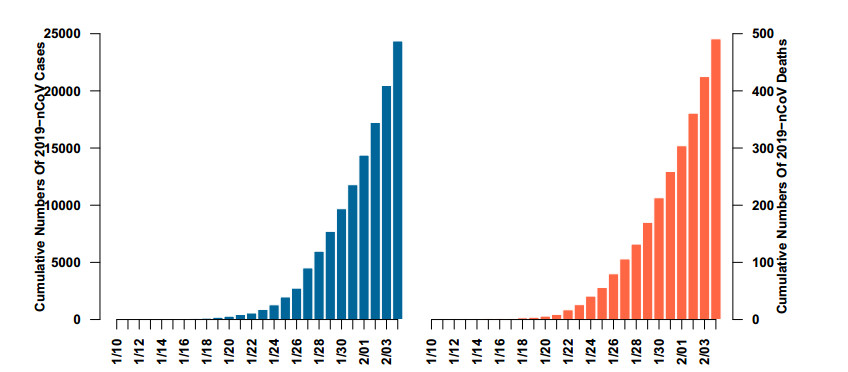
The novel coronavirus disease 2019 (COVID-19) infection broke out in December 2019 in Wuhan, and rapidly overspread 31 provinces in mainland China on 31 January 2020. In the face of the increasing number of daily confirmed infected cases, it has become a common concern and worthy of pondering when the infection will appear the turning points, what is the final size and when the infection would be ultimately controlled. Based on the current control measures, we proposed a dynamical transmission model with contact trace and quarantine and predicted the peak time and final size for daily confirmed infected cases by employing Markov Chain Monte Carlo algorithm. We estimate the basic reproductive number of COVID-19 is 5.78 (95%CI: 5.71–5.89). Under the current intervention before 31 January, the number of daily confirmed infected cases is expected to peak on around 11 February 2020 with the size of 4066 (95%CI: 3898–4472). The infection of COVID-19 might be controlled approximately after 18 May 2020. Reducing contact and increasing trace about the risk population are likely to be the present effective measures.
Citation: Kai Wang, Zhenzhen Lu, Xiaomeng Wang, Hui Li, Huling Li, Dandan Lin, Yongli Cai, Xing Feng, Yateng Song, Zhiwei Feng, Weidong Ji, Xiaoyan Wang, Yi Yin, Lei Wang, Zhihang Peng. Current trends and future prediction of novel coronavirus disease (COVID-19) epidemic in China: a dynamical modeling analysis[J]. Mathematical Biosciences and Engineering, 2020, 17(4): 3052-3061. doi: 10.3934/mbe.2020173
[1] | Damilola Olabode, Jordan Culp, Allison Fisher, Angela Tower, Dylan Hull-Nye, Xueying Wang . Deterministic and stochastic models for the epidemic dynamics of COVID-19 in Wuhan, China. Mathematical Biosciences and Engineering, 2021, 18(1): 950-967. doi: 10.3934/mbe.2021050 |
[2] | Weike Zhou, Aili Wang, Fan Xia, Yanni Xiao, Sanyi Tang . Effects of media reporting on mitigating spread of COVID-19 in the early phase of the outbreak. Mathematical Biosciences and Engineering, 2020, 17(3): 2693-2707. doi: 10.3934/mbe.2020147 |
[3] | Sha He, Sanyi Tang, Libin Rong . A discrete stochastic model of the COVID-19 outbreak: Forecast and control. Mathematical Biosciences and Engineering, 2020, 17(4): 2792-2804. doi: 10.3934/mbe.2020153 |
[4] | Junyuan Yang, Guoqiang Wang, Shuo Zhang . Impact of household quarantine on SARS-Cov-2 infection in mainland China: A mean-field modelling approach. Mathematical Biosciences and Engineering, 2020, 17(5): 4500-4512. doi: 10.3934/mbe.2020248 |
[5] | Tao Chen, Zhiming Li, Ge Zhang . Analysis of a COVID-19 model with media coverage and limited resources. Mathematical Biosciences and Engineering, 2024, 21(4): 5283-5307. doi: 10.3934/mbe.2024233 |
[6] | Liping Wang, Jing Wang, Hongyong Zhao, Yangyang Shi, Kai Wang, Peng Wu, Lei Shi . Modelling and assessing the effects of medical resources on transmission of novel coronavirus (COVID-19) in Wuhan, China. Mathematical Biosciences and Engineering, 2020, 17(4): 2936-2949. doi: 10.3934/mbe.2020165 |
[7] | Chayu Yang, Jin Wang . A mathematical model for the novel coronavirus epidemic in Wuhan, China. Mathematical Biosciences and Engineering, 2020, 17(3): 2708-2724. doi: 10.3934/mbe.2020148 |
[8] | Hamdy M. Youssef, Najat A. Alghamdi, Magdy A. Ezzat, Alaa A. El-Bary, Ahmed M. Shawky . A new dynamical modeling SEIR with global analysis applied to the real data of spreading COVID-19 in Saudi Arabia. Mathematical Biosciences and Engineering, 2020, 17(6): 7018-7044. doi: 10.3934/mbe.2020362 |
[9] | Fulian Yin, Jiahui Lv, Xiaojian Zhang, Xinyu Xia, Jianhong Wu . COVID-19 information propagation dynamics in the Chinese Sina-microblog. Mathematical Biosciences and Engineering, 2020, 17(3): 2676-2692. doi: 10.3934/mbe.2020146 |
[10] | Ling Xue, Caterina Scoglio . Network-level reproduction number and extinction threshold for vector-borne diseases. Mathematical Biosciences and Engineering, 2015, 12(3): 565-584. doi: 10.3934/mbe.2015.12.565 |
The novel coronavirus disease 2019 (COVID-19) infection broke out in December 2019 in Wuhan, and rapidly overspread 31 provinces in mainland China on 31 January 2020. In the face of the increasing number of daily confirmed infected cases, it has become a common concern and worthy of pondering when the infection will appear the turning points, what is the final size and when the infection would be ultimately controlled. Based on the current control measures, we proposed a dynamical transmission model with contact trace and quarantine and predicted the peak time and final size for daily confirmed infected cases by employing Markov Chain Monte Carlo algorithm. We estimate the basic reproductive number of COVID-19 is 5.78 (95%CI: 5.71–5.89). Under the current intervention before 31 January, the number of daily confirmed infected cases is expected to peak on around 11 February 2020 with the size of 4066 (95%CI: 3898–4472). The infection of COVID-19 might be controlled approximately after 18 May 2020. Reducing contact and increasing trace about the risk population are likely to be the present effective measures.
On 31 December 2019, the novel coronavirus disease 2019 (COVID-19) infection broke out in Wuhan, China. As shown in Figure 1, the reported number of COVID-19 cases gradually increases every day, and the cumulative number of confirmed cases and death cases has rapidly multiplied since 18 January 2020. From the point of view for the spread of COVID-19, the epidemic situation is severe and spreads to all over the country (the mainland China) with the arrival of Chunyun (see the Figure 2). Up to 20 January 2020, the confirmed infected cases have been found in 31 provinces of mainland China. By the end of 4 February 2020, there have been 24, 363 confirmed cases of COVID-19 infections in mainland China, including 491 deaths and 892 hospital released cases, and 23, 260 suspected cases under follow-up [1]. So far, in addition to China, the United States, Thailand, Japan, South Korea and other countries have confirmed COVID-19 cases.
Since the 21st century, the global epidemics of severe acute respiratory syndrome coronavirus (SARS-CoV) and Middle East respiratory syndrome coronavirus (MERS-CoV) have been caused by human coronavirus, with alarming morbidity and mortality [2]. The novel coronavirus, occurring in Wuhan, is a novel coronavirus strain that has never been found in humans [3], which is defined as the seventh member of the family of coronaviruses that infect humans [4]. Within a few weeks, the infection of COVID-19 has caused a global awareness of the public health emergency [5].
Recently, there have been some relative studies about the estimation for the infection of COVID-19, the risk of transmission et al. For example, Li et al. [6] reached a conclusion that human-to-human transmission has occurred among close contacts since the middle of December 2019, and they estimated the epidemic doubling time and the basic reproductive number. Their findings [7] were consistent with person-to-person transmission of COVID-19 in hospital and family settings, and the reports of infected travelers in other geographical regions. Nishiura et al. [8] used a spatial back-calculation method and analyzed thirteen exported cases to estimate the cumulative incidence of COVID-19 cases in China in real time. Wu et al. [9] adopted a metapopulation SEIR model to simulate the epidemic across the many affected cities in mainland China. Zhao et al. [10] considered the under-reporting was likely to have resulted in 469 unreported cases during the first half of January 2020 and estimated the basic reproduction number of COVID-19 at 2.56.
In this paper, by taking into account the contact trace and then quarantine measure, we propose a compartmental model to simulate the data on the number of cumulative confirmed cases from 10 January 2020 to 4 February 2020 (in mainland China) and predict the trend of the infection.
We stratify the total population as the susceptible (not traced) denoted by S, the latent (not traced) denoted by E, the infectious (not traced) denoted by I, the uninfected but traced (these individuals stay at home and take self isolation) denoted by ST, the infected and traced denoted by ET, the infectious and quarantined (traced) denoted by Iq, the recovered (through self-isolation or treatment in hospital settings) denoted by R and the dead D.
Because in the whole country, most population is impossible to contact the COVID-19 except (for) the population from Hubei province, i.e., a certain fraction of the population is protected from the COVID-19. In this paper, we assumed that only a small proportion of the whole population is susceptible. The date, 10 January 2020, is the starting date for the dynamical model (i.e., t = 0). We do not consider the birth and death for the total population because the time of the study is short (that is, the total population N(t)=S(t)+E(t)+I(t)+ST(t)+ET(t)+Iq(t)+R(t)+D(t)≡S0). Let β is the contact rate, ϕ is the probability of infection and the trace rate is ρ. Because the latent individuals have the strongly infectious ability [6], however, no stronger than the ability of infectious individuals. So, we use ε to denote the proportion of infectious ability in latent individuals relative to that in infectious individuals. Then the variation of the susceptible S consists of three parts: the uninfected but traced ρ(1−ϕ)βS(I+εE), the infected and traced ρϕβS(I+εE) and the infected but not traced (1−ρ)ϕβS(I+εE). The infected but not traced move to the latent compartment E, while the infected and traced move to Eq, and the uninfected but traced move to ST. The latent individuals no matter whether they were quarantined are becoming the infectious with the rate α. The infectious but not quarantined individuals are sent to some hospital settings (i.e., they move to Iq) with the rate θγ. The infectious but not quarantined individuals are dead with the rate (1−θ)ηγ. The infectious and quarantined individuals are dead with the rate ηqγq. The infectious but not quarantined individuals recover with the rate (1−θ)(1−η)γ. The infectious and quarantined individuals recover with the rate (1−θq)γ. Given the model structure (Figure 3), we obtain that the following dynamical model described by eight differential equations.
{dSdt=−ρ(1−ϕ)βS(I+εE)⏟uninfectedbuttraced−ρϕβS(I+εE)⏟infectedandtraced−(1−ρ)ϕβS(I+εE)⏟infectedbutnottraced+ωST⏟releaseofthosetracedbutuninfected,dEdt=(1−ρ)ϕβS(I+εE)⏟infectedbutnottraced−αE⏟becominginfectiousarenotquarantined,dIdt=αE⏟becominginfectiousarenotquarantined−(1−θ)(1−η)γI⏟notquarantinedandrecover−(1−θ)ηγI⏟notquarantinedanddead−θγI⏟becominginfectiousarequarantined,dRdt=(1−θ)(1−η)γI⏟notquarantinedandrecover+(1−ηq)γIq⏟quarantinedandrecover,dSTdt=ρ(1−ϕ)βS(I+εE)⏟uninfectedbuttraced−ωST⏟releaseofthosetracedbutuninfected,dETdt=ρϕβS(I+εE)⏟infectedandtraced−αET⏟tracedcontactsbecominginfectiousandquarantined,dIqdt=αET⏟tracedcontactsbecominginfectiousandquarantined+θγI⏟becominginfectiousarequarantined−(1−ηq)γqIq⏟quarantinedandrecover−ηqγqIq⏟quarantinedanddead,dDdt=(1−θ)ηγI⏟notquarantinedanddead+ηqγqIq⏟quarantinedanddead, | (2.1) |
the interpretations of all parameters are listed in Table 1.
Parameters | Value (95% CI) | Dimension | Interpretation | Source |
β | 2.6×10−6(2.4×10−6,2.9×10−6) | dimensionless | Contact rate | MCMC |
ϕ | 0.0923 (0.0917, 0.0963) | dimensionless | Probability of transmission per contact | MCMC |
ρ | 0.0171 (0.0151, 0.0198) | dimensionless | Traced rate | MCMC |
ε | 0.914 (0.860, 0.939) | dimensionless | Infectious ability of the latent | MCMC |
1α | 5.2 | day | Mean incubation period | [6] |
θ | 0.0952 (0.0890, 0.0977) | day−1 | Quarantined rate of the infectious | MCMC |
ηq | 0.00161 (0.0145, 0.0177) | day−1 | Disease induced death rate for the infectious and quarantined | MCMC |
η | 0.00392 (0.00352, 0.00427) | day−1 | Disease induced death rate for the infectious not quarantined | MCMC |
γ | 0.071 (0.063, 0.078) | day−1 | Remove rate for the infected not quarantined | MCMC |
γq | 1/12.5 | day−1 | Remove rate for the infected and quarantined | [14] |
ω | 1/14 | day−1 | Release rate for the uninfected but traced | [15] |
S(0) | 1326000(1324000, 1328000) | Humans | Initial susceptible population | MCMC |
E(0) | 3349 (2764, 4327) | Humans | Initial latent population | MCMC |
I(0) | 28 (0, 102) | Humans | Initial infectious population | MCMC |
ST(0) | 509 (344, 674) | Humans | Initial uninfected but traced population | MCMC |
ET(0) | 80 (25, 97) | Humans | Initial infected and traced population | MCMC |
The basic reproduction number for model (2.1) is defined by R0=(1−ρ)ϕβ(εα+1γ)S0 according to the concepts of next generation matrix and reproduction number presented in [12,13].
We collected the data on the cumulative number for the confirmed infected cases and death cases from 10 January 2020 (as the starting date for the dynamical model, i.e., t=0) to 4 February 2020 from the National Health Commission of the People's Republic of China [1].
The mean incubation period for COVID-19 was 5.2 days [6]. The mean latent period for COVID-19 was not clear, so we take 1/α=5.2. The mean hospital stay was 12.5 days (1/γq=12.5) [14]. Suspected cases can be released after 14 days of follow-up [15], so we take ω=1/14. The remaining parameters in the model need to be estimated. We model cumulative cases as a Poisson-distributed random variable because the Poisson distribution describes the number of observed events in an interval of time. We calibrate the model by sampling from the posterior distribution of parameter vector θ|y={β,ϕ,ρ,ε,θ,η,ηq,γ}|y, where vector y is derived from ddtY(t)=αET+θγI and Y(t) denotes the reported cumulative cases. We conduct sampling via Markov Chain Monte Carlo using a Metropolis-Hastings acceptance rule. The posterior density is
fΘ|y(θ|y)=∏TL(Y(t)|θ)fΘ(θ). |
The likelihood L(Y(t)|θ) describes the probability of the data with a Poisson distribution. The prior density fΘ(θ) is the joint probability of eight univariate priors. We consider that β, ϕ, ρ, ε, θ, η, ηq and γ are distributed according to U(0,1). The program was implemented in R version 3.6.0 deBInfer package [16]. We sampled from 100, 000 MCMC iterations and discarded the first 90, 000 samples as a burn-in period. On the basis of these 10, 000 samples, the point estimates and 95% confidence intervals for those parameters were calculated. The initial conditions can be obtained from the actual data as R(0)=2, Iq(0)=41, D(0)=1. We left S(0), E(0), I(0), ST(0) and ET(0) as estimated parameters. The data from 10 January to 31 January were used for modeling and the one from 1 February to 4 February were used for validation. The results are shown in Table 1.
Based on the fitted model, we estimate the based reproductive number as R0≈5.78 (95%CI: 5.71–5.89), which means each infected person might infect an average of 5.78 people during the period of infection. The daily confirmed data from 1 February to 4 February were used to verify the model, which showed good prediction effect. Using the estimated parameter values, we can predict the trend of COVID-19 infection (see Figure 4). Under the current intervention (before 31 January)– intensive intensive contact tracing followed by quarantine and isolation, the number of daily confirmed infected individuals is expected to peak on around 11 February with a peak size of 4066 (95%CI: 3898–4472) daily confirmed infected individuals. Starting from 18 May, there will be no new confirmed cases, the cumulative number of cases will not increase, and the outbreak will be over.
We plot the cumulative infected cases and daily infected cases with varying contact rate β and traced rate ρ to examine the possible impact of enhanced interventions on COVID-19 infection (see Figure 5). As shown in Figure 5(a), reducing contact rate persistently decreases the peak value and may delay the peak. To be specific, it shows that reducing contact β by 0.5 times or 0.25 times will delay the peak by 28 days or 113 days, and lead to reduction of peak value in the number of daily infected cases by 54% or by 92%. Meanwhile, increasing the trace proportion of susceptible people decreases the peak value but may not delay the peak, as shown in Figure 5(b). This result indicates that interventions to reduce contact rate come first in control of the outbreak.
We estimated that the basic reproductive number for COVID-19 was 5.78 (95%CI: 5.71–5.89), which is higher than the basic reproductive number in previous studies, (for instance, 2.68 (95%CI: 2.47–2.86) in [9], 2.56 (95% CI: 2.49–2.63) in [10].) This may be due to the fact that there are some under-reported new cases in the early outbreak. We did not considered this factor and directly fitted the data without adjustment, then the basic reproductive number may be overestimated, which is in line with previous estimated [17] (6.47 (95%CI: 5.71–7.23)).
Based on our predictions, around 11 February, the number of daily confirmed infected individuals may reach a peak, and the outbreak will appear an inflection point. Although the inflection point may occur in the near future, it is important to keep it under control to avoid a new wave or outbreak, as secondary outbreaks tend to be more severe.
Reducing contact rate persistently decreases the peak value and may delay the peak, which is in line with the government's call for a reduction in unnecessary gathering. The delay in peak could buy time for new drug and vaccine development. Though increasing the trace proportion of susceptible people may not delay the peak, it is still a necessity to reduce the peak value. The resumption of work after the Spring Festival may bring potential risks to the control of the epidemic. It is suggested to pay close attention to the changes of the epidemic and choose the time of resumption carefully.
Because the daily data could contain more noise during the early stage, we use the cumulative incidence data rather than the daily incidence to make the simulations. Cumulative cases eliminate data fluctuations and will lead to a narrow 95% confidence interval. This is a limitation of the estimation in our paper. In our future work, we would use more detailed and accurate daily data to improve the estimation. A model is always an abstraction of reality and never a mirror of reality. This paper is only a mathematical results for reference. As more data on outbreaks follow, more accurate mathematical models will emerge.
In this paper, it is demonstrated that the current trends and future prediction of COVID-19 can be investigated by an appropriate mathematical model. Our model predicts that the daily confirmed COVID-19 cases will continuously increase in the next several days and reach the peak around 11 February. The outbreak is expected to end around 18 May. The basic reproductive number is estimated to be 5.78 (95%CI: 5.71–5.89) which means this outbreak is severe. At present, the most effective control is to reduce the contact rate which can not only reduce the peak value but also delay the peak.
This work was supported in part by the Natural Science Foundation of China (11961071, 82041026, 81673275, 91846302), the National S & T Major Project Foundation of China (2018ZX10715002, 2018ZX10713001).
The authors declare no conflict of interest.
[1] | Chinese Center for Disease Control and Prevention, Report about 2019-nCoV, 2020. Available from: http://www.chinacdc.cn/yyrdgz/202001/P020200128523354919292.pdf. |
[2] |
C. I. Paules, H. D. Marston, A. S. Fauci, Coronavirus infections-more than just the common cold, JAMA., 323 (2020), 707-708. doi: 10.1001/jama.2020.0757
![]() |
[3] |
J. F. Chan, K. H. Kok, Z. Zhu, H. CHu, K. K. To, S. Yuan, et al., Genomic characterization of the 2019 novel human-pathogenic coronavirus isolated from a patient with atypical pneumonia after visiting Wuhan, Emerg. Microbes Infec., 9 (2020), 221-236. doi: 10.1080/22221751.2020.1719902
![]() |
[4] |
N. Zhu, D. Y. Zhang, W. L. Wang, X. W. Li, B. Yang, J. Song, et al., A novel coronavirus from patients with pneumonia in China, 2019, N. Engl. J. Med., 382 (2020), 727-733. doi: 10.1056/NEJMoa2001017
![]() |
[5] |
C. Wang, P. W. Horby, F. G. Hayden, G. Gao, A novel coronavirus outbreak of global health concern, Lancet., 395 (2020), 470-473. doi: 10.1016/S0140-6736(20)30185-9
![]() |
[6] |
Q. Li, X. H. Guan, P. Wu, X. Wang, L. Zhou, Y. Tong, et al., Early transmission dynamics in Wuhan, China, of novel coronavirus infected pneumonia, N. Engl. J. Med., 382 (2020), 1199-1207. doi: 10.1056/NEJMoa2001316
![]() |
[7] |
J. F. Chan, S. F. Yuan, K. H. Kok, K. K. To, H. Chu, J. Yang, et al., A familial cluster of pneumonia associated with the 2019 novel coronavirus indicating person-to-person transmission: a study of a family cluster, Lancet., 395 (2020), 514-523. doi: 10.1016/S0140-6736(20)30154-9
![]() |
[8] |
H. Nishiura, S. M. Jung, N. M. Linton, R. Kinoshita, Y. Yang, K. Hayashi, et al., The Extent of Transmission of Novel Coronavirus in Wuhan, J. Clin. Med., 9 (2020), 330. doi: 10.3390/jcm9020330
![]() |
[9] | J. T. Wu, K. Leung, G. M. Leung, Nowcasting and forecasting the potential domestic and international spread of the 2019-nCoV outbreak originating in Wuhan, China: a modelling study, Lancet., 395 (2020), 689-697. |
[10] |
S. Zhao, S. S. Musa, Q. Lin, J. Ran, G. Yang, W. Wang, et al., Estimating the Unreported Number of Novel Coronavirus 2019-nCoV Cases in China in the First Half of January 2020: A Data-Driven Modelling Analysis of the Early Outbreak, J. Clin. Med., 9 (2020), 388. doi: 10.3390/jcm9020388
![]() |
[11] | Situation report of the pneumonia cases caused by the novel coronavirus, released on January 31, 2020 by the National Health Commission of the People's Republic of China (in Chinese), 2020. Available from: http://www.nhc.gov.cn/xcs/yqtb/202002/84faf71e096446fdb1ae44939ba5c528.shtml. |
[12] | O. Diekmann, J. A. P. Heesterbeek, J. A. J. Metz, On the definition and the computation of the basic reproduction ratio R0, in models for infectious diseases in heterogeneous populations, J. Math. Biol., 28 (1990), 365-382. |
[13] |
P. Driessche, J. Watmough, Reproduction numbers and sub-threshold endemic equilibria for compartmental models of disease transmission, Math. Biosci., 180 (2002), 29-48. doi: 10.1016/S0025-5564(02)00108-6
![]() |
[14] | Central People's Government of the People's Republic of China, Available from: http://www.gov.cn/xinwen/2020-02/01/content$_$5473742.htm. |
[15] | National Health Commission of the People's Republic of China, Available from: http://www.nhc.gov.cn/xcs/kpzs/202001/a4499dd30c484457b1c05e3590fae794.shtml. |
[16] |
P. H. Boersch-Supan, S. J. Ryan, L. R. Johnson, deBInfer: Bayesian inference for dynamical models of biological systems in R, Methods Ecol. Evol., 8 (2017), 511-518. doi: 10.1111/2041-210X.12679
![]() |
[17] |
B. Tang, X. Wang, Q. Li, N. L. Bragazzi, S. Tang, Y. xiao, et al., Estimation of the Transmission Risk of the 2019-nCoV and Its Implication for Public Health Interventions, J. Clin. Med., 9 (2020), 462. doi: 10.3390/jcm9020462
![]() |
1. | Le Khanh Ngan Nguyen, Susan Howick, Dennis McLafferty, Gillian H. Anderson, Sahaya J. Pravinkumar, Robert Van Der Meer, Itamar Megiddo, Evaluating intervention strategies in controlling coronavirus disease 2019 (COVID-19) spread in care homes: An agent-based model, 2020, 0899-823X, 1, 10.1017/ice.2020.1369 | |
2. | Marcello Trovati, 2021, chapter 3, 9781799867364, 44, 10.4018/978-1-7998-6736-4.ch003 | |
3. | Marcelo M. Morato, Igor M.L. Pataro, Marcus V. Americano da Costa, Julio E. Normey-Rico, A parametrized nonlinear predictive control strategy for relaxing COVID-19 social distancing measures in Brazil, 2020, 00190578, 10.1016/j.isatra.2020.12.012 | |
4. | Hamdy M. Youssef, Najat A. Alghamdi, Magdy A. Ezzat, Alaa A. El-Bary, Ahmed M. Shawky, A modified SEIR model applied to the data of COVID-19 spread in Saudi Arabia, 2020, 10, 2158-3226, 125210, 10.1063/5.0029698 | |
5. | Jianli Liu, Yuan Zhou, Chuanyu Ye, Guangming Zhang, Feng Zhang, Chunjuan Song, The spatial transmission of SARS-CoV-2 in China under the prevention and control measures at the early outbreak, 2021, 79, 2049-3258, 10.1186/s13690-021-00529-z | |
6. | Khagendra Adhikari, Ramesh Gautam, Anjana Pokharel, Kedar Nath Uprety, Naveen K. Vaidya, Transmission dynamics of COVID-19 in Nepal: Mathematical model uncovering effective controls, 2021, 521, 00225193, 110680, 10.1016/j.jtbi.2021.110680 | |
7. | Haitao Song, Zhongwei Jia, Zhen Jin, Shengqiang Liu, Estimation of COVID-19 outbreak size in Harbin, China, 2021, 0924-090X, 10.1007/s11071-021-06406-2 | |
8. | Hamdy Youssef, Najat Alghamdi, Magdy A. Ezzat, Alaa A. El-Bary, Ahmed M. Shawky, Study on the SEIQR model and applying the epidemiological rates of COVID-19 epidemic spread in Saudi Arabia, 2021, 6, 24680427, 678, 10.1016/j.idm.2021.04.005 | |
9. | Tanvir Ahammed, Aniqua Anjum, Mohammad Meshbahur Rahman, Najmul Haider, Richard Kock, Md Jamal Uddin, Estimation of novel coronavirus ( COVID ‐19) reproduction number and case fatality rate: A systematic review and meta‐analysis , 2021, 4, 2398-8835, 10.1002/hsr2.274 | |
10. | M. A. El-Shorbagy, Mati ur Rahman, Maryam Ahmed Alyami, On the analysis of the fractional model of COVID-19 under the piecewise global operators, 2023, 20, 1551-0018, 6134, 10.3934/mbe.2023265 | |
11. | Moirangthem Bidyaluxmi Devi, Arpita Devi, Praveen Kumar Gupta, Dharmendra Tripathi, Response of vaccination on community transmission of COVID-19: a dynamical approach, 2022, 231, 1951-6355, 3749, 10.1140/epjs/s11734-022-00652-0 | |
12. | Indrajit Ghosh, Tanujit Chakraborty, An integrated deterministic–stochastic approach for forecasting the long-term trajectories of COVID-19, 2021, 12, 1793-9623, 2141001, 10.1142/S1793962321410014 | |
13. | Sanjoy Basu, R. Prem Kumar, P.K. Santra, G.S. Mahapatra, A.A. Elsadany, Preventive control strategy on second wave of Covid-19 pandemic model incorporating lock-down effect, 2022, 61, 11100168, 7265, 10.1016/j.aej.2021.12.066 | |
14. | Sui Zhang, Zhao Yang, Minghao Wang, Baolei Zhang, “Distance‐Driven” Versus “Density‐Driven”: Understanding the Role of “Source‐Case” Distance and Gathering Places in the Localized Spatial Clustering of COVID‐19—A Case Study of the Xinfadi Market, Beijing (China), 2021, 5, 2471-1403, 10.1029/2021GH000458 | |
15. | Tailei Zhang, Zhimin Li, Analysis of COVID-19 epidemic transmission trend based on a time-delayed dynamic model, 2023, 22, 1534-0392, 1, 10.3934/cpaa.2021088 | |
16. | Shengtao Wang, Yan Li, Ximei Wang, Yuanyuan Zhang, Yiyi Yuan, Yong Li, Giulia Morsica, The Impact of Lockdown, Patient Classification, and the Large-Scale Case Screening on the Spread of the Coronavirus Disease 2019 (COVID-19) in Hubei, 2022, 2022, 2314-6141, 1, 10.1155/2022/8920117 | |
17. | Zhen Wang, Sajjad Shaukat Jamal, Baonan Yang, Viet-Thanh Pham, Complex behavior of COVID-19’s mathematical model, 2022, 231, 1951-6355, 885, 10.1140/epjs/s11734-021-00309-4 | |
18. | Yan Wang, Feng Qing, Haozhan Li, Xuteng Wang, Timely and effective media coverage's role in the spread of Corona Virus Disease 2019, 2022, 0170-4214, 10.1002/mma.8732 | |
19. | Jinxing Guan, Yang Zhao, Yongyue Wei, Sipeng Shen, Dongfang You, Ruyang Zhang, Theis Lange, Feng Chen, Transmission dynamics model and the coronavirus disease 2019 epidemic: applications and challenges, 2022, 2, 2749-9642, 89, 10.1515/mr-2021-0022 | |
20. | Pablo Abuin, Alejandro Anderson, Antonio Ferramosca, Esteban A. Hernandez-Vargas, Alejandro H. Gonzalez, Dynamical characterization of antiviral effects in COVID-19, 2021, 52, 13675788, 587, 10.1016/j.arcontrol.2021.05.001 | |
21. | R. Prem Kumar, Sanjoy Basu, Dipankar Ghosh, Prasun Kumar Santra, G. S. Mahapatra, Dynamical analysis of novel COVID ‐19 epidemic model with non‐monotonic incidence function , 2022, 22, 1472-3891, 10.1002/pa.2754 | |
22. | Tshilidzi Marwala, Wilson Tsakane Mongwe, Rendani Mbuvha, 2023, 9780443190353, 123, 10.1016/B978-0-44-319035-3.00023-9 | |
23. | Hamdy M. Youssef, Najat Alghamdi, Magdy A. Ezzat, Alaa A. El-Bary, Ahmed M. Shawky, A proposed modified SEIQR epidemic model to analyze the COVID-19 spreading in Saudi Arabia, 2022, 61, 11100168, 2456, 10.1016/j.aej.2021.06.095 | |
24. | 2023, 9780443190353, 175, 10.1016/B978-0-44-319035-3.00033-1 | |
25. | Haitao Song, Fang Liu, Feng Li, Xiaochun Cao, Hao Wang, Zhongwei Jia, Huaiping Zhu, Michael Y. Li, Wei Lin, Hong Yang, Jianghong Hu, Zhen Jin, Modeling the second outbreak of COVID-19 with isolation and contact tracing, 2022, 27, 1531-3492, 5757, 10.3934/dcdsb.2021294 | |
26. | M. El Sayed, M. A. El Safty, M. K. El-Bably, Topological approach for decision-making of COVID-19 infection via a nano-topology model, 2021, 6, 2473-6988, 7872, 10.3934/math.2021457 | |
27. | Brandon Kaiheng Tay, Carvalho Andrea Roby, Jodi Wenjiang Wu, Da Yang Tan, Dynamical Analysis of Universal Masking on the Pandemic, 2021, 18, 1660-4601, 9027, 10.3390/ijerph18179027 | |
28. | Zhengyu Shi, Haoqi Qian, Yao Li, Fan Wu, Libo Wu, Machine learning based regional epidemic transmission risks precaution in digital society, 2022, 12, 2045-2322, 10.1038/s41598-022-24670-z | |
29. | Le Khanh Ngan Nguyen, Susan Howick, Dennis McLafferty, Gillian Hopkins Anderson, Sahaya Josephine Pravinkumar, Robert Van Der Meer, Itamar Megiddo, Impact of visitation and cohorting policies to shield residents from covid-19 spread in care homes: an agent-based model, 2021, 49, 01966553, 1105, 10.1016/j.ajic.2021.07.001 | |
30. | Srikanth Aravamuthan, Juan Francisco Mandujano Reyes, Brian S. Yandell, Dörte Döpfer, Real-time estimation and forecasting of COVID-19 cases and hospitalizations in Wisconsin HERC regions for public health decision making processes, 2023, 23, 1471-2458, 10.1186/s12889-023-15160-6 | |
31. | Xin Tang, Hui Cong Wu, Yi Chen, 2022, Prediction of COVID-19 Confirmed Cases Combining The LSTM Model and Evolutionary Strategy, 979-8-3503-2000-8, 637, 10.1109/IAECST57965.2022.10061986 | |
32. | Jorge M. Mendes, Pedro S. Coelho, The effect of non-pharmaceutical interventions on COVID-19 outcomes: A heterogeneous age-related generalisation of the SEIR model, 2023, 8, 24680427, 742, 10.1016/j.idm.2023.05.009 | |
33. | R. Prem Kumar, Sanjoy Basu, P. K. Santra, Abdelalim A. Elsadany, Amr Elsonbaty, G. S. Mahapatra, A. Al-khedhairi, Global stability and sensitivity analysis of parameters of Omicron variant epidemic in diverse susceptible classes incorporating vaccination stages, 2024, 28, 1432-7643, 4689, 10.1007/s00500-023-09170-0 |
Parameters | Value (95% CI) | Dimension | Interpretation | Source |
β | 2.6×10−6(2.4×10−6,2.9×10−6) | dimensionless | Contact rate | MCMC |
ϕ | 0.0923 (0.0917, 0.0963) | dimensionless | Probability of transmission per contact | MCMC |
ρ | 0.0171 (0.0151, 0.0198) | dimensionless | Traced rate | MCMC |
ε | 0.914 (0.860, 0.939) | dimensionless | Infectious ability of the latent | MCMC |
1α | 5.2 | day | Mean incubation period | [6] |
θ | 0.0952 (0.0890, 0.0977) | day−1 | Quarantined rate of the infectious | MCMC |
ηq | 0.00161 (0.0145, 0.0177) | day−1 | Disease induced death rate for the infectious and quarantined | MCMC |
η | 0.00392 (0.00352, 0.00427) | day−1 | Disease induced death rate for the infectious not quarantined | MCMC |
γ | 0.071 (0.063, 0.078) | day−1 | Remove rate for the infected not quarantined | MCMC |
γq | 1/12.5 | day−1 | Remove rate for the infected and quarantined | [14] |
ω | 1/14 | day−1 | Release rate for the uninfected but traced | [15] |
S(0) | 1326000(1324000, 1328000) | Humans | Initial susceptible population | MCMC |
E(0) | 3349 (2764, 4327) | Humans | Initial latent population | MCMC |
I(0) | 28 (0, 102) | Humans | Initial infectious population | MCMC |
ST(0) | 509 (344, 674) | Humans | Initial uninfected but traced population | MCMC |
ET(0) | 80 (25, 97) | Humans | Initial infected and traced population | MCMC |
Parameters | Value (95% CI) | Dimension | Interpretation | Source |
β | 2.6×10−6(2.4×10−6,2.9×10−6) | dimensionless | Contact rate | MCMC |
ϕ | 0.0923 (0.0917, 0.0963) | dimensionless | Probability of transmission per contact | MCMC |
ρ | 0.0171 (0.0151, 0.0198) | dimensionless | Traced rate | MCMC |
ε | 0.914 (0.860, 0.939) | dimensionless | Infectious ability of the latent | MCMC |
1α | 5.2 | day | Mean incubation period | [6] |
θ | 0.0952 (0.0890, 0.0977) | day−1 | Quarantined rate of the infectious | MCMC |
ηq | 0.00161 (0.0145, 0.0177) | day−1 | Disease induced death rate for the infectious and quarantined | MCMC |
η | 0.00392 (0.00352, 0.00427) | day−1 | Disease induced death rate for the infectious not quarantined | MCMC |
γ | 0.071 (0.063, 0.078) | day−1 | Remove rate for the infected not quarantined | MCMC |
γq | 1/12.5 | day−1 | Remove rate for the infected and quarantined | [14] |
ω | 1/14 | day−1 | Release rate for the uninfected but traced | [15] |
S(0) | 1326000(1324000, 1328000) | Humans | Initial susceptible population | MCMC |
E(0) | 3349 (2764, 4327) | Humans | Initial latent population | MCMC |
I(0) | 28 (0, 102) | Humans | Initial infectious population | MCMC |
ST(0) | 509 (344, 674) | Humans | Initial uninfected but traced population | MCMC |
ET(0) | 80 (25, 97) | Humans | Initial infected and traced population | MCMC |