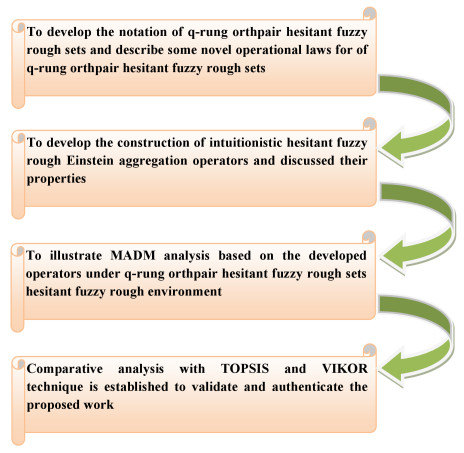
Environmental science and pollution research has benefits around the globe. Human activity produces more garbage throughout the day as the world's population and lifestyles rise. Choosing a garbage disposal site (GDS) is crucial to effective disposal. In illuminated of the advancements in society, decision-makers concede a significant challenge for assessing an appropriate location for a garbage disposal site. This research used a multi-attribute decision-making (MADM) approach based on q-rung orthopair hesitant fuzzy rough (q-ROHFR) Einstein aggregation information for evaluating GDS selection schemes and providing decision-making (DM) support to select a suitable waste disposal site. In this study, first, q-ROHFR Einstein average aggregation operators are integrated. Some intriguing characteristics of the suggested operators, such as monotonicity, idempotence and boundedness were also explored. Then, a MADM technique was established using the novel concept of q-ROHFR aggregation operators under Einstein t-norm and t-conorm. In order to help the decision makers (DMs) make a final choice, this technique aims to rank and choose an alternative from a collection of feasible alternatives, as well as to propose a solution based on the ranking of alternatives for a problem with conflicting criteria. The model's adaptability and validity are then demonstrated by an analysis and solution of a numerical issue involving garbage disposal plant site selection. We performed a the sensitivity analysis of the proposed aggregation operators to determine the outcomes of the decision-making procedure. To highlight the potential of our new method, we performed a comparison study using the novel extended TOPSIS and VIKOR schemes based on q-ROHFR information. Furthermore, we compared the results with those existing in the literature. The findings demonstrate that this methodology has a larger range of information representation, more flexibility in the assessment environment, and improved consistency in evaluation results.
Citation: Attaullah, Asghar Khan, Noor Rehman, Fuad S. Al-Duais, Afrah Al-Bossly, Laila A. Al-Essa, Elsayed M Tag-eldin. A novel decision model with Einstein aggregation approach for garbage disposal plant site selection under q-rung orthopair hesitant fuzzy rough information[J]. AIMS Mathematics, 2023, 8(10): 22830-22874. doi: 10.3934/math.20231163
[1] | Attaullah, Shahzaib Ashraf, Noor Rehman, Asghar Khan, Muhammad Naeem, Choonkil Park . Improved VIKOR methodology based on q-rung orthopair hesitant fuzzy rough aggregation information: application in multi expert decision making. AIMS Mathematics, 2022, 7(5): 9524-9548. doi: 10.3934/math.2022530 |
[2] | Attaullah, Shahzaib Ashraf, Noor Rehman, Asghar Khan, Choonkil Park . A decision making algorithm for wind power plant based on q-rung orthopair hesitant fuzzy rough aggregation information and TOPSIS. AIMS Mathematics, 2022, 7(4): 5241-5274. doi: 10.3934/math.2022292 |
[3] | Jia-Bao Liu, Rashad Ismail, Muhammad Kamran, Esmail Hassan Abdullatif Al-Sabri, Shahzaib Ashraf, Ismail Naci Cangul . An optimization strategy with SV-neutrosophic quaternion information and probabilistic hesitant fuzzy rough Einstein aggregation operator. AIMS Mathematics, 2023, 8(9): 20612-20653. doi: 10.3934/math.20231051 |
[4] | Muhammad Saqlain, Xiao Long Xin, Rana Muhammad Zulqarnain, Imran Siddique, Sameh Askar, Ahmad M. Alshamrani . Energy supplier selection using Einstein aggregation operators in an interval-valued q-rung orthopair fuzzy hypersoft structure. AIMS Mathematics, 2024, 9(11): 31317-31365. doi: 10.3934/math.20241510 |
[5] | Adel Fahad Alrasheedi, Jungeun Kim, Rukhsana Kausar . q-Rung orthopair fuzzy information aggregation and their application towards material selection. AIMS Mathematics, 2023, 8(8): 18780-18808. doi: 10.3934/math.2023956 |
[6] | Sumbal Ali, Asad Ali, Ahmad Bin Azim, Ahmad ALoqaily, Nabil Mlaiki . Averaging aggregation operators under the environment of q-rung orthopair picture fuzzy soft sets and their applications in MADM problems. AIMS Mathematics, 2023, 8(4): 9027-9053. doi: 10.3934/math.2023452 |
[7] | Yasir Yasin, Muhammad Riaz, Kholood Alsager . Synergy of machine learning and the Einstein Choquet integral with LOPCOW and fuzzy measures for sustainable solid waste management. AIMS Mathematics, 2025, 10(1): 460-498. doi: 10.3934/math.2025022 |
[8] | Sumbal Ali, Asad Ali, Ahmad Bin Azim, Abdul Samad Khan, Fuad A. Awwad, Emad A. A. Ismail . TOPSIS method based on q-rung orthopair picture fuzzy soft environment and its application in the context of green supply chain management. AIMS Mathematics, 2024, 9(6): 15149-15171. doi: 10.3934/math.2024735 |
[9] | Ghous Ali, Kholood Alsager, Asad Ali . Novel linguistic q-rung orthopair fuzzy Aczel-Alsina aggregation operators for group decision-making with applications. AIMS Mathematics, 2024, 9(11): 32328-32365. doi: 10.3934/math.20241551 |
[10] | Ahmad Bin Azim, Ahmad ALoqaily, Asad Ali, Sumbal Ali, Nabil Mlaiki, Fawad Hussain . q-Spherical fuzzy rough sets and their usage in multi-attribute decision-making problems. AIMS Mathematics, 2023, 8(4): 8210-8248. doi: 10.3934/math.2023415 |
Environmental science and pollution research has benefits around the globe. Human activity produces more garbage throughout the day as the world's population and lifestyles rise. Choosing a garbage disposal site (GDS) is crucial to effective disposal. In illuminated of the advancements in society, decision-makers concede a significant challenge for assessing an appropriate location for a garbage disposal site. This research used a multi-attribute decision-making (MADM) approach based on q-rung orthopair hesitant fuzzy rough (q-ROHFR) Einstein aggregation information for evaluating GDS selection schemes and providing decision-making (DM) support to select a suitable waste disposal site. In this study, first, q-ROHFR Einstein average aggregation operators are integrated. Some intriguing characteristics of the suggested operators, such as monotonicity, idempotence and boundedness were also explored. Then, a MADM technique was established using the novel concept of q-ROHFR aggregation operators under Einstein t-norm and t-conorm. In order to help the decision makers (DMs) make a final choice, this technique aims to rank and choose an alternative from a collection of feasible alternatives, as well as to propose a solution based on the ranking of alternatives for a problem with conflicting criteria. The model's adaptability and validity are then demonstrated by an analysis and solution of a numerical issue involving garbage disposal plant site selection. We performed a the sensitivity analysis of the proposed aggregation operators to determine the outcomes of the decision-making procedure. To highlight the potential of our new method, we performed a comparison study using the novel extended TOPSIS and VIKOR schemes based on q-ROHFR information. Furthermore, we compared the results with those existing in the literature. The findings demonstrate that this methodology has a larger range of information representation, more flexibility in the assessment environment, and improved consistency in evaluation results.
Abbreviation | Description |
MAGDM | Multi-attribute group decision making |
MADM | Multi-attribute decision making |
FS | Fuzzy set |
IFS | Intuitionistic fuzzy set |
PFS | Pythagorean fuzzy set |
q-ROFS | q-rung orthopair fuzzy set |
DMs | Decision-makers |
AOps | Aggregation operators |
q-ROHFRS | q-rung orthopair hesitant fuzzy rough set |
In the current economic and social management system, we need to deal with numerous MADM challenges. Many researchers in the fields of operations research and decision sciences have taken keen interest in MADM theories and techniques, and their noteworthy contributions have been acknowledged [1,2,3,4,5,6,7,8]. Choosing a suitable GDS is one of the most important steps for proper disposal of rubbish. Garbage has risen to be a real problem for international society, causing an increasing hazard to human health [9]. Garbage disposal which is not harmful to the environment and the conversion of garbage into money have become vital aspects for each community in planning and executing sustainable growth and maintaining an intellectual atmosphere [10]. At the start of the twenty-first century, the worldwide average annual waste growth rate has accelerated to 8.42 %, while in developing regions, the figure has even reached 10% [11]. The implementation of waste disposal systems begins with the identification of a location. The choice of selecting a GDS is complicated because so many elements are taken into consideration, such as the local economy, climate, transport services, ground hydrological factors, and geological conditions in the environment. In practical DM challenges, several influencing circumstances, such as lack of understanding of DMs, tight schedules, and restricted budgets, prevent DMs from providing correct assessment values of alternatives with regard to qualities [12]. Imprecise assessments play a crucial role in DM techniques in these situations. It can be seen that the MADM approaches have been used in the assessment of the GDS selection scheme. However, most existing studies were conducted using Zadeh's fuzzy sets (FSs) [13] and Attansov's intuitionistic fuzzy sets (IFSs) [14]. Existing research of IFSs-based MAGDM approaches has demonstrated the effectiveness and efficiency of IFSs in coping with challenging assessment schemes presented by DMs [15]. Afterwards, Yager [16] suggested a novel extension of IFSs called Pythagorean FSs (PFSs). The constraint of IFSs is that the sum of membership and nonmembership degree must be less than or equal to 1, while PFSs fulfill the constraint that the sum of the square of membership and nonmembership degree must not be greater than 1. Obviously, PFSs provide a wider range of information than IFSs, and PFS-based MADM methodologies have emerged as a novel and dynamic research field in comparison to IFSs [17]. Despite their capability to deal with complex fuzzy information in certain situations in real life, it is found that both IFSs and PFSs still have some shortcomings. Later on, Yager [18] introduced the notion of a q-rung orthopair fuzzy (q-ROF) set, which is an extension of the conventional IFS. The q-ROF set has the constraint that the sum of the qth-powers of membership and non-membership degree must be less than or equal to 1.
There are several situations in which DMs have strong opinions regarding evaluating or assessing plans, initiatives or governmental statements issued by a government. For example, let an organization start a huge construction project in order to demonstrate its success and performance. Members of the organization may attribute favorable membership (β=0.9) to their initiative, whereas others may assess the same exertion as a wastage of resources and strive to invalidate it by providing fiercely contrasting perspectives. Therefore, they ascribe a negative membership (ψ=0.7). In this situation, βϝ(ℓ)+ψϝ(ℓ)>1, and (βϝ(ℓ))2+(ψϝ(ℓ))2>1, but (βϝ(ℓ))q+(ψϝ(ℓ))q<1 for q>3. Therefore, (β,ψ) is a q-rung orthopair fuzzy number (q-ROFN) rather than an intuitionistic fuzzy number (IFN) or a Pythagorean fuzzy number (PFN). Thus, Yager's q-ROFNs are effective in addressing information imprecision. The disadvantages of the aforementioned information make evaluating the selection process under such complicated information complex. Zhang et al. [19] integrated q-ROFSs into a multi-granular three-way decision framework and explored a unique MADM technique in q-ROF information systems. Zhang et al. [20] investigated a fuzzy intelligence learning technique based on limited rationality in internet of medical things systems, giving a suitable scheme for biomedical data processing. Zhang et al. [21] investigated MADM with imprecise and incomplete information in incomplete q-ROF information systems using multi-granulation probabilistic models, MULTIMOORA (multi-objective optimisation by ratio analysis plus the full multiplicative form) and the technique of precise order preference. For MADM, Hussain et al. [22] discussed a covering-based q-rung orthopair fuzzy rough set (q-ROFRS) model hybrid with technique for order preference by similarity to ideal solution (TOPSIS) approach. Chakraborty [23] conducted thorough simulation-based comparisons and mathematical analyses of TOPSIS and modified TOPSIS approaches in order to clear up incorrect assumptions about their applications in MADM resolving issues. Hanine et al. [24] proposed and explored the use of two well-known MCDM approaches, the analytic hierarchy process and TOPSIS methodologies, in decision-making situations. Gupta et al. [25] introduced a trapezoidal IF number-based decision approach for MADM challenges, including plant site selection. They additionally applied the VIKOR technique based on IF environment. A VIKOR approach was proposed by Hafezalkotob et al. [26] with both interval target values of attributes and interval ratings of alternatives on attributes. Additionally, they sought to use the power of interval estimations to reduce the degeneration of undetermined data. Soner et al. [27] employed the analytic hierarchy process and the VIKOR scheme to develop a complete framework for dealing with uncertainty during linguistic evaluation of decision-makers in marine transportation enterprises. Gul et al. [28] carried out an innovative literature review in order to categorize, analyze and evaluate current research on VIKOR applications. They also explored the applications of the VIKOR approach in fuzzy settings. Opricovi et al. [29] enhanced the VIKOR approach with a stability analysis identifying the weight stability intervals and with a trade-offs analysis, demonstrating its application in MCDM challenges with conflicting and non-commensurable criteria.
Several approaches have been presented to address data uncertainty. The rough set (RS) theory, proposed by Pawlak [30] in 1982, is a non-statistical mathematical technique for dealing with the difficulties of ambiguity and uncertainty. This theory is based on associating a subset with two crisp sets known as lower and upper approximations, which are used to establish the subset's boundary region and accuracy measure [31]. Several extensions to the RS concept have been explored. Fuzzy-rough set (FRS) theory and RS theory may be integrated to organize information with continuous features and evaluate information discrepancies. The FRS approach has proven to be extremely beneficial in a wide range of application fields because it is an appropriate approach for evaluating inconsistent and conflicting information. The FRS theory, introduced in [34], is an enhancement of the RS theory that can support information with continuous numerical properties. The importance of FRS theory is highlighted in a variety of applications. Pan et al. [35] modified the fuzzy preference relation RS framework with an additive consistent fuzzy preference relation. Li et al. [36] proposed an effective FRS technique for feature evaluation. The problem of continuous observer design for integer order nonlinear systems has received a lot of consideration in the literature. However, there are a couple of investigations that are directly connected to granular computing theory in order to better estimate system states. Zhan [37] discussed the granular function description and provided a unique impulsive observer design technique to assure error dynamic system cognitive convergence. The derived criteria, in particular, accurately captured the relationship between the fractional order and the pulse signal. A numerical simulation was presented to demonstrate the efficacy of the suggested approach. Ren et al. [38] investigated logical systems with mixed decision implications. The mixed decision implications semantical system was designed to express and originate acceptable mixed decision implications while avoiding contradicting mixed decision implications. The syntactical system introduced mixed augmentation and mixed combination, and the soundness, completeness, and non-redundancy of these two inference rules were demonstrated. Lian et al. [39] investigated the concept of relative knowledge distances in light of relative cognitive principles. Then in the context of precise and fuzzy settings, they depicted the transformation challenges between any two types of knowledge given the condition of specific knowledge, and they further provided the newly possessed features due to the increase of relative knowledge distances and the refinement of conditional knowledge granularities, which can well reflect progressive features. Feng et al. [40] minimized multi-granulation by using uncertainty measures based on FRSs by minimizing both the negative and positive regions. Sun et al. [41] employed a constructive approach to generate three varieties of multi-granulation FRSs over two universes. Zhang et al. [42] discussed the FRS theory for feature selection based on information entropy. Based on FRS theory, Vluymans et al. [43] proposed a novel type of classification for unbalanced multi-instance data. Wang and Hu [44] proposed new L-FRSs to generalize the concept of L-FRSs. Zhang et al. [45] suggested the dual hesitant FRS and its application. Peng et al. [46] suggested an interactive fuzzy linguistic term set to represent interactive information in a MADM problem. The geometric features and benefits of the interactive fuzzy linguistic term set for decision information consistency were examined. Numerical examples illustrated its application to a MADM problem using interactive information, which may enhance decision outcomes and improve artificial intelligence. An improved evaluation based on the distance from average solution (EDAS) of the interval-valued intuitionistic trapezoidal fuzzy set was proposed by Peng et al. [47]. They provided a numerical example of flood disaster rescue to demonstrate the feasibility and efficacy of the suggested strategy, which was also compared to current methods. Tang et al. [48] introduced a decision-theoretic RS model using q-ROF information with their applications in stock investment assessment. For three-way decisions under GDM, Liang et al. [49] suggested q-ROFSs on decision-theoretic FRSs. Zhang et al. [50] proposed GDM using incomplete q-ROF preference relations. Peng et al. [51] suggested similarity measures for linguistic q-ROF MADM using a projection technique and presented a numerical example to demonstrate its feasibility and efficacy by comparing it to existing approaches. Sensitivity and stability analyses of the suggested approach were also provided. These q-ROFRS extensions have been demonstrated to be beneficial in resolving DM evaluation values in MADM challenges in applications.
In recent years, researchers have significantly merged the MADM technique with uncertainty theories in response to the rising ambiguity and the complexity of real-world problems. Motivated by the above description, existing research piqued our interest in investigating the generalization of FRS theory to propose a substantial information representation. The innovative features of generalized RS theory addressed the limitations of traditional fuzzy set theory extensions such as FS, IFS, PFS, q-ROFS, LDFS, and many more. Several approaches to fuzzy generalisations of RS theory have been developed and implemented. Considering situations where experts hesitate among several evaluation values, q-rung orthopair hesitant FRSs (q-ROHFRSs), as a new generalizations of intuitionistic fuzzy rough sets, can describe uncertain information flexibly in the decision-making process. The q-ROHFRSs, a hybrid intelligent structure of rough sets, and q-rung orthopair hesitant fuzzy sets (q-ROHFSs) are advanced classification strategies that have captured our interest in working with ambiguous and incomplete data. The q-ROHFRSs improves on traditional models that utilize the value of lower and upper approximations with membership and non- membership from the unit interval to represent the ambiguity of real world challenges, which can effectively describe the uncertainty of complicated problems and the vagueness of human cognition. In accordance with the assessments, aggregation operators (AOps) play a key role in DM in collecting information from diverse sources into a single value. According to existing knowledge, the establishment of AOps with the hybridization of q-ROHFS with RS is not found in the q-ROF information. As a result, the current q-ROHF rough structure has captivated our attention, and we construct a list of algebraic AOps based on rough information, such as q-ROHF weighted averaging (WA), order WA and hybrid WA operators, using the algebraic t-norm and t-conorm.
In light of the above research motivations, this paper enhances the existing literature of FRS theory by introducing novel ideas. The following describes the major contributions of the present work.
(ⅰ) We establish novel q-ROHFRS concepts and examine their fundamental operational rules.
(ⅱ) To construct AOps, q-ROHFREWA, q-ROHFREOWA and q-ROHFREHWA operators, based on Einstein's t-norm and t-conorm and thoroughly investigate their associated features.
(ⅲ) To establish a DM approach based on the specified aggregation operators to synthesize ambiguous information in real-world DM challenges.
(ⅳ) Carried out the sensitivity analysis of the proposed aggregation operators with decision-making outcomes.
(ⅴ) A real-world case study with DM complications in GDS selection assessment has been established.
(ⅵ) The outcomes have been compared to those demonstrated in the available the scientific literature.
(ⅶ) Finally, enhanced q-ROHFR-TOPSIS and VIKOR approaches are employed, and we compare the findings obtained through the suggested operators to validate the proposed DM methodology. The main contributions of the study are summarized in Figure 1.
The remainder of this article is outlined as follows: Section 2 briefly explores several fundamental concepts of IFSs, q-ROFSs and RS theory. In Section 3, an innovative notion of q-ROHFRSs is described together with their fundamental relevant operating rules. Section 4 displays several Einstein AOps that are constructed to aggregate ambiguous information and are based on Einstein operational laws. Section 5 is devoted to a DM approach based on the established AOps. Section 6 provides a numerical example of the assessment of site selection for garbage disposal site selection. This part also discusses the application of the established approach. The sensitivity analysis of ranking order for different values of parameters is presented in Section 7. Section 8 addresses the comparison of suggested AOps with previous outcomes and the enhanced q-ROHFR-TOPSIS and VIKOR methods for evaluating the proposed AOps-based MADM methodology. This manuscript is concluded at Section 9.
In this section, we provide some relevant fundamental information, such as IFS, q-ROFS, q-ROHFS, RS and q-ROFRS, and some related operational laws, which are listed below. These core concepts will assist readers in comprehending the proposed framework.
Definition 1. [14] Let U={∝1,∝2,∝3,...,∝n} be a universal set, an IFS ϝ over U is described by
ϝ={⟨∝ı,βϝ(∝ı),ψϝ(∝ı)⟩|∝ı∈U}. |
For each ∝ı∈U, the functions βϝ:U→[0, 1] and ψϝ:U→[0, 1] symbolize the membership grade (MG) and non-membership grade (NMG), which must fulfill the property 0≤βϝ(∝ı)+ψϝ(∝ı)≤1.
Definition 2. [18] Let U={∝1,∝2,∝3,...,∝n} be a universal set, a q-ROFS F over U is defined by
F={⟨∝ı,βF(∝ı),ψF(∝ı)⟩|∝ı∈U}. |
For each ∝ı∈U, the functions βF:U→[0, 1] and ψF:U→[0, 1] signify the MG and NMG, which must satisfy the property that (ψF(∝ı))q+(βF(∝ı))q≤1,(q>2∈Z). Figure 2 depicts a diagrammatic portrayal of an IFS and q-ROFSs (q=1−5).
Definition 3. [58] Let U={∝1,∝2,∝3,...,∝n} be a universal set, a q-ROHFS F is defined by
F={⟨∝ı,βhF(∝ı),ψhF(∝ı)⟩|∝ı∈U}, |
where βhF(∝ı) and ψhF(∝ı) are sets of some values in [0,1] symbolizing the MG and NMG, respectively. Which must satisfy the following properties: ∀∝ı∈U, ∀ϖF(∝ı)∈βhF(∝ı), ∀νF(∝ı)∈ψhF(∝ı) with (max(βhF(∝ı)))q+(max(ψhF(∝ı)))q≤1. We utilize a pair F= (βhF,ψhF) for simplicity to mean a q-ROHF number.
Definition 4. [58] Let F1=(βhF1,ψhF1) and F2=(βhF2,ψhF2) be two q-ROHFNs. The fundamental set theoretic operations are as follows:
(1) F1⊕F2=∪μ1∈ψhF1,μ2∈ψhF2ν1∈βhF1,ν2∈βhF2{((μ1)q+(μ2)q−(μ1)q⋅(μ2)q)1q,(ν1⋅ν2)};
(2) F1⊗F2=∪μ1∈ψhF1,μ2∈ψhF2ν1∈βhF1,ν2∈βhF2{(μ1⋅μ2),((ν1)q+(ν2)q−(ν1)q⋅(ν2)q)1q};
(3) γ⋅F1=∪μ1∈ψhF1,ν1∈βhF1{(1−(1−μq1)γ)1q,(ν1)γ};
(4) (F1)γ=∪μ1∈ψhF1,ν1∈βhF1{(μ1)γ,(1−(1−νq1)γ)1q};
(5) Fc1={ψhF1,βhF1}.
Definition 5. Let F1=(βhF1,ψhF1) and F2=(βhF2,ψhF2) be two q-ROHFNs, q>2 and γ>0 be any real number. The fundamental operations based on Einstein's t-norm and t-conorm are described in the following:
(1) F1⨁F2={∪μ1∈βhF1μ2∈βhF2{q√μq1+μq2q√1+μq1.μq2},∪ν1∈ψhF1ν2∈ψhF2{ν1.ν2q√1+(1−νq1).(1−νq2)}};
(2) F1⨂F2={∪μ1∈βhF1μ2∈βhF2{μ1.μ2q√1+(1−μq1).(1−μq2)},∪ν1∈ψhF1ν2∈ψhF2{q√νq1+νq2q√1+νq1.νq2}};
(3) F∧γ1={∪μ1∈βhF1{q√2(μq1)γq√(2−μq1)γ+(μq1)γ}, ∪ν1∈ψhF1{q√(1+νq1)γ−(1−νq1)γq√(1+νq1)γ+(1−νq1)γ}};
(4) γ.F1={∪μ1∈βhF1{q√(1+μq1)γ−(1−μq1)γq√(1+μq1)γ+(1−μq1)γ},∪ν1∈ψhF1{q√2(νq1)γq√(2−νq1)γ+(νq1)γ}}.
Definition 6. Let U={∝1,∝2,∝3,...,∝n} be a universal set and ℶ⊆U×U be a (crisp) relation. Then,
(1)The relation ℶ is reflexive iff ∝ıℶ∝ı for all ∝ı∈U
(2)The relation ℶ is symmetric if ∝ıℶ∝j then ∝jℶ∝ı for all ∝ı,∝j∈U
(3)The relation ℶ is transitive if ∝ıℶ∝j and ∝jℶ∝k implies ∝ıℶ∝k∀∝ı,∝j,∝k∈U.
Definition 7. [32] Let U={∝1,∝2,∝3,...,∝n} be a universal set and ℶ be any relation on U. Define ℶ∗:U→M(U) by ℶ∗(∝)={φ∈U|(∝,φ)∈ℶ}, for ∝∈U where ℶ∗(∝) is known as a successor neighborhood of the element ∝ with respect to the relation ℶ. The pair (U,ℶ) is called a (crisp) approximation space. Now, for any set £⊆U, the lower approximation (LA) and upper approximation (UA) of ∝ with respect to approximation space (U,ℶ) are defined as
ℶ_(£)={∝∈U|ℶ∗(∝)⊆£},¯ℶ(£)={∝∈U|ℶ∗(∝)∩£≠ϕ}. |
The pair (ℶ_(£),¯ℶ(£)) is called a rough set if ℶ_(£)≠¯ℶ(£) and both ℶ_(£),¯ℶ(£):M(U)→M(U) are LA and UA operators.
Definition 8. Let U={∝1,∝2,∝3,...,∝n} be a universal set, and ℶ∈IFS(U×U) be an IF relation. Then,
(1) ℶ is reflexive if μℶ(∝ı,∝ı)=1 and νℶ(∝ı,∝ı)=0,∀∝ı∈U,
(2) ℶ is symmetric if ∀(∝ı,∝ȷ)∈U×U, μℶ(∝ı,∝κ)=μℶ(∝ȷ,∝ı) and νℶ(∝ı,∝κ)=νℶ(∝κ,∝ı);
(3) ℶ is transitive if ∀(∝ı,∝ȷ)∈U×U,
μℶ(∝ı,∝κ)≥⋁∝ȷ∈U[μℶ(∝ı,∝ȷ)∧μℶ(∝ȷ,∝κ)]; |
and
νℶ(∝ı,∝κ)=⋀∝ȷ∈U[νℶ(∝ı,∝ȷ)∧νℶ(∝ȷ,∝κ)]. |
Definition 9. [18] Let U={∝1,∝2,∝3,...,∝n} be a universal set. Then, any ℶ∈q−RFS(U×U) is called a q-rung relation. The pair (U×ℶ) is said to be a q-rung approximation space. Now, for any T⊆q−RFS(U), the LA and UA of T with respect to q-RF approximation space (U,ℶ) are two q-RFSs, which are represented by ¯ℶ(T) and ℶ_(T) and characterized as
¯ℶ(T)={⟨∝ı,μ¯ℶ(T)(∝ı),ν¯ℶ(T)(∝ı)⟩|∝ı∈U},ℶ_(T)={⟨∝ı,μℶ_(T)(∝ı),νℶ_(T)(∝ı)⟩|∝ı∈U}, |
where
μ¯ℶ(T)(∝ı)=⋁g∈U[μℶ(∝ı,g)⋁μT(g)],ν¯ℶ(T)(∝ı)=⋀g∈U[νℶ(∝ı,c)⋀νT(g)],μℶ_(T)(∝ı)=⋀g∈U[μℶ(∝ı,c)⋀μT(g)],νℶ_(T)(∝ı)=⋁g∈U[νℶ(∝ı,c)⋁νT(g)], |
such that 0≤((μ¯ℶ(T)(∝ı))q+(ν¯ℶ(T)(∝ı))q)≤1, and 0≤((μℶ_(T)(∝ı))q+(νℶ_(T)(∝ı))q)≤1. As (ℶ_(T),¯ℶ(T)) are q−RFSs, ℶ_(T), ¯ℶ(T):q−RFS(U)→q−RFS(U) are LA and UA operators. The pair ℶ(T)=(ℶ_(T),¯ℶ(T)) ={⟨∝ı,(μℶ_(T)(∝ı),νℶ_(T)(∝ı),(μ¯ℶ(T)(∝ı),ν¯ℶ(T)(∝ı))⟩|∝ı∈T} is known as a q-ROFS. For simplicity ℶ(T)={⟨∝ı,μℶ_(T)(∝ı),νℶ_(T)(∝ı),(μ¯ℶ(T)(∝ı),ν¯ℶ(T)(∝ı))⟩|∝ı∈U} is represented as ℶ(T)=((μ_,ν_),(¯μ,¯ν)) and is referred to as a q-ROFR values.
This section introduces the concept of a q-ROHFRS, which is a rough set and q-ROFS hybrid structure. In addition, we initiate the new score and accuracy functions to assess the q-ROHFRS and provide its key operational rules.
Definition 10. Let U={∝1,∝2,∝3,...,∝n} be a universal set. Then, any subset ℶ∈q−ROHFS(U×U) is said to be a q-RHF relation. The pair (U,ℶ) is said to be q-ROHF approximation space. For any T⊆q−ROHFS(U), then the LA and UA of T with respect to q-ROHF approximation space (U,ℶ) are two q-ROHFSs, which are represented by ¯ℶ(T) and ℶ_(T) and characterized as
¯ℶ(T)={⟨∝ı,βh¯ℶ(T)(∝ı),ψh¯ℶ(T)(∝ı)⟩|∝ı∈U},ℶ_(T)={⟨∝ı,βhℶ_(T)(∝ı),ψhℶ_(T)(∝ı)⟩|∝ı∈U}, |
where
βh¯ℶ(T)(∝ı)=⋁k∈U[βhℶ(∝ı,k)⋁βhT(k)],ψh¯ℶ(T)(∝ı)=⋀k∈U[ψhℶ(∝ı,k)⋀ψhT(k)],βhℶ_(T)(∝ı)=⋀k∈U[βhℶ(∝ı,k)⋀βhT(k)],ψhℶ_(T)(∝ı)=⋁k∈U[ψhℶ(∝ı,k)⋁ψhT(k)], |
such that 0≤(max(βh¯ℶ(T)(∝ı)))q+(min(ψh¯ℶ(T)(∝ı)))q≤1 and 0≤(min(βhℶ_(T)(∝ı))q+(max(ψhℶ_(T)(∝ı)))q≤1. As (¯ℶ(T),ℶ_(T)) are q−ROHFSs, ℶ_(T),¯ℶ(T):q−ROHFS(U)→q−RFS(U) are LA and UA operators. The pair
ℶ(T)=(ℶ_(T),¯ℶ(T))={⟨∝ı,(βhℶ_(T)(∝ı),ψhℶ_(T)(∝ı)),(βh¯ℶ(T)(∝ı),ψh¯ℶ(T)(∝ı))⟩|∝ı∈T} |
will be referred to as a q-ROHFRS. For simplicity,
ℶ(T)={⟨∝ı,(βhℶ_(T)(∝ı),ψhℶ_(T)(∝ı)),(βh¯ℶ(T)(∝ı),ψh¯ℶ(T)(∝ı))⟩|∝ı∈T} |
is symbolized as ℶ(T)=((β_,ψ_),(¯β,¯ψ)) and is referred to as a q-ROHFR values. For explanation of the above concept of a q-ROHFRS, we present the following example.
Example 1. Let U={∝1,∝2,∝3,∝4} be any arbitrary set, and (U,ℶ) is a q-ROHF approximation space where ℶ∈q−ROHFRS(U×U) is the q -ROHFR relation as displayed in Table 1, where the value of q is 3. A decision expert provides the decision evaluation in the form of a q-ROHFS as follows:
T | c1 | c2 | c3 | c4 |
∝1 | ({0.1,0.3,0.4},{0.2,0.5,0.7}) | ({0.2,0.3},{0.7,0.9}) | ({0.2,0.5,0.7},{0.2,0.3}) | ({0.3,0.5},{0.8}) |
∝2 | ({0.2,0.3,0.5},{0.2,0.7}) | ({0.2,0.3,,0.5},{0.3,0.4}) | ({0.1,0.4,0.6},{0.7,0.9}) | ({0.2,0.4},{0.7}) |
∝3 | ({0.5,0.6},{0.7,0.9}) | ({0.5,0.8,0.9},{0.1,0.9}) | ({0.2,0.3},{0.5,0.9}) | ({0.7,0.9},{0.1,0.2,0.3}) |
∝4 | ({0.2,0.5,0.9},{0.6,0.7,0.9}) | ({0.3,0.8,0.9},{0.4,0.8}) | ({0.2,0.5},{0.6,0.9}) | ({0.5,0.7},{0.1,0.8}) |
and
T={⟨∝1,{0.2,0.3,0.4},{0.5,0.7}⟩,⟨∝2,{0.2,0.3,0.7},{0.1,0.7,0.8}⟩,⟨∝3,{0.5,0.7,0.8},{0.1,0.5,0.7}⟩,⟨∝4,{0.6,0.8,0.9},{0.2,0.6,0.7}⟩}. |
It follows that
βh¯ℶ(T)(∝1)=⋁k∈U[βhℶ(∝,c)⋁βhT(k)]={{0.1∨0.2,0.3∨0.3,0.4∨0.4}∨{0.2∨0.2,0.3∨0.3,0∨0.7}∨{0.2∨0.5,0.5∨0.7,0.7∨0.8}∨{0.3∨0.6,0.5∨0.8,0∨0.9}}={{0.2,0.3,0.4}∨{0.2,0.3,0}∨{0.5,0.7,0.8}∨{0.6,0.8,0.9}}={0.6,0.8,0.9}. |
The other values are determined in a similar manner as follows:
βh¯ℶ(T)(∝2)={0.6,0.8,0.9},βh¯ℶ(T)(∝3)={0.7,0.9},βh¯ℶ(T)(∝4)={0.6,0.8,0.9}. |
Similarly,
ψh¯ℶ(T)(∝1)=⋀k∈U[ψhℶ(∝,c)⋀ψhT(k)]={{0.2∧0.5,0.5∧0.7,0∧0.7}∧{0.7∧0.1,0.9∧0.7,0∧0.8}∧{0.2∧0.1,0.3∧0.5,0∧0.7}∧{0.8∧0.2,0∧0.6,0∧0.7}}={{0.2,0.5}∧{0.1,0.7}∧{0.2,0.3}∧{0.2}},={0.2}. |
By routine calculations, we get
ψh¯ℶ(T)(∝2)={0.1},ψh¯ℶ(T)(∝3)={0.1,0.2},ψh¯ℶ(T)(∝4)={0.1,0.5}. |
Further,
βhℶ_(T)(∝1)=⋀k∈U[βhℶ(∝,c)⋀βhT(k)]={{0.1∧0.2,0.3∧0.3,0.4∧0.4}∧{0.2∧0.2,0.3∧0.3,0∧0.7}∧{0.2∧0.5,0.5∧0.7,0.7∧0.8}∧{0.3∧0.6,0.5∧0.8,0∧0.9}}={{0.1,0.3,0.4}∧{0.2,0.3}∧{0.2,0.5,0.7}∧{0.3,0.5}}={0.1,0.3}. |
By routine calculations, we get
βhℶ_(T)(∝2)={0.1,0.3},βhℶ_(T)(∝3)={0.2,0.3},βhℶ_(T)(∝4)={0.2,0.3}. |
Now,
ψhℶ_(T)(∝1)=⋁k∈U[ψhℶ(∝,c)⋁ψhT(k)]={{0.2∨0.5,0.5∨0.7,0.7∨0}∨{0.7∨0.2,0.9∨0.3,0∨0.7}∨{0.2∨0.1,0.3∨0.5,0∨0.7}∨{0.8∨0.2,0∨0.6,0∨0.7}}={{0.5,0.7,0.7}∨{0.7,0.9,0.7}∨{0.2,0.5,0.7}∨{0.8,0.6,0.7}}={0.8,0.9,0.7}. |
Following the same procedure, we obtain the other values,
ψhℶ_(T)(∝2)={0.7,0.9},ψhℶ_(T)(∝3)={0.7,0.9,0.8},ψhℶ_(T)(∝4)={0.6,0.9,0.9}. |
Thus, the LA and UA operators based on the q-ROHFR are presented as follows:
ℶ_(T)={⟨∝1,{0.1,0.3},{0.8,0.9,0.7}⟩,⟨∝2,{0.1,0.3},{0.7,0.9}⟩,⟨∝3,{0.2,0.3},{0.7,0.9,0.8}⟩,⟨∝3,{0.2,0.3},{0.6,0.9,0.9}⟩}, |
¯ℶ(T)={⟨∝1,{0.6,0.8,0.9},{0.2}⟩,⟨∝2,{0.6,0.8,0.9},{0.1}⟩,⟨∝3,{0.7,0.9,0.9},{0.1,0.2}⟩,⟨∝4,{0.6,0.8,0.9},{0.1,0.5}⟩}. |
Hence,
ℶ(T)=(ℶ_(T),¯ℶ(T))={⟨∝1,({0.1,0.3},{0.8,0.9,0.7}),({0.6,0.8,0.9},{0.2})⟩,⟨∝2,({0.1,0.3},{0.7,0.9}),({0.6,0.8,0.9},{0.1})⟩,⟨∝3,({0.2,0.3},{0.7,0.9,0.8}),({0.7,0.9,0.9},{0.1,0.2})⟩,⟨∝3,({0.2,0.3},{0.6,0.9,0.9}),({0.6,0.8,0.9},{0.1,0.5})⟩}. |
Definition 11. Let ℶ(T1)=(ℶ_(T1),¯ℶ(T1)) and ℶ(T2)=(ℶ_(T2),¯ℶ(T2)) be two q-ROHFRSs. Then, we have the following.
(1) ℶ(T1)∪ ℶ(T2)={(ℶ_(T1)∪ℶ_(T2)),(¯ℶ(T1)∪¯ℶ(T2))}
(2) ℶ(T1)∩ ℶ(T2)={(ℶ_(T1)∩ℶ_(T2)),(¯ℶ(T1)∩¯ℶ(T2))}
(3) ℶ(T1)⨁ ℶ(T2)={(ℶ_(T1)⨁ℶ_(T2)),(¯ℶ(T1)⨁¯ℶ(T2))}
(4) ℶ(T1)⨂ ℶ(T2)={(ℶ_(T1)⨂ℶ_(T2)),(¯ℶ(T1)⨂¯ℶ(T2))}
(5) ℶ(T1)⊆ ℶ(T2)={(ℶ_(T1)⊆ℶ_(T2)) and (¯ℶ(T1)⊆¯ℶ(T2))}
(6) γℶ(T1)=(γℶ_(T1), γ¯ℶ(T1)) for γ≥1
(7) (ℶ(T1))γ=((ℶ_(T1))γ, (¯ℶ(T1))γ) for γ≥1
(8) ℶ(T1)c=(ℶ_(T1)c, ¯ℶ(T1)c) where ℶ_(T1)c and ¯ℶ(T1)c represent the complement of q-rung fuzzy rough approximation operators ℶ_(T1) and ¯ℶ(T1), that is, ℶ_(T1)c=(ψhℶ_(T),βhℶ_(T))
(9) ℶ(T1)= ℶ(T2) iff ℶ_(T1)=ℶ_(T2) and ¯ℶ(T1)=¯ℶ(T2).
Definition 12. The score function for q-ROHFRN ℶ(T)=(ℶ_(T),¯ℶ(T))=((β_,ψ_),(¯β,¯ψ)) is given as:
SR(ℶ(T))=14(2+1MF∑μı_∈βhℶ_(T){μı_}+1NF∑¯μı∈βh¯ℶ(T){¯μı}−1MF∑νı_∈ψhℶ_(T)(νı_)−1MF∑¯νı∈ψh¯ℶ(T)(¯νı)). |
The accuracy function for q-ROHFRV ℶ(T)=(ℶ_(T),¯ℶ(T))=((β_,ψ_),(¯β,¯ψ)) is defined by:
ACℶ(T)=14(1MF∑μı∈βh¯ℶ(T)(¯μı)+1MF∑μı∈βh¯ℶ(T)(¯μı)+1MF∑νı_∈ψhℶ_(T)(νı_)+1MF∑¯νı∈ψh¯ℶ(T)(¯νı)), |
where MF and NF indicate the numbers of elements in βhg and ψhg, respectively. We will use the score function to compare and rank two or more q-ROHFR values. The q-ROHFR number with the highest score is considered greater, while the q-ROHFRNs with the lowest score is considered smaller. If the score values are equal, the accuracy function will be employed. The q-ROHFRN having higher accuracy values are considered greater, whereas lower accuracy q-ROHFR values are considered smaller.
Definition 13. Suppose ℶ(T1)=(ℶ_(T1),¯ℶ(T1)) and ℶ(T2)=(ℶ_(T2),¯ℶ(T2)) are two q-ROHFRVs. Then, we have the following.
(1) If SR(ℶ(T1))>SR(ℶ(T2)), then ℶ(T1)>ℶ(T2).
(2) If SR(ℶ(T1))≺SR(ℶ(T2)), then ℶ(T1)≺ℶ(T2).
(3) If SR(ℶ(T1))=SR(ℶ(T2)), then we will find the accuracy functions and there are three possibilities that are as follows:
(a) If ACℶ(T1)>ACℶ(T2) then, ℶ(T1)>ℶ(T2).
(b) If ACℶ(T1)≺ACℶ(T2) then, ℶ(T1)≺ℶ(T2).
(c) If ACℶ(T1)=ACℶ(T2) then, ℶ(T1)=ℶ(T2).
In this part, we introduce the new concept of q-ROHF rough AOps by integrating RSs and q-ROPHF AOps to get aggregation notions of q-ROHFREWA, q-ROHFREOWA, and q-ROHFREHWA. In addition, certain important characteristics of the ideas are explored.
Definition 14. Let ℶ(κt)=(ℶ_(κt),¯ℶ(κt)) (t=1,2,3,...,r) be the collection of q-ROHFRVs. The q-ROHFREWA operator is designated as
q−ROHFREWA(ℶ(κ1),ℶ(κ2),...,ℶ(κn))=(n⨁t=1γtℶ_(κt),r⊗t=1γt¯ℶ(κt)), |
where γ=(γ1,γ2,...,γr)T is the weights vector such that r⊗t=1γt=1 and 0≤ γt≤1.
Theorem 4.1. Let ℶ(κt)=(ℶ_(κt),¯ℶ(κt)) (t=1,2,3,...,r) be the collection of q-ROHFRVs. Then, the q-ROHFREWA operator is defined by
q−ROHFREWA(ℶ(κ1),ℶ(κ2),...,ℶ(κr))=(r⊗t=1γtℶ_(κt),r⊗t=1γt¯ℶ(κt))=({⋃μht_∈βhℶ_(κ)(q√r⊗t=1(1+μht_q)γt−r⊗t=1(1−μht_q)γtq√r⊗t=1(1+μht_q)γt+r⊗t=1(1−μht_q)γt),⋃νht_∈ψhℶ_(κ)(q√2r⊗t=1(νqht_)γtq√r⊗t=1(2−νqht_)γt+r⊗t=1(νqht_)γt)}{⋃¯μht∈ψh¯ℶ(κ),¯ðht∈ðh¯ℶ(κ)(q√r⊗t=1(1+¯μqht)γt−r⊗t=1(1−¯μqht)γtq√r⊗t=1(1+¯μqht)γt+r⊗t=1(1−¯μqht)γt),⋃¯νht∈ψh¯ℶ(κ),¯∂ht∈∂h¯ℶ(κ)(q√2r⊗t=1(¯νqht)γtq√r⊗t=1(2−¯νqht)γt+r⊗t=1(¯νqht)γt)}), |
where γ=(γ1,γ2,...,γr)T is the weight vector such that ⨁rt=1γt=1 and 0≤ γt≤1.
Proof. We will use mathematical induction to prove this theorem, starting with r=2.
(ℶ(κ1)⨁ℶ(κ2))=(ℶ_(κ1)⨁ℶ_(κ2),¯ℶ(κ1)⨁¯ℶ(κ2)) |
q−ROHFREWA(ℶ(κ1),ℶ(κ2))=(2⊗t=1γtℶ_(κt),2⊗t=1γt¯ℶ(κt))=( {⋃μht_∈βhℶ_(κ)(q√2⊗t=1(1+μqht_)γt−2⊗t=1(1−μqht_)γtq√2⊗t=1(1+μht_q)γt+2⊗t=1(1−μht_q)γt),⋃νht_∈ψhℶ_(κ)(q√22⊗t=1(νqht_)γtq√2⊗t=1(2−νqht_)γt+2⊗t=1(νqht_)γt)}{⋃¯μht∈ψh¯ℶ(κ),¯ðht∈ðh¯ℶ(κ)(q√2⊗t=1(1+¯μqht)γt−2⊗t=1(1−¯μqht)γtq√2⊗t=1(1+¯μqht)γt+2⊗t=1(1−¯μqht)γt),⋃¯νht∈ψh¯ℶ(κ),¯∂ht∈∂h¯ℶ(κ)(q√22⊗t=1(¯νqht)γtq√2⊗t=1(2−¯νqht)γt+2⊗t=1(¯νqht)γt)}), |
so the result is true for r=2. Now, suppose that the result is true for r=k, that is,
q−ROHFREWA(ℶ(κ1),ℶ(κ2),...,ℶ(κk))=(k⨁t=1γtℶ_(κt),k⨁t=1γt¯ℶ(κt))=( {⋃μht_∈βhℶ_(κ)(q√k⊗t=1(1+μht_q)γt−k⊗t=1(1−μht_q)γtq√k⊗t=1(1+μht_q)γt+k⊗t=1(1−μht_q)γt),⋃νht_∈ψhℶ_(κ)(q√2k⊗t=1(νqht_)γtq√k⊗t=1(2−νqht_)γt+k⊗t=1(νqht_)γt)}{⋃¯μht∈ψh¯ℶ(κ),¯ðht∈ðh¯ℶ(κ)(q√k⊗t=1(1+¯μqht)γt−k⊗t=1(1−¯μqht)γtq√k⊗t=1(1+¯μqht)γt+k⊗t=1(1−¯μqht)γt),⋃¯νht∈ψh¯ℶ(κ),¯∂ht∈∂h¯ℶ(κ)(q√2k⊗t=1(¯νqht)γtq√k⊗t=1(2−¯νqht)γt+k⊗t=1(¯νqht)γt)}). |
Now, we have to prove that the result holds for r=k+1. It follows that
q−ROHFREWA(ℶ(κ1),ℶ(κ2),...,ℶ(κk),ℶ(κk+1))=(k⨁t=1γtℶ_(κt)⨁(ℶ_(κk+1))wk+1,k⨁t=1γt¯ℶ(κt)⨁(¯ℶ(κk+1))wk+1)=( {⋃μht_∈βhℶ_(κ)(q√k⊗t=1+1(1+μht_q)γt−k⊗t=1+1(1−μht_q)γtq√k⊗t=1+1(1+μht_q)γt+k⊗t=1+1(1−μht_q)γt),⋃νht_∈ψhℶ_(κ)(q√2k⊗t=1+1(νqht_)γtq√k⊗t=1+1(2−νqht_)γt+k⊗t=1+1(νqht_)γt)}{⋃¯μht∈ψh¯ℶ(κ),¯ðht∈ðh¯ℶ(κ)(q√k⊗t=1+1(1+¯μqht)γt−k⊗t=1+1(1−¯μqht)γtq√k⊗t=1+1(1+¯μqht)γt+k⊗t=1+1(1−¯μqht)γt),⋃¯νht∈ψh¯ℶ(κ),¯∂ht∈∂h¯ℶ(κ)(q√2k⊗t=1+1(¯νqht)γtq√k⊗t=1+1(2−¯νqht)γt+k⊗t=1+1(¯νqht)γt)}). |
Thus, the result is valid for r=k+1. Therefore, it holds for every r≥1.
Theorem 4.2. Let ℶ(κt)=(ℶ_(κt),¯ℶ(κt)) (t=1,2,3,...,r) be the collection of q-ROPHFRVs, and γ=(γ1,γ2,...,γr)T is the weight vector such that γt∈[0,1] and ⨁rt=1γt=1. The q-ROHFREWA operator must fulfill the following features:
(1) Idempotency: If ℶ(κt)=Q(κ) for t=1,2,3,...,r where Q(κ)=(Q_(κ),¯Q(κ))=((bh(∝ı)_,dh(∝ı)_),(¯bh(∝ı),¯dh(∝ı)), then
q−ROHFREWA(ℶ(κ1),ℶ(κ2),...,ℶ(κr))=Q(κ). |
(2) Boundedness: Let (ℶ(κ))min=(mintℶ_(κt),maxt¯ℶ(κt)) and (ℶ(κ))max= (maxtℶ_(κt),mint¯ℶ(κt)). Then,
(ℶ(κ))min≤q−ROHFREWGA(ℶ(κ1),ℶ(κ2),...,ℶ(κr))≤(ℶ(κ))max. |
(3) Monotonicity: Suppose Q(κ)=(Q_(κt),¯Q(κt))(t=1,2,...,r) is another collection of q-ROPHFRVs such that Q_(κt)≤ℶ_(κt) and ¯Q(κt)≤¯ℶ(κt). Then,
q−ROHFREWA(Q(κ1),Q(κ2),...,Q(κr))≤q−ROHFREWA(ℶ(κ1),ℶ(κ2),...,ℶ(κr)). |
Proof. (1) Idempotency: As ℶ(κt)=Q(κ) (for all t=1,2,3,...,r) where Q(κt)=(Q_(κ),¯Q(κ))=((bh(∝ı)_,dh(∝ı)_),(¯bh(∝ı),¯dh(∝ı))), it follows that
q−ROHFREWA(ℶ(κ1),ℶ(κ2),...,ℶ(κr))=(r⊗t=1γtℶ_(κt),r⊗t=1γt¯ℶ(κt))=( {⋃μht_∈βhℶ_(κ)(q√r⊗t=1(1+μht_q)γt−r⊗t=1(1−μht_q)γtq√r⊗t=1(1+μht_q)γt+r⊗t=1(1−μht_q)γt),⋃νht_∈ψhℶ_(κ)(q√2r⊗t=1(νqht_)γtq√r⊗t=1(2−νqht_)γt+r⊗t=1(νqht_)γt),}{⋃¯μht∈ψh¯ℶ(κ),¯ðht∈ðh¯ℶ(κ)(q√r⊗t=1(1+¯μqht)γt−r⊗t=1(1−¯μqht)γtq√r⊗t=1(1+¯μqht)γt+r⊗t=1(1−¯μqht)γt),⋃¯νht∈ψh¯ℶ(κ),¯∂ht∈∂h¯ℶ(κ)(q√2r⊗t=1(¯νqht)γtq√r⊗t=1(2−¯νqht)γt+r⊗t=1(¯νqht)γt)}), |
for all t, ℶ(κt)=Q(κ)= (Q_(κ),¯Q(κ))=((bh(∝ı)_,dh(∝ı)_),(¯bh(∝ı),¯dh(∝ı))). Hence,
=[(⋃bh(∝ı)_∈βhℶ_(κ)(q√r⊗t=1(1+(bh(∝ı)_)q)γt−n⊗t=1(1−(bh(∝ı)_)q)γtq√r⊗t=1(1+(bh(∝ı)_)q)γt+r⊗t=1(1−(bh(∝ı)_)q)γt), ⋃dh(∝ı)_∈ψhℶ_(κ)(q√2r⊗t=1((dh(∝ı)_)q)γtq√r⊗t=1(2−(dh(∝ı)_)q)γt+r⊗t=1((dh(∝ı)_)q)γt))(⋃¯bh(∝ı)∈βh¯ℶ(κ)(q√r⊗t=1(1+(¯bh(∝ı))q)γt−r⊗t=1(1−(¯bh(∝ı))q)γtq√r⊗t=1(1+(¯bh(∝ı))q)γt+r⊗t=1(1−(¯bh(∝ı))q)γt), ⋃¯dh(∝ı)∈ψh¯ℶ(κ)(q√2r⊗t=1((¯dh(∝ı))q)γtq√r⊗t=1(2−(¯dh(∝ı))q)γt+r⊗t=1((¯dh(∝ı))q)γt))]=[((q√(1+(bh(∝ı)_)q)−(1−(bh(∝ı)_)q)q√(1+(bh(∝ı)_)q)+(1−(bh(∝ı)_)q)),(q√2((dh(∝ı)_)q)q√(2−(dh(∝ı)_)q)+((dh(∝ı)_)q))),(q√(1+(¯bh(∝ı))q)−(1−(¯bh(∝ı))q)q√(1+(¯bh(∝ı))q)+(1−(¯bh(∝ı))q)),(q√2((¯dh(∝ı))q)q√(2−(¯dh(∝ı))q)+((¯dh(∝ı))q))]=(Q_(κ),¯Q(κ))=Q(κ). |
Therefore, q-ROHFREWA(ℶ(κ1),ℶ(κ2),...,ℶ(κr))=Q(κ).
(2) Boundedness: As
(ℶ_(κ))−=[(mint{μht_},maxt{νht_}),(mint{¯μht},maxt{¯νhı})](ℶ_(κ))+=[(maxt{μht_},mint{νht_}),(maxt{¯μht/¯ðht},mint{¯νhı})] |
and ℶ(κt)=[(βt_,ψt_),(¯βt,¯ψt)]. To prove that
(ℶ(κ))−≤q−ROHFREWA(ℶ(κ1),ℶ(κ2),...,ℶ(κr))≤(ℶ(κ))+, |
let $ f(\mathcal{G}) = \sqrt[3]{\frac{1-\mathcal{G}^{3}}{1+\mathcal{G}^{3}}}, \mathcal{G}\in \lbrack 0, 1]. Then, f^{^{\prime }}(\mathcal{G}) = \frac{-2\mathcal{G}}{\left(1+\mathcal{G}^{3}\right) ^{3}}\sqrt[3]{\left(\frac{1-\mathcal{G}^{3}}{1+\mathcal{G}^{3}}\right) ^{-2}} < 0. Thus, f(\mathcal{G}) isadecreasingfunctionover [0, 1]. Since \{\underline{\mu _{h_{\max }}}\}\leq \{ \underline{\mu _{h_{t}}}\}\leq \{\underline{\mu _{h_{\min }}}\} forall \ t, \ g\left(\underline{\mu _{h_{\min }}}\right) \leq g\left(\underline{\mu _{h_{t}}}\right) \leq g\left(\underline{\mu _{h_{\max }}}\right) (t = 1, 2, 3, ..., n) $ i.e.,
⇔3√1−(μhmin_)31+(μhmin_)3≤3√1−(μht_)31+(μht_)3≤3√1−(μhmax_)31+(μhmax_)3, (t=1,2,3,...,n). |
Let γ=(γ1,γ2,...,γr)T be a weight vector such that γt∈[0,1] and ⨁rt=1γt=1, and we have
⇔3√r⊗t=1(1−(μhmin_)31+(μhmin_)3)γt≤3√r⊗t=1(1−(μht_)31+(μht_)3)γt≤3√r⊗t=1(1−(μhmax_)31+(μhmax_)3)γt⇔3√(1−(μhmin_)31+(μhmin_)3)⨁rt=1γt≤3√r⊗t=1(1−(μht_)31+(μht_)3)γt≤3√(1−(μhmax_)31+(μhmax_)3)⨁rt=1γt | (4.1) |
⇔μhmax_≤3√r⊗t=1(1−(μht_)31+(μht_)3)γt≤μhmin_⇔μhmax_≤3√r⊗t=1(1−(μht_)31+(μht_)3)γt≤μhmin_. | (4.2) |
In a similar way, we can show that
⇔¯μhmax≤3√r⊗t=1(2−(¯μht)3(¯μht)3)γt≤¯μhmin. |
Again, let S(ϰ) = $ \sqrt[3]{\frac{2-\varkappa ^{3}}{\varkappa ^{3}}}, \varkappa \in (0, 1]. Then, \mathcal{S}^{\prime }(\varkappa) = \frac{-2}{\varkappa ^{4} }\sqrt[3]{\left(\frac{2-\varkappa ^{3}}{\varkappa ^{3}}\right) ^{-2}} < 0.\ So, \mathcal{S}(\varkappa) isadecreasingfunctionon (0, 1] .Since \{\underline{ \nu _{h_{\min }}}\}\leq \{\underline{\nu _{h_{t}}}\}\leq \{\underline{\nu _{h_{\max }}}\} forall \ t .So, \mathcal{S}\left(\underline{\nu _{h_{\max }}} \right) \leq \mathcal{S}\left(\underline{\nu _{h_{t}}}\right) \leq \mathcal{S}\left(\underline{ \nu _{h_{\min }}}\right) (t = 1, 2, 3, ..., r), $ i.e.,
3√2−(νhmax_)3(νhmax_)3≤3√2−(νht_)3(νht_)3≤3√2−(νhmin_)3(νhmin_)3. |
Let γ=(γ1,γ2,...,γr)T be a weight vector such that γt∈[0,1] and ⨁rt=1γt=1, and we have
⇔3√r⊗t=1(2−(νhmax_)3(νhmax_3))γt≤3√r⊗t=1(2−(νht_)3(νht_3))γt≤3√r⊗t=1(2−(νhmax_)3(νhmax_)3)γt⇔3√(2−(νhmax_)3(νhmax_)3)⨁rt=1γt≤3√r⊗t=1(2−(νht_3)(νht_)3)γt≤3√r⊗t=1(2−(νhmax_)3(νhmax_)3)⨁rt=1γt⇔νhmin_≤3√r⊗t=1(2−(νht_)3(νht_)3)γt≤νhmax_. | (4.3) |
Likewise, we can show that
⇔¯νhmin≤3√r⊗t=1(2−(¯νht)3(¯νht)3)γt≤¯νhmax. | (4.4) |
By routine calculation, we can show that it is true for q>3. Thus, from Eqs (4.1)–(4.4), we have
(ℶ(κ))−≤q−ROHFREWG(ℶ(κ1),ℶ(κ2),...,ℶ(κr))≤(ℶ(κ))+. |
(3) Monotonicity: The proof is similar to the proof of Theorem 4.1.
In this subsection, we introduce the concept of the q-ROHFREOWA operator and suggest the essential features of the operator.
Definition 15. Let ℶ(κt)=(ℶ_(κt),¯ℶ(κt)) (t=1,2,3,4,...,r) be the collection of q-ROHFRNs. Then, the q-ROHFREOWA operator is defined as
q−ROHFREOWA(ℶ(κ1),ℶ(κ2),...,ℶ(κr))=(r⊗t=1γtℶ_ρ(t)(κt),r⊗t=1γt¯ℶρ(t)(κt)), |
where (ρ(1),ρ(2),...,ρ(n)) is a permutation in such a way that ℶ_ρ(κt)≤ℶ_ρ(κt−1), and the weights vector γ=(γ1,γ2,...,γr)T such that r⊗t=1γt=1 and 0≤ γt≤1.
Theorem 4.3. Let ℶ(κt)=(ℶ_(κt),¯ℶ(κt)) (t=1,2,3,...,r) be the collection of q -ROHFRVs. Then, the q-ROHFREOWA operator is defined as:
q−ROHFREOWA(ℶ(κ1),ℶ(κ2),...,ℶ(κr))=(r⊗t=1γtℶ_ρ(κt),r⊗t=1γt¯ℶρ(κt))=({⋃μρht_∈βhℶ_ρ(κ)(q√r⊗t=1(1+μρht_q)γt−r⊗t=1(1−μρht_q)γtq√r⊗t=1(1+μρht_q)γt+r⊗t=1(1−μρht_q)γt)⋃νρht_∈ψhℶ_ρ(κ)(q√2r⊗t=1(νqρht_)γtq√r⊗t=1(2−νqρht_)γt+r⊗t=1(νρhtq_)γt),}{⋃¯μρht∈ψh¯ℶρ(κ)(q√r⊗t=1(1+¯μqρht)γt−r⊗t=1(1−¯μqρht)γtq√r⊗t=1(1+¯μqρht)γt+r⊗t=1(1−¯μqρht)γt),⋃¯νρht∈ψh¯ℶρ(κ)(q√2r⊗t=1(¯νqρht)γtq√r⊗t=1(2−¯νqρht)γt+r⊗t=1(¯νqρht)γt)}), |
where γ=(γ1,γ2,...,γr)T is a weight vector such that ⨁rt=1γt=1 and 0≤ γt≤1.
Proof. It follows from Theorem 4.1.
Theorem 4.4. Let ℶ(κt)=(ℶ_(κt),¯ℶ(κt)) (t=1,2,3,...,r) be the collection of q-ROHFRVs, and γ=(γ1,γ2,...,γr)T is a weights vector such that γt∈[0,1] and ⨁rt=1γt=1. Then, the q-ROHFREOWA operator must fulfill the following features:
(1) Idempotency: If ℶ(κt)=Q(κ) for (t=1,2,3,...,r) where
Q(κ)=(Q_(κ),¯Q(κ))=((bh(∝ı)_,dh(∝ı)_),(¯bh(∝ı),¯dh(∝ı)), |
then
q−ROHFREOWA(ℶ(κ1),ℶ(κ2),...,ℶ(κr))=Q(κ). |
(2) Boundedness: Let (ℶ(κ))min=(mintℶ_(κt),maxt¯ℶ(κt)) and (ℶ(κ))max= (maxtℶ_(κt),mint¯ℶ(κt)). Then,
(ℶ(κ))min≤q−ROHFREOWA(ℶ(κ1),ℶ(κ2),...,ℶ(κr))≤(ℶ(κ))max. |
(3) Monotonicity: Suppose Q(κ)=(Q_(κt),¯Q(κt))(t=1,2,3,...,n) is another collection of q-ROHFRVs such that Q_(κt)≤ℶ_(κt) and ¯Q(κt)≤¯ℶ(κt). Then,
q−ROHFREOWA(Q(κ1),Q(κ2),...,Q(κr))≤q−ROHFREOWA(ℶ(κ1),ℶ(κ2),...,ℶ(κr)). |
Proof. The proof is comparable to the proof of Theorem 4.2.
In this part, we present the q-ROHFREHWA operator, which simultaneously weights the value and ordered position of q-ROHFR information. The key features of the proposed operator are described in detail as follows:
Definition 16. Let ℶ(κt)=(ℶ_(κt),¯ℶ(κt)) (t=1,2,3,...,n) be the collection of q-ROHFRVs and let the weight vector γ=(γ1,γ2,...,γr)T of the given collection of q-ROHFRVs such that r⊗t=1γt=1 and 0≤ γt≤1. Let (ϱ1,ϱ2,...,ϱr)T be the associated weight vector such that r⊗t=1ϱt=1 and 0≤ ϱt≤1. Then, the q-ROHFREHWA operator is determined as
q−ROHFREHWA(ℶ(κ1),ℶ(κ2),...,ℶ(κr))=(r⊗t=1γt^ℶ_ρ(κt),r⊗t=1γt¯ˆℶρ(κt)), |
where (^ℶ_ρ(κt),¯ˆℶρ(κt))=(nγtℶ_ρ(κt),nγt¯ℶρ(κt)).
Theorem 4.5. Let ℶ(κt)=(ℶ_(κt),¯ℶ(κt)) (t=1,2,3,4,...,r) be the collection of q -ROHFRVs. Then, the q-ROHFREHWA operator is defined as:
q−ROHFREHWA(ℶ(κ1),ℶ(κ2),...ℶ(κr))=(r⊗t=1ϱt^ℶ_ρ(κt),r⊗t=1ϱt¯ˆℶρ(κt))=({⋃μρht_∈βh^ℶ_ρ(κ)(q√r⊗t=1(1+ˆμρht_q)ϱt−r⊗t=1(1−ˆμρht_q)ϱtq√r⊗t=1(1+ˆμρht_q)ϱt+r⊗t=1(1−ˆμρht_q)ϱt)⋃νρht_∈ψh^ℶ_ρ(κ)(q√2r⊗t=1(ˆνqρht_)ϱtq√r⊗t=1(2−ˆνqρht_)ϱt+r⊗t=1(ˆνqρht_)ϱt),}{⋃¯μρht∈ψh¯ˆℶρ(κ)(q√r⊗t=1(1+¯ˆμqρht)ϱt−r⊗t=1(1−¯ˆμqρht)ϱtq√r⊗t=1(1+¯ˆμqρht)ϱt+r⊗t=1(1−¯ˆμqρht)ϱt),⋃¯νρht∈ψh¯ˆℶρ(κ)(q√2r⊗t=1(¯ˆνqρht)ϱtq√r⊗t=1(2−¯ˆνqρht)ϱt+r⊗t=1(¯ˆνqρht)ϱt)}), |
where (^ℶ_ρ(κt),¯ˆℶρ(κt))=(nγtℶ_ρ(κt),nγt¯ℶρ(κt)) and (ϱ1,ϱ2,...,ϱr)T is the associated weight vector such that r⊗t=1ϱt=1 and 0≤ ϱt≤1.
Proof. The proof follows from the proof of Theorem 4.1.
Theorem 4.6. Let ℶ(κt)=(ℶ_(κt),¯ℶ(κt)) (t=1,2,3,...,n) be the collection of q-ROHFRVs and let (ϱ1,ϱ2,...,ϱr)T be the associated weight vector such that r⊗t=1ϱt=1 and 0≤ ϱt≤1. Let the weight vector of the given collection of q-ROHFRVs be γ=(γ1,γ2,...,γr)T such that r⨁t=1γt=1 and 0≤ γt≤1. Then, q-ROHFREHWA operator satisfies the following properties:
(1) Idempotency: If ℶ(κt)=Q(κ) for t=1,2,...,r where Q(κ)=(Q_(κ),¯Q(κ))=((bh(∝ı)_,dh(∝ı)_),(¯bh(∝ı),¯dh(∝ı)), then
q−ROHFREHWA(ℶ(κ1),ℶ(κ2),...,ℶ(κr))=Q(κ). |
(2) Boundedness: Let (ℶ(κ))min=(mintℶ_(κt),maxt¯ℶ(κt)) and (ℶ(κ))max= (maxtℶ_(κt),mint¯ℶ(κt)). Then,
(ℶ(κ))min≤q−ROHFREHWA(ℶ(κ1),ℶ(κ2),...,ℶ(κr))≤(ℶ(κ))max. |
(3) Monotonicity: Suppose Q(κ)=(Q_(κt),¯Q(κt))(t=1,2,3,...,r) is another collection of q-ROHFRVs such that Q_(κt)≤ℶ_(κt) and ¯Q(κt)≤¯ℶ(κt). Then,
q−ROHFREHWA(Q(κ1),Q(κ2),...,Q(κr))≤q−ROHFREHWA(ℶ(κ1),ℶ(κ2),...,ℶ(κr)). |
Proof. The proof is similar to the proof of Theorem 4.2.
Here, we develop an algorithm in order to deal with uncertainty in MAGDM under q-ROHFR information. Consider a DM problem with a set {A1,A2,...,Ar} of n alternatives and a set of n attributes {χ1,χ2,...,χr} with the weight vector (γ1,γ2,...,γr)T, that is, γt∈[0,1], ⨁rt=1γt=1. To test the reliability of kth alternative At under the attribute χt, let {˚D1,˚D2,...,˚Dˆz} be a set of DMs. The expert assessment matrix is defined as follows:
M=[¯ℶ(κˆztj)]m×n=[(ℶ_(κ11),¯ℶ(κ11))(ℶ_(κ12),¯ℶ(κ12))⋯(ℶ_(κ1j),¯ℶ(κ1j))(ℶ_(κ21),¯ℶ(κ21))(ℶ_(κ22),¯ℶ(κ22))⋯(ℶ_(κ2j),¯ℶ(κ2j))(ℶ_(κ31),¯ℶ(κ31))(ℶ_(κ32),¯ℶ(κ32))⋯(ℶ_(κ3j),¯ℶ(κ3j))⋮⋮⋱⋮(ℶ_(κt1),¯ℶ(κt1))(ℶ_(κt2),¯ℶ(κt2))⋯(ℶ_(κtj),¯ℶ(κtj))], |
where
ℶ_(κ)={⟨∝ı,βhℶ_(κ)(∝ı),ψhℶ_(κ)(∝ı)⟩|∝ı∈U}, |
and
¯ℶ(κtj)={⟨∝ı,βh¯ℶ(κ)(∝ı),ψh¯ℶ(κ)(∝ı)⟩|∝ı∈U}, |
0≤(max(βh¯ℶ(κ)(∝ı)))q+(min(ψh¯ℶ(κ)(∝ı)))q≤1, |
and
0≤(min(βhℶ_(κ)(∝ı)))q+(max(ψhℶ_(κ)(∝ı)))q≤1, |
are the q-ROHFR values. The main steps for MAGDM are as follows:
Step-1. Construct the experts evaluation matrices as
(E)^zı=[(ℶ_(κ^zı11),¯ℶ(κ^zı11))(ℶ_(κ^zı12),¯ℶ(κ^zı12))⋯(ℶ_(κˆ∝ı1j),¯ℶ(κ^zı1j))(ℶ_(κ^zı21),¯ℶ(κ^zı21))(ℶ_(κ^zı22),¯ℶ(κ^zı22))⋯(ℶ_(κˆ∝ı2j),¯ℶ(κ^zı2j))(ℶ_(κ^zı31),¯ℶ(κ^zı31))(ℶ_(κ^zı32),¯ℶ(κ^zı32))⋯(ℶ_(κˆ∝ı3j),¯ℶ(κ^zı3j))⋮⋮⋱⋮(ℶ_(κ^zıt1),¯ℶ(κ^zıt1))(ℶ_(κ^zıt2),¯ℶ(κ^zıt2))⋯(ℶ_(κˆ∝ıtj),¯ℶ(κ^zıtj))], |
where ^zı shows the number of experts.
Step-2. Evaluate the normalized expert matrices (N)^zı, as
(N)^zı=ℶ(κtj)=(ℶ_(κtj),¯ℶ(κtj))if forbenefit,(ℶ(κtj))c=((ℶ_(κtj))c,(¯ℶ(κtj))c)iffor cost . |
Step-3. Compute the q-ROHFRVs for each considered alternative with respect to the given list of criteria/attributes by utilizing the proposed aggregation information.
Step-4. Determine the ranking of alternatives based on the score function as follows:
SR(ℶ(κ))=14(2+1MF∑μht_∈βhℶ_(κ)(μht_)+1NF∑¯μht∈ψh¯ℶ(κ)(¯μht)1MF∑νht_∈ψhℶ_(κ)(νht_)−1MF∑¯νht∈ψh¯ℶ(κ)(¯νht)). |
Step-5. Rank all the alternative scores in descending order. The alternative with the largest value will be superior/best.
To validate the established operators, we consider a numerical MCGDM example for the analysis of the site selection for a garbage disposal plant.
The production of garbage in human activities is growing day by day as the world population grows and people's living standards improve. Garbage has become significant problem for international society, creating an increasing threat to human health [43]. Environmentally friendly garbage disposal and the conversion of garbage into wealth have become critical issues for all countries to achieve sustainable growth and establish a scientific ecosystem [10]. Choosing the most appropriate location for garbage disposal is one of the most essential elements in assuring successful garbage disposal. An ideal garbage disposal location will minimize the enterprise's operating costs, increase the efficiency of rubbish disposal and improve the preservation of the city's environment. Environment, natural conditions, social factors and economic factors are all significant in GDS selection. Soil, water and air pollution are the three most significant environmental problems [54]. The waste water produced from a high number of landfills in a short period of time may rupture the leachate collection system, damaging the other cover film. Plastic garbage particles are so small that they can enter into the human body through water, causing a great risk for the health of an individual [53]. Plastic materials, heavy metals, cleaning products and other harmful chemicals in the garbage will end up in the soil while occupying many areas. Air pollution primarily implies that many germs are transported in the garbage, and the transmission of these germs to humans poses a severe health threat. Further, poisonous gases, dust and tiny particles produced by garbage decomposition spread into the air, causing suspended sulfur dioxide particles to reach the atmosphere, resulting in pollution from acid rain and dust. The primary and essential factor is the natural conditions for GDS selection. To maintain the water resistance of soil and rock density, the site must be kept away from unfavorable construction locations, water sources, rivers, and streams. Moreover, places far below ground level must be addressed [33,52]. This eliminates the need for many people to dig trenches and makes waste disposal more simple. Moreover, part of social factors is that allowing the public to participate and evaluate the establishment of the GDS is essential. Garbage disposal facilities will become interconnected and interdependent with society in the future as people's knowledge of environmental protection grows, as do quality of life and living environment needs [54]. If the public's negative perception of the GDS cannot be changed, and the odor is detected on a daily basis, negative impressions will grow. Economic factors are identified as the income and expenditure [53].
The evaluation procedure for selecting a GDS: Suppose an organization wants to select a GDS for plant. They invite a panel of experts to analyze an appropriate GDS location. Let {A1,A2,A3,A4} be four alternatives for a garbage disposal site, and we must select the ideal one. Let {χ1,χ2,χ3,χ4} be the attributes of each alternative based on the influencing factors determined as follows: environmental protection level (χ1), suitability of natural conditions (χ2), social benefit (χ3) and economic benefit (χ4) of the GDS. Because of uncertainty, the DMs' selection information is presented as q-ROHFR information. The weight vector for criteria is γ=(0.13,0.27,0.29,0.31)T. The following computations are carried out in order to evaluate the MCDM problem utilizing the defined approach for evaluating alternatives:
Step 1. The information of a professional expert is presented in the form of q-ROHFRSs, where the value of q is 3, as shown in Tables 2–5.
χ1 | χ2 | |
A1 | (((0.1,0.2,0.5),(0.3,0.4)),((0.8),(0.4,0.6))) | (((0.5,0.7),(0.5,0.6)),((0.4,0.5),(0.7,0.9))) |
A2 | (((0.6,0.7),(0.7,0.9)),((0.3,0.5),(0.6))) | (((0.2,0.4,0.5),(0.5)),((0.6,0.7),(0.3))) |
χ3 | χ4 | |
A1 | (((0.4),(0.3,0.7)),((0.5),(0.9))) | (((0.6),(0.7)),((0.6,0.8,0.9),(0.7,0.9))) |
A2 | (((0.8),(0.4,0.5,0.7)),((0.2,0.5),(0.4,0.5))) | (((0.8),(0.5)),((0.7),(0.1,0.3,0.4))) |
χ1 | χ2 | |
A3 | (((0.4,0.5,0.6),(0.6,0.7)),((0.9),(0.5))) | (((0.1),(0.5,0.6)),((0.4,0.6,0.7),(0.5,0.7))) |
A4 | (((0.4),(0.5,0.6)),((0.3,0.4),(0.8))) | (((0.4,0.5),(0.4)),((0.1,0.2),(0.2,0.3))) |
χ3 | χ4 | |
A3 | (((0.3),(0.7,0.8)),((0.7,0.8),(0.1,0.4,0.7))) | (((0.3,0.6),(0.8)),((0.7),(0.3))) |
A4 | (((0.3),(0.7,0.8)),((0.7),(0.6))) | (((0.6,0.7,0.9),(0.3,0.4)),((0.2,0.7),(0.7,0.8,0.9))) |
Step 2. The expert information is benefit type, so in this case, we do not need to normalize the q-ROHFRVs.
Step 3. In this problem, we consider only one expert for the collection of uncertain information. So, we do not need to find the collected information.
Step 4. Aggregation information of the alternative under the given list of attributes is evaluated using proposed AOps as follows:
Case 1. Aggregation information using the Einstein weighted averaging operator is presented in Table 6.
A1 | (({0.4934,0.5659,0.9634,0.56680.5143,0.5817},{0.0047,0.0113,0.0057,0.0136,0.0063,0.0150,0.0076,0.0181}),({0.5932,0.6759,0.9871,0.5777,0.6647,0.7320},{0.0281,0.0371,0.0370,0.0488,0.0423,0.0558,0.0556,0.0735})) |
A2 | (({0.7074,0.7160,0.8976,0.7074,0.7160,0.7252},{0.0105,0.0132,0.0187,0.0137,0.0171,0.0243}),({0.5584,0.5915,0.9867,0.6235,0.5714,0.6030,0.6057,0.6336},{0.0011,0.0032,0.0043,0.0013,0.0040,0.0054})) |
A3 | (({0.2916,0.4384,0.9834,0.4518,0.3556,0.4707},{0.0262,0.0302,0.0315,0.0364,0.0306,0.0353,0.0369,0.0426}),({0.6968,0.7310,0.9674,0.7522,0.7408,0.7695},{0.0011,0.0063,0.0079,0.0016,0.0063,0.0112})) |
A4 | (({0.4659,0.5233,0.9234,0.4899,0.5424,0.6876},{0.0063,0.0084,0.0073,0.0097,0.0076,0.0102,0.0088,0.0117}),({0.4784,0.5990,0.9543,0.6006,0.4852,0.6032,0.4879,0.6048},{0.0103,0.0119,0.0136,0.0155,0.0179,0.0204})) |
Case 2. The score values from Tables 2–5 have been computed and are presented in Table 7. The information has been ordered on the basis of the score values, as shown in Tables 8–11. Aggregation information using the Einstein ordered weighted averaging operator is given in Table 12.
χ1 | χ2 | χ3 | χ4 | |
A1 | 0.5542 | 0.4250 | 0.3750 | 0.4667 |
A2 | 0.4000 | 0.5542 | 0.5417 | 0.6833 |
A3 | 0.5625 | 0.3792 | 0.4750 | 0.5125 |
A4 | 0.3500 | 0.4875 | 0.4125 | 0.5083 |
χ1 | χ2 | |
A1 | (((0.1,0.2,0.5),(0.3,0.4)),((0.8),(0.4,0.6))) | (((0.6),(0.7)),((0.6,0.8,0.9),(0.7,0.9))) |
A2 | (((0.8),(0.5)),((0.7),(0.1,0.3,0.4))) | (((0.2,0.4,0.5),(0.5)),((0.6,0.7),(0.3))) |
χ3 | χ4 | |
A1 | (((0.5,0.7),(0.5,0.6)),((0.4,0.5),(0.7,0.9))) | (((0.4),(0.3,0.7)),((0.5),(0.9))) |
A2 | (((0.8),(0.4,0.5,0.7)),((0.2,0.5),(0.4,0.5))) | (((0.6,0.7),(0.7,0.9)),((0.3,0.5),(0.6))) |
χ1 | χ2 | |
A3 | (((0.4,0.5,0.6),(0.6,0.7)),((0.9),(0.5))) | (((0.3,0.6),(0.8)),((0.7),(0.3))) |
A4 | (((0.6,0.7,0.9),(0.3,0.4)),((0.2,0.7),(0.7,0.8,0.9))) | (((0.4,0.5),(0.4)),((0.1,0.2),(0.2,0.3))) |
χ3 | χ4 | |
A3 | (((0.3),(0.7,0.8)),((0.7,0.8),(0.1,0.4,0.7))) | (((0.1),(0.5,0.6)),((0.4,0.6,0.7),(0.5,0.7))) |
A4 | (((0.3),(0.7,0.8)),((0.7),(0.6))) | (((0.4),(0.5,0.6)),((0.3,0.4),(0.8))) |
A1 | (({0.4865,0.5656,0.4878,0.5665,0.5081,0.5814},{0.4419,0.5744,0.4669,0.6049,0.4583,0.5945,0.4841,0.6257}),({0.5898,0.5729,0.6640,0.6513,0.7238,0.7139},{0.7150,0.7732,0.7692,0.8277,0.7481,0.8067,0.8026,0.8609})) |
A2 | (({0.6682,0.6957,0.6783,0.7048,0.6889,0.7144},{0.5235,0.5766,0.5573,0.6127,0.6149,0.6737}),({0.4876,0.5265,0.5306,0.5637,0.5343,0.5669,0.5704,0.5991},{0.3535,0.3777,0.4065,0.4339,0.4217,0.4500})) |
A3 | (({0.2875,0.4372,0.3167,0.4507,0.3529,0.4697},{0.6461,0.6810,0.6738,0.7094,0.6591,0.6944,0.6872,0.7230}),({0.6896,0.7180,0.7408,0.7247,0.7495,0.7695},{0.2751,0.3088,0.4092,0.4578,0.4847,0.5407})) |
A4 | (({0.4187,0.4480,0.4502,0.4758,0.5473,0.5647},{0.4897,0.5188,0.5127,0.5429,0.5075,0.5375,0.5313,0.5622}),({0.4833,0.4989,0.4859,0.5014,0.5400,0.5525,0.5421,0.5545},{0.5111,0.5659,0.5217,0.5773,0.5325,0.5889})) |
Case 3. The weighted information is depicted in Tables 13 and 14, and the score values of the weighted matrix are given in Table 15. The ordered information is presented in Tables 16 and 17, respectively. Finally, the aggregation information using the Einstein hybrid weighted averaging operator is shown in Table 18.
χ1 | χ2 | |
A1 | (((0.0479,0.0958,0.2400),(0.9159,0.9339)),((0.3960),(0.9339,0.9599))) | (((0.3276,0.4638),(0.8609,0.8933)),((0.3276,0.2618),(0.8989,0.9734))) |
A2 | (((0.2890,0.3400),(0.9703,0.9897)),((0.1438,0.2400),(0.9599))) | (((0.1308,0.2618,0.3276),(0.8609)),((0.3944,0.4638),(0.7285))) |
A3 | (((0.1917,0.2400,0.2890),(0.9599,0.9703)),((0.4666),(0.9481))) | (((0.0654),(0.8609,0.8933)),((0.2618,0.3944,0.4638),(0.8609,0.9217))) |
A4 | (((0.1917),(0.9481,0.9599)),((0.1438,0.1917),(0.9801))) | (((0.2618,0.3276),(0.8222)),((0.0654,0.1308),(0.7058,0.7733))) |
χ3 | χ4 | |
A1 | (((0.2679),(0.7563,0.9158)),((0.3352),(0.9715))) | (((0.4080),(0.9128)),((0.4080,0.5578,0.6539),(0.9128,0.9705))) |
A2 | (((0.5518),(0.8087,0.8503,0.9158)),((0.1339,0.3352),(0.8087,0.8503))) | (((0.5578),(0.8450)),((0.4797),(0.5574,0.7478,0.8020))) |
A3 | (((0.2008),(0.9158,0.9440)),((0.4745,0.5518),(0.5704,0.8087,0.9158))) | (((0.2031,0.4080),(0.9421)),((0.4797),(0.7478))) |
A4 | (((0.2008),(0.9158,0.9440)),((0.4745),(0.8851))) | (((0.4080,0.4797,0.6539),(0.7478,0.8020)),((0.1354,0.4797),(0.9128,0.9421,0.9705))) |
χ1 | χ2 | χ3 | χ4 | |
A1 | 0.1630 | 0.2193 | 0.1989 | 0.2734 |
A2 | 0.1416 | 0.2700 | 0.2746 | 0.3725 |
A3 | 0.1984 | 0.1676 | 0.2548 | 0.2738 |
A4 | 0.1064 | 0.2078 | 0.2151 | 0.2762 |
χ1 | χ2 | |
A1 | (((0.4080),(0.9128)),((0.4080,0.5578,0.6539),(0.9128,0.9705))) | (((0.3276,0.4638),(0.8609,0.8933)),((0.3276,0.2618),(0.8989,0.9734))) |
A2 | (((0.5578),(0.8450)),((0.4797),(0.55740.74780.8020))) | (((0.5518),(0.80870.8503,0.9158)),((0.13390.3352),(0.80870.8503))) |
A3 | (((0.2031,0.4080),(0.9421)),((0.4797),(0.7478))) | (((0.2008),(0.9158,0.9440)),((0.4745,0.5518),(0.5704,0.8087,0.9158))) |
A4 | (((0.4080,0.4797,0.6539),(0.7478,0.8020)),((0.1354,0.4797),(0.9128,0.9421,0.9705))) | (((0.2008),(0.9158,0.9440)),((0.4745),(0.8851))) |
χ3 | χ4 | |
A1 | (((0.2679),(0.7563,0.9158)),((0.3352),(0.9715))) | (((0.0479,0.0958,0.2400),(0.9159,0.9339)),((0.3960),(0.9339,0.9599))) |
A2 | (((0.1308,0.2618,0.3276),(0.8609)),((0.3944,0.4638),(0.7285))) | (((0.2890,0.3400),(0.9703,0.9897)),((0.1438,0.2400),(0.9599))) |
A3 | (((0.1917,0.2400,0.2890),(0.9599,0.9703)),((0.4666),(0.9481))) | (((0.0654),(0.8609,0.8933)),((0.2618,0.3944,0.4638),(0.8609,0.9217))) |
A4 | (((0.2618,0.3276),(0.8222)),((0.0654,0.1308),(0.7058,0.7733))) | (((0.1917),(0.9481,0.9599)),((0.1438,0.1917),(0.9801))) |
A1 | (({0.3647,0.3527,0.3967,0.3866,0.42520.4165},{0.9331,0.9412,0.9531,0.9610,0.9406,0.9487,0.9604,0.9681}),({0.3647,0.3527,0.3967,0.3866,0.4252,0.4165},{0.9331,0.9412,0.9531,0.9610,0.9406,0.9487,0.9604,0.9683})) |
A2 | (({0.4246,0.4331,0.4329,0.4410,0.4415,0.4494},{0.8802,0.8869,0.8912,0.8978,0.9087,0.9152}),({0.3233,0.3336,0.3556,0.3643,0.3511,0.3600,0.3791,0.3867},{0.7996,0.8109,0.8260,0.8373,0.8331,0.8443})) |
A3 | (({0.1755,0.1946,0.2180,0.2361,0.2473,0.2626},{0.9157,0.9254,0.9189,0.9286,0.9234,0.9330,0.9266,0.9362}),({0.4279,0.4510,0.4696,0.4564,0.4768,0.4935},{0.7915,0.8110,0.8585,0.8776,0.8874,0.9061})) |
A4 | (({0.2641,0.2861,0.2884,0.6061,0.3606,0.3729},{0.8781,0.8821,0.8861,0.8900,0.8850,0.8889,0.8929,0.8968}),({0.3117,0.3159,0.3136,0.3178,0.3539,0.3573,0.3554,0.3587},{0.8686,0.8879,0.8726,0.8918,0.8765,0.8957})) |
Step 5. Table 19 and Figure 3 show the score values for all alternatives under established aggregation operators.
Operators | SR(A1) | SR(A2) | SR(A3) | SR(A4) |
q-ROHFREWA | 0.4690 | 0.6064 | 0.5051 | 0.5181 |
q-ROHFREOWA | 0.4665 | 0.5597 | 0.5052 | 0.4823 |
q-ROHFRHWA | 0.2198 | 0.2680 | 0.2259 | 0.2322 |
Step-6. The alternatives Ak(k=1,2,...,4) have been arranged in ascending order, as shown in Table 20.
Operator | Scores | Best Alternative |
q-ROHFREWA | SR(A2)>SR(A4)>SR(A3)>SR(A1) | A2 |
q-ROHFREOWA | SR(A2)>SR(A3)>SR(A4)>SR(A1) | A2 |
q-ROHFRHWA | SR(A2)>SR(A4)>SR(A3)>SR(A1) | A2 |
Based on the results of the preceding computational procedure, we determined that the alternative A2 is the best option among all and is thus strongly suggested.
Garbage disposal plant site selection involves a complex process that requires a thorough understanding of the determination aspects of the process. The following are some of the most significant factors that should be considered while selecting a location for a garbage disposal plant:
(i) Environmental protection level: One of the most critical aspects of site selection is considering the environmental factors. This includes the potential impact on air and water quality, noise pollution, land use and local biodiversity. The site should be located in an area that minimizes any negative environmental impact.
(ii) Suitability of natural conditions: The suitability of natural conditions and the geographic location of the site is another critical factor in the site selection process. The site should be easily accessible and located in a region with a significant population and a consistent supply of waste materials. It includes the availability of infrastructure, such as roads, water supply, electricity, and communication systems.
(iii) Social benefit: Social factors such as the local community's attitude towards waste disposal, employment opportunities, and economic benefits are also important in the site selection process.
(iv) Economic benefit: The economic viability of the site is a crucial factor in site selection. The site should be cost-effective to operate, and the waste disposal fees should be competitive with other options. It includes the availability of infrastructure, such as roads, water supply, electricity, and communication systems.
Garbage disposal plant site selection requires a thorough consideration of environmental, geographic, technical, social, legal and economic factors to ensure safe and efficient operation. The present study demonstrates a prudent development in selection of a site for garbage disposal by incorporating the key variables for assessment, namely environmental, geographic, technical, social, legal, and economic factors. This has been accomplished to ensure a safe and efficient operation, and requires the implementation of appropriate strategies through a compact model that is based on a high degree of sustainability. The site selection process should adhere to local, state and federal laws and regulations. Compliance with these regulations ensures that the plant operates in a safe and efficient manner. Global warming and climate change are serious environmental threats. To maintain environmental sustainability, site selection has to contemplate technological feasibility.
The q-ROHFREWAA consists of the parameter q which gives the q-ROHFREWAA significant flexibility in handling fuzzy information. In our case, the value q = 3 was used. We study the possible variation in the results obtained from the q-ROHFREWAA operator. In the following, Table 21 and Figure 4 show the ranking orders based on score values obtained through the q-ROHFREWAA operator. The parameter q, was varied with values of 1 to 5, 10, 15, 20, 50 and 100, and the alternatives were arranged in ascending order based on q-ROHFR score values. When the value q in the q-ROHFREWAA was varied, the optimal alternative was always the same for 1 to 5 and 10, as were the orderings of alternatives (A2≻ A4≻ A3≻ A1). For the values 15, 20, 50 and 100, the optimal alternative was always the same, as were the orderings of alternatives (A2≻ A4≻ A1≻ A3). Using the q-ROHFREWAA operator and the score function, A2 had the highest score value, as the optimal alternative. A3 had the lowest score for the parameter values 1 to 5 and 10, and A1 had the lowest score for the parameter values 15, 20, 50 and 100, as presented in Table 21. Therefore, the most appropriate alternative was the same for all values of q among 1 to 5, 10, 15, 20, 50 and 100. Figure 4 depicts a graphical representation of Table 21. The effect of varying q on ranking order using the q-ROHFREWAA operator is visualized in Figure 4.
q | Score values of alternatives | Ranking | Best Alternative | |||
A1 | A2 | A3 | A4 | |||
1 | 0.5992 | 0.7522 | 0.6395 | 0.6623 | A2≻A4≻ A3≻A1 | A2 |
2 | 0.5122 | 0.6657 | 0.5545 | 0.5698 | A2≻A4≻ A3≻A1 | A2 |
3 | 0.4690 | 0.6064 | 0.5051 | 0.5181 | A2≻A4≻ A3≻A1 | A2 |
4 | 0.4441 | 0.5657 | 0.4734 | 0.4868 | A2≻A4≻ A3≻A1 | A2 |
5 | 0.4282 | 0.5366 | 0.4518 | 0.4660 | A2≻A4≻ A3≻A1 | A2 |
10 | 0.3969 | 0.4658 | 0.4033 | 0.4185 | A2≻A4≻ A3≻A1 | A2 |
15 | 0.3876 | 0.4382 | 0.3874 | 0.4003 | A2≻A4≻ A1≻A3 | A2 |
20 | 0.3833 | 0.4235 | 0.3804 | 0.3905 | A2≻A4≻ A1≻A3 | A2 |
50 | 0.3759 | 0.3953 | 0.3537 | 0.3717 | A2≻A4≻ A1≻A3 | A2 |
100 | 0.2989 | 0.3844 | 0.2306 | 0.3144 | A2≻A4≻ A1≻A3 | A2 |
In this section, we compare the outcomes with Ashraf et al. [55] and the enhanced TOPSIS and VIKOR methodologies for the q-ROHFR information in order to deal with MCGDM problems and to verify the results obtained through the suggested aggregation operators. To demonstrate the importance and credibility of the developed q-ROHFR Einstein weighted averaging aggregation operators, a comparison of their features with those of existing decision support approaches is presented.
Ashraf et al. [55] developed the list of q-rung orthopair fuzzy rough weighted aggregation operators to sort out the best alternative. We take the collective expert information from Ashraf et al. [55] to evaluate the finest alternative. The collective expert information is displayed in Table 22 as follows:
χ1 | χ2 | χ3 | χ4 | χ5 | |
A1 | ((0.64,0.13),(0.46,0.15)) | ((0.8,0.16),(0.63,0.26)) | ((0.75,0.22),(0.58,0.18)) | ((0.67,0.13),(0.60,0.17)) | ((0.78,0.1),(0.67,0.15)) |
A2 | ((0.55,0.15),(0.62,0.24)) | ((0.71,0.18),(0.4,0.26)) | ((0.43,0.17),(0.51,0.20)) | ((0.61,0.26),(0.40,0.20)) | ((0.63,0.19),(0.46,0.26)) |
A3 | ((0.57,0.24),(0.40,0.27)) | ((0.71,0.17),(0.65,0.23)) | ((0.49,0.22),(0.36,0.39)) | ((0.54,0.40),(0.45,0.36)) | ((0.56,0.36),(0.32,0.18)) |
A4 | ((0.56,0.17),(0.59,0.23)) | ((0.50,0.32),(0.43,0.42)) | ((0.27,0.13),(0.36,0.29)) | ((0.46,0.19),(0.37,0.36)) | ((0.49,0.25),(0.37,0.26)) |
Now, we utilize the q-ROHFR Einstein weighted average aggregation operator and the q-ROHFR Einstein ordered weighted average aggregation operator to find out the best alternative presented in Table 23 as follows:
A1 | ((0.7338,0.1396),(0.6058,0.1743)) |
A2 | ((0.5992,0.1959),(0.4774,0.2263)) |
A3 | ((0.5717,0.2878),(0.4502,0.2807)) |
A4 | ((0.4656,0.1988),(0.4204,0.3077)) |
Comparative analysis of the proposed operators with collected expert data by Ashraf et al. [55] is expressed in Table 24:
Score | |||||
Ashraf et al. | SR(A1) | SR(A2) | SR(A3) | SR(A4) | Ranking |
q-ROFREWA | 0.999 | 0.489 | 0.201 | 0.011 | A1>A2>A3>A4 |
Score | |||||
Developed method | SR(A1) | SR(A2) | SR(A3) | SR(A4) | Ranking |
q-ROHFREWA | 0.7564 | 0.6636 | 0.6134 | 0.5949 | A1>A2>A3>A4 |
q-ROHFREOWA | 0.7612 | 0.6741 | 0.6166 | 0.5991 | A1>A2>A3>A4 |
Comparison findings show that the proposed operators are generalized and effective to for aggregating uncertain information as compared to the existing operators developed by Ashraf et al. [55]. For this reason, our recommended techniques are likely to become more comprehensive and more adaptable to control q-rung orthopair hesitant fuzzy rough MADM challenges.
The Technique for Order of Preference by Similarity to Ideal Solution (TOPSIS) method is widely used in various fields, including engineering, management, and social sciences, for decision-making in situations where there are multiple criteria to be considered. It is a simple and effective method that provides a clear and systematic approach to decision-making. However, it requires a clear understanding of the problem and careful consideration of the criteria and their weights. The TOPSIS is a MADM method used for selecting the best option among a set of alternatives based on a set of criteria. This method was first introduced by Hwang and Yoon [57] in 1981 for solutions and allows policy makers and authorities to assess positive ideal solutions (PIS) and negative ideal solutions (NIS). The TOPSIS is established on the assumption that the optimal option is the one that is closest to the ideal while being the furthest distant from the perfect negative solutions [56,59]. The TOPSIS method involves the following steps:
Step 1. Let the set of alternatives be A={A1,A2,A3,...,Am} and χ={χ1,χ2,χ3,...,χr} be the set of criteria. The expert decision matrix is presented as
M=[ℶ_(κ^zııj),¯ℶ(κ^zııj)]m×n=[(ℶ_(κ11),¯ℶ(κ11))(ℶ_(κ12),¯ℶ(κ12))⋯(ℶ_(κ1j),¯ℶ(κ1j))(ℶ_(κ21),¯ℶ(κ21))(ℶ_(κ22),¯ℶ(κ22))⋯(ℶ_(κ2j),¯ℶ(κ2j))(ℶ_(κ31),¯ℶ(κ31))(ℶ_(κ32),¯ℶ(κ32))⋯(ℶ_(κ3j),¯ℶ(κ3j))⋮⋮⋱⋮(ℶ_(κı1),¯ℶ(κı1))(ℶ_(κı2),¯ℶ(κı2))⋯(ℶ_(κıj),¯ℶ(κıj))], |
where
¯ℶ(κıj)={⟨∝ı,βh¯ℶ(κ)(∝ı),ψh¯ℶ(κ)(∝ı)⟩|∝ı∈T} |
and
ℶ_(κ)={⟨∝ı,βhℶ_(κ)(∝ı),ψhℶ_(κ)(∝ı)⟩|∝ı∈T} |
such that
0≤(max(βh¯ℶ(κ)(∝ı)))q+(min(ψh¯ℶ(κ)(∝ı)))q≤1 |
and
0≤(min(βhℶ_(κ)(∝ı))q+(max(ψhℶ_(κ)(∝ı)))q≤1 |
are the q-ROHF rough values.
Step 2. Initially, we collect information from decision makers based of q-ROHFRNs.
Step 3. Second, we normalize the information provided by DMs, since the decision matrix may involve both benefit and cost criteria, as depicted below:
(H)^zı=[(¯ℶ(κ^zı11),ℶ_(κ^zı11))(¯ℶ(κ^zı12),ℶ_(κ^zı12))⋯(¯ℶ(κˆ∝ı1j),ℶ_(κ^zı1j))(¯ℶ(κ^zı21),ℶ_(κ^zı21))(¯ℶ(κ^zı22),ℶ_(κ^zı22))⋯(¯ℶ(κˆ∝ı2j),ℶ_(κ^zı2j))(¯ℶ(κ^zı31),ℶ_(κ^zı31))(¯ℶ(κ^zı32),ℶ_(κ^zı32))⋯(¯ℶ(κˆ∝ı3j),ℶ_(κ^zı3j))⋮⋮⋱⋮(¯ℶ(κ^zıı1),ℶ_(κ^zıı1))(¯ℶ(κ^zıı2),ℶ_(κ^zıı2))⋯(¯ℶ(κˆ∝ııj),ℶ_(κ^zııj))] |
where ^zı represents the number of experts.
Step 4. We examine the expert matrices that are normalized (N)^zı, as
(N)^zı={ℶ(κıj)=(ℶ_(κıj),¯ℶ(κıj))iffor benefit, (ℶ(κıj))c=((ℶ_(κıj))c,(¯ℶ(κıj))c)iffor cost . |
Step 5. The PIS and NIS are computed based on the score value. The PIS and NIS are referred to as ℸ+=(ℷ+1,ℷ+2,ℷ+3,...ℷ+r) and ℸ−=(ℷ−1,ℷ−2,ℷ−3,...,ℷ−r), respectively. PIS ℸ+ is determined using the formula
ℸ+=(ℷ+1,ℷ+2,ℷ+3,...,ℷ+r)=(maxıscore(ℷı1),maxıscoreℷı2,maxıscoreℷı3,...,maxıscoreℷın). |
Similarly, the NIS is determined with the following formula:
ℸ−=(ℷ−1,ℷ−2,ℷ−3,...,ℷ−r)=(minıscoreℷı1,minıscoreℷı2,minıscoreℷı3,...,minıscoreℷır). |
After that, we compute the geometric distances between all of the alternatives and PIS ℸ+ as follows:
d(αıj,ℸ+)=18((1#ℵ∑#ℵs=1|(μ_ıj(s))2−(μ_+ı)2|+|(¯μıj(s))2−(¯μ+ı(s))2|)+(1#H∑#Hs=1|(ν_ıj(s))2−(ν_+ı(s))2|+|(¯νhıj)2−(¯νh+ı)2|)), where ı=1,2,3,...,r,and j=1,2,3,...,m. |
Where #ℵ and #H represents the number of elements in membership and non-membership, respectively. Consequently, the geometric distances between all alternatives and NIS ℸ− are described as follows:
d(αıj,ℸ−)=18((1#ℵ∑#ℵs=1|(μ_ıj(s))2−(μ_−ı(s))2|+|(¯μıj(s))2−(¯μ−ı(s))2|)+(1#H∑#Hs=1|(ν_ıj(s))2−(ν_−ı(s))2|+|(¯νhıj)2−(¯νh−ı)2|)),where ı=1,2,3,...,r,and j=1,2,3,...,m. |
Step 6. The following is a description of the relative closeness indices that were calculated for each decision maker based on the alternatives:
RC(αıj)=d(αıj,ℸ+)d(αıj,ℸ−)+d(αıj,ℸ+). |
Step 7. The most desirable alternative with the shortest distance may be chosen by determining the ranking orders of the alternatives.
To illustrate the viability of the method, a numerical example applicable to "site selection for a garbage disposal plant" is presented as follows:
Step 1. Tables 2–5 contain decision makers information based on q-ROHFRNs.
Step 2. Table 25 determines PIS and NIS as follows:
Criteria | ℸ+ | ℸ− |
χ1 | (((0.4,0.5,0.6),(0.6,0.7)),((0.9),(0.5))) | (((0.4),(0.5,0.6)),((0.3,0.4),(0.8))) |
χ2 | (((0.2,0.4,0.5),(0.5)),((0.6,0.7),(0.3))) | (((0.1),(0.5,0.6)),((0.4,0.6,0.7),(0.5,0.7))) |
χ3 | (((0.8),(0.4,0.5,0.7)),((0.2,0.5),(0.4,0.5))) | (((0.4),(0.3,0.7)),((0.5),(0.9))) |
χ4 | (((0.8),(0.5)),((0.7),(0.1,0.3,0.4))) | (((0.6),(0.7)),((0.6,0.8,0.9),(0.7,0.9))) |
Step 3. The PIS and NIS distances are calculated as follows:
![]() |
and
![]() |
Step 4. The following are the relative closeness indices of the alternatives for decision makers:
![]() |
Step 5. According to the aforementioned ranking of alternatives visualized in Figure 5, A2 has the shortest distance. Hence, A2 is the best option.
VIKOR (Vise Kriterijumska Optimizacija I Kompromisno Resenje), is a decision-making method used to rank a set of alternatives based on multiple criteria. The VIKOR technique is particularly useful in situations where the decision-maker needs to consider both the optimal solution and the level of compromise among the alternatives. The method allows for the consideration of multiple criteria and multiple alternatives, and it can help to identify the best compromise solution. The VIKOR method is widely used in various fields, including engineering, management, and social sciences, for decision-making in situations where there are multiple criteria to be considered. It provides a systematic and objective approach to decision-making and allows the decision-maker to identify the best compromise solution among a set of alternatives. The following is a detailed explanation of the modified form of the VIKOR approach based on q-ROHFR information:
Step 1. Identify the problem and the decision criteria. Construct evaluation matrices for the experts in the form of q-ROHFRVs that shows the performance of each alternative on each criterion.
Step 2. Through using the q-ROHFRWA aggregation operator, compute the collected information of decision makers along their weights vector and obtain the aggregated decision matrix.
Step 3. Compute the positive ideal solutions (PIS) ℸ+ and negative ideal solutions (NIS) ℸ− in the form of q-ROHFR information as follows:
ℸ+=(Y+1,Y+2,Y+3,...,Y+ℓ)=(maxıYı1,maxıYı2,maxıYı3,...,maxıYın.),ℸ−=(Y−1,Y−2,Y−3,...,Y−ℓ)=(minıYı1,minıYı2,minıYı3,...,minıYın.). |
Step 4. Determine the q-ROHFR group utility measure Sı(ı=1,2,3,...,ℓ) and the regret measure Rı(ı=1,2,3,...,ℓ) for all alternatives L=(A1,A2,A3,...,Aℓ) applying the formulas mentioned below:
Sı=ℓ⨁j=1∝jd(Yıj,Y+j)d(Y+j,Y−j), ı=1,2,3,4,...,m,Rı=max∝jd(Yıj,Y+j)d(Y+j,Y−j), ı=1,2,3,4,...,m. |
Step 5. Determine the maximum and minimum values of S and R, respectively, as follows:
S⋆=minıSı, S◃=maxıSı, R⋆=minıRı, R◃=maxıRı, ı=1,2,3,...,ℓ. |
Lastly, we integrate the features of both the group utility Sı and the individual regret Rı in order to assess the ranking measure Qı for the alternatives L=(A1,A2,A3,...,Aℓ) as follows:
Qı=χSı−S⋆S◃−S⋆+(1−χ)Rı−R⋆R◃−R⋆,Rı, ı=1,2,3,...,ℓ, |
where χ is the strategic weight of the majority of parameters (the parameter with the largest group utility) and is essential for assessing the compromise solution. The value is chosen from the range [0,1], and 0.5 is a common number and was utilized it.
Step 6. Furthermore, the alternatives are ordered in decreasing order for the group utility measure Si, individual regret measure Rı, and ranking measure Qı. Here, we obtained three ranking lists that will help us determine the best compromise alternative.
In this section, we implement an improved q-ROHFR-VIKOR approach to the MAGDM problem in order to identify the best location for a GDS using four criteria mentioned in the following numerical example.
Step 1. The information based on q-ROHFRVs of the three professional experts is analyzed in Tables 2–5.
Step 2. The aggregated information of the team of experts, as determined by the q-ROHFRWA aggregation operator, is summarized in Table 6.
Step 3. The q-ROHFR PIS (ℸ+) and the q-ROHFR NIS (ℸ−) are computed in Table 25.
Step 4. The q-ROHFR group utility measure values Sı(ı=1,2,3,4) and the regret measure Rı(ı=1,2,3,4) of the alternatives under consideration are summarized in Table 26.
alternatives | Sı | Rı | Qı |
A1 | 0.1460 | 0.2472 | 0.2137 |
A2 | 0.5324 | 0.4042 | 0.5631 |
A3 | 0.3637 | 0.3781 | 0.4319 |
A4 | 0.3426 | 0.2682 | 0.3142 |
Steps-5 & 6. Alternative rankings based on the group utility measure Sı, the individual regret measure Rı, and the ranking measure Qı are indicated in Table 27. Figure 6 depicts a graphical illustration of the rankings according to the modified VIKOR approach.
Alternatives | Ranking order of Sı | Ranking order of Rı | Ranking order of Qı |
A1 | 4 | 4 | 4 |
A2 | 1 | 1 | 1 |
A3 | 2 | 2 | 2 |
A4 | 3 | 3 | 3 |
There are numerous factors to consider while choosing a garbage disposal location, but human intelligence has certain limitations. Hence, it is essential to develop an efficient framework for analyzing garbage disposal location. Many researchers have addressed various approaches for evaluating garbage disposal location schemes, particularly the assessment approach based on fuzzy set theory. The basic technology of the ongoing fuzzy theory-based evaluation technique is to provide the assessment value via the membership function of the fuzzy set, then utilize the operational rules between fuzzy numbers for information aggregation and, lastly, obtain the evaluation result. However, the expression space for analyzing the information in fuzzy sets is restricted, making it difficult to handle problems of assessing GDS options in complex environmental situations. Therefore, a novel approach based on q-ROHFRS has been proposed for evaluation to overcome these limitations. It is worth noting that the approach presented in this study provides a broad space for analyzing information, enabling DMs to incorporate the features of uncertain data and have high computing capabilities for imprecise information. On the basis of the Einstein t-norm and t-conorm, several aggregation operators, the q-ROHFREWA, q-ROHFREOWA and q-ROHFREHWA operators, are described. In addition, a comprehensive discussion is presented on the fundamental concepts exhibited by the developed operators. The aforementioned operators consider the complexity of the MADM approach based on the q-ROHFR operators, and the evaluation information has significant rationality. A case study in the assessment of GDS location is also utilized to show the applicability and validity of the proposed technique. According to the empirical results, utilizing the suggested approach to perform the final site selection based on the ranking findings is appropriate and practical. We assessed the impact of variations of parameters on the decision-making outcomes through sensitivity analysis of the proposed aggregation operators. The findings indicated that the outcomes were consistent. The present study involves a comparative analysis of the proposed models with the existing literature outcomes. Additionally, an evaluation is carried out utilizing the extended q-ROHFR-TOPSIS and VIKOR methodologies in order to demonstrate the viability, superiority and reliability of the proposed techniques. The findings of the comparison indicated that the novel approach provides numerous benefits in assessing and analyzing the consequences of high-value assessment information.
Future recommendations and limitations of the study: The method is beneficial for top-level executives who make decisions with conflicting paths in areas such as planning and control, industrial production, financing decisions, and health-care planning processes. There are numerous directions and ideas for future research in this field. As a follow-up to this study, future work could investigate the application of linguistic term usage to the structure explored here. Since q-ROHF rough set theory presupposes discrete values, it might not be applicable for all types data. The q-ROHF rough set theory may not work for continuous or mixed information. Fuzzy rough set theory computational complexity grows significantly with dataset advancement. This makes the theory inappropriate for enormous data sets.
The authors declare they have not used Artificial Intelligence (AI) tools in the creation of this article.
The authors acknowledge Princess Nourah bint Abdulrahman University Researchers Supporting Project number (PNURSP2023R443), Princess Nourah bint Abdulrahman University, Riyadh, Saudi Arabia. This study was supported via funding from Prince Sattam Bin Abdulaziz University project number (PSAU/2023/R/1444).
The authors declare no competing interests.
[1] |
Z. Raizah, U. K. K. Nanjappa, H. U. A. Shankar, U. Khan, S. M. Eldin, R. Kumar, et al., Windmill global sourcing in an initiative using a spherical fuzzy multiple-criteria decision prototype, Energies, 15 (2022), 8000. https://doi.org/10.3390/en15218000 doi: 10.3390/en15218000
![]() |
[2] |
M. M. Lashin, M. I. Khan, N. B. Khedher, S. M. Eldin, Optimization of display window design for females clothes for fashion stores through artificial intelligence and fuzzy system, Appl. Sci., 12 (2022), 11594. https://doi.org/10.3390/app122211594 doi: 10.3390/app122211594
![]() |
[3] |
G. Shahzadi, F. Zafar, M. A. Alghamdi, Multiple-attribute decision-making using Fermatean fuzzy Hamacher interactive geometric operators, Math. Prob. Eng., 2021, 1–20. https://doi.org/10.1155/2021/5150933 doi: 10.1155/2021/5150933
![]() |
[4] |
S. Ashraf, S. N. Abbasi, M. Naeem, S. M. Eldin, Novel decision aid model for green supplier selection based on extended EDAS approach under pythagorean fuzzy Z-numbers, Front. Env. Sci., 11 (2023), 342. https://doi.org/10.3389/fenvs.2023.1137689 doi: 10.3389/fenvs.2023.1137689
![]() |
[5] |
Attaullah, S. Ashraf, N. Rehman, A. Khan, M. Naeem, C. Park, A wind power plant site selection algorithm based on q-rung orthopair hesitant fuzzy rough Einstein aggregation information, Sci. Rep., 12 (2022), 5443. https://doi.org/10.1038/s41598-022-09323-5 doi: 10.1038/s41598-022-09323-5
![]() |
[6] |
Attaullah, S. Ashraf, N. Rehman, H. AlSalman, A. H. Gumaei, A decision-making framework using q-rung orthopair probabilistic hesitant fuzzy rough aggregation information for the drug selection to treat COVID-19, Complexity, 2022. https://doi.org/10.1155/2022/5556309 doi: 10.1155/2022/5556309
![]() |
[7] |
Attaullah, S. Ashraf, N. Rehman, A. Khan, C. Park, A decision making algorithm for wind power plant based on q-rung orthopair hesitant fuzzy rough aggregation information and TOPSIS, AIMS Math., 7 (2022), 5241–5274. https://doi.org/10.3934/math.2022292 doi: 10.3934/math.2022292
![]() |
[8] |
Attaullah, N. Rehman, A. Khan, G. Santos-Garcia, Fermatean hesitant fuzzy rough aggregation operators and their applications in multiple criteria group decision-making, Sci. Rep., 13 (2023), 6676. https://doi.org/10.1038/s41598-023-28722-w doi: 10.1038/s41598-023-28722-w
![]() |
[9] |
V. Nevrlý, R. Šomplák, O. Putna, M. Pavlas, Location of mixed municipal waste treatment facilities: Cost of reducing greenhouse gas emissions, J. Clean. Prod., 239 (2019), 118003. https://doi.org/10.1016/j.jclepro.2019.118003 doi: 10.1016/j.jclepro.2019.118003
![]() |
[10] |
A. Kumar, S. R. Samadder, A review on technological options of waste to energy for effective management of municipal solid waste, Waste Manage., 69 (2017), 407–422. https://doi.org/10.1016/j.wasman.2017.08.046 doi: 10.1016/j.wasman.2017.08.046
![]() |
[11] |
J. Song, Y. Sun, L. Jin, PESTEL analysis of the development of the waste-to-energy incineration industry in China, Renew. Sust. Energ. Rev., 80 (2017), 276–289. https://doi.org/10.1016/j.rser.2017.05.066 doi: 10.1016/j.rser.2017.05.066
![]() |
[12] |
H. Jiang, J. Zhan, D. Chen, PROMETHEE-Ⅱ method based on variable precision fuzzy rough sets with fuzzy neighborhoods, Artif. Intell. Rev., 54 (2021), 1281–1319. https://doi.org/10.1007/s10462-020-09878-7 doi: 10.1007/s10462-020-09878-7
![]() |
[13] |
L. A. Zadeh, Fuzzy sets, Inform. Control, 8 (1965), 338–353. https://doi.org/10.1016/S0019-9958(65)90241-X doi: 10.1016/S0019-9958(65)90241-X
![]() |
[14] | K. T. Atanassov, Intuitionistic fuzzy sets, In: Intuitionistic fuzzy sets, 1999, 1–137, Physica, Heidelberg. https://doi.org/10.1007/978-3-7908-1870-3_1 |
[15] |
S. Singh, S. Sharma, S. Lalotra, Generalized correlation coefficients of intuitionistic fuzzy sets with application to MAGDM and clustering analysis, Int. J. Fuzzy Syst., 22 (2020), 1582–1595. https://doi.org/10.3390/e23050563 doi: 10.3390/e23050563
![]() |
[16] |
R. R. Yager, A. M. Abbasov, Pythagorean membership grades, complex numbers, and decision making, Int. J. Intell. Syst., 28 (2013), 436–452. https://doi.org/10.1002/int.21584 doi: 10.1002/int.21584
![]() |
[17] |
O. Yanmaz, Y. Turgut, E. N. Can, C. Kahraman, Interval-valued Pythagorean fuzzy EDAS method: An application to car selection problem, J. Intell. Fuzzy Syst., 38 (2020), 4061–4077. https://doi.org/10.3233/JIFS-182667 doi: 10.3233/JIFS-182667
![]() |
[18] |
R. R. Yager, Generalized orthopair fuzzy sets, IEEE T. Fuzzy Syst., 25 (2016), 1222–1230. https://doi.org/10.1109/TFUZZ.2016.2604005 doi: 10.1109/TFUZZ.2016.2604005
![]() |
[19] |
C. Zhang, J. Ding, D. Li, J. Zhan, A novel multi-granularity three-way decision making approach in q-rung orthopair fuzzy information systems, Int. J. Approx. Reason., 138 (2021), 161–187. https://doi.org/10.1016/j.ijar.2021.08.004 doi: 10.1016/j.ijar.2021.08.004
![]() |
[20] |
C. Zhang, J. Ding, J. Zhan, A. K. Sangaiah, D. Li, Fuzzy intelligence learning based on bounded rationality in IoMT systems: A case study in Parkinson Disease, IEEE T. Comput. Soc. Syst., 2022. https://doi.org/10.1109/TCSS.2022.3221933 doi: 10.1109/TCSS.2022.3221933
![]() |
[21] |
C. Zhang, W. Bai, D. Li, J. Zhan, Multiple attribute group decision making based on multigranulation probabilistic models, MULTIMOORA and TPOP in incomplete q-rung orthopair fuzzy information systems, Int. J. Approx. Reason., 143 (2022), 102–120. https://doi.org/10.1016/j.ijar.2022.01.002 doi: 10.1016/j.ijar.2022.01.002
![]() |
[22] |
A. Hussain, M. I. Ali, T. Mahmood, Covering based q-rung orthopair fuzzy rough set model hybrid with TOPSIS for multi-attribute decision making, J. Intell. Fuzzy Syst., 37 (2019), 981–993. https://doi.org/10.3233/JIFS-181832 doi: 10.3233/JIFS-181832
![]() |
[23] |
S. Chakraborty, TOPSIS and modified TOPSIS: A comparative analysis, Decis. Anal. J., 2 (2022), 100021. https://doi.org/10.1016/j.dajour.2021.100021 doi: 10.1016/j.dajour.2021.100021
![]() |
[24] |
M. Hanine, O. Boutkhoum, A. Tikniouine, T. Agouti, Application of an integrated multi-criteria decision making AHP-TOPSIS methodology for ETL software selection, SpringerPlus, 5 (2016), 1–17. https://doi.org/10.1186/s40064-016-2233-2 doi: 10.1186/s40064-016-2233-2
![]() |
[25] |
P. Gupta, M. K. Mehlawat, N. Grover, Intuitionistic fuzzy multi-attribute group decision-making with an application to plant location selection based on a new extended VIKOR method, Inform. Sci., 370 (2016), 184–203. https://doi.org/10.1016/j.ins.2016.07.058 doi: 10.1016/j.ins.2016.07.058
![]() |
[26] |
A. Hafezalkotob, A. Hafezalkotob, Interval target-based VIKOR method supported on interval distance and preference degree for machine selection, Eng. Appl. Artif. Intel., 57 (2017), 184–196. https://doi.org/10.1016/j.engappai.2016.10.018 doi: 10.1016/j.engappai.2016.10.018
![]() |
[27] |
O. Soner, E. Celik, E. Akyuz, Application of AHP and VIKOR methods under interval type 2 fuzzy environment in maritime transportation, Ocean Eng., 129 (2017), 107–116. https://doi.org/10.3390/math11102249 doi: 10.3390/math11102249
![]() |
[28] |
M. Gul, E. Celik, N. Aydin, A. T. Gumus, A. F. Guneri, A state of the art literature review of VIKOR and its fuzzy extensions on applications, Appl. Soft Comput., 46 (2016), 60–89. https://doi.org/10.1016/j.asoc.2016.04.040 doi: 10.1016/j.asoc.2016.04.040
![]() |
[29] |
S. Opricovic, G. H. Tzeng, Extended VIKOR method in comparison with outranking methods, Eur. J. Oper. Res., 178 (2007), 514–529. https://doi.org/10.1016/j.ejor.2006.01.020 doi: 10.1016/j.ejor.2006.01.020
![]() |
[30] |
Z. Pawlak, Rough sets, Int. J. Comput. Inform. Sci., 11 (1982), 341–356. https://doi.org/10.1007/BF01001956 doi: 10.1016/j.ins.2021.04.016
![]() |
[31] |
T. M. Al-shami, An improvement of rough sets accuracy measure using containment neighborhoods with a medical application, Inform. Sci., 569 (2021), 110–124. https://doi.org/10.1016/j.ins.2021.04.016 doi: 10.1016/j.ins.2021.04.016
![]() |
[32] |
Z. Pawlak, A. Skowron, Rudiments of rough sets, Inform. Sci., 177 (2007), 3–27. https://doi.org/10.1016/j.ins.2006.06.003 doi: 10.1016/j.ins.2006.06.003
![]() |
[33] |
S. Sadek, M. El-Fadel, F. Freiha, Compliance factors within a GIS-based framework for landfill siting, Int. J. Env. Stud., 63 (2006), 71–86. https://doi.org/10.1080/00207230600562213 doi: 10.1080/00207230600562213
![]() |
[34] |
A. M. Radzikowska, E. E. Kerre, A comparative study of fuzzy rough sets, Fuzzy Set. Syst., 126 (2002), 137–155. https://doi.org/10.1016/S0165-0114(01)00032-X doi: 10.1016/S0165-0114(01)00032-X
![]() |
[35] |
W. Pan, K. She, P. Wei, Multi-granulation fuzzy preference relation rough set for ordinal decision system, Fuzzy Set. Syst., 312 (2017), 87–108. https://doi.org/10.1016/j.fss.2016.08.002 doi: 10.1016/j.fss.2016.08.002
![]() |
[36] |
Y. Li, S. Wu, Y. Lin, J. Liu, Different classes' ratio fuzzy rough set based robust feature selection, Knowl.-Based Syst., 120 (2017), 74–86. https://doi.org/10.1155/2021/6685396 doi: 10.1155/2021/6685396
![]() |
[37] |
T. Zhan, Granular-based state estimation for nonlinear fractional control systems and its circuit cognitive application, Int. J. Cogn. Comput. Eng., 4 (2023), 1–5. https://doi.org/10.1016/j.ijcce.2022.12.001 doi: 10.1016/j.ijcce.2022.12.001
![]() |
[38] |
X. Ren, D. Li, Y. Zhai, Research on mixed decision implications based on formal concept analysis, Int. J. Cogn. Comput. Eng., 4 (2023), 71–77. https://doi.org/10.1016/j.ijcce.2023.02.007 doi: 10.1016/j.ijcce.2023.02.007
![]() |
[39] |
K. Lian, T. Wang, B. Wang, M. Wang, W. Huang, J. Yang, The research on relative knowledge distances and their cognitive features, Int. J. Cogn. Comput. Eng., 2023. https://doi.org/10.1016/j.ijcce.2023.03.004 doi: 10.1016/j.ijcce.2023.03.004
![]() |
[40] |
T. Feng, H. T. Fan, J. S. Mi, Uncertainty and reduction of variable precision multigranulation fuzzy rough sets based on three-way decisions, Int. J. Approx. Reason., 85 (2017), 36–58. https://doi.org/10.1016/j.ins.2022.05.122 doi: 10.1016/j.ins.2022.05.122
![]() |
[41] |
B. Sun, W. Ma, X. Chen, X. Zhang, Multigranulation vague rough set over two universes and its application to group decision making, Soft Comput., 23 (2019), 8927–8956. https://doi.org/10.1007/s00500-018-3494-1 doi: 10.1007/s00500-018-3494-1
![]() |
[42] |
C. Y. Wang, B. Q. Hu, Granular variable precision fuzzy rough sets with general fuzzy relations, Fuzzy Set. Syst., 275 (2015), 39–57. https://doi.org/10.1007/s40314-023-02245-6 doi: 10.1007/s40314-023-02245-6
![]() |
[43] |
S. Vluymans, D. S. Tarragó, Y. Saeys, C. Cornelis, F. Herrera, Fuzzy rough classifiers for class imbalanced multi-instance data, Pattern Recog., 53 (2016), 36–45. https://doi.org/10.1016/j.patcog.2015.12.002 doi: 10.1016/j.patcog.2015.12.002
![]() |
[44] |
C. Y. Wang, B. Q. Hu, Fuzzy rough sets based on generalized residuated lattices, Inform. Sci., 248 (2013), 31–49. https://doi.org/10.1016/j.ins.2013.03.051 doi: 10.1016/j.ins.2013.03.051
![]() |
[45] |
H. Zhang, L. Shu, S. Liao, C. Xiawu, Dual hesitant fuzzy rough set and its application, Soft Comput., 21 (2017), 3287–3305. https://doi.org/10.1007/s00500-015-2008-7 doi: 10.1007/s00500-015-2008-7
![]() |
[46] |
D. Peng, J. Wang, D. Liu, Y. Cheng, The interactive fuzzy linguistic term set and its application in multi-attribute decision making, Artif. Intell. Medicine, 131 (2022), 102345. https://doi.org/10.1016/j.artmed.2022.102345 doi: 10.1016/j.artmed.2022.102345
![]() |
[47] |
D. Peng, J. Wang, D. Liu, Z. Liu, An improved EDAS method for the multi-attribute decision making based on the dynamic expectation level of decision makers, Symmetry, 14 (2022), 979. https://doi.org/10.3390/sym14050979 doi: 10.3390/sym14050979
![]() |
[48] |
G. Tang, F. Chiclana, P. Liu, A decision-theoretic rough set model with q-rung orthopair fuzzy information and its application in stock investment evaluation, Appl. Soft Comput., 91 (2020), 106212. https://doi.org/10.1016/j.asoc.2020.106212 doi: 10.1016/j.asoc.2020.106212
![]() |
[49] |
D. Liang, W. Cao, q-Rung orthopair fuzzy sets-based decision-theoretic rough sets for three-way decisions under group decision making, Int. J. Intell. Syst., 34 (2019), 3139–3167. https://doi.org/10.1002/int.22187 doi: 10.1002/int.22187
![]() |
[50] | Z. Zhang, S. M. Chen, Group decision making with incomplete q-rung orthopair fuzzy preference relations, Inform. Sci., 553 (2021), 376–396. |
[51] |
D. Peng, J. Wang, D. Liu, Z. Liu, The similarity measures for linguistic q-rung orthopair fuzzy multi-criteria group decision making using projection method, IEEE Access, 7 (2019), 176732–176745. https://doi.org/10.1109/ACCESS.2019.2957916 doi: 10.1109/ACCESS.2019.2957916
![]() |
[52] |
K. Charnpratheep, Q. Zhou, B. Garner, Preliminary landfill site screening using fuzzy geographical information systems, Waste Manag. Res., 15 (1997), 197–215. https://doi.org/10.1177/0734242X9701500207 doi: 10.1177/0734242X9701500207
![]() |
[53] |
O. E. Demesouka, A. P Vavatsikos, K. P. Anagnostopoulos, Suitability analysis for siting MSW landfills and its multicriteria spatial decision support system: Method, implementation and case study, Waste Manage., 33 (2013), 1190–1206. https://doi.org/10.1016/j.wasman.2013.01.030 doi: 10.1016/j.wasman.2013.01.030
![]() |
[54] |
M. Ekmekçioǧlu, T. Kaya, C. Kahraman, Fuzzy multicriteria disposal method and site selection for municipal solid waste, Waste Manage., 30 (2010), 1729–1736. https://doi.org/10.1016/j.wasman.2010.02.031 doi: 10.1016/j.wasman.2010.02.031
![]() |
[55] |
S. Ashraf, N. Rehman, A. Hussain, H. AlSalman, A. H. Gumaei, q-Rung orthopair fuzzy 380 rough Einstein aggregation information-based EDAS method: Applications in robotic agrifarming, Comput. Intell. Neurosci., 2021. https://doi.org/10.1155/2021/5520264 doi: 10.1155/2021/5520264
![]() |
[56] |
P. F. Hsu, M. G. Hsu, Optimizing the information outsourcing practices of primary care medical organizations using entropy and TOPSIS, Qual. Quant., 42 (2008), 181–201. https://doi.org/10.1007/s11135-006-9040-8 doi: 10.1007/s11135-006-9040-8
![]() |
[57] | C. L. Hwang, K. Yoon, Methods for multiple attribute decision making, In: Multiple attribute decision making, 1981, 58–191. Springer, Berlin, Heidelberg. https://doi.org/10.1007/978-3-642-48318-9_3 |
[58] |
D. Liu, D. Peng, Z. Liu, The distance measures between q-rung orthopair hesitant fuzzy sets and their application in multiple criteria decision making, Int. J. Intell. Syst., 34 (2019), 2104–2121. https://doi.org/10.1002/int.22133 doi: 10.1002/int.22133
![]() |
[59] | G. H. Tzeng, J. J. Huang, Multiple attribute decision making: Methods and applications, CRC Press, 2011. https://doi.org/10.1201/b11032 |
1. | Cengiz Kahraman, Proportional picture fuzzy sets and their AHP extension: Application to waste disposal site selection, 2024, 238, 09574174, 122354, 10.1016/j.eswa.2023.122354 | |
2. | Omar Barukab, Asghar Khan, Sher Afzal Khan, Fermatean fuzzy Linguistic term set based on linguistic scale function with Dombi aggregation operator and their application to multi criteria group decision –making problem, 2024, 10, 24058440, e36563, 10.1016/j.heliyon.2024.e36563 | |
3. | Yushuo Cao, Xuzhong Wu, Ling Ding, Weizhong Wang, A Hybrid Framework for Selecting Food Waste Treatment Techniques Using q-Rung Orthopair Fuzzy CRITIC-Generalized TODIM Method, 2024, 26, 1562-2479, 1916, 10.1007/s40815-024-01714-2 | |
4. | Ertugrul Ayyildiz, Melike Erdogan, Literature analysis of the location selection studies related to the waste facilities within MCDM approaches, 2024, 1614-7499, 10.1007/s11356-024-34370-y | |
5. | Mery Ellen Brandt de Oliveira, Francisco Rodrigues Lima Junior, José Marcelo Almeida Prado Cestari , Hesitant Fuzzy Vikor e suas extensões: uma revisão sistemática de literatura, 2024, 15, 2178-9010, e4287, 10.7769/gesec.v15i10.4287 | |
6. | Zihang Jia, Junsheng Qiao, Minghao Chen, Multi-level discrimination index for intuitionistic fuzzy coverings and its applications in feature selection, 2025, 263, 09574174, 125735, 10.1016/j.eswa.2024.125735 |
T | c1 | c2 | c3 | c4 |
∝1 | ({0.1,0.3,0.4},{0.2,0.5,0.7}) | ({0.2,0.3},{0.7,0.9}) | ({0.2,0.5,0.7},{0.2,0.3}) | ({0.3,0.5},{0.8}) |
∝2 | ({0.2,0.3,0.5},{0.2,0.7}) | ({0.2,0.3,,0.5},{0.3,0.4}) | ({0.1,0.4,0.6},{0.7,0.9}) | ({0.2,0.4},{0.7}) |
∝3 | ({0.5,0.6},{0.7,0.9}) | ({0.5,0.8,0.9},{0.1,0.9}) | ({0.2,0.3},{0.5,0.9}) | ({0.7,0.9},{0.1,0.2,0.3}) |
∝4 | ({0.2,0.5,0.9},{0.6,0.7,0.9}) | ({0.3,0.8,0.9},{0.4,0.8}) | ({0.2,0.5},{0.6,0.9}) | ({0.5,0.7},{0.1,0.8}) |
χ1 | χ2 | |
A1 | (((0.1,0.2,0.5),(0.3,0.4)),((0.8),(0.4,0.6))) | (((0.5,0.7),(0.5,0.6)),((0.4,0.5),(0.7,0.9))) |
A2 | (((0.6,0.7),(0.7,0.9)),((0.3,0.5),(0.6))) | (((0.2,0.4,0.5),(0.5)),((0.6,0.7),(0.3))) |
χ3 | χ4 | |
A1 | (((0.4),(0.3,0.7)),((0.5),(0.9))) | (((0.6),(0.7)),((0.6,0.8,0.9),(0.7,0.9))) |
A2 | (((0.8),(0.4,0.5,0.7)),((0.2,0.5),(0.4,0.5))) | \left( \begin{array}{c} \left( \begin{array}{c} \left( 0.8\right), \\ \left( 0.5\right) \end{array} \right), \\ \left( \begin{array}{c} \left( 0.7\right), \\ \left( 0.1, 0.3, 0.4\right) \end{array} \right) \end{array} \right) |
\chi _{1} | \chi_{2} | |
A_{3} | \left( \begin{array}{c} \left( \begin{array}{c} \left( 0.4, 0.5, 0.6\right), \\ \left( 0.6, 0.7\right) \end{array} \right), \\ \left( \begin{array}{c} \left( 0.9\right), \\ \left( 0.5\right) \end{array} \right) \end{array} \right) | \left( \begin{array}{c} \left( \begin{array}{c} \left( 0.1\right), \\ \left( 0.5, 0.6\right) \end{array} \right), \\ \left( \begin{array}{c} \left( 0.4, 0.6, 0.7\right), \\ \left( 0.5, 0.7\right) \end{array} \right) \end{array} \right) |
A_{4} | \left( \begin{array}{c} \left( \begin{array}{c} \left( 0.4\right), \\ \left( 0.5, 0.6\right) \end{array} \right), \\ \left( \begin{array}{c} \left( 0.3, 0.4\right), \\ \left( 0.8\right) \end{array} \right) \end{array} \right) | \left( \begin{array}{c} \left( \begin{array}{c} \left( 0.4, 0.5\right), \\ \left( 0.4\right) \end{array} \right), \\ \left( \begin{array}{c} \left( 0.1, 0.2\right), \\ \left( 0.2, 0.3\right) \end{array} \right) \end{array} \right) |
\chi _{3} | \chi _{4} | |
A_{3} | \left( \begin{array}{c} \left( \begin{array}{c} \left( 0.3\right), \\ \left( 0.7, 0.8\right) \end{array} \right), \\ \left( \begin{array}{c} \left( 0.7, 0.8\right), \\ \left( 0.1, 0.4, 0.7\right) \end{array} \right) \end{array} \right) | \left( \begin{array}{c} \left( \begin{array}{c} \left( 0.3, 0.6\right), \\ \left( 0.8\right) \end{array} \right), \\ \left( \begin{array}{c} \left( 0.7\right), \\ \left( 0.3\right) \end{array} \right) \end{array} \right) |
A_{4} | \left( \begin{array}{c} \left( \begin{array}{c} \left( 0.3\right), \\ \left( 0.7, 0.8\right) \end{array} \right), \\ \left( \begin{array}{c} \left( 0.7\right), \\ \left( 0.6\right) \end{array} \right) \end{array} \right) | \left( \begin{array}{c} \left( \begin{array}{c} \left( 0.6, 0.7, 0.9\right), \\ \left( 0.3, 0.4\right) \end{array} \right), \\ \left( \begin{array}{c} \left( 0.2, 0.7\right), \\ \left( 0.7, 0.8, 0.9\right) \end{array} \right) \end{array} \right) |
A_{1} | \left( \begin{array}{c} \left( \begin{array}{c} \left\{ 0.4934, 0.5659, 0.9634, 0.56680.5143, 0.5817\right\}, \\ \left\{ 0.0047, 0.0113, 0.0057, 0.0136, 0.0063, 0.0150, 0.0076, 0.0181\right\} \end{array} \right) {\bf{, }} \\ \left( \begin{array}{c} \left\{ 0.5932, 0.6759, 0.9871, 0.5777, 0.6647, 0.7320\right\}, \\ \left\{ 0.0281, 0.0371, 0.0370, 0.0488, 0.0423, 0.0558, 0.0556, 0.0735\right\} \end{array} \right) \end{array} \right) |
A_{2} | \left( \begin{array}{c} \left( \begin{array}{c} \left\{ 0.7074, 0.7160, 0.8976, 0.7074, 0.7160, 0.7252\right\}, \\ \left\{ 0.0105, 0.0132, 0.0187, 0.0137, 0.0171, 0.0243\right\} \end{array} \right) {\bf{, }} \\ \left( \begin{array}{c} \left\{ 0.5584, 0.5915, 0.9867, 0.6235, 0.5714, 0.6030, 0.6057, 0.6336\right\}, \\ \left\{ 0.0011, 0.0032, 0.0043, 0.0013, 0.0040, 0.0054\right\} \end{array} \right) \end{array} \right) |
A_{3} | \left( \begin{array}{c} \left( \begin{array}{c} \left\{ 0.2916, 0.4384, 0.9834, 0.4518, 0.3556, 0.4707\right\}, \\ \left\{ 0.0262, 0.0302, 0.0315, 0.0364, 0.0306, 0.0353, 0.0369, 0.0426\right\} \end{array} \right) {\bf{, }} \\ \left( \begin{array}{c} \left\{ 0.6968, 0.7310, 0.9674, 0.7522, 0.7408, 0.7695\right\}, \\ \left\{ 0.0011, 0.0063, 0.0079, 0.0016, 0.0063, 0.0112\right\} \end{array} \right) \end{array} \right) |
A_{4} | \left( \begin{array}{c} \left( \begin{array}{c} \left\{ 0.4659, 0.5233, 0.9234, 0.4899, 0.5424, 0.6876\right\}, \\ \left\{ 0.0063, 0.0084, 0.0073, 0.0097, 0.0076, 0.0102, 0.0088, 0.0117\right\} \end{array} \right) {\bf{, }} \\ \left( \begin{array}{c} \left\{ 0.4784, 0.5990, 0.9543, 0.6006, 0.4852, 0.6032, 0.4879, 0.6048\right\}, \\ \left\{ 0.0103, 0.0119, 0.0136, 0.0155, 0.0179, 0.0204\right\} \end{array} \right) \end{array} \right) |
\chi _{1} | \chi _{2} | \chi _{3} | \chi _{4} | |
A_{1} | 0.5542 | 0.4250 | 0.3750 | 0.4667 |
A_{2} | 0.4000 | 0.5542 | 0.5417 | 0.6833 |
A_{3} | 0.5625 | 0.3792 | 0.4750 | 0.5125 |
A_{4} | 0.3500 | 0.4875 | 0.4125 | 0.5083 |
\chi _{1} | \chi _{2} | |
A_{1} | \left( \begin{array}{c} \left( \begin{array}{c} \left( 0.1, 0.2, 0.5\right), \\ \left( 0.3, 0.4\right) \end{array} \right), \\ \left( \begin{array}{c} \left( 0.8\right), \\ \left( 0.4, 0.6\right) \end{array} \right) \end{array} \right) | \left( \begin{array}{c} \left( \begin{array}{c} \left( 0.6\right) {\bf{, }} \\ \left( 0.7\right) \end{array} \right), \\ \left( \begin{array}{c} \left( 0.6, 0.8, 0.9\right), \\ \left( 0.7, 0.9\right) \end{array} \right) \end{array} \right) |
A_{2} | \left( \begin{array}{c} \left( \begin{array}{c} \left( 0.8\right), \\ \left( 0.5\right) \end{array} \right), \\ \left( \begin{array}{c} \left( 0.7\right), \\ \left( 0.1, 0.3, 0.4\right) \end{array} \right) \end{array} \right) | \left( \begin{array}{c} \left( \begin{array}{c} \left( 0.2, 0.4, 0.5\right), \\ \left( 0.5\right) \end{array} \right), \\ \left( \begin{array}{c} \left( 0.6, 0.7\right), \\ \left( 0.3\right) \end{array} \right) \end{array} \right) |
\chi _{3} | \chi _{4} | |
A_{1} | \left( \begin{array}{c} \left( \begin{array}{c} \left( 0.5, 0.7\right), \\ \left( 0.5, 0.6\right) \end{array} \right), \\ \left( \begin{array}{c} \left( 0.4, 0.5\right), \\ \left( 0.7, 0.9\right) \end{array} \right) \end{array} \right) | \left( \begin{array}{c} \left( \begin{array}{c} \left( 0.4\right), \\ \left( 0.3, 0.7\right) \end{array} \right) {\bf{, }} \\ \left( \begin{array}{c} \left( 0.5\right), \\ \left( 0.9\right) \end{array} \right) \end{array} \right) |
A_{2} | \left( \begin{array}{c} \left( \begin{array}{c} \left( 0.8\right), \\ \left( 0.4, 0.5, 0.7\right) \end{array} \right), \\ \left( \begin{array}{c} \left( 0.2, 0.5\right), \\ \left( 0.4, 0.5\right) \end{array} \right) \end{array} \right) | \left( \begin{array}{c} \left( \begin{array}{c} \left( 0.6, 0.7\right), \\ \left( 0.7, 0.9\right) \end{array} \right), \\ \left( \begin{array}{c} \left( 0.3, 0.5\right), \\ \left( 0.6\right) \end{array} \right) \end{array} \right) |
\chi _{1} | \chi _{2} | |
A_{3} | \left( \begin{array}{c} \left( \begin{array}{c} \left( 0.4, 0.5, 0.6\right), \\ \left( 0.6, 0.7\right) \end{array} \right), \\ \left( \begin{array}{c} \left( 0.9\right), \\ \left( 0.5\right) \end{array} \right) \end{array} \right) | \left( \begin{array}{c} \left( \begin{array}{c} \left( 0.3, 0.6\right), \\ \left( 0.8\right) \end{array} \right), \\ \left( \begin{array}{c} \left( 0.7\right), \\ \left( 0.3\right) \end{array} \right) \end{array} \right) |
A_{4} | \left( \begin{array}{c} \left( \begin{array}{c} \left( 0.6, 0.7, 0.9\right), \\ \left( 0.3, 0.4\right) \end{array} \right), \\ \left( \begin{array}{c} \left( 0.2, 0.7\right), \\ \left( 0.7, 0.8, 0.9\right) \end{array} \right) \end{array} \right) | \left( \begin{array}{c} \left( \begin{array}{c} \left( 0.4, 0.5\right), \\ \left( 0.4\right) \end{array} \right), \\ \left( \begin{array}{c} \left( 0.1, 0.2\right), \\ \left( 0.2, 0.3\right) \end{array} \right) \end{array} \right) |
\chi _{3} | \chi _{4} | |
A_{3} | \left( \begin{array}{c} \left( \begin{array}{c} \left( 0.3\right), \\ \left( 0.7, 0.8\right) \end{array} \right), \\ \left( \begin{array}{c} \left( 0.7, 0.8\right), \\ \left( 0.1, 0.4, 0.7\right) \end{array} \right) \end{array} \right) | \left( \begin{array}{c} \left( \begin{array}{c} \left( 0.1\right), \\ \left( 0.5, 0.6\right) \end{array} \right), \\ \left( \begin{array}{c} \left( 0.4, 0.6, 0.7\right), \\ \left( 0.5, 0.7\right) \end{array} \right) \end{array} \right) |
A_{4} | \left( \begin{array}{c} \left( \begin{array}{c} \left( 0.3\right), \\ \left( 0.7, 0.8\right) \end{array} \right), \\ \left( \begin{array}{c} \left( 0.7\right), \\ \left( 0.6\right) \end{array} \right) \end{array} \right) | \left( \begin{array}{c} \left( \begin{array}{c} \left( 0.4\right), \\ \left( 0.5, 0.6\right) \end{array} \right), \\ \left( \begin{array}{c} \left( 0.3, 0.4\right), \\ \left( 0.8\right) \end{array} \right) \end{array} \right) |
A_{1} | \left( \begin{array}{c} \left( \begin{array}{c} \left\{ 0.4865, 0.5656, 0.4878, 0.5665, 0.5081, 0.5814\right\}, \\ \left\{ 0.4419, 0.5744, 0.4669, 0.6049, 0.4583, 0.5945, 0.4841, 0.6257\right\} \end{array} \right) {\bf{, }} \\ \left( \begin{array}{c} \left\{ 0.5898, 0.5729, 0.6640, 0.6513, 0.7238, 0.7139\right\}, \\ \left\{ 0.7150, 0.7732, 0.7692, 0.8277, 0.7481, 0.8067, 0.8026, 0.8609\right\} \end{array} \right) \end{array} \right) |
A_{2} | \left( \begin{array}{c} \left( \begin{array}{c} \left\{ 0.6682, 0.6957, 0.6783, 0.7048, 0.6889, 0.7144\right\}, \\ \left\{ 0.5235, 0.5766, 0.5573, 0.6127, 0.6149, 0.6737\right\} \end{array} \right) {\bf{, }} \\ \left( \begin{array}{c} \left\{ 0.4876, 0.5265, 0.5306, 0.5637, 0.5343, 0.5669, 0.5704, 0.5991\right\}, \\ \left\{ 0.3535, 0.3777, 0.4065, 0.4339, 0.4217, 0.4500\right\} \end{array} \right) \end{array} \right) |
A_{3} | \left( \begin{array}{c} \left( \begin{array}{c} \left\{ 0.2875, 0.4372, 0.3167, 0.4507, 0.3529, 0.4697\right\}, \\ \left\{ 0.6461, 0.6810, 0.6738, 0.7094, 0.6591, 0.6944, 0.6872, 0.7230\right\} \end{array} \right) {\bf{, }} \\ \left( \begin{array}{c} \left\{ 0.6896, 0.7180, 0.7408, 0.7247, 0.7495, 0.7695\right\}, \\ \left\{ 0.2751, 0.3088, 0.4092, 0.4578, 0.4847, 0.5407\right\} \end{array} \right) \end{array} \right) |
A_{4} | \left( \begin{array}{c} \left( \begin{array}{c} \left\{ 0.4187, 0.4480, 0.4502, 0.4758, 0.5473, 0.5647\right\}, \\ \left\{ 0.4897, 0.5188, 0.5127, 0.5429, 0.5075, 0.5375, 0.5313, 0.5622\right\} \end{array} \right) {\bf{, }} \\ \left( \begin{array}{c} \left\{ 0.4833, 0.4989, 0.4859, 0.5014, 0.5400, 0.5525, 0.5421, 0.5545\right\}, \\ \left\{ 0.5111, 0.5659, 0.5217, 0.5773, 0.5325, 0.5889\right\} \end{array} \right) \end{array} \right) |
\chi _{1} | \chi _{2} | |
A_{1} | \left( \begin{array}{c} \left( \begin{array}{c} \left( 0.0479, 0.0958, 0.2400\right), \\ \left( 0.9159, 0.9339\right) \end{array} \right), \\ \left( \begin{array}{c} \left( 0.3960\right), \\ \left( 0.9339, 0.9599\right) \end{array} \right) \end{array} \right) | \left( \begin{array}{c} \left( \begin{array}{c} \left( 0.3276, 0.4638\right) {\bf{, }} \\ \left( 0.8609, 0.8933\right) \end{array} \right), \\ \left( \begin{array}{c} \left( 0.3276, 0.2618\right), \\ \left( 0.8989, 0.9734\right) \end{array} \right) \end{array} \right) |
A_{2} | \left( \begin{array}{c} \left( \begin{array}{c} \left( 0.2890, 0.3400\right), \\ \left( 0.9703, 0.9897\right) \end{array} \right), \\ \left( \begin{array}{c} \left( 0.1438, 0.2400\right), \\ \left( 0.9599\right) \end{array} \right) \end{array} \right) | \left( \begin{array}{c} \left( \begin{array}{c} \left( 0.1308, 0.2618, 0.3276\right), \\ \left( 0.8609\right) \end{array} \right), \\ \left( \begin{array}{c} \left( 0.3944, 0.4638\right), \\ \left( 0.7285\right) \end{array} \right) \end{array} \right) |
A_{3} | \left( \begin{array}{c} \left( \begin{array}{c} \left( 0.1917, 0.2400, 0.2890\right), \\ \left( 0.9599, 0.9703\right) \end{array} \right), \\ \left( \begin{array}{c} \left( 0.4666\right), \\ \left( 0.9481\right) \end{array} \right) \end{array} \right) | \left( \begin{array}{c} \left( \begin{array}{c} \left( 0.0654\right), \\ \left( 0.8609, 0.8933\right) \end{array} \right), \\ \left( \begin{array}{c} \left( 0.2618, 0.3944, 0.4638\right), \\ \left( 0.8609, 0.9217\right) \end{array} \right) \end{array} \right) |
A_{4} | \left( \begin{array}{c} \left( \begin{array}{c} \left( 0.1917\right), \\ \left( 0.9481, 0.9599\right) \end{array} \right), \\ \left( \begin{array}{c} \left( 0.1438, 0.1917\right), \\ \left( 0.9801\right) \end{array} \right) \end{array} \right) | \left( \begin{array}{c} \left( \begin{array}{c} \left( 0.2618, 0.3276\right), \\ \left( 0.8222\right) \end{array} \right), \\ \left( \begin{array}{c} \left( 0.0654, 0.1308\right), \\ \left( 0.7058, 0.7733\right) \end{array} \right) \end{array} \right) |
\chi _{3} | \chi _{4} | |
A_{1} | \left( \begin{array}{c} \left( \begin{array}{c} \left( 0.2679\right), \\ \left( 0.7563, 0.9158\right) \end{array} \right), \\ \left( \begin{array}{c} \left( 0.3352\right), \\ \left( 0.9715\right) \end{array} \right) \end{array} \right) | \left( \begin{array}{c} \left( \begin{array}{c} \left( 0.4080\right), \\ \left( 0.9128\right) \end{array} \right) {\bf{, }} \\ \left( \begin{array}{c} \left( 0.4080, 0.5578, 0.6539\right), \\ \left( 0.9128, 0.9705\right) \end{array} \right) \end{array} \right) |
A_{2} | \left( \begin{array}{c} \left( \begin{array}{c} \left( 0.5518\right), \\ \left( 0.8087, 0.8503, 0.9158\right) \end{array} \right), \\ \left( \begin{array}{c} \left( 0.1339, 0.3352\right), \\ \left( 0.8087, 0.8503\right) \end{array} \right) \end{array} \right) | \left( \begin{array}{c} \left( \begin{array}{c} \left( 0.5578\right), \\ \left( 0.8450\right) \end{array} \right), \\ \left( \begin{array}{c} \left( 0.4797\right), \\ \left( 0.5574, 0.7478, 0.8020\right) \end{array} \right) \end{array} \right) |
A_{3} | \left( \begin{array}{c} \left( \begin{array}{c} \left( 0.2008\right), \\ \left( 0.9158, 0.9440\right) \end{array} \right), \\ \left( \begin{array}{c} \left( 0.4745, 0.5518\right), \\ \left( 0.5704, 0.8087, 0.9158\right) \end{array} \right) \end{array} \right) | \left( \begin{array}{c} \left( \begin{array}{c} \left( 0.2031, 0.4080\right), \\ \left( 0.9421\right) \end{array} \right), \\ \left( \begin{array}{c} \left( 0.4797\right), \\ \left( 0.7478\right) \end{array} \right) \end{array} \right) |
A_{4} | \left( \begin{array}{c} \left( \begin{array}{c} \left( 0.2008\right), \\ \left( 0.9158, 0.9440\right) \end{array} \right), \\ \left( \begin{array}{c} \left( 0.4745\right), \\ \left( 0.8851\right) \end{array} \right) \end{array} \right) | \left( \begin{array}{c} \left( \begin{array}{c} \left( 0.4080, 0.4797, 0.6539\right), \\ \left( 0.7478, 0.8020\right) \end{array} \right), \\ \left( \begin{array}{c} \left( 0.1354, 0.4797\right), \\ \left( 0.9128, 0.9421, 0.9705\right) \end{array} \right) \end{array} \right) |
\chi _{1} | \chi _{2} | \chi _{3} | \chi _{4} | |
A_{1} | 0.1630 | 0.2193 | 0.1989 | 0.2734 |
A_{2} | 0.1416 | 0.2700 | 0.2746 | 0.3725 |
A_{3} | 0.1984 | 0.1676 | 0.2548 | 0.2738 |
A_{4} | 0.1064 | 0.2078 | 0.2151 | 0.2762 |
\chi _{1} | \chi _{2} | |
A_{1} | \left( \begin{array}{c} \left( \begin{array}{c} \left( 0.4080\right), \\ \left( 0.9128\right) \end{array} \right) {\bf{, }} \\ \left( \begin{array}{c} \left( 0.4080, 0.5578, 0.6539\right), \\ \left( 0.9128, 0.9705\right) \end{array} \right) \end{array} \right) | \left( \begin{array}{c} \left( \begin{array}{c} \left( 0.3276, 0.4638\right) {\bf{, }} \\ \left( 0.8609, 0.8933\right) \end{array} \right), \\ \left( \begin{array}{c} \left( 0.3276, 0.2618\right), \\ \left( 0.8989, 0.9734\right) \end{array} \right) \end{array} \right) |
A_{2} | \left( \begin{array}{c} \left( \begin{array}{c} \left( 0.5578\right), \\ \left( 0.8450\right) \end{array} \right), \\ \left( \begin{array}{c} \left( 0.4797\right), \\ \left( 0.55740.74780.8020\right) \end{array} \right) \end{array} \right) | \left( \begin{array}{c} \left( \begin{array}{c} \left( 0.5518\right), \\ \left( 0.80870.8503, 0.9158\right) \end{array} \right), \\ \left( \begin{array}{c} \left( 0.13390.3352\right), \\ \left( 0.80870.8503\right) \end{array} \right) \end{array} \right) |
A_{3} | \left( \begin{array}{c} \left( \begin{array}{c} \left( 0.2031, 0.4080\right), \\ \left( 0.9421\right) \end{array} \right), \\ \left( \begin{array}{c} \left( 0.4797\right), \\ \left( 0.7478\right) \end{array} \right) \end{array} \right) | \left( \begin{array}{c} \left( \begin{array}{c} \left( 0.2008\right), \\ \left( 0.9158, 0.9440\right) \end{array} \right), \\ \left( \begin{array}{c} \left( 0.4745, 0.5518\right), \\ \left( 0.5704, 0.8087, 0.9158\right) \end{array} \right) \end{array} \right) |
A_{4} | \left( \begin{array}{c} \left( \begin{array}{c} \left( 0.4080, 0.4797, 0.6539\right), \\ \left( 0.7478, 0.8020\right) \end{array} \right), \\ \left( \begin{array}{c} \left( 0.1354, 0.4797\right), \\ \left( 0.9128, 0.9421, 0.9705\right) \end{array} \right) \end{array} \right) | \left( \begin{array}{c} \left( \begin{array}{c} \left( 0.2008\right), \\ \left( 0.9158, 0.9440\right) \end{array} \right), \\ \left( \begin{array}{c} \left( 0.4745\right), \\ \left( 0.8851\right) \end{array} \right) \end{array} \right) |
\chi _{3} | \chi _{4} | |
A_{1} | \left( \begin{array}{c} \left( \begin{array}{c} \left( 0.2679\right), \\ \left( 0.7563, 0.9158\right) \end{array} \right), \\ \left( \begin{array}{c} \left( 0.3352\right), \\ \left( 0.9715\right) \end{array} \right) \end{array} \right) | \left( \begin{array}{c} \left( \begin{array}{c} \left( 0.0479, 0.0958, 0.2400\right), \\ \left( 0.9159, 0.9339\right) \end{array} \right), \\ \left( \begin{array}{c} \left( 0.3960\right), \\ \left( 0.9339, 0.9599\right) \end{array} \right) \end{array} \right) |
A_{2} | \left( \begin{array}{c} \left( \begin{array}{c} \left( 0.1308, 0.2618, 0.3276\right), \\ \left( 0.8609\right) \end{array} \right), \\ \left( \begin{array}{c} \left( 0.3944, 0.4638\right), \\ \left( 0.7285\right) \end{array} \right) \end{array} \right) | \left( \begin{array}{c} \left( \begin{array}{c} \left( 0.2890, 0.3400\right), \\ \left( 0.9703, 0.9897\right) \end{array} \right), \\ \left( \begin{array}{c} \left( 0.1438, 0.2400\right), \\ \left( 0.9599\right) \end{array} \right) \end{array} \right) |
A_{3} | \left( \begin{array}{c} \left( \begin{array}{c} \left( 0.1917, 0.2400, 0.2890\right), \\ \left( 0.9599, 0.9703\right) \end{array} \right), \\ \left( \begin{array}{c} \left( 0.4666\right), \\ \left( 0.9481\right) \end{array} \right) \end{array} \right) | \left( \begin{array}{c} \left( \begin{array}{c} \left( 0.0654\right), \\ \left( 0.8609, 0.8933\right) \end{array} \right), \\ \left( \begin{array}{c} \left( 0.2618, 0.3944, 0.4638\right), \\ \left( 0.8609, 0.9217\right) \end{array} \right) \end{array} \right) |
A_{4} | \left( \begin{array}{c} \left( \begin{array}{c} \left( 0.2618, 0.3276\right), \\ \left( 0.8222\right) \end{array} \right), \\ \left( \begin{array}{c} \left( 0.0654, 0.1308\right), \\ \left( 0.7058, 0.7733\right) \end{array} \right) \end{array} \right) | \left( \begin{array}{c} \left( \begin{array}{c} \left( 0.1917\right), \\ \left( 0.9481, 0.9599\right) \end{array} \right), \\ \left( \begin{array}{c} \left( 0.1438, 0.1917\right), \\ \left( 0.9801\right) \end{array} \right) \end{array} \right) |
A_{1} | \left( \begin{array}{c} \left( \begin{array}{c} \left\{ 0.3647, 0.3527, 0.3967, 0.3866, 0.42520.4165\right\}, \\ \left\{ 0.9331, 0.9412, 0.9531, 0.9610, 0.9406, 0.9487, 0.9604, 0.9681\right\} \end{array} \right) {\bf{, }} \\ \left( \begin{array}{c} \left\{ 0.3647, 0.3527, 0.3967, 0.3866, 0.4252, 0.4165\right\}, \\ \left\{ 0.9331, 0.9412, 0.9531, 0.9610, 0.9406, 0.9487, 0.9604, 0.9683\right\} \end{array} \right) \end{array} \right) |
A_{2} | \left( \begin{array}{c} \left( \begin{array}{c} \left\{ 0.4246, 0.4331, 0.4329, 0.4410, 0.4415, 0.4494\right\}, \\ \left\{ 0.8802, 0.8869, 0.8912, 0.8978, 0.9087, 0.9152\right\} \end{array} \right) {\bf{, }} \\ \left( \begin{array}{c} \left\{ 0.3233, 0.3336, 0.3556, 0.3643, 0.3511, 0.3600, 0.3791, 0.3867\right\}, \\ \left\{ 0.7996, 0.8109, 0.8260, 0.8373, 0.8331, 0.8443\right\} \end{array} \right) \end{array} \right) |
A_{3} | \left( \begin{array}{c} \left( \begin{array}{c} \left\{ 0.1755, 0.1946, 0.2180, 0.2361, 0.2473, 0.2626\right\}, \\ \left\{ 0.9157, 0.9254, 0.9189, 0.9286, 0.9234, 0.9330, 0.9266, 0.9362\right\} \end{array} \right) {\bf{, }} \\ \left( \begin{array}{c} \left\{ 0.4279, 0.4510, 0.4696, 0.4564, 0.4768, 0.4935\right\}, \\ \left\{ 0.7915, 0.8110, 0.8585, 0.8776, 0.8874, 0.9061\right\} \end{array} \right) \end{array} \right) |
A_{4} | \left( \begin{array}{c} \left( \begin{array}{c} \left\{ 0.2641, 0.2861, 0.2884, 0.6061, 0.3606, 0.3729\right\}, \\ \left\{ 0.8781, 0.8821, 0.8861, 0.8900, 0.8850, 0.8889, 0.8929, 0.8968\right\} \end{array} \right) {\bf{, }} \\ \left( \begin{array}{c} \left\{ 0.3117, 0.3159, 0.3136, 0.3178, 0.3539, 0.3573, 0.3554, 0.3587\right\}, \\ \left\{ 0.8686, 0.8879, 0.8726, 0.8918, 0.8765, 0.8957\right\} \end{array} \right) \end{array} \right) |
Operators | SR\left( A_{1}\right) | SR\left( A_{2}\right) | SR\left( A_{3}\right) | SR\left( A_{4}\right) |
q-ROHFREWA | 0.4690 | 0.6064 | 0.5051 | 0.5181 |
q-ROHFREOWA | 0.4665 | 0.5597 | 0.5052 | 0.4823 |
q-ROHFRHWA | 0.2198 | 0.2680 | 0.2259 | 0.2322 |
Operator | Scores | Best Alternative |
q-ROHFREWA | SR\left( A_{2}\right) >SR\left( A_{4}\right) >SR\left( A_{3}\right) >SR\left( A_{1}\right) | A_{2} |
q-ROHFREOWA | SR\left( A_{2}\right) >SR\left( A_{3}\right) >SR\left( A_{4}\right) >SR\left( A_{1}\right) | A_{2} |
q-ROHFRHWA | SR\left( A_{2}\right) >SR\left( A_{4}\right) >SR\left( A_{3}\right) >SR\left( A_{1}\right) | A_{2} |
q | Score values of alternatives | Ranking | Best Alternative | |||
A_{1} | A_{2} | A_{3} | A_{4} | |||
1 | 0.5992 | 0.7522 | 0.6395 | 0.6623 | A_{2}\succ A_{4}\succ \ A_{3}\succ A_{1} | A_{2} |
2 | 0.5122 | 0.6657 | 0.5545 | 0.5698 | A_{2}\succ A_{4}\succ \ A_{3}\succ A_{1} | A_{2} |
3 | 0.4690 | 0.6064 | 0.5051 | 0.5181 | A_{2}\succ A_{4}\succ \ A_{3}\succ A_{1} | A_{2} |
4 | 0.4441 | 0.5657 | 0.4734 | 0.4868 | A_{2}\succ A_{4}\succ \ A_{3}\succ A_{1} | A_{2} |
5 | 0.4282 | 0.5366 | 0.4518 | 0.4660 | A_{2}\succ A_{4}\succ \ A_{3}\succ A_{1} | A_{2} |
10 | 0.3969 | 0.4658 | 0.4033 | 0.4185 | A_{2}\succ A_{4}\succ \ A_{3}\succ A_{1} | A_{2} |
15 | 0.3876 | 0.4382 | 0.3874 | 0.4003 | A_{2}\succ A_{4}\succ \ A_{1}\succ A_{3} | A_{2} |
20 | 0.3833 | 0.4235 | 0.3804 | 0.3905 | A_{2}\succ A_{4}\succ \ A_{1}\succ A_{3} | A_{2} |
50 | 0.3759 | 0.3953 | 0.3537 | 0.3717 | A_{2}\succ A_{4}\succ \ A_{1}\succ A_{3} | A_{2} |
100 | 0.2989 | 0.3844 | 0.2306 | 0.3144 | A_{2}\succ A_{4}\succ \ A_{1}\succ A_{3} | A_{2} |
\chi _{1} | \chi _{2} | \chi _{3} | \chi _{4} | \chi _{5} | |
A_{1} | \left( \begin{array}{c} \left( 0.64, 0.13\right), \\ \left( 0.46, 0.15\right) \end{array} \right) | \left( \begin{array}{c} \left( 0.8, 0.16\right), \\ \left( 0.63, 0.26\right) \end{array} \right) | \left( \begin{array}{c} \left( 0.75, 0.22\right), \\ \left( 0.58, 0.18\right) \end{array} \right) | \left( \begin{array}{c} \left( 0.67, 0.13\right), \\ \left( 0.60, 0.17\right) \end{array} \right) | \left( \begin{array}{c} \left( 0.78, 0.1\right), \\ \left( 0.67, 0.15\right) \end{array} \right) |
A_{2} | \left( \begin{array}{c} \left( 0.55, 0.15\right), \\ \left( 0.62, 0.24\right) \end{array} \right) | \left( \begin{array}{c} \left( 0.71, 0.18\right), \\ \left( 0.4, 0.26\right) \end{array} \right) | \left( \begin{array}{c} \left( 0.43, 0.17\right), \\ \left( 0.51, 0.20\right) \end{array} \right) | \left( \begin{array}{c} \left( 0.61, 0.26\right), \\ \left( 0.40, 0.20\right) \end{array} \right) | \left( \begin{array}{c} \left( 0.63, 0.19\right), \\ \left( 0.46, 0.26\right) \end{array} \right) |
A_{3} | \left( \begin{array}{c} \left( 0.57, 0.24\right), \\ \left( 0.40, 0.27\right) \end{array} \right) | \left( \begin{array}{c} \left( 0.71, 0.17\right), \\ \left( 0.65, 0.23\right) \end{array} \right) | \left( \begin{array}{c} \left( 0.49, 0.22\right), \\ \left( 0.36, 0.39\right) \end{array} \right) | \left( \begin{array}{c} \left( 0.54, 0.40\right), \\ \left( 0.45, 0.36\right) \end{array} \right) | \left( \begin{array}{c} \left( 0.56, 0.36\right), \\ \left( 0.32, 0.18\right) \end{array} \right) |
A_{4} | \left( \begin{array}{c} \left( 0.56, 0.17\right), \\ \left( 0.59, 0.23\right) \end{array} \right) | \left( \begin{array}{c} \left( 0.50, 0.32\right), \\ \left( 0.43, 0.42\right) \end{array} \right) | \left( \begin{array}{c} \left( 0.27, 0.13\right), \\ \left( 0.36, 0.29\right) \end{array} \right) | \left( \begin{array}{c} \left( 0.46, 0.19\right), \\ \left( 0.37, 0.36\right) \end{array} \right) | \left( \begin{array}{c} \left( 0.49, 0.25\right), \\ \left( 0.37, 0.26\right) \end{array} \right) |
A_{1} | \left( \left( 0.7338, 0.1396\right), \left( 0.6058, 0.1743\right) \right) |
A_{2} | \left( \left( 0.5992, 0.1959\right), \left( 0.4774, 0.2263\right) \right) |
A_{3} | \left( \left( 0.5717, 0.2878\right), \left( 0.4502, 0.2807\right) \right) |
A_{4} | \left( \left( 0.4656, 0.1988\right), \left( 0.4204, 0.3077\right) \right) |
Score | |||||
Ashraf et al. | SR\left( A_{1}\right) | SR\left( A_{2}\right) | SR\left( A_{3}\right) | SR\left( A_{4}\right) | Ranking |
q-ROFREWA | 0.999 | 0.489 | 0.201 | 0.011 | A_{1}>A_{2}>A_{3}>A_{4} |
Score | |||||
Developed method | SR\left( A_{1}\right) | SR\left( A_{2}\right) | SR\left( A_{3}\right) | SR\left( A_{4}\right) | Ranking |
q-ROHFREWA | 0.7564 | 0.6636 | 0.6134 | 0.5949 | A_{1}>A_{2}>A_{3}>A_{4} |
q-ROHFREOWA | 0.7612 | 0.6741 | 0.6166 | 0.5991 | A_{1}>A_{2}>A_{3}>A_{4} |
Criteria | \daleth ^{+} | \daleth ^{-} |
\chi _{1} | \left( \begin{array}{c} \left( \begin{array}{c} \left( 0.4, 0.5, 0.6\right), \\ \left( 0.6, 0.7\right) \end{array} \right), \\ \left( \begin{array}{c} \left( 0.9\right), \\ \left( 0.5\right) \end{array} \right) \end{array} \right) | \left( \begin{array}{c} \left( \begin{array}{c} \left( 0.4\right), \\ \left( 0.5, 0.6\right) \end{array} \right), \\ \left( \begin{array}{c} \left( 0.3, 0.4\right), \\ \left( 0.8\right) \end{array} \right) \end{array} \right) |
\chi _{2} | \left( \begin{array}{c} \left( \begin{array}{c} \left( 0.2, 0.4, 0.5\right), \\ \left( 0.5\right) \end{array} \right), \\ \left( \begin{array}{c} \left( 0.6, 0.7\right), \\ \left( 0.3\right) \end{array} \right) \end{array} \right) | \left( \begin{array}{c} \left( \begin{array}{c} \left( 0.1\right), \\ \left( 0.5, 0.6\right) \end{array} \right), \\ \left( \begin{array}{c} \left( 0.4, 0.6, 0.7\right), \\ \left( 0.5, 0.7\right) \end{array} \right) \end{array} \right) |
\chi _{3} | \left( \begin{array}{c} \left( \begin{array}{c} \left( 0.8\right), \\ \left( 0.4, 0.5, 0.7\right) \end{array} \right), \\ \left( \begin{array}{c} \left( 0.2, 0.5\right), \\ \left( 0.4, 0.5\right) \end{array} \right) \end{array} \right) | \left( \begin{array}{c} \left( \begin{array}{c} \left( 0.4\right), \\ \left( 0.3, 0.7\right) \end{array} \right) {\bf{, }} \\ \left( \begin{array}{c} \left( 0.5\right), \\ \left( 0.9\right) \end{array} \right) \end{array} \right) |
\chi _{4} | \left( \begin{array}{c} \left( \begin{array}{c} \left( 0.8\right), \\ \left( 0.5\right) \end{array} \right), \\ \left( \begin{array}{c} \left( 0.7\right), \\ \left( 0.1, 0.3, 0.4\right) \end{array} \right) \end{array} \right) | \left( \begin{array}{c} \left( \begin{array}{c} \left( 0.6\right) {\bf{, }} \\ \left( 0.7\right) \end{array} \right), \\ \left( \begin{array}{c} \left( 0.6, 0.8, 0.9\right), \\ \left( 0.7, 0.9\right) \end{array} \right) \end{array} \right) |
alternatives | S_{\imath} | R_{\imath} | Q_{\imath} |
A _{1} | 0.1460 | 0.2472 | 0.2137 |
A _{2} | 0.5324 | 0.4042 | 0.5631 |
A _{3} | 0.3637 | 0.3781 | 0.4319 |
A _{4} | 0.3426 | 0.2682 | 0.3142 |
Alternatives | Ranking order of S_{\imath} | Ranking order of R_{\imath} | Ranking order of Q_{\imath} |
A _{1} | 4 | 4 | 4 |
A _{2} | 1 | 1 | 1 |
A _{3} | 2 | 2 | 2 |
A _{4} | 3 | 3 | 3 |
\mathcal{T} | c_{1} | c_{2} | c_{3} | c_{4} |
\propto _{1} | \left( \begin{array}{c} \left\{ 0.1, 0.3, 0.4\right\}, \\\left\{ 0.2, 0.5, 0.7\right\} \end{array} \right) | \left( \begin{array}{c} \left\{ 0.2, 0.3\right\}, \\\left\{ 0.7, 0.9\right\} \end{array} \right) | \left( \begin{array}{c} \left\{ 0.2, 0.5, 0.7\right\}, \\\left\{ 0.2, 0.3\right\} \end{array} \right) | \left( \begin{array}{c} \left\{ 0.3, 0.5\right\}, \\\left\{ 0.8\right\} \end{array} \right) |
\propto _{2} | \left( \begin{array}{c} \left\{ 0.2, 0.3, 0.5\right\}, \\\left\{ 0.2, 0.7\right\} \end{array} \right) | \left( \begin{array}{c} \left\{ 0.2, 0.3, , 0.5\right\}, \\\left\{ 0.3, 0.4\right\} \end{array} \right) | \left( \begin{array}{c} \left\{ 0.1, 0.4, 0.6\right\}, \\\left\{ 0.7, 0.9\right\} \end{array} \right) | \left( \begin{array}{c} \left\{ 0.2, 0.4\right\}, \\\left\{ 0.7\right\} \end{array} \right) |
\propto _{3} | \left( \begin{array}{c} \left\{ 0.5, 0.6\right\}, \\\left\{ 0.7, 0.9\right\} \end{array} \right) | \left( \begin{array}{c} \left\{ 0.5, 0.8, 0.9\right\}, \\\left\{ 0.1, 0.9\right\} \end{array} \right) | \left( \begin{array}{c} \left\{ 0.2, 0.3\right\}, \\\left\{ 0.5, 0.9\right\} \end{array} \right) | \left( \begin{array}{c} \left\{ 0.7, 0.9\right\}, \\\left\{ 0.1, 0.2, 0.3\right\} \end{array} \right) |
\propto _{4} | \left( \begin{array}{c} \left\{ 0.2, 0.5, 0.9\right\}, \\\left\{ 0.6, 0.7, 0.9\right\} \end{array} \right) | \left( \begin{array}{c} \left\{ 0.3, 0.8, 0.9\right\}, \\\left\{ 0.4, 0.8\right\} \end{array} \right) | \left( \begin{array}{c} \left\{ 0.2, 0.5\right\}, \\\left\{ 0.6, 0.9\right\} \end{array} \right) | \left( \begin{array}{c} \left\{ 0.5, 0.7\right\}, \\\left\{ 0.1, 0.8\right\} \end{array} \right) |
\chi _{1} | \chi _{2} | |
A_{1} | \left( \begin{array}{c} \left( \begin{array}{c} \left( 0.1, 0.2, 0.5\right), \\ \left( 0.3, 0.4\right) \end{array} \right), \\ \left( \begin{array}{c} \left( 0.8\right), \\ \left( 0.4, 0.6\right) \end{array} \right) \end{array} \right) | \left( \begin{array}{c} \left( \begin{array}{c} \left( 0.5, 0.7\right), \\ \left( 0.5, 0.6\right) \end{array} \right), \\ \left( \begin{array}{c} \left( 0.4, 0.5\right), \\ \left( 0.7, 0.9\right) \end{array} \right) \end{array} \right) |
A_{2} | \left( \begin{array}{c} \left( \begin{array}{c} \left( 0.6, 0.7\right), \\ \left( 0.7, 0.9\right) \end{array} \right), \\ \left( \begin{array}{c} \left( 0.3, 0.5\right), \\ \left( 0.6\right) \end{array} \right) \end{array} \right) | \left( \begin{array}{c} \left( \begin{array}{c} \left( 0.2, 0.4, 0.5\right), \\ \left( 0.5\right) \end{array} \right), \\ \left( \begin{array}{c} \left( 0.6, 0.7\right), \\ \left( 0.3\right) \end{array} \right) \end{array} \right) |
\chi _{3} | \chi _{4} | |
A_{1} | \left( \begin{array}{c} \left( \begin{array}{c} \left( 0.4\right), \\ \left( 0.3, 0.7\right) \end{array} \right) \bf{, } \\ \left( \begin{array}{c} \left( 0.5\right), \\ \left( 0.9\right) \end{array} \right) \end{array} \right) | \left( \begin{array}{c} \left( \begin{array}{c} \left( 0.6\right) \bf{, } \\ \left( 0.7\right) \end{array} \right), \\ \left( \begin{array}{c} \left( 0.6, 0.8, 0.9\right), \\ \left( 0.7, 0.9\right) \end{array} \right) \end{array} \right) |
A_{2} | \left( \begin{array}{c} \left( \begin{array}{c} \left( 0.8\right), \\ \left( 0.4, 0.5, 0.7\right) \end{array} \right), \\ \left( \begin{array}{c} \left( 0.2, 0.5\right), \\ \left( 0.4, 0.5\right) \end{array} \right) \end{array} \right) | \left( \begin{array}{c} \left( \begin{array}{c} \left( 0.8\right), \\ \left( 0.5\right) \end{array} \right), \\ \left( \begin{array}{c} \left( 0.7\right), \\ \left( 0.1, 0.3, 0.4\right) \end{array} \right) \end{array} \right) |
\chi _{1} | \chi_{2} | |
A_{3} | \left( \begin{array}{c} \left( \begin{array}{c} \left( 0.4, 0.5, 0.6\right), \\ \left( 0.6, 0.7\right) \end{array} \right), \\ \left( \begin{array}{c} \left( 0.9\right), \\ \left( 0.5\right) \end{array} \right) \end{array} \right) | \left( \begin{array}{c} \left( \begin{array}{c} \left( 0.1\right), \\ \left( 0.5, 0.6\right) \end{array} \right), \\ \left( \begin{array}{c} \left( 0.4, 0.6, 0.7\right), \\ \left( 0.5, 0.7\right) \end{array} \right) \end{array} \right) |
A_{4} | \left( \begin{array}{c} \left( \begin{array}{c} \left( 0.4\right), \\ \left( 0.5, 0.6\right) \end{array} \right), \\ \left( \begin{array}{c} \left( 0.3, 0.4\right), \\ \left( 0.8\right) \end{array} \right) \end{array} \right) | \left( \begin{array}{c} \left( \begin{array}{c} \left( 0.4, 0.5\right), \\ \left( 0.4\right) \end{array} \right), \\ \left( \begin{array}{c} \left( 0.1, 0.2\right), \\ \left( 0.2, 0.3\right) \end{array} \right) \end{array} \right) |
\chi _{3} | \chi _{4} | |
A_{3} | \left( \begin{array}{c} \left( \begin{array}{c} \left( 0.3\right), \\ \left( 0.7, 0.8\right) \end{array} \right), \\ \left( \begin{array}{c} \left( 0.7, 0.8\right), \\ \left( 0.1, 0.4, 0.7\right) \end{array} \right) \end{array} \right) | \left( \begin{array}{c} \left( \begin{array}{c} \left( 0.3, 0.6\right), \\ \left( 0.8\right) \end{array} \right), \\ \left( \begin{array}{c} \left( 0.7\right), \\ \left( 0.3\right) \end{array} \right) \end{array} \right) |
A_{4} | \left( \begin{array}{c} \left( \begin{array}{c} \left( 0.3\right), \\ \left( 0.7, 0.8\right) \end{array} \right), \\ \left( \begin{array}{c} \left( 0.7\right), \\ \left( 0.6\right) \end{array} \right) \end{array} \right) | \left( \begin{array}{c} \left( \begin{array}{c} \left( 0.6, 0.7, 0.9\right), \\ \left( 0.3, 0.4\right) \end{array} \right), \\ \left( \begin{array}{c} \left( 0.2, 0.7\right), \\ \left( 0.7, 0.8, 0.9\right) \end{array} \right) \end{array} \right) |
A_{1} | \left( \begin{array}{c} \left( \begin{array}{c} \left\{ 0.4934, 0.5659, 0.9634, 0.56680.5143, 0.5817\right\}, \\ \left\{ 0.0047, 0.0113, 0.0057, 0.0136, 0.0063, 0.0150, 0.0076, 0.0181\right\} \end{array} \right) {\bf{, }} \\ \left( \begin{array}{c} \left\{ 0.5932, 0.6759, 0.9871, 0.5777, 0.6647, 0.7320\right\}, \\ \left\{ 0.0281, 0.0371, 0.0370, 0.0488, 0.0423, 0.0558, 0.0556, 0.0735\right\} \end{array} \right) \end{array} \right) |
A_{2} | \left( \begin{array}{c} \left( \begin{array}{c} \left\{ 0.7074, 0.7160, 0.8976, 0.7074, 0.7160, 0.7252\right\}, \\ \left\{ 0.0105, 0.0132, 0.0187, 0.0137, 0.0171, 0.0243\right\} \end{array} \right) {\bf{, }} \\ \left( \begin{array}{c} \left\{ 0.5584, 0.5915, 0.9867, 0.6235, 0.5714, 0.6030, 0.6057, 0.6336\right\}, \\ \left\{ 0.0011, 0.0032, 0.0043, 0.0013, 0.0040, 0.0054\right\} \end{array} \right) \end{array} \right) |
A_{3} | \left( \begin{array}{c} \left( \begin{array}{c} \left\{ 0.2916, 0.4384, 0.9834, 0.4518, 0.3556, 0.4707\right\}, \\ \left\{ 0.0262, 0.0302, 0.0315, 0.0364, 0.0306, 0.0353, 0.0369, 0.0426\right\} \end{array} \right) {\bf{, }} \\ \left( \begin{array}{c} \left\{ 0.6968, 0.7310, 0.9674, 0.7522, 0.7408, 0.7695\right\}, \\ \left\{ 0.0011, 0.0063, 0.0079, 0.0016, 0.0063, 0.0112\right\} \end{array} \right) \end{array} \right) |
A_{4} | \left( \begin{array}{c} \left( \begin{array}{c} \left\{ 0.4659, 0.5233, 0.9234, 0.4899, 0.5424, 0.6876\right\}, \\ \left\{ 0.0063, 0.0084, 0.0073, 0.0097, 0.0076, 0.0102, 0.0088, 0.0117\right\} \end{array} \right) {\bf{, }} \\ \left( \begin{array}{c} \left\{ 0.4784, 0.5990, 0.9543, 0.6006, 0.4852, 0.6032, 0.4879, 0.6048\right\}, \\ \left\{ 0.0103, 0.0119, 0.0136, 0.0155, 0.0179, 0.0204\right\} \end{array} \right) \end{array} \right) |
\chi _{1} | \chi _{2} | \chi _{3} | \chi _{4} | |
A_{1} | 0.5542 | 0.4250 | 0.3750 | 0.4667 |
A_{2} | 0.4000 | 0.5542 | 0.5417 | 0.6833 |
A_{3} | 0.5625 | 0.3792 | 0.4750 | 0.5125 |
A_{4} | 0.3500 | 0.4875 | 0.4125 | 0.5083 |
\chi _{1} | \chi _{2} | |
A_{1} | \left( \begin{array}{c} \left( \begin{array}{c} \left( 0.1, 0.2, 0.5\right), \\ \left( 0.3, 0.4\right) \end{array} \right), \\ \left( \begin{array}{c} \left( 0.8\right), \\ \left( 0.4, 0.6\right) \end{array} \right) \end{array} \right) | \left( \begin{array}{c} \left( \begin{array}{c} \left( 0.6\right) {\bf{, }} \\ \left( 0.7\right) \end{array} \right), \\ \left( \begin{array}{c} \left( 0.6, 0.8, 0.9\right), \\ \left( 0.7, 0.9\right) \end{array} \right) \end{array} \right) |
A_{2} | \left( \begin{array}{c} \left( \begin{array}{c} \left( 0.8\right), \\ \left( 0.5\right) \end{array} \right), \\ \left( \begin{array}{c} \left( 0.7\right), \\ \left( 0.1, 0.3, 0.4\right) \end{array} \right) \end{array} \right) | \left( \begin{array}{c} \left( \begin{array}{c} \left( 0.2, 0.4, 0.5\right), \\ \left( 0.5\right) \end{array} \right), \\ \left( \begin{array}{c} \left( 0.6, 0.7\right), \\ \left( 0.3\right) \end{array} \right) \end{array} \right) |
\chi _{3} | \chi _{4} | |
A_{1} | \left( \begin{array}{c} \left( \begin{array}{c} \left( 0.5, 0.7\right), \\ \left( 0.5, 0.6\right) \end{array} \right), \\ \left( \begin{array}{c} \left( 0.4, 0.5\right), \\ \left( 0.7, 0.9\right) \end{array} \right) \end{array} \right) | \left( \begin{array}{c} \left( \begin{array}{c} \left( 0.4\right), \\ \left( 0.3, 0.7\right) \end{array} \right) {\bf{, }} \\ \left( \begin{array}{c} \left( 0.5\right), \\ \left( 0.9\right) \end{array} \right) \end{array} \right) |
A_{2} | \left( \begin{array}{c} \left( \begin{array}{c} \left( 0.8\right), \\ \left( 0.4, 0.5, 0.7\right) \end{array} \right), \\ \left( \begin{array}{c} \left( 0.2, 0.5\right), \\ \left( 0.4, 0.5\right) \end{array} \right) \end{array} \right) | \left( \begin{array}{c} \left( \begin{array}{c} \left( 0.6, 0.7\right), \\ \left( 0.7, 0.9\right) \end{array} \right), \\ \left( \begin{array}{c} \left( 0.3, 0.5\right), \\ \left( 0.6\right) \end{array} \right) \end{array} \right) |
\chi _{1} | \chi _{2} | |
A_{3} | \left( \begin{array}{c} \left( \begin{array}{c} \left( 0.4, 0.5, 0.6\right), \\ \left( 0.6, 0.7\right) \end{array} \right), \\ \left( \begin{array}{c} \left( 0.9\right), \\ \left( 0.5\right) \end{array} \right) \end{array} \right) | \left( \begin{array}{c} \left( \begin{array}{c} \left( 0.3, 0.6\right), \\ \left( 0.8\right) \end{array} \right), \\ \left( \begin{array}{c} \left( 0.7\right), \\ \left( 0.3\right) \end{array} \right) \end{array} \right) |
A_{4} | \left( \begin{array}{c} \left( \begin{array}{c} \left( 0.6, 0.7, 0.9\right), \\ \left( 0.3, 0.4\right) \end{array} \right), \\ \left( \begin{array}{c} \left( 0.2, 0.7\right), \\ \left( 0.7, 0.8, 0.9\right) \end{array} \right) \end{array} \right) | \left( \begin{array}{c} \left( \begin{array}{c} \left( 0.4, 0.5\right), \\ \left( 0.4\right) \end{array} \right), \\ \left( \begin{array}{c} \left( 0.1, 0.2\right), \\ \left( 0.2, 0.3\right) \end{array} \right) \end{array} \right) |
\chi _{3} | \chi _{4} | |
A_{3} | \left( \begin{array}{c} \left( \begin{array}{c} \left( 0.3\right), \\ \left( 0.7, 0.8\right) \end{array} \right), \\ \left( \begin{array}{c} \left( 0.7, 0.8\right), \\ \left( 0.1, 0.4, 0.7\right) \end{array} \right) \end{array} \right) | \left( \begin{array}{c} \left( \begin{array}{c} \left( 0.1\right), \\ \left( 0.5, 0.6\right) \end{array} \right), \\ \left( \begin{array}{c} \left( 0.4, 0.6, 0.7\right), \\ \left( 0.5, 0.7\right) \end{array} \right) \end{array} \right) |
A_{4} | \left( \begin{array}{c} \left( \begin{array}{c} \left( 0.3\right), \\ \left( 0.7, 0.8\right) \end{array} \right), \\ \left( \begin{array}{c} \left( 0.7\right), \\ \left( 0.6\right) \end{array} \right) \end{array} \right) | \left( \begin{array}{c} \left( \begin{array}{c} \left( 0.4\right), \\ \left( 0.5, 0.6\right) \end{array} \right), \\ \left( \begin{array}{c} \left( 0.3, 0.4\right), \\ \left( 0.8\right) \end{array} \right) \end{array} \right) |
A_{1} | \left( \begin{array}{c} \left( \begin{array}{c} \left\{ 0.4865, 0.5656, 0.4878, 0.5665, 0.5081, 0.5814\right\}, \\ \left\{ 0.4419, 0.5744, 0.4669, 0.6049, 0.4583, 0.5945, 0.4841, 0.6257\right\} \end{array} \right) {\bf{, }} \\ \left( \begin{array}{c} \left\{ 0.5898, 0.5729, 0.6640, 0.6513, 0.7238, 0.7139\right\}, \\ \left\{ 0.7150, 0.7732, 0.7692, 0.8277, 0.7481, 0.8067, 0.8026, 0.8609\right\} \end{array} \right) \end{array} \right) |
A_{2} | \left( \begin{array}{c} \left( \begin{array}{c} \left\{ 0.6682, 0.6957, 0.6783, 0.7048, 0.6889, 0.7144\right\}, \\ \left\{ 0.5235, 0.5766, 0.5573, 0.6127, 0.6149, 0.6737\right\} \end{array} \right) {\bf{, }} \\ \left( \begin{array}{c} \left\{ 0.4876, 0.5265, 0.5306, 0.5637, 0.5343, 0.5669, 0.5704, 0.5991\right\}, \\ \left\{ 0.3535, 0.3777, 0.4065, 0.4339, 0.4217, 0.4500\right\} \end{array} \right) \end{array} \right) |
A_{3} | \left( \begin{array}{c} \left( \begin{array}{c} \left\{ 0.2875, 0.4372, 0.3167, 0.4507, 0.3529, 0.4697\right\}, \\ \left\{ 0.6461, 0.6810, 0.6738, 0.7094, 0.6591, 0.6944, 0.6872, 0.7230\right\} \end{array} \right) {\bf{, }} \\ \left( \begin{array}{c} \left\{ 0.6896, 0.7180, 0.7408, 0.7247, 0.7495, 0.7695\right\}, \\ \left\{ 0.2751, 0.3088, 0.4092, 0.4578, 0.4847, 0.5407\right\} \end{array} \right) \end{array} \right) |
A_{4} | \left( \begin{array}{c} \left( \begin{array}{c} \left\{ 0.4187, 0.4480, 0.4502, 0.4758, 0.5473, 0.5647\right\}, \\ \left\{ 0.4897, 0.5188, 0.5127, 0.5429, 0.5075, 0.5375, 0.5313, 0.5622\right\} \end{array} \right) {\bf{, }} \\ \left( \begin{array}{c} \left\{ 0.4833, 0.4989, 0.4859, 0.5014, 0.5400, 0.5525, 0.5421, 0.5545\right\}, \\ \left\{ 0.5111, 0.5659, 0.5217, 0.5773, 0.5325, 0.5889\right\} \end{array} \right) \end{array} \right) |
\chi _{1} | \chi _{2} | |
A_{1} | \left( \begin{array}{c} \left( \begin{array}{c} \left( 0.0479, 0.0958, 0.2400\right), \\ \left( 0.9159, 0.9339\right) \end{array} \right), \\ \left( \begin{array}{c} \left( 0.3960\right), \\ \left( 0.9339, 0.9599\right) \end{array} \right) \end{array} \right) | \left( \begin{array}{c} \left( \begin{array}{c} \left( 0.3276, 0.4638\right) {\bf{, }} \\ \left( 0.8609, 0.8933\right) \end{array} \right), \\ \left( \begin{array}{c} \left( 0.3276, 0.2618\right), \\ \left( 0.8989, 0.9734\right) \end{array} \right) \end{array} \right) |
A_{2} | \left( \begin{array}{c} \left( \begin{array}{c} \left( 0.2890, 0.3400\right), \\ \left( 0.9703, 0.9897\right) \end{array} \right), \\ \left( \begin{array}{c} \left( 0.1438, 0.2400\right), \\ \left( 0.9599\right) \end{array} \right) \end{array} \right) | \left( \begin{array}{c} \left( \begin{array}{c} \left( 0.1308, 0.2618, 0.3276\right), \\ \left( 0.8609\right) \end{array} \right), \\ \left( \begin{array}{c} \left( 0.3944, 0.4638\right), \\ \left( 0.7285\right) \end{array} \right) \end{array} \right) |
A_{3} | \left( \begin{array}{c} \left( \begin{array}{c} \left( 0.1917, 0.2400, 0.2890\right), \\ \left( 0.9599, 0.9703\right) \end{array} \right), \\ \left( \begin{array}{c} \left( 0.4666\right), \\ \left( 0.9481\right) \end{array} \right) \end{array} \right) | \left( \begin{array}{c} \left( \begin{array}{c} \left( 0.0654\right), \\ \left( 0.8609, 0.8933\right) \end{array} \right), \\ \left( \begin{array}{c} \left( 0.2618, 0.3944, 0.4638\right), \\ \left( 0.8609, 0.9217\right) \end{array} \right) \end{array} \right) |
A_{4} | \left( \begin{array}{c} \left( \begin{array}{c} \left( 0.1917\right), \\ \left( 0.9481, 0.9599\right) \end{array} \right), \\ \left( \begin{array}{c} \left( 0.1438, 0.1917\right), \\ \left( 0.9801\right) \end{array} \right) \end{array} \right) | \left( \begin{array}{c} \left( \begin{array}{c} \left( 0.2618, 0.3276\right), \\ \left( 0.8222\right) \end{array} \right), \\ \left( \begin{array}{c} \left( 0.0654, 0.1308\right), \\ \left( 0.7058, 0.7733\right) \end{array} \right) \end{array} \right) |
\chi _{3} | \chi _{4} | |
A_{1} | \left( \begin{array}{c} \left( \begin{array}{c} \left( 0.2679\right), \\ \left( 0.7563, 0.9158\right) \end{array} \right), \\ \left( \begin{array}{c} \left( 0.3352\right), \\ \left( 0.9715\right) \end{array} \right) \end{array} \right) | \left( \begin{array}{c} \left( \begin{array}{c} \left( 0.4080\right), \\ \left( 0.9128\right) \end{array} \right) {\bf{, }} \\ \left( \begin{array}{c} \left( 0.4080, 0.5578, 0.6539\right), \\ \left( 0.9128, 0.9705\right) \end{array} \right) \end{array} \right) |
A_{2} | \left( \begin{array}{c} \left( \begin{array}{c} \left( 0.5518\right), \\ \left( 0.8087, 0.8503, 0.9158\right) \end{array} \right), \\ \left( \begin{array}{c} \left( 0.1339, 0.3352\right), \\ \left( 0.8087, 0.8503\right) \end{array} \right) \end{array} \right) | \left( \begin{array}{c} \left( \begin{array}{c} \left( 0.5578\right), \\ \left( 0.8450\right) \end{array} \right), \\ \left( \begin{array}{c} \left( 0.4797\right), \\ \left( 0.5574, 0.7478, 0.8020\right) \end{array} \right) \end{array} \right) |
A_{3} | \left( \begin{array}{c} \left( \begin{array}{c} \left( 0.2008\right), \\ \left( 0.9158, 0.9440\right) \end{array} \right), \\ \left( \begin{array}{c} \left( 0.4745, 0.5518\right), \\ \left( 0.5704, 0.8087, 0.9158\right) \end{array} \right) \end{array} \right) | \left( \begin{array}{c} \left( \begin{array}{c} \left( 0.2031, 0.4080\right), \\ \left( 0.9421\right) \end{array} \right), \\ \left( \begin{array}{c} \left( 0.4797\right), \\ \left( 0.7478\right) \end{array} \right) \end{array} \right) |
A_{4} | \left( \begin{array}{c} \left( \begin{array}{c} \left( 0.2008\right), \\ \left( 0.9158, 0.9440\right) \end{array} \right), \\ \left( \begin{array}{c} \left( 0.4745\right), \\ \left( 0.8851\right) \end{array} \right) \end{array} \right) | \left( \begin{array}{c} \left( \begin{array}{c} \left( 0.4080, 0.4797, 0.6539\right), \\ \left( 0.7478, 0.8020\right) \end{array} \right), \\ \left( \begin{array}{c} \left( 0.1354, 0.4797\right), \\ \left( 0.9128, 0.9421, 0.9705\right) \end{array} \right) \end{array} \right) |
\chi _{1} | \chi _{2} | \chi _{3} | \chi _{4} | |
A_{1} | 0.1630 | 0.2193 | 0.1989 | 0.2734 |
A_{2} | 0.1416 | 0.2700 | 0.2746 | 0.3725 |
A_{3} | 0.1984 | 0.1676 | 0.2548 | 0.2738 |
A_{4} | 0.1064 | 0.2078 | 0.2151 | 0.2762 |
\chi _{1} | \chi _{2} | |
A_{1} | \left( \begin{array}{c} \left( \begin{array}{c} \left( 0.4080\right), \\ \left( 0.9128\right) \end{array} \right) {\bf{, }} \\ \left( \begin{array}{c} \left( 0.4080, 0.5578, 0.6539\right), \\ \left( 0.9128, 0.9705\right) \end{array} \right) \end{array} \right) | \left( \begin{array}{c} \left( \begin{array}{c} \left( 0.3276, 0.4638\right) {\bf{, }} \\ \left( 0.8609, 0.8933\right) \end{array} \right), \\ \left( \begin{array}{c} \left( 0.3276, 0.2618\right), \\ \left( 0.8989, 0.9734\right) \end{array} \right) \end{array} \right) |
A_{2} | \left( \begin{array}{c} \left( \begin{array}{c} \left( 0.5578\right), \\ \left( 0.8450\right) \end{array} \right), \\ \left( \begin{array}{c} \left( 0.4797\right), \\ \left( 0.55740.74780.8020\right) \end{array} \right) \end{array} \right) | \left( \begin{array}{c} \left( \begin{array}{c} \left( 0.5518\right), \\ \left( 0.80870.8503, 0.9158\right) \end{array} \right), \\ \left( \begin{array}{c} \left( 0.13390.3352\right), \\ \left( 0.80870.8503\right) \end{array} \right) \end{array} \right) |
A_{3} | \left( \begin{array}{c} \left( \begin{array}{c} \left( 0.2031, 0.4080\right), \\ \left( 0.9421\right) \end{array} \right), \\ \left( \begin{array}{c} \left( 0.4797\right), \\ \left( 0.7478\right) \end{array} \right) \end{array} \right) | \left( \begin{array}{c} \left( \begin{array}{c} \left( 0.2008\right), \\ \left( 0.9158, 0.9440\right) \end{array} \right), \\ \left( \begin{array}{c} \left( 0.4745, 0.5518\right), \\ \left( 0.5704, 0.8087, 0.9158\right) \end{array} \right) \end{array} \right) |
A_{4} | \left( \begin{array}{c} \left( \begin{array}{c} \left( 0.4080, 0.4797, 0.6539\right), \\ \left( 0.7478, 0.8020\right) \end{array} \right), \\ \left( \begin{array}{c} \left( 0.1354, 0.4797\right), \\ \left( 0.9128, 0.9421, 0.9705\right) \end{array} \right) \end{array} \right) | \left( \begin{array}{c} \left( \begin{array}{c} \left( 0.2008\right), \\ \left( 0.9158, 0.9440\right) \end{array} \right), \\ \left( \begin{array}{c} \left( 0.4745\right), \\ \left( 0.8851\right) \end{array} \right) \end{array} \right) |
\chi _{3} | \chi _{4} | |
A_{1} | \left( \begin{array}{c} \left( \begin{array}{c} \left( 0.2679\right), \\ \left( 0.7563, 0.9158\right) \end{array} \right), \\ \left( \begin{array}{c} \left( 0.3352\right), \\ \left( 0.9715\right) \end{array} \right) \end{array} \right) | \left( \begin{array}{c} \left( \begin{array}{c} \left( 0.0479, 0.0958, 0.2400\right), \\ \left( 0.9159, 0.9339\right) \end{array} \right), \\ \left( \begin{array}{c} \left( 0.3960\right), \\ \left( 0.9339, 0.9599\right) \end{array} \right) \end{array} \right) |
A_{2} | \left( \begin{array}{c} \left( \begin{array}{c} \left( 0.1308, 0.2618, 0.3276\right), \\ \left( 0.8609\right) \end{array} \right), \\ \left( \begin{array}{c} \left( 0.3944, 0.4638\right), \\ \left( 0.7285\right) \end{array} \right) \end{array} \right) | \left( \begin{array}{c} \left( \begin{array}{c} \left( 0.2890, 0.3400\right), \\ \left( 0.9703, 0.9897\right) \end{array} \right), \\ \left( \begin{array}{c} \left( 0.1438, 0.2400\right), \\ \left( 0.9599\right) \end{array} \right) \end{array} \right) |
A_{3} | \left( \begin{array}{c} \left( \begin{array}{c} \left( 0.1917, 0.2400, 0.2890\right), \\ \left( 0.9599, 0.9703\right) \end{array} \right), \\ \left( \begin{array}{c} \left( 0.4666\right), \\ \left( 0.9481\right) \end{array} \right) \end{array} \right) | \left( \begin{array}{c} \left( \begin{array}{c} \left( 0.0654\right), \\ \left( 0.8609, 0.8933\right) \end{array} \right), \\ \left( \begin{array}{c} \left( 0.2618, 0.3944, 0.4638\right), \\ \left( 0.8609, 0.9217\right) \end{array} \right) \end{array} \right) |
A_{4} | \left( \begin{array}{c} \left( \begin{array}{c} \left( 0.2618, 0.3276\right), \\ \left( 0.8222\right) \end{array} \right), \\ \left( \begin{array}{c} \left( 0.0654, 0.1308\right), \\ \left( 0.7058, 0.7733\right) \end{array} \right) \end{array} \right) | \left( \begin{array}{c} \left( \begin{array}{c} \left( 0.1917\right), \\ \left( 0.9481, 0.9599\right) \end{array} \right), \\ \left( \begin{array}{c} \left( 0.1438, 0.1917\right), \\ \left( 0.9801\right) \end{array} \right) \end{array} \right) |
A_{1} | \left( \begin{array}{c} \left( \begin{array}{c} \left\{ 0.3647, 0.3527, 0.3967, 0.3866, 0.42520.4165\right\}, \\ \left\{ 0.9331, 0.9412, 0.9531, 0.9610, 0.9406, 0.9487, 0.9604, 0.9681\right\} \end{array} \right) {\bf{, }} \\ \left( \begin{array}{c} \left\{ 0.3647, 0.3527, 0.3967, 0.3866, 0.4252, 0.4165\right\}, \\ \left\{ 0.9331, 0.9412, 0.9531, 0.9610, 0.9406, 0.9487, 0.9604, 0.9683\right\} \end{array} \right) \end{array} \right) |
A_{2} | \left( \begin{array}{c} \left( \begin{array}{c} \left\{ 0.4246, 0.4331, 0.4329, 0.4410, 0.4415, 0.4494\right\}, \\ \left\{ 0.8802, 0.8869, 0.8912, 0.8978, 0.9087, 0.9152\right\} \end{array} \right) {\bf{, }} \\ \left( \begin{array}{c} \left\{ 0.3233, 0.3336, 0.3556, 0.3643, 0.3511, 0.3600, 0.3791, 0.3867\right\}, \\ \left\{ 0.7996, 0.8109, 0.8260, 0.8373, 0.8331, 0.8443\right\} \end{array} \right) \end{array} \right) |
A_{3} | \left( \begin{array}{c} \left( \begin{array}{c} \left\{ 0.1755, 0.1946, 0.2180, 0.2361, 0.2473, 0.2626\right\}, \\ \left\{ 0.9157, 0.9254, 0.9189, 0.9286, 0.9234, 0.9330, 0.9266, 0.9362\right\} \end{array} \right) {\bf{, }} \\ \left( \begin{array}{c} \left\{ 0.4279, 0.4510, 0.4696, 0.4564, 0.4768, 0.4935\right\}, \\ \left\{ 0.7915, 0.8110, 0.8585, 0.8776, 0.8874, 0.9061\right\} \end{array} \right) \end{array} \right) |
A_{4} | \left( \begin{array}{c} \left( \begin{array}{c} \left\{ 0.2641, 0.2861, 0.2884, 0.6061, 0.3606, 0.3729\right\}, \\ \left\{ 0.8781, 0.8821, 0.8861, 0.8900, 0.8850, 0.8889, 0.8929, 0.8968\right\} \end{array} \right) {\bf{, }} \\ \left( \begin{array}{c} \left\{ 0.3117, 0.3159, 0.3136, 0.3178, 0.3539, 0.3573, 0.3554, 0.3587\right\}, \\ \left\{ 0.8686, 0.8879, 0.8726, 0.8918, 0.8765, 0.8957\right\} \end{array} \right) \end{array} \right) |
Operators | SR\left( A_{1}\right) | SR\left( A_{2}\right) | SR\left( A_{3}\right) | SR\left( A_{4}\right) |
q-ROHFREWA | 0.4690 | 0.6064 | 0.5051 | 0.5181 |
q-ROHFREOWA | 0.4665 | 0.5597 | 0.5052 | 0.4823 |
q-ROHFRHWA | 0.2198 | 0.2680 | 0.2259 | 0.2322 |
Operator | Scores | Best Alternative |
q-ROHFREWA | SR\left( A_{2}\right) >SR\left( A_{4}\right) >SR\left( A_{3}\right) >SR\left( A_{1}\right) | A_{2} |
q-ROHFREOWA | SR\left( A_{2}\right) >SR\left( A_{3}\right) >SR\left( A_{4}\right) >SR\left( A_{1}\right) | A_{2} |
q-ROHFRHWA | SR\left( A_{2}\right) >SR\left( A_{4}\right) >SR\left( A_{3}\right) >SR\left( A_{1}\right) | A_{2} |
q | Score values of alternatives | Ranking | Best Alternative | |||
A_{1} | A_{2} | A_{3} | A_{4} | |||
1 | 0.5992 | 0.7522 | 0.6395 | 0.6623 | A_{2}\succ A_{4}\succ \ A_{3}\succ A_{1} | A_{2} |
2 | 0.5122 | 0.6657 | 0.5545 | 0.5698 | A_{2}\succ A_{4}\succ \ A_{3}\succ A_{1} | A_{2} |
3 | 0.4690 | 0.6064 | 0.5051 | 0.5181 | A_{2}\succ A_{4}\succ \ A_{3}\succ A_{1} | A_{2} |
4 | 0.4441 | 0.5657 | 0.4734 | 0.4868 | A_{2}\succ A_{4}\succ \ A_{3}\succ A_{1} | A_{2} |
5 | 0.4282 | 0.5366 | 0.4518 | 0.4660 | A_{2}\succ A_{4}\succ \ A_{3}\succ A_{1} | A_{2} |
10 | 0.3969 | 0.4658 | 0.4033 | 0.4185 | A_{2}\succ A_{4}\succ \ A_{3}\succ A_{1} | A_{2} |
15 | 0.3876 | 0.4382 | 0.3874 | 0.4003 | A_{2}\succ A_{4}\succ \ A_{1}\succ A_{3} | A_{2} |
20 | 0.3833 | 0.4235 | 0.3804 | 0.3905 | A_{2}\succ A_{4}\succ \ A_{1}\succ A_{3} | A_{2} |
50 | 0.3759 | 0.3953 | 0.3537 | 0.3717 | A_{2}\succ A_{4}\succ \ A_{1}\succ A_{3} | A_{2} |
100 | 0.2989 | 0.3844 | 0.2306 | 0.3144 | A_{2}\succ A_{4}\succ \ A_{1}\succ A_{3} | A_{2} |
\chi _{1} | \chi _{2} | \chi _{3} | \chi _{4} | \chi _{5} | |
A_{1} | \left( \begin{array}{c} \left( 0.64, 0.13\right), \\ \left( 0.46, 0.15\right) \end{array} \right) | \left( \begin{array}{c} \left( 0.8, 0.16\right), \\ \left( 0.63, 0.26\right) \end{array} \right) | \left( \begin{array}{c} \left( 0.75, 0.22\right), \\ \left( 0.58, 0.18\right) \end{array} \right) | \left( \begin{array}{c} \left( 0.67, 0.13\right), \\ \left( 0.60, 0.17\right) \end{array} \right) | \left( \begin{array}{c} \left( 0.78, 0.1\right), \\ \left( 0.67, 0.15\right) \end{array} \right) |
A_{2} | \left( \begin{array}{c} \left( 0.55, 0.15\right), \\ \left( 0.62, 0.24\right) \end{array} \right) | \left( \begin{array}{c} \left( 0.71, 0.18\right), \\ \left( 0.4, 0.26\right) \end{array} \right) | \left( \begin{array}{c} \left( 0.43, 0.17\right), \\ \left( 0.51, 0.20\right) \end{array} \right) | \left( \begin{array}{c} \left( 0.61, 0.26\right), \\ \left( 0.40, 0.20\right) \end{array} \right) | \left( \begin{array}{c} \left( 0.63, 0.19\right), \\ \left( 0.46, 0.26\right) \end{array} \right) |
A_{3} | \left( \begin{array}{c} \left( 0.57, 0.24\right), \\ \left( 0.40, 0.27\right) \end{array} \right) | \left( \begin{array}{c} \left( 0.71, 0.17\right), \\ \left( 0.65, 0.23\right) \end{array} \right) | \left( \begin{array}{c} \left( 0.49, 0.22\right), \\ \left( 0.36, 0.39\right) \end{array} \right) | \left( \begin{array}{c} \left( 0.54, 0.40\right), \\ \left( 0.45, 0.36\right) \end{array} \right) | \left( \begin{array}{c} \left( 0.56, 0.36\right), \\ \left( 0.32, 0.18\right) \end{array} \right) |
A_{4} | \left( \begin{array}{c} \left( 0.56, 0.17\right), \\ \left( 0.59, 0.23\right) \end{array} \right) | \left( \begin{array}{c} \left( 0.50, 0.32\right), \\ \left( 0.43, 0.42\right) \end{array} \right) | \left( \begin{array}{c} \left( 0.27, 0.13\right), \\ \left( 0.36, 0.29\right) \end{array} \right) | \left( \begin{array}{c} \left( 0.46, 0.19\right), \\ \left( 0.37, 0.36\right) \end{array} \right) | \left( \begin{array}{c} \left( 0.49, 0.25\right), \\ \left( 0.37, 0.26\right) \end{array} \right) |
A_{1} | \left( \left( 0.7338, 0.1396\right), \left( 0.6058, 0.1743\right) \right) |
A_{2} | \left( \left( 0.5992, 0.1959\right), \left( 0.4774, 0.2263\right) \right) |
A_{3} | \left( \left( 0.5717, 0.2878\right), \left( 0.4502, 0.2807\right) \right) |
A_{4} | \left( \left( 0.4656, 0.1988\right), \left( 0.4204, 0.3077\right) \right) |
Score | |||||
Ashraf et al. | SR\left( A_{1}\right) | SR\left( A_{2}\right) | SR\left( A_{3}\right) | SR\left( A_{4}\right) | Ranking |
q-ROFREWA | 0.999 | 0.489 | 0.201 | 0.011 | A_{1}>A_{2}>A_{3}>A_{4} |
Score | |||||
Developed method | SR\left( A_{1}\right) | SR\left( A_{2}\right) | SR\left( A_{3}\right) | SR\left( A_{4}\right) | Ranking |
q-ROHFREWA | 0.7564 | 0.6636 | 0.6134 | 0.5949 | A_{1}>A_{2}>A_{3}>A_{4} |
q-ROHFREOWA | 0.7612 | 0.6741 | 0.6166 | 0.5991 | A_{1}>A_{2}>A_{3}>A_{4} |
Criteria | \daleth ^{+} | \daleth ^{-} |
\chi _{1} | \left( \begin{array}{c} \left( \begin{array}{c} \left( 0.4, 0.5, 0.6\right), \\ \left( 0.6, 0.7\right) \end{array} \right), \\ \left( \begin{array}{c} \left( 0.9\right), \\ \left( 0.5\right) \end{array} \right) \end{array} \right) | \left( \begin{array}{c} \left( \begin{array}{c} \left( 0.4\right), \\ \left( 0.5, 0.6\right) \end{array} \right), \\ \left( \begin{array}{c} \left( 0.3, 0.4\right), \\ \left( 0.8\right) \end{array} \right) \end{array} \right) |
\chi _{2} | \left( \begin{array}{c} \left( \begin{array}{c} \left( 0.2, 0.4, 0.5\right), \\ \left( 0.5\right) \end{array} \right), \\ \left( \begin{array}{c} \left( 0.6, 0.7\right), \\ \left( 0.3\right) \end{array} \right) \end{array} \right) | \left( \begin{array}{c} \left( \begin{array}{c} \left( 0.1\right), \\ \left( 0.5, 0.6\right) \end{array} \right), \\ \left( \begin{array}{c} \left( 0.4, 0.6, 0.7\right), \\ \left( 0.5, 0.7\right) \end{array} \right) \end{array} \right) |
\chi _{3} | \left( \begin{array}{c} \left( \begin{array}{c} \left( 0.8\right), \\ \left( 0.4, 0.5, 0.7\right) \end{array} \right), \\ \left( \begin{array}{c} \left( 0.2, 0.5\right), \\ \left( 0.4, 0.5\right) \end{array} \right) \end{array} \right) | \left( \begin{array}{c} \left( \begin{array}{c} \left( 0.4\right), \\ \left( 0.3, 0.7\right) \end{array} \right) {\bf{, }} \\ \left( \begin{array}{c} \left( 0.5\right), \\ \left( 0.9\right) \end{array} \right) \end{array} \right) |
\chi _{4} | \left( \begin{array}{c} \left( \begin{array}{c} \left( 0.8\right), \\ \left( 0.5\right) \end{array} \right), \\ \left( \begin{array}{c} \left( 0.7\right), \\ \left( 0.1, 0.3, 0.4\right) \end{array} \right) \end{array} \right) | \left( \begin{array}{c} \left( \begin{array}{c} \left( 0.6\right) {\bf{, }} \\ \left( 0.7\right) \end{array} \right), \\ \left( \begin{array}{c} \left( 0.6, 0.8, 0.9\right), \\ \left( 0.7, 0.9\right) \end{array} \right) \end{array} \right) |
alternatives | S_{\imath} | R_{\imath} | Q_{\imath} |
A _{1} | 0.1460 | 0.2472 | 0.2137 |
A _{2} | 0.5324 | 0.4042 | 0.5631 |
A _{3} | 0.3637 | 0.3781 | 0.4319 |
A _{4} | 0.3426 | 0.2682 | 0.3142 |
Alternatives | Ranking order of S_{\imath} | Ranking order of R_{\imath} | Ranking order of Q_{\imath} |
A _{1} | 4 | 4 | 4 |
A _{2} | 1 | 1 | 1 |
A _{3} | 2 | 2 | 2 |
A _{4} | 3 | 3 | 3 |