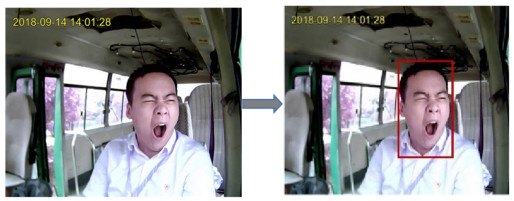
In order to avoid traffic accidents caused by driver fatigue, smoking and talking on the phone, it is necessary to design an effective fatigue detection algorithm. Firstly, this paper studies the detection algorithms of driver fatigue at home and abroad, and analyzes the advantages and disadvantages of the existing algorithms. Secondly, a face recognition module is introduced to crop and align the acquired faces and input them into the Facenet network model for feature extraction, thus completing the identification of drivers. Thirdly, a new driver fatigue detection algorithm based on deep learning is designed based on Single Shot MultiBox Detector (SSD) algorithm, and the additional layer network structure of SSD is redesigned by using the idea of reverse residual. By adding the detection of drivers' smoking and making phone calls, adjusting the size and number of prior boxes of SSD algorithm, improving FPN network and SE network, the identification and verification of drivers can be realized. The experimental results showed that the number of parameters decreased from 96.62 MB to 18.24 MB. The average accuracy rate increased from 89.88% to 95.69%. The projected number of frames per second increased from 51.69 to 71.86. When the confidence threshold was set to 0.5, the recall rate of closed eyes increased from 46.69% to 65.87%, that of yawning increased from 59.72% to 82.72%, and that of smoking increased from 65.87% to 83.09%. These results show that the improved network model has better feature extraction ability for small targets.
Citation: Yuhua Ma, Ye Tao, Yuandan Gong, Wenhua Cui, Bo Wang. Driver identification and fatigue detection algorithm based on deep learning[J]. Mathematical Biosciences and Engineering, 2023, 20(5): 8162-8189. doi: 10.3934/mbe.2023355
[1] | Feng You, Yunbo Gong, Xiaolong Li, Haiwei Wang . R2DS: A novel hierarchical framework for driver fatigue detection in mountain freeway. Mathematical Biosciences and Engineering, 2020, 17(4): 3356-3381. doi: 10.3934/mbe.2020190 |
[2] | Jing Zhang, Ting Fan, Ding Lang, Yuguang Xu, Hong-an Li, Xuewen Li . Intelligent crowd sensing pickpocketing group identification using remote sensing data for secure smart cities. Mathematical Biosciences and Engineering, 2023, 20(8): 13777-13797. doi: 10.3934/mbe.2023613 |
[3] | Qi Cui, Ruohan Meng, Zhili Zhou, Xingming Sun, Kaiwen Zhu . An anti-forensic scheme on computer graphic images and natural images using generative adversarial networks. Mathematical Biosciences and Engineering, 2019, 16(5): 4923-4935. doi: 10.3934/mbe.2019248 |
[4] | Tingxi Wen, Hanxiao Wu, Yu Du, Chuanbo Huang . Faster R-CNN with improved anchor box for cell recognition. Mathematical Biosciences and Engineering, 2020, 17(6): 7772-7786. doi: 10.3934/mbe.2020395 |
[5] | Muhammad Hassan Jamal, Muazzam A Khan, Safi Ullah, Mohammed S. Alshehri, Sultan Almakdi, Umer Rashid, Abdulwahab Alazeb, Jawad Ahmad . Multi-step attack detection in industrial networks using a hybrid deep learning architecture. Mathematical Biosciences and Engineering, 2023, 20(8): 13824-13848. doi: 10.3934/mbe.2023615 |
[6] | Qihan Feng, Xinzheng Xu, Zhixiao Wang . Deep learning-based small object detection: A survey. Mathematical Biosciences and Engineering, 2023, 20(4): 6551-6590. doi: 10.3934/mbe.2023282 |
[7] | Lixiang Zhang, Yian Zhu, Jie Ren, Wei Lu, Ye Yao . A method for detecting abnormal behavior of ships based on multi-dimensional density distance and an abnormal isolation mechanism. Mathematical Biosciences and Engineering, 2023, 20(8): 13921-13946. doi: 10.3934/mbe.2023620 |
[8] | C Willson Joseph, G. Jaspher Willsie Kathrine, Shanmuganathan Vimal, S Sumathi., Danilo Pelusi, Xiomara Patricia Blanco Valencia, Elena Verdú . Improved optimizer with deep learning model for emotion detection and classification. Mathematical Biosciences and Engineering, 2024, 21(7): 6631-6657. doi: 10.3934/mbe.2024290 |
[9] | Jin Zhang, Nan Ma, Zhixuan Wu, Cheng Wang, Yongqiang Yao . Intelligent control of self-driving vehicles based on adaptive sampling supervised actor-critic and human driving experience. Mathematical Biosciences and Engineering, 2024, 21(5): 6077-6096. doi: 10.3934/mbe.2024267 |
[10] | Giuseppe Ciaburro . Machine fault detection methods based on machine learning algorithms: A review. Mathematical Biosciences and Engineering, 2022, 19(11): 11453-11490. doi: 10.3934/mbe.2022534 |
In order to avoid traffic accidents caused by driver fatigue, smoking and talking on the phone, it is necessary to design an effective fatigue detection algorithm. Firstly, this paper studies the detection algorithms of driver fatigue at home and abroad, and analyzes the advantages and disadvantages of the existing algorithms. Secondly, a face recognition module is introduced to crop and align the acquired faces and input them into the Facenet network model for feature extraction, thus completing the identification of drivers. Thirdly, a new driver fatigue detection algorithm based on deep learning is designed based on Single Shot MultiBox Detector (SSD) algorithm, and the additional layer network structure of SSD is redesigned by using the idea of reverse residual. By adding the detection of drivers' smoking and making phone calls, adjusting the size and number of prior boxes of SSD algorithm, improving FPN network and SE network, the identification and verification of drivers can be realized. The experimental results showed that the number of parameters decreased from 96.62 MB to 18.24 MB. The average accuracy rate increased from 89.88% to 95.69%. The projected number of frames per second increased from 51.69 to 71.86. When the confidence threshold was set to 0.5, the recall rate of closed eyes increased from 46.69% to 65.87%, that of yawning increased from 59.72% to 82.72%, and that of smoking increased from 65.87% to 83.09%. These results show that the improved network model has better feature extraction ability for small targets.
With the improvement of people's living standard, there are more and more vehicles in life for the convenience of travel and the demand of work. There are not only personal cars, but also other vehicles used for transportation, such as taxis, buses, school buses, trucks, forklifts and other models. These means of transportation bring great convenience to our life, but they also bring a lot of problems. The most prominent problem is that traffic accidents happen more frequently, resulting in great losses for both the country and the people. According to survey statistics, more than 60,000 people die in traffic accidents every year in China, accounting for more than 2% of the total number of deaths [1]. Meanwhile, the fatality rate in traffic accidents is more than 20% every year. This is a terrible figure compared to other countries, such as the fatality rate of traffic accidents in Japan is only 0.8%, and the fatality rate of traffic accidents in the United States is only 1.5% [2]. According to the Traffic Administration Bureau of the Ministry of Public Security, a total of 248,553 traffic accidents occurred in 2020. Among them, 67,759 people died and 269,127 were injured to varying degrees, resulting in an economic loss of 1.29 billion yuan [3]. After investigating the causes of these traffic accidents, it was found that more than 30 percent of the traffic accidents were caused by incorrect driving conditions. The most incorrect driving conditions are fatigue driving, and a small part of them are caused by drivers smoking and talking on the phone while driving [4]. It can be seen that more than 90% of traffic accidents could have been avoided if the driver had been in the correct driving condition before the accident occurred.
The facial feature analysis and detection algorithm imports the video data obtained by the camera into the target detection algorithm for detection. A video of the driver's driving state is captured by a camera, and then computer vision algorithms are used to extract and analyze each frame of the characteristic video stream. The features analyzed in fatigue driving detection are mainly eyes, mouth, cigarette and mobile phone. Through the identification and statistics of these features, it is possible to judge whether the driver is fatigued. For example, when it is detected that the driver closes his eyes for too long, or blinks too frequently or yawns for a period of time, it can be determined that the current state of the driver is fatigue driving. When smoking is detected, it is judged that the current state of the driver is smoking. When it is detected that there is a mobile phone on the driver's face, it is determined that the current state of the driver is a call. Wang et al. [5] studied the influence of wearing glasses on the driver's operation, and analyzed the relationship between the driver's eye area data and the fatigue after wearing glasses. After locating the eye, the eye region is processed using a Kalman filter. Driver fatigue is then detected based on blink rate over time. Wang et al. [6] studied the relationship between the driver's sight and fatigue, established a three-dimensional model of the driver's head area, and calculated the driver's head rotation angle. Liu and He [7] focused on analyzing the eye area in the face image after face detection, and used the Proportion of Eye Closure Time (PERCLOS) value to detect whether the driver was fatigued. Liu et al. [8] described integration work with RGB-D cameras and deep learning, using generative adversarial networks and multi-channel schemes to improve performance.
The detection cost of driver facial feature analysis is lower, the detection effect is better, and the method of data collection is relatively simple. With the continuous optimization of the computer vision algorithm, the driver's facial feature analysis and detection algorithm will also continue to improve, so this paper uses this type of algorithm to realize the driver's fatigue detection.
The above-mentioned driver fatigue detection algorithm model is too large and requires too much configuration of on-board equipment, so real-time monitoring of the driver's working state has been difficult to be popularized. In this paper, the traditional SSD algorithm is improved and a fatigue driving detection algorithm is designed to monitor the driver's face in real time. This algorithm reduces the model size of the original algorithm and is convenient for the algorithm to be embedded in some on-board equipment. The detection speed and accuracy of the algorithm are improved. Added the detection of two dangerous behaviors: smoking and talking on the phone.
The construction of the face identification network is mainly divided into three parts. The first part is face detection, and the detected face image is cut out; the second part is face feature extraction, which converts the intercepted face into a face feature vector. The third part is face identification, which compares the face feature vector with the face database to identify the face.
The Retinaface algorithm is a one-stage face detection algorithm proposed in 2019. It is an optimized version of the Retinanet algorithm [9]. Compared with the Retinanet algorithm, the Retinaface algorithm adds feature fusion (FPN) and enhanced feature extraction (SSH). Thereby, the detection effect of the algorithm is improved. The detection effect of the Retinaface algorithm is shown in Figure 1.
The entire Retinaface algorithm is mainly composed of three parts, as shown in Figure 2. The first part is the backbone feature extraction network, which performs preliminary feature extraction on the features input to the network. The second part is to strengthen the feature extraction network. The obtained features are fused, and an enhanced feature extraction is performed at the same time. The third part is the prediction layer of the network, and the features obtained in the second part are used to obtain the final prediction result.
Through the network in the Retinaface algorithm, it can be judged whether these a priori boxes contain faces, so that the positions of these a priori boxes can be determined. And adjust the position of these a priori boxes to obtain the final prediction box.
In the Retinaface face detection algorithm, the network not only obtains the position of the face frame, but also obtains the positions of five key points of the face, namely the left eye, the right eye, the nose, the left side of the mouth, and the right side of the mouth. The horizontal and vertical coordinates of these key points are obtained by offsetting the center of the box prior.
1) Face detection dataset
The face detection dataset uses the public Wider-Face dataset [10]. There are a total of 32,203 images in the dataset, and each image is marked with faces, and a total of 393,703 faces are marked. This dataset is then divided into three parts, the training set (40%, 12881), the validation set (10%, 3221) and the test set (50%, 16101).
2) Image enhancement processing
•Image light compensation
Taking the driver's driving as an example for comparative analysis, the light on the driver's face during driving will be affected by the external environment, which will affect the accuracy of the detection algorithm. Some original information is missing to varying degrees, resulting in low accuracy of feature extraction. Therefore, in order to minimize the interference and save the valuable data information in the original image, it is necessary to use image light compensation [11]. The light compensation method selected in this paper is the reference white algorithm [12] and the histogram equalization algorithm.
• Image noise reduction
Performing light compensation and histogram equalization processing on the image is just a simple preprocessing, and the good effect of these processing is based on the condition that the image is not polluted. However, in fact, the image is affected by light and monitoring equipment during the acquisition process, and will also be disturbed during the transmission process, which will inevitably be disturbed by noise. The existence of noise will interfere with or even drown out the useful feature information, so there will be problems such as false detection and missed detection during detection [13], so it is necessary to preprocess the image before image detection to reduce noise. Commonly used image noise reduction methods are: mean filter, Gaussian filter, median filter, bilateral filter. The preprocessing module in this paper adopts the bilateral filtering method, and the bilateral filtering operation is realized by calling the bilateralFilter interface in the OpenCV library.
3) Selection and comparative analysis of backbone network
There are many choices for the backbone network of the Retinaface algorithm. Due to the recent release of Mobilenet V3, two relatively stable and fast models, mobilenet V2 backbone network model and ResNet backbone network model, are selected respectively in this paper. A network model with Mobilenet V2 as the backbone and a network model with ResNet as the backbone are respectively built. The Retinaface face detection algorithm is used in two backbone networks. The following results are compared as shown in Table 1.
backbone network | model size | Enter the image size | Easy | Medium | Hard |
Mobilenet V2 | 2 M | 1280 × 1280 | 88.94% | 86.76% | 73.83% |
ResNet | 105 M | 1280 × 1280 | 94.69% | 93.08% | 84.31% |
Figure 3 shows the feature extraction network with Mobilenet V2 as the backbone. Figure (a) shows the structure of the conv_bn module, (b) shows the structure of the conv_dw module, and (c) shows the conv_bn module. The structure diagram of the backbone neural network composed of the conv_dw module and the conv_dw module can be seen from (c), after the backbone network, three effective feature layers of C1, C2 and C3 will be obtained as the input of the lower network.
4) Construction of FPN feature pyramid and enhanced feature extraction layer SSH
After obtaining the three effective feature layers C1, C2 and C3, the three effective feature layers are fused by constructing the FPN feature pyramid [14]. The construction of the FPN feature pyramid is shown in Figure 4. Perform 1 × 1 convolution for each effective feature layer, adjust the number of channels, and then perform upsampling operation on the obtained feature layer with the smallest length and width. After upsampling, the effective feature layer becomes larger. After it becomes larger, adjust the number of channels of the second feature layer and add it to it. This addition operation is the operation of feature fusion. After the feature fusion, a 64-channel 1 × 1 convolution will be performed to adjust the number of channels. After the convolution, upsampling is performed, and then the first feature layer is adjusted for the number of channels and added to it. After the addition, a 1 × 1 convolution of 64 channels is performed to adjust the number of channels.
After the operation of the FPN network, three preliminary prediction feature layers of P1, P2 and P3 are obtained, and then the SSH module is used to perform feature extraction on these three preliminary prediction feature layers again. The advantage of the SSH module is that it can enhance the receptive field of the feature layer [15]. The structure diagram of the SSH module is shown in Figure 5. SSH adopts three parallel structures, which are one 3 × 3 ordinary convolution, two 3 × 3 ordinary convolutions (Its role is to simulate the effect of 5 × 5 convolution), three 3 × 3 ordinary convolutions (Its role is to simulate the effect of 7 × 7 convolution).
5) Analysis of the prediction results of Retinaface
After passing the SSH module, the system will obtain three final effective feature layers, namely SSH1, SSH2, and SSH3, and the system will predict the results through these three effective feature layers. The prediction results of Retinaface are divided into three parts, which are the face classification prediction results, the regression prediction results of the face frame and the regression prediction results of the key points of the face.
• Prediction results of face classification
The interior of the a priori box on each grid point is judged whether it contains a face. By using 1 × 1 convolution to adjust the number of input feature layer channels to num_anchors × 2, the meaning of num_anchors is the number of prior boxes in each grid point. In the Retinaface face detection algorithm in this paper, the value of num_anchors is 2, and num_anchors is multiplied by 2 to determine whether the prior frame contains a face. If the value of the serial number 1 here is relatively large, it means that the a priori frame contains a human face. If the value with the serial number 0 here is relatively large, it means that the a priori frame does not contain a face.
• The regression prediction result of the face frame
Adjust the width and height of the prior frame and the coordinates of the center point to obtain the prediction frame. Because the coordinates of the width and height and the center point require a total of four parameters to determine, the system adjusts the number of input feature layer channels to num_anchors × 4 by using a 1 × 1 convolution.
• Regression prediction results of face key points
Predict the position coordinates of five key points on the face. The system adjusts the number of input feature layer channels to num_anchors × 10 through a 1 × 1 convolution, 10 can be divided into 5 × 2, 5 represents the number of key points on the face, and 2 is the adjustment parameter of five face key points.
1) Improved FPN Feature Pyramid
The FPN feature pyramid mainly solves the multi-scale problem in object detection. Through simple network connections, the performance of small object detection is greatly improved without increasing the amount of calculation of the original model. However, through the analysis of the original FPN feature pyramid, it is found that the Retinaface algorithm only uses the last three feature layers at the FPN, and does not fully utilize the previous feature layers. Therefore, there are three design flaws. First, the semantic difference between features at different levels before feature summation. Second, the information of the features at the highest pyramid level is lost. Third, the heuristic Rol assignment is unreasonable.
Therefore, this paper conducts experimental research on the above three problems, and improves the network structure on the basis of the original. The improved backbone network structure is shown in Figure 6 and the improved FPN structure is shown in Figure 7. The feature layer in the red box is used as the new effective feature layer C0, and then the newly added effective feature layer C0 is also involved in the FPN feature pyramid, so that the improvement will make more full use of the feature layer in the network. Moreover, this improvement is more helpful for the detection of small objects.
2) Experimental results and analysis
When training the Retinaface network model, this article is divided into two steps of training. The first step is to load the pre-training weights of the backbone network, and freeze the weight training of the backbone network during training. At this time, the learning rate is set to 1e-3, Batch_size It is set to 8, and the number of training is set to 50; the second step is to unfreeze the weight training of the backbone network part during training. At this time, the learning rate is set to 1e-4, the Batch_size is set to 4, and the number of training is set to 50. Then this paper uses the trained weights to make predictions, and uses MAP, FPS, and Estimated Total Size to evaluate the network model.
The experimental results are shown in Table 2. It can be found that the MAP value of the improved Retinaface network model on the Easy sample is 3.82% higher than the original model, and the value on the Medium sample is 4.32% higher than the original model., the value on the Hard sample is increased by 5.48% compared with the original model, and the size of the network model after improvement is only 0.05 MB compared with the original model, and the FPS value is only reduced by 5.42% compared with the original model, The FPS of the improved network model is still as high as 72.32%, and the detection speed is still very fast, which shows that the improved Retinaface network model in this paper is better than the original Retinaface network model in most application scenarios.
Network | Model size Enter image size | Model size Enter image size | Easy | Medium | Hard | FPS |
Original network | 2 M | 1280 × 1280 | 88.94% | 86.76% | 73.83% | 77.74 |
Improved network | 2.05 M | 1280 × 1280 | 92.76% | 91.08% | 79.31% | 72.32 |
Figure 8 shows the detection results of the face detection algorithm based on Retinaface before and after the improvement. The comparison of the detection results shows that there is a false detection of the face before the improvement, and the steering wheel is also judged as a face in the figure, and the improved network can recognize faces more correctly and reduce the occurrence of false detections.
After the face detection is performed by the Retinaface algorithm, the Facenet network can be used to extract the features of the face detected by the system.
1) Face recognition dataset
The face recognition data set is the public CASIA-WebFace data set [16], in which this paper selects the available faces and aligns them. Many face pictures belonging to the same person are stored in a subdirectory.
2) Face correction processing
In the process of face detection and recognition, most of the collected faces are offset. This phenomenon will affect the detection effect of the system's face recognition. In order to solve this problem, this paper uses a related algorithm to rotate the face to adjust to the facial features are in the horizontal state, which is more convenient for the system to recognize the face [17].
The first step is to obtain the relative coordinates of the face key points in the face frame. The second step is to use the coordinates of the eyes in the face key points to obtain the inclination angle of the face. The third step is to obtain the center value of the corrected image, this The value is the center of the image after the face frame is intercepted. The fourth step is to use the center of the corrected image and the correction angle to calculate the rotation matrix. The fifth step is to use the rotation matrix and the warpAffine function in the OpenCV library to correct the face. The sixth step is to calculate the coordinates of the key points of the face after alignment. The result is shown in Figure 9.
3) Workflow of Facenet
As shown in Figure 10, the Facenet algorithm can be divided into three parts according to the workflow. The first part is the backbone feature extraction network, which performs preliminary feature extraction on the image, and the second part, a global average pooling operation is performed on the obtained preliminary feature layer [18]. In the third part, L2 normalization is performed on the obtained feature vector to increase the stability of the network and facilitate the face comparison operation of the network.
As shown in formula (1), L2 normalization is to find each element in the eigenvector divided by the L2 norm. The L2 norm is the Euclidean norm. L2 is computed by taking the absolute value of each vector element in the eigenvector, taking the sum of squares, and taking the square root.
(1) |
When the Facenet algorithm is used for face recognition, when the face feature strip with a length of 128 that has been standardized by L2 is obtained, then the face feature vector needs to be compared with the face feature vector already in the face database. If there is a face feature vector in the database that is very close to the Euclidean distance of the currently obtained face feature vector. In other words, the distance between the two faces is very close, which indicates that the two It is very likely that the face images belong to the same person.
4) Facenet prediction process
The input of the Facenet network is two pictures. Before inputting the two pictures into the Facenet network model, the pictures are preprocessed. The specific operation is to first perform an undistorted resize operation on the two pictures. Adjust the image size to the value required by the Facenet network model. In this paper, the input image size of the Facenet network model is required to be 160 × 160. Then the image is normalized. The normalization process includes the adjustment of the input image data dimension and the dimension adjustment. After the preprocessing of the image is completed, the image is input into the Facenet network model, and then the feature vectors output by the two images are obtained, and the Euclidean distance of the two feature vectors is calculated and compared. When the Euclidean distance of the two pictures is less than the set threshold, it is determined to be the same face; when the Euclidean distance of the two pictures is greater than the set threshold, it is determined not to be the same face [19].
The entire face recognition process can be divided into three parts. The first part is to use the Retinaface algorithm to perform face detection on the picture input to the network. After the face detection is completed, the system will obtain the coordinates of the face frame in the picture, and then Use the obtained face frame coordinates to intercept and align the face in the picture; the second part is to use the Facenet network to encode the intercepted face to obtain a feature vector with a length of 128. This feature vector is the concentration of the face data in the input image; the third part is the face comparison, the process of face comparison is to subtract the obtained feature vector from the face feature vector that already exists in the database, and then calculate the European The value of the distance, after the cyclic comparison, find the face with the shortest Euclidean distance between the database and the detected face. If the Euclidean distance between the face and the detected face meets the threshold, it indicates that the person who entered the network The face and the face of the database are the face of the same person, thereby identifying the identity of the face.
1) Establish database and data initialization
Database initialization refers to the initialization of the face database. To achieve face recognition, you must first know which faces you need to recognize. In this step, the faces that need to be recognized are encoded and put into the database. The specific execution process of database initialization is as follows.
• Traverse all the pictures in the database.
• Use Retinaface to detect the face position in each image.
• Cut out the face.
• Align the acquired faces.
• Use Facenet to encode the face.
• Put all face encoding results in a list.
The list obtained in step 6 is the list of known features of all faces. The faces in the real-time pictures obtained later need to be compared with the known faces. This is how you know everyone's identity.
2) Compare the face characteristics in real-time pictures with the database
Figure 11 shows the comparison process of face and database, this alignment process requires a loop implementation, which is specifically looped for each face in real-time pictures.
• Get every face feature in real-time pictures.
• Compare each face feature and all faces in the database, calculate the distance. If the distance is less than the threshold, it is considered to have a certain similarity.
• Get the serial number of each face in the database in the database.
• Determine whether the face distance of this serial number is less than the threshold. If it is less than the threshold, it is considered that the face recognition is successful, and he is this person.
3) Display of prediction results
The prediction results are shown in Figure 12. (a) contains a picture of Qian Er, and (b) contains a picture of Zhang San. Because the faces of Qian Er and Zhang San are collected in the database, after passing through the network, not only will the The faces of Qian Er and Zhang San are framed, and the name of the corresponding face will be marked on the lower left of the face frame. However, the faces in the dataset are not included in (c) and (d), so through the network After that, only the face in the picture can be framed, and the identity information of the face in the picture cannot be recognized.
The SSD algorithm is a target detection algorithm. After comparing the SSD algorithm with other mainstream target detection algorithms, it is concluded that the detection speed and detection accuracy have quite good results [20]. The running speed of SSD algorithm is similar to YOLO, and the detection accuracy is similar to Faster RCNN. So this paper uses the SSD algorithm to achieve Monitoring of the driver's working status. In practical applications, the devices that detect the driver's working state are all in-vehicle devices or portable devices. Although the traditional SSD algorithm model is relatively small, it cannot be embedded for some devices with low memory. Therefore, the main purpose of improving SSD in this paper is It is to reduce the model algorithm, and the second is to improve the detection accuracy and detection speed.
1) Network structure of SSD
The network structure of SSD is shown in Figure 13. SSD uses VGG16 as the basic model, and adds a convolutional layer on the basis of VGG16 to obtain more feature maps for detection [21]. After the extra layers of the backbone feature extraction network VGG and SSD, 6 effective feature layers will be obtained. The sizes of these 6 effective feature layers are 1 × 1. Among them, the two feature layers of 38 × 38 and 19 × 19 are used to detect small objects, the two feature layers of 10 × 10 and 5 × 5 are used to detect medium objects, and the two feature layers of 3 × 3 and 1 × 1 used to detect large objects.
In the middle of the VGG and SSD extra layers, the SSD algorithm uses the expansion convolution (Expansion convolution) [22]. The expansion convolution can exponentially increase the receptive field of the convolution without increasing the parameters and model complexity, so that each convolution output contains a large range of information, which uses the dilation rate parameter to indicate the size of the dilation. As shown in Figure 14, (a) is an ordinary 3 × 3 convolution, and its field of view is 3 × 3. Figure (b) is a dilated convolution with a dilation rate of 2, and the field of view becomes 7 × 7.
2) Network structure of SSD
In the traditional SSD algorithm, the setting of the prior frame size is divided from small to large, and the correspondence between the prior frame and the feature map is that the smaller the feature map scale, the larger the corresponding prior frame size [23].
The calculation method of the prior frame scale is shown in Eq (2), where is the number of feature graphs, is the serial number of feature graphs. In the traditional SSD algorithm, the first feature map is defined separately, represents the ratio of the prior frame size to the original image size, and and represent the minimum and maximum ratios, respectively. The values set in traditional SSD algorithms are 0.2 and 0.9 [24]. For the first feature map, the ratio of the prior box size to the original image is set as . Calculated according to Eq (2), the final values are 0.1, 0.2, 0.37, 0.54, 0.71 and 0.88. In the traditional SSD algorithm, the size of the original image is 300 × 300, and these ratios are corresponding to the original image size, and the scale values of each feature map are finally obtained as shown in Table 3.
(2) |
feature map | min_size | max_size |
conv4_3 | 30 | 60 |
fc7 | 60 | 111 |
conv6_2 | 111 | 162 |
conv7_2 | 162 | 213 |
conv8_2 | 213 | 264 |
conv9_2 | 264 | 315 |
The size calculation of the default box is shown in Figure 15. In the traditional SSD algorithm, each grid center of each feature map will generate 2 square default boxes of different sizes [25]. In addition, each time aspect_ratio is set, two rectangular default boxes are added to the center of each grid of each feature map. In the traditional SSD algorithm, the value of aspect_ratio is set to [[2,2,3,2,3,2,3,2,2]], and the corresponding number is 1, 2, 2, 2, 1, 1, so in the traditional SSD algorithm, 4, 6, 6, 6, 4, 4 default boxes are generated at each grid center of each feature map [26].
This paper studies the detection of the driver's working state, and the device used is a vehicle-mounted device or a portable device. The configuration of these devices is not too high, so the first improvement of the algorithm in this paper is to reduce the size of the detection system network model. The object detected by the SSD algorithm in this paper is the driver's working state feature. Specific features include the opening and closing of the eyes, the opening and closing of the mouth, a burning cigarette, and a mobile phone on the side of the face. Among them, the detection of eyes and mouths belong to the detection of small objects, so the second improvement of the algorithm in this paper is to optimize the detection effect of the network model on small objects.
For the above two improvement directions, this paper has four improvements to the SSD algorithm. First, to modify the backbone network from VGG to MobileNet V2, and rebuild a new extra layer feature extraction network. Second, adjust the size and number of prior boxes in the network model. Third, a feature pyramid structure is added to the SSD to perform feature fusion on the obtained effective feature layers. Fourth, an attention mechanism is added before the head layer of the network.
1) Adjust the backbone network
The original backbone feature extraction network of the SSD algorithm is VGG16. VGG16 is composed of five groups of convolutional layers and three fully connected layers. In each group, the maximum pooling method is used to reduce the size of the feature layer to half of the original. VGG has a good detection effect in model size, detection accuracy, and detection speed. At present, the SSD backbone feature extraction network is improved towards more complex models. For example, replace the SSD backbone network with resnet50 [27]. These methods improve the detection accuracy of the network model, but will increase the size of the network model and reduce the detection speed. At present, the problem encountered in the practical application of driver working state detection is that the detection network model is too large, which leads to the high configuration and high price of detection equipment [28]. Therefore, increasing the size of the network model is not suitable for the research topic of this paper. To sum up, this paper adjusts the backbone network of SSD to MobileNet V2, and the changed network structure is shown in Figure 16. When MobileNet V2 is selected as the backbone network of SSD, part of the network structure of MobileNet V2 needs to be modified. Remove the last fully connected layer of MobileNet V2. In addition, this paper imitates the extra layer feature extraction network of the original SSD and reconstructs a new extra layer feature extraction network. The new extra feature layer construction uses four InvertedResidual structures with stride of 2, and the values of expand_ratio are 0.2, 0.25, 0.25 and 0.25, respectively.
2) Adjustment of a priori box size and number
In the SSD network model, network predictions are made by setting multiple scales of prior boxes on the grid of each feature layer. According to this idea, if the a priori frame size of the network is set enough, in principle, all detection targets can be covered. But this will lead to a large increase in the number of a priori boxes in the network, and there will be a particularly large number of invalid a priori boxes overlaid on the feature layer. As a result, the detection speed of the algorithm will also drop significantly [29]. Therefore, in the experiment, the number of a priori boxes was adjusted many times, and the optimal value was finally selected.
In the original SSD network, the scale of the feature layer responsible for detecting small objects is 38 × 38. Its corresponding prior box scale is 0.1 to 0.2. This means that the minimum detection scale of the SSD network model is 0.1. When the detection target is much smaller than 0.1 scale, the SSD network model will not be able to detect this object. In the SSD network model in this paper, the input size of the model is set to 300 × 300. The value corresponding to the 0.1 scale is 30, and the detection scale of 30 is still very large for small features such as eyes and mouths in the working state. Therefore, this paper decides to moderately adjust the scale of the prior frame to a small direction.
The scales of the original SSD prior boxes are (0.1, 0.2, 0.37, 0.54, 0.71, 0.88). This article has tried a variety of adjustment methods, here are two adjustments.
• The scale of the prior box is (0.05, 0.12, 0.29, 0.46, 0.62, 0.80), the minimum size is 0.05 and 0.12, and the maximum scale is 0.80. So it can be calculated that the interval is still 0.17, and the number of a priori boxes is (6, 6, 6, 6, 6, 6).
• (0.07, 0.15, 0.32, 0.49, 0.66, 0.83), the minimum size is 0.07 and 0.15, and the maximum size is 0.83. Therefore, the interval after calculation is still 0.17, and the number of a priori boxes is (6, 6, 6, 6, 6, 6).
When adjusting the prior frame, this paper also tried the K-nearest neighbor clustering algorithm to classify and arrange the real frames of the dataset. The obtained data is then used as the prior frame of the network, but the experimental detection effect is not good.
3) Construction of feature pyramid structure
The traditional SSD algorithm detection adopts multi-scale prediction, and uses effective feature layers of different sizes to predict objects of different sizes on the picture. The feature layer with large size detects small objects, and the feature layer with small size detects large objects. However, there is a problem with such prediction, that is, the feature layer with large size is too shallow because of the shallow number of layers in the network, resulting in too little feature semantic information extracted. Although the feature layer with small size extracts sufficient semantic features, the location information is lost too much due to multiple downsampling. Therefore, in the original multi-scale prediction of SSD, the FPN module was constructed for the four feature layers with sizes of 38 × 38, 19 × 19, 10 × 10, and 5 × 5 to enhance the detection effect of the network on small objects.
The module construction of FPN is shown in Figure 17. The number of layers and the size of layers is the usual number. The method used in the feature fusion part is add. A 1 × 1 convolution operation is performed before and after the add operation. The 1 × 1 convolution before adding is to adjust the number of channels and reduce the computational load of the network. The 1 × 1 convolution after adding is for better feature fusion, thereby improving the detection effect of the algorithm in this paper.
After the FPN module is performed, the obtained shallow feature layer will contain more semantic information, which will improve the small target detection effect.
4) Add attention mechanism
The main role of the attention mechanism is to optimize the extraction ability of useful information in the feature layer [30]. The main structure is shown in Figure 18. The importance of each feature channel is used to enhance the ability of the network to extract useful information, while suppressing the ability of the network to extract useless information.
In Figure 18, first, an h × w × c feature layer is input. Second, perform a global average pooling on it, resulting in a 1 × 1 × c feature layer. Thirdly, two fully connected layers are used to increase and reduce the dimension of the obtained feature layer. In this way, the relationship between the feature layer channels can be better obtained. Fourth, a sigmod layer is processed, and a 1 × 1 × c feature vector is obtained at this time. Finally, this feature vector is fully multiplied with the original feature layer, which improves the ability of the feature layer to obtain useful information.
In the experiments in this section, there are two choices for where to add the attention mechanism module. The first option is to place the attention mechanism module before the FPN module. The second option is to place the attention mechanism module after the FPN module. In this section, experiments are carried out on both of the above options. The experiments show that placing the attention mechanism module after the FPN module improves the detection of MAP by 5% compared to placing it before the FPN module. Therefore, this paper chooses to place the attention mechanism module (AMM) after the FPN module, and the network model is shown in Figure 19.
1) Driver fatigue detection dataset
At present, there is no open-source large-scale dataset for the algorithm research of driver fatigue detection. Therefore, in order to support the application research of driver fatigue detection algorithm, this paper downloads 4932 pictures about driver fatigue driving from the Internet. Since this paper not only detects the fatigue state of the driver, but also detects the state of smoking and calling, so 4829 pictures of smoking and calling are downloaded from the Internet. Then, these 9761 pictures were marked with working status features, including open and closed eyes, yawning and normal mouths, burning cigarettes, mobile phones attached to the side of the face, etc.
The pictures in the dataset are shown in Figure 20. The production process of data set includes obtaining images, labeling images with labelimg, obtaining xml and txt files, saving data and outputting. After labeling the dataset, make sure that each feature of the data is labeled and that the labels are correct. Then put the dataset in the correct directory. The effect of Labellmg labeling the dataset is shown in Figure 21.
After all the data sets are labeled, the data of this data set is enhanced. The method chosen in this paper is the spatial transformation of pictures. Firstly, the images are rotated clockwise by 30, and then the annotation of the data set is changed by the rotation algorithm, and a data set of 19,522 images is obtained. Finally, the training set, verification set and test set are divided according to the ratio of 5:2:3, and 9761 training sets, 3905 verification sets and 5856 test sets are obtained. And the data set contains faces of all skin colors.
2) Experimental design and result analysis
In this paper, many attempts have been made in the process of improving SSD algorithm, and finally good detection results have been obtained. The training of models is based on self-made data sets, and the models will be loaded with corresponding pre-training weights to improve the training speed and training effect of algorithm models. The training methods of the model are freezing backbone network weight training and thawing backbone network weight training, and the number of epoch trained is 120. The 1st-50th epoch is the weight that the network will freeze the backbone network. In this stage, the learning rate is set to 5e-4, the Batch size is set to 4, and the optimizer is Adam optimizer. In the 50th-120th epoch, unfreeze the backbone network weight training. In this stage, set the learning rate to 1e-4 and the Batch size to 4. In the training process of the model, generally around the 100th epoch, the training loss and verification loss of the model tend to be stable and converge.
As shown in Table 4, six experiments were conducted in the process of improving SSD. Experiment 1 is a fatigue detection system built with traditional SSD network. Experiment 2 to Experiment 6 all improved the traditional SSD network. Experiment 6 is the final designed algorithm.
Serial number | model | Eye closure recall rate | Yawning recall rate |
Parameter quantity | MAP | FPS |
1 | Vgg16 backbone | 46.69% | 59.72% | 92.62 MB | 86.00% | 51.69 |
2 | MobileNetV2 Backbone | 58.43% | 74.51% | 16.05 MB | 85.94% | 85.86 |
3 | MobileNetV2 Backbone + Adjustment 1 of prior box |
53.86% | 69.89% | 16.05 MB | 91.60% | 85.47 |
4 | MobileNetV2 Backbone + Adjustment 2 of prior box |
59.71% | 75.74% | 16.05 MB | 93.68% | 86.94 |
5 | MobileNetV2 Backbone + Adjustment of prior box + FPN module |
62.94% | 78.97% | 17.62 MB | 94.55% | 76.35 |
6 | MobileNetV2 Backbone + Adjustment of prior box + FPN module + SE module |
65.87% | 82.72% | 18.24 MB | 95.69% | 71.86 |
After the training of the network model, several weights with small training loss and verification loss are selected and loaded into the network for measurement and evaluation in turn. This paper mainly evaluates the model from Precision, Recall, average accuracy (MAP), number of predicted video frames per second (FPS) and the size of parameters, and determines whether the improved network model in this paper is superior or not.
The comparison of ablation experiments and data shows that the improved driver fatigue detection algorithm based on SSD algorithm has two improvements compared with the traditional SSD algorithm.
•The network size is greatly reduced, and the detection speed is increased.
This paper studies the detection of driver's fatigue state, and the purpose of the designed system is to embed in the vehicle-mounted equipment to realize the real-time monitoring of driver's fatigue state. The network model size of the improved algorithm is 92.62 MB and the FPS is 51.69. The network model size of the improved algorithm is 18.24 MB, which is reduced by 74.38 MB. FPS is 71.86, an increase of 20.17. It can be seen that compared with the traditional SSD algorithm, the network model size of the improved driver fatigue detection algorithm is greatly reduced, and the detection speed is also improved.
•The extraction ability and detection effect of small targets are improved.
As shown in Figure 22, (a) and (c) are the recall rates of eye-closing feature and yawning feature of traditional SSD network model respectively, and (b) and (d) are the recall rates of eye-closing features and yawning features of the improved network model based on SSD algorithm, respectively. According to the data in the figure, the recall rate of eyes closed and yawning in the network model before improvement is 46.69% and 59.72%, respectively. In the improved network model, the recall rate of eye-closing is 65.87%, up by 19.18%, and that of yawning is 82.72%, up by 23%. Therefore, compared with the traditional SSD algorithm, the improved driver fatigue detection algorithm improves the ability to extract small targets.
As shown in Figure 23, (a) is the MAP of the traditional SSD network model, and (b) is the MAP of the improved network model based on SSD algorithm. According to the data in the figure, the MAP of the network model before improvement is 86.00%. Among them, AP with eyes closed is 76%, and AP with yawning is 86%. The MAP of the improved network model is 95.69%, which is increased by 9.69%. Among them, AP with eyes closed is 93%, and AP with yawning is 7%. Therefore, compared with the traditional SSD algorithm, the improved driver fatigue detection algorithm improves the detection effect of small targets.
Compared with the above ablation experiments and data analysis, MobileNetV2 Backbone + Adjustment of prior box + FPN module + SE module algorithm has the best detection effect. In addition, this paper also compares the detection effects of the improved SSD algorithm, YOLOv4 algorithm and Faster RCNN algorithm in the same configuration environment, and the results are shown in Table 5. Because YOLOv5, v6 and v7 occupy a large amount of storage space, YOLO v4, which occupies a small amount of space, is used here for comparison.
Model | Eye closure recall rate | Yawning recall rate | Parameter quantity | MAP | FPS |
Improved SSD | 65.87% | 82.72% | 18.24 MB | 95.69% | 71.86 |
YOLOv4 | 60.14% | 77.35% | 244.42 MB | 91.56% | 55 |
Faster RCNN | 55.58% | 72.89% | 530.37 MB | 87.53% | 31 |
According to the comparison of the detection effects of different algorithms above, the improved SSD algorithm is better than YOLOv4 algorithm and Faster RCNN algorithm in the same configuration environment.
In this paper, the characteristics of the driver's working state include open and closed eyes, open and shut mouth, a lit cigarette, and a mobile phone held to the side of the face. After the SSD algorithm is used to detect the above features, the system compares the number of features in each frame and the next frame, and makes a cyclic judgment to get the working state of the driver. When the algorithm in this paper uses the computer with the CPU Core i5-1135G7 and the memory of 16 GB for video detection, the FPS value is about 40, that is, the detection time of a frame picture is 0.025 seconds.
1) Eye feature determination
According to research statistics, when people are awake, the time to close their eyes during a single blink is between 0.2 seconds and 0.4 seconds. When people are fatigued, their eyes will be closed for 1 to 2 seconds. Through these data, eye features can be determined according to the number of frames of the video [31]. In this paper, a single eye closure of 2 seconds is selected as the threshold for judging eye fatigue. When the eye-closing time exceeds 2 seconds and the number of video frames converted into 80 frames, the eye fatigue is judged. When the working state is detected, it is detected every 4 frames for 0.1 second. When the video stream is input into the network, SSD algorithm will first detect whether the driver has eyes closed or mouth opened. If eyes closed or mouths opened are not detected, the number of eyes closed or mouths opened will be reset, and then the next video stream will be read in to continue detection. If eyes closed or mouth opened is detected, the number of eyes closed or mouth opened is increased by 1, and then it is determined whether the number of eyes closed or mouth opened is greater than 20. If it is greater than 20, it means that the driver is in a state of fatigue at this time. If it is not greater than 20, it is read into the next video stream to continue detection.
2) Mouth feature judgment
When people are tired, they will yawn, and the time to open their mouths when yawning is usually more than two seconds [32]. Therefore, in actual detection, the mouth feature judgment process is the same as the eye feature judgment process. When the result of 20 consecutive frames is mouth opening, it is judged as yawning state, that is, fatigue state.
3) Smoking judgment and calling judgment
Smoking and calling are relatively simple. When the video stream is transmitted to the network, it is only necessary to detect whether the current frame contains smoking features or calling features. If yes, it is determined that its status is smoking or calling.
The actual detection effect is shown in Figure 24. In (a), the driver's eyes are open, his mouth is closed, and there is no smoking or phone call. Therefore, the detection result is that the driving state and driving behavior are normal; In (b), the driver's eyes are closed, his mouth is shut, and he doesn't smoke or make phone calls. Therefore, the test results are fatigue in driving state and normal driving behavior; In (c), the driver's eyes are open, his mouth is open, and he has no smoking or phone calls. Therefore, the test results are fatigue in driving state and normal driving behavior; In (d), the driver's eyes are open, his mouth is closed, he smokes, and he doesn't call. Therefore, the test result is that the driving state is normal and the driving behavior is smoking; In (e), the driver's eyes are all open, his mouth is closed, he has no smoking characteristics, and he has the characteristics of making phone calls. Therefore, the test result is that the driving state is normal and the driving behavior is calling.
Driver fatigue driving is the most common cause of traffic accidents. Because the traditional model of driver fatigue detection algorithm is too large, and the configuration requirements of vehicle-mounted equipment are too high, it is difficult to popularize the real-time monitoring of driver's working state. Aiming at the above problems, this paper designs a fatigue driving detection algorithm based on SSD algorithm to monitor the driver's face in real time. The traditional SSD algorithm is improved, and the model size of the original algorithm is reduced, so that the algorithm can be embedded in some vehicle-mounted devices. The detection speed and accuracy of the algorithm are improved. At the same time, two dangerous behaviors of drivers, smoking and making phone calls, are added to the fatigue detection. In addition, in order to prevent non-designated drivers from driving vehicles and realize effective monitoring of vehicle operation, an improved face recognition module is added to realize the identification and verification of drivers.
Firstly, this paper designs a network model for face identification. This paper introduces the process of Retinface algorithm to realize face detection. The advantages and disadvantages of the network model with MobileNet V2 backbone network and the network model with ResNet backbone network are compared. The FPN module in Retinface algorithm is optimized. After training the network model, it is known that the MAP of the improved Retinaface model is 3.82% higher than that of the original model. Therefore, the improved face detection algorithm based on Retinaface is more superior.
Secondly, the process of Facenet algorithm to extract face features is introduced. The Facenet network model with MobileNet as the backbone and the network model with Inception-ResNetV1 as the backbone are constructed respectively. Through model training, it is found that the detection accuracy difference between the two network models is only 0.55%. The parameters of Facenet network model with MobileNet as the backbone only account for 14% of the parameters of the network model with Inception-ResNetV1 as the backbone. Therefore, the Facenet network model with MobileNet as the backbone is more advantageous. This paper introduces the construction and initialization of face recognition database, and shows the recognition effect of face recognition network.
Thirdly, the improved SSD algorithm is used to realize the real-time monitoring of driver fatigue. According to the working principle of SSD algorithm, the traditional SSD algorithm is improved by adjusting the backbone network, adjusting the size and number of prior frames, constructing the characteristic pyramid structure and adding attention mechanism. Combined with the comparative analysis of ablation experiments in each improvement process, the optimal improvement algorithm is selected. The improved SSD algorithm surpasses the traditional SSD algorithm in detection accuracy and detection speed, and also reduces the size of the algorithm model. This is more conducive to the embedding and implementation of vehicle-mounted equipment. The judgment basis of fatigue detection is designed, and the detection effect of this fatigue detection algorithm is demonstrated.
In future studies, the face data set should be rebuilt according to the driver's driving situation, and the algorithm model of face detection should be further optimized.
This work was supported by Joint Fund Project of the National Natural Science Foundation of China (U1908218), the Natural Science Foundation project of Liaoning Province (2021-KF-12-06), the Department of Education of Liaoning Province (LJKFZ20220197), and the National College Students Innovation and Entrepreneurship Training Program of Liaoning University of Science and Technology (202110146004).
[1] |
D. Shi, C. Sun, X. Sheng, X. Bi, Design of monitoring system for driving safety based on convolutional neural network, J. Hebei North Univ., 36 (2020), 57–61. https://doi.org/10.3969/j.issn.1673-1492.2020.09.011 doi: 10.3969/j.issn.1673-1492.2020.09.011
![]() |
[2] |
X. Meng, Driving fatigue caused by tramc accident characteristics and effective prevention analysis, Logist. Eng. Manage., 8 (2014), 187–188. https://doi.org/10.3969/j.issn.1674-4993.2014.08.073 doi: 10.3969/j.issn.1674-4993.2014.08.073
![]() |
[3] |
X. Gong, J. Fang, X. Tan, A. Liao, C. Xiao, Analysis of the current situation of road traffic accidents in the 31 provinces/municipalities of China and the projection for achieving the SDGs target of halving the numbers of death and injury, Chin. J. Dis. Control Prev., 24 (2020), 4–8. http://doi.org/10.16462/j.cnki.zhjbkz.2020.01.002 doi: 10.16462/j.cnki.zhjbkz.2020.01.002
![]() |
[4] |
S. Chen, J. Hu, Causative analysis of road traffic accidents and research on safety prevention measures, Leg. Syst. Soc., 27 (2020), 143–144. https://doi.org/10.19387/j.cnki.1009-0592.2020.09.247 doi: 10.19387/j.cnki.1009-0592.2020.09.247
![]() |
[5] |
J. Wang, X. Yu, Q. Liu, Y. Zhou, Research on key technologies of intelligent transportation based on image recognition and anti-fatigue driving, EURASIP J. Image Video Process., 1 (2019), 33–45. https://doi.org/10.1186/s13640-018-0403-6 doi: 10.1186/s13640-018-0403-6
![]() |
[6] |
X. Wang, R. Chen, B. Huang, Implementation of driver driving safety monitoring system based on android system, Electron. Meas. Technol., 42 (2019), 56–60. https://doi.org/10.19651/j.cnki.emt.1802406 doi: 10.19651/j.cnki.emt.1802406
![]() |
[7] |
S. Liu, L. He, Fatigue driving detection system based on image processing, J. Yuncheng Univ., 39 (2021), 51–54. https://doi.org/10.15967/j.cnki.cn14-1316/g4.2021.06.013 doi: 10.15967/j.cnki.cn14-1316/g4.2021.06.013
![]() |
[8] |
F. Liu, D. Chen, J. Zhou, F. Xu, A review of driver fatigue detection and its advances on the use of RGB-D camera and deep learning, Eng. Appl. Artif. Intell., 116 (2022), 105399. https://doi.org/10.1016/j.engappai.2022.105399 doi: 10.1016/j.engappai.2022.105399
![]() |
[9] |
Y. Sui, Z. Yan, L. Dai. H. Jing, Face multi-attribute detection algorithm based on RetinaFace, Railway Comput. Appl., 30 (2021), 1–4. https://doi.org/10.3969/j.issn.1005-8451.2021.03.001 doi: 10.3969/j.issn.1005-8451.2021.03.001
![]() |
[10] | S. Yang, P. Luo, C. C. Loy, X. Tang, Wider face: a face detection benchmark, in 2016 IEEE Conference on Computer Vision and Pattern Recognition (CVPR), (2016), 5525–5533. https://doi.org/10.1109/CVPR.2016.596 |
[11] |
G. M. Clayton, S. Devasia, Image-based compensation of dynamic effects in scanning tunnelling microscopes, Nanotechnology, 16 (2005), 809–818. https://doi.org/10.1088/0957-4484/16/6/032 doi: 10.1088/0957-4484/16/6/032
![]() |
[12] |
L. Huang, H. Yang, B. Wang, Research and improvement of multi-method combined face image illumination compensation algorithm, J. Chongqing Univ. Technol., 31 (2017), 6–12. https://doi.org/10.3969/j.issn.1674-8425(z).2017.11.027 doi: 10.3969/j.issn.1674-8425(z).2017.11.027
![]() |
[13] |
L. Shao, R. Yan, X. Li, Y. Liu, From heuristic optimization to dictionary learning: A review and comprehensive comparison of Image denoising algorithms, IEEE Trans. Cybern., 44 (2017), 1001–1013. https://doi.org/10.1109/TCYB.2013.2278548 doi: 10.1109/TCYB.2013.2278548
![]() |
[14] |
C. Shi, C. Zhang, Q. He, H. Wang, Target detection based on improved feature pyramid, Electron. Meas. Technol., 44 (2021), 150–156. https://doi.org/10.19651/j.cnki.emt.2107598 doi: 10.19651/j.cnki.emt.2107598
![]() |
[15] | X. Guo, Research on Multi-Scale Face Detection Based on Convolution Neural Networks, M.S thesis, North China Electric Power University in Hebei, 2020. |
[16] | F. Chen, Research on Cosine Loss Algorithm for Face Verification, M.S thesis, Xiangtan University in Hunan, 2020. https://doi.org/10.27426/d.cnki.gxtdu.2020.001269 |
[17] |
Z. Yang, L. Hou, D. Yang, lmproved face recognition algorithm of attitude correction, Cyber Secur. Data Governance, 35 (2016), 56–60. https://doi.org/10.19358/j.issn.1674-7720.2016.03.019 doi: 10.19358/j.issn.1674-7720.2016.03.019
![]() |
[18] | S. Preetha, S. V. Sheela, Security monitoring system using facenet for wireless sensor network, preprint, arXiv: 2112.01305. |
[19] | X. Li, R. Huang, Z. Chen, Y. Long, L. Xu, An improved face detection and recognition algorithm based on FaceNet and MTCNN, J. Guangdong Univ. of Petrochem. Technol., 31 (2021), 45–47. |
[20] |
J. Wang, J. Li, X. Zhou, X. Zhang, Improved SSD algorithm and its performance analysis of small target detection in remote sensing images, Acta Opt. Sin. 39 (2019), 10. https://doi.org/10.3788/AOS201939.0628005 doi: 10.3788/AOS201939.0628005
![]() |
[21] | S. Mao, H. Li, Research on improved SSD algorithm for detection in traffic, Microprocessors, 43 (2022), 26–29. |
[22] | B. Wang, Y. Lv, X. Hei, H. Jin, Lightweight deep convolutional neural network model based on dilated convolution, 2020. Available from: https://kns.cnki.net/kcms2/article/abstract?v = kxaUMs6x7-4I2jr5WTdXti3zQ9F92xu0dKxhnJcY9pxwfrkG2rAGFOJWdZMiOIJjZJ9FLVWmYcCCgfpgeyHSjqedCLDh_ut5 & uniplatform = NZKPT |
[23] |
L. Jiang, J. Li, B. Huang, Research on face feature detection algorithm based on improved SSD, Mach. Des. Manuf. Eng., 50 (2021), 82–86. https://doi.org/10.3969/j.issn.2095-509X.2021.07.017 doi: 10.3969/j.issn.2095-509X.2021.07.017
![]() |
[24] |
X. Zhang, A. Jiang, SSD Small Target detection algorithm combining feature enhancement and self-attention, Comput. Eng. Appl., 58 (2022), 247–255. https://doi.org/10.3778/j.issn.1002-8331.2109-0356 doi: 10.3778/j.issn.1002-8331.2109-0356
![]() |
[25] | J. Guo, T. Yu, Y. Cui, X. Zhou, Research on vehicle small target detection algorithm based on improved SSD, Comput. Technol. Dev., 32 (2022), 1–7. |
[26] | Q. Zheng, L. Wang, F. Wang, Candidate box generation method based on improved ssd network, 2020. Available from: https://kns.cnki.net/kcms2/article/abstract?v = kxaUMs6x7-4I2jr5WTdXti3zQ9F92xu0ManZHCyoNk-lwS3y-OLIR4fcD18PUKrUkLhyHScAkvpkTgimuL-OfVjGi7Jisy2h & uniplatform = NZKPT |
[27] |
Q. Song, X. Wang, C. Zhang, Y. Chen, H. Song, A residual SSD model based on window size clustering for traffic sign detection, J. Hunan Univ., 46 (2019), 133–140. https://doi.org/10.16339/j.cnki.hdxbzkb.2019.10.016 doi: 10.16339/j.cnki.hdxbzkb.2019.10.016
![]() |
[28] |
W. Chen, Lightweight convolutional neural network remote sensing image target detection, Beijing Surv. Mapp., 36 (2018), 178–183. https://doi.org/10.19580/j.cnki.1007-3000.2022.02.014 doi: 10.19580/j.cnki.1007-3000.2022.02.014
![]() |
[29] | K. Chen, Research on SSD-based Multi-scale Detection Algorithm, M.S thesis, Beijing Jiaotong University in Beijing, 2020. https://doi.org/10.26944/d.cnki.gbfju.2020.002225 |
[30] |
H. Zhang, M. Zhang, SSD Target Detection Algorithm with Channel Attention Mechanism, Comput. Eng., 46 (2020), 264–270. https://doi.org/10.19678/j.issn.1000-3428.0054946 doi: 10.19678/j.issn.1000-3428.0054946
![]() |
[31] | Z. A. Haq, Z. Hasan, Eye-blink rate detection for fatigue determination, in 2016 1st India International Conference on Information Processing (IICIP), (2016), 1–5. https://doi.org/10.1109/IICIP.2016.7975348 |
[32] |
X. Zhou, S. Wang, W. Zhao, X. Zhao, T. Li, Fatigue Driving Detection Based on State Recognition of Eyes and Mouth, J. Jilin Univ., 35 (2017), 204–211. https://doi.org/10.19292/j.cnki.jdxxp.2017.02.015 doi: 10.19292/j.cnki.jdxxp.2017.02.015
![]() |
1. | Jiayi Yu, Ye Tao, Huan Zhang, Zhibiao Wang, Wenhua Cui, Tianwei Shi, Age estimation algorithm based on deep learning and its application in fall detection, 2023, 31, 2688-1594, 4907, 10.3934/era.2023251 | |
2. | Ayan Bin Rafaih, Kaso Ari, Artificial Intelligence-Driven Approaches to Managing Surgeon Fatigue and Improving Performance, 2024, 2168-8184, 10.7759/cureus.75717 | |
3. | Yanfan Duan, Zheng Li, Yanchen Gao, Diancai Yang, 2024, Lightweight YOLOv8n with Cross-Scale Feature Fusion for Driver Fatigue Detection, 979-8-3503-6828-4, 191, 10.1109/ICHCI63580.2024.10808105 | |
4. | Shanmeng Zhao, Yaxue Peng, Yaqing Wang, Gang Li, Mohammed Al-Mahbashi, Lightweight YOLOM-Net for Automatic Identification and Real-Time Detection of Fatigue Driving, 2025, 82, 1546-2226, 4995, 10.32604/cmc.2025.059972 |
backbone network | model size | Enter the image size | Easy | Medium | Hard |
Mobilenet V2 | 2 M | 1280 × 1280 | 88.94% | 86.76% | 73.83% |
ResNet | 105 M | 1280 × 1280 | 94.69% | 93.08% | 84.31% |
Network | Model size Enter image size | Model size Enter image size | Easy | Medium | Hard | FPS |
Original network | 2 M | 1280 × 1280 | 88.94% | 86.76% | 73.83% | 77.74 |
Improved network | 2.05 M | 1280 × 1280 | 92.76% | 91.08% | 79.31% | 72.32 |
feature map | min_size | max_size |
conv4_3 | 30 | 60 |
fc7 | 60 | 111 |
conv6_2 | 111 | 162 |
conv7_2 | 162 | 213 |
conv8_2 | 213 | 264 |
conv9_2 | 264 | 315 |
Serial number | model | Eye closure recall rate | Yawning recall rate |
Parameter quantity | MAP | FPS |
1 | Vgg16 backbone | 46.69% | 59.72% | 92.62 MB | 86.00% | 51.69 |
2 | MobileNetV2 Backbone | 58.43% | 74.51% | 16.05 MB | 85.94% | 85.86 |
3 | MobileNetV2 Backbone + Adjustment 1 of prior box |
53.86% | 69.89% | 16.05 MB | 91.60% | 85.47 |
4 | MobileNetV2 Backbone + Adjustment 2 of prior box |
59.71% | 75.74% | 16.05 MB | 93.68% | 86.94 |
5 | MobileNetV2 Backbone + Adjustment of prior box + FPN module |
62.94% | 78.97% | 17.62 MB | 94.55% | 76.35 |
6 | MobileNetV2 Backbone + Adjustment of prior box + FPN module + SE module |
65.87% | 82.72% | 18.24 MB | 95.69% | 71.86 |
Model | Eye closure recall rate | Yawning recall rate | Parameter quantity | MAP | FPS |
Improved SSD | 65.87% | 82.72% | 18.24 MB | 95.69% | 71.86 |
YOLOv4 | 60.14% | 77.35% | 244.42 MB | 91.56% | 55 |
Faster RCNN | 55.58% | 72.89% | 530.37 MB | 87.53% | 31 |
backbone network | model size | Enter the image size | Easy | Medium | Hard |
Mobilenet V2 | 2 M | 1280 × 1280 | 88.94% | 86.76% | 73.83% |
ResNet | 105 M | 1280 × 1280 | 94.69% | 93.08% | 84.31% |
Network | Model size Enter image size | Model size Enter image size | Easy | Medium | Hard | FPS |
Original network | 2 M | 1280 × 1280 | 88.94% | 86.76% | 73.83% | 77.74 |
Improved network | 2.05 M | 1280 × 1280 | 92.76% | 91.08% | 79.31% | 72.32 |
feature map | min_size | max_size |
conv4_3 | 30 | 60 |
fc7 | 60 | 111 |
conv6_2 | 111 | 162 |
conv7_2 | 162 | 213 |
conv8_2 | 213 | 264 |
conv9_2 | 264 | 315 |
Serial number | model | Eye closure recall rate | Yawning recall rate |
Parameter quantity | MAP | FPS |
1 | Vgg16 backbone | 46.69% | 59.72% | 92.62 MB | 86.00% | 51.69 |
2 | MobileNetV2 Backbone | 58.43% | 74.51% | 16.05 MB | 85.94% | 85.86 |
3 | MobileNetV2 Backbone + Adjustment 1 of prior box |
53.86% | 69.89% | 16.05 MB | 91.60% | 85.47 |
4 | MobileNetV2 Backbone + Adjustment 2 of prior box |
59.71% | 75.74% | 16.05 MB | 93.68% | 86.94 |
5 | MobileNetV2 Backbone + Adjustment of prior box + FPN module |
62.94% | 78.97% | 17.62 MB | 94.55% | 76.35 |
6 | MobileNetV2 Backbone + Adjustment of prior box + FPN module + SE module |
65.87% | 82.72% | 18.24 MB | 95.69% | 71.86 |
Model | Eye closure recall rate | Yawning recall rate | Parameter quantity | MAP | FPS |
Improved SSD | 65.87% | 82.72% | 18.24 MB | 95.69% | 71.86 |
YOLOv4 | 60.14% | 77.35% | 244.42 MB | 91.56% | 55 |
Faster RCNN | 55.58% | 72.89% | 530.37 MB | 87.53% | 31 |