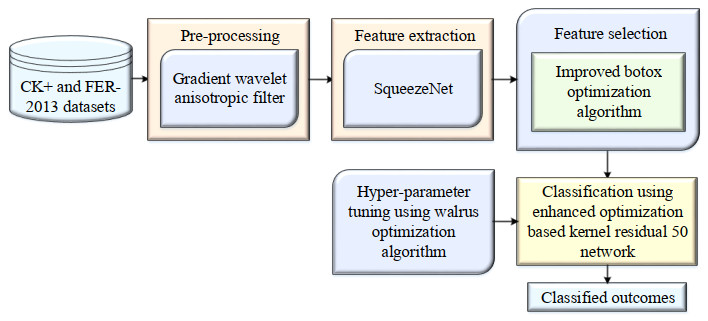
Facial emotion recognition (FER) is largely utilized to analyze human emotion in order to address the needs of many real-time applications such as computer-human interfaces, emotion detection, forensics, biometrics, and human-robot collaboration. Nonetheless, existing methods are mostly unable to offer correct predictions with a minimum error rate. In this paper, an innovative facial emotion recognition framework, termed extended walrus-based deep learning with Botox feature selection network (EWDL-BFSN), was designed to accurately detect facial emotions. The main goals of the EWDL-BFSN are to identify facial emotions automatically and effectively by choosing the optimal features and adjusting the hyperparameters of the classifier. The gradient wavelet anisotropic filter (GWAF) can be used for image pre-processing in the EWDL-BFSN model. Additionally, SqueezeNet is used to extract significant features. The improved Botox optimization algorithm (IBoA) is then used to choose the best features. Lastly, FER and classification are accomplished through the use of an enhanced optimization-based kernel residual 50 (EK-ResNet50) network. Meanwhile, a nature-inspired metaheuristic, walrus optimization algorithm (WOA) is utilized to pick the hyperparameters of EK-ResNet50 network model. The EWDL-BFSN model was trained and tested with publicly available CK+ and FER-2013 datasets. The Python platform was applied for implementation, and various performance metrics such as accuracy, sensitivity, specificity, and F1-score were analyzed with state-of-the-art methods. The proposed EWDL-BFSN model acquired an overall accuracy of 99.37 and 99.25% for both CK+ and FER-2013 datasets and proved its superiority in predicting facial emotions over state-of-the-art methods.
Citation: C Willson Joseph, G. Jaspher Willsie Kathrine, Shanmuganathan Vimal, S Sumathi., Danilo Pelusi, Xiomara Patricia Blanco Valencia, Elena Verdú. Improved optimizer with deep learning model for emotion detection and classification[J]. Mathematical Biosciences and Engineering, 2024, 21(7): 6631-6657. doi: 10.3934/mbe.2024290
[1] | Xiaodan Zhang, Shuyi Wang, Kemeng Xu, Rui Zhao, Yichong She . Cross-subject EEG-based emotion recognition through dynamic optimization of random forest with sparrow search algorithm. Mathematical Biosciences and Engineering, 2024, 21(3): 4779-4800. doi: 10.3934/mbe.2024210 |
[2] | Binju Saju, Neethu Tressa, Rajesh Kumar Dhanaraj, Sumegh Tharewal, Jincy Chundamannil Mathew, Danilo Pelusi . Effective multi-class lungdisease classification using the hybridfeature engineering mechanism. Mathematical Biosciences and Engineering, 2023, 20(11): 20245-20273. doi: 10.3934/mbe.2023896 |
[3] | Basem Assiri, Mohammad Alamgir Hossain . Face emotion recognition based on infrared thermal imagery by applying machine learning and parallelism. Mathematical Biosciences and Engineering, 2023, 20(1): 913-929. doi: 10.3934/mbe.2023042 |
[4] | Yufeng Qian . Exploration of machine algorithms based on deep learning model and feature extraction. Mathematical Biosciences and Engineering, 2021, 18(6): 7602-7618. doi: 10.3934/mbe.2021376 |
[5] | Xu Yin, Ming Meng, Qingshan She, Yunyuan Gao, Zhizeng Luo . Optimal channel-based sparse time-frequency blocks common spatial pattern feature extraction method for motor imagery classification. Mathematical Biosciences and Engineering, 2021, 18(4): 4247-4263. doi: 10.3934/mbe.2021213 |
[6] | Kunpeng Li, Zepeng Wang, Yu Zhou, Sihai Li . Lung adenocarcinoma identification based on hybrid feature selections and attentional convolutional neural networks. Mathematical Biosciences and Engineering, 2024, 21(2): 2991-3015. doi: 10.3934/mbe.2024133 |
[7] | Yan Yan, Yong Qian, Hongzhong Ma, Changwu Hu . Research on imbalanced data fault diagnosis of on-load tap changers based on IGWO-WELM. Mathematical Biosciences and Engineering, 2023, 20(3): 4877-4895. doi: 10.3934/mbe.2023226 |
[8] | Dingxin Xu, Xiwen Qin, Xiaogang Dong, Xueteng Cui . Emotion recognition of EEG signals based on variational mode decomposition and weighted cascade forest. Mathematical Biosciences and Engineering, 2023, 20(2): 2566-2587. doi: 10.3934/mbe.2023120 |
[9] | Jie Bai, Heru Xue, Xinhua Jiang, Yanqing Zhou . Classification and recognition of milk somatic cell images based on PolyLoss and PCAM-Reset50. Mathematical Biosciences and Engineering, 2023, 20(5): 9423-9442. doi: 10.3934/mbe.2023414 |
[10] | Yuzhuo Shi, Huijie Zhang, Zhisheng Li, Kun Hao, Yonglei Liu, Lu Zhao . Path planning for mobile robots in complex environments based on improved ant colony algorithm. Mathematical Biosciences and Engineering, 2023, 20(9): 15568-15602. doi: 10.3934/mbe.2023695 |
Facial emotion recognition (FER) is largely utilized to analyze human emotion in order to address the needs of many real-time applications such as computer-human interfaces, emotion detection, forensics, biometrics, and human-robot collaboration. Nonetheless, existing methods are mostly unable to offer correct predictions with a minimum error rate. In this paper, an innovative facial emotion recognition framework, termed extended walrus-based deep learning with Botox feature selection network (EWDL-BFSN), was designed to accurately detect facial emotions. The main goals of the EWDL-BFSN are to identify facial emotions automatically and effectively by choosing the optimal features and adjusting the hyperparameters of the classifier. The gradient wavelet anisotropic filter (GWAF) can be used for image pre-processing in the EWDL-BFSN model. Additionally, SqueezeNet is used to extract significant features. The improved Botox optimization algorithm (IBoA) is then used to choose the best features. Lastly, FER and classification are accomplished through the use of an enhanced optimization-based kernel residual 50 (EK-ResNet50) network. Meanwhile, a nature-inspired metaheuristic, walrus optimization algorithm (WOA) is utilized to pick the hyperparameters of EK-ResNet50 network model. The EWDL-BFSN model was trained and tested with publicly available CK+ and FER-2013 datasets. The Python platform was applied for implementation, and various performance metrics such as accuracy, sensitivity, specificity, and F1-score were analyzed with state-of-the-art methods. The proposed EWDL-BFSN model acquired an overall accuracy of 99.37 and 99.25% for both CK+ and FER-2013 datasets and proved its superiority in predicting facial emotions over state-of-the-art methods.
An emotion is a powerful and intuitive sensation that arises in humans as a result of their conditions, circumstances, moods, and relationships with other people. Therefore, emotional recognition is a crucial topic to discuss when talking about human-machine connection as well as when it comes to analyzing and exercising control over feelings. There are a variety of methods, including psychological signals, bodily gestures, speech, and facial expressions, that may be used to decipher an individual's emotional state. Alterations in the human body's physiological states, such as variations in heart rate (measurable by an electrocardiogram (ECG)), temperature, skin conductance, muscular tension, and brain waves are examples of these alterations [1,2,3,4,5].
Emotion detection, a fundamental aspect of affective computing, involves using advanced technologies, specifically deep learning, to recognize and interpret human emotions from various inputs such as text, speech, facial expressions, and other multimodal sources. The ability to understand human emotions automatically has gained increasing significance due to its wide range of applications, including sentiment analysis, mental health assessment, human-computer interaction, and personalized services [6,7,8]. Deep learning, with its capability to automatically learn intricate patterns and features from large and complex datasets, has emerged as a powerful tool for enhancing the accuracy and robustness of emotion detection systems [9,10,11,12,13].
Deep learning is a very important component of the process of identifying a variety of feelings based on acoustic speech information. The detection and classification of emotions represent a vital aspect of human-computer interaction, sentiment analysis, and affective computing. Existing frameworks and techniques have made substantial progress in this domain, yet they are not without their challenges. One of the primary challenges in emotion classification is the availability of high-quality and diverse datasets [14,15,16]. Many existing deep learning models for emotion detection are regarded as "black boxes", making it difficult to understand how and why they make certain predictions. Emotion classification often requires substantial computational resources, especially when dealing with large-scale datasets or complex deep-learning models [17,18,19,20]. These resource requirements can limit the widespread adoption of emotion recognition systems, particularly in resource-constrained environments or on edge devices. Optimizing existing frameworks for efficiency while maintaining accuracy is an ongoing challenge. Addressing these challenges is crucial for creating more accurate and adaptable emotion recognition systems that can be applied across various domains and cultures. In this paper, a novel optimization-based extended deep learning strategy with optimal feature selection is performed to accurately identify facial emotions by addressing the existing problems. The major contributions of the proposed method are as follows.
● To design an innovative FER model, extended walrus-based deep learning with Botox feature selection network (EWDL-BFSN) for effectually predicting facial emotions.
● To apply a new meta-heuristic optimization algorithm, the improved Botox optimization algorithm (IBoA), to select optimal features for minimizing computational complexity.
● To design an effective enhanced optimization-based kernel residual 50 (EK-ResNet50) network for predicting facial emotions with maximum accuracy rate by minimizing the errors.
● To offer a nature-inspired metaheuristic walrus optimization algorithm (WOA) for tuning the network parameters of the EK-ResNet50 model and lessen the false positive (FP) rates.
● To estimate the performance of the EWDL-BFSN model by relating it with state-of-the-art methods using two different publicly available datasets.
The remaining paper structure is as follows: the relevant studies addressing the prediction of facial emotions are identified in Section 2. In Section 3, the EWDL-BFSN model is discussed. The outcome and discussion are presented in Section 4. Section 5 brings an illustration of the conclusion and future directions.
Alzahrani [21] introduced a bioinspired image processing-enabled facial emotion recognition (BIPFER-EOHDL) model that incorporates an equilibrium optimizer (EO) and hybrid deep learning. The median filtering (MF) was used to pre-process the input image. Next, the EfficientNetB7 model was used to extract features. Meanwhile, the EO technique was used to select the hyperparameters of the EfficientNetB7 model. Lastly, the categorization of facial emotions was accomplished through a multi-head attention bi-directional long short-term memory (MA-BLSTM) model. Even with improved performances, this model would require minimizing the false rates.
Tao and Duan [22] suggested a hierarchical attention network with progressive feature fusion for the purpose of classifying facial expressions. First, a diverse feature extraction module that depends on multiple feature aggregation blocks was used to exploit both high-level and low-level features, as well as gradient features in order to aggregate diverse complementary features. Second, a hierarchical attention module (HAM) was constructed to gradually boost discriminative characteristics from significant regions of the face images and suppress task-irrelevant features from distracting facial regions to efficiently fuse the dissimilar features. According to extensive trials, it achieved the best performance; however, the accuracy was not satisfying.
Alamgir and Alam [23] offered a hybrid deep belief rain optimization (HDBRO) model for FER. The first step used in the HDBRO model was pre-processing the images obtained from the dataset in order to remove noise. Then, the primary geometric and appearance-based features were retrieved from the pre-processed image. From the retrieved feature set, the most significant features were chosen and classified into seven distinct emotions using DBRO. The HDBRO method's overall performance attained 97% accuracy values, superior to those of the other categorization models. However, a better optimal parameter tuning algorithm was required to improve the classification performance.
Kumar et al. [24] recommended an improved FER method for recognizing facial emotions using neural networks and optimal descriptor selection. At first, the Viola-Jones method was used to extract the face from the input image. Then, the Gabor filtering effectively filtered the noise, and a modified version of the SIFT approach called affine-scale-invariant feature transform (ASIFT) was used to extract the facial components as features. Subsequently, the neural network used the retrieved descriptors for classification. The findings showed that this system was capable of classifying seven different emotions accurately. The drawbacks of this method include less texture and edge improvements, less accuracy, and more complexity.
Kumari and Bhatia [25] introduced an enhanced FER technique based on deep learning. At first, the collected dataset was subjected to a combined trilateral filter in order to eliminate noise. Then, the filtered images underwent contrast-limited adaptive histogram equalization (CLAHE) to improve image visibility. Lastly, training accomplished a deep convolutional neural network (CNN). The cost function of deep CNN was also optimized by means of the Nadam optimizer. This model outperformed the competing models in terms of precision, recall, accuracy, and other metrics, according to comparative analysis. However, it had limitations such as overfitting issues, vanishing gradient problems, less accuracy, and higher false rates.
Although several deep learning-based FER models have made great progress, they still present several problems and restrictions. For training, emotion detection models mainly rely on labeled datasets. It is difficult to find large and diverse labeled datasets for emotion identification. Besides, accurate emotion labeling is difficult to achieve, and different annotators can interpret emotions differently. As a result, the training data can contain discrepancies that could have an impact on the model's performance. Deep learning models, particularly intricate ones like deep neural networks, are frequently referred to as "black boxes" due to their level of intricacy. Building trust and comprehending the model's decision-making process is crucial, especially in sensitive areas like mental health; however, it is difficult to understand the underlying workings of these models and provide reasons for the predictions they make. Deep learning algorithms undergo overfitting problems, especially if the training data is sparse or unbalanced. In emotion detection tasks, striking a balance between accurately fitting the training data and generalizing to new data remains difficult. Thereby, in this paper, a novel optimization-driven enhanced deep learning model focuses on overcoming the limitations of existing methods.
In this section, a novel EWDL-BFSN model is proposed for accurately predicting facial emotions. The EWDL-BFSN model includes different phases such as data collection, pre-processing, feature extraction, feature selection, and facial emotion classification. Initially, the data collection stage gathers the input images from the publically available dataset. The raw input image typically needs to be pre-processed to enhance the visual representation using a gradient wavelet anisotropic filter (GWAF). Next, a feature extraction method based on SqueezeNet is utilized to extract the features representing facial emotions. Then, the feature selection process is performed using IBoA to obtain optimized features with lower sizes. Lastly, the selected features are fed to EK-ResNet50 to achieve enhanced classification. The block diagram of the EWDL-BFSN model is presented in Figure 1.
The pre-processing is the initial stage of the proposed EWDL-BFSN model, which is employed to boost the visual representation of the acquired input image. In the EWDL-BFSN model, a gradient wavelet anisotropic filter (GWAF) is applied to pre-process the input image acquired from the datasets. Anisotropic diffusion is an extensively renowned method for alleviating noise in images when the existence of edges is more significant. However, when the level of noise is high, the anisotropic diffusion approach is ineffective, and it is considered the primary drawback. Preserving information structures in facial images, like object boundaries and edges, is a major challenge in the field of image processing but is essential to reach better results. The wavelet-based transformation has attained greater importance owing to its capability to perform multi-scale analysis and detect both low- and high-frequency components. Therefore, wavelet transformation is employed in dissimilar image processing applications, including speech recognition, computer graphics, and multi-fractal analysis, biology for cell membrane recognition, fingerprint verification, and smoothing and image de-noising, among others. A wavelet transform-based anisotropic diffusion method is proposed for the filtering of facial images to address the drawbacks of anisotropic diffusion and the benefits of wavelet transform. Here, no smoothing method nor even a Gaussian smoothing is applied.
ωn,p2kL(b,c,u+1)=ωn,p2kL(b,c,u)+κ|μ(b,c)|∑(q,r)∈μ(b,c)g(|∇ωn,p2kL(b,c,u)|,ς)|∇ωn,p2kL(b,c,u)|, | (1) |
where ωn,p2kL(b,c,u) represents the wavelet coefficient at iteration u or time constant at position (b,c), n=(1,2) implies the horizontal and vertical direction, g() indicates the gradient in the DWT domain, κ resembles the stability constant < = 0.25, and μ describes the neighborhoods of the central pixel. The threshold employed to tune the gradient magnitude is specified as ς, which is computed as
ς=√5ς, | (2) |
where ςe=1.4285υl(∇l−υ(|∇l|)). In this case, the filtering equation has removed the Gaussian smoothing component. Owing to this removal, this procedure is more ideal and efficient. The gradient is computed ωn,p2kL(b,c,u) in four dissimilar directions as
∇Nωn,p2kL(b,c,u+1)=ωn,p2kL(b,c−1,u)−ωn,p2kL(b,c,u), | (3) |
∇Sωn,p2kL(b,c,u+1)=ωn,p2kL(b,c−1,u)−ωn,p2kL(b,c,u), | (4) |
∇Eωn,p2kL(b,c,u+1)=ωn,p2kL(b,c−1,u)−ωn,p2kL(b,c,u), | (5) |
∇Wωn,p2kL(b,c,u+1)=ωn,p2kL(b,c−1,u)−ωn,p2kL(b,c,u). | (6) |
For noise reduction, the anisotropic diffusion is executed iteratively on each scale of wavelet transform components ω1,p2kL,ω2,p2kL. As wavelets can break down complex information into simpler forms at dissimilar locations and scales, they are used in this situation. After pre-processing, the pre-processed images are fed to SqueezeNet model for feature extraction.
For emotion recognition, feature extraction encompasses a number of crucial steps and methods to precisely recognize and classify human emotions. Feature extraction involves identifying and isolating particular attributes or specific characteristics. To guarantee the consistency and accuracy of the input, the process usually starts with image pre-processing. This is followed by the identification and localization of the face within the image. The detection of significant face landmarks, comprising the nose, mouth, and eyes, is essential to realize the spatial arrangement of facial features. Methods such as geometric-based techniques examine the angles and distances between landmarks, whereas appearance-based methods acquire fine variations and texture of the muscles and skin of the face. However, deep learning models, mainly convolutional neural networks (CNNs), and advanced techniques automatically recognize and extract the features from raw pixel data. To appropriately classify the emotions, these obtained features are subsequently fed into classifiers by minimizing the dimensionality.
The choice of the initial feature extraction layer is significant for achieving both speed and precision in FER, and it normally entails a trade-off between accuracy and speed. SqueezeNet [26] is designed with the objective of decreasing both the model's size and number of parameters [27]. SqueezeNet lessens the model size to about 4.8 MB while preserving recognition accuracy by compressing the parameters to around 1/50 of AlexNet. SqueezeNet uses the convolutional separation method to transform a standard 3 × 3 convolution into a fire unit by exchanging a 1 × 1 convolution kernel for a part of 3 × 3 convolution kernel. Each module has a rectified linear unit (ReLU) activation, and the fire module encompasses two layers, namely a squeeze layer and an expand layer to upsurge the depth of network. There are 1 × 1 convolution kernels in the squeeze layer, as well as 1 × 1 and 3 × 3 convolution kernels in the expand layer. The 3 × 3 convolution kernel can guarantee the precision of network, while the 1 × 1 convolution kernel can minimize the weight parameters. SqueezeNet is selected as a FER feature extraction network to diminish the time of feature extraction and accelerate detection and recognition owing to its excellent precision and compact size. The architecture of SqueezeNet for extracting features is given in Figure 2.
To give the network a specific depth, the first 17 layers of the SqueezeNet FER feature extraction network are used, and the last convolution and average pooling layers are eliminated. First, the pre-processed input image goes through Conv1 and Max1, Fire2−Fire4 and Max4, then Fire5−Fire8 and Max8, and finally through Fire9. A set of convolutions is employed to extract the image's FER feature map.
Every layer in the SqueezeNet feature extraction network is comprised of three max-pooling layers with a stride of 2, eight fire units, and one convolutional layer. Each fire module has a similar structure, which encompasses the squeeze layer and an expand layer. The depth of the network is given as 2. The 1 × 1 and 3 × 3 convolutional output feature maps are split together in the channel of the expand layer to form the fire module's channel. The below expression is fulfilled by the quantity of convolution kernels in the squeeze and expand layer:
Y<Z1+Z2, | (7) |
where Y indicates the number of 1 × 1 convolution kernels in squeeze layer, Z1 specifies the number of 1 × 1 convolution kernels in the expand layer, and Z2 resembles the number of 3 × 3 convolution kernels in the expand layer.
The SqueezeNet's input size for feature extraction is set to 300 × 300 × 3, and the feature maps' size is minimized to half of its original size using 3 × 3 max pooling layer with a stride of 2. Lastly, Fire9 is utilized to obtain the 19 × 19 × 512 feature map. It has been demonstrated in [28] that large feature maps are favorable for detecting smaller objects, but smaller feature maps are convenient for detecting large objects. The proposed method uses 10 × 10 × 1024 feature map as its input to expand the detection of facial emotions. This is accomplished by passing the 19 × 19 × 512 feature map through a 3 × 3 convolutional layer with 1024 channels and a step size of 2. Moreover, there are more 1 × 1 convolution kernels in the fire module than 3 × 3 convolution kernels. Minimum information loss happens if the network dimension is minimized using the 1 × 1 convolution kernel. As a result, while retaining more facial emotion information, the feature extraction speed of SqueezeNet can be increased. The experiment will demonstrate a higher performance of SqueezeNet for FER feature extraction. In addition, the SqueezeNet model works well for tasks requiring higher real-time performance because of its speedy performance. After feature extraction, the extracted features are given as input to the feature selection algorithm.
The process of discovering the optimal subset of features is termed feature selection. It is vital to build high-performance models and minimize computational complexity. In the proposed EWDL-BFSN model, the feature selection is performed using the improved Botox optimization algorithm (IBoA). IBoA combines the Botox optimization algorithm (BoA) [29] and dynamic weight factor to improve feature selection performance. BoA is a general metaheuristic optimization algorithm stimulated through Botox operation mechanism. The tenacity of BoA is to overcome the optimization issues by engaging in a human-based policy. The BoA is considered and mathematically established by taking hints from Botox treatments, in which the defects are targeted and cured to increase beauty. Moreover, it has great capability to accomplish a balance between exploration and exploitation. Each individual demanding Botox treatments is reflected as a BoA member. BoA models the way a doctor would inject Botox into specific facial muscles to diminish wrinkles and increase beauty. Correspondingly, the BoA strategy incorporates picking decision factors and including a specific value, like Botox, to boost the candidate solution.
Similar to many optimization techniques, BoA may find it difficult to escape local optima. In some cases, the BoA fails to find the actual global optimum and converges on a suboptimal solution. For complex problems, strategies to prevent trapping in local optima and improve the exploration can be required. Dynamic weights are incorporated into the BoA to prevent it from becoming stuck in local optima, which are poor solutions that are mistaken for the best. When considering dynamic weights, BOA can then concentrate on areas with the greatest decrease in wrinkles (exploit) based on the outcomes that have been observed. Moreover, BoA is capable of striking a balance between exploring the search space of promising areas that have been identified so far and searching the search space for potentially superior solutions (avoiding local optima) through the inclusion of dynamic weights. This can greatly enhance the algorithm's chance of determining the true global optimum. For feature selection, the population members of BoA are characterized as features. Each member contributes to the decision variable values according to their location in the problem-solving space, statistically characterized as a vector. The below equation delivers the population matrix from this vector, which comprehends the decision variables.
Z=[→Z1⋮→Zj⋮→ZQ]Q×p=[z1,1⋯y1,f⋯y1,p⋮zk,1⋯yk,f⋯yk,p⋮zQ,1⋯yQ,f⋯yQ,p]Q×p. | (8) |
The Eq (9) is engaged to randomly assign the position of each BoA member during initialization:
zk,f=LowBoftk,f.(UppBof−LowBof),k=1,2,...,Q,f=1,2,...,p, | (9) |
where Z designates the population matrix of BoA, Q characterizes the number of population members, →Zj defines the kth member of BoA (candidate solution), p specifies the number of decision variables, tk,f symbolizes the random numbers from the interval [0, 1], and UppBo and LowBo define the upper and lower bounds of eth decision variable, respectively.
For each individual, it is possible to calculate the fitness function of the problem. The fitness function is employed to rate each feature's excellence (potential solution). The objective of IBoA is to recognize the subset of ideal features in a search area that has minimum feature subset size and lower classification error rate. For feature selection, the fitness function used in the IBoA is conveyed as follows:
I(fit)k=(1−δ)×S+δ×(PDime), | (10) |
where S describes the classification accuracy, δexemplifies the weight parameter set to 0.01, P outlines the size of the selected feature subset, and Dime suggests the dimension. The below expression delivers the array of fitness function values to be categorized as a vector:
→H=[H1⋮Hk⋮HQ]Q×1=[H(→Z1)⋮H(→Zk)⋮H(→ZQ)]Q×1, | (11) |
where →H characterizes the vector of the assessed fitness function, and Hk indicates the evaluated fitness function that relies on kth BoA member.
According to BoA design, the number of facial muscles that demand Botox injections is diminished as the algorithm iterates. Consequently, the following equation is exploited to calculate the number of decision variables (i.e., chosen muscles) for Botox injection:
Qd=[1+pv]⩽p, | (12) |
where v characterizes the present value of the iteration counter and Qd defines the number of muscles that necessitate Botox injections.
The doctor determines which muscles to inject Botox depending on the person's face and wrinkles. By this circumstance, the below formulation is engaged to choose the variables to be injected for each population member.
Dbsk={g1,g2,...,gl,...,gQd},gl∈{1,2,3,...,p}and∀j,m∈{1,2,3,..,Qd}:gj≠gm, | (13) |
where Dbsk signifies the set of potential decision variables for the kth population members that are preferred for Botox injection, and gk states the location of the lth decision variable preferred for Botox injection.
The amount of Botox injections for each population member is calculated using the below equation, which is equivalent to the doctor's decision in determining the drug quantity for Botox injection based on patient desires and expertise:
→Dk={→ZMean−→Zk,v<V2→ZBest−→Zk,else, | (14) |
where →Dk=(dk,1,...,dk,l,....,dk,p) characterizes the considered amount for Botox injection to the kth member, V suggests the total number of iterations, →ZBest defines the best member of the population, and →ZMean signifies the mean population position (i.e., →ZMean=1Q∑Qk=1→Zk). In this phase of BoA, a dynamic weight factor Ψ is included to assist the walrus in constantly updating their location. The equation of Ψ is mathematically stated as follows:
Ψ=e2(1−m/M)−e−2(1−m/M)e2(1−m/M)+e−2(1−m/M). | (15) |
If the BoA is able to achieve an enhanced global search, the value of Ψ at the beginning of the iteration is larger, and at the end of the iteration, the value minimizes adaptively. Now, the BoA can maximize convergence speed and perform local searches more effectively.
→Dk={(→ZMean−→Zk)ψ,v<V2(→ZBest−→Zk)ψ,else. | (16) |
The appearance of faces is altered by the wrinkles disappearing after a Botox injection into the facial muscles. Initially, a new location is calculated for each BoA member depending on Botox injection based on the below equation:
→ZNewk:zNewk,gl=zk,g;+tk,gl.dk,gl, | (17) |
where →ZNewk characterizes the new location of kth member after injecting Botox, dk,gl indicates the dimension of Botox injection forkth member (i.e., →Dk), zNewk,gl specifies its gthl dimension, and tk,gl designates a random number with uniform distribution on the interval. If the value of fitness increases, this new position exchanges the resultant member's preceding location in accordance with the below expression:
→Zk={→ZNewk,HNewk<Hk→Zk,else, | (18) |
where HNewk characterizes the fitness function value. The pseudocode of feature selection using IBoA is delivered in Algorithm 1.
Algorithm 1: Feature selection using IBoA |
Start |
Initialize the size of population Q and total number of iteration V |
Set the fitness function, constraints and variables |
Build the initial population matrix in random manner |
Determine the fitness function |
Assess the best candidate solution →ZBest |
For v=1to V |
Update the number of decision variables for injecting Botox using Eq (12) |
For k=1to Q |
Describe the variables that are imitated for Botox injection based on Eq (13) |
Calculate the amount of Botox injection based on Eq (16) |
For k=1to Qd |
Calculate the new location of kthIBoA member based on Eq (17) |
End |
Compute the fitness function depending on →ZNewj |
Update the kth member of IBoA using Eq (18) |
End |
Save the best candidate solution so far obtained |
End |
Output the best solution (features) |
Stop |
The proposed EWDL-BFSN model has utilized an enhanced optimization-based kernel residual 50 (EK-ResNet50) network for the detection and classification of facial emotions. EK-ResNet50 network resembles a sophisticated method to enhance the efficiency and accuracy of emotion detection from facial images. The foundation of this network is the ResNet-50 architecture, a deep convolutional neural network renowned for its capability to handle vanishing gradients and facilitate residual learning training of very deep networks. To enhance feature learning and classification, optimization-based approaches and kernel techniques are incorporated. Kernel methods, which are popular for their capability to map input data into higher-dimensional spaces, are specially utilized to acquire variations and complex patterns in facial expressions. These kernel policies allow the network to acquire more complex and subtle properties, which are essential for differentiating between emotions, by embedding them into the residual blocks of ResNet-50. The optimization-based techniques improve the network's performance even further by optimizing the learning process. Overfitting can be avoided, and generalization can be strengthened with the use of adaptive learning rate modifications and sophisticated regularization techniques. Through these modifications, the network is assured to learn relevant features more efficiently and remain robust when dealing with a variety of datasets.
Furthermore, the residual connections in ResNet-50 aid in reducing the degradation issue and provide stable gradient flow even in very deep networks by enabling the network to learn identity mappings. When it comes to intricate tasks such as FER, where it is critical to capture fine-grained features and variations, this stability is significant to support good performance. The EK-ResNet50 network can perform outstandingly better in real-world scenarios than conventional models, offering faster convergence and greater accuracy. These advantages can greatly benefit real-time emotion detection systems that are utilized in psychological analysis, automated customer service, and human-computer interaction. Thus, the strong framework of ResNet-50 associated with sophisticated optimization strategy and kernel delivers an influential mechanism for furthering the field of FER. In addition, the ResNet50 model has obtained greater results in the ImageNet classification challenge and addressed the gradient explosion and disappearance issues. Nevertheless, certain issues remain, particularly the inability to learn subtle features, a lengthy operation time, and the large number of parameters. The EWDL-BFSN model suggests an EK-ResNet50 network by considering the issues of current ResNet50 [30] as well as the features of facial emotion images. The EK-ResNet50 network takes the optimal features selected through IBoA as input. The structure of the EK-ResNet50 network is provided in Figure 3. The enhancements made with the standard ResNet50 model are also provided.
The features of facial emotions are difficult to learn because the richness of detailed information is fundamentally proportionate to the number of pixels they occupy. A convolution layer with a 7 × 7 convolution kernel, which can learn apparent features, makes up the network input part of ResNet50. Nonetheless, the complex image background makes it challenging to learn the texture and color information of facial features using a 7 × 7 convolution, which influences the network model's ability to identify facial emotions. More effective subtle feature learning is necessary to correctly identify facial emotions. Consequently, to better adapt the network design for FET, the 7 × 7 convolution of the first layer is decomposed. In EK-ResNet50, the 7 × 7 convolutional layer is replaced with three 3 × 3 stacked convolutional layers. Besides, the thorough inspection of the ResNet50 structure reveals a greater number of 3 × 3 convolutions within the residual module. Thereby, the 3 × 3 convolution is decomposed into 3 × 1 and 1 × 3 convolutions in series. One way to improve the network's capacity for nonlinear fitting is by using additional nonlinear activation functions in the tiny convolution layer of the series. However, there are fewer parameters in the calculation.
Identity mapping is incorporated into the residual term by the ResNet50 network, and a part of the 1 × 1 convolution layer in the identity mapping is employed to maximize channel dimensions for ensuring the computation of eigen matrix summation. In stages 2–4, the identity mapping of the first residual module is a 1 × 1 convolution layer with a step size of 2. As the step size exceeds the convolution kernel's size, some image information is not convolutionally processed, building it to learn useful features. The identity mapping tactic is enriched in accordance with the features of the facial emotions. In the EK-ResNet50 network, identity mapping is accomplished by employing the convolution layer and the max-pool layer in series. With a step size of 2, the 3 × 3 max-pool layer computes all feature map information while retaining the important features in the area.
In order to maximize the network's ability for generalization, batch normalization (BN) executes global normalization along the batch dimension of the sample. The BN is sensitive to the value of batch size, resulting in data deviation while determining the BN layer at network training, because the standard deviation and average value of BN are derived from the batch size. Switchable normalization (SN) [31] stabilizes the network under dissimilar batch sizes, resolves the issue of computation deviation occurring by BN, and achieves model optimality. In the EK-ResNet50 network, the BN layer in the four phases of the standard ResNet50 model is exchanged with the SN layer. This significantly enhances the model's stability as well as the convergence speed in the FER task.
The walrus optimization algorithm (WOA) is one of the most recent swarm intelligence algorithms inspired by how walruses breed, migrate, roost, escape, gather, and feed based on critical signals (safety and danger signals) [32]. The danger signal is employed in WOA to decide whether to perform the exploration stage or the exploitation stage. During the algorithm's early exploration, the walrus herd moves to a new domain in the solution space if the danger signal reaches specific requirements. On the other hand, the walrus herd reproduces, which defines the exploitation stage. During the exploitation stage, the safety signal is important in defining whether a walrus selects foraging or roosting behavior. In this roosting behavior, male, female, and juvenile walruses communicate with one another in order to move the population in a path that is favorable to survival. Typical foraging characteristics cover gathering and fleeing away, which are managed by danger signals.
In an environment with ordered policing, the walrus herd can accomplish population expansion (looking for the global optimum) and avoid being killed or captured by predators (searching for the local optimum). The experimentation on different test suits shows that the WOA can handle high-dimensional benchmarks and real-world issues with unique stability properties and highly competitive performance. Moreover, the WOA increases the effectiveness of optimization calculations and encourages the ongoing development and application extension of artificial intelligence. Moreover, it becomes a strong tool for resolving challenging issues in the real world. Thereby, the proposed EWDL-BFSN model has selected WOA to tune the hyperparameters of the classification model. Here, the walrus is considered as the tunable parameter, and the gathering behavior of the exploitation stage is imitated to update the optimal values of hyperparameters as follows:
Yu+1j,k=(Y1+Y2)/(Y1+Y2)22, | (19) |
Y1=Yubest−b1×c1×|Yubest−Yuj,k|, | (20) |
Y2=Yusecond−b2×c2×|Yusecond−Yuj,k|, | (21) |
b=χ×s1−χ, | (22) |
c=tan(φ), | (23) |
where Y1 and Y2 resemble the two weights influencing the gathering behavior of walrus, Yusecond signifies the location of the second walrus in the current iteration, and s1 indicates a random number that falls between 0 and 1. |Yusecond−Yuj,k| indicates the separation between the current walrus and the second walrus, b and c characterize the gathering coefficients, and φ represents the values between 0 and π.
This section offers the results of the EWDL-BFSN model and specifies its advantages over modern architectures. The EWDL-BFSN model is executed using the Python platform on a personal computer (PC) with 16 GB of RAM. A testing process is conveyed using an Intel(R) Core (TM) i5-4770CPU@3.20GHz processor, which functions on a 64-bit operating system. For experimentation, the EWDL-BFSN model used two publicly available datasets, namely the extended Cohn-Kanade (CK+) dataset and the FER-2013 dataset. The dropout, learning rate, and activation function are set to 0.001, 0.3, and ReLU. The optimizer used in the classification model is WOA. Numerous performance indicators such as accuracy, sensitivity, specificity, and F1-score are analyzed, and the efficacy of EWDL-BFSN is established over state-of-the-art methods. The below sub-sections cover detailed descriptions of datasets, performance indicators, performance analysis, and discussion of results.
In the EWDL-BFSN model, two different datasets, namely CK+ dataset and FER-2013 dataset, are used for FER experimentation. Comprehensive data for the face emotion classification model is available in the FER2013 for the Kaggle Competition. The FER2013 database was created as part of the ICML 2013 Kaggle challenges [33]. Since then, scientific research on FER has been assessed using this data collection. The images are registered automatically, ensuring that the face of every image is centered and roughly equal in size. The purpose is to use the emotions revealed in a facial expression to classify all faces into eight groups: happiness, sadness, anger, disgust, surprise, contempt, fear, and neutral. This dataset covers 35,887 grayscale images with 48 × 48 pixel resolution. Among all images, 28,709 are used for training and the remaining 3589 for testing. Each image is associated with one of eight emotional states. The other dataset, called CK+3 [34], is a frequently utilized database for FER investigation. It has eight gestures for 123 different individuals. In addition, based on each participant's appearance, 961 image data are included, with each subject resembling one of the eight basic emotional categories (happiness, sadness, fear, disgust, anger, surprise, neutral, and contempt).
This section explains the descriptions and mathematical formulas for several performance measures, including accuracy, F1-score, sensitivity (also known as recall), and specificity. The ratio of accurate FER to complete data elements is distinguished as classification accuracy. Sensitivity is revealed as the ratio of accurate positive outcomes to the total number of matters in the positive class. Specificity measures how well the model differentiates instances that cannot belong to a specified emotion class. The F1-score is a measure that thoroughly replicates the average of recall and precision. The below expression demonstrates the equations of various performance indicators:
Accuracy=TP+TNTP+TN+FP+FN, | (24) |
ℜecall=TPTP+FN, | (25) |
Specificity=TNTN+FP, | (26) |
F1−score=2∗Precision∗ℜecalPrecision+ℜecall, | (27) |
where TN specifies true negative (TN), FP designates false positive (FP), TP indicates true positive (TP), and FN symbolizes false negative (FN).
This section encompasses a detailed analysis and results comparison for classifying facial emotions as anger, contempt, disgust, fear, happiness, neutral, sadness, and surprise. In the evaluation, numerous state-of-the-art models are used to define the effectiveness of the EWDL-BFSN model. Convolutional neural network (CNN), ResNet-101, AlexNet, CNN-VGG-19, inception v3, MobileNet, support vector machine (SVM), ResNet50, HGSO-DLFER, and BIPFER-EOHDL [21,35] are the techniques being compared for classifying facial emotions.
Table 1 presents an analysis of FER results of the EWDL-BFSN model with different classes under an 80:20 ratio of training and testing stages using the CK+ dataset. The results reveal that the EWDL-BFSN model performs successfully across all emotion classes (anger, contempt, disgust, fear, happiness, neutral, sadness, and surprise). In the same way, Table 2 offers the same analysis using the FER-2013 dataset.
Training stage (80%) | Testing stage (20%) | |||||||
Class | Accuracy | Sensitivity | Specificity | F1-score | Accuracy | Sensitivity | Specificity | F1-score |
Surprise | 99.24 | 88.22 | 99.51 | 90.82 | 99.56 | 94.15 | 98.97 | 87.82 |
Sadness | 98.08 | 99.71 | 99.45 | 99.08 | 98.74 | 95.58 | 98.31 | 91.61 |
Neutral | 99.54 | 99.06 | 99.41 | 96.73 | 99.37 | 97.10 | 98.94 | 95.09 |
Happiness | 99.70 | 96.00 | 99.71 | 97.39 | 98.71 | 94.05 | 98.25 | 87.55 |
Fear | 98.52 | 98.01 | 99.79 | 91.90 | 98.61 | 95.52 | 98.72 | 95.89 |
Disgust | 98.64 | 97.15 | 99.50 | 97.62 | 98.46 | 91.96 | 98.99 | 99.72 |
Contempt | 99.25 | 96.29 | 100.00 | 99.55 | 98.55 | 92.34 | 100.00 | 100.00 |
Anger | 99.24 | 96.35 | 99.62 | 96.16 | 98.86 | 94.39 | 98.88 | 93.95 |
Training stage (70%) | Testing stage (30%) | |||||||
Class | Accuracy | Sensitivity | Specificity | F1-score | Accuracy | Sensitivity | Specificity | F1-score |
Surprise | 99.06 | 88.2 | 99.43 | 90.76 | 99.42 | 94.11 | 98.78 | 87.67 |
Sadness | 98.03 | 99.67 | 99.41 | 99.05 | 98.46 | 95.52 | 98.24 | 91.46 |
Neutral | 99.80 | 99.02 | 99.36 | 96.27 | 99.17 | 96.89 | 98.26 | 95.01 |
Happiness | 99.61 | 95.89 | 99.65 | 97.28 | 98.56 | 94.01 | 98.51 | 87.23 |
Fear | 98.25 | 98 | 99.71 | 91.82 | 98.47 | 95.05 | 98.27 | 95.35 |
Disgust | 98.39 | 97.05 | 99.43 | 97.32 | 98.4 | 91.35 | 98.82 | 99.47 |
Contempt | 99.18 | 96.21 | 99.32 | 99.25 | 98.46 | 92.21 | 99.76 | 100.00 |
Anger | 99.21 | 96.3 | 99.38 | 96.07 | 98.57 | 94.27 | 98.57 | 93.53 |
The comparison of the accuracy of the proposed EWDL-BFSN and other state-of-the-art methods regarding FER is shown in Figure 4. The comparison demonstrates how accurate the EWDL-BFSN model is compared to CNN, ResNet-101, AlexNet, CNN-VGG-19, inception v3, MobileNet, SVM, ResNet50, HGSO-DLFER, and BIPFER-EOHDL [21,35]. By using the CK+ and FER-2013 datasets, the overall accuracy accomplished by the EWDL-BFSN for FER is 99.37 and 99.25%, respectively, which is clearly shown in the graphical representation. The reason for these high values is the usage of EK-ResNet50 with effective IBoA for feature selection. The state-of-the-art methods show certain insufficiencies in FER compared with the EWDL-BFSN model. From the graphical demonstration, it is shown that the ResNet and Naïve Bayes models reach the lowest accuracy scores among all state-of-the-art methods, while the BIPFER-EOHDL model has a higher accuracy but still lower than that of the EWDL-BFSN model.
The sensitivity for effectively predicting facial emotions using the CK+ and FER-2013 datasets of the EWDL-BFSN model compared with the state-of-the-art methods is shown in Figure 5. This comparison demonstrates how well the EWDL-BFSN can predict facial emotions (anger, contempt, disgust, fear, happiness, neutral, sadness, and surprise). From the graphical demonstration, it is shown that the EWDL-BFSN model accomplishes a better recall value than the other state-of-the-art methods. As the complex feature vectors are nominated through IBoA, the EWDL-BFSN model can classify facial emotion classes with greater exactness. The overall sensitivity value accomplished by the EWDL-BFSN model is 99.2 and 98.21% using the CK+ and FER-2013 datasets, respectively.
Figure 6 compares the specificity of the EWDL-BFSN model and other state-of-the-art methods in effectively predicting facial emotions using the CK+ and FER-2013 datasets. From the graphical demonstration, it is demonstrated that the EWDL-BFSN model has a higher specificity value than the other state-of-the-art methods. The primary cause for this enhancement is the selection of the EK-ResNet50 network, which uses the WOA for classifying facial emotions. Among the state-of-the-art methods, the BIPFER-EOHDL has better specificity, closer to the EWDL-BFSN model, while ResNet101 and Naïve Bayes have relatively little specificity. Subsequently, it is recognized that the EWDL-BFSN model effectively identifies the facial emotion classes and measures the rate of incorrect cases.
Figure 7 displays the F1-score of the EWDL-BFSN model and the other state-of-the-art methods for effectively predicting facial emotions using the CK+ and FER-2013 datasets. The EWDL-BFSN model can properly recognize the class labels for a given input data. The graphical depiction illustrates that the EWDL-BFSN model outperforms the state-of-the-art methods by around 98.21 and 98.15% for the CK+ and FER-2013 datasets, respectively. Furthermore, the graphical examination clearly demonstrates that the optimal parameter selection has allowed the EWDL-BFSN model to surpass numerous deep learning models regarding their F1-score value. Consequently, the findings prove that the EWDL-BFSN model outperforms the other state-of-the-art methods in exactly classifying facial emotions.
The comparative analysis of EWDL-BFSN and state-of-the-art models using the CK+ dataset is presented in Table 3, clearly showing that the proposed EWDL-BFSN model has superior performance for FER. Similarly, the comparative analysis of EWDL-BFSN and state-of-the-art models using the FER-2013 dataset is presented in Table 4.
Methods | Performances (%) | |||
Accuracy | Sensitivity | Specificity | F1-score | |
CNN [21] | 94.76 | 78.5 | 78.53 | 78.08 |
ResNet-10 [21] | 93.89 | 73.65 | 76.11 | 74.29 |
AlexNet [21] | 95.88 | 83.25 | 83.1 | 82.5 |
CNN-VGG19 [35] | 94.03 | 82.95 | 81.59 | 81.75 |
Inception V3 [35] | 93.74 | 80.23 | 84.06 | 73.82 |
MobileNet [35] | 92.32 | 89.74 | 83.81 | 86.52 |
SVM [35] | 91.64 | 89.17 | 82.18 | 87.55 |
ResNet50 [35] | 88.54 | 90.96 | 83.65 | 85.99 |
HGSO-DLFER [35] | 98.65 | 98.45 | 84.99 | 87.78 |
BIPFER-EOHDL [21] | 99.05 | 88.5 | 99 | 90.93 |
Proposed | 99.37 | 99.2 | 99.48 | 98.21 |
Methods [21] | Performances (%) | |||
Accuracy | Sensitivity | Specificity | F1-score | |
Naïve Bayes | 40.55 | 40.57 | 45.59 | 40.51 |
Decision tree | 51.52 | 51.79 | 51.64 | 51.71 |
SVM | 62.58 | 62.52 | 63.58 | 59.53 |
Random forest | 62.51 | 63.5 | 64.51 | 60.54 |
Logistic regression | 51.55 | 51.66 | 49.65 | 49.66 |
CNN-3 | 80.79 | 81.63 | 81.79 | 81.73 |
CNN | 64.8 | 61.59 | 64.25 | 61.69 |
ResNet-101 | 63.43 | 60.11 | 62.99 | 60.52 |
AlexNet | 65.3 | 61.76 | 64.34 | 62.03 |
BIPFER-EOHDL | 88.5 | 83.42 | 83.48 | 83.5 |
Proposed | 99.25 | 98.21 | 97.41 | 98.15 |
The training and testing data from both CK+ and FER-2013 datasets are combined to examine the accuracy and loss of the EWDL-BFSN model for predicting facial emotions. Around 20% of the data is applied for testing and the remaining 80% is considered for training the EWDL-BFSN model. Figure 8 establishes the testing and training accuracy of the EWDL-BFSN model for FER. Here, the accuracy performance of the EWDL-BFSN model is assessed by fluctuating the epoch size from 0 to 300 and observed in a graphical way. In terms of accuracy during testing and training, the graphical portrayal seems to be closely identical. Correspondingly, the EWDL-BFSN model that is trained for 300 epochs and tested using the CK+ and FER-2013 datasets is examined regarding testing and training loss. The EWDL-BFSN model accomplishes a little loss once the epoch size is extended, as depicted in the graphical representation. The loss performance accomplished using CK+ and FER-2013 datasets is disclosed in Figure 9. The proposed study got minimum loss performance due to an efficient data training procedure over the EWDL-BFSN model.
To calculate the robustness of each phase of the EWDL-BFSN model, a number of ablation studies are presented for accurately classifying facial emotions. To conduct the ablation investigation, the EWDL-BFSN model is split into four distinct components: module-A, module-B, module-C, and module-D. The effectiveness of each stage in the EWDL-BFSN model is assessed independently based on the accuracy, sensitivity, specificity, and F1-score provided by these modules. Module-A suggests that the EWDL-BFSN model operates without executing pre-processing; Module-B indicates that no feature extraction process is performed; Module-C indicates that the feature selection process is not performed; and Module-D suggests that the EWDL-BFSN model performs classification without using WOA.
The performance obtained for the EWDL-BFSN model in the ablation study is shown in Table 5. Compared to all other modules, module-A has a decrease in performance. This results from the pre-processing stage being excluded. Here, the process is accomplished by feeding the obtained input image directly into the stage of feature extraction. Due to noisy images, the EWDL-BFSN model cannot achieve better performance without pre-processing the input image. In module-B, the pre-processed images are sent directly to IBoA for FER, eliminating the feature extraction procedure. Nevertheless, feature selection is used to reduce feature dimensionality, which enhances the EWDL-BFSN model's effectiveness. In the same way, module-C excludes the optimal feature selection process and provides the output from SqueezeNet feature extraction directly to the classification model. Additionally, module-D analyzes the performance without using a WOA in EK-ResNet50 network for FER classification and proves that WOA is necessary to achieve better FER performance. Consequently, it is believed that every step is important for improving the EWDL-BFSN model's performance in accurately predicting facial emotions.
Metrics | Module-A | Module-B | Module-C | Module-D |
Accuracy | 93.67 | 95.24 | 94.97 | 96.75 |
Sensitivity | 93.89 | 94.16 | 95.48 | 96.67 |
Specificity | 95.17 | 96.78 | 95.89 | 97.73 |
F1-score | 93.24 | 94.02 | 93.78 | 97.27 |
In this section, the EWDL-BFSN model is evaluated using other standard datasets in order to infer its applicability in different scenarios. For comparison, other state-of-the-art methods [deep neural network (DNN) [36], hybrid deep CNN [37], appearance-based fused descriptors model [38], distance and shape signature features–based multilayer perceptron model [39], and deep CNN with bilinear pooling [40] are utilized. Table 6 offers the analysis of the EWDL-BFSN model with other standard datasets: Japanese female facial expression (JAFFE), Karolinska directed emotional faces (KDEF), Radboud faces database (RaFD), and Indian movie face benchmark database (IMFDB). It is clear that the proposed EWDL-BFSN model has better performance than the other state-of-the-art methods for different datasets.
Method | Dataset | Accuracy (%) |
Deep neural network (DNN) [36] | JAFFE | 96.91 |
Hybrid deep CNN [37] | JAFFE | 98.14 |
KDEF | 95.29 | |
RaFD | 98.86 | |
Appearance-based fused descriptors model [38] | KDEF | 90.12 |
RaFD | 95.54 | |
JAFFE | 96.17 | |
Distance and shape signature features–based multilayer perceptron model [39] | JAFFE | 96.4 |
Deep CNN with bilinear pooling [40] | IMFDB | 64.17 |
Proposed model | JAFFE | 99.26 |
KDEF | 98.76 | |
RaFD | 98.65 | |
IMFDB | 97.56 |
Recently, deep learning–based methods have been suggested to be most effective for image sentiment analysis methodologies. In [41], the sentiment conveyed in tweets was examined regarding a potential emergency at various points within a specified region. Two scenarios for binary and multi-class were considered to test the RASA model. In order to obtain keywords based on tweet feeds and interpretations, it employed the LSTM technique with word embedding. A CNN model for human facial expression identification was studied in [42]; seven emotions were predicted and identified using the facial action coding system model. The findings show that 79.8% accuracy was achieved without the use of optimization techniques. The ResNet18 BNN network, which is based on the conventional Bayesian neural network design, was introduced in [43] for classifying human facial expressions into seven major classes. When tested on the FER-2013 test set, this model obtained 71.5 and 73.1% accuracy in the PublicTestSet and PrivateTestSet. Besides, a facial emotion identification system with automatic face detection and facial expression recognition was presented in [44]. Four deep CNNs and a label smoothing technique were employed to deal with mislabeled training data, as opposed to an ensemble model. The accuracy of the ExpW, FER-2013, and SFEW 2.0 datasets was 72.72, 51.97, and 71.82%, respectively. In [45], a face-sensitive CNN (FS-CNN) was offered for the recognition of human emotions. A deep learning–based technique that recognizes online students' real-time activity based on their facial emotions was suggested in [46], and a FER system that can recognize emotions from mask-covered faces was given in [47]. The ability of the developed FER system to identify emotions and their valence only from the eye region was assessed and contrasted with the outcomes attained when the entire face was taken into consideration.
This research presents the EWDL-BFSN model, which integrates numerous sophisticated methods to introduce a comprehensive solution to FER. With a set of well-defined processes covering pre-processing, feature extraction, feature selection, and classification, the EWDL-BFSN model is methodologically created to improve efficacy as well as the accuracy of emotion detection. The EWDL-BFSN model's advanced feature selection and classification model with parameter tuning procedures are its main advantages. The best possible input images for feature extraction are guaranteed when GWAF is used for image pre-processing. After that, features are extracted from the pre-processed images using SqueezeNet. By choosing the best features, the IBoA further improves the EWDL-BFSN model and ensures that the most pertinent features are used for FER. The EK-ResNet50 network manages the classification and effectively identifies and classifies face emotions by employing the chosen features. The WOA, a nature-inspired metaheuristic algorithm, is a crucial constituent in optimizing the tunable parameters of the EK-ResNet50 model, thereby improving its overall performance. Tests of the EWDL-BFSN model on the CK+ and FER-2013 datasets yielded very encouraging results, with overall accuracies of 99.37 and 99.25%, respectively. These measures show the EWDL-BFSN model's superiority over other state-of-the-art techniques for classifying facial emotions. Performance indicators including sensitivity, F1-score, specificity, and accuracy are included to give a thorough assessment of the model's abilities. However, there are some cases in which the model may fail to correctly classify emotions. For instance, the EWDL-BFSN can misclassify the emotions in situations where the facial expressions are subtle or ambiguous, such as expressions with low intensity or mixed emotions. Misclassifications can also result from incomplete training data representation of certain emotions, illumination, position, face occlusion variability, and data imbalances where specific emotion classes are underrepresented. In order to handle failure instances and enhance overall performance, it is required to further improve the robustness of the model by using strategies like data augmentation and regularization. Furthermore, even if the model works well on the FER-2013 and CK+ datasets, it is still unclear whether it can be employed in other less regulated datasets. The necessity for exact hyperparameter tuning also highlights a possible area for development, because poor hyperparameter selection can be compromised by suboptimal hyperparameters.
This paper proposes a novel EWDL-BFSN model for effectively identifying facial emotions. The EWDL-BFSN model chooses the best features and modifies classifier hyperparameters in order to automatically and effectively recognize facial emotions. The EWDL-BFSN uses GWAF for pre-processing collected images and SqueezeNet for extracting key features. The optimal features are chosen by IBoA, whereas the emotion recognition and classification are handled by the EK-ResNet50 network. Furthermore, the hyperparameters of EK-ResNet50 model are optimized through the application of WOA. The CK+ and FER-2013 publicly accessible datasets are used to train and evaluate the model using the Python platform. A comprehensive simulation study is conducted to verify the higher FER results obtained by the EWDL-BFSN model. According to the simulation results, FER outcomes of the EWDL-BFSN model are more effective than the other current methods. The overall accuracy of the EWDL-BFSN model on CK+ and FER-2013 datasets is 99.37 and 99.25%, respectively. Even if a high accuracy rate is obtained through the EWDL-BFSN model, it requires more computation resources due to complexity at both the training and testing phases. Besides, the datasets employed to test the model's performance cannot accurately reflect the diversity of real-world situations. To overcome the limitations, more effective hybrid models and optimization strategies that minimize computational load without minimizing performance will be developed in future work. To ensure generalizability and robustness, more evaluation is made by including a wider range of datasets with more variability.
The authors declare they have not used Artificial Intelligence (AI) tools in the creation of this article.
The authors declare there is no conflict of interest.
[1] | S. K. Singh, R. K. Thakur, S. Kumar, R. Anand, Deep learning and machine learning based facial emotion detection using CNN, in 2022 9th International Conference on Computing for Sustainable Global Development (INDIACom), New Delhi, India, (2022), 530–535. https://doi.org/10.23919/INDIACom54597.2022.9763165 |
[2] |
A. R. Khan, Facial emotion recognition using conventional machine learning and deep learning methods: current achievements, analysis and remaining challenges, Information, 13 (2022), 268. https://doi.org/10.3390/info13060268 doi: 10.3390/info13060268
![]() |
[3] |
V. M. Joshi, R. B. Ghongade, A. M. Joshi, R. V. Kulkarni, Deep BiLSTM neural network model for emotion detection using cross-dataset approach, Biomed. Signal Process. Control, 73 (2022), 103407. https://doi.org/10.1016/j.bspc.2021.103407 doi: 10.1016/j.bspc.2021.103407
![]() |
[4] |
A. Aggarwal, A. Srivastava, A. Agarwal, N. Chahal, D. Singh, A. A. Alnuaim, et al., Two-way feature extraction for speech emotion recognition using deep learning, Sensors, 22 (2022), 2378. https://doi.org/10.3390/s22062378 doi: 10.3390/s22062378
![]() |
[5] |
M. F. Bashir, A. R. Javed, M. U. Arshad, T. R. Gadekallu, W. Shahzad, M. O. Beg, Context-aware emotion detection from low-resource URDU language using deep neural network, ACM Trans. Asian Low-Resour. Lang. Inf. Process., 22 (2023), 1–30. https://doi.org/10.1145/3528576 doi: 10.1145/3528576
![]() |
[6] |
I. Lasri, A. Riadsolh, M. Elbelkacemi, Facial emotion recognition of deaf and hard-of-hearing students for engagement detection using deep learning, Educ. Inf. Technol., 28 (2023), 4069–4092. https://doi.org/10.1007/s10639-022-11370-4 doi: 10.1007/s10639-022-11370-4
![]() |
[7] |
M. Mukhiddinov, O. Djuraev, F. Akhmedov, A. Mukhamadiyev, J. Cho, Masked face emotion recognition based on facial landmarks and deep learning approaches for visually impaired people, Sensors, 23 (2023), 1080. https://doi.org/10.3390/s23031080 doi: 10.3390/s23031080
![]() |
[8] |
F. M. Talaat, Z. H. A. Zainab, R. R. Mostafa, N. El-Rashidy, Real-time facial emotion recognition model based on kernel autoencoder and convolutional neural network for autism children, Soft Comput., 28 (2024), 1–14. https://doi.org/10.21203/rs.3.rs-2387030/v1 doi: 10.21203/rs.3.rs-2387030/v1
![]() |
[9] |
B. Sowmya, S. A. Alex, A. Kanavalli, S. Supreeth, G. Shruthi, S. Rohith, Machine learning model for emotion detection and recognition using an enhanced convolutional neural network, J. Integr. Sci. Technol., 12 (2024), 786. https://doi.org/10.62110/sciencein.jist.2024.v12.786 doi: 10.62110/sciencein.jist.2024.v12.786
![]() |
[10] |
B. Bakariya, A. Singh, H. Singh, P. Raju, R. Rajpoot, K. K. Mohbey, Facial emotion recognition and music recommendation system using CNN-based deep learning techniques, Evol. Syst., 15 (2024), 641–658. https://doi.org/10.1007/s12530-023-09506-z doi: 10.1007/s12530-023-09506-z
![]() |
[11] | K. Jhadi, N. Tiwari, M. Chawla, Review of machine and deep learning techniques for expression based facial emotion recognition, in 2024 IEEE International Students' Conference on Electrical, Electronics and Computer Science (SCEECS), Bhopal, India, (2024), 1–6. https://doi.org/10.1109/SCEECS61402.2024.10482176 |
[12] |
H. B. U. Haq, W. Akram, M. N. Irshad, A. Kosar, M. Abid, Enhanced real-time facial expression recognition using deep learning, Acadlore Trans. AI Mach. Learn., 3 (2024), 24–35. https://doi.org/10.56578/ataiml030103 doi: 10.56578/ataiml030103
![]() |
[13] | A. Jaiswal, A. K. Raju, S. Deb, Facial emotion detection using deep learning, in 2020 international conference for emerging technology (INCET), Belgaum, India, (2020), 1–5. https://doi.org/10.1109/INCET49848.2020.9154121 |
[14] | E. Pranav, S. Kamal, C. S. Chandran, M. H. Supriya, Facial emotion recognition using deep convolutional neural network, in 2020 6th International Conference on Advanced Computing and Communication Systems (ICACCS), Coimbatore, India, (2020), 317–320. https://doi.org/10.1109/ICACCS48705.2020.9074302 |
[15] |
W. Mellouk, W. Handouzi, Facial emotion recognition using deep learning: review and insights, Procedia Comput. Sci., 175 (2020), 689–694. https://doi.org/10.1016/j.procs.2020.07.101 doi: 10.1016/j.procs.2020.07.101
![]() |
[16] |
S. A. Hussain, A. S. A. Al Balushi, A real time face emotion classification and recognition using deep learning model, J. Phys. Conf. Ser., 1432 (2020), 012087. https://doi.org/10.1088/1742-6596/1432/1/012087 doi: 10.1088/1742-6596/1432/1/012087
![]() |
[17] |
M. A. H. Akhand, S. Roy, N. Siddique, M. A. S. Kamal, T. Shimamura, Facial emotion recognition using transfer learning in the deep CNN, Electronics, 10 (2021), 1036. https://doi.org/10.3390/electronics10091036 doi: 10.3390/electronics10091036
![]() |
[18] |
M. K. Chowdary, T. N. Nguyen, D. J. Hemanth, Deep learning-based facial emotion recognition for human–computer interaction applications, Neural Comput. Appl., 35 (2023), 23311–23328. https://doi.org/10.1007/s00521-021-06012-8 doi: 10.1007/s00521-021-06012-8
![]() |
[19] | I. P. R. E. Wicaksana, G. R. Davinsi, M. A. Afriyanto, A. Wibowo, P. A. Suri, Systematic literature review: The influence and effectiveness of deep learning in image processing for emotion recognition, 2024. https://doi.org/10.21203/rs.3.rs-3856084/v1 |
[20] |
G. Meena, K. K. Mohbey, A. Indian, M. Z. Khan, S. Kumar, Identifying emotions from facial expressions using a deep convolutional neural network-based approach, Multimedia Tools Appl., 83 (2024), 15711–15732. https://doi.org/10.1007/s11042-023-16174-3 doi: 10.1007/s11042-023-16174-3
![]() |
[21] |
A. A. Alzahrani, Bioinspired image processing enabled facial emotion recognition using equilibrium optimizer with a hybrid deep learning model, IEEE Access, 12 (2024), 22219–22229. https://doi.org/10.1109/ACCESS.2024.3359436 doi: 10.1109/ACCESS.2024.3359436
![]() |
[22] |
H. Tao, Q. Duan, Hierarchical attention network with progressive feature fusion for facial expression recognition, Neural Networks, 170 (2024), 337–348. https://doi.org/10.1016/j.neunet.2023.11.033 doi: 10.1016/j.neunet.2023.11.033
![]() |
[23] |
F. M. Alamgir, M. S. Alam, An artificial intelligence driven facial emotion recognition system using hybrid deep belief rain optimization, Multimedia Tools Appl., 82 (2023), 2437–2464. https://doi.org/10.1007/s11042-022-13378-x doi: 10.1007/s11042-022-13378-x
![]() |
[24] |
P. M. A. Kumar, J. B. Maddala, K. M. Sagayam, Enhanced facial emotion recognition by optimal descriptor selection with neural network, IETE J. Res., 69 (2023), 2595–2614. https://doi.org/10.1080/03772063.2021.1902868 doi: 10.1080/03772063.2021.1902868
![]() |
[25] |
N. Kumari, R. Bhatia, Efficient facial emotion recognition model using deep convolutional neural network and modified joint trilateral filter, Soft Comput., 26 (2022), 7817–7830. https://doi.org/10.1007/s00500-022-06804-7 doi: 10.1007/s00500-022-06804-7
![]() |
[26] | B. Koonce, B. E. Koonce, Convolutional Neural Networks with Swift for Tensorflow: Image Recognition and Dataset Categorization, USA: Apress, New York, NY, (2021), 109–123. https://doi.org/10.1007/978-1-4842-6168-2_10 |
[27] | F. N. Iandola, S. Han, M. W. Moskewicz, K. Ashraf, W. J. Dally, K. Keutzer, SqueezeNet: AlexNet-level accuracy with 50x fewer parameters and < 0.5 MB model size, preprint, arXiv: 1602.07360. |
[28] | W. Liu, D. Anguelov, D. Erhan, C. Szegedy, S. Reed, C. Y. Fu, et al., SSD: Single shot multibox detector, in Computer Vision–ECCV 2016: 14th European Conference, Amsterdam, Proceedings, Part I, Springer International Publishing, The Netherlands, 14 (2016), 21–37. https://doi.org/10.1007/978-3-319-46448-0_2 |
[29] |
M. Hubálovská, Š. Hubálovský, P. Trojovský, Botox optimization algorithm: a new human-based metaheuristic algorithm for solving optimization problems, Biomimetics, 9 (2024), 137. https://doi.org/10.3390/biomimetics9030137 doi: 10.3390/biomimetics9030137
![]() |
[30] |
W. Islam, M. Jones, R. Faiz, N. Sadeghipour, Y. Qiu, B. Zheng, Improving performance of breast lesion classification using a ResNet50 model optimized with a novel attention mechanism, Tomography, 8 (2022), 2411–2425. https://doi.org/10.3390/tomography8050200 doi: 10.3390/tomography8050200
![]() |
[31] |
P. Luo, R. Zhang, J. Ren, Z. Peng, J. Li, Switchable normalization for learning-to-normalize deep representation, IEEE Trans. Pattern Anal. Mach. Intell., 43 (2019), 712–728. https://doi.org/10.1109/TPAMI.2019.2932062 doi: 10.1109/TPAMI.2019.2932062
![]() |
[32] |
M. Han, Z. Du, K. F. Yuen, H. Zhu, Y. Li, Q. Yuan, Walrus optimizer: A novel nature-inspired metaheuristic algorithm, Expert Syst. Appl., 239 (2024), 122413. https://doi.org/10.1016/j.eswa.2023.122413 doi: 10.1016/j.eswa.2023.122413
![]() |
[33] | I. J. Goodfellow, D. Erhan, P. L. Carrier, A. Courville, M. Mirza, B. Hamner, et al., Challenges in representation learning: A report on three machine learning contests, in Neural Information Processing: 20th International Conference, ICONIP 2013, Proceedings, Part Ⅲ, Springer-Verlag Berlin Heidelberg, Daegu, Korea, 20 (2013), 117–124. https://doi.org/10.1007/978-3-642-42051-1_16 |
[34] | P. Lucey, J. F. Cohn, T. Kanade, J. Saragih, Z. Ambadar, I. Matthews, The extended Cohn-Kanade dataset (CK+): A complete dataset for action unit and emotion-specified expression, in 2010 IEEE Computer Society Conference on Computer Vision and Pattern Recognition-Workshops, San Francisco, CA, USA, (2010), 94–101. https://doi.org/10.1109/CVPRW.2010.5543262 |
[35] |
H. N. AlEisa, F. Alrowais, N. Negm, N. Almalki, M. Khalid, R. Marzouk, et al., Henry gas solubility optimization with deep learning based facial emotion recognition for human computer interface, IEEE Access, 11 (2023), 62233–62241. https://doi.org/10.1109/ACCESS.2023.3284457 doi: 10.1109/ACCESS.2023.3284457
![]() |
[36] |
S. Benisha, T. T. Mirnalinee, Human facial emotion recognition using deep neural networks, Int. Arab J. Inf. Technol., 20 (2023), 303–309. https://doi.org/10.34028/iajit/20/3/2 doi: 10.34028/iajit/20/3/2
![]() |
[37] |
A. J. Obaid, H. K. Alrammahi, An intelligent facial expression recognition system using a hybrid deep convolutional neural network for multimedia applications, Appl. Sci., 13 (2023), 12049. https://doi.org/10.3390/app132112049 doi: 10.3390/app132112049
![]() |
[38] |
Y. Yaddaden, An efficient facial expression recognition system with appearance-based fused descriptors, Intell. Syst. Appl., 17 (2023), 200166. https://doi.org/10.1016/j.iswa.2022.200166 doi: 10.1016/j.iswa.2022.200166
![]() |
[39] |
A. Barman, P. Dutta, Facial expression recognition using distance and shape signature features, Pattern Recognit. Lett., 145 (2021), 254–261. https://doi.org/10.1016/j.patrec.2017.06.018 doi: 10.1016/j.patrec.2017.06.018
![]() |
[40] |
S. Hossain, S. Umer, R. K. Rout, M. Tanveer, Fine-grained image analysis for facial expression recognition using deep convolutional neural networks with bilinear pooling, Appl. Soft Comput., 134 (2023), 109997. https://doi.org/10.1016/j.asoc.2023.109997 doi: 10.1016/j.asoc.2023.109997
![]() |
[41] |
M. Parimala, R. M. S. Priya, M. P. K. Reddy, C. L. Chowdhary, R. K. Poluru, S. Khan, Spatiotemporal‐based sentiment analysis on tweets for risk assessment of event using deep learning approach, Softw.: Pract. Exper., 51 (2021), 550–570. https://doi.org/10.1002/spe.2851 doi: 10.1002/spe.2851
![]() |
[42] | P. Babajee, G. Suddul, S. Armoogum, R. Foogooa, Identifying human emotions from facial expressions with deep learning, in 2020 Zooming Innovation in Consumer Technologies Conference (ZINC), (2020), 36–39. https://doi.org/10.1109/ZINC50678.2020.9161445 |
[43] | Y. Tai, Y. Tan, W. Gong, H. Huang, Bayesian convolutional neural networks for seven basic facial expression classifications, preprint, arXiv: 2107.04834. |
[44] |
N. K. Benamara, M. Val-Calvo, J. R. Alvarez-Sanchez, A. Diaz-Morcillo, J. M. Ferrandez-Vicente, E. Fernandez-Jover, et al., Real-time facial expression recognition using smoothed deep neural network ensemble, Integr. Comput.-Aided Eng., 28 (2021), 97–111. https://doi.org/10.3233/ICA-200643 doi: 10.3233/ICA-200643
![]() |
[45] |
Y. Said, M. Barr, Human emotion recognition based on facial expressions via deep learning on high-resolution images, Multimedia Tools Appl., 80 (2021), 25241–25253. https://doi.org/10.1007/s11042-021-10918-9 doi: 10.1007/s11042-021-10918-9
![]() |
[46] |
S. Gupta, P. Kumar, R. K. Tekchandani, Facial emotion recognition based real-time learner engagement detection system in online learning context using deep learning models, Multimedia Tools Appl., 82 (2023), 11365–11394. https://doi.org/10.1007/s11042-022-13558-9 doi: 10.1007/s11042-022-13558-9
![]() |
[47] |
G. Castellano, B. De Carolis, N. Macchiarulo, Automatic facial emotion recognition at the COVID-19 pandemic time, Multimedia Tools Appl., 82 (2023), 12751–12769. https://doi.org/10.1007/s11042-022-14050-0 doi: 10.1007/s11042-022-14050-0
![]() |
Training stage (80%) | Testing stage (20%) | |||||||
Class | Accuracy | Sensitivity | Specificity | F1-score | Accuracy | Sensitivity | Specificity | F1-score |
Surprise | 99.24 | 88.22 | 99.51 | 90.82 | 99.56 | 94.15 | 98.97 | 87.82 |
Sadness | 98.08 | 99.71 | 99.45 | 99.08 | 98.74 | 95.58 | 98.31 | 91.61 |
Neutral | 99.54 | 99.06 | 99.41 | 96.73 | 99.37 | 97.10 | 98.94 | 95.09 |
Happiness | 99.70 | 96.00 | 99.71 | 97.39 | 98.71 | 94.05 | 98.25 | 87.55 |
Fear | 98.52 | 98.01 | 99.79 | 91.90 | 98.61 | 95.52 | 98.72 | 95.89 |
Disgust | 98.64 | 97.15 | 99.50 | 97.62 | 98.46 | 91.96 | 98.99 | 99.72 |
Contempt | 99.25 | 96.29 | 100.00 | 99.55 | 98.55 | 92.34 | 100.00 | 100.00 |
Anger | 99.24 | 96.35 | 99.62 | 96.16 | 98.86 | 94.39 | 98.88 | 93.95 |
Training stage (70%) | Testing stage (30%) | |||||||
Class | Accuracy | Sensitivity | Specificity | F1-score | Accuracy | Sensitivity | Specificity | F1-score |
Surprise | 99.06 | 88.2 | 99.43 | 90.76 | 99.42 | 94.11 | 98.78 | 87.67 |
Sadness | 98.03 | 99.67 | 99.41 | 99.05 | 98.46 | 95.52 | 98.24 | 91.46 |
Neutral | 99.80 | 99.02 | 99.36 | 96.27 | 99.17 | 96.89 | 98.26 | 95.01 |
Happiness | 99.61 | 95.89 | 99.65 | 97.28 | 98.56 | 94.01 | 98.51 | 87.23 |
Fear | 98.25 | 98 | 99.71 | 91.82 | 98.47 | 95.05 | 98.27 | 95.35 |
Disgust | 98.39 | 97.05 | 99.43 | 97.32 | 98.4 | 91.35 | 98.82 | 99.47 |
Contempt | 99.18 | 96.21 | 99.32 | 99.25 | 98.46 | 92.21 | 99.76 | 100.00 |
Anger | 99.21 | 96.3 | 99.38 | 96.07 | 98.57 | 94.27 | 98.57 | 93.53 |
Methods | Performances (%) | |||
Accuracy | Sensitivity | Specificity | F1-score | |
CNN [21] | 94.76 | 78.5 | 78.53 | 78.08 |
ResNet-10 [21] | 93.89 | 73.65 | 76.11 | 74.29 |
AlexNet [21] | 95.88 | 83.25 | 83.1 | 82.5 |
CNN-VGG19 [35] | 94.03 | 82.95 | 81.59 | 81.75 |
Inception V3 [35] | 93.74 | 80.23 | 84.06 | 73.82 |
MobileNet [35] | 92.32 | 89.74 | 83.81 | 86.52 |
SVM [35] | 91.64 | 89.17 | 82.18 | 87.55 |
ResNet50 [35] | 88.54 | 90.96 | 83.65 | 85.99 |
HGSO-DLFER [35] | 98.65 | 98.45 | 84.99 | 87.78 |
BIPFER-EOHDL [21] | 99.05 | 88.5 | 99 | 90.93 |
Proposed | 99.37 | 99.2 | 99.48 | 98.21 |
Methods [21] | Performances (%) | |||
Accuracy | Sensitivity | Specificity | F1-score | |
Naïve Bayes | 40.55 | 40.57 | 45.59 | 40.51 |
Decision tree | 51.52 | 51.79 | 51.64 | 51.71 |
SVM | 62.58 | 62.52 | 63.58 | 59.53 |
Random forest | 62.51 | 63.5 | 64.51 | 60.54 |
Logistic regression | 51.55 | 51.66 | 49.65 | 49.66 |
CNN-3 | 80.79 | 81.63 | 81.79 | 81.73 |
CNN | 64.8 | 61.59 | 64.25 | 61.69 |
ResNet-101 | 63.43 | 60.11 | 62.99 | 60.52 |
AlexNet | 65.3 | 61.76 | 64.34 | 62.03 |
BIPFER-EOHDL | 88.5 | 83.42 | 83.48 | 83.5 |
Proposed | 99.25 | 98.21 | 97.41 | 98.15 |
Metrics | Module-A | Module-B | Module-C | Module-D |
Accuracy | 93.67 | 95.24 | 94.97 | 96.75 |
Sensitivity | 93.89 | 94.16 | 95.48 | 96.67 |
Specificity | 95.17 | 96.78 | 95.89 | 97.73 |
F1-score | 93.24 | 94.02 | 93.78 | 97.27 |
Method | Dataset | Accuracy (%) |
Deep neural network (DNN) [36] | JAFFE | 96.91 |
Hybrid deep CNN [37] | JAFFE | 98.14 |
KDEF | 95.29 | |
RaFD | 98.86 | |
Appearance-based fused descriptors model [38] | KDEF | 90.12 |
RaFD | 95.54 | |
JAFFE | 96.17 | |
Distance and shape signature features–based multilayer perceptron model [39] | JAFFE | 96.4 |
Deep CNN with bilinear pooling [40] | IMFDB | 64.17 |
Proposed model | JAFFE | 99.26 |
KDEF | 98.76 | |
RaFD | 98.65 | |
IMFDB | 97.56 |
Training stage (80%) | Testing stage (20%) | |||||||
Class | Accuracy | Sensitivity | Specificity | F1-score | Accuracy | Sensitivity | Specificity | F1-score |
Surprise | 99.24 | 88.22 | 99.51 | 90.82 | 99.56 | 94.15 | 98.97 | 87.82 |
Sadness | 98.08 | 99.71 | 99.45 | 99.08 | 98.74 | 95.58 | 98.31 | 91.61 |
Neutral | 99.54 | 99.06 | 99.41 | 96.73 | 99.37 | 97.10 | 98.94 | 95.09 |
Happiness | 99.70 | 96.00 | 99.71 | 97.39 | 98.71 | 94.05 | 98.25 | 87.55 |
Fear | 98.52 | 98.01 | 99.79 | 91.90 | 98.61 | 95.52 | 98.72 | 95.89 |
Disgust | 98.64 | 97.15 | 99.50 | 97.62 | 98.46 | 91.96 | 98.99 | 99.72 |
Contempt | 99.25 | 96.29 | 100.00 | 99.55 | 98.55 | 92.34 | 100.00 | 100.00 |
Anger | 99.24 | 96.35 | 99.62 | 96.16 | 98.86 | 94.39 | 98.88 | 93.95 |
Training stage (70%) | Testing stage (30%) | |||||||
Class | Accuracy | Sensitivity | Specificity | F1-score | Accuracy | Sensitivity | Specificity | F1-score |
Surprise | 99.06 | 88.2 | 99.43 | 90.76 | 99.42 | 94.11 | 98.78 | 87.67 |
Sadness | 98.03 | 99.67 | 99.41 | 99.05 | 98.46 | 95.52 | 98.24 | 91.46 |
Neutral | 99.80 | 99.02 | 99.36 | 96.27 | 99.17 | 96.89 | 98.26 | 95.01 |
Happiness | 99.61 | 95.89 | 99.65 | 97.28 | 98.56 | 94.01 | 98.51 | 87.23 |
Fear | 98.25 | 98 | 99.71 | 91.82 | 98.47 | 95.05 | 98.27 | 95.35 |
Disgust | 98.39 | 97.05 | 99.43 | 97.32 | 98.4 | 91.35 | 98.82 | 99.47 |
Contempt | 99.18 | 96.21 | 99.32 | 99.25 | 98.46 | 92.21 | 99.76 | 100.00 |
Anger | 99.21 | 96.3 | 99.38 | 96.07 | 98.57 | 94.27 | 98.57 | 93.53 |
Methods | Performances (%) | |||
Accuracy | Sensitivity | Specificity | F1-score | |
CNN [21] | 94.76 | 78.5 | 78.53 | 78.08 |
ResNet-10 [21] | 93.89 | 73.65 | 76.11 | 74.29 |
AlexNet [21] | 95.88 | 83.25 | 83.1 | 82.5 |
CNN-VGG19 [35] | 94.03 | 82.95 | 81.59 | 81.75 |
Inception V3 [35] | 93.74 | 80.23 | 84.06 | 73.82 |
MobileNet [35] | 92.32 | 89.74 | 83.81 | 86.52 |
SVM [35] | 91.64 | 89.17 | 82.18 | 87.55 |
ResNet50 [35] | 88.54 | 90.96 | 83.65 | 85.99 |
HGSO-DLFER [35] | 98.65 | 98.45 | 84.99 | 87.78 |
BIPFER-EOHDL [21] | 99.05 | 88.5 | 99 | 90.93 |
Proposed | 99.37 | 99.2 | 99.48 | 98.21 |
Methods [21] | Performances (%) | |||
Accuracy | Sensitivity | Specificity | F1-score | |
Naïve Bayes | 40.55 | 40.57 | 45.59 | 40.51 |
Decision tree | 51.52 | 51.79 | 51.64 | 51.71 |
SVM | 62.58 | 62.52 | 63.58 | 59.53 |
Random forest | 62.51 | 63.5 | 64.51 | 60.54 |
Logistic regression | 51.55 | 51.66 | 49.65 | 49.66 |
CNN-3 | 80.79 | 81.63 | 81.79 | 81.73 |
CNN | 64.8 | 61.59 | 64.25 | 61.69 |
ResNet-101 | 63.43 | 60.11 | 62.99 | 60.52 |
AlexNet | 65.3 | 61.76 | 64.34 | 62.03 |
BIPFER-EOHDL | 88.5 | 83.42 | 83.48 | 83.5 |
Proposed | 99.25 | 98.21 | 97.41 | 98.15 |
Metrics | Module-A | Module-B | Module-C | Module-D |
Accuracy | 93.67 | 95.24 | 94.97 | 96.75 |
Sensitivity | 93.89 | 94.16 | 95.48 | 96.67 |
Specificity | 95.17 | 96.78 | 95.89 | 97.73 |
F1-score | 93.24 | 94.02 | 93.78 | 97.27 |
Method | Dataset | Accuracy (%) |
Deep neural network (DNN) [36] | JAFFE | 96.91 |
Hybrid deep CNN [37] | JAFFE | 98.14 |
KDEF | 95.29 | |
RaFD | 98.86 | |
Appearance-based fused descriptors model [38] | KDEF | 90.12 |
RaFD | 95.54 | |
JAFFE | 96.17 | |
Distance and shape signature features–based multilayer perceptron model [39] | JAFFE | 96.4 |
Deep CNN with bilinear pooling [40] | IMFDB | 64.17 |
Proposed model | JAFFE | 99.26 |
KDEF | 98.76 | |
RaFD | 98.65 | |
IMFDB | 97.56 |