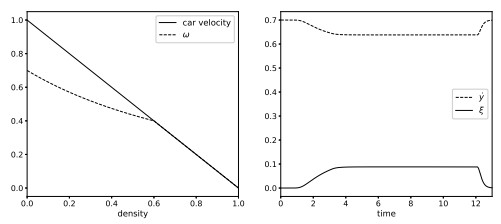
Citation: Kai Su, Xuan Zhang, Qing Liu, Bin Xiao. Strategies of similarity propagation in web service recommender systems[J]. Mathematical Biosciences and Engineering, 2021, 18(1): 530-550. doi: 10.3934/mbe.2021029
[1] | Abraham Sylla . Influence of a slow moving vehicle on traffic: Well-posedness and approximation for a mildly nonlocal model. Networks and Heterogeneous Media, 2021, 16(2): 221-256. doi: 10.3934/nhm.2021005 |
[2] | Paola Goatin, Chiara Daini, Maria Laura Delle Monache, Antonella Ferrara . Interacting moving bottlenecks in traffic flow. Networks and Heterogeneous Media, 2023, 18(2): 930-945. doi: 10.3934/nhm.2023040 |
[3] | Maria Laura Delle Monache, Paola Goatin . Stability estimates for scalar conservation laws with moving flux constraints. Networks and Heterogeneous Media, 2017, 12(2): 245-258. doi: 10.3934/nhm.2017010 |
[4] | Felisia Angela Chiarello, Giuseppe Maria Coclite . Nonlocal scalar conservation laws with discontinuous flux. Networks and Heterogeneous Media, 2023, 18(1): 380-398. doi: 10.3934/nhm.2023015 |
[5] | Raimund Bürger, Harold Deivi Contreras, Luis Miguel Villada . A Hilliges-Weidlich-type scheme for a one-dimensional scalar conservation law with nonlocal flux. Networks and Heterogeneous Media, 2023, 18(2): 664-693. doi: 10.3934/nhm.2023029 |
[6] | Markus Musch, Ulrik Skre Fjordholm, Nils Henrik Risebro . Well-posedness theory for nonlinear scalar conservation laws on networks. Networks and Heterogeneous Media, 2022, 17(1): 101-128. doi: 10.3934/nhm.2021025 |
[7] | Gabriella Bretti, Roberto Natalini, Benedetto Piccoli . Numerical approximations of a traffic flow model on networks. Networks and Heterogeneous Media, 2006, 1(1): 57-84. doi: 10.3934/nhm.2006.1.57 |
[8] | Christophe Chalons, Paola Goatin, Nicolas Seguin . General constrained conservation laws. Application to pedestrian flow modeling. Networks and Heterogeneous Media, 2013, 8(2): 433-463. doi: 10.3934/nhm.2013.8.433 |
[9] | Jan Friedrich, Oliver Kolb, Simone Göttlich . A Godunov type scheme for a class of LWR traffic flow models with non-local flux. Networks and Heterogeneous Media, 2018, 13(4): 531-547. doi: 10.3934/nhm.2018024 |
[10] | Martin Gugat, Alexander Keimer, Günter Leugering, Zhiqiang Wang . Analysis of a system of nonlocal conservation laws for multi-commodity flow on networks. Networks and Heterogeneous Media, 2015, 10(4): 749-785. doi: 10.3934/nhm.2015.10.749 |
Delle Monache and Goatin developed in [20] a macroscopic model aiming at describing the situation in which a slow moving large vehicle – a bus for instance – reduces the road capacity and thus generates a moving bottleneck for the surrounding traffic flow. Their model is given by a Cauchy problem for Lightwill-Whitham-Richards scalar conservation law in one space dimension with local point constraint. The constraint is prescribed along the slow vehicle trajectory
˙y(t)=ω(ρ(y(t)+,t)). | (A) |
Above,
˙y(t)=ω(∫Rρ(x+y(t),t)μ(x)dx), | (B) |
where
The paper is organized as follows. Sections 2 and 3 are devoted to the proof of the well-posedness of the model. In Section 4 we introduce the numerical finite volume scheme and prove its convergence. An important step of the reasoning is to prove a
Note that we find it convenient to study the problem in the bus frame, which means setting
{∂tρ+∂x(F(˙y(t),ρ))=0R×(0,T)ρ(x,0)=ρ0(x+y0)x∈RF(˙y(t),ρ)|x=0≤Q(˙y(t))t∈(0,T)˙y(t)=ω(∫Rρ(x,t)μ(x)dx)t∈(0,T)y(0)=y0. | (1) |
Above,
F(˙y(t),ρ)=f(ρ)−˙y(t)ρ |
denotes the normal flux through the curve
f(ρ)≥0, f(0)=f(R)=0, ∃! ¯ρ∈(0,R), f′(ρ)(¯ρ−ρ)>0 for a.e. ρ∈(0,R). | (2) |
In [20], the authors chose the function
Q:[0,‖ω‖L∞]→R+ |
can be any Lipschitz continuous function. It is a well known fact that in general, the total variation of an entropy solution to a constraint Cauchy problem may increase (see [17,Section 2] for an example). However, this increase can be controlled if the constraint level does not reach the maximum level. A mild assumption on
ρ0∈L1(R;[0,R])∩BV(R). |
Throughout the paper, we denote by
Φ(a,b)=sign(a−b)(f(a)−f(b))andΦ˙y(t)(a,b)=Φ(a,b)−˙y(t)|a−b| |
the entropy fluxes associated with the Kružkov entropy
Definition 2.1. A couple
(ⅰ) the following regularity is fulfilled:
ρ∈C([0,T];L1loc(R)); | (3) |
(ⅱ) for all test functions
∫τ′τ∫R|ρ−κ|∂tφ+Φ˙y(t)(ρ,κ)∂xφdxdt+∫R|ρ(x,τ)−κ|φ(x,τ)dx−∫R|ρ(x,τ′)−κ|φ(x,τ′)dx+2∫τ′τR˙y(t)(κ,q(t))φ(0,t)dt≥0, | (4) |
where
R˙y(t)(κ,q(t))=F(˙y(t),κ)−min{F(˙y(t),κ),q(t)}andq(t)=Q(˙y(t)); |
(ⅲ) for all test functions
−∫τ′τ∫R+ρ∂t(φψ)+F(˙y(t),ρ)∂x(φψ)dxdt−∫R+ρ(x,τ)φ(x)ψ(τ)dx+∫R+ρ(x,τ′)φ(x)ψ(τ′)dx≤∫τ′τq(t)ψ(t)dt; | (5) |
(ⅳ) the following weak ODE formulation is verified for all
y(t)=y0+∫t0ω(∫Rρ(x,s)μ(x)dx)ds. | (6) |
Definition 2.2. We will call
ρ∈L∞((0,T);BV(R)). |
Remark 1. It is more usual to formulate (4) with
Remark 2. As it happens, the time-continuity regularity (3) is actually a consequence of inequalities (4). Indeed, we will use the result [12,Theorem 1.2] which states that if
∫T0∫Ω|ρ−κ|∂tφ+Φ˙y(t)(ρ,κ)∂xφdxdt+∫Ω|ρ0(x)−κ|φ(x,0)dx≥0, |
then
Remark 3. Any admissible weak solution
∫τ′τ∫R−ρ∂t(φψ)+F(˙y(t),ρ)∂x(φψ)dxdt+∫R−ρ(x,τ)φ(x)ψ(τ)dx−∫R−ρ(x,τ′)φ(x)ψ(τ′)dx≤∫τ′τq(t)ψ(t)dt, |
where
The interest of weak formulations (5)-(6) for the flux constraint and for the ODE governing the slow vehicle lies in their stability with respect to
In this section, we prove stability with respect to the initial data and uniqueness for
Lemma 2.3. If
Proof. Denote for all
s(t)=ω(∫Rρ(x,t)μ(x)dx). |
Since
‖μn−μ‖L1 ⟶n→+∞ 0andTV(μn) ⟶n→+∞ TV(μ). |
Introduce for all
ξn(t)=∫Rρ(x,t)μn(x)dx. |
Fix
∫T0ξn(t)˙ψ(t)dt=∫T0∫Rρ∂t(ψμn)dxdt=−∫T0∫RF(˙y(t),ρ)∂x(ψμn)dxdt=−∫T0(∫RF(˙y(t),ρ)μ′n(x)dx)ψ(t)dt, |
which means that for all
˙ξn(t)=∫RF(˙y(t),ρ)μ′n(x)dx. |
In particular, since both the sequences
‖ξn‖L∞≤RCand‖˙ξn‖L∞≤C(‖f‖L∞+‖ω‖L∞R). |
Therefore, the sequence
|s(t)−s(τ)|≤2‖ω′‖L∞R‖μn−μ‖L1+‖ω′‖L∞|∫R(ρ(x,t)−ρ(x,τ))μn(x)dx|=2‖ω′‖L∞R‖μn−μ‖L1+‖ω′‖L∞|ξn(t)−ξn(τ)|≤2‖ω′‖L∞R‖μn−μ‖L1+C‖ω′‖L∞(‖f‖L∞+‖ω‖L∞R)⏟K|t−τ|. |
Letting
Before stating the uniqueness result, we make the following additional assumption:
∀s∈[0,‖ω‖L∞], argmaxρ∈[0,R] F(s,ρ)>0. | (7) |
This ensures that for all
Theorem 2.4. Suppose that
for a.e. t∈(0,T), ‖ρ1(t)−ρ2(t)‖L1≤(|y10−y20|TV(ρ10)+‖ρ10−ρ20‖L1)exp(αt) | (8) |
and
∀t∈[0,T], |y1(t)−y2(t)|≤|y10−y20|+(β|y10−y20|+γ‖ρ10−ρ20‖L1)(exp(αt)−1). | (9) |
In particular, Problem (1) admits at most one
Proof. Since
∀t∈[0,T], TV(ρ1(t))≤C. |
Lemma 2.3 ensures that
‖ρ1(t)−ρ2(t)‖L1≤|y10−y20|TV(ρ10)+‖ρ10−ρ20‖L1+(2‖Q′‖L∞+2R+C)∫t0|˙y1(s)−˙y2(s)|ds. | (10) |
Moreover, since for a.e.
|˙y1(t)−˙y2(t)|≤‖ω′‖L∞‖μ‖L∞‖ρ1(t)−ρ2(t)‖L1, |
Gronwall's lemma yields (8) with
|y1(t)−y2(t)|≤|y10−y20|+∫t0|˙y1(s)−˙y2(s)|ds≤|y10−y20|+‖ω′‖L∞‖μ‖L∞∫t0‖ρ1(s)−ρ2(s)‖L1ds≤|y10−y20|+(β|y10−y20|+γ‖ρ10−ρ20‖L1)(exp(αt)−1), |
where
β=TV(ρ10)2‖Q′‖L∞+2R+Candγ=12‖Q′‖L∞+2R+C. |
The uniqueness of a
Remark 4. Up to inequality (10), our proof was very much following the one of [21,Theorem 2.1]. However, the authors of [21] faced an issue to derive a Lipschitz stability estimate between the car densities and the slow vehicle velocities starting from
|ω(ρ1(0+,t))−ω(ρ2(0+,t))|. |
For us, due to the nonlocality of our problem, it was straightforward to obtain the bound
|ω(∫Rρ1(x,t)μ(x)dx)−ω(∫Rρ2(x,t)μ(x)dx)|≤‖ω′‖L∞‖μ‖L∞‖ρ1(t)−ρ2(t)‖L1. |
Remark 5. A noteworthy consequence of Theorem 2.4 is that existence of a
In [20], to prove existence for their problem, the authors took a wave-front tracking approach. We choose here to use a time-splitting technique. The main advantage of this technique is that it relies on a ready-to-use theory. More precisely, at each time step, we will deal with exact solutions to a conservation law with a flux constraint, which have now become standard, see [17,6,15].
Fix
∀t∈R, ρ0(t)=ρ0(⋅+y0)and∀t∈[0,T], y0(t)=y0. |
Fix
σn(t)=ω(∫Rρn−1(x,t−δ)μ(x)dx), sn=σn(tn) and qn=Q(sn). |
Since both
{∂tρ+∂x(F(sn,ρ))=0R×(tn−1,tn)ρ(x,tn−1)=ρn−1(x,tn−1)x∈RF(sn,ρ)|x=0≤qnt∈(tn−1,tn), |
in the sense that
∙ ρδ(t)=ρ01R−(t)+N+1∑n=1ρn(t)1(tn−1,tn](t)∙ σδ(t),qδ(t),sδ(t)=σn(t),qn,snif t∈(tn−1,tn]∙ yδ(t)=y0+∫t0σδ(u)du. |
First, let us prove that
Proposition 1. The couple
{∂tρδ+∂x(F(sδ(t),ρδ))=0R×(0,T)ρδ(x,0)=ρ0(x+y0)x∈RF(sδ(t),ρδ)|x=0≤qδ(t)t∈(0,T)˙yδ(t)=ω(∫Rρδ(x,t−δ)μ(x)dx)t∈(0,T)yδ(0)=y0, | (11) |
in the sense that
∀t∈[0,T], yδ(t)=y0+∫t0ω(∫Rρδ(x,s−δ)μ(x)dx)ds. |
Proof. By construction, for all
yδ(t)−y0=n−1∑k=1∫tktk−1σk(s)ds+∫ttn−1σn(s)ds=n−1∑k=1∫tktk−1ω(∫Rρk−1(x,s−δ)⏟ρδ(x,s−δ)μ(x)dx)ds+∫ttn−1ω(∫Rρn−1(x,s−δ)⏟ρδ(x,s−δ)μ(x)dx)ds=∫t0ω(∫Rρδ(x,s−δ)μ(x)dx)ds, | (12) |
which proves that
∫tmtn∫R|ρδ−κ|∂tφ+Φsδ(t)(ρδ,κ)∂xφdxdt=m∑k=n+1∫tktk−1∫R|ρk−κ|∂tφ+Φsk(ρk,κ)∂xφdxdt≥m∑k=n+1{∫R|ρk(x,tk)−κ|φ(x,tk)dx−∫R|ρk(x,tk−1)⏟ρk−1(x,tk−1)−κ|φ(x,tk−1)dx−2∫tktk−1Rsk(κ,qk)φ(0,t)dt}=∫R|ρδ(x,tm)−κ|φ(x,tm)dx−∫R|ρδ(x,tn)−κ|φ(x,tn)dx−2∫tmtnRsδ(t)(κ,qδ(t))φ(0,t)dt. |
It is then straightforward to prove that for all
∫τ′τ∫R|ρδ−κ|∂tφ+Φsδ(t)(ρδ,κ)∂xφ dxdt+∫R|ρδ(x,τ)−κ|φ(x,τ)dx−∫R|ρδ(x,τ′)−κ|φ(x,τ′)dx+2∫τ′τRsδ(t)(κ,qδ(t))φ(0,t)dt≥0. | (13) |
Proving that
−∫τ′τ∫R+ρδ∂t(φψ)+F(sδ(t),ρδ)∂x(φψ) dxdt |
and make use once again of the construction of the sequence
−∫τ′τ∫R+ρδ∂t(φψ)+F(sδ(t),ρδ)∂x(φψ) dxdt−∫R+ρδ(x,τ)φ(x)ψ(τ)dx+∫R+ρδ(x,τ′)φ(x)ψ(τ′)dx≤∫τ′τqδ(t)ψ(t)dt. | (14) |
This concludes the proof.
Remark 6. Remark that we have for all
‖σδ‖L∞≤‖ω‖L∞and‖yδ‖L∞≤|y0|+T‖ω‖L∞. |
This means that the sequence
At this point, we propose two ways to obtain compactness for the sequence
Theorem 3.1. Fix
for a.e. s∈(0,‖ω‖L∞), mes{ρ∈[0,R] | f′(ρ)−s=0}=0. | (15) |
Then Problem (1) admits at least one admissible weak solution.
Proof. Condition (15) combined with the obvious uniform
∀δ>0, ∀(x,t)∈R×[0,T], ρδ(x,t)∈[0,R], |
and the results proved by Panov in [30,31] ensure the existence of a subsequence – which we do not relabel – that converges in
For all
yδ(t)−y0=∫t0ω(∫Rρδ(x,s−δ)μ(x)dx)ds=∫t−δ−δω(∫Rρδ(x,s)μ(x)dx)ds=∫t0ω(∫Rρδ(x,s)μ(x)dx)ds+(∫0−δ−∫tt−δ)ω(∫Rρδ(x,s)μ(x)dx)ds. |
The last term vanishes as
yδ(t) ⟶δ→0 y0+∫t0ω(∫Rρ(x,s)μ(x)dx)ds. |
This last quantity is also equal to
‖μn−μ‖L1 ⟶n→+∞ 0andTV(μn) ⟶n→+∞ TV(μ). |
Introduce for every
ξnδ(t)=∫Rρδ(x,t)μn(x)dx. |
Since for all
˙ξnδ(t)=∫RF(sδ(t),ρδ)μ′n(x)dx. |
Moreover, since both the sequences
|σδ(t)−ω(∫Rρ(x,t)μ(x)dx)|≤2‖ω′‖L∞R‖μn−μ‖L1+δ supn∈N ‖ω(ξnδ)‖W1,∞+‖ω′‖L∞|∫R(ρδ(x,t)−ρ(x,t))μ(x)dx|⟶δ→0n→+∞0, |
which proves that
∫T0∫R|ρδ−κ|∂tφ+Φσδ(t)(ρδ,κ)∂xφ dxdt+∫R|ρ0(x+y0)−κ|φ(x,0)dx≥0. |
Then, we let
∫T0∫R|ρ−κ|∂tφ+Φ˙y(t)(ρ,κ)∂xφdxdt+∫R|ρ0(x+y0)−κ|φ(x,0)dx≥0. |
Consequently,
∫τ′τ∫R|ρ−κ|∂tφ+Φ˙y(t)(ρ,κ)∂xφ dxdt+∫R|ρ(x,τ)−κ|φ(x,τ)dx−∫R|ρ(x,τ′)−κ|φ(x,τ′)dx+2∫τ′τR˙y(t)(κ,q(t))φ(0,t)dt≥0. |
Observe that the expression in the left-hand side of the previous inequality is a continuous function of
In this section, we proved an existence result for
Assumption (15) ensures the compactness for sequences of entropy solutions to conservation laws with flux function
To obtain compactness for
Theorem 3.2. Fix
∀s∈[0,‖ω‖L∞], F(s,⋅)∈C1([0,R]∖{¯ρs}), | (16) |
where
∃ε>0, ∀s∈[0,‖ω‖L∞], Q(s)≤maxρ∈[0,R] F(s,ρ)−ε. | (17) |
Then Problem (1) admits a unique admissible weak solution, which is also
Proof. Fix
σδ(t)=ω(∫Rρδ(x,t−δ)μ(x)dx). |
Following the steps of the proof of Lemma 2.3, we can show that for all
TV(ρδ(t))≤TV(ρ0)+4R+Cε(TV(qδ)+TV(sδ))≤TV(ρ0)+4R+Cε(1+‖Q′‖L∞)TV(sδ). | (18) |
Consequently for all
∀t∈[0,T], ρδ(t) ⟶δ→0 ρ(t) in L1loc(R). |
With this convergence, we can follow the proof of Theorem 3.1 to show that
To end this section, we now study the stability of Problem (1) with respect to the weight function
∀g∈L∞(R), ∫Rg(x)μℓ(x)dx ⟶ℓ→+∞ ∫Rg(x)μ(x)dx. | (19) |
Let
{∂tρℓ+∂x(F(˙yℓ(t),ρℓ))=0R×(0,T)ρℓ(x,0)=ρℓ0(x+yℓ0)x∈RF(˙yℓ(t),ρℓ)|x=0≤Q(˙yℓ(t))t∈(0,T)˙yℓ(t)=ω(∫Rρℓ(x,t)μℓ(x)dx)t∈(0,T)yℓ(0)=yℓ0. |
Remark 7. Using the same arguments as in Remark 6 and as in the proof of Theorem 3.1, we get that up to the extraction of a subsequence,
Theorem 3.3. The couple
Proof. The sequence
∀ε>0, ∃α>0, ∀A∈B(R), mes(A)<α⟹ ∀ℓ∈N, ∫Aμℓ(x)dx≤ε | (20) |
and
∀ε>0, ∃X>0, ∀ℓ∈N, ∫|x|≥Xμℓ(x)dx≤ε. | (21) |
Fix
mes([−X,X]∖Et)<α and ρℓ(⋅,t)⟶ρ(⋅,t) uniformly on Et. |
For a sufficiently large
|∫Rρℓ(x,t)μℓ(x)dx−∫Rρ(x,t)μ(x)dx|≤∫|x|≥X|ρℓ−ρ|μℓdx+|∫Et(ρℓ−ρ)μℓdx|+|∫[−X,X]∖Et(ρℓ−ρ)μℓdx|+|∫Rρμℓdx−∫Rρμdx|≤Rε+‖ρℓ−ρ‖L∞(Et)∫Etμℓ(x)dx+R∫[−X,X]∖Etμℓ(x)dx+ε≤2(R+1)ε, |
which proves that for a.e.
∫Rρℓ(x,t)μℓ(x)dx ⟶ℓ→+∞ ∫Rρ(x,t)μ(x)dx. | (22) |
We get that
yℓ(t)=yℓ0+∫t0ω(∫Rρℓ(x,s)μℓ(x)dx)ds. |
By definition, for all
∫T0∫R|ρℓ−κ|∂tφ+Φ˙yℓ(t)(ρℓ,κ)∂xφdxdt+∫R|ρℓ0(x+yℓ0)−κ|φ(x,0)dx≥0. |
The continuity of
∫T0∫R|ρ−κ|∂tφ+Φ˙y(t)(ρ,κ)∂xφdxdt+∫R|ρ0(x+y0)−κ|φ(x,0)dx≥0. |
Consequently
The last section concludes the theoretical analysis of Problem (1). The nonlocality in space of the constraint delivers an easy proof of stability with respect to the initial data in the
As evoked earlier, the nonlocality in space of the constraint makes the mathematical study of the model easier. But in the modeling point of view, this choice also makes sense for several reasons. First of all, one can think that the velocity
In this section, we aim at constructing a finite volume scheme and at proving its convergence toward the
a∨b=max{a,b}anda∧b=min{a,b}. |
Fix
For a fixed spatial mesh size
R×[0,T]⊂N⋃n=0⋃j∈ZPnj+1/2,Pnj+1/2=Kj+1/2×[tn,tn+1). |
We choose to discretize the initial data
Remark 8. Others choice could be made, for instance in the case
∀j∈Z, ρ0j+1/2∈[0,R]andρ0Δ=∑j∈Zρ0j+1/21Kj+1/2 ⟶Δx→0 ρ0(⋅+y0) in L1loc(R). |
Fix
sn+1=ω(∑j∈Zρnj+1/2μj+1/2Δx),qn+1=Q(sn+1). | (23) |
With these values, we update the approximate traffic density with the marching formula for all
ρn+1j+1/2=ρnj+1/2−ΔtΔx(Fn+1j+1(ρnj+1/2,ρnj+3/2)−Fn+1j(ρnj−1/2,ρnj+1/2)), | (24) |
where, following the recipe of [6,15],
Fn+1j(a,b)={Fn+1(a,b)if j≠0min{Fn+1(a,b),qn+1}if j=0, | (25) |
ρn+1j+1/2=Hn+1j(ρnj−1/2,ρnj+1/2,ρnj+3/2), | (26) |
where
∙ ρΔ(x,t)=N∑n=0∑j∈Zρnj+1/21Pnj+1/2(x,t)∙ sΔ(t),qΔ(t)=sn+1,qn+1if t∈[tn,tn+1)∙ yΔ(t)=y0+∫t0sΔ(u)du. |
Let
λsups∈[0,‖ω‖L∞] (‖∂Fs∂a‖L∞+‖∂Fs∂b‖L∞)⏟L≤1, | (27) |
where
Remark 9. When considering the Rusanov flux or the Godunov one, (27) is guaranteed when
2λ(‖f′‖L∞+‖ω‖L∞)≤1. |
Proposition 2 (
(i) monotone: for all
(ii) stable:
∀n∈{0,…,N}, ∀j∈Z,ρnj+1/2∈[0,R]. | (28) |
Proof. (i) In the classical case –
(ii) The
Hn+1j(0,0,0)=0,Hn+1j(R,R,R)=R. |
In order to show that the limit of
Proposition 3 (Discrete entropy inequalities). The numerical scheme (26) fulfills the following inequalities for all
(|ρn+1j+1/2−κ|−|ρnj+1/2−κ|)Δx+(Φnj+1−Φnj)Δt≤Rsn+1(κ,qn+1)Δt δj∈{−1,0}+(Φn0−Φnint)Δt(δj=−1−δj=0), | (29) |
where
Φnj=Fn+1(ρnj−1/2∨κ,ρnj+1/2∨κ)−Fn+1(ρnj−1/2∧κ,ρnj+1/2∧κ),Φnint=min{Fn+1(ρn−1/2∨κ,ρn1/2∨κ),qn+1}−min{Fn+1(ρn−1/2∧κ,ρn1/2∧κ),qn+1} |
and
Rsn+1(κ,qn+1)=F(sn+1,κ)−min{F(sn+1,κ),qn+1}. |
Proof. This result is a direct consequence of the scheme monotonicity. When the constraint does not enter the calculations i.e.
Hn+1−1(κ,κ,κ)=κ+λRsn+1(κ,qn+1). |
Consequently, we have both
ρn+1−1/2∨κ≤Hn+1−1(ρn−3/2∨κ,ρn−1/2∨κ,ρn1/2∨κ) |
and
ρn+1−1/2∧κ≥Hn+1−1(ρn−3/2∧κ,ρn−1/2∧κ,ρn1/2∧κ)−λRsn+1(κ,qn+1). |
By substracting these last two inequalities, we get
|ρn+1−1/2−κ|=ρn+1−1/2∨κ−ρn+1−1/2∧κ≤Hn+1−1(ρn−3/2∨κ,ρn−1/2∨κ,ρn1/2∨κ)−Hn+1−1(ρn−3/2∧κ,ρn−1/2∧κ,ρn1/2∧κ)+λRsn+1(κ,qn+1)=|ρn−1/2−κ|−λ(min{Fn+1(ρn−1/2∨κ,ρn1/2∨κ),qn+1}−Fn+1(ρn−1/2∨κ,ρn1/2∨κ))+λ(min{Fn+1(ρn−1/2∧κ,ρn1/2∧κ),qn+1}−Fn+1(ρn−1/2∧κ,ρn1/2∧κ))+λRsn+1(κ,qn+1)=|ρn−1/2−κ|−λ(Φn0−Φn−1)+λ(Φn0−Φnint)+λRsn+1(κ,qn+1), |
which is exactly (29) in the case
Starting from (24) and (29), we can obtain approximate versions of (4) and (5). Let us introduce the functions:
ΦΔ(ρΔ,κ)=N∑n=0∑j∈ZΦnj1Pnj+1/2;FΔ(sΔ,ρΔ)=N∑n=0∑j∈ZFn+1(ρnj−1/2,ρnj+1/2)1Pnj+1/2. |
Proposition 4 (Approximate entropy/constraint inequalities). (i) Fix
∫τ′τ∫R|ρΔ−κ|∂tφ+ΦΔ(ρΔ,κ)∂xφdxdt+∫R|ρΔ(x,τ)−κ|φ(x,τ)dx−∫R|ρΔ(x,τ′)−κ|φ(x,τ′)dx+2∫τ′τRsΔ(t)(κ,qΔ(t))φ(0,t)dt≥−Cφ1(Δt+Δx). | (30) |
(ii) Fix
−∫τ′τ∫R+ρΔ∂t(φψ)+FΔ(sΔ,ρΔ)∂x(φψ)dxdt−∫R+ρΔ(x,τ)φ(x)ψ(τ)dx+∫R+ρΔ(x,τ′)φ(x)ψ(τ′)dx≤∫τ′τqΔ(t)ψ(t)dt+Cφ,ψ2(Δx+Δt). | (31) |
Proof. Fix
(i) Define for all
A+B+C+D+E≥0, | (32) |
with
A=m−1∑n=k+1∑j∈Z|ρnj+1/2−κ|(φnj+1/2−φn−1j+1/2)ΔxB=m−1∑n=k∑j∈ZΦnj(φnj+1/2−φnj−1/2)ΔtC=∑j∈Z|ρkj+1/2−κ|φkj+1/2Δx−∑j∈Z|ρmj+1/2−κ|φm−1j+1/2ΔxD=m−1∑n=kRsn+1(κ,qn+1)(φn−1/2+φn1/2)ΔtE=m−1∑n=k(Φn0−Φnint)(φn−1/2−φn1/2)Δt. |
Inequality (30) follows from (32) with
Cφ1=R(T maxt∈[0,T]‖∂2ttφ(⋅,t)‖L1+4 maxt∈[0,T] ‖∂tφ(⋅,t)‖L1)+RL(T maxt∈[0,T] ‖∂2xxφ(⋅,t)‖L1+2 maxt∈[0,T] ‖∂xφ(⋅,t)‖L1+4‖φ‖L∞+2T‖∂xφ‖L∞), |
making use of the bounds:
|A−∫τ′τ∫R|ρΔ−κ|∂tφdxdt|≤R(T maxt∈[0,T] ‖∂2ttφ(⋅,t)‖L1+2 maxt∈[0,T] ‖∂tφ(⋅,t)‖L1)Δt,|B−∫τ′τ∫RΦΔ(ρΔ,κ)∂xφdxdt|≤RL(T maxt∈[0,T] ‖∂2xxφ(⋅,t)‖L1Δx,+2 maxt∈[0,T] ‖∂xφ(⋅,t)‖L1Δt)|C−∫R|ρΔ(x,τ)−κ|φ(x,τ)dx+∫R|ρΔ(x,τ′)−κ|φ(x,τ′)dx|≤2R maxt∈[0,T] ‖∂tφ(⋅,t)‖L1Δt,|D−2∫τ′τRsΔ(t)(κ,qΔ(t))φ(0,t)dt|≤RL(4‖φ‖L∞Δt+T‖∂xφ‖L∞Δx)and|E|≤2RTL‖∂xφ‖L∞Δx. |
(ii) In this case, the constant
Cφ,ψ2=R‖φ‖L1(T‖ψ″‖L∞+4‖ψ′‖L∞)+‖Q‖L∞‖ψ‖L∞(2+T‖φ′‖L∞)+RL‖ψ‖L∞(2‖φ′‖L1+T‖φ′‖L1+T‖φ″‖L1). |
Following the proof of (30), define for all
ψn=1Δt∫tn+1tnψ(t)dt,andφj+1/2=1Δx∫xj+1xjφ(x)dx, |
multiply the scheme (24) by
The final step is to obtain compactness for the sequences
Proposition 5. For all
yΔ(t)=y0+∫t0ω(∫RρΔ(x,u)μ(x)dx)du. | (33) |
Consequently, there exists
Proof. For all
yΔ(t)−y0=n−1∑k=0∫tk+1tksk+1du+∫ttnsn+1du=n−1∑k=0∫tk+1tkω(∑j∈Z∫Rρkj+1/2μj+1/2Δx)du+∫ttnω(∑j∈Z∫Rρnj+1/2μj+1/2Δx)du=∫t0ω(∫RρΔ(x,u)μ(x)dx)du. |
Let us also point out that from (23), we get that for all
sΔ(t)=ω(∫RρΔ(x,t)μ(x)dx). | (34) |
Combining (33) and (34), we obtain that for all
‖˙yΔ‖L∞=‖sΔ‖L∞≤‖ω‖L∞and‖yΔ‖L∞≤|y0|+T‖ω‖L∞. |
The sequence
The presence of a time dependent flux in the conservation law of (1) complicates the obtaining of compactness for
First, we choose to adapt techniques and results put forward by Towers in [33]. With this in mind, we suppose in this section that
∃α>0, ∀ρ∈[0,R],f″(ρ)≤−α. | (35) |
Though this assumption is stronger than the nondegeneracy one (15), since
the numerical flux chosen in (24) is eitherthe Engquist-Osher one or the Godunov one. | (36) |
To be precise, the choice made for the numerical flux at the interface – i.e. when
Dnj=max{ρnj−1/2−ρnj+1/2,0}. |
We will also use the notation
ˆZ=Z∖{−1,0,1}. |
In [33], the author dealt with a discontinuous in both time and space flux and the specific "vanishing viscosity" coupling at the interface. The discontinuity in space was localized along the curve
Lemma 4.1. Let
Dn+1j≤max{Dnj−1,Dnj,Dnj+1}−a(max{Dnj−1,Dnj,Dnj+1})2 | (37) |
and
Dn+1j≤1min{|j|−1,n+1}a. | (38) |
Proof. (Sketched) Inequality (38) is an immediate consequence of inequality (37), see [33,Lemma 4.3]. Obtaining inequality (37) however, is less immediate. Let us give some details of the proof.
First, note that by introducing the function
Dn+1j≤ψ(max{Dnj−1,Dnj,Dnj+1}). | (39) |
Then, one can show – only using the monotonicity of both the scheme and the function
(ρnj+3/2−ρnj+1/2), (ρnj−1/2−ρnj−3/2)≤0, | (40) |
it follows that inequality (39) holds for all cases. And finally in [33,Page 23], the author proves that if the flux considered is either the Engquist-Osher flux or the Godunov flux, then (40) holds.
The following lemma is an immediate consequence of inequality (38).
Lemma 4.2. Fix
J−1∑j=i|ρnj+1/2−ρnj−1/2|,−i−1∑j=−J+1|ρnj+1/2−ρnj−1/2|≤B | (41) |
and
J−2∑j=i|ρn+1j+1/2−ρnj+1/2|,−i−2∑j=−J+1|ρn+1j+1/2−ρnj+1/2|≤2λLB. | (42) |
Proposition 6. There exists
Proof. Fix
(n+1)Δt>t>λε≥λ(i Δx)=iΔt, |
i.e.
TV(ρΔ(t)|Ω(X,ε))≤2B | (43) |
and from (42), we deduce
∫Ω(X,ε)|ρΔ(x,t+Δt)−ρΔ(x,t)|dx≤4LBΔt. | (44) |
Combining (43)-(44) and the
Theorem 4.3. Fix
Proof. We have shown that – up to the extraction of a subsequence –
Recall that for all
yΔ(t)=y0+∫t0ω(∫RρΔ(x,u)μ(x)dx)du. |
When letting
∫T0∫R|ρΔ−κ|∂tφ+ΦΔ(ρΔ,κ)∂xφdxdt+∫R|ρ0Δ−κ|φ(x,0)dx≥−Cφ1(Δx+Δt). |
Then the a.e. convergence of
∫T0∫R|ρ−κ|∂tφ+Φ˙y(t)(ρ,κ)∂xφdxdt+∫R|ρ0(x+y0)−κ|φ(x,0)dx≥0, |
and consequently
∫τ′τ∫R|ρ−κ|∂tφ+Φ˙y(t)(ρ,κ)∂xφdxdt+∫R|ρ(x,τ)−κ|φ(x,τ)dx−∫R|ρ(x,τ′)−κ|φ(x,τ′)dx+2∫τ′τR˙y(t)(κ,q(t))φ(0,t)dt≥0. |
To conclude, note that the expression in the left-hand side of the previous inequality is a continuous function of
We proved than in the
The following result is the discrete version of Lemma 2.3 so it is consistent that the proof uses the discrete analogous arguments of the ones we used in the proof of Lemma 2.3.
Lemma 4.4. Introduce for all
ξΔ(t)=∫RρΔ(x,t)μ(x)dx. |
Then
Proof. Since
‖μℓ−μ‖L1 ⟶ℓ→+∞ 0andTV(μℓ) ⟶ℓ→+∞ TV(μ). |
Introduce for all
∀ℓ∈N,‖μℓ‖L1,TV(μℓ)≤K. |
For all
|ξΔ,ℓ(t)−ξΔ,ℓ(s)|=|ξΔ,ℓ(tk)−ξΔ,ℓ(tm)|=|∫RρΔ(x,tk)μℓ(x)dx−∫RρΔ(x,tm)μℓ(x)dx|=|∑j∈Z(ρkj+1/2−ρmj+1/2)μℓj+1/2Δx|,μℓj+1/2=1Δx∫xj+1xjμℓ(x)dx=|∑j∈Zk−1∑τ=m(ρτ+1j+1/2−ρτj+1/2)μℓj+1/2Δx|=|k−1∑τ=m∑j∈Z(Fτ+1j(ρτj−1/2,ρτj+1/2)−Fτ+1j+1(ρτj+1/2,ρτj+3/2))μℓj+1/2Δt|=|k−1∑τ=m∑j∈ZFτ+1j+1(ρτj+1/2,ρτj+3/2)(μℓj+3/2−μℓj+1/2)Δt|≤RLk−1∑τ=mTV(μℓ)Δt≤RLK(|t−s|+2Δt). |
Consequently, for all
|ξΔ(t)−ξΔ(τ)|≤2R‖μ−μℓ‖L1+RLK(|t−τ|+2Δt). |
Letting
|ξΔ(t)−ξΔ(τ)|≤RLK(|t−τ|+2Δt), |
which leads to
TV(ξΔ)=N∑k=0|ξΔ(tk+1)−ξΔ(tk)|≤3RLK(T+Δt). |
This proves that
Theorem 4.5. Fix
Proof. All the hypotheses of Lemma A.4 are fulfilled. Consequently, there exists a constant
TV(ρΔ(tn+1))≤TV(ρ0)+4R+Cε(n∑k=0|qk+1−qk|+n∑k=0|sk+1−sk|)≤TV(ρ0)+4R+Cε(1+‖Q′‖L∞)n∑k=0|sk+1−sk|. | (45) |
Making use of Lemma 4.4, we obtain that for all
n∑k=0|sk+1−sk|=n∑k=0|sΔ(tk+1)−sΔ(tk)|≤‖ω‖L∞n∑k=0|ξΔ(tk+1)−ξΔ(tk)|≤3RLK‖ω‖L∞(T+Δt). |
where the constant
TV(ρΔ(t))≤TV(ρ0)+4R+3Cε(1+‖Q′‖L∞)‖ω‖L∞RLK(T+Δt). | (46) |
Therefore, the sequence
∀t∈[0,T], ρΔ(t) ⟶Δ→0 ρ(t) in L1loc(R). |
Following the proof of Theorem 4.3, we show that
Remark 10. Note the complementarity of the hypotheses made in the above theorem with the ones of Theorem 4.3. Recall that in Theorem 4.3, we needed the Godunov flux only away from the interface.
In this section we present some numerical tests performed with the scheme analyzed in Section 4. In all the simulations we take the uniformly concave flux
μk(x)=2k1[0;12k](x), |
for one (in Section 5.1) or several (in Section 5.3) values of
In this section, consider a two-lane road on which a bus travels with a speed given by the function
ω(ρ)={α(β+ρ)2if0≤ρ≤0.61−ρif0.6≤ρ≤1, |
where
ρ0(x)=0.51[0.5;1](x),y0=1.5,Q(s)=0.75×(1−s2)2. |
The idea behind the choice of
Remark 11. The function
We also perform a convergence analysis for this test. In Table 1, we computed the relative errors
EΔρ=‖ρΔ−ρΔ/2‖L1((0,T);L1(R))andEΔy=‖yΔ−yΔ/2‖L∞, |
Number of cells | ||
160 | ||
320 | ||
640 | ||
1280 | ||
2560 | ||
5120 | ||
10240 |
for different number of space cells at the final time
Now we confront the numerical tests performed with our model with the tests done by the authors in [14] approximating the original problem of [20]. We deal with a road of length
ω(ρ)=min{0.3; 1−ρ}andQ(s)=0.6×(1−s2)2. |
First, consider the initial datum
ρ0(x)={0.4ifx<0.50.5ifx>0.5 y0=0.5. | (47) |
The numerical solution is composed of two classical shocks separated by a nonclassical discontinuity, as illustrated in Figure 4 (left).
Next, we choose
ρ0(x)={0.8ifx<0.50.5ifx>0.5 y0=0.5. | (48) |
The values of the initial condition create a rarefaction wave followed by a nonclassical and classical shocks, as illustrated in Figure 4 (right).
Finally, still following [14], we consider
ρ0(x)={0.8ifx<0.50.4ifx>0.5 y0=0.4. | (49) |
Here the solution is composed of a rarefaction wave followed by nonclassical and classical shocks on the density that are created when the slow vehicle approaches the rarefaction and initiates a moving bottleneck, as illustrated in Figure 5.
With these three tests, we can already see – in a qualitative way – the resemblance between the numerical approximations to the solutions to our model and the numerical approximations of [14]. One way to quantify their proximity is for example to evaluate the
replacingsn+1=ω(∑j∈Zρnj+1/2μj+1/2Δx)bysn+1=ω(ρn1/2). |
Let us precise that this is not the scheme the authors of [14] proposed. However, this scheme is consistent with the problem
{∂tρ+∂x(F(˙y(t),ρ))=0R×(0,T)ρ(x,0)=ρ0(x+y0)x∈RF(˙y(t),ρ)|x=0≤Q(˙y(t))t∈(0,T)˙y(t)=ω(ρ(0+,t))t∈(0,T)y(0)=y0 | (50) |
and behaves in a stable way in the calculations we performed. Therefore, the couple
EΔL1=‖ρΔ−¯ρΔ‖L1((0,T);L1(R))andEΔL∞=‖yΔ−¯yΔ‖L∞. |
Number of cells | ||
160 | ||
320 | ||
640 | ||
1280 | ||
2560 | ||
5120 | ||
10240 | ||
20480 | ||
40960 |
These calculations indicate that for a sufficiently large number of cells
EΔL1≃2.7×10−4andEΔL∞≃7.6×10−3. |
This indicates the discrepancy between our nonlocal and the local model (50) of [20]. The idea is now to fix the number of cells
weight function | ||
Remark 12. The previous simulations show a closeness between our model as
ρ0(x)={1ifx<xb0ifx>xb. | (51) |
Indeed, for such data, there exists a small time interval
Even if we are unable, at this time, to rigorously link our problem (1) with
The author is most grateful to Boris Andreianov for his constant support and many enlightening discussions.
We focus on the study of the following class of models:
{∂tρ+∂x(F(s(t),ρ))=0R×(0,T)ρ(x,0)=ρ0(x)x∈RF(s(t),ρ)|x=0≤q(t)t∈(0,T), | (52) |
where
∀s∈[0,Σ], F(s,0)=0, F(s,R)≤0and∃! ¯ρs∈(0,R), ∂ρF(s,ρ)(¯ρs−ρ)>0 for a.e. ρ∈(0,R). | (53) |
This framework covers the particular case when
F(s(t),ρ)=f(ρ)−s(t)ρ, |
with bell-shaped
ρ0∈L1(R;[0,R])∩BV(R). |
Throughout the appendix, for all
Φs(a,b)=sign(a−b)(F(s,a)−F(s,b)) |
the classical Kružkov entropy flux associated with the Kružkov entropy
Let us first recall the following definition.
Definition A.1. A bounded function
(ⅰ) the following regularity is fulfilled:
(ⅱ) for all test functions
∫τ′τ∫R|ρ−κ|∂tφ+Φs(t)(ρ,κ)∂xφdxdt+∫R|ρ(x,τ)−κ|φ(x,τ)dx−∫R|ρ(x,τ′)−κ|φ(x,τ′)dx+2∫τ′τRs(t)(κ,q(t))φ(0,t)dt≥0, |
where
Rs(t)(κ,q(t))=F(s(t),κ)−min{F(s(t),κ),q(t)}; |
(ⅲ) for all test functions
−∫τ′τ∫R+ρ∂t(φψ)+F(s(t),ρ)∂x(φψ) dxdt−∫R+ρ(x,τ)φ(x)ψ(τ)dx+∫R+ρ(x,τ′)φ(x)ψ(τ′)dx≤∫τ′τq(t)ψ(t)dt. |
Definition A.2. An admissible weak solution
As we pointed out before, this notion of solution is well suited for passage to the limit of a.e. convergent sequences of exact or approximate solutions. However, it is not so well-adapted to prove uniqueness. An equivalent notion of solution, based on explicit treatment of traces of
Theorem A.3. Fix
‖ρ1(t)−ρ2(t)‖L1≤‖ρ10−ρ20‖L1+2∫t0|q1(τ)−q2(τ)|dτ+2∫t0‖F(s1(τ),⋅)−F(s2(τ),⋅)‖L∞dτ+∫t0||∂ρF(s1(τ),⋅)−∂ρF(s2(τ),⋅)||L∞TV(ρ1(τ))dτ. | (54) |
In particular, Problem (52) admits at most one
Proof. Since our interest to details lies rather on the numerical approximation point of view, we do not fully prove this statement but we give the essential steps leading to this stability result.
● Definition of solution. First, the authors of [7] introduce a subset of
● Equivalence of the two definitions. The next step is to prove that this latter definition of solution is equivalent to Definition A.1. This part is done using good choices of test functions, see [7,Theorem 3.18] or [6,Proposition 2.5,Theorem 2.9].
● First stability estimate. One first shows that if
‖ρ1(t)−ρ2(t)‖L1≤‖ρ10−ρ20‖L1+2∫t0|q1(τ)−q2(τ)|dτ. | (55) |
The proof starts with the classical doubling of variables method of Kružkov [25,Theorem 1] and then uses the germ structure, what the authors of [7] called
● Proof of estimate (54). The proof is based upon estimate (55) and elements borrowed from [8,18]. Most details can be found in the proof of [21,Theorem 2.1].
Remark 13. Though the definition of solutions with the germ explicitly involves the traces of
We now turn to the proof of the existence of
Fix
R×[0,T]⊂N⋃n=0⋃j∈ZPnj+1/2,Pnj+1/2=Kj+1/2×[tn,tn+1). |
We choose to discretize the initial data
ρn+1j+1/2=ρnj+1/2−ΔtΔx(Fnj+1(ρnj+1/2,ρnj+3/2)−Fnj(ρnj−1/2,ρnj+1/2)), | (56) |
where
Fnj(a,b)={Fn(a,b)if j≠0min{Fn(a,b),qn)}if j=0, | (57) |
ρΔ(x,t)=ρnj+1/2 if (x,t)∈Pnj+1/2andsΔ(t),qΔ(t)=sn,qn if t∈[tn,tn+1). |
Let
λsups∈[0,Σ](‖∂Fs∂a‖L∞+‖∂Fs∂b‖L∞)⏟L≤1, | (58) |
where
Lemma A.4. We suppose that
∃ε>0, ∀t∈[0,T], ∀s∈[0,Σ], q(t)≤maxρ∈[0,R]F(s,ρ)−ε:=qε(s). | (59) |
Then there exists a constant
\begin{equation} \mathbf{TV}(\rho_{\Delta}(t^{n+1})) \leq \mathbf{TV}(\rho_{0}) + 4R + \mathbf{C}^{{ \varepsilon}} \left( \sum\limits_{k = 0}^{n} |q^{k+1} - q^{k}| + \sum\limits_{k = 0}^{n} |s^{k+1} - s^{k}| \right), \end{equation} | (60) |
where
Proof. Fix
\begin{split} \sum\limits_{j \in \mathbb{Z}} |\rho_{j+1/2}^{n+1} - \rho_{j-1/2}^{n+1}| \leq & \mathbf{TV}(\rho_{0}) + 4R \\ & + 2 \sum\limits_{k = 0}^{n} \left| \left( \widehat{\rho}_{s^{k+1}}(q^{k+1}) - \widehat{\rho}_{s^{k}}(q^{k}) \right) - \left(\breve{\rho}_{s^{k+1}}(q^{k+1}) - \breve{\rho}_{s^k}(q^{k}) \right) \right|, \end{split} |
where for all
F(s^{k},\widehat{\rho}_{s^k}(q^{k})) = F(s^{k},\breve{\rho}_{s^k}(q^{k})) = q^{k} \quad \text{and} \quad \widehat{\rho}_{s^{k}}(q^{k}) > \breve{\rho}_{s^k}(q^{k}). |
Denote by
\Omega( \varepsilon) = \bigcup\limits_{s \in [0,\Sigma]} \Omega_{s}( \varepsilon) |
where for all
\forall q \in [0,q_{ \varepsilon}(s)], \ \left|(I_{s}^{-1})^{'}(q) \right| \leq \frac{1}{\text{C}_{0}}. |
Then, for all
\begin{split} \left| \breve{\rho}_{s^{k+1}}(q^{k+1}) - \breve{\rho}_{s^{k}}(q^{k}) \right| = & \left| (I_{s^{k+1}}^{-1})(q^{k+1}) - \breve{\rho}_{s^{k}}(q^{k}) \right| \\ \leq & \frac{1}{\text{C}_{0}} |q^{k+1} - q^{k}| + \left| (I_{s^{k+1}}^{-1})(q^{k}) - \breve{\rho}_{s^{k}}(q^{k}) \right| \\ = & \frac{1}{\text{C}_{0}} |q^{k+1} - q^{k}| + \left| (I_{s^{k+1}}^{-1})(q^{k}) - (I_{s^{k+1}}^{-1}) \ \circ \ I_{s^{k+1}} \left( \breve{\rho}_{s^{k}}(q^{k}) \right) \right| \\ \leq & \frac{1}{\text{C}_{0}} \left( |q^{k+1} - q^{k}| + \left| q^{k} - I_{s^{k+1}} \left( \breve{\rho}_{s^{k}}(q^{k}) \right) \right| \right) \\ = & \frac{1}{\text{C}_{0}} \left( |q^{k+1} - q^{k}| + \left| F \left(s^{k}, \breve{\rho}_{s^{k}}(q^{k}) \right) - F\left(s^{k+1}, \breve{\rho}_{s^{k}}(q^{k}) \right) \right| \right)\\ \leq & \frac{1}{\text{C}_{0}} \left( |q^{k+1} - q^{k}| + \| \partial_s F \|_{\mathbf{L}^{{\infty}}} |s^{k+1}-s^{k}| \right) \\ \leq & \frac{1 + \| \partial_s F \|_{\mathbf{L}^{{\infty}}}}{\text{C}_{0}} \left( |q^{k+1} - q^{k}| + |s^{k+1}-s^{k}| \right). \end{split} |
Using the same techniques, one can show that the same inequality holds when considering
\mathbf{C}^{{ \varepsilon}} = 4 \times \left( \frac{1 + \| \partial_s F \|_{\mathbf{L}^{{\infty}}}}{\text{C}_{0}} \right). |
Remark 14. Recall we suppose that
\forall s \in [0,\Sigma], \ F(s,\cdot) \in \mathbf{C}^{{1}}([0,R] \backslash \{ \overline{\rho}_s\}), \quad \overline{\rho}_s = \underset{\rho \in [0,R]}{\text{argmax}} \ F(s,\rho). |
Corollary 1. Fix
\forall t \in [0,T], \ \rho_{\Delta}(t) {\ \underset{{\Delta} \to {0}}{\longrightarrow} \ } \rho(t) \ {\rm{in}} \ \mathbf{L}_{\mathbf{loc}}^{{1}}( \mathbb{R}). |
Proof. Since
Theorem A.5. Fix
\begin{equation} \forall t \in [0,T], \ \mathbf{TV}(\rho(t)) \leq \mathbf{TV}(\rho_{0}) + 4R + \mathbf{C}^{{ \varepsilon}} \left( \mathbf{TV}(q) + \mathbf{TV}(s) \right). \end{equation} | (61) |
Proof. From the scheme (56), one can derive approximate entropy/constraint inequalities analogous to (30)-(31) of Section 4. Let
Corollary 2. Fix
Proof. Uniqueness comes from Theorem 54, the existence and the
Remark 15. Under the hypotheses of Corollary 2, if we prove the existence of an other admissible weak solution
[1] | A. Abdullah, X. Li, An integrated-model QoS-based graph for web service recommendation, IEEE International Conference on Web Services, 2015. |
[2] | W. Shi, X. Liu, Q. Yu, Correlation-Aware Multi-Label Active Learning for Web Service Tag Recommendation, IEEE International Conference on Web Services, 2017. |
[3] |
X. Luo, M. Zhou, Z. Wang, Y. Xia, Q. Zhu, An Effective Scheme for QoS Estimation via Alternating Direction Method-Based Matrix Factorization, IEEE Trans. Serv. Comput., 12 (2019), 503–518. doi: 10.1109/TSC.2016.2597829
![]() |
[4] |
Y. Zhang, Y. Fan, W. Tan, J. Zhang, Web Service Recommendation with Reconstructed Profile from Mashup Descriptions, IEEE Trans. Autom. Sci. Eng., 15 (2018), 468–478. doi: 10.1109/TASE.2016.2624310
![]() |
[5] |
K. Su, B. Xiao, B. Liu, Z. Zhang, TAP: A personalized trust-aware QoS prediction approach for web service recommendation, Knowl. Based Syst., 115 (2017), 55–65. doi: 10.1016/j.knosys.2016.09.033
![]() |
[6] |
J. Liu, Y. Chen, A personalized clustering-based and reliable trust-aware QoS prediction approach for cloud service recommendation in cloud manufacturing, Knowl. Based Syst., 174 (2019), 43–56. doi: 10.1016/j.knosys.2019.02.032
![]() |
[7] |
C. Yu, L. Huang, A Web service QoS prediction approach based on time and location aware collaborative filtering, Serv. Oriented Comput. Appl., 10 (2016), 135–149. doi: 10.1007/s11761-014-0168-4
![]() |
[8] |
R. Xiong, J. Wang, N. Zhang, Y. Ma, Deep hybrid collaborative filtering for web service recommendation, Expert Syst. Appl., 110 (2018), 191–205. doi: 10.1016/j.eswa.2018.05.039
![]() |
[9] |
L. Ren, W. Wang, An SVM-based collaborative filtering approach for Top-N web services recommendation, Future Gener. Comput. Syst., 78 (2018), 531–543. doi: 10.1016/j.future.2017.07.027
![]() |
[10] |
Z. Chen, L. Shen, F. Li, Exploiting Web service geographical neighborhood for collaborative QoS prediction, Future Gener. Comput. Syst., 68 (2017), 248–259. doi: 10.1016/j.future.2016.09.022
![]() |
[11] |
Z. Zheng, H. Ma, M. Hao, M. R. Lyu, I. King, QoS-aware web service recommendation by collaborative filtering, IEEE Trans. Serv. Comput., 4 (2011), 140–152. doi: 10.1109/TSC.2010.52
![]() |
[12] |
X. Chen, Z. Zheng, X. Liu, Z. Huang, Personalized QoS-aware web service recommendation and visualization, IEEE Trans. Serv. Comput., 6 (2013), 35–47. doi: 10.1109/TSC.2011.35
![]() |
[13] | L. Fletcher, X. Liu, A Collaborative Filtering Method for Personalized Preference-based Service Recommendation, IEEE International Conference on Web Services, 2015. |
[14] |
Y. Ma, S. Wang, P. Hung, C. Hsu, Q. Sun, F. Yang, A Highly Accurate Prediction Algorithm for Unknown Web Service QoS Values, IEEE Trans. Serv. Comput., 9 (2016), 511–523. doi: 10.1109/TSC.2015.2407877
![]() |
[15] |
N. Idrissi, A. Zellou, A systematic literature review of sparsity issues in recommender systems, Soc. Network Anal. Min., 10 (2020), 1–23. doi: 10.1007/s13278-019-0612-8
![]() |
[16] | F. Gohari, F. Aliee, H. Haghighi, A Dynamic Local-Global Trust-aware Recommendation approach, Electron. Commer. Res. Appl., 34 (2019), 1–23. |
[17] |
Y. Kim, An enhanced trust propagation approach with expertise and homophily-based trust networks, Knowl. Based Syst., 82 (2015), 20–28. doi: 10.1016/j.knosys.2015.02.023
![]() |
[18] |
S. Lyu, J. Liu, M. Tang, Y. Xu, J. Chen, Efficiently Predicting Trustworthiness of Mobile Services Based on Trust Propagation in Social Networks, Mobile Networks Appl., 20 (2015), 840–852. doi: 10.1007/s11036-015-0619-y
![]() |
[19] |
J. Yin, Y. Xu, Personalized QoS-based Web Service Recommendation with Service Neighborhood-Enhanced Matrix Factorization, Int. J. Web Grid Serv., 11 (2015), 39–56. doi: 10.1504/IJWGS.2015.067156
![]() |
[20] | K. Qi, H. Hu, W. Song, J. Ge, J. Lü, Personalized QoS Prediction via Matrix Factorization Integrated with Neighborhood Information, IEEE International Conference on Services Computing, 2015. |
[21] | Y. Yin, L. Chen, Y. Xu, J. Wan, H. Zhang, Z. Mai, QoS Prediction for Service Recommendation with Deep Feature Learning in Edge Computing Environment, Mobile Networks Appl., 2019 (2019), 1–11. |
[22] | D. Ryu, K. Lee, J. Baik, Location-based Web Service QoS Prediction via Preference Propagation to address Cold Start Problem, IEEE Trans. Serv. Comput., 2018 (2018). |
[23] | X. Zhu, X. Jing, D. Wu, Z. He, J. Cao, D. Yue, et al., Similarity-maintaining Privacy Preservation and Location-aware Low-rank Matrix Factorization for QoS Prediction based Web Service Recommendation, IEEE Trans. Serv. Comput., 2018 (2018). |
[24] | K. Su, L. Ma, B. Xiao, H. Zhang, Web service QoS prediction by neighbor information combined non-negative matrix factorization, J. Int. Fuzzy Syst., 30 (2016), 3593–3604. |
[25] |
Z. Zheng, H. Ma, M. Hao, M. R. Lyu, I. King, Collaborative web service QoS prediction via neighborhood integrated matrix factorization, IEEE Trans. Serv. Comput., 6 (2013), 289–299. doi: 10.1109/TSC.2011.59
![]() |
[26] | J. Golbeck, J. Hendler, Inferring binary trust relationships in web-based social networks. ACM Trans. Int. Technol., 6 (2006), 497–529. |
[27] |
Y. Kim, H. Song, Strategies for predicting local trust based on trust propagation in social networks, Knowl. Based Syst., 24 (2011), 1360–1371. doi: 10.1016/j.knosys.2011.06.009
![]() |
[28] | D. Wei, An Optimized Floyd Algorithm for the Shortest Path Problem, J. Networks, 12 (2010), 1469–1504. |
[29] | D. Rafailidis, F. Crestani, A Regularization Method with Inference of Trust and Distrust in Recommender Systems, Joint European Conference on Machine Learning and Knowledge Discovery in Databases, 2017. |
[30] | H. Rong, P. Pearl, Enhancing collaborative filtering systems with personality information, Proceedings of The 5th ACM Conference on Recommender Systems, 2011. |
[31] | L. Shao, J. Zhang, Y. Wei, J. Zhao, B. Xie, H. Mei, Personalized QoS prediction for Web services via collaborative filtering, IEEE International Conference on Web Services, 2007. |
[32] | B. Sarwar, G. Karypis, J. Konstan, J. Riedl, Item-based collaborative filtering recommendation algorithms, Proceedings of the 10th international conference on World Wide Web, 2001. |
1. | Paola Goatin, Chiara Daini, Maria Laura Delle Monache, Antonella Ferrara, Interacting moving bottlenecks in traffic flow, 2023, 18, 1556-1801, 930, 10.3934/nhm.2023040 | |
2. | Abraham Sylla, A LWR model with constraints at moving interfaces, 2022, 56, 2822-7840, 1081, 10.1051/m2an/2022030 | |
3. | Boris Andreianov, Abraham Sylla, Existence analysis and numerical approximation for a second-order model of traffic with orderliness marker, 2022, 32, 0218-2025, 1295, 10.1142/S0218202522500294 | |
4. | Boris Andreianov, Carlotta Donadello, Massimiliano D. Rosini, Entropy solutions for a two-phase transition model for vehicular traffic with metastable phase and time depending point constraint on the density flow, 2021, 28, 1021-9722, 10.1007/s00030-021-00689-5 | |
5. | Boris Andreianov, Abraham Sylla, Finite volume approximation and well-posedness of conservation laws with moving interfaces under abstract coupling conditions, 2023, 30, 1021-9722, 10.1007/s00030-023-00857-9 | |
6. | Boris Andreianov, Massimiliano D. Rosini, Graziano Stivaletta, On existence, stability and many-particle approximation of solutions of 1D Hughes' model with linear costs, 2023, 369, 00220396, 253, 10.1016/j.jde.2023.06.004 | |
7. | Boris Andreianov, Theo Girard, Existence of Solutions for a Class of One-Dimensional Models of Pedestrian Evacuations, 2024, 56, 0036-1410, 3386, 10.1137/23M1550256 | |
8. | Malaya Mohanty, Madhu Lisha Pattanaik, Satya Ranjan Samal, Shivam Shivam, Assessing the Impact of Three-Wheelers on Traffic Flow in India: A Case Study Using Ann, 2024, 13354205, 10.26552/com.C.2024.033 |
Number of cells | ||
160 | ||
320 | ||
640 | ||
1280 | ||
2560 | ||
5120 | ||
10240 |
Number of cells | ||
160 | ||
320 | ||
640 | ||
1280 | ||
2560 | ||
5120 | ||
10240 | ||
20480 | ||
40960 |
weight function | ||
Number of cells | ||
160 | ||
320 | ||
640 | ||
1280 | ||
2560 | ||
5120 | ||
10240 |
Number of cells | ||
160 | ||
320 | ||
640 | ||
1280 | ||
2560 | ||
5120 | ||
10240 | ||
20480 | ||
40960 |
weight function | ||