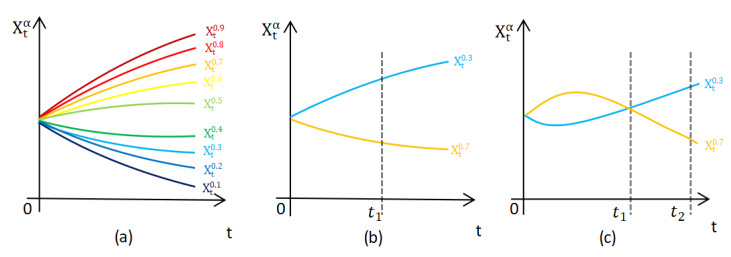
In this paper, we study the higher-order uncertain differential equations (UDEs) as defined by Kaixi Zhang [
Citation: Zeman Wang, Zhong Liu, Zikun Han, Xiuying Guo, Qiubao Wang. The inverse uncertainty distribution of the solutions to a class of higher-order uncertain differential equations[J]. AIMS Mathematics, 2024, 9(11): 33023-33061. doi: 10.3934/math.20241579
[1] | Qinyun Lu, Ya Li, Hai Zhang, Hongmei Zhang . Uncertainty distributions of solutions to nabla Caputo uncertain difference equations and application to a logistic model. AIMS Mathematics, 2024, 9(9): 23752-23769. doi: 10.3934/math.20241154 |
[2] | Nana Tao, Chunxiao Ding . Global attractivity for uncertain differential systems. AIMS Mathematics, 2022, 7(2): 2142-2159. doi: 10.3934/math.2022122 |
[3] | Guiwen Lv, Ping Xu, Yanxue Zhang . Pricing of vulnerable options based on an uncertain CIR interest rate model. AIMS Mathematics, 2023, 8(5): 11113-11130. doi: 10.3934/math.2023563 |
[4] | Zhifu Jia, Xinsheng Liu . New stability theorems of uncertain differential equations with time-dependent delay. AIMS Mathematics, 2021, 6(1): 623-642. doi: 10.3934/math.2021038 |
[5] | Xiaodie Lv, Yi Liu, Yihua Zhong . A novel method to solve the optimization problem of uncertain network system based on uncertainty theory. AIMS Mathematics, 2023, 8(3): 5445-5461. doi: 10.3934/math.2023274 |
[6] | Lin Fan, Shunchu Li, Dongfeng Shao, Xueqian Fu, Pan Liu, Qinmin Gui . Elastic transformation method for solving the initial value problem of variable coefficient nonlinear ordinary differential equations. AIMS Mathematics, 2022, 7(7): 11972-11991. doi: 10.3934/math.2022667 |
[7] | Feng Qi, Da-Wei Niu, Bai-Ni Guo . Simplifying coefficients in differential equations associated with higher order Bernoulli numbers of the second kind. AIMS Mathematics, 2019, 4(2): 170-175. doi: 10.3934/math.2019.2.170 |
[8] | Guijun Xing, Huatao Chen, Zahra S. Aghayan, Jingfei Jiang, Juan L. G. Guirao . Tracking control for a class of fractional order uncertain systems with time-delay based on composite nonlinear feedback control. AIMS Mathematics, 2024, 9(5): 13058-13076. doi: 10.3934/math.2024637 |
[9] | Shuai Huang, Youwu Lin, Jing Zhang, Pei Wang . Chance-constrained approach for decentralized supply chain network under uncertain cost. AIMS Mathematics, 2023, 8(5): 12217-12238. doi: 10.3934/math.2023616 |
[10] | Muhammad Akram, Ghulam Muhammad, Tofigh Allahviranloo, Ghada Ali . New analysis of fuzzy fractional Langevin differential equations in Caputo's derivative sense. AIMS Mathematics, 2022, 7(10): 18467-18496. doi: 10.3934/math.20221016 |
In this paper, we study the higher-order uncertain differential equations (UDEs) as defined by Kaixi Zhang [
Events with known frequencies of occurrence are classified as random, while those with unknown frequencies are termed uncertain [1]. With the rapid advancement of science and technology, a multitude of uncertain factors have emerged in real life, rendering the phenomena of uncertainty in the objective world undeniable. Consequently, scholars have begun to incorporate these uncertain factors into the establishment of mathematical models, leading to the research and development of uncertainty theory.
Integrating uncertain factors into differential equations results in the formation of UDEs, a type of differential equation established by Liu in 2007 [1], designed to describe the dynamics of uncertain phenomena. Yao and Chen provided an effective formula for calculating the inverse uncertainty distribution of the solutions to UDEs, known as the Yao-Chen formula [2].
The Yao-Chen formula yields a family of solutions to ordinary differential equations, denoted as α-paths, and it has been indicated [2] that these α-paths represent the inverse uncertainty distribution of the solutions. Therefore, to determine the inverse uncertainty distribution of the solution, we need to solve a family of ordinary differential equations to obtain the α-paths. For first-order scalar UDE, the inverse uncertainty distribution of the solutions can be determined using the Yao-Chen formula. Building on this foundation, research has been conducted in many fields, including finance [3], optimal control [4], population growth [5], pharmacokinetics [6,7], epidemiology [8], and heat conduction [9,10]. However, in many practical contexts, first-order scalar UDEs may not fully capture the complexity of real-world scenarios. Often, more intricate cases emerge, such as higher-order, fractional-order, functional differential equations, etc. Thus, investigating theories concerning those UDEs is of significant importance. This paper primarily focuses on the inverse uncertainty distribution problem of a class of higher-order UDEs.
Zhang [11] has rigorously defined the concept of multiple integrals and higher-order derivatives of uncertain processes, establishing the framework for higher-order UDEs. These contributions have addressed previous theoretical deficiencies in higher-order UDEs and provided new analytical methods for solving more complex uncertain system problems. However, the definition of the α-path, which is crucial for determining the inverse uncertainty distribution of UDEs' solutions, was not provided. Therefore, in this article, we define the α-path for second-order UDEs and a class of higher-order UDEs, and determine the corresponding inverse uncertainty distributions for the solutions of these types of UDEs. This will enable a more comprehensive understanding and analysis of higher-order uncertain processes, offering new perspectives and tools for the theoretical development and practical application of uncertain calculus.
To determine the inverse uncertainty distribution of the solutions to UDEs, it is essential to identify the α-paths. The formation of α-paths must satisfy fundamental conditions, primarily concerning the monotonicity with respect to α. That is, at any given time t, the value of Xαt on an α-path should monotonically increase with respect to α, as illustrated in Figure 1(a). It is impermissible to encounter a scenario as depicted in Figure 1(c) and Figure 2(b). The main focus of this paper is to study under what conditions the α-paths of UDEs behave like the one in Figure 1(a), rather than exhibiting the situations shown in (b) and (c).
The rest of this paper is organized as follows: In Section 2, we review related concepts and conclusions of uncertainty theory. In Section 3, we define the α-paths for some second-order UDEs and a class of higher-order UDEs, deriving the inverse uncertainty distribution corresponding to the solutions. In Section 4, we provide positive and negative instances of the theorem in Section 3. In Section 5, we give a brief summary of this paper. Figure 2 illustrates the conceptual framework of this manuscript, encapsulating the core ideas and interconnections presented in the paper.
In this section, we introduce some basic concepts and theorems about uncertain processes and uncertain calculus.
Definition 2.1 (Liu [12]) Let Γ be a non-empty set, let Λ be a σ-algebra over Γ, and let M be an uncertain measure. Then the triplet (Γk,Lk,Mk) is called an uncertainty space.
Theorem 2.1. [13] (Measure Inversion Theorem) Let ξ be an uncertain variable with uncertainty distribution Φ. Then for any real number x, we have
M{ξ≤x}=Φ(x),M{ξ>x}=1−Φ(x). |
Definition 2.2 (Liu [14]) An uncertain process Ct is said to be a Liu process if
(i) C0=0 and almost all sample paths are Lipschitz continuous,
(ii) Ct has stationary and independent increments,
(iii) every increment Cs+t-Cs is a normal uncertain variable with an expected value of 0 and variance t2.
Definition 2.3 (Yao-Chen[2]) Let α be a number between 0 and 1. An uncertain differential equation
dXt=f(t,Xt)dt+g(t,Xt)dCt |
is said to have an α-path Xαt if it solves the corresponding ordinary differential equation
dXαt=f(t,Xαt)dt+|g(t,Xαt)|Φ−1(α)dt |
where Φ−1(α) is the inverse standard normal uncertainty distribution, i.e.,
Φ−1(α)=√3πlnα1−α. |
Definition 2.4 (Liu[12]) Suppose f and g are continuous functions. An uncertain differential equation
dXt=f(t,Xt)dt+g(t,Xt)dCt |
is said to satisfy the regular condition if
g(t,Xt)>0,∀t≥0. |
Theorem 2.2. (Liu[12]) The uncertain differential equation
dXt=f(t,Xt)dt+g(t,Xt)dCt |
has a unique solution if the functions f(t,x) and g(t,x) satisfy the linear growth condition
|f(t,x)|+|g(t,x)|≤L(1+|x|),∀x∈ℜ,t≥0 |
and the Lipschitz condition
|f(t,x)−f(t,y)|+|g(t,x)−g(t,y)|≤L(|x−y|),∀x,y∈ℜ,t≥0. |
Without loss of generality, suppose L. Moreover, the solution is sample continuous.
Theorem 2.3. (Liu[12]) Let Xαt be the α-path of the regular uncertain differential equation
dXt=f(t,Xt)dt+g(t,Xt)dCt. |
If the linear growth, Lipschitz, and regular conditions hold, then Xαt is a strictly increasing function with respect to α at each time t>0.
Theorem 2.4. (Liu[12]) Let Xαt be the α-path of the regular uncertain differential equation
dXt=f(t,Xt)dt+g(t,Xt)dCt. |
Then Xαt is a continuous function with respect to α at each time t>0.
Theorem 2.5. (Yao-Chen formula [2]) Let Xt and Xαt be the solution and α-path of the regular uncertain differential equation
dXt=f(t,Xt)dt+g(t,Xt)dCt, |
respectively. Then
M{Xt≤Xαt,∀t}=α, |
M{Xt>Xαt,∀t}=1−α. |
Definition 2.5. (Liu [14]; Chen and Ralescu[15]; Ye[16]) Let Ct be a Liu process, and let Zt be an uncertain process. If there exist two sample-continuous uncertain processes μt and δt such that
Zt=Z0+∫t0μsds+∫t0δsdCs, |
for any t≥0, then Zt is called a general Liu process with drift μt and diffusion δt. Furthermore, Zt has an uncertain differential
dZt=μtdt+δtdCt, |
and a first-order derivative
˙Zt=μt+δt˙Ct, |
where ˙Ct is the formal derivative dCt/dt.
Definition 2.6 (Zhang [11]) Let Ct be a Liu process, and let Zt be an uncertain process. If there exist sample-continuous uncertain processes μt and δt such that
Zt=Z0+˙Z0t+∫t0∫s0μrdrds+∫t0∫s0δrdCrds |
for any t≥0, then Zt is called a second-order Liu process and has a second-order derivative
¨Zt=μt+δt˙Ct, |
where ¨Zt is the formal second-order derivative d2Zt/dt2.
Theorem 2.6. (Zhang [11]) Let f(t,s) be an uncertain field, and let Xt and Ys be general Liu processes. For any partition of the closed region [0,a]×[0,b] with
0=t1<t2<⋯<tn+1=a,0=s1<s2<⋯<sm+1=b, |
the mesh is written as
Δ=max1≤i≤n1≤j≤m √(ti+1−ti)2+(si+1−si)2. |
Then the double Liu integral of f(t,s) with respect to Xt and Ys is defined as
∬[0,a]×[0,b]f(t,s)dXtdYs=limΔ→0n∑i=1m∑j=1f(ti,si)(Xti+1−Xti)(Ysj+1−Ysj), |
provided that there is an uncertain variable to which the above sum converges almost surely as Δ→0. In this case, the uncertain field f(t,s) is said to be integrable with respect to Xt and Ys.
Theorem 2.7. Zhang [11] (Fubini's Theorem) Let Xt and Ys be general Liu processes. Suppose f(t,s) is an integrable uncertain field with respect to Xt and Ys. Then
(1) ∫a0f(t,s)dXt and ∫b0f(t,s)dYs exist almost surely;
(2) ∫a0f(t,s)dXt and ∫b0f(t,s)dYs are integrable with respect to Ys and Xt, respectively;
(3)
∬[0,a]×[0,b]f(t,s)dYsdXt=∫b0∫a0f(t,s)dXtdYs,a.s. |
Definition 2.7. (Liu[12]) Suppose f and g are continuous functions. An uncertain differential equation
dXt=f(t,Xt)dt+g(t,Xt)dCt |
is said to be regular if
g(t,Xt)>0,∀t>0. |
Definition 2.8. (Zhang [11]) An uncertain process Xt is called a solution of the higher-order uncertain integral equation if
Xt=n−1∑i=0tii!Xi0+∫t0∫t10…∫tn−10f(t,Xt,X(1)t,X(2)t,…,X(n−1)t)dtndtn−1…dt2dt1+∫t0∫t10…∫tn−10g(t,Xt,X(1)t,X(2)t,…,X(n−1)t)dCtndtn−1…dt2dt1, |
where Ct is a Liu process, and f and g are continuous functions. Equivalently, the above equation can be simply written as the differential form
X(n)t=f(t,Xt,X(1)t,X(2)t,…,X(n−1)t)+g(t,Xt,X(1)t,X(2)t,…,X(n−1)t)˙Ct, |
that is called a higher-order uncertain diferential equation.
Theorem 2.8. (Liu [17]) A function Φ−1:(0,1)→ℜ is the inverse uncertainty distribution of an uncertain variable ξ if and only if it is continuous and
M{ξ≤Φ−1(α)}=α. |
Initially, for the significant research conclusions obtained in this paper, such as Theorem 3.6, a schematic diagram is employed for a more intuitive representation of the research process, as shown in Figure 3.
Next, we introduce Lemma 3.1, which serves as a foundation for the proof of Theorems 3.3, 3.4, 3.7, and 3.8.
Lemma 3.1. Lemma 3.1. Let Xt satisfies the following higher-order UDE:
{X(n)t=f(t,Xt,X(1)t,X(2)t,…,X(n−1)t)+g(t,Xt,X(1)t,X(2)t,…,X(n−1)t˙Ct,X0(γ)=X0,X(k)0(γ)=Xk0,k=1,2,…,n−1. |
Then, Xt also satisfies the following integral equation:
Xt=n−1∑k=0tkk!Xk0+1(n−1)!∫t0(t−s)n−1f(s,X(1)s,X(2)s,…,X(n−1)s)ds+1(n−1)!∫t0(t−s)n−1g(s,Xs,X(1)s,X(2)s,…,X(n−1)s)dCs. |
For simplicity and clarity, we denote Xt(γ) as Xt. Henceforth, we shall use Xt to represent the solution to the UDE and Xαt to denote its corresponding α-path.
Proof. Introduce two variables, Zt and Wt, which respectively satisfy the following two equations:
{Z(n)t=0,Zt|t=0=Z0,Z(k)t|t=0=Zk0,k=1,2,…,n−1. |
{W(n)t=f(t,Wt,W(1)t,W(2)t,…,W(n−1)t)+g(t,Wt,W(1)t,W(2)t,…,W(n−1)t)˙Ct,W(k)t|t=0=0. |
It can be easily derived that Xt=Zt+Wt. For Zt, we integrate both sides directly:
Zt=∫t0Z(1)sds=−∫t0Z(1)sd(t−s)=−Z(1)s(t−s)|t0−12∫t0Z(2)sd(t−s)2=Z10t+12Z20t2−13×2∫t0Z(3)s(s)d(t−s)3…=Z10t+12Z20t2+13×2Z30t3+⋯+1(n−1)!Zn−10t(n−1)=n−1∑k=0tkk!Zk0. | (3.1) |
For Wt, we use intregration by parts repeatedly as follows:
Wt=∫t0W(1)sds=−∫t0W(1)sd(t−s)=−W(1)s(t−s)|t0+∫t0(t−s)W(2)sds=−12W20t2+12∫t0(t−s)2W(3)sds…=1(n−1)!∫t0(t−s)n−1f(s,Ws,W(1)s,W(2)s,…,W(n−1)s)ds+1(n−1)!∫t0(t−s)n−1g(s,Ws,W(1)s,W(2)s,…,W(n−1)s)dCs. | (3.2) |
Thus, adding Eqs (3.1) and (3.2), we derive the integral equation satisfied by Xt:
Xt=Zt+Wt=n−1∑k=0tkk!Xk0+1(n−1)!∫t0(t−s)n−1X(n)s(s)ds=n−1∑k=0tkk!Xk0+1(n−1)!∫t0(t−s)n−1f(s,Xs,X(1)s,X(2)s,…,X(n−1)s)ds+1(n−1)!∫t0(t−s)n−1g(s,Xs,X(1)s,X(2)s,…,X(n−1)s)dCs. |
Definition 3.1. Let α be a number between 0 and 1. An uncertain differential equation
{dnXtdtn=f(t,Xt,…,dn−1Xtdtn−1)+g(t,Xt,…,dn−1Xtdtn−1)dCtdt,Xt|t=0=X0,dXtdt|t=0=X1,…,dnXtdtn|t=0=Xn |
is said to have an α-path Xαt if it solves the corresponding ordinary differential equation
{dnXαtdtn=f(t,Xαt,…,dn−1Xαtdtn−1)+|g(t,Xαt,…,dn−1Xαtdtn−1)|Φ−1(α),Xαt|t=0=X0,dXαtdt|t=0=X1,…,dnXαtdtn|t=0=Xn, |
where Φ−1(α) is the inverse standard normal uncertainty distribution, i.e.,
Φ−1(α)=√3πlnα1−α. |
Example 1. In the uncertain spring vibration equation [18] denoted as
{d2Xtdt2+20dXtdt+64Xt=dCtdt,Xt|t=0=0,dXtdt|t=0=0. |
The solution is given by
Xt=112∫t0(exp(−4t+4s)−exp(−16t+16s))dCs, |
it has an α-path
Xαt=(316−14exp(−4α)+116exp(−16α))√312πlnt1−t, |
and the inverse uncertainty distribution is expressed as
Ψ−1t(α)=(316−14exp(−4t)+116exp(−16t))√312πlnα1−α. |
Note 1.
(1) There are generally two approaches to obtaining the inverse uncertain distribution Ψ−1t(α) of the solution to a UDE. One approach involves directly solving the equation to obtain Ψ−1t(α)[18], and the other involves solving its α-path, which entails solving a family of deterministic ordinary differential equations to determine Ψ−1t(α).
(2) The first method is straightforward, allowing for the direct computation of the solution Xt, and consequently, the inverse uncertainty distribution. Example 1 is linear and thus allows for the computation of Xt. However, for the majority of equations, analytical solutions cannot be derived, and currently, there are no numerical methods available for directly solving uncertain systems. Therefore, this method has significant limitations. The second method does not suffer from such limitations, as it involves solving a family of ordinary differential equations for the α-path, which has a variety of solution methods. Consequently, the second method is more meaningful in practical applications and represents the direction of research in this paper.
(3) Regarding Xαt and Ψ−1t(α), if we consider them as bivariate functions, they represent the same function. If we view them as univariate functions with a single parameter, Xαt is a function with α as the parameter and t as the independent variable. Ψ−1t(α) is a function with t as the parameter and α as the independent variable. Therefore, knowing Xαt allows us to determine Ψ−1t(α) by simply interchanging the roles of the parameter and the independent variable.
Definition 3.2. Suppose f and g are continuous functions. An uncertain differential equation
dnXtdtn=f(t,Xt,…,dn−1Xtdtn−1)+g(t,Xt,…,dn−1Xtdtn−1)dCtdt |
is said to be regular if
g(t,Xt,…,dn−1Xtdtn−1)>0,∀t>0. |
Theorem 3.1. The uncertain differential equation is
{X(n)t=f(t,Xt,X(1)t,X(2)t,…,X(n−1)t)+g(t,Xt,X(1)t,X(2)t,…,X(n−1)t)˙Ct,Xt|t=0=y0,X(1)t|t=0=y1,…,X(n−1)t|t=0=yn−1 |
where
X=(Xt,X(1)t,X(2)t,…,X(n−1)t). |
It has a unique solution if the functions f(t,X) and g(t,X) satisfy the linear growth condition
|f(t,X)|+|g(t,X)|≤L(1+||X||),∀X∈ℜn,t≥0,||X||=n∑i=1|Xi|, |
and the Lipschitz condition
|f(t,X)−f(t,Z)|+|g(t,X)−g(t,Z)|≤L(|X−Z|),∀X,Z∈ℜn,t≥0. |
Without loss of generality, suppose L≥2. Moreover, the solution is sample continuous.
Proof. At first, Theorem 14.1 in [12] says that there exists an event Λ with M{Λ}=1, such that Ct(γ) is Lipschitz continuous with respect to t for each γ∈Λ. Next, we prove the existence and continuity of the solution by a successive approximation method. Define
X(0)t(γ)=y0,…,Y(0)tn−1(γ)=yn−1, |
and
{dXt=Yt,1dt,dYt,1=Yt,2dt,…dYt,n−1=f(t,Xt,Yt,1,…,Yt,n−1)dt+g(t,Xt,Yt,2,…,Yt,n−1)dCt,Xt|t=0=y0,Yt,1|t=0=y1,…,Yt,n−1|t=0=yn−1. |
By integrating,
{X(n)t(γ)=y0+∫t0Y(n−1)t,1(γ)ds,Y(n)t,1(γ)=y1+∫t0Y(n−1)t,2(γ)ds,…Y(n)t,n−1(γ)=yn−1+∫t0f(s,X(n−1)s(γ),…,Y(n−1)s,n−1(γ))ds+∫t0g(s,X(n−1)s(γ),…,Y(n−1)s,n−1(γ))dCs(γ),Xt|t=0=y0,Yt,1|t=0=y1,…,Yt,n−1|t=0=yn−1, |
for each integer n. It follows from the linear growth condition that
|X(1)t(γ)−X(0)t(γ)|+|Y(1)t,1(γ)−Y(0)s,1(γ)|+⋯+|Y(1)t,n−1(γ)−Y(0)t,n−1(γ)|=|∫t0Y0s,1(γ)ds|+|∫t0Y0s,2(γ)ds|+⋯+|∫t0f(s,X0s(γ),…,Y0s,n−1(γ))ds|+|∫t0g(s,X0s(γ),…,Y0s,n−1(γ))dCs(γ)|≤∫t0|y1|ds+∫t0|y2|ds+⋯+∫t0|f(s,X0s(γ),…,Y0s,n−1(γ))|ds+Kγ∫t0|g(s,X0s(γ),…,Y0s,n−1(γ))|ds≤∫t0(|y1|+⋯+|yn−1|)ds+∫t0L(1+|y0|+|y1|+⋯+|yn−1|)ds+Kγ∫t0L(1+|y0|+|y1|+⋯+|yn−1|)ds≤∫t0(1+|y0|+|y1|+⋯+|yn−1|)ds+∫t0L(1+|y0|+|y1|+⋯+|yn−1|)ds+Kγ∫t0L(1+|y0|+|y1|+⋯+|yn−1|)ds=∫t0(1+L+Kγ⋅L)(1+|y0|+|y1|+⋯+|yn−1|)ds≤∫t0(2+Kγ)L(1+|y0|+|y1|+⋯+|yn−1|)ds=(1+|y0|+|y1|+⋯+|yn−1|)L(2+Kγ)t |
for any t≥0, where Kγ is the Lipschitz constant to the sample path Ct(γ). Assume
|X(k)t(γ)−X(k−1)t(γ)|+|Y(k)t,1(γ)−Y(k−1)t,1(γ)|+⋯+|Y(k)t,n−1(γ)−Y(k−1)t,n−1(γ)|≤(1+|y0|+|y1|+⋯+|yn−1|)Lk(2+Kγ)kk!tk. |
It follows from the Lipschitz condition that
|X(k+1)t(γ)−X(k)t(γ)|+|Y(k+1)t,1(γ)−Y(k)t,1(γ)|+⋯+|Y(k+1)tn−1(γ)−Y(k)tn−1(γ)|≤∫t0|Y(k)s,1(γ)−Y(k−1)s,1(γ)|ds+⋯+∫t0|Y(k)s,n−1(γ)−Y(k−1)s,n−1(γ)|ds+∫t0|f(s,X(k)s(γ),…,Y(k)s,n−1(γ))−f(s,X(k−1)s(γ),…,Y(k−1)s,n−1(γ))|ds+Kγ∫t0|g(s,X(k)s(γ),…,Y(k)s,n−1(γ))−g(s,X(k−1)s(γ),…,Y(k−1)s,n−1(γ))|ds≤∫t0(|X(k)s(γ)−X(k−1)s(γ)|+|Y(k)s,1(γ)−Y(k−1)s,1(γ)|+⋯+|Y(k)s,n−1(γ)−Y(k−1)s,n−1(γ)|)ds+L∫t0(|X(k)s(γ)−X(k−1)s(γ)|+|Y(k)s,1(γ)−Y(k−1)s,1(γ)|+⋯+|Y(k)s,n−1(γ)−Y(k−1)s,n−1(γ)|)ds+KγL∫t0(|X(k)s(γ)−X(k−1)s(γ)|+|Y(k)s,1(γ)−Y(k−1)s,1(γ)|+⋯+|Y(k)s,n−1(γ)−Y(k−1)s,n−1(γ)|)ds≤(1+|y0|+|y1|+⋯+|yn−1|)Lk+1(2+Kγ)k+1(k+1)!tk+1. |
The above induction shows that
|Y(k+1)t,n−1(γ)−Y(k)t,n−1(γ)|≤(1+|y0|+|y1|+⋯+|yn−1|)Ln+1(2+Kγ)n+1(n+1)!tn+1 |
for each integer n. This means that, for each γ∈Λ, the sequence X(n)t(γ) converges uniformly on any given time interval as n→∞. Furthermore, since X(0)t is constant, obviously it is continuous. Suppose X(k)t is continuous. Then, X(k)t is also continuous with respect to t. So, by induction, X(n)t is continuous for all n. Provided X(n)t converges uniformly, that is limn→∞X(n)t(γ)=Xt(γ), the limit function Xt is also continuous.
The solution to the first equation below is Xt(γ).
{Xt(γ)=y0+∫t0Yt,1(γ)ds,Yt,1(γ)=y1+∫t0Yt,2(γ)ds,…Yt,n−1(γ)=yn−1+∫t0f(s,Xs(γ),…,Ys,n−1(γ))ds+∫t0g(s,Xs(γ),…,Ys,n−1(γ))dCs(γ),Xt|t=0=y0,Yt,1|t=0=y1,…,Yt,n−1|t=0=yn−1. |
We then prove that the solution is unique. Assume that both Xt and X∗t are solutions of the UDE. For each γ∈Λ, it follows from the Lipschitz condition that
Xt(γ)−X∗t(γ)≤L(2+Kγ)∫t0(Xs(γ)−X∗s(γ))ds. |
By using the Gronwall inequality, we obtain
Xt(γ)−X∗t(γ)=0⋅exp(L(2+Kγ)t)=0. |
Hence Xt=X∗t. The uniqueness is verified. The theorem is proved.
Note 2. The conditions on f and g in the aforementioned theorem are stringent, leading to an ideal conclusion: the global existence of solutions. However, in practical problems, such a perfect conclusion is not necessary. Therefore, we can relax the condition, for instance, by discussing the properties of solutions within a bounded domain. In this case, the linear growth condition for f and g is not required. Instead, it is sufficient that f and g have an upper bound M on the domain G. This also allows us to derive th einequality
|X(1)t(γ)−X(0)t(γ)|+|Y(1)t1(γ)−Y(0)s1(γ)|+⋯+|Y(1)tn−1(γ)−Y(0)tn−1(γ)|≤(1+|y0|+|y1|+⋯+|yn−1|)L(2+Kγ)t. |
From this inequality, we can infer the existence, continuity, and uniqueness of the solution on the domain. These are more practical conditions for applications, and the examples provided later in the paper all meet this criterion.
Theorem 3.2. Let Xαt be the α-path of the uncertain differential equation
{X(n)t=f(t,Xt,X(1)t,X(2)t,…,X(n−1)t)+g(t,Xt,X(1)t,X(2)t,…,X(n−1)t)˙Ct,Xt|t=0=y0,X(1)t|t=0=y1,…,X(n−1)t|t=0=yn−1. |
If f and g satisfy the Lipschitz and regular conditions hold, then Xαt is a continuous function with respect to α at each time t>0.
Proof. Since f and g satisfy the Lipschitz conditions, it follows from Theorem 3.1 that the UDEs
{dXαt=Yαt,1dt,dYαt,1=Yαt,2dt,…dYαt,n−1=f(t,Xαt,Yαt,1,…,Yαt,n−1)dt+g(t,Xαt,Yαt,2,…,Yαt,n−1)Φ−1(α)dt,Xt|t=0=y0,Yt1|t=0=y1,…,Yt,n−1|t=0=yn−1. |
have a unique solution Xαt, where Φ−1(α) is the inverse standard normal uncertainty distribution. For any numbers α and β between 0 and 1, it follows from the Lipschitz condition that
|Xαt−Xβt|+|Yαt,1−Yβt,1|+⋯+|Yαt,n−1−Yβt,n−1|≤∫t0|Yαs,1−Yβs,1|ds+⋯+∫t0|Yαs,n−1−Yβs,n−1|ds+∫t0|f(s,Xαs,…,Yαs,n−1)−f(s,Xβs,…,Yβs,n−1)|ds+∫t0|g(s,Xαs,…,Yαs,n−1)−g(s,Xβs,…,Yβs,n−1)||Φ−1(α)|ds+∫t0|g(s,Xβs,…,Yβs,n−1)||Φ−1(α)−Φ−1(β)| |
≤∫t0(|Xαs−Xβs|+|Yαs,1−Yβs,1|+⋯+|Yαs,n−1−Yβs,n−1|)ds+L∫t0(|Xαs−Xβs|+|Yαs,1−Yβs,1|+⋯+|Yαs,n−1−Yβs,n−1|)ds+L|Φ−1(α)|∫t0(|Xαs−Xβs|+|Yαs,1−Yβs,1|+⋯+|Yαs,n−1−Yβs,n−1|)ds+|Φ−1(α)−Φ−1(β)|∫t0|g(s,Xβs,…,Yβs,n−1)|ds≤(2+Φ−1(α))L∫t0(|Xαs−Xβs|+|Yαs,1−Yβs,1|+⋯+|Yαs,n−1−Yβs,n−1|)ds+|Φ−1(α)−Φ−1(β)|∫t0|g(s,Xβs,…,Yβs,n−1)|ds. |
By using the Gronwall inequality, we obtain
|Xαt−Xβt|+|Yαt,1−Yβt,1|+⋯+|Yαt,n−1−Yβt,n−1|≤|Φ−1(α)−Φ−1(β)|∫t0|g(s,Xβs,…,Yβs,n−1)|ds⋅L(2+Φ−1(α))t. |
Thus
limα→β|Xαt−Xβt|=0 |
for each time t>0. Hence Xαt is continuous with respect to α.
Note 3. The proof of Theorem 3.2 is pivotal as it establishes a necessary condition for Xαt to serve as an inverse uncertainty distribution. This condition will be instrumental in the subsequent proofs of Theorems 3.6 and 3.10.
Theorem 3.3. Let Xαt be the α-path of the regular uncertain differential equation
d2Xtdt2=f(t,Xt,dXtdt)+g(t,Xt,dXtdt)dCtdt. |
If the conditions
f(x,y1,z)≤f(x,y2,z),g(x,y1,z)≤g(x,y2,z),∀y1<y2 | (H) |
are met and the linear growth, Lipschitz, and regular conditions hold, then Xαt is a continuous and strictly increasing function with respect to α at each time t>0.
Proof. Since f and g satisfy the linear growth and Lipschitz conditions, the α-path Xαt is continuous with respect to t. Let Φ−1 be the inverse standard normal uncertainty distribution, and let α and β be numbers with 0<α<β<1. Write the second-order UDEs in the form of a system of equations:
{dXαt=Yαtdt,dYαt=f(t,Xαt,Yαt)+g(t,Xαt,Yαt)Φ−1(α)dt,Xα0=X0,Yα0=Y0. | (3.3) |
{dXβt=Yβtdt,dYβt=f(t,Xβt,Yβt)+g(t,Xβt,Yβt)Φ−1(β)dt,Xβ0=X0,Yβ0=Y0. | (3.4) |
Define μ and ν as:
μ(T,t)=(T−t)[f(t,Xαt,Yαt)+g(t,Xαt,Yαt)Φ−1(α)], |
ν(T,t)=(T−t)[f(t,Xβt,Yβt)+g(t,Xβt,Yβt)Φ−1(β)]. |
We have
μ(T,0)=T[f(0,X0,Y0)+g(0,X0,Y0)Φ−1(α)], |
ν(T,0)=T[f(0,X0,Y0)+g(0,X0,Y0)Φ−1(β)]. |
Since g(0,X0,Y0)>0 (regular condition), we have
μ(T,0)<ν(T,0). |
Due to the continuous nature of f and g, and the continuity of Xαt and Yαt, we can infer that the composite functions μ and ν are also continuous with respect to t.
By the continuity of μ and ν, there exists a small number r>0 such that
μ(T,t)<ν(T,t),∀t∈[0,r]. |
Thus, by Lemma 3.1, we have:
XαT=X0+Y0T+∫T0(T−t)f(t,Xαt,Yαt)dt+∫T0(T−t)g(t,Xαt,Yαt)Φ−1(α)dt<X0+Y0T+∫T0(T−t)f(t,Xβt,Yβt)dt+∫T0(T−t)g(t,Xβt,Yβt)Φ−1(β)dt=XβT |
for any time T∈(0,r].
If for any t>r, Xαt<Xβt, and the theorem holds. We will prove that by contradiction.
Suppose there exists a time b>r at which Xαt and Xβt first meet, i.e.,
Xαb=Xβb,Xαt<Xβt,∀t∈(0,b). |
The next phase of our proof will be to compare Yαt and Yβt in a similar way. Write
¯μ(t)=f(t,Xαt,Yαt)+g(t,Xαt,Yαt)Φ−1(α), |
¯ν(t)=f(t,Xβt,Yβt)+g(t,Xβt,Yβt)Φ−1(β). |
Since g(0,X0,Y0)>0, we have
¯μ(0)<¯ν(0). |
By the continuity of ¯μ and ¯ν, there exists a small number ¯r>0 such that
¯μ(t)<¯ν(t),∀t∈[0,¯r]. |
Thus,
Yαt=Y0+∫t0f(s,Xαs,Yαs)ds+∫t0g(s,Xαs,Yαs)Φ−1(α)ds<Y0+∫t0f(s,Xβs,Yβs)ds+∫t0g(s,Xβs,Yβs)Φ−1(β)ds=Yβt, |
for any time t∈(0,¯r].
We will prove Yαt<Yβt for ¯r<t<b by contradiction.
Suppose there exists a time ¯r<¯b<b at which Yαt and Yβt first meet, i.e.,
Yα¯b=Yβ¯b,Yαt<Yβt,∀t∈(0,¯b). |
Due to conditions (H), we have
f(¯b,Xα¯b,Yα¯b)<f(¯b,Xβ¯b,Yβ¯b),0<g(¯b,Xα¯b,Yα¯b)<g(¯b,Xβ¯b,Yβ¯b). |
Then
¯μ(¯b)<¯ν(¯b). |
By the continuity of ¯μ and ¯ν, there exists a time ¯a∈(0,¯b) such that
¯μ(t)<¯ν(t),t∈[¯a,¯b]. |
Thus,
Yα¯b=Yα¯a+∫¯b¯af(s,Xαs,Yαs)ds+∫¯b¯ag(s,Xαs,Yαs)Φ−1(α)ds<Yβ¯a+∫¯b¯af(s,Xβs,Yβs)ds+∫¯b¯ag(s,Xβs,Yβs)Φ−1(β)ds=Yβ¯b, |
which is in contradiction with the assumption Yα¯b=Yβ¯b. Therefore,
Yαt<Yβt,∀0<t<b. |
Integrate Eqs (3.3) and(3.4)
Xαb=X0+∫b0Yαtdt, |
Xβb=X0+∫b0Yαtdt, |
and we have
Xαb<Xβb, |
which is in contradiction with the assumption Xαb=Xβb. The theorem is proved.
Note 4. Condition (H) is indispensable in the proof of the theorem. Should condition (H) not hold, it would be impossible to establish the relationship between Xα¯b and Xβ¯b when proving ¯μ(¯b)<¯ν(¯b) and thus the proof would be untenable.
By Theorem 3.3, we have established that Xt is a continuous and strictly increasing function with respect to α. We wonder whether this collection of α-paths can determine the inverse uncertainty distribution of the solutions to second-order UDEs. The following Theorem 3.4 provides a definitive answer.
Theorem 3.4. Let Xt and Xαt be the solution and α-path of a regular uncertain differential equation
d2Xtdt2=f(t,Xt,dXtdt)+g(t,Xt,dXtdt)dCtdt. |
If the conditions (H) are met and the linear growth, Lipschitz, and regular conditions hold, then we have
M{Xt≤Xαt,∀t}=α, |
M{Xt>Xαt,∀t}=1−α. |
Proof. Theorem 14.3 in [12] constructs an event Λ1 with M{Λ1}=α, and shows that for each γ∈Λ1, there exists a small number δ>0 such that
Cs(γ)−Ct(γ)s−t<Φ−1(α−δ) | (3.5) |
for any times s and t with s>t, where Φ−1 is the inverse standard normal uncertainty distribution. Since f and g satisfy the linear growth and Lipschitz conditions, Xαt and Xt(γ) are continuous with respect to t. Write the second-order UDE in the form of a system of equations:
{dXt=Ytdt,dYt=f(t,Xt,Yt)dt+g(t,Xt,Yt)dCt,Xt|t=0=X0,Yt|t=0=Y0. | (3.6) |
Define λ, μ, and ν as:
λ(T,t,s)=(T−t)[f(t,Xt(γ),Yt(γ))+g(t,Xt(γ),Yt(γ))Cs(γ)−Ct(γ)s−t], |
μ(T,t)=(T−t)[f(t,Xt(γ),Yt(γ))+g(t,Xt(γ),Yt(γ))Φ−1(α−δ)], |
ν(T,t)=(T−t)[f(t,Xαt,Yαt)+g(t,Xαt,Yαt)Φ−1(α)]. |
Due to the continuous nature of f and g, and the continuity of Xt(γ) and Yt(γ), we can infer that the composite functions λ, μ, and ν are also continuous with respect to t.
Since g(0,X0,Y0)>0 (regular condition), we have
μ(T,0)<ν(T,0). |
By the continuity of μ and ν, there exists a small number r>0 such that
μ(T,t)<ν(T,t),∀t∈[0,r]. |
By inequality (3.5), for any time t∈[0,r] and any time s∈(t,∞), we have
λ(T,t,s)=(T−t)[f(t,Xt(γ),Yt(γ))+g(t,Xt(γ),Yt(γ))Cs(γ)−Ct(γ)s−t]<(T−t)[f(t,Xt(γ),Yt(γ))+g(t,Xt(γ),Yt(γ))Φ−1(α−δ)]=μ(T,t)<ν(T,t). |
By Lemma 3.1, it follows that
XT(γ)=X0+Y0T+∫T0(T−t)f(t,Xt(γ),Yt(γ))dt+∫T0(T−t)g(t,Xt(γ),Yt(γ))dCt(γ)=X0+Y0T+limΔ→0k∑i=1λ(T,ti+1,ti)(ti+1−ti)≤X0+Y0T+limΔ→0k∑i=1μ(T,ti)(ti+1−ti)=X0+Y0T+∫T0μ(T,t)dt<X0+Y0T+∫T0ν(T,t)dt=X0+Y0T+∫T0(T−t)f(t,Xαt,Yαt)dt+∫T0(T−t)g(t,Xαt,Yαt)Φ−1(α)dt=XαT |
for any time T∈(0,r].
We will then prove Xt(γ)<Xαt for all t>r by contradiction.
Suppose there exists a time b>r at which Xt(γ) and Xαt first meet, i.e.,
Xb(γ)=Xαb,Xt(γ)<Xαt,∀t∈(0,b). |
The next phase of our proof will be to compare Yαt and Yt(γ) in a similar way. Write
¯λ(t,s)=f(t,Xt(γ),Yt(γ))+g(t,Xt(γ),Yt(γ))Cs(γ)−Ct(γ)s−t, |
¯μ(t)=f(t,Xt(γ),Yt(γ))+g(t,Xt(γ),Yt(γ))Φ−1(α−δ), |
¯ν(t)=f(t,Xαt,Yαt)+g(t,Xαt,Yαt)Φ−1(α). |
Since g(0,X0,Y0)>0, we have
¯μ(0)<¯ν(0). |
By the continuity of ¯μ and ¯ν, there exists a small number ¯r>0 such that
¯μ(t)<¯ν(t),∀t∈[0,¯r]. |
By inequality (3.5), for any time t∈[0,¯r] and any time s∈(t,∞), we have
¯λ(t,s)=f(t,Xt(γ),Yt(γ))+g(t,Xt(γ),Yt(γ))Cs(γ)−Ct(γ)s−t<f(t,Xt(γ),Yt(γ))+g(t,Xt(γ),Yt(γ))Φ−1(α−δ)=¯μ(t)<¯ν(t). |
Thus,
Yt(γ)=Y0+∫t0f(s,Xs(γ),Ys(γ))ds+∫t0g(s,Xs(γ),Ys(γ))dCs(γ)=Y0+limΔ→0k∑i=1¯λ(ti+1,ti)(ti+1−ti)≤Y0+limΔ→0k∑i=1¯μ(ti)(ti+1−ti)=Y0+∫t0¯μ(t)dt<Y0+∫t0¯ν(t)dt=Y0+∫t0f(s,Xαs,Yαs)ds+∫t0g(s,Xαs,Yαs)Φ−1(α)ds=Yαt |
for any time t∈(0,¯r].
We will prove Yt(γ)<Yαt for all ¯r<t<b by contradiction.
Suppose there exists a time ¯r<¯b<b at which Yt(γ) and Yαt first meet, i.e.,
Y¯b(γ)=Yα¯b,Yt(γ)<Yαt,∀t∈(0,¯b). |
Due to conditions (H), we have
f(¯b,X¯b(γ),Y¯b(γ))<f(¯b,Xα¯b,Yα¯b),0<g(¯b,X¯b(γ),Y¯b(γ))<g(¯b,Xα¯b,Yα¯b), |
and then
¯μ(¯b)<¯ν(¯b). |
By the continuity of ¯μ and ¯ν, there exists a time ¯a∈(0,¯b) such that
¯μ(t)<¯ν(t),t∈[¯a,¯b]. |
By inequality (3.5), for any time t∈[¯a,¯b] and any time s∈(t,∞), we have
¯λ(t,s)=f(t,Xt(γ),Yt(γ))+g(t,Xt(γ),Yt(γ))Cs(γ)−Ct(γ)s−t<f(t,Xt(γ),Yt(γ))+g(t,Xt(γ),Yt(γ))Φ−1(α−δ)=¯μ(t)<¯ν(t). |
Thus,
Y¯b(γ)=Y¯a(γ)+∫¯b¯af(s,Xs(γ),Ys(γ))ds+∫¯b¯ag(s,Xs(γ),Ys(γ))dCs(γ)=Y¯a(γ)+limΔ→0k∑i=1¯λ(ti+1,ti)(ti+1−ti)≤Y¯a(γ)+limΔ→0k∑i=1¯μ(ti)(ti+1−ti)=Y¯a(γ)+∫¯b¯a¯μ(t)ds<Yα¯a+∫¯b¯a¯ν(t)ds=Yα¯a+∫¯b¯af(s,Xαs,Yαs)ds+∫¯b¯ag(s,Xαs,Yαs)Φ−1(α)ds=Yα¯b, |
which is in contradiction with Y¯b(γ)=Yα¯b. Therefore,
Yt(γ)<Yαt,∀0<t<b. |
Integrate Eq (3.6),
Xb(γ)=X0+∫b0Yt(γ)dt, |
Xαb=X0+∫b0Yαtdt, |
and we have
Xb(γ)<Xαb, |
which is in contradiction with Xb(γ)=Xαb. Therefore,
Xt(γ)<Xαt,∀t>0. |
Since M{Λ1}=α, we have
M{Xt≤Xαt,∀t}≥α. | (3.7) |
Theorem 14.3 in [12] constructs an event Λ2 with M{Λ2}=1−α, and shows that for each γ∈Λ2, there exists a small number δ>0 such that
Cs(γ)−Ct(γ)s−t>Φ−1(α+δ) | (3.8) |
for any times s and t with s>t. Write
λ(T,t,s)=(T−t)[f(t,Xt(γ),Yt(γ))+g(t,Xt(γ),Yt(γ))Cs(γ)−Ct(γ)s−t], |
μ(T,t)=(T−t)[f(t,Xt(γ),Yt(γ))+g(t,Xt(γ),Yt(γ))Φ−1(α+δ)], |
ν(T,t)=(T−t)[f(t,Xαt,Yαt)+g(t,Xαt,Yαt)Φ−1(α)]. |
Since g(0,X0,Y0)>0 (regular condition), we have
μ(T,0)>ν(T,0). |
By the continuity of μ and ν, there exists a small number r>0 such that
μ(T,t)>ν(T,t),∀t∈[0,r]. |
By inequality (3.8), for any time t∈[0,r] and any time s∈(t,∞), we have
λ(T,t,s)=(T−t)[f(t,Xt(γ),Yt(γ))+g(t,Xt(γ),Yt(γ))Cs(γ)−Ct(γ)s−t]>(T−t)[f(t,Xt(γ),Yt(γ))+g(t,Xt(γ),Yt(γ))Φ−1(α+δ)]=μ(T,t)>ν(T,t). |
By Lemma 3.1, it follows that
XT(γ)=X0+Y0T+∫T0(T−t)f(t,Xt(γ),Yt(γ))dt+∫T0(T−t)g(t,Xt(γ),Yt(γ))dCt(γ)=X0+Y0T+limΔ→0k∑i=1λ(T,ti+1,ti)(ti+1−ti)≥X0+Y0T+limΔ→0k∑i=1μ(T,ti)(ti+1−ti)=X0+Y0T+∫T0μ(T,t)dt>X0+Y0T+∫T0ν(T,t)dt=X0+Y0T+∫T0(T−t)f(t,Xαt,Yαt)dt+∫T0(T−t)g(t,Xαt,Yαt)Φ−1(α)dt=XαT |
for any time T∈(0,r].
We will then prove Xt(γ)<Xαt for all t>r by contradiction.
Suppose there exists a time b>r at which Xt(γ) and Xαt first meet, i.e.,
Xb(γ)=Xαb,Xt(γ)>Xαt,∀t∈(0,b). |
The next phase of our proof will be to compare Yαt and Yt(γ) in a similar way. Write
¯λ(t,s)=f(t,Xt(γ),Yt(γ))+g(t,Xt(γ),Yt(γ))Cs(γ)−Ct(γ)s−t, |
¯μ(t)=f(t,Xt(γ),Yt(γ))+g(t,Xt(γ),Yt(γ))Φ−1(α+δ), |
¯ν(t)=f(t,Xαt,Yαt)+g(t,Xαt,Yαt)Φ−1(α). |
Since g(0,X0,Y0)>0, we have
¯μ(0)>¯ν(0). |
By the continuity of ¯μ and ¯ν, there exists a small number ¯r>0 such that
¯μ(t)>¯ν(t),∀t∈[0,¯r]. |
By inequality (3.8), for any time t∈[0,¯r] and any time s∈(t,∞), we have
¯λ(t,s)=f(t,Xt(γ),Yt(γ))+g(t,Xt(γ),Yt(γ))Cs(γ)−Ct(γ)s−t>f(t,Xt(γ),Yt(γ))+g(t,Xt(γ),Yt(γ))Φ−1(α+δ)=¯μ(t)>¯ν(t). |
Thus,
Yt(γ)=Y0+∫t0f(s,Xs(γ),Ys(γ))ds+∫t0g(s,Xs(γ),Ys(γ))dCs(γ)=Y0+limΔ→0k∑i=1¯λ(ti+1,ti)(ti+1−ti)≥Y0+limΔ→0k∑i=1¯μ(ti)(ti+1−ti)=Y0+∫t0¯μ(t)dt>Y0+∫t0¯ν(t)dt=Y0+∫t0f(s,Xαs,Yαs)ds+∫t0g(s,Xαs,Yαs)Φ−1(α)ds=Yαt |
for any time t∈(0,¯r].
We will prove Yt(γ)>Yαt for all ¯r<t<b by contradiction.
Suppose there exists a time ¯r<¯b<b at which Yt(γ) and Yαt first meet, i.e.,
Y¯b(γ)=Yα¯b,Yt(γ)>Yαt,∀t∈(0,b). |
Due to conditions (H), we have
f(¯b,X¯b(γ),Y¯b(γ))>f(¯b,Xα¯b,Yα¯b),g(¯b,X¯b(γ),Y¯b(γ))>g(¯b,Xα¯b,Yα¯b)>0, |
and then
¯μ(¯b)>¯ν(¯b). |
By the continuity of ¯μ and ¯ν, there exists a time ¯a∈(0,¯b) such that
¯μ(t)>¯ν(t),t∈[¯a,¯b]. |
By inequality (3.8), for any time t∈[¯a,¯b] and any time s∈(t,∞), we have
¯λ(t,s)=f(t,Xt(γ),Yt(γ))+g(t,Xt(γ),Yt(γ))Cs(γ)−Ct(γ)s−t>f(t,Xt(γ),Yt(γ))+g(t,Xt(γ),Yt(γ))Φ−1(α+δ)=¯μ(t)>¯ν(t). |
Thus,
Y¯b(γ)=Y¯a(γ)+∫¯b¯af(s,Xs(γ),Ys(γ))ds+∫¯b¯ag(s,Xs(γ),Ys(γ))dCs(γ)=Y¯a(γ)+limΔ→0k∑i=1¯λ(ti+1,ti)(ti+1−ti)≥Y¯a(γ)+limΔ→0k∑i=1¯μ(ti)(ti+1−ti)=Y¯a(γ)+∫¯b¯a¯μ(t)ds>Yα¯a+∫¯b¯a¯ν(t)ds=Yα¯a+∫¯b¯af(s,Xαs,Yαs)ds+∫¯b¯ag(s,Xαs,Yαs)Φ−1(α)ds=Yα¯b, |
which is in contradiction with Y¯b(γ)=Yα¯b. Therefore,
Yt(γ)>Yαt,∀0<t<b. |
Integrate Eq (3.6),
Xb(γ)=X0+∫b0Yt(γ)dt, |
Xαb=X0+∫b0Yαtdt, |
and we have
Xb(γ)>Xαb, |
which is in contradiction with Xb(γ)=Xαb. Therefore,
Xt(γ)>Xαt,∀t>0. |
Since M{Λ2}=1−α, we have
M{Xt>Xαt,∀t}≥1−α. | (3.9) |
It follows from (3.7), (3.9) and
M{Xt≤Xαt,∀t}+M{Xt>Xαt,∀t}≤1, |
that
M{Xt≤Xαt,∀t}=α, |
M{Xt>Xαt,∀t}=1−α. |
The proof is now complete.
Theorem 3.5. Let Xt and Xαt be the solution and α-path of a regular uncertain differential equation
d2Xtdt2=f(t,Xt,dXtdt)+g(t,Xt,dXtdt)dCtdt. |
If the conditions (H) are met, the linear growth, Lipschitz and regular conditions hold, then at any time t>0, we have
M{Xt≤Xαt}=α, |
M{Xt>Xαt}=1−α. |
Proof. Note that {Xt≤Xαt}⊃{Xs≤Xαs,∀s>0} holds for any time t>0. By using the Theorems 3.3 and 3.4, we obtain
M{Xt≤Xαt}≥M{Xs≤Xαs,∀s>0}=α. |
Similarly, we also have
M{Xt>Xαt}≥M{Xs>Xαs,∀s>0}=1−α. |
Since {Xt≤Xαt} and {Xt>Xαt} are opposite events for each t>0, the duality axiom makes
M{Xt≤Xαt}+M{Xt>Xαt}=1. |
The theorem is proved.
Theorem 3.6. Let Xt and Xαt be the solution and α-path of the uncertain differential equation
d2Xtdt2=f(t,Xt,dXtdt)+g(t,Xt,dXtdt)dCtdt, |
respectively. If the conditions (H) are met, the linear growth, Lipschitz and regular conditions hold, then Xt has an inverse uncertainty distribution
Ψ−1t(α)=Xαt. |
Proof. Theorem 3.2 says that Xαt is a continuous function with respect to α at each time t>0. Theorem 3.4 says that
M{Xt≤Xαt}=α |
for any α∈(0,1). It follows from Theorem 2.8 that the inverse uncertainty distribution of Xt is Ψ−1t(α)=Xαt.
Determining the inverse uncertainty distribution of solutions to second-order UDEs is more complex than that for first-order UDEs. In our analysis, we employ Condition H, which is fundamentally derived from the comparison theorem. Readers can refer to publications on the comparison theorem for higher-order differential equations [19], partial order relations, and quasi-monotone functions [20].
We now turn to the discussion of higher-order UDEs. In Definition 2.7, we defined higher-order UDEs and presented them in the form of a system of Eq (3.10). The first component of the solution to system (3.10) is the solution to the equation as defined in Definition 2.7.
{dXt=Yt,1dt,dYt,1=Yt,2dt,…dYt,n−1=f(t,Xt,Yt,1,…,Yt,n−1)dt+g(t,Xt,Yt,1,…,Yt,n−1)dCt,Xt|t=0=X0,Yt1|t=0=Y1,…,Ytn−1|t=0=Yn−1. | (3.10) |
However, since f is an (n+1)-ary function, applying the condition of inverse monotonicity becomes more complex and less straightforward to prove. Yet, if our UDE is formulated as in Eq (3.11), we will proceed to provide a proof for the theorem below.
Theorem 3.7. Let Xαt be the α-path of a regular uncertain differential equation
dnXtdtn=f(t,Xt)+g(t,Xt)dCtdt. | (3.11) |
If the linear growth, Lipschitz and regular conditions hold, then Xαt is a continuous and strictly increasing function with respect to α at each time t>0.
Proof. Since f and g satisfy the linear growth and Lipschitz conditions, the α-path Xαt is continuous with respect to t. Let Φ−1 be the inverse standard normal uncertainty distribution, and let α and β be numbers with 0<α<β<1. Write
{dXαt=Yαt,1dt,dYαt,1=Yαt,2dt,…dYαt,n−1=f(t,Xαt)dt+g(t,Xαt)Φ−1(α)dt,Xαt|t=0=X0,Yαt,1|t=0=Y1,…,Yαt,n−1|t=0=Yn−1. | (3.12) |
{dXβt=Yβt,1dt,dYβt,1=Yβt,2dt,…dYβt,n−1=f(t,Xβt)dt+g(t,Xβt)Φ−1(β)dt,Xβt|t=0=X0,Yβt,1|t=0=Y1,…,Yβt,n−1|t=0=Yn−1. | (3.13) |
μ(T,t)=(T−t)n−1[f(t,Xαt)+g(t,Xαt)Φ−1(α)], |
ν(T,t)=(T−t)n−1[f(t,Xβt)+g(t,Xβt)Φ−1(β)], |
μ1(t)=f(t,Xαt)+g(t,Xαt)Φ−1(α), |
ν1(t)=f(t,Xβt)+g(t,Xβt)Φ−1(β). |
Due to the continuous nature of f and g, and the continuity of Xαt and Yαt, we can infer that the composite functions μ, ν and μ1, ν1 are also continuous with respect to t.
Since g(0,X0)>0 (regular condition), we have
μ(T,0)<ν(T,0). |
By the continuity of μ and ν, there exists a small number r>0 such that
μ(T,t)<ν(T,t),∀t∈[0,r]. |
By Lemma 3.1, it follows that
XαT=X0+n−1∑k=1Tkk!Yk+1(n−1)![∫T0(T−t)n−1f(t,Xαt)dt+∫T0(T−t)n−1g(t,Xαt)Φ−1(α)dt]<X0+n−1∑k=1Tkk!Yk+1(n−1)![∫T0(T−t)n−1f(t,Xβt)dt+∫T0(T−t)n−1g(t,Xβt)Φ−1(β)dt]=XβT |
for any time T∈(0,r].
We will prove Xαt<Xβt for any t>r by contradiction.
Suppose there exists a time b>r at which Xαt and Xβt first meet, i.e.,
Xαb=Xβb,Xαt<Xβt,∀t∈(0,b). |
Since f(b,Xβb)=f(b,Xαb),g(b,Xβb)=g(b,Xαb)>0, and we have
μ1(b)<ν1(b). |
By the continuity of μ1 and ν1, there exists a time a∈(0,b) such that
μ1(t)<ν1(t),t∈[a,b]. |
Then, we have
(b−t)n−1(n−1)!μ1(t)<(b−t)n−1(n−1)!ν1(t),t∈[a,b). |
Choose a1 and b1 such that a<a1<b1<b. Thus, we have
(b−t)n−1(n−1)!μ1(t)<(b−t)n−1(n−1)!ν1(t),t∈[a1,b1]. |
Note that any continuous function defined on a closed interval can always reach its minimum. Without loss of generality, suppose that ν1(t)-μ1(t) reaches its minimum on [a1,b1] at t∗∈[a1,b1]. Then, we have the following inequality:
∫ba(b−t)n−1(n−1)!(ν1(t)−μ1(t))dt⩾∫b1a1(b−t)n−1(n−1)!(ν1(t)−μ1(t))dt⩾∫b1a1(b−b1)n−1(n−1)!(ν1(t∗)−μ1(t∗))dt=(b−b1)n−1(n−1)!(ν1(t∗)−μ1(t∗))(b1−a1)>0. |
Thus,
Xαb=X0+n−1∑k=1(b−a)kk!Yk+1(n−1)![∫ba(b−t)n−1f(t,Xαt)dt+∫ba(b−t)n−1g(t,Xαt)Φ−1(α)dt]<X0+n−1∑k=1(b−a)kk!Yk+1(n−1)![∫ba(b−t)n−1f(t,Xβt)dt+∫ba(b−t)n−1g(t,Xβt)Φ−1(β)dt]=Xβb, |
which is in contradiction with Xb(γ)=Xαb. The theorem is proved.
By Theorem 3.7, we have established that Xt is a continuous and strictly increasing function with respect to α. We wonder whether this collection of α-paths can determine the inverse uncertainty distribution of the solutions to higher-order UDEs. The following Theorem 3.8 provides a definitive answer.
Theorem 3.8. Let Xt and Xαt be the solution and α-path of the regular uncertain differential equation
dnXtdtn=f(t,Xt)+g(t,Xt)dCtdt. |
If the linear growth, Lipschitz and regular conditions hold, then
M{Xt≤Xαt,∀t}=α, |
M{Xt>Xαt,∀t}=1−α. |
Proof. Theorem 14.3 in [12] constructs an event Λ1 with M{Λ1}=α, and shows that for each γ∈Λ1, there exists a small number δ>0 such that
Cs(γ)−Ct(γ)s−t<Φ−1(α−δ), | (3.14) |
for any times s and t with s>t, where Φ−1 is the inverse standard normal uncertainty distribution. Since f and g satisfy the linear growth and Lipschitz conditions, it follows from Theorem 3.1 that Xαt and Xt(γ) exist and are continuous with respect to t. Write the higher-order UDE in the form of a system of equations:
{dXt=Yt,1dt,dYt,1=Yt,2dt,…dYt,n−1=f(t,Xt)dt+g(t,Xt)dCt,Xt|t=0=X0,Yt1|t=0=Y1,…,Ytn−1|t=0=Yn−1. | (3.15) |
λ(T,t,s)=(T−t)n−1[f(t,Xt(γ))+g(t,Xt(γ))Cs(γ)−Ct(γ)s−t], |
μ(T,t)=(T−t)n−1[f(t,Xt(γ))+g(t,Xt(γ))Φ−1(α−δ)], |
ν(T,t)=(T−t)n−1[f(t,Xαt)+g(t,Xαt)Φ−1(α)], |
λ1(t,s)=f(t,Xt(γ))+g(t,Xt(γ))Cs(γ)−Ct(γ)s−t, |
μ1(t)=f(t,Xt(γ))+g(t,Xt(γ))Φ−1(α−δ), |
ν1(t)=f(t,Xαt)+g(t,Xαt)Φ−1(α). |
Due to the continuous nature of f and g, and the continuity of Xt(γ), we can infer that the composite functions λ, μ, ν and λ1, μ1, ν1 are also continuous with respect to t.
Since g(0,X0)>0 (regular condition), we have
μ(T,0)<ν(T,0). |
By the continuity of μ and ν, there exists a small number r>0 such that
μ(T,t)<ν(T,t),∀t∈[0,r]. |
By inequality (3.14), for any time t∈[0,r] and any time s∈(t,∞), we have
λ(T,t,s)=(T−t)n−1[f(t,Xt(γ))+g(t,Xt(γ))Cs(γ)−Ct(γ)s−t]<(T−t)n−1[f(t,Xt(γ))+g(t,Xt(γ))Φ−1(α−δ)=μ(T,t)<ν(T,t). |
By Lemma 3.1, it follows that
XT(γ)=X0+n−1∑k=1Tkk!Yk+1(n−1)![∫T0(T−t)n−1f(t,Xt(γ))dt+∫T0(T−t)n−1g(t,Xt(γ))dCt(γ)]=X0+n−1∑k=1Tkk!Yk+1(n−1)!limΔ→0k∑i=1λ(T,ti+1,ti)(ti+1−ti)≤X0+n−1∑k=1Tkk!Yk+1(n−1)!limΔ→0k∑i=1μ(T,ti)(ti+1−ti)=X0+n−1∑k=1Tkk!Yk+1(n−1)!∫T0μ(T,t)dt<X0+n−1∑k=1Tkk!Yk+1(n−1)!∫T0ν(T,t)dt=X0+n−1∑k=1Tkk!Yk+1(n−1)!∫T0(T−t)n−1f(t,Xαt)dt+1(n−1)!∫T0(T−t)n−1g(t,Xαt)Φ−1(α)dt=XαT |
for any time T∈(0,r].
We will prove Xt(γ)<Xαt for all t>r by contradiction.
Suppose there exists a time b>r at which Xt(γ) and Xαt first meet, i.e.,
Xb(γ)=Xαb,Xt(γ)<Xαt,∀t∈(0,b). |
Since f(b,Xαb)=f(b,Xb(γ)),g(b,Xαb)=g(b,Xb(γ))>0, and we have
μ1(b)<ν1(b). |
By the continuity of μ1 and ν1, there exists a time a∈(0,b) such that
μ1(t)<ν1(t),t∈[a,b]. |
By inequality (3.14), for any time t∈[a,b) and any time s∈(t,∞), we have
(b−t)n−1λ1(t,s)=(b−t)n−1[f(t,Xt(γ))+g(t,Xt(γ))Cs(γ)−Ct(γ)s−t]<(b−t)n−1[f(t,Xt(γ))+g(t,Xt(γ))Φ−1(α−δ)=(b−t)n−1μ1(t)<(b−t)n−1ν1(t). |
By a similar process as in Theorem 3.7, which indicates that the integral of a non-negative function with a positive interval is also positive, we have the following inequality:
Xb(γ)=X0+n−1∑k=1(b−a)kk!Yk+1(n−1)!∫ba(b−t)n−1f(t,Xt(γ))dt+1(n−1)!∫ba(b−t)n−1g(t,Xt(γ))dCt(γ)=X0+n−1∑k=1(b−a)kk!Yk+1(n−1)!limΔ→0k∑i=1(b−ti)n−1λ1(ti+1,ti)(ti+1−ti)≤X0+n−1∑k=1(b−a)kk!Yk+1(n−1)!limΔ→0k∑i=1(b−ti)n−1μ1(ti)(ti+1−ti)=X0+n−1∑k=1(b−a)kk!Yk+1(n−1)!∫ba(b−t)n−1μ1(t)dt<X0+n−1∑k=1(b−a)kk!Yk+1(n−1)!∫ba(b−t)n−1ν1(t)dt=X0+n−1∑k=1(b−a)kk!Yk+1(n−1)!∫ba(b−t)n−1f(t,Xαt)dt+1(n−1)!∫ba(b−t)n−1g(t,Xαt)Φ−1(α)dt=Xαb |
which is in contradiction with Xb(γ)=Xαb. Therefore,
Xt(γ)<Xαt,∀t>0. |
Since M{Λ1}=α, we have
M{Xt≤Xαt,∀t}≥α. | (3.16) |
Theorem 14.3 in [1] constructs an event Λ2 with M{Λ2}=1−α, and shows that for each γ∈Λ2, there exists a small number δ>0 such that
Cs(γ)−Ct(γ)s−t>Φ−1(α+δ) | (3.17) |
for any times s and t with s>t. Write
λ(T,t,s)=(T−t)n−1[f(t,Xt(γ))+g(t,Xt(γ))Cs(γ)−Ct(γ)s−t], |
μ(T,t)=(T−t)n−1[f(t,Xt(γ))+g(t,Xt(γ))Φ−1(α+δ)], |
ν(T,t)=(T−t)n−1[f(t,Xαt)+g(t,Xαt)Φ−1(α)], |
λ1(t,s)=f(t,Xt(γ))+g(t,Xt(γ))Cs(γ)−Ct(γ)s−t, |
μ1(t)=f(t,Xt(γ))+g(t,Xt(γ))Φ−1(α+δ), |
ν1(t)=f(t,Xαt)+g(t,Xαt)Φ−1(α). |
Since g(0,X0)>0, we have
μ(T,0)>ν(T,0). |
By the continuity of μ and ν, there exists a small number r>0 such that
μ(T,t)>ν(T,t),∀t∈[0,r]. |
By inequality (3.17), for any time t∈[0,r] and any time s∈(t,∞), we have
λ(T,t,s)=(T−t)n−1[f(t,Xt(γ))+g(t,Xt(γ))Cs(γ)−Ct(γ)s−t]>(T−t)n−1[f(t,Xt(γ))+g(t,Xt(γ))Φ−1(α+δ)=μ(T,t)>ν(T,t). |
Thus,
XT(γ)=X0+n−1∑k=1Tkk!Yk+1(n−1)!∫T0(T−t)n−1f(t,Xt(γ))dt+1(n−1)!∫T0(T−t)n−1g(t,Xt(γ))dCt(γ)=X0+n−1∑k=1Tkk!Yk+1(n−1)!limΔ→0k∑i=1(T−ti)n−1λ(T,ti+1,ti)(ti+1−ti)≥X0+n−1∑k=1Tkk!Yk+1(n−1)!limΔ→0k∑i=1(T−ti)n−1μ(T,ti)(ti+1−ti)=X0+n−1∑k=1Tkk!Yk+1(n−1)!∫T0μ(T,t)dt>X0+n−1∑k=1Tkk!Yk+1(n−1)!∫T0ν(T,t)dt=X0+n−1∑k=1Tkk!Yk+1(n−1)!∫T0(T−t)n−1f(t,Xαt)dt+1(n−1)!∫T0(T−t)n−1g(t,Xαt)Φ−1(α)dt=XαT |
for any time T∈(0,r].
We will prove Xt(γ)>Xαt for all t>r by contradiction.
Suppose there exists a time b>r at which Xt(γ) and Xαt first meet, i.e.,
Xb(γ)=Xαb,Xt(γ)>Xαt,∀t∈(0,b). |
Since f(b,Xαb)=f(b,Xb(γ)),g(b,Xαb)=g(b,Xb(γ))>0, we have
μ1(b)>ν1(b). |
By the continuity of μ1 and ν1, there exists a time a∈(0,b) such that
μ1(t)>ν1(t),t∈[a,b]. |
By inequality (3.17), for any time t∈[a,b] and any time s∈(t,∞), we have
(b−t)n−1λ1(t,s)=(b−t)n−1[f(t,Xt(γ))+g(t,Xt(γ))Cs(γ)−Ct(γ)s−t]>(b−t)n−1[f(t,Xt(γ))+g(t,Xt(γ))Φ−1(α+δ)=(b−t)n−1μ1(t)>(b−t)n−1ν1(t). |
Thus,
Xb(γ)=X0+n−1∑k=1(b−a)kk!Yk+1(n−1)!∫ba(b−t)n−1f(t,Xt(γ))dt+1(n−1)!∫ba(b−t)n−1g(t,Xt(γ))dCt(γ)=X0+n−1∑k=1(b−a)kk!Yk+1(n−1)!limΔ→0k∑i=1(b−t)n−1λ1(ti+1,ti)(ti+1−ti)≥X0+n−1∑k=1(b−a)kk!Yk+1(n−1)!limΔ→0k∑i=1(b−t)n−1μ1(ti)(ti+1−ti)=X0+n−1∑k=1(b−a)kk!Yk+1(n−1)!∫ba(b−t)n−1μ1(t)dt>X0+n−1∑k=1(b−a)kk!Yk+1(n−1)!∫ba(b−t)n−1ν1(t)dt=X0+n−1∑k=1(b−a)kk!Yk+1(n−1)!∫ba(b−t)n−1f(t,Xαt)dt+1(n−1)!∫ba(b−t)n−1g(t,Xαt)Φ−1(α)dt=Xαb, |
which is in contradiction with Xb(γ)=Xαb. Therefore,
Xt(γ)>Xαt,∀t>0. |
Since M{Λ2}=1−α, we have
M{Xt>Xαt,∀t}≥1−α. | (3.18) |
It follows from (3.16), (3.18) and
M{Xt≤Xαt,∀t}+M{Xt>Xαt,∀t}≤1 |
that
M{Xt≤Xαt,∀t}=α, |
M{Xt>Xαt,∀t}=1−α. |
hold.
Theorem 3.9. Let Xt and Xαt be the solution and α-path of the regular uncertain differential equation
dnXtdtn=f(t,Xt)+g(t,Xt)dCtdt. |
If the linear growth, Lipschitz and regular conditions hold, then at any time t>0, we have
M{Xt≤Xαt}=α, |
M{Xt>Xαt}=1−α. |
Proof. The proof follows a similar process to Theorem 3.5.
Theorem 3.10. Let Xt and Xαt be the solution and α-path of the uncertain differential equation
dnXtdtn=f(t,Xt)+g(t,Xt)dCtdt, |
respectively. If the linear growth, Lipschitz and regular conditions hold, then Xt has an inverse uncertainty distribution
Ψ−1t(α)=Xαt. |
Proof. The proof follows a similar process to Theorem 3.6.
Moving forward, we will provide examples to illustrate the significance of Condition (H).
Example 2. For the uncertain Duffing system, the equation is given by
md2Xtdt2+δdXtdt+αXt+βX3t−ζcosω(t)−γdCtdt=0. |
The initial values are Xt0(γ)=Xαt0=0.1. For convenience, the parameters involved are illustrated in Table 1.
Parameters | Interpretation | Value |
m | Mass | 1kg |
δ | Damping coefficient | 1Ns/m |
E | Elastic modulus | 1000Pa |
A | Cross-sectional area | 0.01m2 |
L | Length | 0.1m |
α | Linear stiffness coefficient | 1N/m |
β | Nonlinear stiffness coefficient | 1N/m3 |
ζ | Excitation coefficient | 1N |
ω | Excitation frequency | 1rads/s |
γ | Uncertain external excitation coefficient | 1Ns/m |
The uncertain external excitation represents the influence of climate on the object within the system. Figure 4 is a schematic diagram of the Duffing system, where the spring is a hardening spring.
We have
f(t,Xt,dXtdt)=cos(t)−dXtdt−Xt−X3t,g(t,Xt,dXtdt)=1. |
It is straightforward to deduce that
∂f(t,Xt,dXtdt)∂Xt=−1−3X2t<0,∂g(t,Xt,dXtdt)∂Xt=0. |
Upon observing Figure 5, it can be seen that the α-path for α=0.1 and α=0.2 intersect at time t1. The set of solutions Xαt derived from the family of ordinary differential equations cannot substitute for the inverse uncertainty distribution of the solution to the UDE.
For α<0, the spring is a softening spring, where β<0, the uncertain Duffing system is for
d2Xtdt2+dXtdt−Xt−X3t−cos(t)−dCtdt=0. |
The initial values are Xt(γ)|t=0=Xαt|t=0=0.1, dXt(γ)dt|t=0=dXαtdt|t=0=0.1. And we have
f(t,Xt,dXtdt)=cos(t)−dXtdt+Xt+X3t,g(t,Xt,dXtdt)=1. |
Verify condition (H),
∂f(t,Xt,dXtdt)∂Xt=1+3X2t>0,∂g(t,Xt,dXtdt)∂Xt=0. |
We define the region G∈[0,100]×[0,10]×[0,10]. It is straightforward to determine that the maximum value of the function f is denoted as M=1100, which satisfies the conditions provided in the remarks. Furthermore, as depicted in Figure 6, there are no intersecting α-path. Hence, the set of solutions Xαt derived from the family of ordinary differential equations corresponding to the α-path can be used to represent the inverse uncertainty distribution of the UDE.
Example 3. The function g represents a commonly utilized activation function within neural networks. Its second-order uncertain differential equation is given by
d2Xtdt2−dXtdt−Xt−X3t−cos(t)−11+exp(−Xt)dCtdt=0. |
The initial values are Xt(γ)|t=0=Xαt|t=0=0.1, dXt(γ)dt|t=0=dXαtdt|t=0=0.1, where
f(t,Xt,dXtdt)=cos(t)−dXtdt+Xt+X3t,g(t,Xt,dXtdt)=11+exp(−Xt), |
∂f(t,Xt,dXtdt)∂Xt=1+3X2t>0,∂g(t,Xt,dXtdt)∂Xt=exp(−Xt)(1+exp(−Xt))−2>0. |
We define the region G∈[0,100]×[0,10]×[0,10]. It is easy to establish that the maximum value of the functions f and g is M=1100, which meets the conditions specified in the notes. Additionally, as shown in Figure 7, there are no intersections among the α-paths, whether in the short term (a) or the long term (b). So the set of solutions Xαt derived from the α-path for a family of ordinary differential equations can be used to represent the inverse uncertainty distribution of the solution to the UDE.
Example 4.
d5Xtdt5−sin(t)−Xt−dCtdt=0. |
The initial values are
Xt(γ)|t=0=Xαt|t=0=0.1,X(k)t(γ)|t=0=Xα(k)t|t=0=0.1,k=1,2,…,5. |
f(t,Xt)=s(t)+Xt,g(t,Xt)=1. |
We define the region G∈[0,100]×[0,10]. It is easy to establish that the maximum value of the function f is M=11, which meets the conditions specified in the notes. The α-path diagram is depicted in Figure 8, from which it is observable that the individual α-path do not intersect at any point in time. Thus, the set of solutions Xαt derived from the α-path for a family of ordinary differential equations can be used to represent the inverse uncertainty distribution of the UDE.
The research presented in this paper is of significant importance and has broad applicability in practical applications. If the correct inverse uncertainty distribution of the solutions to UDEs cannot be obtained, it would be impossible to delve into the study of the behavior of UDEs and their solutions. Consequently, the construction of such UDE models would be meaningless.
This paper presents for the first time the inverse uncertainty distribution for second-order and a class of higher-order UDEs. (1) For second-order UDEs, if the conditions (H) are met and the linear growth, Lipschitz and regular conditions hold, we can determine the inverse uncertainty distribution of the second-order UDEs. (2) For a class of higher-order UDEs, if the linear growth, Lipschitz and regular conditions hold, we can similarly ascertain the inverse uncertainty distribution of the second-order UDEs. Based on the theorems presented in this paper, it is possible to conduct uncertain dynamical analyses of these types of UDEs, which constitutes a foundational work in the field of uncertainty theory. It is undeniable that these conditions have certain limitations. Scholars can continue to explore the statistical properties and other applications of the solutions to UDEs to potentially relax these constraints.
Based on our research, there are three areas for potential future exploration: (1) It merits investigation whether the sufficient conditions for determining the inverse unncertainty distribution of solutions to second-order or higher-order UDEs can be expanded and whether there exist necessary and sufficient conditions. (2) While uncertainty theory and this paper discuss the transition from ordinary differential equations to UDEs, our research group is currently working on more complex equations, such as uncertain fractional-order differential equations and uncertain functional differential equations with constant delays. We have conceived a potential sufficient condition for the α-paths of systems of UDEs to determine the inverse uncertainty distribution of their solutions. Additionally, uncertain partial differential equations also warrant investigation. (3) There is a multitude of differential equations that satisfy our conditions, with numerous examples provided in the final version under submission. On this basis, more in-depth issues can be explored, such as uncertain dynamics, uncertain bifurcation, and uncertain chaos.
Zeman Wang: Conceptualization, Data curation, Formal analysis, Investigation, Methodology, Software, Validation, Writing–original draft, Writing–review & editing; Qiubao Wang: Conceptualization, Funding acquisition, Project administration, Supervision, Validation, Writing–review & editing; Zhong Liu: Data curation, Formal analysis; Zikun Han: Data curation, Investigation; Xiuying Guo: Investigation, Supervision. All authors have read and approved the final version of the manuscript for publication.
This work was supported by the Innovation and Entrepreneurship Curriculum Construction in Hebei Province: Ordinary Differential Equations; Key Project of Natural Science Foundation of Hebei Province (Basic Discipline Research) (A2023210064).
All authors declare no conflict of interest that could affect the publication of this paper.
[1] | B. Liu, Uncertainty theory, 2 Eds., Berlin: Springer Berlin Heidelberg, 2007. |
[2] |
K. Yao, X. Chen, A numerical method for solving uncertain differential equations, J. Intell. Fuzzy Syst., 25 (2013), 825–832. http://doi.org/10.3233/IFS-120688 doi: 10.3233/IFS-120688
![]() |
[3] |
B. Liu, Toward uncertain finance theory, J. Uncertain. Anal. Appl., 1 (2013), 1. https://doi.org/10.1186/2195-5468-1-1 doi: 10.1186/2195-5468-1-1
![]() |
[4] |
L. Sheng, Y. Zhu, Optimistic value model of uncertain optimal control, Int. J. Uncert. Fuzz. Knowledge-Based Syst., 21 (2013), 75–87. https://doi.org/10.1142/S0218488513400060 doi: 10.1142/S0218488513400060
![]() |
[5] |
Z. Zhang, X. Yang, Uncertain population model, Soft Comput., 24 (2020), 2417–2423. https://doi.org/10.1007/s00500-018-03678-6 doi: 10.1007/s00500-018-03678-6
![]() |
[6] |
Z. Liu, X. Yang, A linear uncertain pharmacokinetic model driven by Liu process, Appl. Math. Model., 89 (2021), 1881–1899. https://doi.org/10.1016/j.apm.2020.08.061 doi: 10.1016/j.apm.2020.08.061
![]() |
[7] |
Z. Liu, R. Kang, Pharmacokinetics with intravenous infusion of two-compartment model based on Liu process, Commun. Statistics-Theory Meth., 53 (2024), 4975–4990. https://doi.org/10.1080/03610926.2023.2198626 doi: 10.1080/03610926.2023.2198626
![]() |
[8] |
Z. Li, Y. Sheng, Z. Teng, H. Miao, An uncertain differential equation for SIS epidemic model, J. Intell. Fuzzy Syst., 33 (2017), 2317–2327. https://doi.org/10.3233/JIFS-17354 doi: 10.3233/JIFS-17354
![]() |
[9] |
C. Tian, T. Jin, X. Yang, Q. Liu, Reliability analysis of the uncertain heat conduction model, Comput. Math. Appl., 119 (2022), 131–140. https://doi.org/10.1016/j.camwa.2022.05.033 doi: 10.1016/j.camwa.2022.05.033
![]() |
[10] |
X. Yang, K. Yao, Uncertain partial differential equation with application to heat conduction, Fuzzy Optim. Decis. Making, 16 (2017), 379–403. https://doi.org/10.1007/s10700-016-9253-9 doi: 10.1007/s10700-016-9253-9
![]() |
[11] |
K. Zhang, B. Liu, Higher-order derivative of uncertain process and higher-order uncertain differential equation, Fuzzy Optim. Decis. Making, 23 (2024), 295–318. https://doi.org/10.1007/s10700-024-09422-0 doi: 10.1007/s10700-024-09422-0
![]() |
[12] | B. Liu, Uncertainty theory, 5 Eds., Berlin: Springer, 2024. |
[13] | B. Liu, Uncertainty theory, 3 Eds., Berlin: Springer, 2010. |
[14] | B. Liu, Some research problems in uncertainty theory, J. Uncertain Syst., 3 (2009), 3–10. |
[15] |
X. Chen, D. A. Ralescu, Liu process and uncertain calculus, J. Uncertain. Anal. Appl., 1 (2013), 3. https://doi.org/10.1186/2195-5468-1-3 doi: 10.1186/2195-5468-1-3
![]() |
[16] |
T. Ye, A rigorous proof of fundamental theorem of uncertain calculus, J. Uncertain Syst., 14 (2021), 2150009. https://doi.org/10.1142/S1752890921500094 doi: 10.1142/S1752890921500094
![]() |
[17] | B. Liu, Uncertainty theory, 4 Eds., Berlin: Springer Berlin Heidelberg, 2015. |
[18] |
L. Jia, W. Dai, Uncertain spring vibration equation, J. Indust. Manage. Optim., 18 (2022), 2401. https://doi.org/10.3934/jimo.2021073 doi: 10.3934/jimo.2021073
![]() |
[19] |
A. Shekhovtsov, Higher order maximum persistency and comparison theorems, Comput. Vision Image Underst., 143 (2016), 54–79. https://doi.org/10.1016/j.cviu.2015.05.002 doi: 10.1016/j.cviu.2015.05.002
![]() |
[20] | W. Walter, Ordinary differential equations, Berlin: Springer Science & Business Media, 2013. |
1. | Peng E, Tingting Xu, Linhua Deng, Yulin Shan, Miao Wan, Weihong Zhou, Solutions of a class of higher order variable coefficient homogeneous differential equations, 2025, 20, 1556-1801, 213, 10.3934/nhm.2025011 |
Parameters | Interpretation | Value |
m | Mass | 1kg |
δ | Damping coefficient | 1Ns/m |
E | Elastic modulus | 1000Pa |
A | Cross-sectional area | 0.01m2 |
L | Length | 0.1m |
α | Linear stiffness coefficient | 1N/m |
β | Nonlinear stiffness coefficient | 1N/m3 |
ζ | Excitation coefficient | 1N |
ω | Excitation frequency | 1rads/s |
γ | Uncertain external excitation coefficient | 1Ns/m |
Parameters | Interpretation | Value |
m | Mass | 1kg |
δ | Damping coefficient | 1Ns/m |
E | Elastic modulus | 1000Pa |
A | Cross-sectional area | 0.01m2 |
L | Length | 0.1m |
α | Linear stiffness coefficient | 1N/m |
β | Nonlinear stiffness coefficient | 1N/m3 |
ζ | Excitation coefficient | 1N |
ω | Excitation frequency | 1rads/s |
γ | Uncertain external excitation coefficient | 1Ns/m |