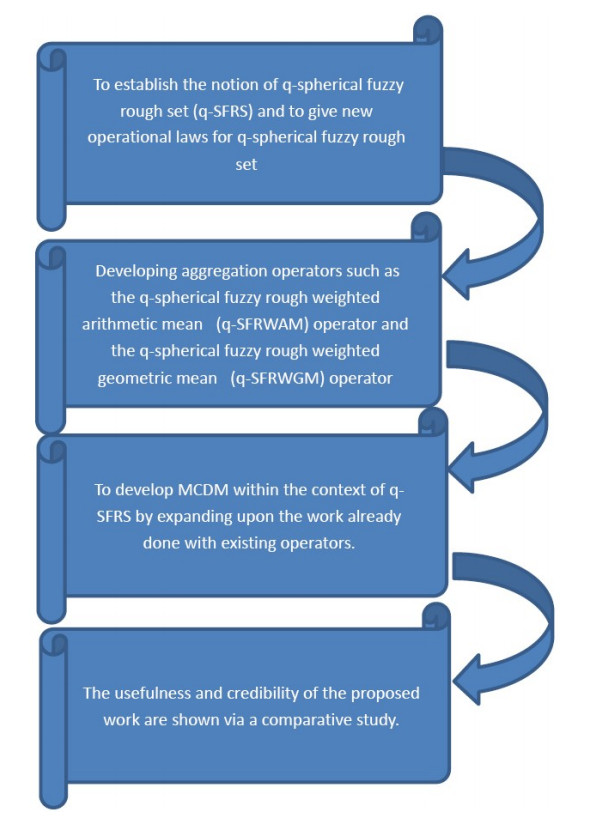
This article's purpose is to investigate and generalize the concepts of rough set, in addition to the q-spherical fuzzy set, and to introduce a novel concept that is called q-spherical fuzzy rough set (q-SFRS). This novel approach avoids the complications of more recent ideas like the intuitionistic fuzzy rough set, Pythagorean fuzzy rough set, and q-rung orthopair fuzzy rough set. Since mathematical operations known as "aggregation operators" are used to bring together sets of data. Popular aggregation operations include the arithmetic mean and the weighted mean. The key distinction between the weighted mean and the arithmetic mean is that the latter allows us to weight the various values based on their importance. Various aggregation operators make different assumptions about the input (data kinds) and the kind of information that may be included in the model. Because of this, some new q-spherical fuzzy rough weighted arithmetic mean operator and q-spherical fuzzy rough weighted geometric mean operator have been introduced. The developed operators are more general. Because the picture fuzzy rough weighted arithmetic mean (PFRWAM) operator, picture fuzzy rough weighted geometric mean (PFRWGM) operator, spherical fuzzy rough weighted arithmetic mean (SFRWAM) operator and spherical fuzzy rough weighted geometric mean (SFRWGM) operator are all the special cases of the q-SFRWAM and q-SFRWGM operators. When parameter q = 1, the q-SFRWAM operator reduces the PFRWAM operator, and the q-SFRWGM operator reduces the PFRWGM operator. When parameter q = 2, the q-SFRWAM operator reduces the SFRWAM operator, and the q-SFRWGM operator reduces the SFRWGM operator. Besides, our approach is more flexible, and decision-makers can choose different values of parameter q according to the different risk attitudes. In addition, the basic properties of these newly presented operators have been analyzed in great depth and expounded upon. Additionally, a technique called multi-criteria decision-making (MCDM) has been established, and a detailed example has been supplied to back up the recently introduced work. An evaluation of the offered methodology is established at the article's conclusion. The results of this research show that, compared to the q-spherical fuzzy set, our method is better and more effective.
Citation: Ahmad Bin Azim, Ahmad ALoqaily, Asad Ali, Sumbal Ali, Nabil Mlaiki, Fawad Hussain. q-Spherical fuzzy rough sets and their usage in multi-attribute decision-making problems[J]. AIMS Mathematics, 2023, 8(4): 8210-8248. doi: 10.3934/math.2023415
[1] | Ahmad Bin Azim, Ahmad ALoqaily, Asad Ali, Sumbal Ali, Nabil Mlaiki . Industry 4.0 project prioritization by using q-spherical fuzzy rough analytic hierarchy process. AIMS Mathematics, 2023, 8(8): 18809-18832. doi: 10.3934/math.2023957 |
[2] | Shahzaib Ashraf, Huzaira Razzaque, Muhammad Naeem, Thongchai Botmart . Spherical q-linear Diophantine fuzzy aggregation information: Application in decision support systems. AIMS Mathematics, 2023, 8(3): 6651-6681. doi: 10.3934/math.2023337 |
[3] | Muhammad Naeem, Muhammad Qiyas, Lazim Abdullah, Neelam Khan, Salman Khan . Spherical fuzzy rough Hamacher aggregation operators and their application in decision making problem. AIMS Mathematics, 2023, 8(7): 17112-17141. doi: 10.3934/math.2023874 |
[4] | Attaullah, Shahzaib Ashraf, Noor Rehman, Asghar Khan, Muhammad Naeem, Choonkil Park . Improved VIKOR methodology based on q-rung orthopair hesitant fuzzy rough aggregation information: application in multi expert decision making. AIMS Mathematics, 2022, 7(5): 9524-9548. doi: 10.3934/math.2022530 |
[5] | Muhammad Akram, Sumera Naz, Feng Feng, Ghada Ali, Aqsa Shafiq . Extended MABAC method based on 2-tuple linguistic T-spherical fuzzy sets and Heronian mean operators: An application to alternative fuel selection. AIMS Mathematics, 2023, 8(5): 10619-10653. doi: 10.3934/math.2023539 |
[6] | Attaullah, Shahzaib Ashraf, Noor Rehman, Asghar Khan, Choonkil Park . A decision making algorithm for wind power plant based on q-rung orthopair hesitant fuzzy rough aggregation information and TOPSIS. AIMS Mathematics, 2022, 7(4): 5241-5274. doi: 10.3934/math.2022292 |
[7] | Muhammad Naeem, Aziz Khan, Shahzaib Ashraf, Saleem Abdullah, Muhammad Ayaz, Nejib Ghanmi . A novel decision making technique based on spherical hesitant fuzzy Yager aggregation information: application to treat Parkinson's disease. AIMS Mathematics, 2022, 7(2): 1678-1706. doi: 10.3934/math.2022097 |
[8] | Ashraf Al-Quran . T-spherical linear Diophantine fuzzy aggregation operators for multiple attribute decision-making. AIMS Mathematics, 2023, 8(5): 12257-12286. doi: 10.3934/math.2023618 |
[9] | Muhammad Ali Khan, Saleem Abdullah, Alaa O. Almagrabi . Analysis of deep learning technique using a complex spherical fuzzy rough decision support model. AIMS Mathematics, 2023, 8(10): 23372-23402. doi: 10.3934/math.20231188 |
[10] | Rabia Mazhar, Shahida Bashir, Muhammad Shabir, Mohammed Al-Shamiri . A soft relation approach to approximate the spherical fuzzy ideals of semigroups. AIMS Mathematics, 2025, 10(2): 3734-3758. doi: 10.3934/math.2025173 |
This article's purpose is to investigate and generalize the concepts of rough set, in addition to the q-spherical fuzzy set, and to introduce a novel concept that is called q-spherical fuzzy rough set (q-SFRS). This novel approach avoids the complications of more recent ideas like the intuitionistic fuzzy rough set, Pythagorean fuzzy rough set, and q-rung orthopair fuzzy rough set. Since mathematical operations known as "aggregation operators" are used to bring together sets of data. Popular aggregation operations include the arithmetic mean and the weighted mean. The key distinction between the weighted mean and the arithmetic mean is that the latter allows us to weight the various values based on their importance. Various aggregation operators make different assumptions about the input (data kinds) and the kind of information that may be included in the model. Because of this, some new q-spherical fuzzy rough weighted arithmetic mean operator and q-spherical fuzzy rough weighted geometric mean operator have been introduced. The developed operators are more general. Because the picture fuzzy rough weighted arithmetic mean (PFRWAM) operator, picture fuzzy rough weighted geometric mean (PFRWGM) operator, spherical fuzzy rough weighted arithmetic mean (SFRWAM) operator and spherical fuzzy rough weighted geometric mean (SFRWGM) operator are all the special cases of the q-SFRWAM and q-SFRWGM operators. When parameter q = 1, the q-SFRWAM operator reduces the PFRWAM operator, and the q-SFRWGM operator reduces the PFRWGM operator. When parameter q = 2, the q-SFRWAM operator reduces the SFRWAM operator, and the q-SFRWGM operator reduces the SFRWGM operator. Besides, our approach is more flexible, and decision-makers can choose different values of parameter q according to the different risk attitudes. In addition, the basic properties of these newly presented operators have been analyzed in great depth and expounded upon. Additionally, a technique called multi-criteria decision-making (MCDM) has been established, and a detailed example has been supplied to back up the recently introduced work. An evaluation of the offered methodology is established at the article's conclusion. The results of this research show that, compared to the q-spherical fuzzy set, our method is better and more effective.
The fuzzy set theory is an extension of the more conventional crisp set theory, which was described for the first time by Zadeh [1]. Also, it was Zadeh who first introduced the idea of a fuzzy set, which considers grades that were earned with a positive grade. The intuitionistic fuzzy set (IFS) was proposed by Atanassov [2] as an extension of the fuzzy set, in which both the positive and negative grades are included, but only if their sum is less than or equal to 1. In other words, both the positive grade and the negative grade are taken into consideration by the IFS. IFS has grown in significance ever since it was first introduced, and it has been put to extensive use in the solution of decision-making issues. In 2014, Cuong [3] expanded the notion of fuzzy sets and IFS and invented a new concept that he referred to as a picture fuzzy (PF) set. In this concept, he specifies positive grades, neutral grades, and negative grades. Multiple attribute group decision-making strategies based on intuitionistic fuzzy frank power aggregation operators were developed by Zhang et al. [4] in 2015. Seikh and Mandal [5] have introduced several intuitionistic fuzzy Dombi aggregation operators and applied them to MCDM issues. Additionally, Zeng et al. [6] established the MCDM by merging social network analysis with an intuitionistic fuzzy hybrid contingent aggregation operator. It is important to keep in mind that the restriction sum (μ+, μ−) ∈ [0, 1] in IFS restricts the potential of obtaining both a good grade "μ+" and a negative grade "μ−" for the same work. To get over this limitation, as an extension of the IFS, Yager [7] constructed what is now known as the Pythagorean fuzzy set (PyFS). For this generalization, we will make use of the required condition, which may be represented as 0≤μ+2+μ−2≤1. PyFS is a stronger mathematical tool, and it provides decision-makers (DMs) in fuzzy set theory with greater flexibility to operate with the data at their fingertips. After PyFS was introduced, other academics sought to construct forks of it in the form of additional aggregation operators (AOs). As a result, Akram et al. [8] presented some Pythagorean Dombi fuzzy AOs. Additionally, Garg [9] design various PyF aggregation operations depending on confidence level and apply them to DM situations. Furthermore, Wang and Garg [10] have devised an algorithm for MADM that operates according to the principle of PyFS and makes use of the interactive Archimedean norm operations. The flexibility of the MAGDM was further shown by Wu et al. [11] via the use of the information fusion approach and uncertain Pythagorean fuzzy sets. PyFS is a restricted concept that has been brought to the attention of researchers because when DMs provide 0.8 as a positive grade (PG) and 0.7 as a negative grade (NG), then sum (0.82+0.72)≤∉[0,1],PyFS cannot be used, so PyFS is unable to resolve this issue as a result of the current circumstances. Yager [12] developed the idea of q-rung orthopair fuzzy set (q-ROFS) as a generalization of IFS and PyFS to reduce the difficulty associated with this task. In a recent article by Xing et al. [13], certain point-weighted AOs for q-ROFSs were shown, and their applicability to MCDM difficulties was discussed. On top of that, Liu, and Wang [14] have introduced various q-ROFS-based AOs for MADM issues. These AOs have been developed. It is important to note that the PG and NG are the only grades that are mentioned in any of the concepts, while the abstinence grade (AG) is completely disregarded, even though it is necessary to discuss the AG in many different types of real-life scenarios. This problem was brought to light by Cuong and Kreinovich [15], and they came up with a novel concept that is referred to as a picture fuzzy set (PFS) in the published works. When it comes to dealing with the ambiguity and uncertainty that MCDM issues present, PFS is a more effective tool. Note that the PFS employs the condition that the sum (μ+, μ′, μ−) ∈ [0, 1], and this feature differentiates the PFS from all other theories to make it more general. In addition, Wei [16], which is derived from PFS, discusses a few PF Hamacher AOs. If the membership grades all end up being 0.5 (positive), 0.4 (negative), or 0.2 (negative), then PFS is not a permissible formation since its primary requirement is not satisfied. This is because the formula 0.5 + 0.2 + 0.4 ∉ [0, 1] does not work in this scenario. Mahmood et al. [17] have proposed the idea of a spherical fuzzy set (SFS) as a solution to this problem to control it. Since it has been introduced, SFS has been receiving increased attention from researchers. Additionally, Deli and Cagman [18] have developed some theories for spherical fuzzy numbers as well as an approach is known as the MCDM method. In addition, some cosine similarity measures based on SFS have been presented by Raq et al. [19], along with their application to DM issues. In addition, Ashraf et al. [20] propose several symmetric-based AOs for the SF data. To deal with uncertainty, Kahraman [21] proposed the q-spherical fuzzy set. He used the idea to solve a decision-making difficulty. It is believed to be an expanded idea of what is known as a spherical fuzzy set. In q-SFS, the element is specified by three degrees: Positive (μ+), neutral (μ′), and negative (μ−) with the requirement that 0≤(μ+)q+(μ′)q+(μ−)q≤1. The opinion in this q-spherical fuzzy set is not restricted to yes or no, but also includes the option to abstain or refuse. The total of the q powers of (μ+), (μ′) and (μ−) must be less than or equal to one. Therefore, q-SFSs (q-Spherical Fuzzy Sets) provide a greater preference volume for decision makers so that they may assign their judgments on membership, non-membership, and hesitant degrees.
Rough sets (RS) are a technique initially suggested by Pawlak [22,23] for handling ambiguity. There is a mathematical resemblance between this setup and ambiguity and uncertainty. Rough set theory (RST), which is an extension of classical set theory, has as its primary instrument the relation, which is a representation of information systems. It has been observed that the equivalence relation in the Pawlak relational semantics theory is restricted in many practical fields. As a result, many authors have extended the Pawlak rough set theory by employing non-equivalence relations, and examples of similarity relations can be found in [24,25].
Although intuitionistic fuzzy sets, Pythagorean fuzzy sets, and q-rung orthopair fuzzy all have their place, they can only consider binary options like yes or no, and human opinion is never just yes or no. Take the act of voting as an example; there are four possible outcomes: voting yes, voting no, not voting at all, or abstaining from voting. Not voting at all means refusing to vote for someone and abstaining from voting means in the parliamentary process when they are there for the vote but don't cast a ballot. No currently accepted theory can account for this phenomenon. Keep in mind that the picture fuzzy set and the spherical fuzzy set can deal with these kinds of problems, despite certain limitations. The q-spherical fuzzy set, however, has shown to be the most effective framework for dealing with problems of this kind. Also, keep in mind that the current theories must overcome obstacles in their structures or conditions, as shown below.
(1) The fuzzy rough set (FRS) can be combined with RS to manage information with continuous attributes and investigate data inconsistencies. The FRS model is highly effective in many application areas because it is a powerful tool for analyzing inconsistent and ambiguous information. FRS set theory is a rough set theory extension that handles continuous numerical attributes [26]. The significance of the FRS theory may be observed in a variety of applications. Pan et al. [27] established the additive consistent fuzzy preference relation to improving the rough set model of the fuzzy preference relation. Li et al. [28] suggested a practical FRS approach for robust feature selection. Feng et al. [29] reduced multi-granulation using uncertainty measures based on FRSs, eliminating the negative and positive regions. Sun et al. [30] used a constructive technique to provide three multi-granulation FRSs over two universes. In this study by Liu [31], in the framework of interval-valued fuzzy and fuzzy probabilistic approximation space models, a decision-theoretic RS model was investigated. Zhang used axiomatic and constructive techniques to integrate rough set theory and interval-valued fuzzy set theory and [32] presented a novel paradigm based on FRSs with extended interval values. Zhang et al. [33] offered FR-based feature selection based on information entropy to minimize heterogeneous data. By combining granular variable precision FRSs and general fuzzy relations, Wang and Hu [34] provided a random set of fuzzy relationships. Vluymans et al. [35] introduced a new type of classifier for unbalanced multi-instance data based on FRS theory. Shaheen et al. [36] described the application of generalized hesitant fuzzy rough sets (GHFRS) in risk analysis. Khan et al. [37] addressed the use of a probabilistic hesitant FRS in a decision support system. Tang et al. [38] proposed the decision-theoretic rough set model with q-rung orthopair fuzzy information, as well as its application in evaluating stock investments. Liang et al. [39] suggested q-Rung orthopair fuzzy sets on decision-theoretic rough sets for three-way decisions under group DM. Zhang et al. [40] proposed group DM using incomplete q-rung orthopair fuzzy preference relations. IFRS [41], PyFRS [42], and q-ROFRS [43,44] are three popular theories that fail miserably when faced with data that includes all three possible grades (positive, neutral, and negative). Some decision-making techniques are discussed in [45,46,47,48,49].
(2) Even though the concept of the picture fuzzy rough set PFSRS allows for the consideration of the voting phenomena, it is constrained by the presence of lower and upper approximations like 0≤μ_++μ_′+μ_−≤1 and 0≤¯μ++¯μ′+¯μ−≤1. On the other hand, when information is presented to decision-makers as q-spherical fuzzy rough sets (q-SFRS), which include lower and upper approximations like {(0.7, 0.8, 0.9), (0.9, 0.7, 0.8)} etc. then, be aware that the sum of the lower and upper approximation values is beyond the range [0, 1], which means that (0≰0.72+0.82+0.92≰1)and(0≰0.92+0.72+0.82≰1) that can never be handled by SFRS and that limit the SFRS concept.
In this article, we propose a new kind of fuzzy set that we name a q-spherical fuzzy rough set. This type of fuzzy set includes the benefits that are associated with both the rough set and the q-spherical fuzzy set. Because of this, the primary contribution that this work makes to the existing body of knowledge is the development of a practical decision-making technique that is relevant to the q-spherical fuzzy rough set. Also, the innovative work has led to the proposal of novel aggregation operators, such as the q-spherical fuzzy rough weighted average mean (q-SFRWAM) and q-spherical fuzzy rough weighted geometric (q-SFRWGM). Also, up for discussion are the fundamental properties of these newly designed operators. In addition, via the use of MCDM, a deconstructive examination of the work that was done in the past as well as an illustrative example to back up our claims. We have supplied an example of the work in the form of a frame diagram, which can be seen in Figure 1. We hope that this will make it simpler for you to understand the work.
The remaining parts of the article can be broken down into the following sections: In the second section of this article, we had a look at the fundamental definitions of FS, PFS, SFS, q-SFS, and RS as well as operational laws and aggregation operators for q-SFNs, q-spherical fuzzy relation, and q-spherical fuzzy rough approximation space. In Section 3, operational laws, and aggregation operators for q-SFNs are discussed. In Section 4, both an algorithm and an example that illustrates its use are provided to demonstrate how these operators function. In Section 5, a comparison assessment is used to discuss how beneficial the planned work would be as well as how credible it will be. The concluding observations are summarized in Section 6, which is the last section.
This section investigates the concepts of fuzzy set (FS), picture fuzzy set (PFS), spherical fuzzy set (SFS), q-spherical fuzzy set (q-SFS), and rough set (RS), in addition to operational laws and aggregation operators for q-spherical fuzzy numbers (q-SFSs), q-spherical fuzzy relation, and q-spherical fuzzy rough approximation space.
Definition 2.1. Zadeh [1] came up with the idea of fuzzy set in 1965. It is a generalization of the crisp set. Mathematically, it's defined as:
A={⟨x,μA(x)⟩:x∊X} | (1) |
with the condition,
0≤μA(x)≤1. |
Where μA(x):X→[0,1] represents the membership function.
Definition 2.2. Picture fuzzy set is the generalization of fuzzy set and intuitionistic fuzzy set, which was proposed by Cuong et al. [3] in 2014. Mathematically it is defined as:
A={⟨x,μ+A(x),μ′A(x),μ−A(x)⟩:x∊X} | (2) |
with the condition,
0≤μ+A(x)+μ′A(x)+μ−A(x)≤1. |
Where μ+A(x):X→[0,1],μ′A(x):X→[0,1] and μ−A(x):X→[0,1] represents the positive, neutral, and negative membership functions respectively.
Definition 2.3. Spherical fuzzy set is the generalization of the picture fuzzy set proposed by Mehmood et al. [17] in 2019. Mathematically it is defined as:
A={⟨x,μ+A(x),μ′A(x),μ−A(x)⟩:x∊X} | (3) |
with the condition,
0≤(μ+A(x))2+(μ′A(x))2+(μ−A(x))2≤1. |
Where μ+A(x):X→[0,1],μ′A(x):X→[0,1] and μ−A(x):X→[0,1] represents the positive, neutral, and negative membership functions respectively.
Definition 2.4. q-Spherical fuzzy set is the generalization of the spherical fuzzy set proposed by Kahraman et al. [21] in 2020. Mathematically it is defined as:
Let X be a non-empty set. A q-spherical fuzzy set Aq−SFS is of the form
Aq−SFS={⟨x,μ+Aq−SFS(x),μ′Aq−SFS(x),μ−Aq−SFS(x)⟩:x∈X} | (4) |
with the condition that(0≤(μ+Aq−SFS(x))q+(μ′Aq−SFS(x))q+(μ−Aq−SFS(x))q≤1,q≥1).
Where μ+Aq−SFS(x):X→[0,1], μ′Aq−SFS(x):X→[0,1] and μ−Aq−SFS(x):X→[0,1] is the positive, neutral and negative membership functions respectively.
Definition 2.5. Pawlak [22] was the first to introduce the concept of a rough set in 1982, which is defined as:
Let R be an arbitrary binary relation on U1×U2, then the triplet (U1,U2,R) is called approximation space. For any, X⊆U1 and A⊆U2 the lower approximation R_(A) and the upper approximation ¯R(A) are defined as
(R_(A)={x∊U1:[x]A⊆X}¯R(A)={x∊U1:[x]A⋂X≠ϕ}). | (5) |
Where [x]A denote indiscernibility.
Then the pair (R_(A),¯R(A)) is called a rough set.
Definition 2.6. Basic operators
Kahraman et al. [21] 2020 defined q-spherical fuzzy set with their basic operators which are defined as:
(ⅰ) Intersection: Let Aq−SFS and Bq−SFSare two q-SF numbers, then
Aq−SFS∩Bq−SFS=⟨min{μ+Aq−SFS ,μ+Bq−SFS },max{μ′Aq−SFS ,μ′Bq−SFS },min{1−((min{μ+Aq−SFS ,μ+Bq−SFS })q+(max{μ′Aq−SFS ,μ′Bq−SFS })q,min{μ−Aq−SFS ,μ−Bq−SFS })}⟩. |
(ⅱ) Union: Let Aq−SFS and Bq−SFSare two q-SFNs, then
Aq−SFS⋃Bq−SFS=⟨max{μ+Aq−SFS , μ+Bq−SFS },min{μ′Aq−SFS , μ′Bq−SFS },max{1−((max{μ+Aq−SFS , μ+Bq−SFS })q+(min{μ′Aq−SFS , μ′Bq−SFS })q,max{μ−Aq−SFS , μ−Bq−SFS })}⟩. |
(ⅲ) Addition: Let Aq−SFS and Bq−SFSare two q-SFNs, then
Aq−SFS⊕Bq−SFS= |
⟨((μ+Aq−SFS )q+(μ+Bq−SFS )q−(μ+Aq−SFS )q(μ+Bq−SFS )q)1q,(μ′Aq−SFS )q(μ′Bq−SFS )q,((1−(μ+Bq−SFS )q)(μ−Aq−SFS )q+(1−(μ+Aq−SFS )q)(μ−Bq−SFS )q)−(μ−Aq−SFS )q(μ−Bq−SFS )q⟩. |
(ⅳ) Multiplication: Let Aq−SFS and Bq−SFSare two q-SFNs, then
Aq−SFS⊗Bq−SFS |
=⟨(μ+Aq−SFS )q(μ+Bq−SFS )q,(μ′Aq−SFS )q+(μ′Bq−SFS )q−(μ′Aq−SFS )(μ′Bq−SFS ),((1−(μ′Bq−SFS )q)(μ−Aq−SFS )q+(1−(μ′Aq−SFS )q)(μ−Bq−SFS )q)−(μ−Aq−SFS )q(μ−Bq−SFS )q⟩. |
(ⅴ) Multiplication by a scaler: For λ>0
λAq−SFS=⟨(1−(1−(μ+Aq−SFS )q)λ)1q,(μ′Aq−SFS )λ,[(1−(μ+Aq−SFS )q)λ−(1−(μ+Aq−SFS )q−(μ−Aq−SFS )q)λ]1q⟩. |
(ⅵ) λλ Power of Aq−SFS;λλ > 0
Aq−SFSλ=⟨(μ+Aq−SFS )λ,(1−(1−(μ′Aq−SFS )q)λ)1q,[(1−(μ′Aq−SFS )q)λ−(1−(μ′Aq−SFS )q−(μ−Aq−SFS )q)λ]1q⟩. |
Definition 2.7. For two q-SFNs
Aq−SFS=⟨μ+Aq−SFS , μ′Aq−SFS , μ−Aq−SFS ⟩ and Bq−SFS=⟨μ+Bq−SFS , μ′Bq−SFS , μ−Bq−SFS ⟩, the following are valid under the condition λ,λ1,λ2 > 0
(i)Aq−SFS⊕Bq−SFS=Bq−SFS⊕Aq−SFS.
(ii)Aq−SFS⊗Bq−SFS=Bq−SFS⊗Aq−SFS.
(iii)λ(Aq−SFS⊕Bq−SFS)=λBq−SFS⊕λAq−SFS.
(iv)λ1(Aq−SFS)⊕λ2(Aq−SFS)=(λ1⊕λ2)Aq−SFS.
(v)(Aq−SFS⊗Bq−SFS)λ=Aq−SFSλ⊗Bq−SFSλ.
(vi)Aq−SFSλ1⊗Aq−SFSλ2=Aq−SFSλ1+λ2.
Definition 2.8. q-Spherical Fuzzy Weighted Arithmetic Operator (q-SFRWAO) for w=(w1,w2,w3,…,wn); wi∈ [0, 1]; ∑ni=1wi=1, q-SFWAO is defined as
q−SFWAOw(Aq−SFS1,Aq−SFS2,Aq−SFS3,…,Aq−SFSn) =w1Aq−SFS1⊕w2Aq−SFS2⊕w3Aq−SFS3⊕,…,⊕wnAq−SFSn |
=⟨[1−∏ni=1(1−(μ+Aq−SFRSi )q)wi]1q,∏ni=1(μ′Aq−SFRSi )wi,[∏ni=1(1−(μ+Aq−SFRSi )q)wi−∏ni=1(1−(μ+Aq−SFRSi )q−(μ−Aq−SFRSi )q)wi]1q⟩. |
Definition 2.9. q-Spherical Fuzzy Weighted Geometric Operator (q-SWGO) for w=(w1,w2,w3,…,wn); wi∈ [0, 1]; ∑ni=1wi=1, q-SFWGO is defined as
q−SFWGOw(Aq−SFS1,Aq−SFS2,Aq−SFS3,…,Aq−SFSn) =w1Aq−SFS1⊗w2Aq−SFS2⊗w3Aq−SFS3⊗,…,⊗wnAq−SFSn |
=⟨∏ni=1(μ+Aq−SFRSi )wi,[1−∏ni=1(1−(μ′Aq−SFRSi )q)wi]1q,[∏ni=1(1−(μ′Aq−SFRSi )q)wi−∏ni=1(1−(μ′Aq−SFRSi )q−(μ−Aq−SFRSi )q)wi]1q⟩. |
Definition 2.10. A q-spherical fuzzy relation R in U1×U2 is a q-spherical fuzzy subset of U1×U2 and is given by
R={⟨(r, s):μ+R(r,s),μ′R(r,s),μ−R(r,s)⟩:((μ+R(r,s),)q+(μ′R(r,s),)q+(μ−R(r,s))q) ≤1:∀r∈U1,s∈U2}, |
where μ+R:U→[0,1], μ′R:U→[0,1] and μ−R:U→[0,1].
The degree of refusal s∈U is defined by r(s)=q√1−(μ′(s)q+μ′(s)q+μ−(s)q).
Definition 2.11. Let R be a q-spherical fuzzy relation R on two universes, then we call the triplet (U1,U2,R) q-spherical fuzzy rough approximation space.
Definition 2.12. Let A⊆U2, then the lower and upper approximation of A for (U1,U2,R) is defined by
Aq−SFRS=(A_q−SFRS , −Aq−SFRS={s,⟨μ+A_q−SFRS (s),μ′A_q−SFRS (s),μ−A_q−SFRS (s),μ+−Aq−SFRS (s),μ′−Aq−SFRS (s),μ−−Aq−SFRS (s)⟩:s∈U1}, |
where,
μ+A_q−SFRS (s)=⋀s∈U2{μ+R(r,s)⋀μ+A(s)} |
μ′A_q−SFRS (s)=⋁s∈U2{μ′R(r,s)⋁μ′A(s)} |
μ−A_q−SFRS (s)=⋁s∈U2{μ−R(r,s)⋁μ−A(s)} |
μ+−Aq−SFRS (s)=⋁s∈U2{μ+R(r,s)⋁μ+A(s)} |
μ′−Aq−SFRS (s)=⋀s∈U2{μ′R(r,s)⋀μ′A(s)} |
μ−−Aq−SFRS (s)=⋀s∈U2{μ−R(r,s)⋀μ−A(s)}, |
with the condition that
{(0≤(μ+A_q−SFRS (s))q+(μ′A_q−SFRS (s))q+(μ−A_q−SFRS (s))q≤1),(0≤(μ+−Aq−SFRS (s))q+(μ′−Aq−SFRS (s))q+(μ−−Aq−SFRS (s))q≤1)}. |
The pair of q-spherical fuzzy sets is then said to represent a q-spherical fuzzy rough set (q-SFRS) if A_q−SFRS≠−Aq−SFRS. For simplicity, we write A=(A_q−SFRS , −Aq−SFRS ) and the expression A=(A_q−SFRS , −Aq−SFRS ) is called a q-spherical fuzzy rough number. Aq−SFRSidenotes the collection of all q-SFRNs.
Example 1. Let's say that a person in charge of making decisions, Z, purchases a home, as shown in the set U1={h1,h2,h3,h4,h5} that are now being considered.
Let 𝑈2 = {beautiful (a1), size (a2), expensive (a3) 𝑎𝑛𝑑 location (a4)} is the set of alternatives.
A person who makes decisions Z would want to buy a home from among those that are currently on the market and which, to the greatest degree possible, satisfy the requirements that have been outlined.
Consider the fact that decision-maker Z displays the attractiveness of the homes in the form of a q-SFR relation, which can be found in Table 1 below.
R | a1 | a2 | a3 | a4 |
h1 | (0.7, 0.2, 0.4) | (0.5, 0.2, 0.7) | (0.6, 0.5, 0.3) | (0.7, 0.2, 0.5) |
h2 | (0.6, 0.3, 0.1) | (0.3, 0.2, 0.5) | (0.3, 0.2, 0.4) | (0.5, 0.2, 0.5) |
h3 | (0.3, 0.1, 0.2) | (0.7, 0.4, 0.3) | (0.2, 0.3, 0.7) | (0.3, 0.4, 0.1) |
h4 | (0.4, 0.5, 0.2) | (0.8, 0.4, 0.5) | (0.5, 0.3, 0.4) | (0.4, 0.3, 0.2) |
h5 | (0.6, 0.1, 0.5) | (0.4, 0.3, 0.5) | (0.9, 0.3, 0.5) | (0.3, 0.2, 0.1) |
Consider a decision-maker Z that provides the optimal normal decision object A, which is a q-SFS subset over the attribute set U2; that is to say,
A=(a1/(0.8,0.1,0.3)),(a2/(0.8,0.4,0.3)),(a3/(0.5,0.1,0.4)),(a4/(0.5,0.4,0.6)). |
In this case, we use Definition 3.4 to determine the lower and upper approximations of A approximations regarding (U1, U2, R, A).
A_q−SFR={(h1/(0.5,0.5,0.7)),(h2/(0.3,0.4,0.6)),(h3/(0.2,0.4,0.7)),(h4/(0.4,0.5,0.6)),(h5/(0.3,0.4,0.6))}.ˉAq−SFRS={(h1/(0.8,0.1,0.3)),(h2/(0.8,0.1,0.1)),(h3/(0.8,0.1,0.1)),(h4/(0.8,0.1,0.2)),(h5/(0.9,0.1,0.1))}. Hence Aq−SFRS={(h1/(0.5,0.5,0.7),(0.8,0.1,0.3)),(h2/(0.3,0.4,0.6),(0.8,0.1,0.1)),(h3/(0.2,0.4,0.7),(0.8,0.1,0.1)),(h4/(0.4,0.5,0.6),(0.8,0.1,0.2)),(h5/(0.3,0.4,0.6),(0.9,0.1,0.1))}. |
This section examines the basic operational laws and aggregation operators of q-spherical fuzzy rough numbers.
Definition 3.1. Basic operators
(ⅰ) Intersection: Let Aq−SFRS and Bq−SFRSare two q-SFRNs in (U1, U2, R), then
Aq−SFRS∩Bq−SFRS=A_q−SFRS∩B_q−SFRS , −Aq−SFRS∩−Bq−SFRS where, |
A_q−SFRS∩B_q−SFRS=⟨min{μ+A_q−SFSR , μ+B_q−SFRS },max{μ′A_q−SFRS , μ′B_q−SFRS },min{1−((min{μ+A_q−SFSR , μ+B_q−SFRS })q+(max{μ′A_q−SFSR , μ′B_q−SFRS })q,min{μ−A_q−SFSR , μ−B_q−SFRS })}⟩. |
−Aq−SFRS∩−Bq−SFRS=⟨min{μ+−Aq−SFRS , μ+−Bq−SFRS },max{μ′−Aq−SFRS , μ′−Bq−SFRS },min{1−((min{μ+−Aq−SFRS , μ+−Bq−SFRS })q+(max{μ′−Aq−SFRS , μ′−Bq−SFRS })q,min{μ−−Aq−SFRS , μ−−Bq−SFRS })}⟩. |
(ⅱ) Union: Let Aq−SFRS and Bq−SFRSare two q-SFRNs in (U1, U2, R), then
Aq−SFRS⋃Bq−SFRS=A_q−SFRS⋃B_q−SFRS , −Aq−SFRS⋃−Bq−SFRS where, |
A_q−SFRS⋃B_q−SFSR=⟨max{μ+A_q−SFRS , μ+B_q−SFRS },min{μ′A_q−SFRS , μ′B_q−SFRS },max{1−((max{μ+A_q−SFSR , μ+B_q−SFRS })q+(min{μ′A_q−SFSR , μ′B_q−SFRS })q,max{μ−A_q−SFSR , μ−B_q−SFRS })}⟩. |
−Aq−SFRS⋃−Bq−SFRS=⟨max{μ+−Aq−SFRS , μ+−Bq−SFRS },min{μ′−Aq−SFRS , μ′−Bq−SFRS },max{1−((max{μ+−Aq−SFRS , μ+−Bq−SFRS })q+(min{μ′−Aq−SFRS , μ′−Bq−SFRS })q,max{μ−−Aq−SFRS , μ−−Bq−SFRS })}⟩. |
(ⅲ) Addition: Let Aq−SFS and Bq−SFSare two q-SFRNs in (U1, U2, R), then
Aq−SFRS⊕Bq−SFRS=A_q−SFRS⊕B_q−SFRS , −Aq−SFRS⊕−Bq−SFRS where, |
A_q−SFRS⊕B_q−SFRS=⟨((μ+A_q−SFRS )q+(μ+B_q−SFRS )q−(μ+A_q−SFRS )q(μ+B_q−SFRS )q)1q,(μ′A_q−SFRS )q(μ′B_q−SFRS )q,((1−(μ+B_q−SFRS )q)(μ−A_q−SFRS )q+(1−(μ+A_q−SFRS )q)(μ−B_q−SFRS )q)−(μ−A_q−SFRS )q(μ−B_q−SFRS )q⟩. |
−Aq−SFRS⊕−Bq−SFRS=⟨((μ+−Aq−SFRS )q+(μ+−Bq−SFRS )q−(μ+−Aq−SFRS )q(μ+−Bq−SFRS )q)1q,(μ′−Aq−SFRS )q(μ′−Bq−SFRS )q,((1−(μ+−Bq−SFRS )q)(μ−−Aq−SFRS )q+(1−(μ+−Aq−SFRS )q)(μ−−Bq−SFRS )q)−(μ−−Aq−SFRS )q(μ−−Bq−SFRS )q⟩. |
(ⅳ) Multiplication: Let Aq−SFRS and Bq−SFRSare two q-SFRNs in (U1, U2, R), then
Aq−SFR⊗Bq−SFR=A_q−SFR⊗B_q−SFR,ˉAq−SFR⊗ˉBq−SFR, where, |
A_q−SFRS⊗B_q−SFRS=⟨(μ+A_q−SFRS )q(μ+B_q−SFRS )q,(μ′A_q−SFRS )q+(μ′B_q−SFRS )q−(μ′A_q−SFRS )q(μ′B_q−SFRS )q,((1−(μ′B_q−SFRS )q)(μ−A_q−SFRS )q+(1−(μ′A_q−SFRS )q)(μ−B_q−SFRS )q)−(μ−A_q−SFRS )q(μ−B_q−SFRS )q⟩. |
−Aq−SFRS⊗−Bq−SFRS=⟨(μ+−Aq−SFRS )q(μ+−Bq−SFRS )q,(μ′−Aq−SFRS )q+(μ′−Bq−SFRS )q−(μ′−Aq−SFRS )q(μ′−Bq−SFRS )q,((1−(μ′−Bq−SFRS )q)(μ−−Aq−SFRS )q+(1−(μ′−Aq−SFRS )q)(μ−−Bq−SFRS )q)−(μ−−Aq−SFRS )q(μ−−Bq−SFRS )q⟩. |
(ⅴ) Multiplication by a scaler: Let Aq−SFRS be a q-SFRNs in (U1, U2, R) and λ>0 then
λAq−SFRS=λA_q−SFRS , λ−Aq−SFRS where,
λA_q−SFRS=⟨(1−(1−(μ+A_q−SFRS )q)λ)1q,(μ′A_q−SFRS )λ,[(1−(μ+A_q−SFRS )q)λ−(1−(μ+A_q−SFRS )q−(μ−A_q−SFRS )q)λ]1q⟩. |
λ−Aq−SFRS=⟨(1−(1−(μ+−Aq−SFRS )q)λ)1q,(μ′−Aq−SFRS )λ,[(1−(μ+−Aq−SFRS )q)λ−(1−(μ+−Aq−SFRS )q−(μ−−Aq−SFRS )q)λ]1q⟩. |
(ⅵ) λλ Power of −Aq−SFS:Let Aq−SFRS be a q-SFRNs in (U1, U2, R) and λ>0 then
Aq−SFRSλ=A_q−SFRSλ,−Aq−SFRSλ where,
A_q−SFRSλ=⟨(μ+A_q−SFRS )λ,(1−(1−(μ′A_q−SFRS )q)λ)1q,[(1−(μ′A_q−SFRS )q)λ−(1−(μ′A_q−SFRS )q−(μ−A_q−SFRS )q)λ]1q⟩. |
−Aq−SFRSλ=⟨(μ+−Aq−SFRS )λ,(1−(1−(μ′−Aq−SFRS )q)λ)1q,[(1−(μ′−Aq−SFRS )q)λ−(1−(μ′−Aq−SFRS )q−(μ−−Aq−SFRS )q)λ]1q⟩. |
Definition 3.2. For two q-SFRNs
Aq−SFRS=⟨μ+A_q−SFRS , μ′A_q−SFRS , μ−A_q−SFRS , μ+−Aq−SFRS , μ′−Aq−SFRS , μ−−Aq−SFRS⟩ and Bq−SFRS=⟨μ+B_q−SFRS , μ′B_q−SFRS , μ−B_q−SFRS , μ+−Bq−SFRS , μ′−Bq−SFRS , μ−−Bq−SFRS⟩, the following are valid under the condition λ,λ1,λ2>0
(i)Aq−SFRS⊕Bq−SFRS=Bq−SFRS⊕Aq−SFRS
(ii)Aq−SFRS⊗Bq−SFRS=Bq−SFRS⊗Aq−SFRS
(iii)λ(Aq−SFRS⊕Bq−SFRS )=λAq−SFRS⊕λBq−SFRS
(iv)λ1(Aq−SFRS)⊕λ2(Aq−SFRS )=(λ1⊕λ2)Aq−SFRS
(v)(Aq−SFRS⊗Bq−SFRS )λ=Aq−SFRSλ⊗Bq−SFRSλ
(vi)Aq−SFRSλ1⊗Aq−SFRSλ2=Aq−SFRSλ1+λ2.
Definition 3.3. q-Spherical Fuzzy Rough Arithmetic Mean (q-SFRAM) with respect to, w=(w1,w2,w3,…,wn); wi∈ [0, 1]; ∑ni=1wi=1, q-SFRAM is defined as
q−SFRAMw(Aq−SFRS1,Aq−SFRS2,Aq−SFRS3,…,Aq−SFRSn) =w1Aq−SFRS1⊕w2Aq−SFRS2⊕w3Aq−SFRS3⊕,…,⊕wnAq−SFRSn |
=⟨[1−∏ni=1(1−(μ+A_q−SFRSi )q)wi]1q,∏ni=1(μ′A_q−SFRSi )wi,[∏ni=1(1−(μ+A_q−SFRSi )q)wi−∏ni=1(1−(μ+A_q−SFRSi )q−(μ−A_q−SFRSi )q)wi]1q,[1−∏ni=1(1−(μ+−Aq−SFRSi )q)wi]1q,∏ni=1(μ′−Aq−SFRSi )wi,[∏ni=1(1−(μ+−Aq−SFRSi )q)wi−∏ni=1(1−(μ+−Aq−SFRSi )q−(μ−−Aq−SFRSi )q)wi]1q⟩. |
Definition 3.4: q-Spherical Fuzzy Rough Geometric Mean (q-SFRGM) for w=(w1,w2,w3,…,wn); wi∈ [0, 1]; ∑ni=1wi=1, q-SFRGM is defined as
q−SFRGM(Aq−SFRS1,Aq−SFRS2,Aq−SFRS3,…,Aq−SFRSn) =w1Aq−SFRS1⊗w2Aq−SFRS2⊗w3Aq−SFRS3⊗,…,⊗wnAq−SFRSn |
=⟨∏ni=1(μ+A_q−SFRSi )wi,[1−∏ni=1(1−(μ′A_q−SFRSi )q)wi]1q,[∏ni=1(1−(μ′A_q−SFRSi )q)wi−∏ni=1(1−(μ′A_q−SFRSi )q−(μ−A_q−SFRSi )q)wi]1q,∏nI=1(μ+−Aq−SFRSi )wi,[1−∏ni=1(1−(μ′−Aq−SFRSi )q)wi]1q,[∏ni=1(1−(μ′−Aq−SFRSi )q)wi−∏ni=1(1−(μ′−Aq−SFRSi )q−(μ−−Aq−SFRSi )q)wi]1q⟩. |
Proof. The proof of this definition is like the proof of Definition 3.3, so we omit it here.
Definition 3.5. The score function of sorting q-SFRNs is defined by
Score(Aq−SFRS)=13(2+(μ+A_q−SFRS )q+(μ+−Aq−SFRS )q−(μ′A_q−SFRS )q−(μ′−Aq−SFRS )q−(μ−A_q−SFRS )q−(μ−−Aq−SFRS )q),Score(Aq−SFRS)∈[−1,1],q≥1. |
Note that if Aq−SFRS<Bq−SFRS if and only if
Score(Aq−SFRS)<Score(Bq−SFRS) or |
Score(Aq−SFRS)=Score(Bq−SFRS) and Accuracy(Aq−SFRS)<Accuracy(Bq−SFRS). |
Example 2. Let Aq−SFRS1=⟨(0.6,0.4,0.3),(0.5,0.3,0.2)⟩, Aq−SFRS2=⟨(0.8,0.7,0.2),(0.4,0.6,0.4)⟩, Aq−SFRS3=⟨(0.6,0.4,0.3),(0.5,0.3,0.2)⟩ and Aq−SFRS4=⟨(0.5,0.4,0.3),(0.5,0.3,0.2)⟩ are four q-SFRNs, w=(0.2,0.1,0.3,0.4)T is their associate weight vector and q=3. Then
[1−∏4i=1(1−(μ+A_q−SFRSi )q)wi]1q=[1−(1−(0.6)3)0.2(1−(0.8)3)0.1(1−(0.6)3)0.3(1−(0.5)3)0.4]13=0.6025. |
∏4i=1(μ′A_q−SFRSi)wi=(0.4)0.2(0.7)0.1(0.4)0.3(0.4)0.4=0.0757. |
[∏4i=1(1−(μ+A_q−SFRSi )q)wi−∏4i=1(1−(μ+A_q−SFRSi )q−(μ−A_q−SFRSi )q)wi]1q=[((1−(0.6)3)0.2(1−(0.8)3)0.1(1−(0.6)3)0.3(1−(0.5)3)0.4)−((1−(0.6)3−(0.3)3)0.2(1−(0.8)3−(0.2)3)0.1(1−(0.6)3−(0.3)3)0.3(1−(0.5)3−(0.3)3)0.4)]13=0.2900. |
[1−4∏i=1(1−(μ+−Aq−SFRSi)q)wi]1q =[1−(1−(0.5)3)0.2(1−(0.4)3)0.1(1−(0.5)3)0.3(1−(0.5)3)0.4]13=0.4920 |
∏4i=1(μ′−Aq−SFRSi)wi=(0.3)0.2(0.6)0.1(0.3)0.3(0.3)0.4=0.0332. |
[∏4i=1(1−(μ+−Aq−SFRSi )q)wi−∏4i=1(1−(μ+−Aq−SFRSi )q−(μ−−Aq−SFRSi )q)wi]1q=[((1−(0.5)3)0.2(1−(0.4)3)0.1(1−(0.5)3)0.3(1−(0.5)3)0.4)−((1−(0.5)3−(0.2)3)0.2(1−(0.4)3−(0.4)3)0.1(1−(0.5)3−(0.2)3)0.3(1−(0.5)3−(0.2)3)0.4)]13=0.2376. |
Example 3. Let Aq−SFRS1=⟨(0.6,0.4,0.3),(0.5,0.3,0.2)⟩, Aq−SFRS2=⟨(0.8,0.7,0.2),(0.4,0.6,0.4)⟩, Aq−SFRS3=⟨(0.7,0.4,0.3),(0.6,0.3,0.2)⟩ and Aq−SFRS4=⟨(0.5,0.4,0.3),(0.5,0.3,0.2)⟩ are four q-SFRNs and q=3 then the score function is calculated as follows:
Score(Aq−SFRS1)=13(2+(0.6)3+(0.5)3−(0.4)3−(0.3)3−(0.3)3−(0.2)3) |
Score(Aq−SFRS1)=0.7383. |
Score(Aq−SFRS2)=13(2+(0.8)3+(0.4)3−(0.7)3−(0.6)3−(0.2)3−(0.4)3) |
Score(Aq−SFRS2)=0.6483. |
Score(Aq−SFRS3)=13(2+(0.7)3+(0.6)3−(0.4)3−(0.3)3−(0.3)3−(0.2)3) |
Score(Aq−SFRS3)=0.8110. |
Score(Aq−SFRS4)=13(2+(0.5)3+(0.5)3−(0.4)3−(0.3)3−(0.3)3−(0.2)3) |
Score(Aq−SFRS4)=0.7080. |
In this part, we will investigate a novel approach for MADM problem solving that makes use of q-SFRWAM and q-SFRWGM aggregation operators to operate in an environment that contains q-SFR information.
Let's say that the set of "r" alternatives is denoted by A={A1,A2,A3,…,Ar} and that the set of "n" parameters is denoted by C={C1,C2,C3,…,Cn}. Let us refer to the group of "m" experts as E={E1,E2,E3,…,Em} and let us assume that these "m" experts share their knowledge on "A" Ai{i=1,2,3,…,r}. Let us designate the weight vector of Ei experts as W={W1,W2,W3,…,Wn} with the condition that ∑ni=1Wi=1.
Let's say that the evaluation data that was provided by the intellectual was in the form of q-SFRNs. All individual contributes their opinion in the form of q-SFRNs for each possible alternative Ai. After that, organize the information on the comprehensive assessment in the q-SFR decision matrix M=[Aq−SFRSij]n×m. The MCDM approach includes the following as its primary phases, which are based on the established operators:
Step 1: (Construction of q-SFRNs decision-making matrix): Gather all the information that is associated with each option in terms of q-SFRNs, and as a result, create an overall decision matrix M=[Aq−SFRSij]n×m.
Step 2: (Normalize the q-SFR decision matrix): Normalize the q-SFR decision matrix that was provided in Step 1. If all the criteria have the same type, then there is no need for normalizing; however, if there are multiple kinds of criteria, such as profit and cost, then we transform the cost type criterion into the profit type using the normalized formula that is shown below:
Aq−SFRSij={(Aq−SFRSij)C for cost type criteria(Aq−SFRSij) for benefit type criteria | (6) |
where,
(Aq−SFRSij)C={s,⟨μ−A_q−SFRSij (s),μ′A_q−SFRSij (s),μ+A_q−SFRSij (s),μ−−Aq−SFRSij (s),μ′−Aq−SFRSij (s),μ+−Aq−SFRSij (s)⟩:s∈U1}, |
denote the complement of
Aq−SFRSi={s,⟨μ+A_q−SFRSij (s),μ′A_q−SFRSij (s),μ−A_q−SFRSij (s),μ+−Aq−SFRSij (s),μ′−Aq−SFRSij (s),μ−−Aq−SFRSij (s)⟩:s∈U1}. |
As a result, we can obtain the normalized form of the q-SFR decision matrix
N=[Bq−SFRSij]n×m. |
Step 3: (Compute the aggregated values of alternatives): To each decision matrix N=[Bq−SFRSij]n×mand apply the established operators for each alternative Ai{i=1,2,3,…,r} and calculate the aggregated results
¥i=⟨(μ−A_q−SFRS , μ′A_q−SFRS , μ+A_q−SFRS ),(μ−−Aq−SFRS , μ′−Aq−SFRS , μ+−Aq−SFRS )⟩. |
Step 4: (Computation of score values): To get the score values for each ¥i, use the Definition 3.5 formula.
Step 5: (Ranking of alternatives): Rank the results and choose the best one.
In this part of the article, we illustrated the applicability of the model that was provided by providing a concrete example. The technique by which an operating system distributes available resources between the many tasks is known as job scheduling. The jobs in the job queue are ordered by the job scheduler, and the system gives more processing power to the jobs that are higher on the priority list inside the queue. The process of job scheduling ensures that all jobs are finished in a timely and equitable manner and that no jobs are left without enough CPU allocation. Most operating systems, such as UNIX, Windows, iOS, and Android, among others, come equipped with basic job-scheduling capabilities. In addition to this, a variety of applications, such as Database Management Systems (DBMS), Enterprise Resource Planning (ERP), and Business Process Management (BPM), are equipped with specialized job-scheduling features as well. Suppose a programmer needs to schedule jobs A1, A2, A3, and A4 following the criteria C1, C2, and C3, where C1 stands for automated restart in the event of a failure, C2 stands for the number of parallel jobs that are authorized for a user, and C3 stands for the execution time that is allotted to a user. The weight vector was used to determine the order of importance for each of these tasks w=(0.20,0.38,0.42)T.
Step 1: The data that the decision maker has gathered for the evaluation of the alternativesAi are provided in the form of q-SFRNs as shown in Table 1. Now we put the plan into action by selecting the most suitable alternative Ai. Table 2 represents the preferences of decision makers in the form of q-SFRNs for each job.
C1 | C2 | C3 | |
A1 | ⟨(0.8,0.4,0.3),(0.7,0.5,0.2)⟩ | ⟨(0.7,0.4,0.2),(0.6,0.3,0.4)⟩ | ⟨(0.7,0.3,0.3),(0.8,0.4,0.4)⟩ |
A2 | ⟨(0.9,0.2,0.1),(0.8,0.3,0.2)⟩ | ⟨(0.8,0.2,0.3),(0.5,0.4,0.1)⟩ | ⟨(0.8,0.4,0.2),(0.9,0.3,0.2)⟩ |
A3 | ⟨(0.8,0.4,0.3),(0.9,0.5,0.1)⟩ | ⟨(0.8,0.4,0.3),(0.7,0.3,0.2)⟩ | ⟨(0.8,0.4,0.3),(0.4,0.4,0.2)⟩ |
A4 | ⟨(0.7,0.4,0.5),(0.7,0.6,0.4)⟩ | ⟨(0.9,0.4,0.3),(0.7,0.2,0.1)⟩ | ⟨(0.7,0.3,0.3),(0.6,0.1,0.2)⟩ |
Step 2: Since C1 and C3 belong to the cost type of criterion, it is possible to normalize them by applying Eq (6), and as a result, we get the normalized decision matrix N, which is shown in Table 3. Table 3 represents the values of decision-making data that are normalized and presented in the form of q-SFRNs.
C1 | C2 | C3 | |
A1 | ⟨(0.3,0.4,0.8),(0.2,0.5,0.7)⟩ | ⟨(0.7,0.4,0.2),(0.6,0.3,0.4)⟩ | ⟨(0.3,0.3,0.7),(0.4,0.4,0.8)⟩ |
A2 | ⟨(0.1,0.2,0.9),(0.2,0.3,0.8)⟩ | ⟨(0.8,0.2,0.3),(0.5,0.4,0.1)⟩ | ⟨(0.2,0.4,0.8,),(0.2,0.3,0.9)⟩ |
A3 | ⟨(0.3,0.4,0.8),(0.1,0.5,0.9)⟩ | ⟨(0.8,0.4,0.3),(0.7,0.3,0.2)⟩ | ⟨(0.3,0.4,0.8),(0.2,0.4,0.4)⟩ |
A4 | ⟨(0.5,0.4,0.7),(0.4,0.6,0.7)⟩ | ⟨(0.9,0.4,0.3),(0.7,0.2,0.1)⟩ | ⟨(0.3,0.3,0.7),(0.2,0.1,0.6)⟩ |
Step 3: Apply the q-SFRAM aggregation operators developed for normalized decision matrix N=[Bq−SFRSij]n×m to each alternative Ai to get the aggregated result
¥i=⟨(μ+A_q−SFRS , μ′A_q−SFRS , μ−A_q−SFRS )(μ+−Aq−SFRS , μ′−Aq−SFRS , μ−−Aq−SFRS )⟩. |
¥1=⟨(0.5450,0.3545,0.6211),(0.4859,0.3749,0.6845)⟩ |
¥2=⟨(0.6226,0.2676,0.6961),(0.3784,0.3347,0.7817)⟩ |
¥3=⟨(0.6312,0.4000,0.6601),(0.5320,0.3749,0.6005)⟩ |
¥4=⟨(0.7453,0.3545,0.5444),(0.5447,0.1862,0.5296)⟩. |
Step 4: Compute the score values for each ¥i, use Definition 3.5.
Score(¥1)=0.5397Score(¥2)=0.4746Score(¥3)=0.5937Score(¥4)=0.7382. |
Step 5: Rank each alternative in order of preference ¥i{i=1,2,3,…,r}.
Score(¥4)>Score(¥3)>Score(¥1)>Score(¥2). |
According to these score values, the tasks on the timetable that were taken into consideration should be ranked as follows: A4 > A3 > A1 > A2.
As a result, we have concluded that A4 should be scheduled first.
On the other hand, if we aggregate the data that has been provided by utilizing the q- SFRGM operator, then the steps that have been taken are as follows:
Step 1: All of the information related to each alternative is compiled and presented in the form of q- SFRNs in Table 2.
Step 2: The examined problem's normalized data are shown in Table 3.
Step 3: To get the total performance value of each alternative, indicated by ¥i, we may use the q-SFRGM operator as provided in Definition 3.4 to aggregate the ith row.
¥1=⟨(0.4140,0.3650,0.6519),(0.4062,0.3975,0.7009)⟩ |
¥2=⟨(0.2949,0.3172,0.7614),(0.2833,0.3455,0.7922)⟩ |
¥3=⟨(0.4355,0.4000,0.7176),(0.2803,0.3975,0.6719)⟩ |
¥4=⟨(0.5044,0.3650,0.6179),(0.3698,0.3704,0.5706)⟩. |
Step 4: The score values for these alternatives are
Score(¥1)=0.4684Score(¥2)=0.3455Score(¥3)=0.4350Score(¥4)=0.5526. |
Step 5: As a result of this, the alternative with the highest rating is A4, followed by A1 and then A3, while the task with the lowest ranking is A2. i.e., A4 > A1 > A3 > A2.
The data that was shown previously makes it quite easy for us to conclude that while different methodologies may yield different orderings of alternatives, the ones that are the best stay the same. The q-SFAM and q-SFGM operators, on the other hand, do not consider the interrelationships that exist between fused arguments.
In 2020, Kahraman et al. [21] defined operational laws for q-spherical fuzzy sets, and their aggregation operators and applied the proposed operators to decision-making problems to find the best solution. The q-spherical fuzzy rough set is presented here as part of the work that we have suggested. Also developed in detail are the operational laws for the q-spherical fuzzy rough numbers, aggregation operators (q-SFRAM and q-SFRGM), and the properties of those operators. Further application of the suggested aggregation operators in the issue of decision-making led to the selection of the most effective station for analyzing the job scheduling. From what has been shown so far, it should be clear that q-spherical fuzzy rough information job scheduling offers a wide range of potential applications. Because uncertainty can be effectively measured in terms of q-SFR numbers and because this provides improved results in fuzzy mathematics, the preference values are treated as q-SFR numbers rather than an exact numbers in the work that we have proposed. This is because uncertainty can be effectively measured in terms of q-SFR numbers. Because of this, the technique that is being offered is both innovative and effective. Therefore, the multiple attribute group decision-making models developed in this study are more generalizable and adaptable than the existing multiple attribute group decision-making models under a q-spherical fuzzy rough environment, making them applicable in a wider range of settings where the attribute group decision-making (MADM) procedure is used.
Methods | Score values | Ranking Order |
IFWA [50] | (0.22016,0.33214,0.21173,0.27007) | S2≻S4≻S1≻S3 |
IFWG [50] | (0.46119,0.5572,0.49917,0.55418) | S2≻S4≻S3≻S1 |
PFSWA [51] | (0.52209,0.62190,0.56596,0.59380) | S2≻S4≻S3≻S1 |
PFSWG [51] | (0.46427,0.57343,0.55374,0.51474) | S2≻S3≻S4≻S1 |
q - ROFWA [52] | (0.09958,0.14761,0.07984,0.08157) | S2≻S1≻S4≻S3 |
q - ROFWG [52] | (0.29865,0.40678,0.35021,0.38045) | S2≻S1≻S4≻S3 |
q - ROFStRWA [53] | (0.226074,0.298025,0.241341) | S2≻S3≻S1 |
q - ROFStRWG [53] | (0.06616,0.135502,0.118744) | S2≻S3≻S1 |
SFStRWA [54] | (0.4477,0.5337,0.5188) | S2≻S3≻S1 |
SFStROWA [54] | (0.4317,0.5412,0.4977) | S2≻S3≻S1 |
SFStRHA [54] | (0.4454,0.5202,0.4711) | S2≻S3≻S1 |
q - SFRWA (proposed) | (0.5397,0.4746,0.5937,0.7382) | S4≻S3≻S1≻S2 |
q - SFRWG (proposed) | (0.4684,0.3455,0.4350,0.5526) | S4≻S1≻S3≻S2 |
Approches | Fuzzy information | Parameters information | Lower and upper approximation information |
IFWA [50] | √ | × | × |
IFWG [50] | √ | × | × |
PFSWA [51] | √ | √ | × |
PFSWG [51] | √ | √ | × |
q - ROFWA [52] | √ | × | × |
q - ROFWG [52] | √ | × | × |
q - ROFStRWA [53] | √ | √ | √ |
q - ROFStRWG [53] | √ | √ | √ |
SFStRWA [54] | √ | √ | √ |
SFStROWA [54] | √ | √ | √ |
SFStRHA [54] | √ | √ | √ |
q - SFRWA (proposed) | √ | √ | √ |
q - SFRWG (proposed) | √ | √ | √ |
In this article, we developed a hybrid notion called q-spherical fuzzy rough set, by combining the concepts of q-spherical fuzzy set and rough set, where there are three independent parameters with lower and upper set approximations. We define basic operational laws such as intersection, union, addition, multiplication, and scaler multiplication. We plan to develop new aggregation operators such as q-spherical fuzzy rough weighted arithmetic mean (q-SFRWAM) and q-spherical fuzzy rough weighted geometric mean (q-SFRWGM) operators. For application, we construct an example to show the validity of the developed work. In the end, we established a comparison analysis to show the advantages of the proposed operators. For the sake of future study, we want to create brand new aggregation operators in addition to defuzzification processes. In the future, one of our primary goals will be to expand the application of the methodology that we have created by doing research into increasingly complex theories and algorithms in a variety of subfields, and also, we will aim to use the developed approach in more domains, as well as investigate advanced theories and algorithms in diverse fields. As a result, considering everything, the q-spherical fuzzy rough set seems to be a promising new idea, opening the door to a wide variety of opportunities for study in the future.
The authors A. ALoqaily and N. Mlaiki would like to thank Prince Sultan University for paying the APC and for the support through the TAS research lab.
The authors disclose no conflict of interests in publishing this paper.
Proof 3.2: (ⅰ) Aq−SFRS⊕Bq−SFRS=A_q−SFRS⊕B_q−SFRS , −Aq−SFRS⊕−Bq−SFRS where,
A_q−SFRS⊕B_q−SFRS=⟨((μ+A_q−SFRS )q+(μ+B_q−SFRS )q−(μ+A_q−SFRS )q(μ+B_q−SFRS )q)1q,(μ′A_q−SFRS )q(μ′B_q−SFRS )q,((1−(μ+B_q−SFRS )q)(μ−A_q−SFRS )q+(1−(μ+A_q−SFRS )q)(μ−B_q−SFRS )q)−(μ−A_q−SFRS )q(μ−B_q−SFRS )q⟩, |
B_q−SFRS⊕A_q−SFRS=⟨((μ+B_q−SFRS )q+(μ+A_q−SFRS )q−(μ+B_q−SFRS )q(μ+A_q−SFRS )q)1q,(μ′B_q−SFRS )q(μ′A_q−SFRS )q,((1−(μ−A_q−SFRS )q)(μ+B_q−SFRS )q+(1−(μ−B_q−SFRS )q)(μ+A_q−SFRS )q)−(μ−B_q−SFRS )q(μ−A_q−SFRS )q⟩. |
−Aq−SFRS⊕−Bq−SFRS=⟨((μ+−Aq−SFRS )q+(μ+−Bq−SFRS )q−(μ+−Aq−SFRS )q(μ+−Bq−SFRS )q)1q,(μ′−Aq−SFRS )q(μ′−Bq−SFRS )q,((1−(μ+−Bq−SFRS )q)(μ−−Aq−SFRS )q+(1−(μ+−Aq−SFRS )q)(μ−−Bq−SFRS )q)−(μ−−Aq−SFRS )q(μ−−Bq−SFRS )q⟩, |
−Bq−SFRS⊕−Aq−SFRS=⟨((μ+−Bq−SFRS )q+(μ+−Aq−SFRS )q−(μ+−Bq−SFRS )q(μ+−Aq−SFRS )q)1q,(μ′−Bq−SFRS )q(μ′−Aq−SFRS )q,((1−(μ−−Aq−SFRS )q)(μ+−Bq−SFRS )q+(1−(μ−−Bq−SFRS )q)(μ+−Aq−SFRS )q)−(μ−−Bq−SFRS )q(μ−−Aq−SFRS )q⟩. |
And so, Aq−SFRS⊕Bq−SFRS=Bq−SFRS⊕Aq−SFRS.
(ii) Aq−SFRS⊗Bq−SFRS=A_q−SFRS⊗B_q−SFRS , −Aq−SFRS⊗−Bq−SFRS , where,
A_q−SFRS⊗B_q−SFRS=⟨(μ+A_q−SFRS )q(μ+B_q−SFRS )q,(μ′A_q−SFRS )q+(μ′B_q−SFRS )q−(μ′A_q−SFRS )q(μ′B_q−SFRS )q,((1−(μ′B_q−SFRS )q)(μ−A_q−SFRS )q+(1−(μ′A_q−SFRS )q)(μ−B_q−SFRS )q)−(μ−A_q−SFRS )q(μ−B_q−SFRS )q⟩. |
B_q−SFRS⊗A_q−SFRS=⟨(μ+B_q−SFRS )q(μ+A_q−SFRS )q,(μ′B_q−SFRS )q+(μ′A_q−SFRS )q−(μ′B_q−SFRS )q(μ′A_q−SFRS )q,((1−(μ−A_q−SFRS )q)(μ′B_q−SFRS )q+(1−(μ−B_q−SFRS )q)(μ′A_q−SFRS )q)−(μ−B_q−SFRS )q(μ−A_q−SFRS )q⟩. |
−Aq−SFRS⊗−Bq−SFRS=⟨(μ+−Aq−SFRS )q(μ+−Bq−SFRS )q,(μ′−Aq−SFRS )q+(μ′−Bq−SFRS )q−(μ′−Aq−SFRS )q(μ′−Bq−SFRS )q,((1−(μ′−Bq−SFRS )q)(μ−−Aq−SFRS )q+(1−(μ′−Aq−SFRS )q)(μ−−Bq−SFRS )q)−(μ−−Aq−SFRS )q(μ−−Bq−SFRS )q⟩. |
−Bq−SFRS⊗−Aq−SFRS=⟨(μ+−Bq−SFRS )q(μ+−Aq−SFRS )q,(μ′−Bq−SFRS )q+(μ′−Aq−SFRS )q−(μ′−Bq−SFRS )q(μ′−Aq−SFRS )q,((1−(μ−−Aq−SFRS )q)(μ′−Bq−SFRS )q+(1−(μ−−Bq−SFRS )q)(μ′−Aq−SFRS )q)−(μ−−Bq−SFRS )q(μ−−Aq−SFRS )q⟩. |
And so, Aq−SFRS⊗Bq−SFRS=Bq−SFRS⊗Aq−SFRS.
(iii) λ(Aq−SFRS⊕Bq−SFRS )=λAq−SFRS⊕λBq−SFRS, where
λ(Aq−SFRS⊕Bq−SFRS )=λ(A_q−SFRS⊕B_q−SFRS ),λ(−Aq−SFRS⊕−Bq−SFRS ) and |
λAq−SFRS⊕λBq−SFRS=λA_q−SFRS⊕λB_q−SFRS , λ−Aq−SFRS⊕λ−Bq−SFRS. |
λ(A_q−SFRS⊕B_q−SFRS )=λ⟨((μ+A_q−SFRS )q+(μ+B_q−SFRS )q−(μ+A_q−SFRS )q(μ+B_q−SFRS )q)1q,(μ′A_q−SFRS ) (μ′B_q−SFRS ) , ((1−(μ+B_q−SFRS )q)(μ−A_q−SFRS )q+(1−(μ+A_q−SFRS )q)(μ−B_q−SFRS )q)−(μ−A_q−SFRS )q(μ−B_q−SFRS )q⟩, |
=⟨(1−(1−((μ+A_q−SFRS )q+(μ+B_q−SFRS )q−(μ+A_q−SFRS )q(μ+B_q−SFRS )q))λ)1q,(μ′A_q−SFRS )λ(μ′B_q−SFRS )λ,[(1−((μ+A_q−SFRS )q+(μ+B_q−SFRS )q−(μ+A_q−SFRS )q(μ+B_q−SFRS )q))λ−((1−((μ+A_q−SFRS )q+(μ+B_q−SFRS )q−(μ+A_q−SFRS )q(μ+B_q−SFRS )q))−(1−(μ+B_q−SFRS )q)(μ−A_q−SFRS )q−(1−(μ+A_q−SFRS )q)(μ−B_q−SFRS )q+(μ−A_q−SFRS )q(μ−B_q−SFRS )q)λ]1q⟩. |
λ(−Aq−SFRS⊕−Bq−SFRS )=λ⟨((μ+−Aq−SFRS )q+(μ+−Bq−SFRS )q−(μ+−Aq−SFRS )q(μ+−Bq−SFRS )q)1q,(μ′−Aq−SFRS ) (μ′−Bq−SFRS ) , ((1−(μ+−Bq−SFRS )q)(μ−−Aq−SFRS )q+(1−(μ+−Aq−SFRS )q)(μ−−Bq−SFRS )q)−(μ−−Aq−SFRS )q(μ−−Bq−SFRS )q⟩, |
=⟨(1−(1−((μ+−Aq−SFRS )q+(μ+−Bq−SFRS )q−(μ+−Aq−SFRS )q(μ+−Bq−SFRS )q))λ)1q,(μ′−Aq−SFRS )λ(μ′−Bq−SFRS )λ,[(1−((μ+−Aq−SFRS )q+(μ+−Bq−SFRS )q−(μ+−Aq−SFRS )q(μ+−Bq−SFRS )q))λ−((1−((μ+−Aq−SFRS )q+(μ+−Bq−SFRS )q−(μ+−Aq−SFRS )q(μ+−Bq−SFRS )q))−(1−(μ+−Bq−SFRS )q)(μ−−Aq−SFRS )q−(1−(μ+−Aq−SFRS )q)(μ−−Bq−SFRS )q+(μ−−Aq−SFRS )q(μ−−Bq−SFRS )q)λ]1q⟩. |
λA_q−SFRS⊕λB_q−SFRS=⟨(1−(1−(μ+A_q−SFRS )q)λ)1q,(μ′A_q−SFRS )λ,[(1−(μ+A_q−SFRS )q)λ−(1−(μ+A_q−SFRS )q−(μ−A_q−SFRS )q)λ]1q⟩⊕⟨(1−(1−(μ+B_q−SFRS )q)λ)1q,(μ′B_q−SFRS )λ,[(1−(μ+B_q−SFRS )q)λ−(1−(μ+B_q−SFRS )q−(μ−B_q−SFRS )q)λ]1q⟩, |
=⟨(1−(1−(μ+A_q−SFRS )q)λ+1−(1−(μ+B_q−SFRS )q)λ−(1−(1−(μ+A_q−SFRS )q)λ)(1−(1−(μ+B_q−SFRS )q)λ))1q,(μ′A_q−SFRS )λ(μ′−Bq−SFRS )λ,1−(1−(1−(μ+B_q−SFRS )q)λ)((1−(μ+A_q−SFRS )q)λ−(1−(μ+A_q−SFRS )q−(μ−A_q−SFRS )q)λ)+((1−(1−(μ+A_q−SFRS )q)λ)((1−(μ+B_q−SFRS )q)λ−(1−(μ+B_q−SFRS )q−(μ−B_q−SFRS )q)λ)−((1−(μ+A_q−SFRS )q)λ−(1−(μ+A_q−SFRS )q−(μ−A_q−SFRS )q)λ)((1−(μ+B_q−SFRS )q)λ−(1−(μ+B_q−SFRS )q−(μ−B_q−SFRS )q)λ))1q⟩, |
=⟨(1−(1−(μ+A_q−SFRS )q)λ(1−(μ+B_q−SFRS )q)λ)1q,(μ′A_q−SFRS )λ(μ′B_q−SFRS )λ,((1−(μ+A_q−SFRS )q)λ(1−(μ+B_q−SFRS )q)λ−(1−(μ+A_q−SFRS )q−(μ−A_q−SFRS )q)λ(1−(μ+B_q−SFRS )q−(μ−B_q−SFRS )q)λ)1q⟩, |
=⟨(1−(1−((μ+A_q−SFRS )q+(μ+B_q−SFRS )q−(μ+A_q−SFRS )q(μ+B_q−SFRS )q))λ)1q,(μ′A_q−SFRS )λ(μ′B_q−SFRS )λ,[(1−((μ+A_q−SFRS )q+(μ+B_q−SFRS )q−(μ+A_q−SFRS )q(μ+B_q−SFRS )q))λ−((1−((μ+A_q−SFRS )q+(μ+B_q−SFRS )q−(μ+A_q−SFRS )q(μ+B_q−SFRS )q))−(1−(μ+B_q−SFRS )q)(μ−A_q−SFRS )q−(1−(μ+A_q−SFRS )q)(μ−B_q−SFRS )q+(μ−A_q−SFRS )q(μ−B_q−SFRS )q)λ]1q⟩. |
λ−Aq−SFRS⊕λ−Bq−SFRS=⟨(1−(1−(μ+−Aq−SFRS )q)λ)1q,(μ′−Aq−SFRS )λ,[(1−(μ+−Aq−SFRS )q)λ−(1−(μ+−Aq−SFRS )q−(μ−−Aq−SFRS )q)λ]1q⟩⊕⟨(1−(1−(μ+−Bq−SFRS )q)λ)1q,(μ′−Bq−SFRS )λ,[(1−(μ+−Bq−SFRS )q)λ−(1−(μ+−Bq−SFRS )q−(μ−−Bq−SFRS )q)λ]1q⟩, |
=⟨(1−(1−(μ+−Aq−SFRS )q)λ+1−(1−(μ+−Bq−SFRS )q)λ−(1−(1−(μ+−Aq−SFRS )q)λ)(1−(1−(μ+−Bq−SFRS )q)λ))1q,(μ′−Aq−SFRS )λ(μ′−Bq−SFRS )λ,1−(1−(1−(μ+−Bq−SFRS )q)λ)((1−(μ+−Aq−SFRS )q)λ−(1−(μ+−Aq−SFRS )q−(μ−−Aq−SFRS )q)λ)+((1−(1−(μ+−Aq−SFRS )q)λ)((1−(μ+−Bq−SFRS )q)λ−(1−(μ+−Bq−SFRS )q−(μ−−Bq−SFRS )q)λ)−((1−(μ+−Aq−SFRS )q)λ−(1−(μ+−Aq−SFRS )q−(μ−−Aq−SFRS )q)λ)((1−(μ+−Bq−SFRS )q)λ−(1−(μ+−Bq−SFRS )q−(μ−−Bq−SFRS )q)λ))1q⟩, |
=⟨(1−(1−(μ+−Aq−SFRS )q)λ(1−(μ+−Bq−SFRS )q)λ)1q,(μ′−Aq−SFRS )λ(μ′−Bq−SFRS )λ,((1−(μ+−Aq−SFRS )q)λ(1−(μ+−Bq−SFRS )q)λ−(1−(μ+−Aq−SFRS )q−(μ−−Aq−SFRS )q)λ(1−(μ+−Bq−SFRS )q−(μ−−Bq−SFRS )q)λ)1q⟩, |
=⟨(1−(1−((μ+−Aq−SFRS )q+(μ+−Bq−SFRS )q−(μ+−Aq−SFRS )q(μ+−Bq−SFRS )q))λ)1q,(μ′−Aq−SFRS )λ(μ′−Bq−SFRS )λ,[(1−((μ+−Aq−SFRS )q+(μ+−Bq−SFRS )q−(μ+−Aq−SFRS )q(μ+−Bq−SFRS )q))λ−((1−((μ+−Aq−SFRS )q+(μ+−Bq−SFRS )q−(μ+−Aq−SFRS )q(μ+−Bq−SFRS )q))−(1−(μ+−Bq−SFRS )q)(μ−−Aq−SFRS )q−(1−(μ+−Aq−SFRS )q)(μ−−Bq−SFRS )q+(μ−−Aq−SFRS )q(μ−−Bq−SFRS )q)λ]1q⟩. |
And so, λ(Aq−SFRS⊕Bq−SFRS )=λAq−SFRS⊕λBq−SFRS.
(iv) λ1(Aq−SFRS )⊕λ2(Aq−SFRS )=(λ1+λ2)Aq−SFRS, where
λ1(Aq−SFRS )⊕λ2(Aq−SFRS )=λ1(A_q−SFRS )⊕λ2(A_q−SFRS ),λ1(−Aq−SFRS ))⊕λ2(−Aq−SFRS )) |
(λ1+λ2)Aq−SFRS=((λ1+λ2)A_q−SFRS , (λ1+λ2)−Aq−SFRS ) |
λ1(A_q−SFRS )⊕λ2(A_q−SFRS )=⟨(1−(1−(μ+A_q−SFRS )q)λ1)1q,(μ′A_q−SFRS )λ1,[(1−(μ+A_q−SFRS )q)λ1−(1−(μ+A_q−SFRS )q−(μ−A_q−SFRS )q)λ1]1q⟩⊕⟨(1−(1−(μ+A_q−SFRS )q)λ2)1q,(μ′A_q−SFRS )λ2,[(1−(μ+A_q−SFRS )q)λ2−(1−(μ+A_q−SFRS )q−(μ−A_q−SFRS )q)λ2]1q⟩, |
=⟨(1−(1−(μ+A_q−SFRS )q)λ1+1−(1−(μ+A_q−SFRS )q)λ2−(1−(1−(μ+A_q−SFRS )q)λ1)(1−(1−(μ+A_q−SFRS )q)λ2))1q,(μ′A_q−SFRS )λ1+λ2,((1−(μ+A_q−SFRS )q)λ2((1−(μ+A_q−SFRS )q)λ1(1−(μ+A_q−SFRS )q−(μ−A_q−SFRS )q)λ1)+(1−(μ+A_q−SFRS )q)λ1((1−(μ+A_q−SFRS )q)λ2(1−(μ+A_q−SFRS )q−(μ−A_q−SFRS )q)λ2)−((1−(μ+A_q−SFRS )q)λ1−(1−(μ+A_q−SFRS )q−(μ−A_q−SFRS )q)λ1)−((1−(μ+A_q−SFRS )q)λ2−(1−(μ+A_q−SFRS )q−(μ−A_q−SFRS )q)λ2))1q⟩, |
=⟨(1−(1−(μ+A_q−SFRS )q)(λ1+λ2))1q,(μ′A_q−SFRS )(λ1+λ2),[(1−(μ+A_q−SFRS )q)(λ1+λ2)−(1−(μ+A_q−SFRS )q−(μ−A_q−SFRS )q)(λ1+λ2)]1q⟩. |
(λ1+λ2)A_q−SFRS=⟨(1−(1−(μ+A_q−SFRS )q)(λ1+λ2))1q,(μ′A_q−SFRS )(λ1+λ2),[(1−(μ+A_q−SFRS )q)(λ1+λ2)−(1−(μ+A_q−SFRS )q−(μ−A_q−SFRS )q)(λ1+λ2)]1q⟩. |
λ1(−Aq−SFRS )⊕λ2(−Aq−SFRS )=⟨(1−(1−(μ+−Aq−SFRS )q)λ1)1q,(μ′−Aq−SFRS )λ1,[(1−(μ+−Aq−SFRS )q)λ1−(1−(μ+−Aq−SFRS )q−(μ−−Aq−SFRS )q)λ1]1q⟩⊕⟨(1−(1−(μ+−Aq−SFRS )q)λ2)1q,(μ′−Aq−SFRS )λ2,[(1−(μ+−Aq−SFRS )q)λ2−(1−(μ+−Aq−SFRS )q−(μ−−Aq−SFRS )q)λ2]1q⟩, |
=⟨(1−(1−(μ+−Aq−SFRS )q)λ1+1−(1−(μ+−Aq−SFRS )q)λ2−(1−(1−(μ+−Aq−SFRS )q)λ1)(1−(1−(μ+−Aq−SFRS )q)λ2))1q,(μ′−Aq−SFRS )λ1+λ2,((1−(μ+−Aq−SFRS )q)λ2((1−(μ+−Aq−SFRS )q)λ1(1−(μ+−Aq−SFRS )q−(μ−−Aq−SFRS )q)λ1)+(1−(μ+−Aq−SFRS )q)λ1((1−(μ+−Aq−SFRS )q)λ2(1−(μ+−Aq−SFRS )q−(μ−−Aq−SFRS )q)λ2)−((1−(μ+−Aq−SFRS )q)λ1−(1−(μ+−Aq−SFRS )q−(μ−−Aq−SFRS )q)λ1)−((1−(μ+−Aq−SFRS )q)λ2−(1−(μ+−Aq−SFRS )q−(μ−−Aq−SFRS )q)λ2))1q⟩, |
=⟨(1−(1−(μ+−Aq−SFRS )q)(λ1+λ2))1q,(μ′−Aq−SFRS )(λ1+λ2),[(1−(μ+−Aq−SFRS )q)(λ1+λ2)−(1−(μ+−Aq−SFRS )q−(μ−−Aq−SFRS )q)(λ1+λ2)]1q⟩, |
(λ1+λ2)A_q−SFRS=⟨(1−(1−(μ+−Aq−SFRS )q)(λ1+λ2))1q,(μ′−Aq−SFRS )(λ1+λ2),[(1−(μ+−Aq−SFRS )q)(λ1+λ2)−(1−(μ+−Aq−SFRS )q−(μ−−Aq−SFRS )q)(λ1+λ2)]1q⟩. |
And so, λ1(Aq−SFRS )⊕λ2(Aq−SFRS )=(λ1+λ2)Aq−SFRS.
(v) (Aq−SFRS⊗Bq−SFRS )λ=Aq−SFRSλ⊗Bq−SFRSλ, where
(Aq−SFRS⊗Bq−SFRS )λ=((A_q−SFRS⊗B_q−SFRS )λ,(−Aq−SFRS⊗−Bq−SFRS )λ) |
Aq−SFRSλ⊗Bq−SFRSλ=A_q−SFRSλ⊗B_q−SFRSλ,−Aq−SFRSλ⊗−Bq−SFRSλ |
(A_q−SFRS⊗B_q−SFRS )λ |
=⟨((μ+A_q−SFRS ) (μ+B_q−SFRS ) , ((μ′A_q−SFRS )q+(μ′B_q−SFRS )q−(μ′A_q−SFRS )q(μ′B_q−SFRS )q)1q..,[((1−(μ′B_q−SFRS )q)(μ−A_q−SFRS )q−(1−(μ′A_q−SFRS )q)(μ−B_q−SFRS )q+(μ−A_q−SFRS )q(μ−B_q−SFRS )q)λ]1q)λ⟩, |
=⟨(μ+A_q−SFRS )λ(μ+B_q−SFRS )λ,(1−(1−((μ′A_q−SFRS )q+(μ′B_q−SFRS )q−(μ′A_q−SFRS )q(μ′B_q−SFRS )q))λ)1q,[(1−((μ′A_q−SFRS )q+(μ′B_q−SFRS )q−(μ′A_q−SFRS )q(μ′B_q−SFRS )q))λ−(1−((μ′A_q−SFRS )q+(μ′B_q−SFRS )q−(μ′A_q−SFRS )q(μ′B_q−SFRS )q))−((1−(μ′B_q−SFRS )q)(μ−A_q−SFRS )q−(1−(μ′A_q−SFRS )q)(μ−B_q−SFRS )q+(μ−A_q−SFRS )q(μ−B_q−SFRS )q)λ]1q⟩. |
(−Aq−SFRS⊗−Bq−SFRS )λ |
=⟨((μ+−Aq−SFRS ) (μ+−Bq−SFRS ) , ((μ′−Aq−SFRS )q+(μ′−Bq−SFRS )q−(μ′−Aq−SFRS )q(μ′−Bq−SFRS )q)1q..,[((1−(μ′−Bq−SFRS )q)(μ−−Aq−SFRS )q−(1−(μ′−Aq−SFRS )q)(μ−−Bq−SFRS )q+(μ−−Aq−SFRS )q(μ−−Bq−SFRS )q)λ]1q)λ⟩, |
=⟨(μ+−Aq−SFRS )λ(μ+−Bq−SFRS )λ,(1−(1−((μ′−Aq−SFRS )q+(μ′−Bq−SFRS )q−(μ′−Aq−SFRS )q(μ′−Bq−SFRS )q))λ)1q,[(1−((μ′−Aq−SFRS )q+(μ′−Bq−SFRS )q−(μ′−Aq−SFRS )q(μ′−Bq−SFRS )q))λ−(1−((μ′−Aq−SFRS )q+(μ′−Bq−SFRS )q−(μ′−Aq−SFRS )q(μ′−Bq−SFRS )q))−((1−(μ′−Bq−SFRS )q)(μ−−Aq−SFRS )q−(1−(μ′−Aq−SFRS )q)(μ−−Bq−SFRS )q+(μ−−Aq−SFRS )q(μ−−Bq−SFRS )q)λ]1q⟩. |
A_q−SFRSλ⊗B_q−SFRSλ=⟨(μ+A_q−SFRS )λ,(1−(1−(μ′A_q−SFRS )q)λ)1q,[(1−(μ′A_q−SFRS )q)λ−(1−(μ′A_q−SFRS )q−(μ−A_q−SFRS )q)λ]1q⟩⊗⟨(μ+B_q−SFRS )λ,(1−(1−(μ′B_q−SFRS )q)λ)1q,[(1−(μ′B_q−SFRS )q)λ−(1−(μ′B_q−SFRS )q−(μ−B_q−SFRS )q)λ]1q⟩, |
=⟨(μ+A_q−SFRS )λ(μ+B_q−SFRS )λ,(1−(1−(μ′A_q−SFRS )q)λ+1−(1−(μ′B_q−SFRS )q)λ−(1−(1−(μ′A_q−SFRS )q)λ)(1−(1−(μ′B_q−SFRS )q)λ))1q,(1−(1−(1−(μ′B_q−SFRS )q)λ)((1−(μ′A_q−SFRS )q)λ−(1−(μ′A_q−SFRS )q−(μ−A_q−SFRS )q)λ)+((1−(1−(μ′A_q−SFRS )q)λ)((1−(μ′B_q−SFRS )q)λ−(1−(μ′B_q−SFRS )q−(μ−B_q−SFRS )q)λ)−((1−(μ′A_q−SFRS )q)λ−(1−(μ′A_q−SFRS )q−(μ−A_q−SFRS )q)λ)((1−(μ′B_q−SFRS )q)λ−(1−(μ′B_q−SFRS )q−(μ−B_q−SFRS )q)λ)))1q⟩, |
=⟨(μ+A_q−SFRS )λ(μ+B_q−SFRS )λ,(1−(1−(μ′A_q−SFRS )q)λ(1−(μ′B_q−SFRS )q)λ)1q,((1−(μ′A_q−SFRS )q)λ(1−(μ′B_q−SFRS )q)λ−(1−(μ′A_q−SFRS )q−(μ−A_q−SFRS )q)λ(1−(μ′B_q−SFRS )q−(μ−B_q−SFRS )q)λ)1q⟩, |
=⟨(μ+A_q−SFRS )λ(μ+B_q−SFRS )λ,(1−(1−((μ′A_q−SFRS )q+(μ′B_q−SFRS )q−(μ′A_q−SFRS )q(μ′B_q−SFRS )q))λ)1q,[(1−((μ′A_q−SFRS )q+(μ′B_q−SFRS )q−(μ′A_q−SFRS )q(μ′B_q−SFRS )q))λ−((1−((μ′A_q−SFRS )q+(μ′B_q−SFRS )q−(μ′A_q−SFRS )q(μ′B_q−SFRS )q))−(1−(μ′B_q−SFRS )q)(μ−A_q−SFRS )q−(1−(μ′A_q−SFRS )q)(μ−B_q−SFRS )q+(μ−A_q−SFRS )q(μ−B_q−SFRS )q)λ]1q⟩. |
\begin{array}{c} {{\stackrel{-}{A}}_{q-SFRS}}^{\lambda }\otimes {{\stackrel{-}{B}}_{q-SFRS}}^{\lambda } = ⟨{({{\mu }^{+}}_{{\stackrel{-}{A}}_{q-SFRS}}\ \ \ \ \ )}^{\mathrm{\lambda }}, {(1-{(1-{({{\mu }^{'}}_{{\stackrel{-}{A}}_{q-SFRS}}\ \ \ \ \ )}^{q})}^{\mathrm{\lambda }})}^{\frac{1}{q}}, [{(1-{({{\mu }^{'}}_{{\stackrel{-}{A}}_{q-SFRS}}\ \ \ \ \ )}^{q})}^{\mathrm{\lambda }}-\\ {(1-{({{\mu }^{'}}_{{\stackrel{-}{A}}_{q-SFRS}}\ \ \ \ \ )}^{q}-{({{\mu }^{-}}_{{\stackrel{-}{A}}_{q-SFRS}}\ \ \ \ \ )}^{\mathrm{q}})}^{\mathrm{\lambda }}]^{\frac{1}{q}}⟩\otimes ⟨{({{\mu }^{+}}_{{\stackrel{-}{B}}_{q-SFRS}}\ \ \ \ \ )}^{\mathrm{\lambda }}, {(1-{(1-{({{\mu }^{'}}_{{\stackrel{-}{B}}_{q-SFRS}}\ \ \ \ \ )}^{q})}^{\mathrm{\lambda }})}^{\frac{1}{q}}, [(1-\\ {({{\mu }^{'}}_{{\stackrel{-}{B}}_{q-SFRS}}\ \ \ \ \ )}^{q})^{\mathrm{\lambda }}-{(1-{({{\mu }^{'}}_{{\stackrel{-}{B}}_{q-SFRS}}\ \ \ \ \ )}^{q}-{({{\mu }^{-}}_{{\stackrel{-}{B}}_{q-SFRS}}\ \ \ \ \ )}^{\mathrm{q}})}^{\mathrm{\lambda }}]^{\frac{1}{q}}⟩ , \end{array} |
\begin{array}{c} = ⟨{({{\mu }^{+}}_{{\stackrel{-}{A}}_{q-SFRS}}\ \ \ \ \ )}^{\lambda }{({{\mu }^{+}}_{{\stackrel{-}{B}}_{q-SFRS}}\ \ \ \ \ )}^{\lambda }, (1-{(1-{({\mu {'}}_{{\stackrel{-}{A}}_{q-SFRS}}\ \ \ \ \ )}^{q})}^{\mathrm{\lambda }}+1-{(1-{({\mu {'}}_{{\stackrel{-}{B}}_{q-SFRS}}\ \ \ \ \ )}^{q})}^{\mathrm{\lambda }}-\\ (1-{(1-{({\mu {'}}_{{\stackrel{-}{A}}_{q-SFRS}}\ \ \ \ \ )}^{q})}^{\mathrm{\lambda }})(1-{(1-{({\mu {'}}_{{\stackrel{-}{B}}_{q-SFRS}}\ \ \ \ \ )}^{q})}^{\mathrm{\lambda }}))^{\frac{1}{q}}, (1-(1-(1-\\ {({\mu {'}}_{{\stackrel{-}{B}}_{q-SFRS}}\ \ \ \ \ )}^{q})^{\mathrm{\lambda }})((1-{({\mu {'}}_{{\stackrel{-}{A}}_{q-SFRS}}\ \ \ \ \ )}^{q})^{\mathrm{\lambda }}-{(1-{({\mu {'}}_{{\stackrel{-}{A}}_{q-SFRS}}\ \ \ \ \ )}^{q}-{({{\mu }^{-}}_{{\stackrel{-}{A}}_{q-SFRS}}\ \ \ \ \ )}^{q})}^{\mathrm{\lambda }})+((1-\\ {(1-{({\mu {'}}_{{\stackrel{-}{A}}_{q-SFRS}}\ \ \ \ \ )}^{q})}^{\mathrm{\lambda }})({(1-{({\mu {'}}_{{\stackrel{-}{B}}_{q-SFRS}}\ \ \ \ \ )}^{q})}^{\mathrm{\lambda }}-(1-{({\mu {'}}_{{\stackrel{-}{B}}_{q-SFRS}}\ \ \ \ \ )}^{q}-{({{\mu }^{-}}_{{\stackrel{-}{B}}_{q-SFRS}}\ \ \ \ \ )}^{q})^{\mathrm{\lambda }})-((1-\\ {({\mu {'}}_{{\stackrel{-}{A}}_{q-SFRS}}\ \ \ \ \ )}^{q})^{\mathrm{\lambda }}-{(1-{({\mu {'}}_{{\stackrel{-}{A}}_{q-SFRS}}\ \ \ \ \ )}^{q}-{({{\mu }^{-}}_{{\stackrel{-}{A}}_{q-SFRS}}\ \ \ \ \ )}^{\mathrm{q}})}^{\mathrm{\lambda }})((1-{({\mu {'}}_{{\stackrel{-}{B}}_{q-SFRS}}\ \ \ \ \ )}^{q})^{\mathrm{\lambda }}-(1-\\ {({\mu {'}}_{{\stackrel{-}{B}}_{q-SFRS}}\ \ \ \ \ )}^{q}- {({{\mu }^{-}}_{{\stackrel{-}{B}}_{q-SFRS}}\ \ \ \ \ )}^{\mathrm{q}})^{\mathrm{\lambda }})))^{\frac{1}{q}}⟩ , \end{array} |
\begin{array}{c} = ⟨{({{\mu }^{+}}_{{\stackrel{-}{A}}_{q-SFRS}}\ \ \ \ \ )}^{\lambda }{({{\mu }^{+}}_{{\stackrel{-}{B}}_{q-SFRS}}\ \ \ \ \ )}^{\lambda }, {(1-{(1-{({{\mu }^{'}}_{{\stackrel{-}{A}}_{q-SFRS}}\ \ \ \ \ )}^{q})}^{\mathrm{\lambda }}{(1-{({{\mu }^{'}}_{{\stackrel{-}{B}}_{q-SFRS}}\ \ \ \ \ )}^{q})}^{\mathrm{\lambda }})}^{\frac{1}{q}}, ((1-\\ {({{\mu }^{'}}_{{\stackrel{-}{A}}_{q-SFRS}}\ \ \ \ \ )}^{q})^{\mathrm{\lambda }}{(1-{({{\mu }^{'}}_{{\stackrel{-}{B}}_{q-SFRS}}\ \ \ \ \ )}^{q})}^{\mathrm{\lambda }}-{(1-{({{\mu }^{'}}_{{\stackrel{-}{A}}_{q-SFRS}}\ \ \ \ \ )}^{q}-{({{\mu }^{-}}_{{\stackrel{-}{A}}_{q-SFRS}}\ \ \ \ \ )}^{q})}^{\mathrm{\lambda }}(1-({{\mu }^{'}}_{{\stackrel{-}{B}}_{q-SFRS}}\ \ \ \ )^{q}-\\ {({{\mu }^{-}}_{{\stackrel{-}{B}}_{q-SFRS}}\ \ \ \ \ )}^{q})^{\mathrm{\lambda }})^{\frac{1}{q}}⟩ , \end{array} |
\begin{array}{c} = ⟨{({{\mu }^{+}}_{{\stackrel{-}{A}}_{q-SFRS}}\ \ \ \ \ )}^{\lambda }{({{\mu }^{+}}_{{\stackrel{-}{B}}_{q-SFRS}}\ \ \ \ \ )}^{\lambda }, (1-(1-({({{\mu }^{'}}_{{\stackrel{-}{A}}_{q-SFRS}}\ \ \ \ \ )}^{q}+{({{\mu }^{'}}_{{\stackrel{-}{B}}_{q-SFRS}}\ \ \ \ \ )}^{q}-\\ {({{\mu }^{'}}_{{\stackrel{-}{A}}_{q-SFRS}}\ \ \ \ \ )}^{q}{({{\mu }^{'}}_{{\stackrel{-}{B}}_{q-SFRS}}\ \ \ \ \ )}^{q}))^{\lambda })^{\frac{1}{q}}, [(1-({({{\mu }^{'}}_{{\stackrel{-}{A}}_{q-SFRS}}\ \ \ \ \ )}^{q}+{({{\mu }^{'}}_{{\stackrel{-}{B}}_{q-SFRS}}\ \ \ \ \ )}^{q}-\\ {({{\mu }^{'}}_{{\stackrel{-}{A}}_{q-SFRS}}\ \ \ \ \ )}^{q}{({{\mu }^{'}}_{{\stackrel{-}{B}}_{q-SFRS}}\ \ \ \ \ )}^{q}))^{\lambda }-((1-({({{\mu }^{'}}_{{\stackrel{-}{A}}_{q-SFRS}}\ \ \ \ \ )}^{q}+{({{\mu }^{'}}_{{\stackrel{-}{B}}_{q-SFRS}}\ \ \ \ \ )}^{q}-\\ {({{\mu }^{'}}_{{\stackrel{-}{A}}_{q-SFRS}}\ \ \ \ \ )}^{q}{({{\mu }^{'}}_{{\stackrel{-}{B}}_{q-SFRS}}\ \ \ \ \ )}^{q}))-(1-{({{\mu }^{'}}_{{\stackrel{-}{B}}_{q-SFRS}}\ \ \ \ \ )}^{q}){({{\mu }^{-}}_{{\stackrel{-}{A}}_{q-SFRS}}\ \ \ \ \ )}^{q}-(1-\\ {({{\mu }^{'}}_{{\stackrel{-}{A}}_{q-SFRS}}\ \ \ \ \ )}^{q}){({{\mu }^{-}}_{{\stackrel{-}{B}}_{q-SFRS}}\ \ \ \ \ )}^{q}+{({{\mu }^{-}}_{{\stackrel{-}{A}}_{q-SFRS}}\ \ \ \ \ )}^{q}{({{\mu }^{-}}_{{\stackrel{-}{B}}_{q-SFRS}}\ \ \ \ \ )}^{q})^{\lambda }]^{\frac{1}{q}}⟩ . \end{array} |
And so, {({A}_{q-SFRS}\otimes {B}_{q-SFRS}\ \ \ \ )}^{\lambda } = {{A}_{q-SFRS}}^{\lambda }\otimes {{B}_{q-SFRS}}^{\lambda } .
{{({\bf v}{\bf i})\ A}_{q-SFRS}}^{{\lambda }_{1}}\otimes {{A}_{q-SFRS}}^{{\lambda }_{2}} = {{A}_{q-SFRS}}^{{\lambda }_{1}+{\lambda }_{2}} where,
{{A}_{q-SFRS}}^{{\lambda }_{1}}\otimes {{A}_{q-SFRS}}^{{\lambda }_{2}} = ({{\underline{A}}_{q-SFRS}}^{{\lambda }_{1}}\otimes {{\underline{A}}_{q-SFRS}}^{{\lambda }_{2}}, {{\stackrel{-}{A}}_{q-SFRS}}^{{\lambda }_{1}}\otimes {{\stackrel{-}{A}}_{q-SFRS}}^{{\lambda }_{2}}) |
{{A}_{q-SFRS}}^{{\lambda }_{1}+{\lambda }_{2}} = ({{\underline{A}}_{q-SFRS}}^{{\lambda }_{1}+{\lambda }_{2}}, {{\stackrel{-}{A}}_{q-SFRS}}^{{\lambda }_{1}+{\lambda }_{2}}) |
\begin{array}{c} {{\underline{A}}_{q-SFRS}}^{{\lambda }_{1}}\otimes {{\underline{A}}_{q-SFRS}}^{{\lambda }_{2}} = ⟨{({{\mu }^{+}}_{{\underline{A}}_{q-SFRS}}\ \ \ \ \ )}^{{\lambda }_{1}}, {(1-{(1-{({{\mu }^{'}}_{{\underline{A}}_{q-SFRS}}\ \ \ \ \ )}^{q})}^{{\lambda }_{1}})}^{\frac{1}{q}}, [(1-\\ {({{\mu }^{'}}_{{\underline{A}}_{q-SFRS}}\ \ \ \ \ )}^{q})^{{\lambda }_{1}}-{(1-{({{\mu }^{'}}_{{\underline{A}}_{q-SFRS}}\ \ \ \ \ )}^{q}-{({{\mu }^{-}}_{{\underline{A}}_{q-SFRS}}\ \ \ \ \ )}^{\mathrm{q}})}^{{\lambda }_{1}}]^{\frac{1}{q}}⟩\otimes ⟨{({{\mu }^{+}}_{{\underline{A}}_{q-SFRS}}\ \ \ \ \ )}^{{\lambda }_{2}}, (1-(1-\\ {({{\mu }^{'}}_{{\underline{A}}_{q-SFRS}}\ \ \ \ \ )}^{q})^{{\lambda }_{2}})^{\frac{1}{q}}, {[{(1-{({{\mu }^{'}}_{{\underline{A}}_{q-SFRS}}\ \ \ \ \ )}^{q})}^{{\lambda }_{2}}-{(1-{({{\mu }^{'}}_{{\underline{A}}_{q-SFRS}}\ \ \ \ \ )}^{q}-{({{\mu }^{-}}_{{\underline{A}}_{q-SFRS}}\ \ \ \ \ )}^{\mathrm{q}})}^{{\lambda }_{2}}]}^{\frac{1}{q}}⟩ , \end{array} |
\begin{array}{c} = ⟨{({{\mu }^{+}}_{{\underline{A}}_{q-SFRS}}\ \ \ \ \ )}^{{\lambda }_{1}+{\lambda }_{2}}(1-{(1-{({{\mu }^{'}}_{{\underline{A}}_{q-SFRS}}\ \ \ \ \ )}^{q})}^{{\lambda }_{1}}+1-{(1-{({{\mu }^{'}}_{{\underline{A}}_{q-SFRS}}\ \ \ \ \ )}^{q})}^{{\lambda }_{2}}-(1-(1-\\ {({{\mu }^{'}}_{{\underline{A}}_{q-SFRS}}\ \ \ \ \ )}^{q})^{{\lambda }_{1}})(1-{(1-{({{\mu }^{'}}_{{\underline{A}}_{q-SFRS}}\ \ \ \ \ )}^{q})}^{{\lambda }_{2}}))^{\frac{1}{q}}, ({(1-{({{\mu }^{'}}_{{\underline{A}}_{q-SFRS}}\ \ \ \ \ )}^{q})}^{{\lambda }_{2}}((1-\\ {({{\mu }^{'}}_{{\underline{A}}_{q-SFRS}}\ \ \ \ \ )}^{q})^{{\lambda }_{1}}(1-{({{\mu }^{'}}_{{\underline{A}}_{q-SFRS}}\ \ \ \ \ )}^{q}-{({{\mu }^{-}}_{{\underline{A}}_{q-SFRS}}\ \ \ \ \ )}^{q})^{{\lambda }_{1}})+{(1-{({{\mu }^{'}}_{{\underline{A}}_{q-SFRS}}\ \ \ \ \ )}^{q})}^{{\lambda }_{1}}((1-\\ {({{\mu }^{'}}_{{\underline{A}}_{q-SFRS}}\ \ \ \ \ )}^{q})^{{\lambda }_{2}}(1-{({{\mu }^{'}}_{{\underline{A}}_{q-SFRS}}\ \ \ \ \ )}^{q}-{({{\mu }^{-}}_{{\underline{A}}_{q-SFRS}}\ \ \ \ \ )}^{q})^{{\lambda }_{2}})-({(1-{({{\mu }^{'}}_{{\underline{A}}_{q-SFRS}}\ \ \ \ \ )}^{q})}^{{\lambda }_{1}}-(1-\\ {({{\mu }^{'}}_{{\underline{A}}_{q-SFRS}}\ \ \ \ \ )}^{q}-{({{\mu }^{-}}_{{\underline{A}}_{q-SFRS}}\ \ \ \ \ )}^{q})^{{\lambda }_{1}})-((1-{({{\mu }^{'}}_{{\underline{A}}_{q-SFRS}}\ \ \ \ \ )}^{q})^{{\lambda }_{2}}-(1-{({{\mu }^{'}}_{{\underline{A}}_{q-SFRS}}\ \ \ \ \ )}^{q}-\\ {({{\mu }^{-}}_{{\underline{A}}_{q-SFRS}}\ \ \ \ \ )}^{q})^{{\lambda }_{2}}))^{\frac{1}{q}}⟩ , \end{array} |
\begin{array}{c} = ⟨{({{\mu }^{+}}_{{\underline{A}}_{q-SFRS}}\ \ \ \ \ )}^{{\lambda }_{1}+{\lambda }_{2}}, {(1-{(1-{({{\mu }^{'}}_{{\underline{A}}_{q-SFRS}}\ \ \ \ \ )}^{q})}^{{\lambda }_{1}+{\lambda }_{2}})}^{\frac{1}{q}}, [{(1-{({{\mu }^{'}}_{{\underline{A}}_{q-SFRS}}\ \ \ \ \ )}^{q})}^{{\lambda }_{1}+{\lambda }_{2}}-(1-\\ {({{\mu }^{'}}_{{\underline{A}}_{q-SFRS}}\ \ \ \ \ )}^{q}-{({{\mu }^{-}}_{{\underline{A}}_{q-SFRS}}\ \ \ \ \ )}^{\mathrm{q}})^{{\lambda }_{1}+{\lambda }_{2}}]^{\frac{1}{q}}⟩ . \end{array} |
\begin{array}{c} {{\stackrel{-}{A}}_{q-SFRS}}^{{\lambda }_{1}}\otimes {{\stackrel{-}{A}}_{q-SFRS}}^{{\lambda }_{2}} = ⟨{({{\mu }^{+}}_{{\stackrel{-}{A}}_{q-SFRS}}\ \ \ \ \ )}^{{\lambda }_{1}}, {(1-{(1-{({{\mu }^{'}}_{{\stackrel{-}{A}}_{q-SFRS}}\ \ \ \ \ )}^{q})}^{{\lambda }_{1}})}^{\frac{1}{q}}, [(1-\\ {({{\mu }^{'}}_{{\stackrel{-}{A}}_{q-SFRS}}\ \ \ \ \ )}^{q})^{{\lambda }_{1}}-{(1-{({{\mu }^{'}}_{{\stackrel{-}{A}}_{q-SFRS}}\ \ \ \ \ )}^{q}-{({{\mu }^{-}}_{{\stackrel{-}{A}}_{q-SFRS}}\ \ \ \ \ )}^{\mathrm{q}})}^{{\lambda }_{1}}]^{\frac{1}{q}}⟩\otimes ⟨{({{\mu }^{+}}_{{\stackrel{-}{A}}_{q-SFRS}}\ \ \ \ \ )}^{{\lambda }_{2}}, (1-(1-\\ {({{\mu }^{'}}_{{\stackrel{-}{A}}_{q-SFRS}}\ \ \ \ \ )}^{q})^{{\lambda }_{2}})^{\frac{1}{q}}, {[{(1-{({{\mu }^{'}}_{{\stackrel{-}{A}}_{q-SFRS}}\ \ \ \ \ )}^{q})}^{{\lambda }_{2}}-{(1-{({{\mu }^{'}}_{{\stackrel{-}{A}}_{q-SFRS}}\ \ \ \ \ )}^{q}-{({{\mu }^{-}}_{{\stackrel{-}{A}}_{q-SFRS}}\ \ \ \ \ )}^{\mathrm{q}})}^{{\lambda }_{2}}]}^{\frac{1}{q}}⟩ , \end{array} |
\begin{array}{c} = ⟨{({{\mu }^{+}}_{{\stackrel{-}{A}}_{q-SFRS}}\ \ \ \ \ )}^{{\lambda }_{1}+{\lambda }_{2}}(1-{(1-{({{\mu }^{'}}_{{\stackrel{-}{A}}_{q-SFRS}}\ \ \ \ \ )}^{q})}^{{\lambda }_{1}}+1-{(1-{({{\mu }^{'}}_{{\stackrel{-}{A}}_{q-SFRS}}\ \ \ \ \ )}^{q})}^{{\lambda }_{2}}-(1-(1-\\ {({{\mu }^{'}}_{{\stackrel{-}{A}}_{q-SFRS}}\ \ \ \ \ )}^{q})^{{\lambda }_{1}})(1-{(1-{({{\mu }^{'}}_{{\stackrel{-}{A}}_{q-SFRS}}\ \ \ \ \ )}^{q})}^{{\lambda }_{2}}))^{\frac{1}{q}}, ({(1-{({{\mu }^{'}}_{{\stackrel{-}{A}}_{q-SFRS}}\ \ \ \ \ )}^{q})}^{{\lambda }_{2}}((1-\\ {({{\mu }^{'}}_{{\stackrel{-}{A}}_{q-SFRS}}\ \ \ \ \ )}^{q})^{{\lambda }_{1}}(1-{({{\mu }^{'}}_{{\stackrel{-}{A}}_{q-SFRS}}\ \ \ \ \ )}^{q}-{({{\mu }^{-}}_{{\stackrel{-}{A}}_{q-SFRS}}\ \ \ \ \ )}^{q})^{{\lambda }_{1}})+{(1-{({{\mu }^{'}}_{{\stackrel{-}{A}}_{q-SFRS}}\ \ \ \ \ )}^{q})}^{{\lambda }_{1}}((1-\\ {({{\mu }^{'}}_{{\stackrel{-}{A}}_{q-SFRS}}\ \ \ \ \ )}^{q})^{{\lambda }_{2}}(1-{({{\mu }^{'}}_{{\stackrel{-}{A}}_{q-SFRS}}\ \ \ \ \ )}^{q}-{({{\mu }^{-}}_{{\stackrel{-}{A}}_{q-SFRS}}\ \ \ \ \ )}^{q})^{{\lambda }_{2}})-({(1-{({{\mu }^{'}}_{{\stackrel{-}{A}}_{q-SFRS}}\ \ \ \ \ )}^{q})}^{{\lambda }_{1}}-(1-\\ {({{\mu }^{'}}_{{\stackrel{-}{A}}_{q-SFRS}}\ \ \ \ \ )}^{q}-{({{\mu }^{-}}_{{\stackrel{-}{A}}_{q-SFRS}}\ \ \ \ \ )}^{q})^{{\lambda }_{1}})-({(1-{({{\mu }^{'}}_{{\stackrel{-}{A}}_{q-SFRS}}\ \ \ \ \ )}^{q})}^{{\lambda }_{2}}-(1-{({{\mu }^{'}}_{{\stackrel{-}{A}}_{q-SFRS}}\ \ \ \ \ )}^{q}-\\ {({{\mu }^{-}}_{{\stackrel{-}{A}}_{q-SFRS}}\ \ \ \ \ )}^{q})^{{\lambda }_{2}}))^{\frac{1}{q}}⟩ , \end{array} |
\begin{array}{c} = ⟨{(1-{(1-{({{\mu }^{'}}_{{\stackrel{-}{A}}_{q-SFRS}}\ \ \ \ \ )}^{q})}^{({\lambda }_{1}+{\lambda }_{2})})}^{\frac{1}{q}}, {({{\mu }^{'}}_{{\stackrel{-}{A}}_{q-SFRS}}\ \ \ \ \ )}^{({\lambda }_{1}+{\lambda }_{2})}, [{(1-{({{\mu }^{'}}_{{\stackrel{-}{A}}_{q-SFRS}}\ \ \ \ \ )}^{q})}^{({\lambda }_{1}+{\lambda }_{2})}-\\ {(1-{({{\mu }^{'}}_{{\stackrel{-}{A}}_{q-SFRS}}\ \ \ \ \ )}^{q}-{({{\mu }^{-}}_{{\stackrel{-}{A}}_{q-SFRS}}\ \ \ \ \ )}^{\mathrm{q}})}^{({\lambda }_{1}+{\lambda }_{2})}]^{\frac{1}{q}}⟩ , \end{array} |
\begin{array}{c} = ⟨{({{\mu }^{+}}_{{\stackrel{-}{A}}_{q-SFRS}}\ \ \ \ \ )}^{{\lambda }_{1}+{\lambda }_{2}}, {(1-{(1-{({{\mu }^{'}}_{{\stackrel{-}{A}}_{q-SFRS}}\ \ \ \ \ )}^{q})}^{{\lambda }_{1}+{\lambda }_{2}})}^{\frac{1}{q}}, [{(1-{({{\mu }^{'}}_{{\stackrel{-}{A}}_{q-SFRS}}\ \ \ \ \ )}^{q})}^{{\lambda }_{1}+{\lambda }_{2}}-(1-\\ {({{\mu }^{'}}_{{\stackrel{-}{A}}_{q-SFRS}}\ \ \ \ \ )}^{q}-{({{\mu }^{-}}_{{\stackrel{-}{A}}_{q-SFRS}}\ \ \ \ \ )}^{\mathrm{q}})^{{\lambda }_{1}+{\lambda }_{2}}]^{\frac{1}{q}}⟩ . \end{array} |
\begin{array}{c} {{\underline{A}}_{q-SFRS}}^{{\lambda }_{1}+{\lambda }_{2}} = ⟨{({{\mu }^{+}}_{{\underline{A}}_{q-SFRS}}\ \ \ \ \ )}^{{\lambda }_{1}+{\lambda }_{2}}, {(1-{(1-{({{\mu }^{'}}_{{\underline{A}}_{q-SFRS}}\ \ \ \ \ )}^{q})}^{{\lambda }_{1}+{\lambda }_{2}})}^{\frac{1}{q}}, [(1-\\ {({{\mu }^{'}}_{{\underline{A}}_{q-SFRS}}\ \ \ \ \ )}^{q})^{{\lambda }_{1}+{\lambda }_{2}}-{(1-{({{\mu }^{'}}_{{\underline{A}}_{q-SFRS}}\ \ \ \ \ )}^{q}-{({{\mu }^{-}}_{{\underline{A}}_{q-SFRS}}\ \ \ \ \ )}^{\mathrm{q}})}^{{\lambda }_{1}+{\lambda }_{2}}]^{\frac{1}{q}}⟩ . \end{array} |
Definition 3.3: Proof: We can prove the Eq (1) by using mathematical induction on n: when n = 2 ,
{\mathrm{q}-\mathrm{S}\mathrm{F}\mathrm{R}\mathrm{A}\mathrm{M}}_{w}({A}_{q-SFRS1}, {A}_{q-SFRS2}) = {w}_{1}{A}_{q-SFRS1} \oplus {w}_{2}{A}_{q-SFRS2} . |
According to Definition 3.2, we can see that both {w}_{1}{A}_{q-SFRS1}\ \mathrm{a}\mathrm{n}\mathrm{d}\ {w}_{2}{A}_{q-SFRS2} are q-SFRNs. By the operational law (ⅳ) in Definition 3.2, we have
\begin{array}{c} {w}_{1}{\underline{A}}_{q-SFS1} = ⟨{(1-{(1-{({{\mu }^{+}}_{{\underline{A}}_{q-SFS1}}\ \ \ \ )}^{q})}^{{w}_{1}})}^{\frac{1}{q}}, {({{\mu }^{'}}_{{\underline{A}}_{q-SFS1}}\ \ \ \ )}^{{w}_{1}}, [{(1-{({{\mu }^{+}}_{{\underline{A}}_{q-SFS1}}\ \ \ \ )}^{q})}^{{w}_{1}}-\\ {(1-{({{\mu }^{+}}_{{\underline{A}}_{q-SFS1}}\ \ \ \ )}^{q}-{({{\mu }^{-}}_{{\underline{A}}_{q-SFS1}}\ \ \ \ )}^{\mathrm{q}})}^{{w}_{1}}]^{\frac{1}{q}}⟩ . \end{array} |
\begin{array}{l} {w}_{2}{\underline{A}}_{q-SFS2} = ⟨{(1-{(1-{({{\mu }^{+}}_{{\underline{A}}_{q-SFS2}\ \ \ \ })}^{q})}^{{w}_{2}})}^{\frac{1}{q}}, \\ \ \ \ \ \ \ \ \ \ \ \ \ \ \ \ \ \ \ \ \ \ \ \ \ \ \ {({{\mu }^{'}}_{{\underline{A}}_{q-SFS2}\ \ \ \ })}^{{w}_{2}}, {[{(1-{({{\mu }^{+}}_{{\underline{A}}_{q-SFS2}\ \ \ \ })}^{q})}^{{w}_{2}}-{(1-{({{\mu }^{+}}_{{\underline{A}}_{q-SFS2}\ \ \ \ })}^{q}-{({{\mu }^{-}}_{{\underline{A}}_{q-SFS2}\ \ \ \ })}^{\mathrm{q}})}^{{w}_{2}}]}^{\frac{1}{q}}⟩. \end{array} |
\begin{array}{c} {w}_{1}{\underline{A}}_{q-SFRS1} \oplus {w}_{2}{\underline{A}}_{q-SFRS2} = ⟨{(1-{(1-{({{\mu }^{+}}_{{\underline{A}}_{q-SFS1}}\ \ \ \ )}^{q})}^{{w}_{1}})}^{\frac{1}{q}}, {({{\mu }^{'}}_{{\underline{A}}_{q-SFS1}}\ \ \ \ )}^{{w}_{1}}, [(1-\\ {({{\mu }^{+}}_{{\underline{A}}_{q-SFS1}}\ \ \ \ )}^{q})^{{w}_{1}}-{(1-{({{\mu }^{+}}_{{\underline{A}}_{q-SFS1}}\ \ \ \ )}^{q}-{({{\mu }^{-}}_{{\underline{A}}_{q-SFS1}}\ \ \ \ )}^{\mathrm{q}})}^{{w}_{1}}]^{\frac{1}{q}}⟩ \oplus ⟨{(1-{(1-{({{\mu }^{+}}_{{\underline{A}}_{q-SFS2}\ \ \ \ })}^{q})}^{{w}_{2}})}^{\frac{1}{q}}, \\ {({{\mu }^{'}}_{{\underline{A}}_{q-SFS2}\ \ \ \ })}^{{w}_{2}}, {[{(1-{({{\mu }^{+}}_{{\underline{A}}_{q-SFS2}\ \ \ \ })}^{q})}^{{w}_{2}}-{(1-{({{\mu }^{+}}_{{\underline{A}}_{q-SFS2}\ \ \ \ })}^{q}-{({{\mu }^{-}}_{{\underline{A}}_{q-SFS2}\ \ \ \ })}^{\mathrm{q}})}^{{w}_{2}}]}^{\frac{1}{q}}⟩ , \end{array} |
\begin{array}{c} ⟨{(1-{(1-{({{\mu }^{+}}_{{\underline{A}}_{q-SFS1}}\ \ \ \ )}^{q})}^{{w}_{1}})}^{\frac{1}{q}}+{(1-{(1-{({{\mu }^{+}}_{{\underline{A}}_{q-SFS2}\ \ \ \ })}^{q})}^{{w}_{2}})}^{\frac{1}{q}}-(1-(1-\\ {({{\mu }^{+}}_{{\underline{A}}_{q-SFS1}}\ \ \ \ )}^{q})^{{w}_{1}})^{\frac{1}{q}}{(1-{(1-{({{\mu }^{+}}_{{\underline{A}}_{q-SFS2}\ \ \ \ })}^{q})}^{{w}_{2}})}^{\frac{1}{q}}, {({{\mu }^{'}}_{{\underline{A}}_{q-SFS1}}\ \ \ \ )}^{{w}_{1}}\cdot {({{\mu }^{'}}_{{\underline{A}}_{q-SFS2}\ \ \ \ })}^{{w}_{2}}, [1-(1-\\ {(1-{({{\mu }^{+}}_{{\underline{A}}_{q-SFRS2}\ \ \ \ })}^{q})}^{{w}_{2}})({(1-{({{\mu }^{+}}_{{\underline{A}}_{q-SFRS1}\ \ \ \ })}^{q})}^{{w}_{1}}-(1-{({{\mu }^{+}}_{{\underline{A}}_{q-SFRS1}\ \ \ \ })}^{q}- {({{\mu }^{-}}_{{\underline{A}}_{q-SFRS1}\ \ \ \ })}^{q})^{{w}_{1}})+\\ ((1-{(1-{({{\mu }^{+}}_{{\underline{A}}_{q-SFRS1}\ \ \ \ })}^{q})}^{{w}_{1}})({(1-{({{\mu }^{+}}_{{\underline{A}}_{q-SFRS2}\ \ \ \ })}^{q})}^{{w}_{2}}-(1- {({{\mu }^{+}}_{{\underline{A}}_{q-SFRS2}\ \ \ \ })}^{q}-\\{({{\mu }^{-}}_{{\underline{A}}_{q-SFRS2}\ \ \ \ })}^{q})^{{w}_{2}})-({(1-{({{\mu }^{+}}_{{\underline{A}}_{q-SFRS1}\ \ \ \ })}^{q})}^{{w}_{1}}- (1-{({{\mu }^{+}}_{{\underline{A}}_{q-SFRS1}\ \ \ \ })}^{q}- {({{\mu }^{-}}_{{\underline{A}}_{q-SFRS1}\ \ \ \ })}^{\mathrm{q}})^{{w}_{1}})((1-\\ {({{\mu }^{+}}_{{\underline{A}}_{q-SFRS2}\ \ \ \ })}^{q})^{{w}_{2}}-{(1-{({{\mu }^{+}}_{{\underline{A}}_{q-SFRS2}\ \ \ \ })}^{q}-{({{\mu }^{-}}_{{\underline{A}}_{q-SFRS2}\ \ \ \ })}^{\mathrm{q}})}^{{w}_{2}}))^{\frac{1}{q}}]⟩ , \end{array} |
\begin{array}{c} = ⟨{[1-{(1-{({{\mu }^{+}}_{{\underline{A}}_{q-SFRS1}\ \ \ \ })}^{q})}^{{w}_{1}}{(1-{({{\mu }^{+}}_{{\underline{A}}_{q-SFRS2}\ \ \ \ })}^{q})}^{{w}_{2}}]}^{\frac{1}{q}}, {({{\mu }^{'}}_{{\underline{A}}_{q-SFRS1}\ \ \ \ })}^{{w}_{1}}{({{\mu }^{'}}_{{\underline{A}}_{q-SFRS2}\ \ \ \ })}^{{w}_{2}}, [(1-\\ {({{\mu }^{+}}_{{\underline{A}}_{q-SFRS1}\ \ \ \ })}^{q})^{{w}_{1}}{(1-{({{\mu }^{+}}_{{\underline{A}}_{q-SFRS2}\ \ \ \ })}^{q})}^{{w}_{2}}-{(1-{({{\mu }^{+}}_{{\underline{A}}_{q-SFRS1}\ \ \ \ })}^{q}-{({{\mu }^{-}}_{{\underline{A}}_{q-SFRS2}\ \ \ \ })}^{q})}^{{w}_{1}}(1-\\ {({{\mu }^{+}}_{{\underline{A}}_{q-SFRS2}\ \ \ \ })}^{q}-{({{\mu }^{-}}_{{\underline{A}}_{q-SFRS2}\ \ \ \ })}^{q})^{{w}_{2}}]^{\frac{1}{q}}⟩ . \end{array} |
\begin{array}{c} {w}_{1}{\underline{A}}_{q-SFS1} = ⟨{(1-{(1-{({{\mu }^{+}}_{{\underline{A}}_{q-SFS1}}\ \ \ \ )}^{q})}^{{w}_{1}})}^{\frac{1}{q}}, {({{\mu }^{'}}_{{\underline{A}}_{q-SFS1}}\ \ \ \ )}^{{w}_{1}}, [{(1-{({{\mu }^{+}}_{{\underline{A}}_{q-SFS1}}\ \ \ \ )}^{q})}^{{w}_{1}}-\\ {(1-{({{\mu }^{+}}_{{\underline{A}}_{q-SFS1}}\ \ \ \ )}^{q}-{({{\mu }^{-}}_{{\underline{A}}_{q-SFS1}}\ \ \ \ )}^{\mathrm{q}})}^{{w}_{1}}]^{\frac{1}{q}}⟩ . \end{array} |
\begin{array}{c} {w}_{2}{\underline{A}}_{q-SFS2} = ⟨{(1-{(1-{({{\mu }^{+}}_{{\underline{A}}_{q-SFS2}\ \ \ \ })}^{q})}^{{w}_{2}})}^{\frac{1}{q}}, {({{\mu }^{'}}_{{\underline{A}}_{q-SFS2}\ \ \ \ })}^{{w}_{2}}, [{(1-{({{\mu }^{+}}_{{\underline{A}}_{q-SFS2}\ \ \ \ })}^{q})}^{{w}_{2}}-\\ {(1-{({{\mu }^{+}}_{{\underline{A}}_{q-SFS2}\ \ \ \ })}^{q}-{({{\mu }^{-}}_{{\underline{A}}_{q-SFS2}\ \ \ \ })}^{\mathrm{q}})}^{{w}_{2}}]^{\frac{1}{q}}⟩ . \end{array} |
\begin{array}{c} {w}_{1}{\stackrel{-}{A}}_{q-SFRS1} \oplus {w}_{2}{\stackrel{-}{A}}_{q-SFRS2} = ⟨{(1-{(1-{({{\mu }^{+}}_{{\stackrel{-}{A}}_{q-SFS1}}\ \ \ \ )}^{q})}^{{w}_{1}})}^{\frac{1}{q}}, {({{\mu }^{'}}_{{\stackrel{-}{A}}_{q-SFS1}}\ \ \ \ )}^{{w}_{1}}, [(1-\\ {({{\mu }^{+}}_{{\stackrel{-}{A}}_{q-SFS1}}\ \ \ \ )}^{q})^{{w}_{1}}-{(1-{({{\mu }^{+}}_{{\stackrel{-}{A}}_{q-SFS1}}\ \ \ \ )}^{q}-{({{\mu }^{-}}_{{\stackrel{-}{A}}_{q-SFS1}}\ \ \ \ )}^{\mathrm{q}})}^{{w}_{1}}]^{\frac{1}{q}}⟩ \oplus ⟨{(1-{(1-{({{\mu }^{+}}_{{\stackrel{-}{A}}_{q-SFS2}\ \ \ \ })}^{q})}^{{w}_{2}})}^{\frac{1}{q}}, \\ {({{\mu }^{'}}_{{\stackrel{-}{A}}_{q-SFS2}\ \ \ \ })}^{{w}_{2}}, {[{(1-{({{\mu }^{+}}_{{\stackrel{-}{A}}_{q-SFS2}\ \ \ \ })}^{q})}^{{w}_{2}}-{(1-{({{\mu }^{+}}_{{\stackrel{-}{A}}_{q-SFS2}\ \ \ \ })}^{q}-{({{\mu }^{-}}_{{\stackrel{-}{A}}_{q-SFS2}\ \ \ \ })}^{\mathrm{q}})}^{{w}_{2}}]}^{\frac{1}{q}}⟩ , \end{array} |
\begin{array}{c} = ⟨{(1-{(1-{({{\mu }^{+}}_{{\stackrel{-}{A}}_{q-SFS1}}\ \ \ \ )}^{q})}^{{w}_{1}})}^{\frac{1}{q}}+{(1-{(1-{({{\mu }^{+}}_{{\stackrel{-}{A}}_{q-SFS2}\ \ \ \ })}^{q})}^{{w}_{2}})}^{\frac{1}{q}}-(1-(1-\\ {({{\mu }^{+}}_{{\stackrel{-}{A}}_{q-SFS1}}\ \ \ \ )}^{q})^{{w}_{1}})^{\frac{1}{q}}(1-(1- {({{\mu }^{+}}_{{\stackrel{-}{A}}_{q-SFS2}\ \ \ \ })}^{q})^{{w}_{2}})^{\frac{1}{q}}, {({{\mu }^{'}}_{{\stackrel{-}{A}}_{q-SFS1}}\ \ \ \ )}^{{w}_{1}}\cdot {({{\mu }^{'}}_{{\stackrel{-}{A}}_{q-SFS2}\ \ \ \ })}^{{w}_{2}}, [1-(1-\\ (1- {({{\mu }^{+}}_{{\stackrel{-}{A}}_{q-SFRS2}\ \ \ \ })}^{q})^{{w}_{2}})({(1-{({{\mu }^{+}}_{{\stackrel{-}{A}}_{q-SFRS1}\ \ \ \ })}^{q})}^{{w}_{1}}-{(1-{({{\mu }^{+}}_{{\stackrel{-}{A}}_{q-SFRS1}\ \ \ \ })}^{q}-{({{\mu }^{-}}_{{\stackrel{-}{A}}_{q-SFRS1}\ \ \ \ })}^{q})}^{{w}_{1}})+\\ ((1-{(1-{({{\mu }^{+}}_{{\stackrel{-}{A}}_{q-SFRS1}\ \ \ \ })}^{q})}^{{w}_{1}})({(1-{({{\mu }^{+}}_{{\stackrel{-}{A}}_{q-SFRS2}\ \ \ \ })}^{q})}^{{w}_{2}}-(1-{({{\mu }^{+}}_{{\stackrel{-}{A}}_{q-SFRS2}\ \ \ \ })}^{q}-\\ {({{\mu }^{-}}_{{\stackrel{-}{A}}_{q-SFRS2}\ \ \ \ })}^{q})^{{w}_{2}})-({(1-{({{\mu }^{+}}_{{\stackrel{-}{A}}_{q-SFRS1}\ \ \ \ })}^{q})}^{{w}_{1}}-{(1-{({{\mu }^{+}}_{{\stackrel{-}{A}}_{q-SFRS1}\ \ \ \ })}^{q}-{({{\mu }^{-}}_{{\stackrel{-}{A}}_{q-SFRS1}\ \ \ \ })}^{\mathrm{q}})}^{{w}_{1}})((1-\\ {({{\mu }^{+}}_{{\stackrel{-}{A}}_{q-SFRS2}\ \ \ \ })}^{q})^{{w}_{2}}-{(1-{({{\mu }^{+}}_{{\stackrel{-}{A}}_{q-SFRS2}\ \ \ \ })}^{q}-{({{\mu }^{-}}_{{\stackrel{-}{A}}_{q-SFRS2}\ \ \ \ })}^{\mathrm{q}})}^{{w}_{2}}))^{\frac{1}{q}}]⟩ , \end{array} |
\begin{array}{c} = ⟨{[1-{(1-{({{\mu }^{+}}_{{\stackrel{-}{A}}_{q-SFRS1}\ \ \ \ })}^{q})}^{{w}_{1}}{(1-{({{\mu }^{+}}_{{\stackrel{-}{A}}_{q-SFRS2}\ \ \ \ })}^{q})}^{{w}_{2}}]}^{\frac{1}{q}}, {({{\mu }^{'}}_{{\stackrel{-}{A}}_{q-SFRS1}\ \ \ \ })}^{{w}_{1}}{({{\mu }^{'}}_{{\stackrel{-}{A}}_{q-SFRS2}\ \ \ \ })}^{{w}_{2}}, [(1-\\ {({{\mu }^{+}}_{{\stackrel{-}{A}}_{q-SFRS1}\ \ \ \ })}^{q})^{{w}_{1}}{(1-{({{\mu }^{+}}_{{\stackrel{-}{A}}_{q-SFRS2}\ \ \ \ })}^{q})}^{{w}_{2}}-{(1-{({{\mu }^{+}}_{{\stackrel{-}{A}}_{q-SFRS1}\ \ \ \ })}^{q}-{({{\mu }^{-}}_{{\stackrel{-}{A}}_{q-SFRS2}\ \ \ \ })}^{q})}^{{w}_{1}}(1-\\ {({{\mu }^{+}}_{{\stackrel{-}{A}}_{q-SFRS2}\ \ \ \ })}^{q}-{({{\mu }^{-}}_{{\stackrel{-}{A}}_{q-SFRS2}\ \ \ \ })}^{q})^{{w}_{2}}]^{\frac{1}{q}}⟩ . \end{array} |
Thus, the result is true for n = 2 . Assume that the result is true for n = k, Eq (1) holds, i.e.,
\begin{array}{l} {\mathrm{q}-\mathrm{S}\mathrm{F}\mathrm{R}\mathrm{A}\mathrm{M}}_{w}({\stackrel{-}{A}}_{q-SFRS1}, {\stackrel{-}{A}}_{q-SFRS2}, {\stackrel{-}{A}}_{q-SFRS3}, \dots , {\stackrel{-}{A}}_{q-SFRSk})\\ \ \ \ \ \ \ \ \ \ \ \ \ \ \ \ \ \ = {w}_{1}{\stackrel{-}{A}}_{q-SFRS1} \oplus {w}_{2}{\stackrel{-}{A}}_{q-SFRS2} \oplus {w}_{3}{\stackrel{-}{A}}_{q-SFRS3} \oplus , \dots , { \oplus w}_{k}{\stackrel{-}{A}}_{q-SFRSk} \end{array} |
\begin{array}{c} = ⟨{[1-\prod _{i = 1}^{k}{(1-{({{\mu }^{+}}_{{\stackrel{-}{A}}_{q-SFRSi}}\ \ \ \ \ )}^{q})}^{{w}_{i}}]}^{\frac{1}{q}}, \prod _{i = 1}^{k}{({{\mu }^{'}}_{{\stackrel{-}{A}}_{q-SFRSi}}\ \ \ \ \ )}^{{w}_{i}}, [\prod _{i = 1}^{k}{(1-{({{\mu }^{+}}_{{\stackrel{-}{A}}_{q-SFRSi}}\ \ \ \ \ )}^{q})}^{{w}_{i}}-\\ \ \ \ \ \ \ \ \prod _{i = 1}^{k}{(1-{({{\mu }^{+}}_{{\stackrel{-}{A}}_{q-SFRSi}}\ \ \ \ \ )}^{q}-{({{\mu }^{-}}_{{\stackrel{-}{A}}_{q-SFRSi}}\ \ \ \ \ )}^{q})}^{{w}_{i}}]^{\frac{1}{q}}⟩ . \end{array} |
Then when n = k+1
\begin{array}{c} {\mathrm{q}-\mathrm{S}\mathrm{F}\mathrm{R}\mathrm{A}\mathrm{M}}_{w}({\stackrel{-}{A}}_{q-SFRS1}, {\stackrel{-}{A}}_{q-SFRS2}, {\stackrel{-}{A}}_{q-SFRS3}, \dots , {\stackrel{-}{A}}_{q-SFRSk}, {\stackrel{-}{A}}_{q-SFRSk+1})\\ = {w}_{1}{\stackrel{-}{A}}_{q-SFRS1} \oplus {w}_{2}{\stackrel{-}{A}}_{q-SFRS2} \oplus {w}_{3}{\stackrel{-}{A}}_{q-SFRS3}\\ \oplus , \dots , { \oplus w}_{k}{\stackrel{-}{A}}_{q-SFRSk}{ \oplus w}_{k+1}{\stackrel{-}{A}}_{q-SFRSk+1} \end{array} |
\begin{array}{c} = ⟨{[1-\prod _{i = 1}^{k}{(1-{({{\mu }^{+}}_{{\stackrel{-}{A}}_{q-SFRSi}}\ \ \ \ \ )}^{q})}^{{w}_{i}}]}^{\frac{1}{q}}, \prod _{i = 1}^{k}{({{\mu }^{'}}_{{\stackrel{-}{A}}_{q-SFRSi}}\ \ \ \ \ )}^{{w}_{i}}, [\prod _{i = 1}^{k}{(1-{({{\mu }^{+}}_{{\stackrel{-}{A}}_{q-SFRSi}}\ \ \ \ \ )}^{q})}^{{w}_{i}}-\\ \prod _{i = 1}^{k}{(1-{({{\mu }^{+}}_{{\stackrel{-}{A}}_{q-SFRSi}}\ \ \ \ \ )}^{q}-{({{\mu }^{-}}_{{\stackrel{-}{A}}_{q-SFRSi}}\ \ \ \ \ )}^{q})}^{{w}_{i}}]^{\frac{1}{q}}⟩ \oplus ⟨[1-(1-\\ {({{\mu }^{+}}_{{\stackrel{-}{A}}_{q-SFRSk+1}\ \ \ \ })}^{q})^{{w}_{k+1}}]^{\frac{1}{q}}, {({{\mu }^{'}}_{{\stackrel{-}{A}}_{q-SFRSk+1}\ \ \ \ })}^{{w}_{k+1}}, [(1-{({{\mu }^{+}}_{{\stackrel{-}{A}}_{q-SFRSk+1}\ \ \ \ })}^{q})^{{w}_{k+1}}-(1-\\ {({{\mu }^{+}}_{{\stackrel{-}{A}}_{q-SFRSk+1}\ \ \ \ })}^{q}-{({{\mu }^{-}}_{{\stackrel{-}{A}}_{q-SFRSk+1}\ \ \ \ })}^{q})^{{w}_{k+1}}]^{\frac{1}{q}}⟩ , \end{array} |
\begin{array}{c} = ⟨{[1-\prod _{i = 1}^{k+1}{(1-{({{\mu }^{+}}_{{\stackrel{-}{A}}_{q-SFRSi}}\ \ \ \ \ )}^{q})}^{{w}_{i}}]}^{\frac{1}{q}}, \prod _{i = 1}^{k+1}{({{\mu }^{'}}_{{\stackrel{-}{A}}_{q-SFRSi}}\ \ \ \ \ )}^{{w}_{i}}, [\prod _{i = 1}^{k+1}{(1-{({{\mu }^{+}}_{{\stackrel{-}{A}}_{q-SFRSi}}\ \ \ \ \ )}^{q})}^{{w}_{i}}-\\ \prod _{i = 1}^{k+1}{(1-{({{\mu }^{+}}_{{\stackrel{-}{A}}_{q-SFRSi}}\ \ \ \ \ )}^{q}-{({{\mu }^{-}}_{{\stackrel{-}{A}}_{q-SFRSi}}\ \ \ \ \ )}^{q})}^{{w}_{i}}]^{\frac{1}{q}}⟩ . \end{array} |
i.e., when n = k+1 Eq (1) also holds and therefore the proof is completed.
[1] |
L. A. Zadeh, Fuzzy sets, Inf. Control, 8 (1965), 338–353. https://doi.org/10.1016/S0019-9958(65)90241-X doi: 10.1016/S0019-9958(65)90241-X
![]() |
[2] |
K. T. Atanassov, Intuitionistic fuzzy sets, Fuzzy Set. Syst., 20 (1986), 87–96. https://doi.org/10.1016/S0165-0114(86)80034-3 doi: 10.1016/S0165-0114(86)80034-3
![]() |
[3] | B. C. Cuong, V. Kreinovich, Picture fuzzy sets, J. Comput. Sci. Cyb., 30 (2014), 409–420. |
[4] |
X. Zhang, P. Liu, Y. Wang, Multiple attribute group decision-making methods based on intuitionistic fuzzy frank power aggregation operators, J. Intell. Fuzzy Syst., 29 (2015), 2235–2246. https://doi.org/10.3233/IFS-151699 doi: 10.3233/IFS-151699
![]() |
[5] |
M. R. Seikh, U. Mandal, Intuitionistic fuzzy Dombi aggregation operators and their application to multiple attribute decision-making, Granular Comput., 6 (2021), 473–488. https://doi.org/10.1007/s41066-019-00209-y doi: 10.1007/s41066-019-00209-y
![]() |
[6] |
S. Zeng, N. Zhang, C. Zhang, W. Su, L. A. Carlos, Social network multiple-criteria decision-making approach for evaluating unmanned ground delivery vehicles under the Pythagorean fuzzy environment, Technol. Forecast. Soc., 175 (2022), 121–414. https://doi.org/10.1016/j.techfore.2021.121414 doi: 10.1016/j.techfore.2021.121414
![]() |
[7] | R. R. Yager, Pythagorean fuzzy subsets, In 2013 joint IFSA world congress and NAFIPS annual meeting (IFSA/NAFIPS), IEEE, 2013, 57–61. https://doi.org/10.1109/IFSA-NAFIPS.2013.6608375 |
[8] |
M. Akram, W. A. Dudek, J. M. Dar, Pythagorean Dombi fuzzy aggregation operators with application in multicriteria decision‐making, Int. J. Intell. Syst., 34 (2019), 3000–3019. https://doi.org/10.1002/int.22183 doi: 10.1002/int.22183
![]() |
[9] |
H. Garg, Confidence levels-based Pythagorean fuzzy aggregation operators and its application to the decision-making process, Comput. Math. Organ. Th., 23 (2017), 546–571. https://doi.org/10.1007/s10588-017-9242-8 doi: 10.1007/s10588-017-9242-8
![]() |
[10] |
L. Wang, H. Garg, Algorithm for multiple attribute decision-making with interactive Archimedean norm operations under Pythagorean fuzzy uncertainty, Int. J. Comput. Intell. Syst., 14 (2021), 503–927. https://doi.org/10.2991/ijcis.d.201215.002 doi: 10.2991/ijcis.d.201215.002
![]() |
[11] |
Q. Wu, W. Lin, L. Zhou, Y. Chen, H. Chen, Enhancing multiple attribute group decision-making flexibility based on information fusion technique and hesitant Pythagorean fuzzy sets, Comput. Ind. Eng., 127 (2019), 954–970. https://doi.org/10.1016/j.cie.2018.11.029 doi: 10.1016/j.cie.2018.11.029
![]() |
[12] |
R. R. Yager, Generalized orthopair fuzzy sets, IEEE T. Fuzzy Syst., 25 (2016), 1222–1230. https://doi.org/10.1109/TFUZZ.2016.2604005 doi: 10.1109/TFUZZ.2016.2604005
![]() |
[13] |
Y. Xing, R. Zhang, Z. Zhou, J. Wang, Some q-rung orthopair fuzzy point weighted aggregation operators for multi-attribute decision making, Soft Comput., 23 (2019), 11627–11649. https://doi.org/10.1007/s00500-018-03712-7 doi: 10.1007/s00500-018-03712-7
![]() |
[14] |
P. Liu, P. Wang, Some q‐rung orthopair fuzzy aggregation operators and their applications to multiple‐attribute decision making, Int. J. Intell. Syst., 33 (2018), 259–280. https://doi.org/10.1002/int.21927 doi: 10.1002/int.21927
![]() |
[15] | B. C. Cuong, V. Kreinovich, Picture fuzzy sets-a new concept for computational intelligence problems, In 2013 third world congress on information and communication technologies (WICT 2013), IEEE, 2013, 1–6. https://doi.org/10.1109/WICT.2013.7113099 |
[16] |
G. Wei, Picture fuzzy Hamacher aggregation operators and their application to multiple attribute decision making, Fund. Inform., 157 (2018), 271–320. https://doi.org/10.3233/FI-2018-1628 doi: 10.3233/FI-2018-1628
![]() |
[17] |
T. Mahmood, K. Ullah, Q. Khan, N. Jan, An approach toward decision-making and medical diagnosis problems using the concept of spherical fuzzy sets, Neural Comput. Appl., 31 (2019), 7041–7053. https://doi.org/10.1007/s00521-018-3521-2 doi: 10.1007/s00521-018-3521-2
![]() |
[18] | I. Deli, N. Çağman, Spherical fuzzy numbers and multi-criteria decision-making, In Decision Making with Spherical Fuzzy Sets, Springer, 392 (2021), 53–84. https://doi.org/10.1007/978-3-030-45461-6_3 |
[19] |
M. Rafiq, S. Ashraf, S. Abdullah, T. Mahmood, S. Muhammad, The cosine similarity measures of spherical fuzzy sets and their applications in decision making, J. Intell. Fuzzy Syst., 36 (2019), 6059–6073. https://doi.org/10.3233/JIFS-181922 doi: 10.3233/JIFS-181922
![]() |
[20] |
S. Ashraf, S. Abdullah, M. Aslam, Symmetric sum-based aggregation operators for spherical fuzzy information: Application in multi-attribute group decision making problem, J. Intell. Fuzzy Syst., 38 (2020), 5241–5255. https://doi.org/10.3233/JIFS-191819 doi: 10.3233/JIFS-191819
![]() |
[21] | C. Kahraman, B. Oztaysi, S. C. Onar, I. Otay, q-spherical fuzzy sets and their usage in multi-attribute decision making, In Developments of Artificial Intelligence Technologies in Computation and Robotics, World Scientific, 12 (2020), 217–225. https://doi.org/10.1142/9789811223334_0027 |
[22] |
Z. Pawlak, Rough sets, Int. J. Comput. Inform. Sci., 11 (1982), 341–356. https://doi.org/10.1007/BF01001956 doi: 10.1007/BF01001956
![]() |
[23] |
Z. Pawlak, Rough set theory and its applications to data analysis, Cybern. Syst., 29 (1998), 688–1998. https://doi.org/10.1080/019697298125470 doi: 10.1080/019697298125470
![]() |
[24] |
Y. Yao, Constructive and algebraic methods of the theory of rough sets, Inf. Sci., 109 (1998), 21–47. https://doi.org/10.1016/S0020-0255(98)00012-7 doi: 10.1016/S0020-0255(98)00012-7
![]() |
[25] |
J. Dai, S. Gao, G. Zheng, Generalized rough set models determined by multiple neighborhoods generated from a similarity relation, Soft Comput., 22 (2018), 2081–2094. https://doi.org/10.1007/s00500-017-2672-x doi: 10.1007/s00500-017-2672-x
![]() |
[26] |
A. M. Radzikowska, E. E. Kerre, A comparative study of fuzzy rough sets, Fuzzy Set. Syst., 126 (2002), 137–155. https://doi.org/10.1016/S0165-0114(01)00032-X doi: 10.1016/S0165-0114(01)00032-X
![]() |
[27] |
W. Pan, K. She, P. Wei, Multigranulation fuzzy preference relation rough set for ordinal decision system, Fuzzy Set. Syst., 312 (2017), 87–108. https://doi.org/10.1016/j.fss.2016.08.002 doi: 10.1016/j.fss.2016.08.002
![]() |
[28] |
Y. Li, S. Wu, Y. Lin, J. Liu, Different classes' ratio fuzzy rough set based robust feature selection, Knowl.-Based Syst., 120 (2017), 74–86. https://doi.org/10.1016/j.knosys.2016.12.024 doi: 10.1016/j.knosys.2016.12.024
![]() |
[29] |
T. Feng, H. T. Fan, J. S. Mi, Uncertainty, and reduction of variable precision multigranulation fuzzy rough sets based on three-way decisions, Int. J. Approx. Reason., 85 (2017), 36–58. https://doi.org/10.1016/j.ijar.2017.03.002 doi: 10.1016/j.ijar.2017.03.002
![]() |
[30] |
B. Sun, W. Ma, Y. Qian, Multigranulation fuzzy rough set over two universes and its application to decision making, Knowl.-Based Syst., 123 (2017), 61–74. https://doi.org/10.1016/j.knosys.2017.01.036 doi: 10.1016/j.knosys.2017.01.036
![]() |
[31] |
C. Liu, W. Pedrycz, F. Jiang, M. Wang, Decision-theoretic rough set approaches to multi-covering approximation spaces based on fuzzy probability measure, J. Intell. Fuzzy Syst., 34 (2018), 1917–1931. https://doi.org/10.3233/JIFS-171275 doi: 10.3233/JIFS-171275
![]() |
[32] |
H. Zhang, L. Shu, Generalized interval-valued fuzzy rough set and its application in decision making, Int. J. Fuzzy Syst., 17 (2015), 279–291. https://doi.org/10.1007/s40815-015-0012-9 doi: 10.1007/s40815-015-0012-9
![]() |
[33] |
H. Zhang, L. Shu, S. Liao, C. Xiawu, Dual hesitant fuzzy rough set and its application, Soft Comput., 21 (2017), 3287–3305. https://doi.org/10.1007/s00500-015-2008-7 doi: 10.1007/s00500-015-2008-7
![]() |
[34] |
C. Y. Wang, B. Q. Hu, Granular variable precision fuzzy rough sets with general fuzzy relations, Fuzzy Set. Syst., 275 (2015), 39–57. https://doi.org/10.1016/j.fss.2015.01.016 doi: 10.1016/j.fss.2015.01.016
![]() |
[35] |
S. Vluymans, D. S. Tarrago, Y. Saeys, C. Cornelis, F. Herrera, Fuzzy rough classifiers for class imbalanced multi-instance data, Pattern Recogn., 53 (2016), 36–45. https://doi.org/10.1016/j.patcog.2015.12.002 doi: 10.1016/j.patcog.2015.12.002
![]() |
[36] |
T. Shaheen, M. I. Ali, M. Shabir, Generalized hesitant fuzzy rough sets (GHFRS) and their application in risk analysis, Soft Comput., 24 (2020), 14005–14017. https://doi.org/10.1007/s00500-020-04776-0 doi: 10.1007/s00500-020-04776-0
![]() |
[37] |
M. A. Khan, S. Ashraf, S. Abdullah, F. Ghani, Applications of probabilistic hesitant fuzzy rough set in decision support system, Soft Comput., 24 (2020), 16759–16774. https://doi.org/10.1007/s00500-020-04971-z doi: 10.1007/s00500-020-04971-z
![]() |
[38] |
G. Tang, F. Chiclana, P. Liu, A decision-theoretic rough set model with q-rung orthopair fuzzy information and its application in stock investment evaluation, Appl. Soft Comput., 91 (2020), 106–212. https://doi.org/10.1016/j.asoc.2020.106212 doi: 10.1016/j.asoc.2020.106212
![]() |
[39] |
D. Liang, W. Cao, q‐Rung orthopair fuzzy sets‐based decision‐theoretic rough sets for three‐way decisions under group decision making, Int. J. Intell. Syst., 34 (2019), 3139–3167. https://doi.org/10.1002/int.22187 doi: 10.1002/int.22187
![]() |
[40] |
Z. Zhang, S. M. Chen, Group decision making with incomplete q-rung orthopair fuzzy preference relations, Inf. Sci., 553 (2021), 376–396. https://doi.org/10.1016/j.ins.2020.10.015 doi: 10.1016/j.ins.2020.10.015
![]() |
[41] |
J. Zhan, B. Sun, Covering-based intuitionistic fuzzy rough sets and applications in multi-attribute decision-making, Artif. Intell. Rev., 53 (2020), 671–701. https://doi.org/10.1007/s10462-018-9674-7 doi: 10.1007/s10462-018-9674-7
![]() |
[42] |
B. Sun, S. Tong, W. Ma, T. Wang, C. Jiang, An approach to MCGDM based on multi-granulation Pythagorean fuzzy rough set over two universes and its application to the medical decision problem, Artif. Intell. Rev., 55 (2022), 1887–1913. https://doi.org/10.1007/s10462-021-10048-6 doi: 10.1007/s10462-021-10048-6
![]() |
[43] |
H. Garg, M. Atef, Cq-ROFRS: Covering q-rung orthopair fuzzy rough sets and its application to the multi-attribute decision-making process, Complex Intell. Syst., 8 (2022), 2349–2370. https://doi.org/10.1007/s40747-021-00622-4 doi: 10.1007/s40747-021-00622-4
![]() |
[44] |
S. Ashraf, N. Rehman, H. AlSalman, A. H. Gumaei, A decision-making framework using q-rung orthopair probabilistic hesitant fuzzy rough aggregation information for the drug selection to treat COVID-19, Complexity, 2022 (2022). https://doi.org/10.1155/2022/5556309 doi: 10.1155/2022/5556309
![]() |
[45] |
C. N. Huang, J. J. Liou, H. W. Lo, F. J. Chang, Building an assessment model for measuring airport resilience, J. Air Transp. Manag., 95 (2021) 102101. https://doi.org/10.1016/j.jairtraman.2021.102101 doi: 10.1016/j.jairtraman.2021.102101
![]() |
[46] |
H. W. Lo, C. C. Hsu, C. N. Huang, J. J. Liou, An ITARA-TOPSIS based integrated assessment model to identify potential product and system risks, Mathematics, 9 (2021), 239. https://doi.org/10.3390/math9030239 doi: 10.3390/math9030239
![]() |
[47] |
H. W. Lo, J. J. Liou, C. N. Huang, Y. C. Chuang, A novel failure mode and effect analysis model for machine tool risk analysis, Reliab. Eng. Syst. Safe., 183 (2021), 173–183. https://doi.org/10.1016/j.ress.2018.11.018 doi: 10.1016/j.ress.2018.11.018
![]() |
[48] |
M. Lin, C. Huang, Z. Xu, R. Chen, Evaluating IoT platforms using integrated probabilistic linguistic MCDM method, IEEE Internet Things J., 7 (2020), 11195–11208. https://doi.org/10.1109/JIOT.2020.2997133 doi: 10.1109/JIOT.2020.2997133
![]() |
[49] |
X. Q. Xu, J. L. Xie, N. Yue, H. H. Wang, Probabilistic uncertain linguistic TODIM method based on the generalized Choquet integral and its application, Int. J. Intell. Comput. Cyb., 14 (2021), 122–144. https://doi.org/10.1108/IJICC-09-2020-0108 doi: 10.1108/IJICC-09-2020-0108
![]() |
[50] |
Z. Xu, Intuitionistic fuzzy aggregation operators, IEEE T. Fuzzy Syst., 15 (2007), 1179–1187. https://doi.org/10.1109/TFUZZ.2006.890678 doi: 10.1109/TFUZZ.2006.890678
![]() |
[51] |
R. M. Zulqarnain, X. L. Xin, H. Garg, W. A. Khan, Aggregation operators of Pythagorean fuzzy soft sets with their application for green supplier chain management, J. Intell. Fuzzy Syst., 40 (2021), 5545–5563. https://doi.org/10.3233/JIFS-202781 doi: 10.3233/JIFS-202781
![]() |
[52] |
X. Peng, Z. Luo, A review of q-rung orthopair fuzzy information: Bibliometrics and future directions, Artif. Intell. Rev., 54 (2021), 3361–3430. https://doi.org/10.1007/s10462-020-09926-2 doi: 10.1007/s10462-020-09926-2
![]() |
[53] |
Y. Wang, A. Hussain, T. Mahmood, M. I. Ali, H. Wu, Y. Jin, Decision-making based on q-rung orthopair fuzzy soft rough sets, Math. Probl. Eng., 2020 (2020). https://doi.org/10.1155/2020/6671001 doi: 10.1155/2020/6671001
![]() |
[54] |
L. Zheng, T. Mahmood, J. Ahmmad, U. U. Rehman, S. Zeng, Spherical fuzzy soft rough average aggregation operators and their applications to multi-criteria decision making, IEEE Access, 10 (2022), 27832–27852. https://doi.org/10.1109/ACCESS.2022.3150858 doi: 10.1109/ACCESS.2022.3150858
![]() |
1. | Ahmad Bin Azim, Ahmad ALoqaily, Asad Ali, Sumbal Ali, Nabil Mlaiki, Industry 4.0 project prioritization by using q-spherical fuzzy rough analytic hierarchy process, 2023, 8, 2473-6988, 18809, 10.3934/math.2023957 | |
2. | Ahmad Bin Azim, Asad Ali, Abdul Samad Khan, Sumbal Ali, Fuad A. Awwad, Emad A. A. Ismail, q-Spherical Fuzzy Rough Einstein Geometric Aggregation Operator for Image Understanding and Interpretations, 2024, 12, 2169-3536, 140380, 10.1109/ACCESS.2024.3423387 | |
3. | Ahmad Bin Azim, Asad Ali, Sumbal Ali, Ahmad Aloqaily, Nabil Mlaiki, q-Spherical Fuzzy Rough Frank Aggregation Operators in AI Neural Networks: Applications in Military Transport Systems, 2024, 12, 2169-3536, 104215, 10.1109/ACCESS.2024.3414845 | |
4. | Sumbal Ali, Asad Ali, Ahmad Bin Azim, Ahmad Aloqaily, Nabil Mlaiki, Utilizing aggregation operators based on q-rung orthopair neutrosophic soft sets and their applications in multi-attributes decision making problems, 2024, 10, 24058440, e35059, 10.1016/j.heliyon.2024.e35059 | |
5. | Ahmad Bin Azim, Asad Ali, Abdul Samad Khan, Fuad A. Awwad, Emad A.A. Ismail, Sumbal Ali, Utilizing sine trigonometric q-spherical fuzzy rough aggregation operators for group decision-making and their role in digital transformation, 2024, 10, 24058440, e30758, 10.1016/j.heliyon.2024.e30758 | |
6. | Ahmad Bin Azim, Asad Ali, Abdul Samad Khan, Fuad A. Awwad, Emad A.A. Ismail, Sumbal Ali, Assessing indoor positioning system: A q-spherical fuzzy rough TOPSIS analysis, 2024, 10, 24058440, e31018, 10.1016/j.heliyon.2024.e31018 | |
7. | Ahmad Bin Azim, Asad Ali, Abdul Samad Khan, Fuad A. Awwad, Sumbal Ali, Emad A.A. Ismail, Aggregation operators based on Einstein averaging under q-spherical fuzzy rough sets and their applications in navigation systems for automatic cars, 2024, 10, 24058440, e34698, 10.1016/j.heliyon.2024.e34698 | |
8. | Brajamohan Sahoo, Bijoy Krishna Debnath, A novel hybrid spherical fuzzy multi-criteria decision-making approach to select the best hydroelectric power plant source in India, 2024, 51, 17550084, 100650, 10.1016/j.ref.2024.100650 | |
9. | Ahmad Bin Azim, Asad Ali, Abdul Samad Khan, Fuad A. Awwad, Sumbal Ali, Emad A. A. Ismail, Applications of q-Spherical Fuzzy Rough CODAS to the Assessment of a Problem Involving Renewable Energy Site Selection, 2024, 12, 2169-3536, 114100, 10.1109/ACCESS.2024.3412193 | |
10. | Rabia Mazhar, Shahida Bashir, Muhammad Shabir, Mohammed Al-Shamiri, A soft relation approach to approximate the spherical fuzzy ideals of semigroups, 2025, 10, 2473-6988, 3734, 10.3934/math.2025173 |
R | {a{_1}} | {a{_2}} | {a{_3}} | {a{_4}} |
{h{_1}} | {{\text{(0}}{\text{.7, 0}}{\text{.2, 0}}{\text{.4)}}} | {{\text{(0}}{\text{.5, 0}}{\text{.2, 0}}{\text{.7)}}} | {{\text{(0}}{\text{.6, 0}}{\text{.5, 0}}{\text{.3)}}} | {{\text{(0}}{\text{.7, 0}}{\text{.2, 0}}{\text{.5)}}} |
{h{_2}} | {{\text{(0}}{\text{.6, 0}}{\text{.3, 0}}{\text{.1)}}} | {{\text{(0}}{\text{.3, 0}}{\text{.2, 0}}{\text{.5)}}} | {{\text{(0}}{\text{.3, 0}}{\text{.2, 0}}{\text{.4)}}} | {{\text{(0}}{\text{.5, 0}}{\text{.2, 0}}{\text{.5)}}} |
{h{_3}} | {{\text{(0}}{\text{.3, 0}}{\text{.1, 0}}{\text{.2)}}} | {{\text{(0}}{\text{.7, 0}}{\text{.4, 0}}{\text{.3)}}} | {{\text{(0}}{\text{.2, 0}}{\text{.3, 0}}{\text{.7)}}} | {{\text{(0}}{\text{.3, 0}}{\text{.4, 0}}{\text{.1)}}} |
{h{_4}} | {{\text{(0}}{\text{.4, 0}}{\text{.5, 0}}{\text{.2)}}} | {{\text{(0}}{\text{.8, 0}}{\text{.4, 0}}{\text{.5)}}} | {{\text{(0}}{\text{.5, 0}}{\text{.3, 0}}{\text{.4)}}} | {{\text{(0}}{\text{.4, 0}}{\text{.3, 0}}{\text{.2)}}} |
{h{_5}} | {{\text{(0}}{\text{.6, 0}}{\text{.1, 0}}{\text{.5)}}} | {{\text{(0}}{\text{.4, 0}}{\text{.3, 0}}{\text{.5)}}} | {{\text{(0}}{\text{.9, 0}}{\text{.3, 0}}{\text{.5)}}} | {{\text{(0}}{\text{.3, 0}}{\text{.2, 0}}{\text{.1)}}} |
{{C_1}} | {{C_2}} | {{C_3}} | |
{{A_1}} | {\left\langle {(0.8, \, 0.4, \, 0.3), (0.7, \, 0.5, \, 0.2)} \right\rangle } | {\left\langle {(0.7, \, 0.4, \, 0.2), (0.6, \, 0.3, \, 0.4)} \right\rangle } | {\left\langle {(0.7, \, 0.3, \, 0.3), (0.8, \, 0.4, \, 0.4)} \right\rangle } |
{{A_2}} | {\left\langle {(0.9, \, 0.2, \, 0.1), (0.8, \, 0.3, \, 0.2)} \right\rangle } | {\left\langle {(0.8, \, 0.2, \, 0.3), (0.5, \, 0.4, \, 0.1)} \right\rangle } | {\left\langle {(0.8, \, 0.4, \, 0.2), (0.9, \, 0.3, \, 0.2)} \right\rangle } |
{{A_3}} | {\left\langle {(0.8, \, 0.4, 0.3), (0.9, \, 0.5, 0.1)} \right\rangle } | {\left\langle {(0.8, \, 0.4, \, 0.3), (0.7, \, 0.3, \, 0.2)} \right\rangle } | {\left\langle {(0.8, \, 0.4, \, 0.3), (0.4, \, 0.4, \, 0.2)} \right\rangle } |
{{A_4}} | {\left\langle {(0.7, \, 0.4, \, 0.5), (0.7, \, 0.6, \, 0.4)} \right\rangle } | {\left\langle {(0.9, \, 0.4, \, 0.3), (0.7, \, 0.2, \, 0.1)} \right\rangle } | {\left\langle {(0.7, \, 0.3, \, 0.3), (0.6, \, 0.1, \, 0.2)} \right\rangle } |
{{C_1}} | {{C_2}} | {{C_3}} | |
{{A_1}} | {\left\langle {(0.3, \, 0.4, \, 0.8), (0.2, \, 0.5, \, 0.7)} \right\rangle } | {\left\langle {(0.7, \, 0.4, \, 0.2), (0.6, \, 0.3, \, 0.4)} \right\rangle } | {\left\langle {(0.3, 0.3, 0.7\, ), (0.4, \, 0.4, \, 0.8)} \right\rangle } |
{{A_2}} | {\left\langle {(\, 0.1, 0.2, 0.9), (0.2, \, 0.3, \, 0.8)} \right\rangle } | {\left\langle {(0.8, \, 0.2, \, 0.3), (0.5, \, 0.4, \, 0.1)} \right\rangle } | {\left\langle {(0.2, \, 0.4, \, 0.8, ), (0.2, \, 0.3, \, 0.9)} \right\rangle } |
{{A_3}} | {\left\langle {(0.3, \, 0.4, 0.8), (0.1, \, 0.5, 0.9)} \right\rangle } | {\left\langle {(0.8, \, 0.4, \, 0.3), (0.7, \, 0.3, \, 0.2)} \right\rangle } | {\left\langle {(0.3, \, 0.4, \, 0.8), (0.2, 0.4, \, 0.4)} \right\rangle } |
{{A_4}} | {\left\langle {(0.5, \, 0.4, 0.7\, ), (0.4, 0.6, 0.7\, )} \right\rangle } | {\left\langle {(0.9, \, 0.4, \, 0.3), (0.7, \, 0.2, \, 0.1)} \right\rangle } | {\left\langle {(0.3, \, 0.3, \, 0.7), (0.2, 0.1, \, 0.6)} \right\rangle } |
{{\text{Methods}}} | {{\text{Score values}}} | {{\text{Ranking Order}}} |
{{\text{IFWA}}} [50] | {\left({0.22016, \, 0.33214, \, 0.21173, \, 0.27007} \right)} | {{S_2} \succ {S_4} \succ {S_1} \succ {S_3}} |
{{\text{IFWG}}} [50] | {(0.46119, \, \; 0.5572, \, 0.49917, \, 0.55418)} | {{S_2} \succ {S_4} \succ {S_3} \succ {S_1}} |
{{\text{PFSWA}}} [51] | {(0.52209, \, \; 0.62190, \, 0.56596, \, 0.59380)} | {{S_2} \succ {S_4} \succ {S_3} \succ {S_1}} |
{{\text{PFSWG}}} [51] | {(0.46427, \, \; 0.57343, \, 0.55374, \, 0.51474)} | {{S_2} \succ {S_3} \succ {S_4} \succ {S_1}} |
{{\text{q - ROFWA}}} [52] | {(0.09958, \, \; 0.14761, \, 0.07984, \, 0.08157)} | {{S_2} \succ {S_1} \succ {S_4} \succ {S_3}} |
{{\text{q - ROFWG}}} [52] | {(0.29865, \, \; 0.40678, \, 0.35021, \, 0.38045)} | {{S_2} \succ {S_1} \succ {S_4} \succ {S_3}} |
{{\text{q - ROFS}}{_{\text{t}}}{\text{RWA}}} [53] | {(0.226074, \, \; 0.298025, \, \; 0.241341)} | {{S_2} \succ {S_3} \succ {S_1}} |
{{\text{q - ROFS}}{_{\text{t}}}{\text{RWG}}} [53] | {(0.06616, \, \; 0.135502, \, \; 0.118744)} | {{S_2} \succ {S_3} \succ {S_1}} |
{{\text{SFS}}{_{\text{t}}}{\text{RWA}}} [54] | {(0.4477, \, \; 0.5337, \, \; 0.5188)} | {{S_2} \succ {S_3} \succ {S_1}} |
{{\text{SFS}}{_{\text{t}}}{\text{ROWA}}} [54] | {(0.4317, \, \; 0.5412, \, \; 0.4977)} | {{S_2} \succ {S_3} \succ {S_1}} |
{{\text{SFS}}{_{\text{t}}}{\text{RHA}}} [54] | {(0.4454, \, 0.5202, \, \; 0.4711)} | {{S_2} \succ {S_3} \succ {S_1}} |
{{\text{q - SFRWA }}{\left({{\text{proposed}}} \right)}} | {(0.5397, \, 0.4746, \, 0.5937, \, 0.7382)} | {{S_4} \succ {S_3} \succ {S_1} \succ {S_2}} |
{{\text{q - SFRWG }}{\left({{\text{proposed}}} \right)}} | {(0.4684, \, 0.3455, \, 0.4350, \, 0.5526)} | {{S_4} \succ {S_1} \succ {S_3} \succ {S_2}} |
{{\text{Approches}}} | {{\text{Fuzzy information}}} | {{\text{Parameters information}}} | {{\text{Lower and upper approximation information}}} |
{{\text{IFWA}}} [50] | √ | × | × |
{{\text{IFWG}}} [50] | √ | × | × |
{{\text{PFSWA}}} [51] | √ | √ | × |
{{\text{PFSWG}}} [51] | √ | √ | × |
{{\text{q - ROFWA}}} [52] | √ | × | × |
{{\text{q - ROFWG}}} [52] | √ | × | × |
{{\text{q - ROFS}}{_{\text{t}}}{\text{RWA}}} [53] | √ | √ | √ |
{{\text{q - ROFS}}{_{\text{t}}}{\text{RWG}}} [53] | √ | √ | √ |
{{\text{SFS}}{_{\text{t}}}{\text{RWA}}} [54] | √ | √ | √ |
{{\text{SFS}}{_{\text{t}}}{\text{ROWA}}} [54] | √ | √ | √ |
{{\text{SFS}}{_{\text{t}}}{\text{RHA}}} [54] | √ | √ | √ |
{{\text{q - SFRWA }}{\left({{\text{proposed}}} \right)}} | √ | √ | √ |
{{\text{q - SFRWG }}{\left({{\text{proposed}}} \right)}} | √ | √ | √ |
R | {a{_1}} | {a{_2}} | {a{_3}} | {a{_4}} |
{h{_1}} | {{\text{(0}}{\text{.7, 0}}{\text{.2, 0}}{\text{.4)}}} | {{\text{(0}}{\text{.5, 0}}{\text{.2, 0}}{\text{.7)}}} | {{\text{(0}}{\text{.6, 0}}{\text{.5, 0}}{\text{.3)}}} | {{\text{(0}}{\text{.7, 0}}{\text{.2, 0}}{\text{.5)}}} |
{h{_2}} | {{\text{(0}}{\text{.6, 0}}{\text{.3, 0}}{\text{.1)}}} | {{\text{(0}}{\text{.3, 0}}{\text{.2, 0}}{\text{.5)}}} | {{\text{(0}}{\text{.3, 0}}{\text{.2, 0}}{\text{.4)}}} | {{\text{(0}}{\text{.5, 0}}{\text{.2, 0}}{\text{.5)}}} |
{h{_3}} | {{\text{(0}}{\text{.3, 0}}{\text{.1, 0}}{\text{.2)}}} | {{\text{(0}}{\text{.7, 0}}{\text{.4, 0}}{\text{.3)}}} | {{\text{(0}}{\text{.2, 0}}{\text{.3, 0}}{\text{.7)}}} | {{\text{(0}}{\text{.3, 0}}{\text{.4, 0}}{\text{.1)}}} |
{h{_4}} | {{\text{(0}}{\text{.4, 0}}{\text{.5, 0}}{\text{.2)}}} | {{\text{(0}}{\text{.8, 0}}{\text{.4, 0}}{\text{.5)}}} | {{\text{(0}}{\text{.5, 0}}{\text{.3, 0}}{\text{.4)}}} | {{\text{(0}}{\text{.4, 0}}{\text{.3, 0}}{\text{.2)}}} |
{h{_5}} | {{\text{(0}}{\text{.6, 0}}{\text{.1, 0}}{\text{.5)}}} | {{\text{(0}}{\text{.4, 0}}{\text{.3, 0}}{\text{.5)}}} | {{\text{(0}}{\text{.9, 0}}{\text{.3, 0}}{\text{.5)}}} | {{\text{(0}}{\text{.3, 0}}{\text{.2, 0}}{\text{.1)}}} |
{{C_1}} | {{C_2}} | {{C_3}} | |
{{A_1}} | {\left\langle {(0.8, \, 0.4, \, 0.3), (0.7, \, 0.5, \, 0.2)} \right\rangle } | {\left\langle {(0.7, \, 0.4, \, 0.2), (0.6, \, 0.3, \, 0.4)} \right\rangle } | {\left\langle {(0.7, \, 0.3, \, 0.3), (0.8, \, 0.4, \, 0.4)} \right\rangle } |
{{A_2}} | {\left\langle {(0.9, \, 0.2, \, 0.1), (0.8, \, 0.3, \, 0.2)} \right\rangle } | {\left\langle {(0.8, \, 0.2, \, 0.3), (0.5, \, 0.4, \, 0.1)} \right\rangle } | {\left\langle {(0.8, \, 0.4, \, 0.2), (0.9, \, 0.3, \, 0.2)} \right\rangle } |
{{A_3}} | {\left\langle {(0.8, \, 0.4, 0.3), (0.9, \, 0.5, 0.1)} \right\rangle } | {\left\langle {(0.8, \, 0.4, \, 0.3), (0.7, \, 0.3, \, 0.2)} \right\rangle } | {\left\langle {(0.8, \, 0.4, \, 0.3), (0.4, \, 0.4, \, 0.2)} \right\rangle } |
{{A_4}} | {\left\langle {(0.7, \, 0.4, \, 0.5), (0.7, \, 0.6, \, 0.4)} \right\rangle } | {\left\langle {(0.9, \, 0.4, \, 0.3), (0.7, \, 0.2, \, 0.1)} \right\rangle } | {\left\langle {(0.7, \, 0.3, \, 0.3), (0.6, \, 0.1, \, 0.2)} \right\rangle } |
{{C_1}} | {{C_2}} | {{C_3}} | |
{{A_1}} | {\left\langle {(0.3, \, 0.4, \, 0.8), (0.2, \, 0.5, \, 0.7)} \right\rangle } | {\left\langle {(0.7, \, 0.4, \, 0.2), (0.6, \, 0.3, \, 0.4)} \right\rangle } | {\left\langle {(0.3, 0.3, 0.7\, ), (0.4, \, 0.4, \, 0.8)} \right\rangle } |
{{A_2}} | {\left\langle {(\, 0.1, 0.2, 0.9), (0.2, \, 0.3, \, 0.8)} \right\rangle } | {\left\langle {(0.8, \, 0.2, \, 0.3), (0.5, \, 0.4, \, 0.1)} \right\rangle } | {\left\langle {(0.2, \, 0.4, \, 0.8, ), (0.2, \, 0.3, \, 0.9)} \right\rangle } |
{{A_3}} | {\left\langle {(0.3, \, 0.4, 0.8), (0.1, \, 0.5, 0.9)} \right\rangle } | {\left\langle {(0.8, \, 0.4, \, 0.3), (0.7, \, 0.3, \, 0.2)} \right\rangle } | {\left\langle {(0.3, \, 0.4, \, 0.8), (0.2, 0.4, \, 0.4)} \right\rangle } |
{{A_4}} | {\left\langle {(0.5, \, 0.4, 0.7\, ), (0.4, 0.6, 0.7\, )} \right\rangle } | {\left\langle {(0.9, \, 0.4, \, 0.3), (0.7, \, 0.2, \, 0.1)} \right\rangle } | {\left\langle {(0.3, \, 0.3, \, 0.7), (0.2, 0.1, \, 0.6)} \right\rangle } |
{{\text{Methods}}} | {{\text{Score values}}} | {{\text{Ranking Order}}} |
{{\text{IFWA}}} [50] | {\left({0.22016, \, 0.33214, \, 0.21173, \, 0.27007} \right)} | {{S_2} \succ {S_4} \succ {S_1} \succ {S_3}} |
{{\text{IFWG}}} [50] | {(0.46119, \, \; 0.5572, \, 0.49917, \, 0.55418)} | {{S_2} \succ {S_4} \succ {S_3} \succ {S_1}} |
{{\text{PFSWA}}} [51] | {(0.52209, \, \; 0.62190, \, 0.56596, \, 0.59380)} | {{S_2} \succ {S_4} \succ {S_3} \succ {S_1}} |
{{\text{PFSWG}}} [51] | {(0.46427, \, \; 0.57343, \, 0.55374, \, 0.51474)} | {{S_2} \succ {S_3} \succ {S_4} \succ {S_1}} |
{{\text{q - ROFWA}}} [52] | {(0.09958, \, \; 0.14761, \, 0.07984, \, 0.08157)} | {{S_2} \succ {S_1} \succ {S_4} \succ {S_3}} |
{{\text{q - ROFWG}}} [52] | {(0.29865, \, \; 0.40678, \, 0.35021, \, 0.38045)} | {{S_2} \succ {S_1} \succ {S_4} \succ {S_3}} |
{{\text{q - ROFS}}{_{\text{t}}}{\text{RWA}}} [53] | {(0.226074, \, \; 0.298025, \, \; 0.241341)} | {{S_2} \succ {S_3} \succ {S_1}} |
{{\text{q - ROFS}}{_{\text{t}}}{\text{RWG}}} [53] | {(0.06616, \, \; 0.135502, \, \; 0.118744)} | {{S_2} \succ {S_3} \succ {S_1}} |
{{\text{SFS}}{_{\text{t}}}{\text{RWA}}} [54] | {(0.4477, \, \; 0.5337, \, \; 0.5188)} | {{S_2} \succ {S_3} \succ {S_1}} |
{{\text{SFS}}{_{\text{t}}}{\text{ROWA}}} [54] | {(0.4317, \, \; 0.5412, \, \; 0.4977)} | {{S_2} \succ {S_3} \succ {S_1}} |
{{\text{SFS}}{_{\text{t}}}{\text{RHA}}} [54] | {(0.4454, \, 0.5202, \, \; 0.4711)} | {{S_2} \succ {S_3} \succ {S_1}} |
{{\text{q - SFRWA }}{\left({{\text{proposed}}} \right)}} | {(0.5397, \, 0.4746, \, 0.5937, \, 0.7382)} | {{S_4} \succ {S_3} \succ {S_1} \succ {S_2}} |
{{\text{q - SFRWG }}{\left({{\text{proposed}}} \right)}} | {(0.4684, \, 0.3455, \, 0.4350, \, 0.5526)} | {{S_4} \succ {S_1} \succ {S_3} \succ {S_2}} |
{{\text{Approches}}} | {{\text{Fuzzy information}}} | {{\text{Parameters information}}} | {{\text{Lower and upper approximation information}}} |
{{\text{IFWA}}} [50] | √ | × | × |
{{\text{IFWG}}} [50] | √ | × | × |
{{\text{PFSWA}}} [51] | √ | √ | × |
{{\text{PFSWG}}} [51] | √ | √ | × |
{{\text{q - ROFWA}}} [52] | √ | × | × |
{{\text{q - ROFWG}}} [52] | √ | × | × |
{{\text{q - ROFS}}{_{\text{t}}}{\text{RWA}}} [53] | √ | √ | √ |
{{\text{q - ROFS}}{_{\text{t}}}{\text{RWG}}} [53] | √ | √ | √ |
{{\text{SFS}}{_{\text{t}}}{\text{RWA}}} [54] | √ | √ | √ |
{{\text{SFS}}{_{\text{t}}}{\text{ROWA}}} [54] | √ | √ | √ |
{{\text{SFS}}{_{\text{t}}}{\text{RHA}}} [54] | √ | √ | √ |
{{\text{q - SFRWA }}{\left({{\text{proposed}}} \right)}} | √ | √ | √ |
{{\text{q - SFRWG }}{\left({{\text{proposed}}} \right)}} | √ | √ | √ |