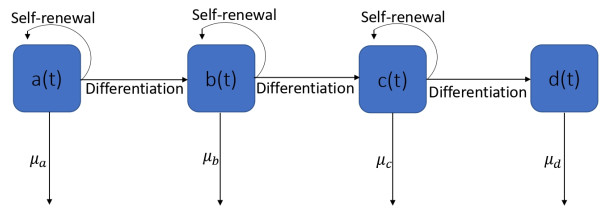
In this study, a mathematical model for the differentiation of stem cells is proposed to understand the dynamics of cell differentiation in a complex network. For this, myeloid cells, which are differentiated from stem cells, are introduced in this study. We introduce the threshold quantity R0 to understand the population dynamics of stem cells. The local stability analysis of three equilibria, namely (i) free equilibrium points, (ii) absence of stem and progenitor cells, and (iii) endemic equilibrium points are investigated in this study. The model is first formulated in non-fractional order and after that converted into a fractional sense by utilizing the Atangana-Baleanu derivative in Caputo (ABC) sense in the form of a non-singular kernel. The model is solved by using numerical techniques. It is seen that the myeloid cell population significantly affects the stem cell population.
Citation: Ram Singh, Attiq U. Rehman, Mehedi Masud, Hesham A. Alhumyani, Shubham Mahajan, Amit K. Pandit, Praveen Agarwal. Fractional order modeling and analysis of dynamics of stem cell differentiation in complex network[J]. AIMS Mathematics, 2022, 7(4): 5175-5198. doi: 10.3934/math.2022289
[1] | Noufe H. Aljahdaly, R. A. Alharbey . Fractional numerical simulation of mathematical model of HIV-1 infection with stem cell therapy. AIMS Mathematics, 2021, 6(7): 6715-6725. doi: 10.3934/math.2021394 |
[2] | Justin Le Sauteur-Robitaille, Zhe Si Yu, Morgan Craig . Impact of estrogen population pharmacokinetics on a QSP model of mammary stem cell differentiation into myoepithelial cells. AIMS Mathematics, 2021, 6(10): 10861-10880. doi: 10.3934/math.2021631 |
[3] | Muhammad Farman, Aqeel Ahmad, Ali Akgül, Muhammad Umer Saleem, Kottakkaran Sooppy Nisar, Velusamy Vijayakumar . Dynamical behavior of tumor-immune system with fractal-fractional operator. AIMS Mathematics, 2022, 7(5): 8751-8773. doi: 10.3934/math.2022489 |
[4] | Shakeel Muhammad, Obaid J. Algahtani, Sayed Saifullah, Amir Ali . Theoretical and numerical aspects of the Malaria transmission model with piecewise technique. AIMS Mathematics, 2023, 8(12): 28353-28375. doi: 10.3934/math.20231451 |
[5] | Abdelatif Boutiara, Jehad Alzabut, Hasib Khan, Saim Ahmed, Ahmad Taher Azar . Qualitative analytical results of complex order nonlinear fractional differential equations with robust control scheme. AIMS Mathematics, 2024, 9(8): 20692-20720. doi: 10.3934/math.20241006 |
[6] | Hengjie Peng, Changcheng Xiang . A Filippov tumor-immune system with antigenicity. AIMS Mathematics, 2023, 8(8): 19699-19718. doi: 10.3934/math.20231004 |
[7] | Ramsha Shafqat, Ateq Alsaadi . Artificial neural networks for stability analysis and simulation of delayed rabies spread models. AIMS Mathematics, 2024, 9(12): 33495-33531. doi: 10.3934/math.20241599 |
[8] | Shabir Ahmad, Aman Ullah, Ali Akgül, Manuel De la Sen . A study of fractional order Ambartsumian equation involving exponential decay kernel. AIMS Mathematics, 2021, 6(9): 9981-9997. doi: 10.3934/math.2021580 |
[9] | Irina Volinsky, Svetlana Bunimovich-Mendrazitsky . Mathematical analysis of tumor-free equilibrium in BCG treatment with effective IL-2 infusion for bladder cancer model. AIMS Mathematics, 2022, 7(9): 16388-16406. doi: 10.3934/math.2022896 |
[10] | Attaullah, Ramzi Drissi, Wajaree Weera . Galerkin time discretization scheme for the transmission dynamics of HIV infection with non-linear supply rate. AIMS Mathematics, 2022, 7(6): 11292-11310. doi: 10.3934/math.2022630 |
In this study, a mathematical model for the differentiation of stem cells is proposed to understand the dynamics of cell differentiation in a complex network. For this, myeloid cells, which are differentiated from stem cells, are introduced in this study. We introduce the threshold quantity R0 to understand the population dynamics of stem cells. The local stability analysis of three equilibria, namely (i) free equilibrium points, (ii) absence of stem and progenitor cells, and (iii) endemic equilibrium points are investigated in this study. The model is first formulated in non-fractional order and after that converted into a fractional sense by utilizing the Atangana-Baleanu derivative in Caputo (ABC) sense in the form of a non-singular kernel. The model is solved by using numerical techniques. It is seen that the myeloid cell population significantly affects the stem cell population.
Stem cells are central to regulate the body mechanism which governs development and tissue regeneration. These cells are self-improving with the capacity to both differentiate and multiply into two hundred cell types that make up the human body. Stem cells are primitive that have the potential to differentiate or develop into a variety of specific cell types. The two main varieties of stem cells in the biological system are embryonic and adult and have a unique capability to develop into well-known cell types in the human body. Moreover, these cells have an important role in the synchronization of the development of human body, tissue homeostasis as well as tissue regeneration. Their potential to differentiate and self-improvement distinguishes them. These two procedures are complementary in several ways in biologically dynamical processes. The self-regeneration ability refers to the stem cells that can create a new cell with similar characteristics to their progenitor, guaranteeing the survival of the basic stem cell population. However, differentiable measures the process by which these offspring become additional specialized and lose stem cell properties, in particular, the potential for self-regeneration [14].
Likewise, a stem cell, a progenitor cell is also a biological cell. Progenitor cells tend to differentiate into a fixed type of cell. But these cells are already more fixed than stem cells and are impelled to differentiate into their presence [7]. The main important difference between progenitor and stem cells is that progenitor cells can differentiate into more than two types of cells based upon their origin and ability but stem cells can reproduce indefinitely [21]. So, the conflict about the accurate definition remains and the abstraction is still in progress [22]. The progenitor cells are early descendents of stem cells that can differentiate to form one or more kinds of cells but they cannot be divided and reproduce indefinitely. There has been very small proof of this oligopotent concept of cells [8]. But progenitor cells speak of being in an extra stage of cell differentiation. Sometimes these cells lie in between stem and fully differentiable cells. This type of ability depends on the differentiation of their big stem cells and likewise on their function. But some progenitor cells could move through the body and migrate towards the tissue where they are needed. So many properties are captured by adult cells as well as progenitor cells.
Marciniak-Czochra and some other mathematical modeller proposed compartmental mathematical models of a discrete group of cell sub-populations to look into possible procedures for stabilization as well as regulation of red blood cell production after perturbations like bone narrow transplantation, which we used as a starting point for our research. Different forms of non-linearities in the mathematical model equations result from various conceivable regulatory feedback processes [18,6]. It was studied that to maintain such a stabilization cell system, two concurrent estimation populations are required [19].
The model provided with six compartments is an isolated negative response curve that describes how mature cells regulate the entire process [3]. In particular, the theories about how external signaling regulates hematopoiesis in feedback to a scarcity of mature cells are being investigated. The first hypothesis argues that the differentiation of cells is controlled solely by increasing the rate of accumulation, whereas the second hypothesis assumes that the prosecution of self-regeneration until differentiation is influenced by outside signals. A unique non-positive regulatory population (matching to hypotheses first or second) is potentially enough to manage the organization. The mathematical modeling in biology has been performed in various age structures [23,24]. The modelling of the COVID-19 pandemic has been observed in the environment [25,26]. We hope to validate this discovery in this work by performing a qualitative analysis of four compartmental mathematical models under the hypothesis first. The structure under Hypothesis Two was examined as well as requirements for the existence of partially free equilibrium points and positively for a four-compartment mathematical model, which have been established in the work of the authors [27]. The following properties of a stem cell population were discovered through mathematical and computational analysis of the biological system of equilibrium points: (i) The mortality rate is lower than the basic reproduction rate for some cytokine levels, and (ii) the signal intensity level of cytokine that is required to maintain the population density is lower than for all supplementary cell populations [16].
In this study, we mainly concentrate on circulation relating to the cell differentiation procedure and its stability analysis. In a few stabilizations cell models, like the cascade of circumstances originating in stem cells earlier than in thoroughly differentiable cells, the hematopoietic system is well outlined [2]. On the other hand, in some blood tissues like the erythrocytes, thrombocytes, and leukocytes, the condition is almost transparent as the total upcoming stages are unknown [13]. As a result, we use mathematical models with as well as without progenitor cells to investigate the impact of intermediary stages among stem, progenitor, and mature cells on stem cell dynamics. For the development of a new concept in differentiation in cell dynamics, incorporated the concept of a myeloid cell. This is the fresh work in cell division modeling through fractional calculus. In hematopoiesis, myelogenous or myeloid cells arise from the progenitor cells after their differentiation [9,10]. They were differentiated from descendants of common progenitors that derived from hematopoietic cells. Also, these cells(common progenitors) are the well-known defenders against infection in the human body.
So, based on cell differentiation facts, we concentrate briefly on the stability analysis of the characterization of stem cells. Here are two important questions still open: (i) Does the stem cell collection remain constant throughout the life of a living entity? (ii) Does it decay the age of a living entity passes? So some moderation of the model from to explore the dynamical behavior of the system less than the satisfaction of all cells, together with stem, progenitor, myeloid and mature cells, explode into replicative senescence has been given in [4,11]. Based on this understanding of differentiation in cell dynamics class in the proposed model. For the implementation of this class, the mathematical model is constructed by using the four compartmental model. The numerical simulations of the proposed model abcd utilizing the estimated data were presented.
This pipeline is described with the assistance of differential as well as delayed differential equations. But in this work, we apply fractional differential equations due to their good explanations in the real world. The mathematical modeling approaches through the fractional differential equation have gained interest as well as another service in the last few years, in the researcher's opinion. This is due to the concept that fractional-order mathematical models have the capability of gaining the crossover dynamical behavior and fading memory that is shown by the biological process. Most of the absorbing facts are the data fitting that can be described as non-integer order rather than ordinary-order [5,20]. Moreover, some fractional derivatives that are identified in their kernels, such as singular and non-singular, are utilized very efficiently to address real-world problems [15]. The fractional differential operator mathematical model generated through the Atangana-Baleanu derivative in the Caputo sense is more important and explains the dynamics systems more effectively than the Caputo-Fabrizio because of its derivative introduced at the basic point of departure in the Mittag-Leffler kernel, which is non-local and non-singular [1]. Thus, the mathematical models created through the Atangana-Baleanu derivative in the Caputo sense are more important and better survey the dynamics of an epidemic. In the ongoing research work, many well-known researchers have established mathematical models with fractional-order derivatives, which are proposed as a good tool for the current life problem response. We extended the model proposed by [28] by using the Atangana-Baleanu derivative in the Caputo sense into 'abcd' cell model by incorporating the myeloid cell class which is an important class of stem cell.
The aim of this mathematical model is to simulate the concentration of stem and progenitor cells. The remaining work on the paper is arranged as follows. Section 2 is devoted to the basic preliminaries related to non-integer fractional derivatives which are utilized in the formulation of mathematical model considerations. The mathematical model description in the Atangana-Baleanu derivative in Caputo sense is given in Section 3. Some basic properties of the mathematical model are given in Section 4. The analysis work is done in Section 5. The numerical solution is carried out in Section 6 by using the Adams Bashforth Moulton method. The numerical results are facilitated in Section 7. Finally, the conclusion is drawn in Section 8.
In this section, we present some valuable concepts and basic definitions of fractional operators.
Definition 2.1. For φ(t)∈Cv, the Caputo derivative is defined as:
C0Dαtφ(t)=1Γ(v−α)∫t0φ(v)(x)(t−x)α−v+1dx,whereα∈(v−1,v],inwhichv∈N. |
Obviously, C0Dαtφ(t)→Dαtφ(t) whenever α→1.
Definition 2.2. The Atangana-Baleanu derivative in the Caputo sense is defined as:\\ ABCaDαtφ(t)=AB(α)1−α∫taφ′(x)×Eα[−α(t−x)α1−α]dx, where 0≤α≤1, φ is in C[a,b] space and AB(α) is the normalization function, such that AB(0)=AB(1)=1.
Definition 2.3. The new fractional derivative associated to integral with the Mittag-Leffler kernel is defined as:
ABCaIαtφ(t)=1−αAB(α)φ(t)+αAB(α)Γ(α)∫taφ(x)(t−x)α−1dx. |
In this section, the integer order mathematical model is formulated. This mathematical model is formulated by the sub-division of the total cell population of stem cell by Δ(t)ast→∞. The proposed model is described by the sub-division of the total cell population given by stem cells a(t), progenitor cells b(t), myeloid cells c(t) and mature cells d(t). The other biological parameters involved in the formulation of the model are ua,ub,uc. These three biological parametric cells are the rate of stem, progenitor, and myeloid cells. The division of stem, progenitor, and myeloid cells that regenerate are represented by va,vb,vc. Also, va(d),vb(d),vc(d) represents the divisional quantities of the stem, progenitor, and myeloid cell dynamical populations, and additionally, μa,μb,μc,μd represents the mortality rate of the corresponding cell populations. On division, the flow of stem cells population is
(2ua−1)va(d(t))a(t). |
The transition diagram of stem cells dynamics is depicted in Figure 1.
The population of progenitor cells is given by
2(1−ua)va(d(t))a(t), |
due to the differentiation of stem cells. Also, the population of progenitor cells is given by
(2ub−1)vb(d(t))b(t). |
In the same fashion, the biological system class of myeloid cells due to differentiation of progenitor cells is
2(1−ub)vb(d(t))b(t). |
Similarly, the flow populations of myeloid cells are
(2uc−1)vc(d(t))c(t). |
Also, in the same fashion, the flow in the class of mature cells due to differentiation of myeloid cells is
2(1−uc)vc(d(t))c(t). |
The model is given as follow:
a′(t)=(2ua−1)va(d(t))a(t)−μaa(t),b′(t)=(2ub−1)vb(d(t))b(t)+2(1−ua)va(d(t))a(t)−μbb(t),c′(t)=(2uc−1)vc(d(t))c(t)+2(1−ub)vb(d(t))b(t)−μcc(t),d′(t)=2(1−uc)vc(d(t))c(t)−μdd(t), | (3.1) |
with initial conditions:
a(0)=a0>0,b(0)=b0≥0,c(0)=c0≥0andd(0)=d0≥0. | (3.2) |
The comprehensive cells division quantities measure the rule of the two procedures by the mature cell production. Take this for ua,ub,uc∈[0,1) and μa,μb,μc,μd>0. The division amounts of the stem, progenitor, and myeloid cells are examined by extracellular biological signaling particles. Specifically, the division amounts of stem, progenitor, and myeloid cells are given by
eaf(t),ebf(t)andecf(t), |
where ea,eb and ec are the uncontrolled divisions of stem, progenitor, myeloid cells, and f(t) is signal strength at time t. Suppose that cytokines are discharged by comprehensive cells and that this production is synchronized by mechanisms sensitive to the rate of mature cells,
f(t)=11+ld(t),wherel>0. |
To estimate the sensitivity to the number of mature cells. This explicit dependency can be rationalized by using a pseudo equilibrium point approximation of the plausible dynamical behavior of the cytokine particles. This estimation throws back the supposition that signal strength attains its efficiency at a lower threshold than the significance of mature cells and mitigates the asymptotic towards zero if the quantity of mature cells increases. The state variables and the biological parameters are shown in Table 1 and Table 2 respectively as below.
State Variables | Biological Description |
a(t) | Stem cell class |
b(t) | Progenitor cell class |
c(t) | Myeloid cell class |
d(t) | Mature cell class |
Parameter | Explanation | Parametric values | Source |
ua | Rate of self-regeneration of stem cells | 0.50−0.60 | [28] |
ub | Rate of self-regeneration of progenitor cells | 0.90−0.95 | [14] |
uc | Rate of self-regeneration of myeloid cells | 0.56−0.66 | Assumed |
va | Division rate of stem cells | 0.1−0.5 | Assumed |
vb | Division rates of progenitor cells | 0.3−0.8 | Assumed |
vc | Division rates of myeloid cells | 0.5−0.9 | Assumed |
μa | Natural death rates of stem cells | 0.01 | [28] |
μb | Natural death rates of progenitor cells | 0.05 | [28] |
μc | Natural death rates of myeloid cells | 0.10 | Assumed |
μd | Natural death rates of mature cells | 0.75 | [28] |
Thus, the spitting amounts of stem, progenitor, and myeloid cells depend on the size of mature cells: va(d)=ea1+ld,vb(d)=eb1+ld,vc(d)=ec1+ld, where ea,eb,ec>0.
We explain the total volume of self-regeneration as the chance that a progeny cell remains in the same position of differentiable as the parent cells. We are additionally supposing that mature cells do not increase and thus really proceed into the mature cell mathematical compartment complementary to differentiable stem cells (two-dimensional mathematical compartmental model), progenitor cells (three-dimensional mathematical compartmental model), or myeloid cells (four-dimensional mathematical compartment model).
We present mathematical model equations in dimensionless expressions divided by μa, and establish the dimensionless time ˜t:=μat :
˜a(˜t):=a(˜tμa),˜b(˜t):=b(˜tμb),˜c(˜t):=c(˜tμc),˜d(˜t):=d(˜tμd), |
sa=eaμa,sb=ebμa,sc=ecμa,gb=μbμa,gc=μcμa,gd=μdμa. |
For the sake of convenience, we are dropping the tilde, Eq (3.1) suit
a′(t)=(2ua−1)sa1+ld(t)a(t)−a(t),b′(t)=(2ub−1)sb1+ld(t)b(t)+2(1−ua)sa1+ld(t)a(t)−gbb(t),c′(t)=(2uc−1)sc1+ld(t)c(t)+2(1−ub)sb1+ld(t)b(t)−gcc(t),d′(t)=2(1−uc)sc1+ld(t)c(t)−gdd(t). | (3.3) |
The fractional-order derivative of Atangana-Baleanu in the Caputo sense is extra comprehensive as the kernel elaborated in this operator is both non-singular as well as non-local. Also, this aforesaid operator tends to catch the memory effects as well as the crossover dynamical behavior that persists in the system of biological cells. On taking these points into mind, in this subsection, we again formulate the abcd mathematical model (3.3) with the assistance of the fractional-order operator of Atangana-Baleanu in the Caputo sense, with the order i.e., α∈[0,1). Therefore, the resulting fractional-order mathematical model demonstrated below is acquired with the substitution of the ordinary derivative by the fractional-order Atangana-Baleanu in the Caputo sense operator:
ABC0Dαta(t)=(2ua−1)sa1+ld(t)a(t)−a(t),ABC0Dαtb(t)=(2ub−1)sb1+ld(t)b(t)+2(1−ua)sa1+ld(t)a(t)−gbb(t),ABC0Dαtc(t)=(2uc−1)sc1+ld(t)c(t)+2(1−ub)sb1+ld(t)b(t)−gcc(t),ABC0Dαtd(t)=2(1−uc)sc1+ld(t)c(t)−gdd(t). | (3.4) |
Thus, in the above proposed mathematical model (3.4), the fractional operator is the Atangana-Baleanu in Caputo sense.
In this section, the well-posedness of the proposed model is discussed below:
In this subsection, we have the powerful feature of the abcd model, said to be existence and uniqueness. For existence and uniqueness purpose we apply the fixed point theory. Initially, the fractional-order model (3.4) is reformulated through the following one-point boundary-value problem:
ABC0DαtΘ(t)=H(t,Θ(t)),Θ(0)=Θ0,0<t<T<∞. | (4.1) |
From the Eq (4.1), the vector demonstrated by Θ=(a(t),b(t),c(t),d(t)) represents the corresponding state variables, and simply the vector Θ0=(a(t0),b(t0),c(t0),d(t0)) demonstrates the corresponding one-point boundary-value conditions. Furthermore, H a continuous vector function as described below:
H=[H1H2H3H4]=[(2ua−1)sa1+ld(t)a(t)−a(t)(2ub−1)sb1+ld(t)b(t)+2(1−ua)sa1+ld(t)a(t)−gbb(t)(2uc−1)sc1+ld(t)c(t)+2(1−ub)sb1+ld(t)b(t)−gcc(t)2(1−uc)sc1+ld(t)c(t)−gdd(t)]. |
Also, the function H satisfies the Lipschitz-criteria demonstrated as below:
‖H(t,Θ1)−H(t,Θ2)‖≤E‖Θ1(t)−Θ2(t)‖,forallE>0. | (4.2) |
Moreover, the below theorem gives out the required existence and uniqueness result.
Theorem 4.1. Let ψ1(α)=1−αABC(α), and ψ2(α)=αABC(α)Γ(α).
If
ψ1(α)E+ψ2(α)TαmaxE<1, | (4.3) |
then the unique solution of the abcd model will exist.
Proof. To take the integral in ABC sense of the Eq (4.1), we have the non-linear Volterra integral equation as follows:
Θ(t)=Θ0(t)+ψ1(α)H(t,Θ)+ψ2(α)∫t0(t−x)α−1H(x,v(x))dx. |
To going next, we interpret ℵ=(0,T) and the correlated function H:C(ℵ,R4+)→C(ℵ,R4+) defined as:
H[Θ(t)]=Θ0(t)+ψ1(α)H(t,Θ)+ψ2(α)∫t0(t−x)α−1H(x,v(x))dx. | (4.4) |
So, the Eq (4.4) takes the following form:
Θ=H[Θ]. | (4.5) |
We represent the supremum norm on ℵ by ‖.‖ℵ states as follows:
‖Θ‖ℵ=supt∈ℵ‖Θ‖,Θ∈C. | (4.6) |
The Banach space is constructed by continuous space C(ℵ,R4+) along with the norm ‖.‖ℵ. The following inequality can be easily demonstrated:
‖∫t0H(t,x)Θ(x)dx‖≤‖H(t,x)‖ℵ‖Θ‖ℵ, | (4.7) |
with Θ∈C(ℵ,R4+) and H(t,x)∈C(ℵ,R) such that
‖H(t,x)‖x=supt∈ℵ|H(t,x)|. | (4.8) |
By the use of Eq (4.6), we have the following result
‖H[Θ1(t)]−H[Θ2(t)]‖ℵ≤‖ψ1(α)(H(t,Θ1(t))−H(t,Θ2(t)))+ψ2(α)×∫t0(t−x)α−1(H(x,Θ1(x))−H(x,Θ2(x)))dx‖ℵ. | (4.9) |
By using Eqs (4.2) and (4.8) as well as triangular inequality, the Eq (4.9) the following form:
‖H[Θ1(t)]−H[Θ2(t)]‖ℵ≤(ψ1(α)E+ψ2(α)TαmaxE)‖Θ1(t)−Θ2(t)‖ℵ. | (4.10) |
Thus, we obtained
‖H[Θ1(t)]−H[Θ2(t)]‖ℵ≤P‖Θ1(t)−Θ2(t)‖ℵ, | (4.11) |
where, P=ψ1(α)E+ψ2(α)TαmaxE. Hence, if the function H holds the Eq (4.3), this function will be a contradiction. This implies that the Eq (4.1) has a unique solution.
In this subsection, we show the feasible region in which our solution lies. The feasible region for cell population is Θ⊂R4+, such that Θ={(a(t),b(t),c(t),d(t))∈R4+,a(t)>0,b(t)≥0,c(t)≥0,d(t)≥0:a(t)+b(t)+c(t)+d(t)≤Θ1+ld(t)}.
Theorem 4.2. The biological feasible region Θ⊂R4+ is positive invariant and attractive for the fractional-order model (3.4) with respect to one-point boundary-value conditions in R4+.
Proof. Let Θ=a(t)+b(t)+c(t)+d(t). By adding all the four equations on the left hand side of model (3.4), we have
ABC0DαtΘ(t)=ABC0Dαta(t)+ABC0Dαtb(t)+ABC0Dαtc(t)+ABC0Dαtd(t),=sa1+ld(t)a(t)+sb1+ld(t)b(t)+sc1+ld(t)c(t)−a(t)−gb(t)−gc(t)−gd(t),=11+ld(t)[saa(t)+sbb(t)+scc(t)]−a(t)−gbb(t)−gcc(t)−gdd(t),=11+ld(t)[Θ−sd]−a(t)−gbb(t)−gcc(t)−gdd(t),≤11+ld(t)[Θ−sd]. | (4.12) |
This implies,
ABC0DαtΘ(t)+Θ1+ld(t)≤sd1+ld(t). |
As a result of the laplace transformation, we have the following:
limt→∞SupΘ(t)≤sd1+ld(t). |
Therefore, Θ(t)→sd1+ld(t) whenever t→∞ for all positive values of time, all the terms given by the closed period with its exact one-point boundary values of the fractional-order abcd model (3.4) factors in Θ persist in Θ. Hence, the biological feasible region Θ is said to be positively invariant and will fascinate all the solutions in R4+.
In this subsection, we explore the positivity of solution of the model (3.4).
Theorem 4.3. For t>0, the solution to the fractional-order abcd model (3.4) is positive as well as bounded for (a(t0),b(t0),c(t0),d(t0))∈R4+.
Proof. We have:
ABC0Dαta(t)(ata(t)=0)=0≥0,ABC0Dαtb(t)(atb(t)=0)=2(1−ua)sa1+ld(t)a(t)≥0,ABC0Dαtc(t)(atc(t)=0)=2(1−ub)sb1+ld(t)b(t)≥0,ABC0Dαtd(t)(atd(t)=0)=2(1−uc)scc(t)≥0. | (4.13) |
Thus, we have the following feasible region: Θ={(a(t0),b(t0),c(t0),d(t0))∈R4+:a(t0),b(t0),c(t0),d(t0)≥0}. Hence, the sum of all four terms is positive.
In this section, we explore the stability of equilibrium points by using the reproduction number of stem cell, progenitor cell and myeloid cell. The reproduction number of stem cell entering in the first compartment mixing the number of stem cell leaving out from the same compartment after division in the life time of stem cell which is denoted by Ra=(2ua−1)sa. Similarly for progenitor cell, the reproduction number is Rb=(2ub−1)sbgb for myeloid cell, the reproduction number is Rc=(2uc−1)scgc.
The combination of the basic reproduction numbers Ra,Rb and Rc characterizes the existence and stability analysis of the equilibrium point. For the sake of convenience, we take the following
fa,1(d)=(2ua−1)sa1+ld(t),fa,2(d)=2(1−ua)sa1+ld(t), | (5.1) |
fb,1(d)=(2ub−1)sb1+ld(t),fb,2(d)=2(1−ub)sb1+ld(t), | (5.2) |
fc,1(d)=(2uc−1)sc1+ld(t),fc,2(d)=2(1−uc)sc1+ld(t). | (5.3) |
In this subsection, we discuss the existence of equilibrium points, namely free equilibrium points, the absence of stem and progenitor cell equilibrium and endemic equilibrium points.
Theorem 5.1. (a) There exists a free equilibrium E∗0=(0,0,0,0) of model (3.4).
(b) There exists a no stem and progenitor cell equilibrium E∗1=(0,0,c1,d1) of model (3.4) given by
E∗1=(0,0,gdRc2(1−uc)scd1,1l(Rc−1)), |
if
Rc>1. | (5.4) |
(c) There exists an endemic equilibrium E2=(a2,b2,c2,d2) of model (3.4) given by
E2=(gb(Ra−Rb)2(1−ua)sab2,gc(Ra−Rc)2(1−ub)sbc2,gdRa2(1−uc)scd2,1l(Ra−1)), |
if
Ra>1, | (5.5) |
and
Ra>Rb&Ra>Rc. | (5.6) |
Theorem 5.2. The free equilibrium point E∗0 of the model (3.4) has four real eigenvalues Ra−1,gb(Rb−1),gc(Rc−1) and −gd and is locally asymptotically stable if
max.{Rc,Rb,Ra}<1, | (5.7) |
and unstable if
max.{Rc,Rb,Ra}>1. | (5.8) |
Proof. At E∗0=(0,0,0,0), the variational matrix of the model (3.4) is described as below:
JE∗0=[(2ua−1)sa−10002(1−ua)sa(2ub−1)sa−gb0002(1−ub)sb(2uc−1)sc−gc0000−gd]=[(2ua−1)sa−10002(1−ua)sa[(2ub−1)sbgb−1]gb0002(1−ub)sb[(2uc−1)scgc−1]gc0000−gd]=[Ra−10002(1−ua)[Rb−1]00sagb02(1−ub)sb[Rc−1]gc0000−gd]. | (5.9) |
From Eq (5.9) we observed that the eigenvalues of the variational matrix at free equilibrium points are λ1=Ra−1,λ2=gb(Rb−1),λ3=gc(Rc−1) and λ4=−gd and is locally asymptotically stable if
max.{Rc,Rb,Ra}<1, | (5.10) |
and unstable if
max.{Rc,Rb,Ra}>1. | (5.11) |
Now, we will discuss the stability analysis of the absence of stem and progenitor cell equilibrium points. Suppose the condition (5.4) holds. We introduced two positive constants for the sake of eigenvalues connected with the absence of stem and progenitor cell equilibrium points.
ϕc1=gd(2−1Rc)andϕc2=gcgd(1−1Rc). | (5.12) |
Theorem 5.3. The absence of stem and progenitor cell equilibrium E∗1 of model (3.4) has four eigen values λi,i=1,2,3,4 where
λ1=RaRb−1,λ2=gbRbRc−gdandλ3,4=12{−ϕc1±((ϕc1)2−4ϕc2)12} | (5.13) |
and λ3,4 lies on the left side of the half plane. The absence of stem and progenitor cell steady point E∗1 is locally asymptotically stable if
Rb>RaandRc>Rb, | (5.14) |
and unstable if
Rb<RaandRc<Rb. | (5.15) |
Proof. Since, we have fc,1(d1)−gc=0andc1=gdd1fc,2(d1). By dropping the sign from c1 and d1, we have the following characteristic equation:
λ{λ−(fa,1(d)−1)}{λ−(fb,1(d1)−gd)}{λ+gd(1−f′c,2(d)fc,2(d)d)}−f′c,1(d)gdd=0. | (5.16) |
We obtain roots,
λ1=fa,1(d)−1=RaRb−1, |
λ2=fb,1(d)−gd=gbRbRc−gd, |
from Eq (5.2) and the identity
1+ld(t)=Rb. |
It follows E∗1 is locally asymptotically stable if Rb>RaandRc>Rb and unstable if Rb<RaandRc<Rb.
Now finally we will look into the stability of the endemic equilibrium points. Suppose that the condition (5.5) holds, and we define
ϵ=(2ub−1)sbandρ(ϵ)=uRa,u∈R. | (5.17) |
Then,
gb>max{0,ρ(ϵ)}. | (5.18) |
Theorem 5.4. If Ra>1 holds, then E2 is locally asymptotically stable if
gb>λ(ϵ,gd), | (5.19) |
and is unstable if
0<gb<λ(ϵ,gd). | (5.20) |
Proof. We have fa,1(d2)=1,b2=gdd2fb,2(d2)anda2=(gb−fb,1(d2))gdd2fa,2(d2)fb,2(d2), by omitting the index from a2,b2 and d2, we have the characteristic equation:
λ[λ(λ−fb,1(d)+gb){λ−gd(f′b,2(d)fb,2(d)d−1)}−gdd{f′b,1(d)+(gb−fb,1)f′a,2(d)fa,2(d)}]−f′a,1(d)(gb−fb,1(d))gdd=0. | (5.21) |
We have the following expressions:
−f′b,2(d)fb,2(d)d=−f′a,2(d)fa,2(d)d=1−1Ra,f′b,1(d)d=fb,1(d)(1−1Ra)=1−1Ra,f′a,1(d)d=fa,1(d)(1−1Ra)=1−1Ra,−fb,1(d)+gb=gb(1−Rb1+ld)=gb(1−RbRa). |
Therefore, Eq (5.21) becomes
λ[λ{λ+gb(1−RbRa)}{λ+gd(2−1Ra)}+gdgb(1−1Ra)]+gdgb(1−RbRa)(1−1Ra)=0. | (5.22) |
By using the gb(Rb/Ra)=ρ(ϵ), in Eq (5.22), we have following characteristic equation
b0λ4+b1λ3+b2λ2+b3λ1+b4=0 | (5.23) |
where,
b0=1,b1=gb(1−RbRa)+gd(2−1Ra),b2=gbgd(1−1Ra)(1−RbRa),b3=gbgd(1−1Ra),b4=gbgd(1−RbRa)(1−1Ra). |
By using the Routh-Hurwitz criteria [12] for Eq (5.23), all the roots have negative real parts if and only if
b0>0,b1>0,b1b2−b0b3>0,b1b2b3−b21b4−b0b23>0,b4>0. |
In this section, we employed the iterative approach of the fractional-order cell division model (3.4) with Atangana-Baleanu in the Caputo sense to obtain the graphical results of abundant biological parameters and memory index on cell division. The well-organized numerical technique [17] is utilized for this determination. First of all, a brief derivation of the approximate approach is introduced for Eq (4.1) and then implemented on the system for (3.4) in compensation.
Using definition 2.3 on both sides of the initial condition (4.1). Then we obtain the integral equation as below:
z(t)−z(0)=ψ1(α)H(t,x)+ψ2(α)∫t0H(y,z(y))(t−y)α−1dy, | (6.1) |
where, ψ1(α)=1−αABC(α) and ψ2(α)=αABC(α)Γ(α).
Moreover, at t=ti+1=(i+1)h, we have
z(ti+1)−z(0)=ψ1(α)H(ti,z(ti))+ψ2(α)∫ti+10H(y,z(y))(ti+1−y)α−1dy,=1AB(α)[(1−α)H(ti,z(ti))+αΓ(α)i∑g=0∫tg+1tgH(y,z(y))(ti+1−y)α−1dy]. | (6.2) |
In addition to the support of a polynomial interpolation scheme, the operator H(y,z(y)) is approximated by employing a two-step Lagrange polynomial on the closed interval [tq,tq+1]. So, we started as follows:
R(y,z(y))˜=Qi(y)=H(tq,z(tq))h(y−tq−1)−H(tq−1,z(tq−1))h(z−tq). | (6.3) |
The Eq (6.2) becomes:
z(ti+1)=z(t0)+1AB(α)[(1−α)H(ti,z(ti))+αhΓ(α)i∑g=0(H(tq,z(tq))∫tg+1tg(y−tq−1)×(ti+1−y)α−1dy−H(tq−1,z(tq−1))∫tg+1tg(y−tq)(ti+1−y)α−1dy)]. | (6.4) |
Furthermore, for the simplification of the Eq (6.4), the approximate solution is considered as follows:
a(ti+1)=a(t0)+1AB(α)[(1−α)H1(ti,z(ti))+αhαΓ(α+2)i∑m=0(H1(ti,z(ti)){pα2p3−pα1p4}−z1{pα+12−pα1p3})],b(ti+1)=b(t0)+1AB(α)[(1−α)H2(ti,z(ti))+αhαΓ(α+2)i∑m=0(H2(ti,z(ti)){pα2p3−pα1p4}−z2{pα+12−pα1p3})],c(ti+1)=c(t0)+1AB(α)[(1−α)H3(ti,z(ti))+αhαΓ(α+2)i∑m=0(H3(ti,z(ti)){pα2p3−pα1p4}−z3{pα+12−pα1p3})],d(ti+1)=d(t0)+1AB(α)[(1−α)H4(ti,z(ti))+αhαΓ(α+2)i∑m=0(H4(ti,z(ti)){pα2p3−pα1p4}−z4{pα+12−pα1p3})], | (6.5) |
where,
p1=i−q,p2=i+1−q,p3=i+2−q+α,p4=i+2−q+2α,
z1=H1(ti−1,z(ti−1)),z2=H2(ti−1,z(ti−1)),z3=H3(ti−1,z(ti−1)),z4=H4(ti−1,z(ti−1)).
In this section, we simulate the mathematical model (3.4) to validate the analytic results. The default parameter are given in Table 2. Figures 2–4 show the variation of all three types of cell population versus time for different of their self-regeneration rates. Figure 2 displays the variation of stem cell population a(t) with respect to time for different values of self-regeneration rate of stem cell. It is seen that as we increase the value of ua from 0.52,0.54,0.56, the population of stem cell increases but it decreases with time. Figure 3 also displays the variation of progenitor cell b(t) with respect to time for different values of self-regeneration rate of progenitor cell. It is seen that as we increases the value of ub from 0.90,0.92,0.94, the population of progenitor cell increases but it also decreases with time. Figure 4 shows the effects of self-generation rate on the dynamics of myeloid cell c(t). It is clear from the figure that the value of self-generation significantly affects the myeloid cell population.
Figure 5 demonstrates the effects of division rate va=0.1,0.2,0.3 on the dynamics of stem cell. It is seen that the population of stem cells decreases with the increase in the values of the division of stem cells. The effects of the self-regeneration rate of the stem cell on the population are seen. Figure 6 shows the effects of the division rate on the population of progenitor cells. It is noticed that as we increase the value of division rate vb from 0.5,0.7,0.9, the population of progenitor cells decreases. It suddenly attains maximum value at t=2 and afterward, it remains almost constant. Figure 7 depicts the variation of myeloid cell with respect to time for different values of division rate of myeloid cell.
Figures 8–11 exhibit the effect of α on all four cell populations. In Figure 8, it is seen that by varying the α from 0.3,0.5,0.7 the stem cell a(t) is affected significantly and it increases with time but decreases with increases in α. We can also find similar trends in Figures 9 and 10, but in Figure 11, the population of d(t) sharply falls and decreasing with time and become almost zero.
In this study, a fractional-order mathematical model is analyzed to understand the dynamical behavior of the stem cell maturation that is responsible for self-renewal, cell death, and regulation of cell division. We considered four compartmental models for a different kind of cell population differentiated for stem cells. The concept of reproduction number is introduced that facilitates our analysis. This reproduction number quality is a threshold parameter that is used to characterize the existence of equilibria and decide the stability of the equilibrium points. It is found that the equilibrium points are stable when max{Ra,Rb,Rc}<1 and unstable when max{Ra,Rb,Rc}>1. Our results indicate that the rate of stem cell division and stem cell self-renewal directly depends upon the environment heterogeneity. In further, these results could be verified by using real data from realistic sources to validate the proposed model.
The authors would like to acknowledge the support from Taif University researchers supporting project (no. TURSP-2020/10), Taif University, Taif, Saudi Arabia.
The authors declare that there are no conflict of interest.
[1] |
A. Atangana, D. Baleanu, New fractional derivatives with the nonlocal and non-singular kernel: Theory and application to heat transfer model, J. Therm. Sci., 20 (2016), 763–769. http://dx.doi.org/10.2298/TSCI160111018A doi: 10.2298/TSCI160111018A
![]() |
[2] |
A. J. Wagers, J. L. Christensen, I. L. Weissman, Cell fate determination from stem cells, Gene Ther., 9 (2002), 606–612. https://doi.org/10.1038/sj.gt.3301717 doi: 10.1038/sj.gt.3301717
![]() |
[3] |
A. Marciniak-Czochra, T. Stiehl, W. Jaeger, A. Ho, W. Wagner, Modeling asymmetric cell division in haemtopoietic stem cells-regulation of self-renewal is essential for efficient re-population, Stem Cells Dev., 17 (2008), 1–10. https://doi.org/10.1089/scd.2008.0143 doi: 10.1089/scd.2008.0143
![]() |
[4] |
A. Marciniak-Czochra, T. Stiehl, W. Wagner, Modeling of replicative senescence in hematopoietic development, Aging, 1 (2009), 723–732. https://dx.doi.org/10.18632/aging.100072 doi: 10.18632/aging.100072
![]() |
[5] |
A. U. Rehman, R. Singh, P. Agarwal, Modeling, analysis and prediction of new variants of covid-19 and dengue co-infection on complex network, Chaos Soliton. Fract., 150 (2021), 111008. https://dx.doi.org/10.1016/j.chaos.2021.111008 doi: 10.1016/j.chaos.2021.111008
![]() |
[6] |
A. U. Rehman, R. Singh, T. Abdeljawad, E. Okyere, L. Guran, Modeling, analysis and numerical solution to malaria fractional model with temporary immunity and relapse, Adv. Differ. Equ., 2021 (2021), 390. https://doi.org/10.1186/s13662-021-03532-4 doi: 10.1186/s13662-021-03532-4
![]() |
[7] | B. Alberts, A. Johnson, J. Lewis, M. Raff, K. Roberts, P. Walter, Molecular biology of the cell, 6 Eds., W.W. Norton & Company, Boca Raton, 2017. |
[8] | B. E. Lawrence, P. M. Horton, Progenitor cells: Biology, characterization and potential clinical applications, Nova Science Publishers. |
[9] |
C. Colijn, M. C. Mackey, A mathematical model of hematopoiesis–-I. Periodic chronic myelogenous leukemia, J. Theor. Biol., 237 (2005), 117–132. https://doi.org/10.1016/j.jtbi.2005.03.033 doi: 10.1016/j.jtbi.2005.03.033
![]() |
[10] |
C. Colijn, M. C. Mackey, A mathematical model of hematopoiesis: II. Cyclical neutropenia, J. Theor. Biol., 237 (2005), 133–146. https://doi.org/10.1016/j.jtbi.2005.03.034 doi: 10.1016/j.jtbi.2005.03.034
![]() |
[11] |
C. Foley, S. Bernard, M. C. Mackey, Cost-effective G-CSF therapy strategies for cyclical neutropenia: Mathematical modelling based hypotheses, J. Theor. Biol., 238 (2006), 754–763. https://doi.org/10.1016/j.jtbi.2005.06.021 doi: 10.1016/j.jtbi.2005.06.021
![]() |
[12] | F. R. Gantmacher, The theory of matrices, Chelsea Publishing Conmpany, New York, 1959. |
[13] |
G. Dontu, M. Al-Hajj, W. M. Abdallah, M. F. Clarke, M. S. Wicha, Stem cells in normal breast development and breast cancer, Cell Proliferat., 36 (2003), 59–72. https://doi.org/10.1046/j.1365-2184.36.s.1.6.x doi: 10.1046/j.1365-2184.36.s.1.6.x
![]() |
[14] |
I. L. Weissman, Stem cells: Units of development, units of regeneration, and units of evolution, Cell, 100 (2000), 157–168. https://doi.org/10.1016/S0092-8674(00)81692-X doi: 10.1016/S0092-8674(00)81692-X
![]() |
[15] | I. Podlubny, Fractional differential equations: An introduction to fractional derivatives, fractional differential equations, to methods of their solution and some of their applications, Elsevier, Amsterdam, 1998. |
[16] |
K. Dietz, J. A. P. Heesterbeek, The concept of R0 in epidemic theory, Stat. Neerl., 50 (1996), 89–110. https://doi.org/10.1111/j.1467-9574.1996.tb01482.x doi: 10.1111/j.1467-9574.1996.tb01482.x
![]() |
[17] |
M. Toufik, A. Atagana, New numerical approximation of fractional derivative with non-local and non-singular kernel: Application to chaotic models, Eur. Phys. J. Plus, 132 (2017), 444. https://doi.org/10.1140/epjp/i2017-11717-0 doi: 10.1140/epjp/i2017-11717-0
![]() |
[18] |
N. Sharma, R. Singh, J. Singh, O. Castillo, Modeling assumptions, optimal control strategies and mitigation through vaccination to zika virus, Chaos Soliton. Fract., 150 (2021), 111137. https://doi.org/10.1016/j.chaos.2021.111137 doi: 10.1016/j.chaos.2021.111137
![]() |
[19] |
O. Arino, M. Kimmel, Stability analysis of models of cell production system, Math. Model., 7 (1986), 1269–1300. https://doi.org/10.1016/0270-0255(86)90081-3 doi: 10.1016/0270-0255(86)90081-3
![]() |
[20] |
P. Agarwal, R. Singh, A. U. Rehman, Numerical solution of a hybrid mathematical model of dengue transmission with relapse and memory via Adam-Bashforth-Moulton predictor-corrector, Chaos Soliton. Fract., 143 (2021), 110564. https://doi.org/10.1016/j.chaos.2020.110564 doi: 10.1016/j.chaos.2020.110564
![]() |
[21] |
P. A. Beachy, S. S. Karhadkar, D. M. Berman, Tissue repair and stem cell renewal in carcinogenesis, Nature, 432 (2004), 324–331. https://doi.org/10.1038/nature03100 doi: 10.1038/nature03100
![]() |
[22] |
R. M. Seaberg, D. Van der Kooy, Stem and progenitor cells: The premature desertion of rigorous definitions, Trends Neurosci., 26 (2003), 125–31. https://doi.org/10.1016/S0166-2236(03)00031-6 doi: 10.1016/S0166-2236(03)00031-6
![]() |
[23] |
S. Bentout, Y. Chen, S. Djilali, Global dynamics of an SEIR model with two age structures and a nonlinear incidence, Acta Appl. Math., 171 (2021). https://doi.org/10.1007/s10440-020-00369-z doi: 10.1007/s10440-020-00369-z
![]() |
[24] |
S. Bentout, S. Djilali, B. Ghanbari, Backward, Hopf bifurcation in a heroin epidemic model with treat age, Int. J. Model. Simul. Sci. Comput., 12 (2021). https://doi.org/10.1142/S1793962321500185 doi: 10.1142/S1793962321500185
![]() |
[25] |
S. Djilali, L. Benahmadi, A. Tridane, K. Niri, Modeling the impact of unreported cases of the COVID-19 in the North African countries, Biology, 9 (2020), 373. https://doi.org/10.3390/biology9110373 doi: 10.3390/biology9110373
![]() |
[26] |
T. Sitthiwirattham, A. Zeb, S. Chasreechai, Z. Eskandari, M. Tilioua, S. Djilali, Analysis of a discrete mathematical COVID-19 model, Results Phys., 28 (2021), 104668. https://doi.org/10.1016/j.rinp.2021.104668 doi: 10.1016/j.rinp.2021.104668
![]() |
[27] |
T. Stiehl, A. Marciniak-Czochra, Characterization of stem cells using mathematical models of multistage cell lineages, Math. Comput. Model., 53 (2011), 1505–1517. https://doi.org/10.1016/j.mcm.2010.03.057 doi: 10.1016/j.mcm.2010.03.057
![]() |
[28] |
Y. Nakata, P. Getto, A. Marciniak-Czochra, T. Alarcoˊn, Stability analysis of multi-compartment models for cell production system, J. Biol. Dynam., 6 (2012), 2–18. https://doi.org/10.1080/17513758.2011.558214 doi: 10.1080/17513758.2011.558214
![]() |
1. | Prem Junswang, Zulqurnain Sabir, Muhammad Asif Zahoor Raja, Waleed Adel, Thongchai Botmart, Wajaree Weera, Intelligent Networks for Chaotic Fractional-Order Nonlinear Financial Model, 2022, 72, 1546-2226, 5015, 10.32604/cmc.2022.027523 | |
2. | Amr Elsonbaty, A. Elsadany, Fatma Kamal, Amin Jajarmi, On Discrete Fractional Complex Gaussian Map: Fractal Analysis, Julia Sets Control, and Encryption Application, 2022, 2022, 1563-5147, 1, 10.1155/2022/8148831 | |
3. | Thongchai Botmart, Wajaree Weera, Muhammad Asif Zahoor Raja, Zulqurnain Sabir, Qusain Hiader, Gilder Cieza Altamirano, Plinio Junior Muro Solano, Alfonso Tesen Arroyo, Dynamics of Fractional Differential Model for Schistosomiasis Disease, 2022, 73, 1546-2226, 981, 10.32604/cmc.2022.028921 | |
4. | Z. Avazzadeh, H. Hassani, M. J. Ebadi, P. Agarwal, M. Poursadeghfard, E. Naraghirad, Optimal Approximation of Fractional Order Brain Tumor Model Using Generalized Laguerre Polynomials, 2023, 2731-8095, 10.1007/s40995-022-01388-1 | |
5. | S. Sujitha, T. Jayakumar, D. Maheskumar, Fractional model of brain tumor with chemo-radiotherapy treatment, 2023, 69, 1598-5865, 3793, 10.1007/s12190-023-01901-8 | |
6. | Neriman Kartal, Dynamics of a Conformable Fractional Order Generalized Richards Growth Model on Star Network with N=20 Nodes, 2024, 45, 2587-2680, 117, 10.17776/csj.1385759 | |
7. | Amit Prakash, Neha Kalyan, Sanjeev Ahuja, Analysis and numerical simulation of fractional-order blood alcohol model with singular and non-singular kernels, 2024, 12, 2544-7297, 10.1515/cmb-2024-0001 | |
8. | Joshua Kiddy K. Asamoah, Gui-Quan Sun, Fractional Caputo and sensitivity heat map for a gonorrhea transmission model in a sex structured population, 2023, 175, 09600779, 114026, 10.1016/j.chaos.2023.114026 |
State Variables | Biological Description |
a(t) | Stem cell class |
b(t) | Progenitor cell class |
c(t) | Myeloid cell class |
d(t) | Mature cell class |
Parameter | Explanation | Parametric values | Source |
u_{a} | Rate of self-regeneration of stem cells | 0.50-0.60 | [28] |
u_{b} | Rate of self-regeneration of progenitor cells | 0.90-0.95 | [14] |
u_{c} | Rate of self-regeneration of myeloid cells | 0.56-0.66 | Assumed |
v_{a} | Division rate of stem cells | 0.1-0.5 | Assumed |
v_{b} | Division rates of progenitor cells | 0.3-0.8 | Assumed |
v_{c} | Division rates of myeloid cells | 0.5-0.9 | Assumed |
\mu_{a} | Natural death rates of stem cells | 0.01 | [28] |
\mu_{b} | Natural death rates of progenitor cells | 0.05 | [28] |
\mu_{c} | Natural death rates of myeloid cells | 0.10 | Assumed |
\mu_{d} | Natural death rates of mature cells | 0.75 | [28] |
State Variables | Biological Description |
a(t) | Stem cell class |
b(t) | Progenitor cell class |
c(t) | Myeloid cell class |
d(t) | Mature cell class |
Parameter | Explanation | Parametric values | Source |
u_{a} | Rate of self-regeneration of stem cells | 0.50-0.60 | [28] |
u_{b} | Rate of self-regeneration of progenitor cells | 0.90-0.95 | [14] |
u_{c} | Rate of self-regeneration of myeloid cells | 0.56-0.66 | Assumed |
v_{a} | Division rate of stem cells | 0.1-0.5 | Assumed |
v_{b} | Division rates of progenitor cells | 0.3-0.8 | Assumed |
v_{c} | Division rates of myeloid cells | 0.5-0.9 | Assumed |
\mu_{a} | Natural death rates of stem cells | 0.01 | [28] |
\mu_{b} | Natural death rates of progenitor cells | 0.05 | [28] |
\mu_{c} | Natural death rates of myeloid cells | 0.10 | Assumed |
\mu_{d} | Natural death rates of mature cells | 0.75 | [28] |