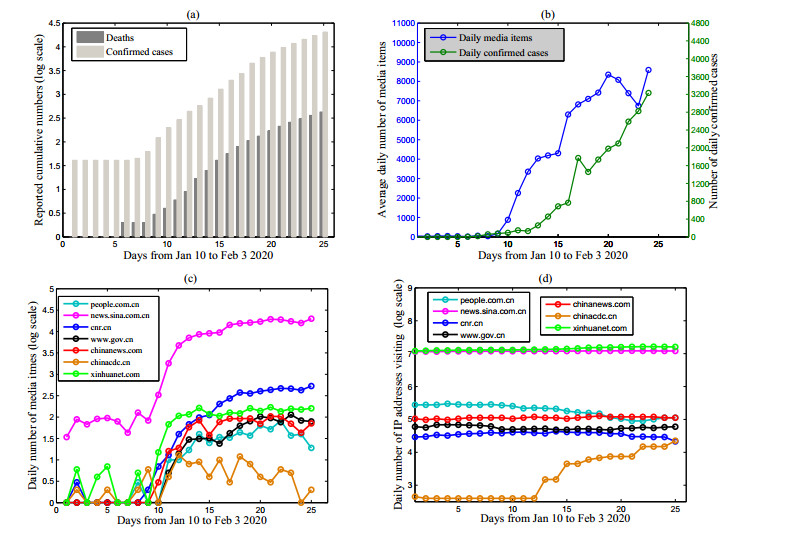
Citation: Weike Zhou, Aili Wang, Fan Xia, Yanni Xiao, Sanyi Tang. Effects of media reporting on mitigating spread of COVID-19 in the early phase of the outbreak[J]. Mathematical Biosciences and Engineering, 2020, 17(3): 2693-2707. doi: 10.3934/mbe.2020147
[1] | Jin Guo, Aili Wang, Weike Zhou, Yinjiao Gong, Stacey R. Smith? . Discrete epidemic modelling of COVID-19 transmission in Shaanxi Province with media reporting and imported cases. Mathematical Biosciences and Engineering, 2022, 19(2): 1388-1410. doi: 10.3934/mbe.2022064 |
[2] | Xiaojing Wang, Yu Liang, Jiahui Li, Maoxing Liu . Modeling COVID-19 transmission dynamics incorporating media coverage and vaccination. Mathematical Biosciences and Engineering, 2023, 20(6): 10392-10403. doi: 10.3934/mbe.2023456 |
[3] | Hamdy M. Youssef, Najat A. Alghamdi, Magdy A. Ezzat, Alaa A. El-Bary, Ahmed M. Shawky . A new dynamical modeling SEIR with global analysis applied to the real data of spreading COVID-19 in Saudi Arabia. Mathematical Biosciences and Engineering, 2020, 17(6): 7018-7044. doi: 10.3934/mbe.2020362 |
[4] | Jiajia Zhang, Yuanhua Qiao, Yan Zhang . Stability analysis and optimal control of COVID-19 with quarantine and media awareness. Mathematical Biosciences and Engineering, 2022, 19(5): 4911-4932. doi: 10.3934/mbe.2022230 |
[5] | Dipo Aldila, Meksianis Z. Ndii, Brenda M. Samiadji . Optimal control on COVID-19 eradication program in Indonesia under the effect of community awareness. Mathematical Biosciences and Engineering, 2020, 17(6): 6355-6389. doi: 10.3934/mbe.2020335 |
[6] | Tao Chen, Zhiming Li, Ge Zhang . Analysis of a COVID-19 model with media coverage and limited resources. Mathematical Biosciences and Engineering, 2024, 21(4): 5283-5307. doi: 10.3934/mbe.2024233 |
[7] | Tianfang Hou, Guijie Lan, Sanling Yuan, Tonghua Zhang . Threshold dynamics of a stochastic SIHR epidemic model of COVID-19 with general population-size dependent contact rate. Mathematical Biosciences and Engineering, 2022, 19(4): 4217-4236. doi: 10.3934/mbe.2022195 |
[8] | Yujie Sheng, Jing-An Cui, Songbai Guo . The modeling and analysis of the COVID-19 pandemic with vaccination and isolation: a case study of Italy. Mathematical Biosciences and Engineering, 2023, 20(3): 5966-5992. doi: 10.3934/mbe.2023258 |
[9] | Li-Xiang Feng, Shuang-Lin Jing, Shi-Ke Hu, De-Fen Wang, Hai-Feng Huo . Modelling the effects of media coverage and quarantine on the COVID-19 infections in the UK. Mathematical Biosciences and Engineering, 2020, 17(4): 3618-3636. doi: 10.3934/mbe.2020204 |
[10] | Allison Fisher, Hainan Xu, Daihai He, Xueying Wang . Effects of vaccination on mitigating COVID-19 outbreaks: a conceptual modeling approach. Mathematical Biosciences and Engineering, 2023, 20(3): 4816-4837. doi: 10.3934/mbe.2023223 |
Recently, a novel coronavirus is spreading across China and causes a severe outbreak of viral pneumonia, catching the eyes of the world. In December 2019, cases of pneumonia of unknown etiology (unknown cause) were reported in Wuhan City, Hubei Province of China [1]. It was found that a novel coronavirus was the causative virus on 7 January 2020, which was provisionally named 2019 novel corona virus (2019-nCoV) [2]. Though similar with SARS coronavirus and MERS coronavirus, the novel coronavirus is distinct from them [3]. It causes a severe acute respiratory illness and the range of symptoms includes fever, cough, and dyspnoea, with chest radiographs revealing invasive lesions in both lungs [4].
The viral pneumonia was initially reported only in Wuhan in early January 2020, while with the coming of the Chinese Spring Festival, people were travelling more frequently, infected cases were informed in other provinces of China in the next few days. Though the government has taken actions such as travel restriction of Wuhan in time, the COVID-19 is still spreading rapidly in China. As of 7 February 2020, 31, 774 confirmed cases and 27, 657 suspected cases of COVID-19 infection have been reported in 31 Chinese provinces or municipalities in mainland China, with 722 deaths and 2050 cured cases, 345, 498 in contact tracing, of which 189, 600 are in clinical medicine observation [5]. In addition, a number of confirmed COVID-19 infection cases have been reported in other countries, including Thailand, Japan, Republic of Korea, USA, Canada, Vietnam, Singapore, France, Germany, Australia, and so on. On 31 January 2020, WHO announced that the COVID-19 outbreak constitutes a Public Heath Emergency of International Concern (PHEIC). On 7 February 2020, the pneumonia infected by the novel coronavirus was provisionally named as Novel Coronavirus Pneumonia (NCP) [6] and formally named as Corona Virus Disease 2019 (COVID-19) by WHO on 11 February [2]. The rapid spread of COVID-19 poses a great burden on the development of the economy and society, causing a great deal of concern among the public and the authorities.
The limitation of the medical resources, the absence of specific anti-COVID-19 therapeutic treatment and effective vaccine against COVID-19, all make it more difficult to curb the transmission of the pneumonia. Thus it's essential to consider some other avenues of control or interventions. Actually, travel restrictions were implemented in China which effectively reduced mobility of millions, and people are requested to stay at home during the outbreak of COVID-19 to reduce the contact rate with others, which indeed contribute to the prevention and control of the disease. However, in the early phase of the outbreak, few information was revealed and few people were aware of the disease, leading to the continuation of frequent movements and the absence of timely control measures of the public, which results in the more severe situation than it is supposed to be. There are evidences shown that media reports and education have the potential to affect the awareness of the public, thus can modify the community's behaviors during the infectious disease outbreak [7,8]. We've known that behavior change during infectious disease outbreaks can curb the disease spread in populations [9], then examining whether and how the media reporting and the infection dynamics correlating becomes very important, especially during the COVID-19 outbreak.
In fact, the relationship between the mass media and the disease spread is complex and mutual. On one hand, the media reporting about the COVID-19 may influence the attitude of the public towards the disease and enhance their self-protecting awareness. People informed by the media reports will change their behaviours and take correct precautions such as frequent hand-washing, wearing protective masks, keeping social distances, and even quarantining themselves at home to avoid contacting with and being infected by others. On the other hand, the degree of mass media attention to COVID-19 is determined by the severity of the outbreak. While how the media reports contributes to the prevention and control of the COVID-19 infection, and how media reports affect the peak time and peak value of the outbreak remain unknown. Thus quantifying the effectiveness of the media reports on mitigating the spread of COVID-19 is of crucial importance during the early stage of the outbreak. This falls within the scope of our study.
In this study, we developed a deterministic dynamical model to describe the human-to-human transmission of COVID-19 considering media impact. Disease progression is characterized by the basic SEIR model and media is portrayed by disease statistics (number of daily confirmed cases) and incorporated as a separate compartment in the model. We estimated the key parameters and the basic reproduction number of the proposed model by using the accumulated number of reported confirmed cases, the accumulated number of reported deaths, and the average daily number of media items. A sensitivity analysis was carried out to identify parameters affecting the peak time and peak size of the outbreak most, and to indicate the effectiveness of enhancing the media reporting intensity and awareness of the public.
We obtained the data of COVID-19 cases in Mainland China from the National Health Commission of the People's Republic of China. The data information includes the reported cumulative number of confirmed cases and reported number of deaths, as shown in Figure 1(a). The number of daily confirmed cases (newly reported) was also shown in Figure 1(b). Though the first case was reported in December 2019, no newly confirmed case was reported until 10 January 2020, and 41 confirmed cases were reported on 10 January 2020. Furthermore, we collected the information of 22 cured cases anonymously, especially their curative hospitalization periods, and calculated the average period from hospitalization to recovery as 7.68 days.
We also collected the daily number of media items on the COVID-19 from seven authoritative or popular websites including cnr.cn (China National Radio), gov.cn (the Central People's Government of the People's Republic of China), chinacdc.cn (Chinese Center for Disease Control and Prevention), xinhuanet.com (Xinhuanet), people.com.cn (People's Daily Online), chinanews.com (China News), and news.sina.com.cn (Sina) from 10 January 2020, by using the key word 'novel coronavirus' included in the title or the full text, as shown in Figure 1(c). In addition, we obtained the daily number of IP addresses that visit each website, as shown in Figure 1(d).
Then we got the average daily number of media items by defining an index 'hotness' as the weighting coefficients to average the number of media items collected in the seven websites, as shown in Figure 1(b). Denote the daily number of media items collected in each website by xi, and the daily number of IP addresses visiting each website as pi, where i=1,2,3,4,5,6,7 denote cnr.cn, gov.cn, chinacdc.cn, xinhuanet.com, people.com.cn, chinanews.com, news.sina.com.cn, respectively. Then the average daily number of media items x is
x=hixi, |
where hi=piΣ7i=1pi represents the index hotness of each website.
We can observe directly from Figure 1(b) that the average daily number of media items and the number of daily confirmed cases are in a strong relationship. Then denote the number of daily confirmed cases of COVID-19 by y, we analyzed the association between the average daily number of media items (x) and the number of daily confirmed cases (y) from January 10 to 31, 2020 at specific lags, by conducting the cross-correlation analysis [10,11].
We proposed a dynamic compartmental model (2.1) incorporating the awareness programs as a separate compartment to describe the transmission of COVID-19 affected by the media reports, based on the disease progression, the intervention measures, and the interconnected relationship between media reports and the disease progression. In this model, the population is divided into seven compartments, including susceptible (S), quarantined susceptible (Sq), infectious without symptoms (or exposed E), quarantined exposed (Eq), infectious with symptoms (or infected I), hospitalized (H) and recovered (R), M represents the cumulative density of awareness programs driven by the media reports.
Here we assumed that contact tracing is implemented and a proportion of q of individuals exposed to the virus is quarantined and the other proportion of 1−q is missed from the contact tracing. The quarantined individuals and the individuals missed from the contact tracing have a probability β of effectively infected and then move to the (quarantined) exposed compartment (Eq or E), while the others, with a probability of 1−β, would stay in the (quarantined) susceptible compartment (Sq or S). We also assume that the quarantined exposed individuals (Eq) would be hospitalized once the detected result is positive. c is the contact rate, β is the transmission probability, θ is the relative transmission probability of exposed individuals to infected individuals, 1/σ is the incubation period and α is the disease-induced death rate, δI and δq are the hospitalized rates for I and Eq, respectively, γI and γH are the cured rates for infected and hospitalized individuals, respectively, λ is the releasing rate of quarantined individuals. For the parameters related with the media reports, e−pM is the media function which means that the contact rate is reduced due to awareness driven by media reports, λM represents the initiative quarantined rate induced by awareness driven by media reports, η is the response intensity of awareness programs on the number of newly confirmed cases, μM is the waning rate of awareness programs due to ineffectiveness, social problems, etc. See more detailed definitions of variables and parameters listed in Table 1.
{S′=−(ce−pMβ+ce−pMq(1−β))S(I+θE)−λMSM+λSq,E′=ce−pMβ(1−q)S(I+θE)−σE−λMEM,I′=σE−(α+δI+γI)I,S′q=ce−pM(1−β)qS(I+θE)+λMSM−λSq,E′q=ce−pMβqS(I+θE)+λMEM−δqEq,H′=δII+δqEq−γHH−αH,R′=γII+γHH,M′=η(δII+δqEq)−μMM. | (2.1) |
Variables | Description | initial value | Resource |
S | Susceptible population | 11081000 | [12, 13] |
E | Exposed population | 118.552 | LS |
I | Infected population with symptomatic | 20 | LS |
Sq | Quarantined susceptible population | 739 | [16] |
Eq | isolated exposed population | 1 | LS |
H | Hospitalized population | 1 | [13] |
R | Recovered population | 2 | [16] |
M | Media items | 16.3 | Data |
Parameters | Description | Value | Resource |
c | Contact rate (per person per day) | 10.582 | LS |
β | Probability of transmission from I to S per contact | 2.010×10−8 | LS |
θ | Relative transmission probability of E compared with I | 1.000×10−6 | LS |
q | Quarantined proportion of latent individuals | 1.000×10−8 | LS |
p | Weight of media effect on contact rate sensitive to media items | 9.003×10−5 | LS |
λM | Quarantined rate of individuals induced by media reporting (per day) | 5.471×10−5 | LS |
λ | Releasing rate of quarantined individuals (per day) | 1/14 | [13] |
σ | Progression rate of exposed individuals to infectives (per day) | 1/5 | [14,17,18] |
α | Disease-induced death rate (per day) | 0.003 | LS |
δI | Progression rate of infectives to hospitalized individuals (per day) | 0.110 | LS |
γI | Recovery rate of infected individuals (per day) | 0.330 | [13] |
δq | Progression rate of quarantined exposed to hospitalized class (per day) | 0.102 | LS |
γH | Recovery rate of hospitalized individuals (per day) | 0.130 | Data |
η | Media reporting rate of number of newly hospital notifications (per person) | 2.951 | LS |
μM | Media waning rate (per day) | 0.735 | LS |
We used data extracted from the National Health Commission of the People's Republic of China from 10 January to 31 January, 2020, to study the effects of media reports. Parameter values for model (2.1) were informed by the literature or further estimated by data fitting using the Least Square Method.
In the initial stage of the epidemic, the COVID-19 was mainly transmitted in Wuhan, where the population of inhabitants was about 11, 081, 000 [12], thus we set S(0)=11,081,000 [13]. The quarantined individuals are required to be isolated 14 days, thus λ=1/14 [13]. The incubation period is 3–7 days [14], here we choose σ=1/5. As illustrated in the previous section, the average period from hospitalization to recovery is 7.68 days, thus we choose γ=1/7.68.
To identify the key parameters that influence the disease infection dynamics, we examined the dependence of the peak time and peak size of the total number of infected and hospitalized individuals ((I(t)+H(t)) on corresponding model parameters, by using Latin Hypercube Sampling (LHS) and partial rank correlation coefficients (PRCCs).
Sample cross-correlation coefficients are shown in Figure 2, from which we can see that x is positive correlated to y at lags ranging from −5 to 3 and the local maximal cross-correlation coefficient occurs at lag = 0. The statistically significant cross-correlation between x and y indicates that the average daily number of media items and the number of daily confirmed cases are strongly interconnected. This confirms the comparatively reasonable modelling of the media compartment in model (2.1).
As we all know, the transmissibility of a virus at the initial stage of an epidemic is measured by the basic reproduction number R0, which measures the average number of new infections generated by one infected individual in the population during the average infection period. By using the next generation matrix, we got the basic reproduction number for system (2.1) as
R0=(cβ(1−q)δI+α+γI+cβ(1−q)θσ)S0. |
Then by fitting the model to the data of accumulated number of confirmed cases, accumulated number of deaths, and average daily number of media items from January 10 to January 31, 2020, the basic reproduction number is estimated as R0=5.3167, which is lower than the result in [13,15] while greater than that in [18,19], and the parameter estimations are shown in Table 1. The good fitness is shown in Figure 3, in which the black markers are the data from January 10–31, the red curves are the fitting curves, and the blue markers are the data from February 1–3 to verify our estimation and prediction of the trend of COVID-19 infection and the media reports.
It follows from Figure 4 that the contact rate c, the transmission probability β, the transition rate from exposed to symptomatic σ (or the incubation period 1/σ) are the most sensitive parameters to the peak time and peak size. In particular, Figure 4 shows that reducing the contact rate c and decreasing the transmission probability β could delay the peak time and lower the peak size of the total number of infected and hospitalized individuals, shortening the incubation period 1/σ and the hospitalized period 1/γH, could bring forward the peak time significantly, increasing the hospitalized rate of infected individuals δI can greatly reduce the peak size of the total number of infected and hospitalized individuals. Despite these significantly sensitive parameters associated with the disease transmissibility, the parameters related to the media reports can also significantly affect the results. To be more detailed, enhancing the response intensity of awareness programs on the number of newly confirmed cases η, or improving the awareness and quarantined rate of individuals to media reports λM could not only bring forward the peak time but also reduce the peak size of the total number of infected and hospitalized individuals significantly. Since we are focusing on the media impact on the transmission of COVID-19, we plotted the contour plots to examine the dependence of the peak time and peak size of the total number of infected and hospitalized individuals on the media reporting rate η and the media waning rate μM (Figure 5), and on the weight of the media impact on the contact rate p and the media-induced quarantined rate λM (Figure 6). The results indicated that, with increasing of η, λM, p, and decreasing of μM, the peak time is brought forward and the peak size is lowered, illustrating that the media reports during the early stage of the disease outbreak would reduce the severity of the epidemic.
To further investigate the explicit effectiveness of media reports on the COVID-19 outbreak, we plotted the total number of infected and hospitalized individuals (I(t)+H(t)) with different values of the media reporting rate η (Figure 7(a)), the media waning rate μM (Figure 7(b)), the weight of the media impact on the contact rate p (Figure 7(c)), the media-induced quarantined rate λM (Figure 7(d)), respectively, with all other parameters fixed. In particular, the results showed that enhancing the response rate of the media reports to the newly confirmed cases, i.e., increasing η by 5 times from its baseline value would lead to 4.35 days earlier of the peak time (from 22.08 days to 17.73 days after January 10) and reduce the peak size from 13, 063 to 2630 (decreased by 79.87%), as shown in Figure 7(a). Reducing the media waning rate μM to 0.1μM could bring forward the peak time to 18.84 days after January 10 (3.24 days earlier) and reduce the peak size to 5112 (reduced by 60.87%), as can be seen in Figure 7(b). Meanwhile, enhancing the response rate of the public to the media reports, for example, increasing the media induced quarantined rate λM by 10 times from its baseline value would bring forward the peak time by 4.28 days (from 22.08 days to 17.8 days after January 10), and reduce the peak size from 13, 063 to 1787 (reduced by 86.31%), as shown in Figure 7(d), or, increasing p to 8p could bring forward the peak time to 20.06 days after January 10 (about 2 days earlier) and reduce the peak size to 5172 (reduced by 60.41%), as shown in Figure 7(c). These sensitivity analysis showed that enhancing the response rate of the media reporting to the severity of COVID-19, and enhancing the response rate of the public awareness to the media reports, both can bring forward the peak time and reduce the peak size of the infection significantly, during the early phase of the COVID-19 outbreak.
Since the first pneumonic case of novel coronavirus was reported in Wuhan City, Hubei Province, China on 12 December 2019, an outbreak of novel coronavirus (COVID-19) has swiftly spread across 34 Chinese provinces or municipalities in China and a number of foreign countries. It is well knowledged that exposed infection and person-to-person transmission appear possible [20] and Chinese Government has implemented timely control measures to mitigate the spread of the virus. However, in the current situation, medical resources are in critical needed and antiviral drugs or vaccine are still in development, control measures mainly include the various social and personal non-pharmaceutical interventions, including media education. The media educated public then take various precautions, for instance, wearing face masks, keeping social distance and frequent hand-washing, to reduce the chance of being infected. This has indeed influenced the pattern of disease transmission and lowered the rate of contact. From the viewpoint of the public health control, it is important to quantify how the number of daily media items provide new insights into the progression of COVID-19 and consequently help curb COVID-19 spreading. To address such an important issue, we have proposed a deterministic dynamic model based on the transmission pattern and clinical feature of COVID-19 in our work. The mass media is represented as a state variable, and a negative exponential function is introduced to the transmission term to describe the effect of media on the contact rate, and also, a media-induced quarantine is considered.
To get a comprehensive understanding of the media effects during the early phase of COVID-19 outbreak, we have collected the data including the daily number of media items and the number of IP addresses visiting seven popular and authoritative websites: cnr.cn, gov.cn, chinacdc.cn, xinhuanet.com, people.com.cn, chinanews.com, and news.sina.com.cn (Figure 1(c) and 1(d)). By introducing the index 'hotness' of a website according to the number of visiting IP addresses, we have calculated the average daily number of media items (Figure 1(b)), from which we can intuitionisticly see that the number of daily confirmed cases and the average daily number of media items are in a strong relationship. The cross-correlation analysis verified that (Figure 2) and confirmed the comparatively reasonable modelling of the media compartment in model (2.1). By using the data of cumulative number of confirmed cases, cumulative number of deaths and average daily number of media items, the unknown parameters were estimated with Least Square Method. Note that the basic reproduction number was calculated as 5.3167, greater than WHO estimates (1.4–2.5) and the results in [18,19,21], but lower than the results in [13,15]. A review research about the basic reproduction number has shown that the WHO may underestimate the R0, which is lower than almost all studies in the early stage [22]. Meanwhile, the estimation of R0 depends on the methods used, the assumptions and the length of time intervals of data. In particular, in the early phase of the outbreak, insufficient data may cause the estimation biased. Thus further work should be conducted based on more explicit data.
We carried out the sensitivity analysis of the peak time and peak size of the total number of infected and hospitalized individuals with respect to the model parameters. The result identified three key parameters: the contact rate c, the transmission probability β, and the progression rate from exposed to symptomatic σ, play an important role in both the peak time and the peak size. This suggested the vital role of quarantine and isolation in the early phase for this deadly infectious disease, besides its high infectivity and long incubation period. The finding also demonstrated that improving the detection rate and hospitalized rate δI, or improving the discharged rate γH can help reduce the severity of the outbreak significantly, or bring forward the peak time.
In addition, it is worth emphasizing that besides the above disease control parameters, media reports can also greatly affect the peak time and peak size. The peak size can be reduced greatly and the peak time can be brought forward by enhancing the response intensity of mass media to the newly confirmed cases η, weakening the waning rate of the awareness programs induced by media reports μM, increasing media-induced quarantined rate λM or raising the weight of public awareness to the media reports p. These results indicated that both enhancing the response rate of the media reporting to the severity of the outbreak, and enhancing the response rate of the public awareness to the media reports, can bring forward the peak time and reduce the peak size of the infection significantly, illustrating the important role of media reports on mitigating the spread of COVID-19 during the early phase of the outbreak.
We have to point out that the disease induced death rate (α=0.003) in our model represents the proportion of deaths to the infecteds per day, which is different from the case fatality rate (the ratio of accumulative deaths to the accumulative confirmed cases). And the disease induced deaths appeared not only in the confirmed cases (i.e., H class in our model) but also in the infecteds (i.e., I class in our model, people had been infected but not been confirmed). In more detail, the infection-fatality rate for infecteds is α/(α+δI+γI)=0.0068, and the hospitalization-fatality-rate for those confirmed cases is α/(α+γH)=0.0226, which is in a reasonable range of the fatality rate derived by the Novel Coronavirus Pneumonia Emergency Response Epidemiology Team [23]. The team have found that a total of 1023 deaths have occurred among 44, 672 confirmed cases for an overall case fatality of 2.3% by studing 72, 314 patient records. Another point we should note is that there were indeed more than 41 cases before Jan 10, 2020 [23]. The long detection period, the lack of public awareness of the virus and the untimely official reporting, may cause the reported confirmed cases lower than the true numbers at the beginning. Here we used the data collected from the National Health Commission of the People's Republic of China without retrospective studying (since in the early stage, data was insufficient). However, in our model fitting, the estimated total number of infectious persons (E+I) was more than 100 on Jan 10, 2020, which also indicated that the cases were more than that was reported. Furthermore, the testing capacity was low in the initial phase of the outbreak, and the detection technology was developing gradually. For example, according to Wuhan Municipal Health Commission [24], the samples could only be tested in the designated organization in Beijing which would take about 5 days to get the results before 16th January 2020, and about 200 samples could be tested in Hubei Provincial CDC every day from 16th to 22nd January. After 22nd January, about 2000 samples could be tested in Wuhan every day, and the capacity was improving gradually with more designated testing organizations. Thus it is more reasonable to incorporate a time varying detection rate in the model, which is a course for our further work.
During the raging of COVID-19 in China and other countries}, mass media plays an important role in curbing the transmission. The average daily number of media reporting, calculated in terms of the index 'hotness', the daily number of media items and daily number of IP visiting addresses, exhibited a strong correlation to the daily number of newly confirmed cases. Cross-correlation analysis further confirmed the interconnection between the case data and media data. In this work, we formulated a dynamic compartment model by setting media programs as a separate state variable to assess the impact of media on disease control. The good fitness of our targeted model to the data confirmed the model advising. The basic reproduction number was estimated as 5.3167, which demonstrated the highly transmissibility of COVID-19. Sensitivity analysis identified three key parameters: the contact rate, the transmission probability, and the progression rate from exposed to symptomatic, all of which had the greatest impact on peak time and peak size. It should be emphasized that the peak time can be brought forward and the peak size can be diminished significantly, if either the response rate of the media reporting to the severity of COVID-19 or the response rate of the public awareness to the media reports is improved. With developing of the testing technology, supply of the detection reagents and more information disclosure, the case detection, diagnosing and official reporting rate would be enhanced, the model should be adjusted and retrospective studies can be done. While in the initial stage of the outbreak, with insufficient resources and data, media coverage can be considered as an effective way to curb the spread of COVID-19.
This research was funded by the China Postdoctoral Science Foundation (grant number 2019M663611 (WZ)), and by the National Natural Science Foundation of China (grant numbers: 11801013(AW), 11631012 (YX, ST), 61772017(ST)).
The authors declare no conflict of interest.
[1] | Wuhan Municipal Health Commission, available from: http://wjw.wuhan.gov.cn/front/web/showDetail/2020010309017 (accessed on 8 February 2020). |
[2] | World Health Organization, available from: https://www.who.int/health-topics/coronavirus (accessed on 8 February 2020). |
[3] | P. Zhou, X. Yang, X. Wang, B. Hu, L. Zhang, W. Zhang, et al., Discovery of a novel coronavirus associated with the recent pneumonia outbreak in humans and its potential bat origin, BioRxiv, (2020). doi: 10.1101/2020.01.22.914952. |
[4] | C. Huang, Y. Wang, X. Li, L. Ren, J. Zhao, Y. Hu, et al., Clinical features of patients infected with 2019 novel coronavirus in Wuhan, China, Lancet, 395 (2020), 497-506. |
[5] | National Health Commission of the People's Republic of China, available from: http://www.nhc.gov.cn/xcs/yqtb/202002/6c305f6d70f545d59548ba17d79b8229.shtml (accessed on 8 February 2020). |
[6] | National Health Commission of the People's Republic of China, available from: http://www.nhc.gov.cn/xcs/zhengcwj/202002/18c1bb43965a4492907957875de02ae7.shtml (accessed on 8 February 2020). |
[7] | I. S. Kristiansen, P. A. Halvorsen, D. Gyrd-Hansen, Influenza pandemic: perception of risk and individual precautions in a general population, Cross sectional study, BMC Public Health, 7 (2007), 48. |
[8] | U. C. de Silva, J. Warachit, S. Waicharoen, M. Chittaganpitch, A preliminary analysis of the epidemiology of influenza A(H1N1) virus infection in Thailand from early outbreak data, June-July 2009, Euresurveilance, 14 (2009), 1-3. |
[9] | D. Roth, B. Henry, Social Distancing as a Pandemic Influenza Prevention Measure, National Collaborating Centre for Infectious Diseases, (2011). |
[10] | F. Huang, S. S. Zhou, S. S. Zhang, H. J. Wang, L. H. Tang, Temporal correlation analysis between malaria and meteorological factors in Motuo County, Tibet, Malaria J., 10 (2011), 54. |
[11] | G. E. Box, G. M. Jenkins, G. C. Reinsel, Time series analysis: forecasting and control, Forth edition, John Wiley & Sons, 2015. |
[12] | Health Commission of Hubei Province, available from: http://wjw.hubei.gov.cn/bmdt/ztzl/fkxxgzbdgrfyyq/ (accessed on 8 February 2020). |
[13] | B. Tang, X. Wang, Q. Li, N. L. Bragazzi, S. Tang, Y. Xiao, et al., Estimation of the transmission risk of the 2019-nCoV and its implication for public health interventions, J. Clin. Med., 9 (2020), 462. |
[14] | Chinese Center for Disease Control and Prevention. Report about 2019-nCoV, available from: http://www.chinacdc.cn/yyrdgz/202001/P020200128523354919292.pdf (accessed on 8 February 2020). |
[15] | M. W. Shen, Z. H. Peng, Y. N. Xiao, L. Zhang, Modelling the epidemic trend of the 2019 novel coronavirus outbreak in China, BioRxiv, (2020). |
[16] | Wuhan Municipal Health Commission, available from: http://wjw.wuhan.gov.cn/front/web/showDetail/2020011109035 (accessed on 8 February 2020). |
[17] | J. A. Backer, D. Klinkenberg, J. Wallinga, The incubation period of 2019-nCoV infections among travellers from Wuhan, China, MedRxiv, (2020). |
[18] | Q. Li, X. Guan, P. Wu, X. Wang, L. Zhou, Y. Tong, Early transmission dynamics in Wuhan, China, of novel coronavirus infected pneumonia, New Engl. J. Med., (2020). |
[19] | S. Zhao, S. Musa, Q. Lin, J. Ran, G. Yang, W. Wang, et al., Estimating the unreported number of novel coronavirus (2019-nCoV) vases in China in the first half of January 2020: a data-driven modelling analysis of the early outbreak, J. Clin. Med., 9 (2020), 388. |
[20] | J. Chan, S. Yuan, K. Kok, K. To, H. Chu, J. Yang, et al., A familial cluster of pneumonia associated with the 2019 novel coronavirus indicating person-to-person transmission: a study of a family cluster, Lancet, 395 (2020), 514-523. |
[21] | J. T. Wu, K. Leung, G. M. Leung, Nowcasting and forecasting the potential domestic and international spread of the 2019-nCoV outbreak originating in Wuhan, China: a modelling study, Lancet, 395 (2020), 689-697. |
[22] | Y. Liu, A. A. Gayle, A. Wilder-Smith, J. Rocklov, The reproductive number of COVID-19 is higher compared to SARS coronavirus, J. Travel. Med., (2020), 1-4. |
[23] | The Novel Coronavirus Pneumonia Emergency Response Epidemiology Team, Vital Surveillances: The Epidemiological Characteristics of an Outbreak of 2019 Novel Coronavirus Diseases (COVID-19)-China, 2020, China CDC weekly, 2 (2020), 113-122. |
[24] | Wuhan Municipal Health Commission, available from: http://wjw.wuhan.gov.cn/front/web/showDetail/2020012409132 (accessed on 4 March 2020). |
1. | Oberiri Destiny Apuke, Bahiyah Omar, Fake news and COVID-19: modelling the predictors of fake news sharing among social media users, 2021, 56, 07365853, 101475, 10.1016/j.tele.2020.101475 | |
2. | Ali Traoré, Fourtoua Victorien Konané, Modeling the effects of contact tracing on COVID-19 transmission, 2020, 2020, 1687-1847, 10.1186/s13662-020-02972-8 | |
3. | Piotr Staszkiewicz, Iwona Chomiak-Orsa, Igor Staszkiewicz, Dynamics of the COVID-19 Contagion and Mortality: Country Factors, Social Media, and Market Response Evidence From a Global Panel Analysis, 2020, 8, 2169-3536, 106009, 10.1109/ACCESS.2020.2999614 | |
4. | Ayse Konac, Yuksel Barut, 2021, chapter 26, 9781799868255, 433, 10.4018/978-1-7998-6825-5.ch026 | |
5. | Jing Ge, Daihai He, Zhigui Lin, Huaiping Zhu, Zian Zhuang, Four-tier response system and spatial propagation of COVID-19 in China by a network model, 2020, 330, 00255564, 108484, 10.1016/j.mbs.2020.108484 | |
6. | Chandan Maji, Mayer Humi, Impact of Media-Induced Fear on the Control of COVID-19 Outbreak: A Mathematical Study, 2021, 2021, 1687-9651, 1, 10.1155/2021/2129490 | |
7. | Mohammad Hussein, Eman Toraih, Rami Elshazli, Manal Fawzy, August Houghton, Danielle Tatum, Mary Killackey, Emad Kandil, Juan Duchesne, Meta-analysis on Serial Intervals and Reproductive Rates for SARS-CoV-2, 2021, 273, 0003-4932, 416, 10.1097/SLA.0000000000004400 | |
8. | Oberiri Destiny Apuke, Bahiyah Omar, How do Nigerian newspapers report COVID-19 pandemic? The implication for awareness and prevention, 2020, 35, 0268-1153, 471, 10.1093/her/cyaa031 | |
9. | Amparo Güemes, Soumyajit Ray, Khaled Aboumerhi, Michael R. Desjardins, Anton Kvit, Anne E. Corrigan, Brendan Fries, Timothy Shields, Robert D. Stevens, Frank C. Curriero, Ralph Etienne-Cummings, A syndromic surveillance tool to detect anomalous clusters of COVID-19 symptoms in the United States, 2021, 11, 2045-2322, 10.1038/s41598-021-84145-5 | |
10. | Rajanish Kumar Rai, Subhas Khajanchi, Pankaj Kumar Tiwari, Ezio Venturino, Arvind Kumar Misra, Impact of social media advertisements on the transmission dynamics of COVID-19 pandemic in India, 2021, 1598-5865, 10.1007/s12190-021-01507-y | |
11. | Justin Wong, Liling Chaw, Wee Chian Koh, Mohammad Fathi Alikhan, Sirajul Adli Jamaludin, Wan Wen Patricia Poh, Lin Naing, Epidemiological Investigation of the First 135 COVID-19 Cases in Brunei: Implications for Surveillance, Control, and Travel Restrictions, 2020, 103, 0002-9637, 1608, 10.4269/ajtmh.20-0771 | |
12. | Filip Raciborski, Mateusz Jankowski, Mariusz Gujski, Jarosław Pinkas, Piotr Samel-Kowalik, Artur Zaczyński, Igor Pańkowski, Kamil Rakocy, Waldemar Wierzba, Prevention of SARS-CoV-2 Infection Among Police Officers in Poland—Implications for Public Health Policies, 2020, 17, 1660-4601, 9072, 10.3390/ijerph17239072 | |
13. | Qianqian Cui, Zengyun Hu, Yingke Li, Junmei Han, Zhidong Teng, Jing Qian, Dynamic variations of the COVID-19 disease at different quarantine strategies in Wuhan and mainland China, 2020, 13, 18760341, 849, 10.1016/j.jiph.2020.05.014 | |
14. | Eladio J. Collado-Boira, Estefanía Ruiz-Palomino, Pablo Salas-Media, Ana Folch-Ayora, Maria Muriach, Pablo Baliño, “The COVID-19 outbreak”—An empirical phenomenological study on perceptions and psychosocial considerations surrounding the immediate incorporation of final-year Spanish nursing and medical students into the health system, 2020, 92, 02606917, 104504, 10.1016/j.nedt.2020.104504 | |
15. | V. S. Smirnov, Areg A. Totolian, Some opportunities for immunotherapy in coronavirus infection, 2020, 10, 2313-7398, 446, 10.15789/2220-7619-SPO-1470 | |
16. | L.H.A. Monteiro, V.C. Fanti, A.S. Tessaro, On the spread of SARS-CoV-2 under quarantine: A study based on probabilistic cellular automaton, 2020, 44, 1476945X, 100879, 10.1016/j.ecocom.2020.100879 | |
17. | L.H.A. Monteiro, An epidemiological model for SARS-CoV-2, 2020, 43, 1476945X, 100836, 10.1016/j.ecocom.2020.100836 | |
18. | Guo-Rong Xing, Ming-Tao Li, Li Li, Gui-Quan Sun, The Impact of Population Migration on the Spread of COVID-19: A Case Study of Guangdong Province and Hunan Province in China, 2020, 8, 2296-424X, 10.3389/fphy.2020.587483 | |
19. | Cheng-Cheng Zhu, Jiang Zhu, Dynamic analysis of a delayed COVID-19 epidemic with home quarantine in temporal-spatial heterogeneous via global exponential attractor method, 2021, 143, 09600779, 110546, 10.1016/j.chaos.2020.110546 | |
20. | Md. Arif Billah, Md. Mamun Miah, Md. Nuruzzaman Khan, Maria Elena Flacco, Reproductive number of coronavirus: A systematic review and meta-analysis based on global level evidence, 2020, 15, 1932-6203, e0242128, 10.1371/journal.pone.0242128 | |
21. | Lin Hu, Lin‐Fei Nie, Dynamic modeling and analysis of COVID‐19 in different transmission process and control strategies, 2021, 44, 0170-4214, 1409, 10.1002/mma.6839 | |
22. | Min Zhou, Piao Long, Nan Kong, Kathryn S. Campy, Characterizing Wuhan residents’ mask-wearing intention at early stages of the COVID-19 pandemic, 2020, 07383991, 10.1016/j.pec.2020.12.020 | |
23. | Seo Yoon Chae, KyoungEun Lee, Hyun Min Lee, Nam Jung, Quang Anh Le, Biseko Juma Mafwele, Tae Ho Lee, Doo Hwan Kim, Jae Woo Lee, Estimation of Infection Rate and Predictions of Disease Spreading Based on Initial Individuals Infected With COVID-19, 2020, 8, 2296-424X, 10.3389/fphy.2020.00311 | |
24. | Prasantha Bharathi Dhandapani, Dumitru Baleanu, Jayakumar Thippan, Vinoth Sivakumar, On stiff, fuzzy IRD-14 day average transmission model of COVID-19 pandemic disease, 2020, 7, 2375-1495, 208, 10.3934/bioeng.2020018 | |
25. | Oberiri Destiny Apuke, Bahiyah Omar, User motivation in fake news sharing during the COVID-19 pandemic: an application of the uses and gratification theory, 2020, 45, 1468-4527, 220, 10.1108/OIR-03-2020-0116 | |
26. | Hong Wu, Zhijian Zhang, Yabo Fang, Shaotang Zhang, Zuo Jiang, Jian Huang, Ping Li, Containment of rumor spread by selecting immune nodes in social networks, 2021, 18, 1551-0018, 2614, 10.3934/mbe.2021133 | |
27. | Akihiro Hisaka, Hideki Yoshioka, Hiroto Hatakeyama, Hiromi Sato, Yoshihiro Onouchi, Naohiko Anzai, Global Comparison of Changes in the Number of Test-Positive Cases and Deaths by Coronavirus Infection (COVID-19) in the World, 2020, 9, 2077-0383, 1904, 10.3390/jcm9061904 | |
28. | Csaba Farkas, David Iclanzan, Boróka Olteán-Péter, Géza Vekov, Estimation of parameters for a humidity-dependent compartmental model of the COVID-19 outbreak, 2021, 9, 2167-8359, e10790, 10.7717/peerj.10790 | |
29. | Zhiming Li, Zhidong Teng, Changxing Ma, 2019-nCoV Transmission in Hubei Province, China: Stochastic and Deterministic Analyses, 2020, 2020, 1076-2787, 1, 10.1155/2020/9012178 | |
30. | Nzaji Michel-Kabamba, Nlandu Ngatu, Ngombe Leon-Kabamba, Astrid Katumbo-Mukemo, Olivier Mukuku, Jean Ngoyi-Mukonkole, Guillaume Ngoie-Mwamba, Elie Kilolo-Ngoie, Ignace Bwana-Kangulu, Dora Kafusthi-Mukemo, Deca Banza-Ndala, Denis Kabila-Mutombo, Marie-Claire Balela-Kabasu, Moise Kanyiki-Katala, Al Syed-Mahfuz, Akitsu Murakami, Kanae Kanda, Yukinori Mashima, Numbi Oscar-Luboya, Tomohiro Hirao, Occupational COVID-19 Prevention among Congolese Healthcare Workers: Knowledge, Practices, PPE Compliance, and Safety Imperatives, 2020, 6, 2414-6366, 6, 10.3390/tropicalmed6010006 | |
31. | Gregory L. Watson, Di Xiong, Lu Zhang, Joseph A. Zoller, John Shamshoian, Phillip Sundin, Teresa Bufford, Anne W. Rimoin, Marc A. Suchard, Christina M. Ramirez, Virginia E. Pitzer, Pandemic velocity: Forecasting COVID-19 in the US with a machine learning & Bayesian time series compartmental model, 2021, 17, 1553-7358, e1008837, 10.1371/journal.pcbi.1008837 | |
32. | Richard B. Yapi, Clarisse A. Houngbedji, Daniel K.G. N’Guessan, Arlette O. Dindé, Aimé R. Sanhoun, Ariane Amin, Kossia D.T. Gboko, Kathrin Heitz-Tokpa, Gilbert Fokou, Bassirou Bonfoh, Knowledge, Attitudes, and Practices (KAP) Regarding the COVID-19 Outbreak in Côte d’Ivoire: Understanding the Non-Compliance of Populations with Non-Pharmaceutical Interventions, 2021, 18, 1660-4601, 4757, 10.3390/ijerph18094757 | |
33. | Deliana Kostova, Patricia Richter, Gretchen Van Vliet, Michael Mahar, Ronald L. Moolenaar, The Role of Noncommunicable Diseases in the Pursuit of Global Health Security, 2021, 2326-5094, 10.1089/hs.2020.0121 | |
34. | Cheng-Jun Yu, Zi-Xiao Wang, Yue Xu, Ming-Xia Hu, Kai Chen, Gang Qin, Assessment of basic reproductive number for COVID-19 at global level, 2021, 100, 0025-7974, e25837, 10.1097/MD.0000000000025837 | |
35. | Herman Wasserman, Wallace Chuma, Tanja Bosch, Chikezie E. Uzuegbunam, Rachel Flynn, South African newspaper coverage of COVID-19: A content analysis, 2021, 13, 2040-199X, 333, 10.1386/jams_00052_1 | |
36. | Li-Xiang Feng, Shuang-Lin Jing, Shi-Ke Hu, De-Fen Wang, Hai-Feng Huo, Modelling the effects of media coverage and quarantine on the COVID-19 infections in the UK, 2020, 17, 1551-0018, 3618, 10.3934/mbe.2020204 | |
37. | Ao Li, Yang Wang, Pingping Cong, Xingfu Zou, Re-examination of the impact of some non-pharmaceutical interventions and media coverage on the COVID-19 outbreak in Wuhan, 2021, 6, 24680427, 975, 10.1016/j.idm.2021.07.001 | |
38. | Xia Ma, Xiao-Feng Luo, Li Li, Yong Li, Gui-Quan Sun, The influence of mask use on the spread of COVID-19 during pandemic in New York City, 2022, 34, 22113797, 105224, 10.1016/j.rinp.2022.105224 | |
39. | Haifeng Li, Mo Hai, Wenxun Tang, Prior Knowledge-Based Causal Inference Algorithms and Their Applications for China COVID-19 Analysis, 2022, 10, 2227-7390, 3568, 10.3390/math10193568 | |
40. | James Georgalakis, Countdown to a Pandemic: BBC News representation of the response to COVID-19 as a global crisis approached, 2021, 1556-5068, 10.2139/ssrn.3931855 | |
41. | Brinda Sampat, Sahil Raj, Fake or real news? Understanding the gratifications and personality traits of individuals sharing fake news on social media platforms, 2022, 74, 2050-3806, 840, 10.1108/AJIM-08-2021-0232 | |
42. | Tao Zheng, Yantao Luo, Xinran Zhou, Long Zhang, Zhidong Teng, Spatial dynamic analysis for COVID-19 epidemic model with diffusion and Beddington-DeAngelis type incidence, 2023, 22, 1534-0392, 365, 10.3934/cpaa.2021154 | |
43. | Shaharior Rahman Razu, Nishana Afrin Nishu, Md. Fajlay Rabbi, Ashis Talukder, Paul R. Ward, Knowledge, Attitudes, and Practices Concerning COVID-19 in Bangladesh: A Qualitative Study of Patients With Chronic Illnesses, 2021, 9, 2296-2565, 10.3389/fpubh.2021.628623 | |
44. | Joanna Sooknanan, Terence A. R. Seemungal, FOMO (fate of online media only) in infectious disease modeling: a review of compartmental models, 2022, 2195-268X, 10.1007/s40435-022-00994-6 | |
45. | Bunmi O. Olatunji, Rebecca C. Cox, David A. Cole, Longitudinal trajectories of coronavirus anxiety and health behavior use before and after the U.S. 2020 presidential election: The effects of political orientation, 2022, 92, 08876185, 102643, 10.1016/j.janxdis.2022.102643 | |
46. | Edgar Steiger, Tobias Mussgnug, Lars Eric Kroll, Sungwoo Lim, Causal graph analysis of COVID-19 observational data in German districts reveals effects of determining factors on reported case numbers, 2021, 16, 1932-6203, e0237277, 10.1371/journal.pone.0237277 | |
47. | Temple Uwalaka, ‘Abba Kyari did not die of Coronavirus’: Social media and fake news during a global pandemic in Nigeria, 2022, 1329-878X, 1329878X2211012, 10.1177/1329878X221101216 | |
48. | Medhavi Gupta, Vikash Ranjan Keshri, Pompy Konwar, Katherine L Cox, , and Jagnoor Jagnoor, Media coverage of COVID-19 health information in India: a content analysis, 2022, 37, 1460-2245, 10.1093/heapro/daab116 | |
49. | Yang Liu, Yi Chen, Kefan Xie, Jia Liu, Effectiveness and policies analysis of pool testing method for COVID-19, 2023, 52, 0368-492X, 64, 10.1108/K-01-2021-0052 | |
50. | Omaji Samuel, Akogwu Blessing Omojo, Abdulkarim Musa Onuja, Yunisa Sunday, Prayag Tiwari, Deepak Gupta, Ghulam Hafeez, Adamu Sani Yahaya, Oluwaseun Jumoke Fatoba, Shahab Shamshirband, IoMT: A COVID-19 Healthcare System Driven by Federated Learning and Blockchain, 2023, 27, 2168-2194, 823, 10.1109/JBHI.2022.3143576 | |
51. | Ying-Qi Wang, Qing-Sheng Li, Xin-Qiang Zheng, Jian-Liang Lu, Yue-Rong Liang, Antiviral Effects of Green Tea EGCG and Its Potential Application against COVID-19, 2021, 26, 1420-3049, 3962, 10.3390/molecules26133962 | |
52. | Chao Zuo, Fenping Zhu, Yuting Ling, Analyzing COVID-19 Vaccination Behavior Using an SEIRM/V Epidemic Model With Awareness Decay, 2022, 10, 2296-2565, 10.3389/fpubh.2022.817749 | |
53. | İlkin Esen YILDIRIM, Deniz SEZGİN, COVID-19 Salgınında Türkiye’de Normalleşme Sürecine Geçiş: Ankara İli Örneğinde Toplumsal Bir Bakış, 2021, 4, 2667-4343, 92, 10.53048/johass.888234 | |
54. | Xinghua Chang, Jianrong Wang, Maoxing Liu, Zhen Jin, Dun Han, Study on an SIHRS Model of COVID-19 Pandemic With Impulse and Time Delay Under Media Coverage, 2021, 9, 2169-3536, 49387, 10.1109/ACCESS.2021.3064632 | |
55. | Chentong Li, Huan Lei, Zhanhu Hu, Xinwang Li, Junxiong Liang, Zhenyu Zhong, 2021, A Stochastic Model with Optimal Control Strategy of the Transmission of Covid-19, 978-1-6654-3531-4, 62, 10.1109/ICESIT53460.2021.9697050 | |
56. | Maheswari Rangasamy, Nazek Alessa, Prasantha Bharathi Dhandapani, Karuppusamy Loganathan, Dynamics of a Novel IVRD Pandemic Model of a Large Population over a Long Time with Efficient Numerical Methods, 2022, 14, 2073-8994, 1919, 10.3390/sym14091919 | |
57. | Anuj Kumar Gupta, Manvinder Sharma, Ankit Sharma, Vikas Menon, A Study on SARS-CoV-2 (COVID-19) and Machine Learning Based Approach to Detect COVID-19 Through X-Ray Images, 2022, 22, 0219-4678, 10.1142/S0219467821400106 | |
58. | Jinxing Guan, Yongyue Wei, Yang Zhao, Feng Chen, Modeling the transmission dynamics of COVID-19 epidemic: a systematic review, 2020, 34, 1674-8301, 422, 10.7555/JBR.34.20200119 | |
59. | Gürkan MURATDAĞI, Elif SARICA, Elif KÖSE, Serap UNGAN, Muhammet Raşit AYDIN, Abdülkadir AYDIN, Elif AKAR, Ahmet TÜRKMEN, Erkut ETÇİOĞLU, Emine Melike ARSLAN, Aslıhan ARIKAN, Ahmet ÖKSÜZ, Yıldız BÜYÜKDERELİ ATADAĞ, The evaluation of the news related to coronavirus in national media before and after the first declared case inTurkey, 2021, 1309-3878, 10.18521/ktd.885006 | |
60. | Tridip Sardar, Sk Shahid Nadim, Sourav Rana, Detection of multiple waves for COVID-19 and its optimal control through media awareness and vaccination: study based on some Indian states, 2023, 111, 0924-090X, 1903, 10.1007/s11071-022-07887-5 | |
61. | Yang Deng, Yi Zhao, Mathematical modeling for COVID-19 with focus on intervention strategies and cost-effectiveness analysis, 2022, 110, 0924-090X, 3893, 10.1007/s11071-022-07777-w | |
62. | Gloria C. Ugwu, Christian S. Ugwuanyi, Eucharia N. Aye, Celestine O. Eze, Nkiru Christiana Ohia, Emmanuel C. Okenyi, Theresa O. Oforka, Charles A. Oraelosi, Obiageli C. Njoku, Victor S. Ezema, Kelechi O. Ifekoya, Chinyere Theresa Nwoga, Alexander Okondugba, Julia Amobi Onumonu, Rosemary Chinyere Ohaneme, Malachy A. Ezeah, Validation of coronavirus-2019 phobia scale using preschool practitioners in urban and rural communities in Nigeria, 2022, 101, 0025-7974, e29209, 10.1097/MD.0000000000029209 | |
63. | Haiyin Li, Yan Wu, Toshikazu Kuniya, Dynamics of SCIR Modeling for COVID-19 with Immigration, 2022, 2022, 1099-0526, 1, 10.1155/2022/9182830 | |
64. | Ulva Noviana, M. Hasinuddin, M. Suhron, Sumarti Endah, Exploring Perception and Role of Nurses during COVID-19 Pandemic: Experiences of Frontline Workers in East Java, Indonesia, 2022, 18, 1675-8544, 72, 10.47836/mjmhs.18.6.11 | |
65. | Fang Keren, Ahmad Nabeel Siddiquei, Muhammad Azfar Anwar, Fahad Asmi, Qing Ye, What Explains Natives and Sojourners Preventive Health Behavior in a Pandemic: Role of Media and Scientific Self-Efficacy, 2021, 12, 1664-1078, 10.3389/fpsyg.2021.664399 | |
66. | Tingting Ding, Tongqian Zhang, Asymptotic behavior of the solutions for a stochastic SIRS model with information intervention, 2022, 19, 1551-0018, 6940, 10.3934/mbe.2022327 | |
67. | Maheswari Rangasamy, Christophe Chesneau, Carlos Martin-Barreiro, Víctor Leiva, On a Novel Dynamics of SEIR Epidemic Models with a Potential Application to COVID-19, 2022, 14, 2073-8994, 1436, 10.3390/sym14071436 | |
68. | Youming Guo, Tingting Li, Modeling the transmission of second‐wave COVID‐19 caused by imported cases: A case study, 2022, 45, 0170-4214, 8096, 10.1002/mma.8041 | |
69. | Deepika Varshney, Dinesh Kumar Vishwakarma, Framework for detection of probable clues to predict misleading information proliferated during COVID-19 outbreak, 2023, 35, 0941-0643, 5999, 10.1007/s00521-022-07938-3 | |
70. | Jinxing Guan, Yang Zhao, Yongyue Wei, Sipeng Shen, Dongfang You, Ruyang Zhang, Theis Lange, Feng Chen, Transmission dynamics model and the coronavirus disease 2019 epidemic: applications and challenges, 2022, 2, 2749-9642, 89, 10.1515/mr-2021-0022 | |
71. | Ráchael A. Powers, Kacy Bleeker, Crystal Alcalde, Anti-Asian Hate Crime in U.S. National News: A Content Analysis of Coverage and Narratives from 2010–2021, 2023, 13, 2153-3687, 32, 10.1177/21533687221120949 | |
72. | Xiunan Wang, Hao Wang, Pouria Ramazi, Kyeongah Nah, Mark Lewis, A Hypothesis-Free Bridging of Disease Dynamics and Non-pharmaceutical Policies, 2022, 84, 0092-8240, 10.1007/s11538-022-01012-8 | |
73. | Shanlang Lin, Chao Ma, Ruofei Lin, Research on the Influence of Information Diffusion on the Transmission of the Novel Coronavirus (COVID-19), 2022, 19, 1660-4601, 6801, 10.3390/ijerph19116801 | |
74. | Jyu-Lin Chen, Chen-Xi Lin, Mijung Park, Jerry John Nutor, Rosalind de Lisser, Thomas J. Hoffmann, Hannah J. Kim, Rapid response nursing triage outcomes for COVID-19: factors associated with patient’s participation in triage recommendations, 2023, 23, 1472-6947, 10.1186/s12911-023-02139-x | |
75. | Tangjuan Li, Yanni Xiao, Complex dynamics of an epidemic model with saturated media coverage and recovery, 2022, 107, 0924-090X, 2995, 10.1007/s11071-021-07096-6 | |
76. | Hao Wang, Di Zhu, Shiqi Li, Robert A. Cheke, Sanyi Tang, Weike Zhou, Home quarantine or centralized quarantine? A mathematical modelling study on the COVID-19 epidemic in Guangzhou in 2021, 2022, 19, 1551-0018, 9060, 10.3934/mbe.2022421 | |
77. | Yan Wang, Feng Qing, Haozhan Li, Xuteng Wang, Timely and effective media coverage's role in the spread of Corona Virus Disease 2019, 2022, 0170-4214, 10.1002/mma.8732 | |
78. | Suihong Lan, Jiaxiang Li, Chao Zhao, 2022, Chapter 18, 978-3-031-06049-6, 241, 10.1007/978-3-031-06050-2_18 | |
79. | Michał Górski, Marta Buczkowska, Mateusz Grajek, Jagoda Garbicz, Beata Całyniuk, Kamila Paciorek, Aleksandra Głuszek, Renata Polaniak, Assessment of the Risk of Depression in Residents Staying at Long-Term Care Institutions in Poland During the COVID-19 Pandemic Depending on the Quality of Cognitive Functioning, 2022, 12, 1664-1078, 10.3389/fpsyg.2021.766675 | |
80. | Shuang Zhang, Peng Jing, Daibiao Yuan, Chenlu Yang, On parents' choice of the school travel mode during the COVID-19 pandemic, 2022, 19, 1551-0018, 9412, 10.3934/mbe.2022438 | |
81. | Felix Olajide Talabi, Ikechukwu Peter Ugbor, Moyinoluwa Joseph Talabi, Joel C Ugwuoke, David Oloyede, Ayodeji Boluwatife Aiyesimoju, Amaka B Ikechukwu-Ilomuanya, Effect of a social media-based counselling intervention in countering fake news on COVID-19 vaccine in Nigeria, 2022, 37, 1460-2245, 10.1093/heapro/daab140 | |
82. | Shraddha Ramdas Bandekar, Tanuja Das, Akhil Kumar Srivastav, Anuradha Yadav, Anuj Kumar, Prashant K Srivastava, Mini Ghosh, Modeling and prediction of the third wave of COVID-19 spread in India, 2022, 10, 2544-7297, 231, 10.1515/cmb-2022-0138 | |
83. | Xinghua Chang, Maoxing Liu, Zhen Jin, Jianrong Wang, Studying on the impact of media coverage on the spread of COVID-19 in Hubei Province, China, 2020, 17, 1551-0018, 3147, 10.3934/mbe.2020178 | |
84. | Xinjie Fu, JinRong Wang, Dynamic stability and optimal control of SISqIqRS epidemic network, 2022, 163, 09600779, 112562, 10.1016/j.chaos.2022.112562 | |
85. | Temple Uwalaka, Bigman Nwala, Amadi Confidence Chinedu, Social media, fake news and fake COVID-19 cures in Nigeria, 2021, 13, 2040-199X, 435, 10.1386/jams_00058_1 | |
86. | Yinjiao Gong, Aili Wang, Jin Guo, Stacey R. Smith?, Jorge E. Macias-Diaz, Modelling the Impact of Media-Induced Social Distancing on the Containment of COVID-19 in Beijing, 2022, 2022, 1607-887X, 1, 10.1155/2022/3954598 | |
87. | Sangeeta Saha, Protyusha Dutta, Guruprasad Samanta, Dynamical behavior of SIRS model incorporating government action and public response in presence of deterministic and fluctuating environments, 2022, 164, 09600779, 112643, 10.1016/j.chaos.2022.112643 | |
88. | Biao Tang, Weike Zhou, Xia Wang, Hulin Wu, Yanni Xiao, Controlling Multiple COVID-19 Epidemic Waves: An Insight from a Multi-scale Model Linking the Behaviour Change Dynamics to the Disease Transmission Dynamics, 2022, 84, 0092-8240, 10.1007/s11538-022-01061-z | |
89. | Tangjuan Li, Yanni Xiao, Linking the disease transmission to information dissemination dynamics: An insight from a multi-scale model study, 2021, 526, 00225193, 110796, 10.1016/j.jtbi.2021.110796 | |
90. | Mengyao Ren, Sangwon Park, Yang Xu, Xiao Huang, Lei Zou, Man Sing Wong, Sun-Young Koh, Impact of the COVID-19 pandemic on travel behavior: A case study of domestic inbound travelers in Jeju, Korea, 2022, 92, 02615177, 104533, 10.1016/j.tourman.2022.104533 | |
91. | Qing Ye, Rongting Zhou, Fahad Asmi, Evaluating the Impact of the Pandemic Crisis on the Aviation Industry, 2022, 0361-1981, 036119812211257, 10.1177/03611981221125741 | |
92. | Glenn Marion, Liza Hadley, Valerie Isham, Denis Mollison, Jasmina Panovska-Griffiths, Lorenzo Pellis, Gianpaolo Scalia Tomba, Francesca Scarabel, Ben Swallow, Pieter Trapman, Daniel Villela, Modelling: Understanding pandemics and how to control them, 2022, 39, 17554365, 100588, 10.1016/j.epidem.2022.100588 | |
93. | Qiaojuan Jia, Yafei Lou, Feilong Rong, Shuai Zhang, Minghua Wang, Linghao He, Zhihong Zhang, Miao Du, Silver nanoparticle embedded polymer–zirconium-based metal–organic framework (polyUiO-66) for electrochemical biosensors of respiratory viruses, 2021, 9, 2050-7526, 14190, 10.1039/D1TC03100G | |
94. | Asma Alwreikat, Sharing of Misinformation during COVID-19 Pandemic: Applying the Theory of Planned Behavior with the Integration of Perceived Severity, 2022, 41, 0194-262X, 133, 10.1080/0194262X.2021.1960241 | |
95. | Seon-Woo Kim, Martina Santia, Raymond J. Pingree, Ayla Oden, Kirill Bryanov, Jessica Wyers, Natalie J. Shook, The curve not taken: Effects of COVID-19 international comparison news, 2022, 17, 1932-6203, e0271041, 10.1371/journal.pone.0271041 | |
96. | Ousmane Koutou, Abou Bakari Diabaté, Boureima Sangaré, Mathematical analysis of the impact of the media coverage in mitigating the outbreak of COVID-19, 2023, 205, 03784754, 600, 10.1016/j.matcom.2022.10.017 | |
97. | Jin Guo, Aili Wang, Weike Zhou, Yinjiao Gong, Stacey R. Smith?, Discrete epidemic modelling of COVID-19 transmission in Shaanxi Province with media reporting and imported cases, 2021, 19, 1551-0018, 1388, 10.3934/mbe.2022064 | |
98. | Suleyman Utku UZUN, Ozgur SEVINC, Ahmet ERGIN, Prevalence of correct face mask usage among general public during COVID-19 pandemic in Denizli, Turkey, 2022, 1019-1941, 10.5472/marumj.1191644 | |
99. | Olusegun Emmanuel Akinwale, Uchechi C. Onokala, 2022, 978-1-80117-903-4, 53, 10.1108/978-1-80117-902-720221005 | |
100. | Juan Mejía-Trejo, Ismael Loza-Vega, Social Media Information Literacy vs. Fake News: Probing the Business Decisions under COVID-19 times as Innovation skills with fsQCA, 2021, 1, 27, 10.55965/setp.1.02.a2 | |
101. | Ishita Vohra, Meher Shashwat Nigam, Aryan Sakaria, Amey Kudari, Nimmi Rangaswamy, 2022, Is Twitter Enough? Investigating Situational Awareness in Social and Print Media during the Second COVID-19 Wave in India, 978-1-6654-5661-6, 339, 10.1109/ASONAM55673.2022.10068667 | |
102. | Himanshu Grover, Public Risk Perception of Covid Transmission and Support for Smart Growth Policies in Seattle Metro Region, 2022, 1556-5068, 10.2139/ssrn.4122786 | |
103. | Elena E. Popadyuk, Tatyana E. Sizikova, Aleksey L. Khmelev, Mikhail A. Timofeev, Vitaliy N. Lebedev, Sergey V. Borisevich, The use of immunoglobulins and monoclonal antibodies against COVID-19, 2024, 69, 2411-2097, 119, 10.36233/0507-4088-225 | |
104. | S. Sankaranarayanan, An approach to statistical analysis-using the average transmission model of Covid 19, 2020, 8, 23193786, 2158, 10.26637/MJM0804/0141 | |
105. | Corlia Meyer, François van Schalkwyk, Framing Covid-19 in the South African News Media: An Analysis of 22 Months of Reporting, 2024, 25, 1461-670X, 480, 10.1080/1461670X.2023.2232473 | |
106. | Anna D. Fome, Herieth Rwezaura, Mamadou L. Diagne, Shannon Collinson, Jean M. Tchuenche, A deterministic Susceptible–Infected–Recovered model for studying the impact of media on epidemic dynamics, 2023, 3, 27724425, 100189, 10.1016/j.health.2023.100189 | |
107. | Shimli Dutta, Protyusha Dutta, Guruprasad Samanta, Modelling disease transmission through asymptomatic carriers: a societal and environmental perspective, 2024, 12, 2195-268X, 3100, 10.1007/s40435-024-01387-7 | |
108. | Ann Osi, Navid Ghaffarzadegan, Claudio José Struchiner, Parameter estimation in behavioral epidemic models with endogenous societal risk-response, 2024, 20, 1553-7358, e1011992, 10.1371/journal.pcbi.1011992 | |
109. | Aliasghar Kheirkhah Vakiabad, Ehsan Movahed, Fereshte Gheybi, Fatemeh Kave Farsani, Fatemeh Rigabadi, Is Strengths, Weaknesses, Opportunities, and Threats Analysis Effective in Preventing and Controlling Coronavirus Disease-19? A qualitative study, 2024, 13, 2645-6109, 131, 10.34172/jqr.2024.19 | |
110. | Yunbo Tu, Xinzhu Meng, A reaction–diffusion epidemic model with virus mutation and media coverage: Theoretical analysis and numerical simulation, 2023, 214, 03784754, 28, 10.1016/j.matcom.2023.06.023 | |
111. | Xueli Wang, Suxia Zhang, Coupling media coverage and susceptibility for modeling epidemic dynamics: An application to COVID-19, 2024, 217, 03784754, 374, 10.1016/j.matcom.2023.10.026 | |
112. | Rebecca E. Fuoco, Carol F. Kwiatkowski, Linda S. Birnbaum, Arlene Blum, Effective communications strategies to increase the impact of environmental health research, 2023, 22, 1476-069X, 10.1186/s12940-023-00997-6 | |
113. | 秀 秦, Dynamic Analysis of SEIRS-M Epidemic Mod-el with Media Effect Delay, 2023, 12, 2324-7991, 4338, 10.12677/AAM.2023.1210427 | |
114. | Jacques Demongeot, Pierre Magal, Data-driven mathematical modeling approaches for COVID-19: A survey, 2024, 50, 15710645, 166, 10.1016/j.plrev.2024.08.004 | |
115. | Darla Spence Coffey, Deana F. Morrow, 2024, Chapter 12, 978-3-031-66558-5, 159, 10.1007/978-3-031-66559-2_12 | |
116. | Weiyuan Ma, Nuri Ma, Changping Dai, YangQuan Chen, Xinwei Wang, Fractional modeling and optimal control strategies for mutated COVID‐19 pandemic, 2023, 0170-4214, 10.1002/mma.9313 | |
117. | Kangguo Li, Jiayi Wang, Jiayuan Xie, Jia Rui, Buasiyamu Abudunaibi, Hongjie Wei, Hong Liu, Shuo Zhang, Qun Li, Yan Niu, Tianmu Chen, Advancements in Defining and Estimating the Reproduction Number in Infectious Disease Epidemiology, 2023, 5, 2097-3101, 829, 10.46234/ccdcw2023.158 | |
118. | Lamia Alyami, Saptarshi Das, Stuart Townley, Charin Modchang, Bayesian model selection for COVID-19 pandemic state estimation using extended Kalman filters: Case study for Saudi Arabia, 2024, 4, 2767-3375, e0003467, 10.1371/journal.pgph.0003467 | |
119. | Makayla Preston, Alexandria Carter, Eric Numfor, Modeling the Effects of Media Awareness on SARS-CoV-2 Transmission in Georgia, 2024, 10, 2349-5103, 10.1007/s40819-024-01759-9 | |
120. | Oberiri Destiny Apuke, Bahiyah Omar, Television News Coverage of COVID-19 Pandemic in Nigeria: Missed Opportunities to Promote Health Due to Ownership and Politics, 2021, 11, 2158-2440, 10.1177/21582440211032675 | |
121. | Sonu Lamba, Prashant K. Srivastava, Cost-effective optimal control analysis of a COVID-19 transmission model incorporating community awareness and waning immunity, 2023, 11, 2544-7297, 10.1515/cmb-2023-0154 | |
122. | Arpita Devi, Praveen Kumar Gupta, Impact of media information and reinfection in a COVID-19 dynamical model: emergence of periodic orbits through Hopf bifurcation, 2024, 99, 0031-8949, 075207, 10.1088/1402-4896/ad4f2e | |
123. | Verónica Israel-Turim, Valentina Laferrara, Ana Regina Rego, Josep Lluís Micó-Sanz, Misinformation about the COVID-19 Vaccine in Online Catholic Media, 2023, 11, 2076-393X, 1054, 10.3390/vaccines11061054 | |
124. | Dandan Sun, Wan-Tong Li, Ming-Zhen Xin, Dynamics of an age-structured SIS epidemic model with local dispersal and general incidence functions, 2025, 140, 10075704, 108364, 10.1016/j.cnsns.2024.108364 | |
125. | Naba Kumar Goswami, Samson Olaniyi, Sulaimon F. Abimbade, Furaha M. Chuma, A mathematical model for investigating the effect of media awareness programs on the spread of COVID-19 with optimal control, 2024, 5, 27724425, 100300, 10.1016/j.health.2024.100300 | |
126. | Ruijie Shu, Hua Xia, Oberiri Destiny Apuke, The Mechanism Through Which Corporate Social Responsibility (CSR) Affects Customer Patronage of Manufacturing Companies: Modeling the Mediating Role of Corporate Reputation and the Moderating Role of Customer Awareness of CSR Activities, 2024, 14, 2158-2440, 10.1177/21582440241305320 | |
127. | Shardul Shankar, Vijayshri Tewari, Shashwat Shankar, Measuring Emotional Wellbeing and Emotional Contagion Through Sentiments and Emotions Evoked by Social Media for COVID-19, 2024, 14, 2158-2440, 10.1177/21582440241285771 | |
128. | Leah LeJeune, Navid Ghaffarzadegan, Lauren M. Childs, Omar Saucedo, Formulating human risk response in epidemic models: Exogenous vs endogenous approaches, 2025, 03772217, 10.1016/j.ejor.2025.01.004 | |
129. | Guowei Sun, Ali Mai, Zhen Jin, Modeling precaution, immunity loss and dispersal on disease dynamics: a two-patch SIRS model, 2025, 2025, 2731-4235, 10.1186/s13662-024-03862-z | |
130. | Fahad Al Basir, Kottakkaran Sooppy Nisar, Ibraheem M. Alsulami, Amar Nath Chatterjee, Dynamics and optimal control of an extended SIQR model with protected human class and public awareness, 2025, 140, 2190-5444, 10.1140/epjp/s13360-025-06108-3 | |
131. | Xiaojun Ding, Bingxing Shang, Caifeng Xie, Jiayi Xin, Feng Yu, Artificial intelligence in the COVID-19 pandemic: balancing benefits and ethical challenges in China’s response, 2025, 12, 2662-9992, 10.1057/s41599-025-04564-x | |
132. | Arif Satriantoro, Mohamad Saifudin Mohamad Saleh, A content analysis of COVID-19 news coverage of The Jakarta Post , The Star , and Bangkok Post , 2025, 0739-5329, 10.1177/30497841251325811 | |
133. | Vasiliy Osipov, Marina Osipova, Sergey Kuleshov, Alexandra Zaytseva, Aleksey Aksenov, Epidemiological Informing of the Population in Cities: Models and Their Application, 2022, 16, 2500-2597, 80, 10.17323/2500-2597.2022.2.80.89 | |
134. | Xinghua Chang, Jianrong Wang, Maoxing Liu, Xue Yan, An SIS infectious disease model with nonlinear incidence and disease awareness on complex networks, 2025, 196, 09600779, 116349, 10.1016/j.chaos.2025.116349 |
Variables | Description | initial value | Resource |
S | Susceptible population | 11081000 | [12, 13] |
E | Exposed population | 118.552 | LS |
I | Infected population with symptomatic | 20 | LS |
Sq | Quarantined susceptible population | 739 | [16] |
Eq | isolated exposed population | 1 | LS |
H | Hospitalized population | 1 | [13] |
R | Recovered population | 2 | [16] |
M | Media items | 16.3 | Data |
Parameters | Description | Value | Resource |
c | Contact rate (per person per day) | 10.582 | LS |
β | Probability of transmission from I to S per contact | 2.010×10−8 | LS |
θ | Relative transmission probability of E compared with I | 1.000×10−6 | LS |
q | Quarantined proportion of latent individuals | 1.000×10−8 | LS |
p | Weight of media effect on contact rate sensitive to media items | 9.003×10−5 | LS |
λM | Quarantined rate of individuals induced by media reporting (per day) | 5.471×10−5 | LS |
λ | Releasing rate of quarantined individuals (per day) | 1/14 | [13] |
σ | Progression rate of exposed individuals to infectives (per day) | 1/5 | [14,17,18] |
α | Disease-induced death rate (per day) | 0.003 | LS |
δI | Progression rate of infectives to hospitalized individuals (per day) | 0.110 | LS |
γI | Recovery rate of infected individuals (per day) | 0.330 | [13] |
δq | Progression rate of quarantined exposed to hospitalized class (per day) | 0.102 | LS |
γH | Recovery rate of hospitalized individuals (per day) | 0.130 | Data |
η | Media reporting rate of number of newly hospital notifications (per person) | 2.951 | LS |
μM | Media waning rate (per day) | 0.735 | LS |
Variables | Description | initial value | Resource |
S | Susceptible population | 11081000 | [12, 13] |
E | Exposed population | 118.552 | LS |
I | Infected population with symptomatic | 20 | LS |
Sq | Quarantined susceptible population | 739 | [16] |
Eq | isolated exposed population | 1 | LS |
H | Hospitalized population | 1 | [13] |
R | Recovered population | 2 | [16] |
M | Media items | 16.3 | Data |
Parameters | Description | Value | Resource |
c | Contact rate (per person per day) | 10.582 | LS |
β | Probability of transmission from I to S per contact | 2.010×10−8 | LS |
θ | Relative transmission probability of E compared with I | 1.000×10−6 | LS |
q | Quarantined proportion of latent individuals | 1.000×10−8 | LS |
p | Weight of media effect on contact rate sensitive to media items | 9.003×10−5 | LS |
λM | Quarantined rate of individuals induced by media reporting (per day) | 5.471×10−5 | LS |
λ | Releasing rate of quarantined individuals (per day) | 1/14 | [13] |
σ | Progression rate of exposed individuals to infectives (per day) | 1/5 | [14,17,18] |
α | Disease-induced death rate (per day) | 0.003 | LS |
δI | Progression rate of infectives to hospitalized individuals (per day) | 0.110 | LS |
γI | Recovery rate of infected individuals (per day) | 0.330 | [13] |
δq | Progression rate of quarantined exposed to hospitalized class (per day) | 0.102 | LS |
γH | Recovery rate of hospitalized individuals (per day) | 0.130 | Data |
η | Media reporting rate of number of newly hospital notifications (per person) | 2.951 | LS |
μM | Media waning rate (per day) | 0.735 | LS |