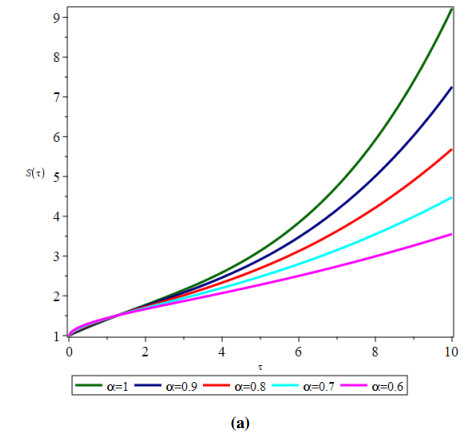
The present research investigates the Susceptible-Infected-Recovered (SIR) epidemic model of childhood diseases and its complications with the Atangana-Baleanu fractional derivative operator in the Caputo sense (ABC). With the aid of the Elzaki Adomian decomposition method (EADM), the approximate solutions of the aforesaid model are discussed by exerting the Adomian decomposition method. By employing the fixed point postulates and the Picard–Lindelöf approach, the stability, existence, and uniqueness consequences of the model are demonstrated. Furthermore, we illustrate the essential hypothesis for disease control in order to find the role of unaware infectives in the spread of childhood diseases. Besides that, simulation results and graphical illustrations are presented for various fractional-orders. A comparison analysis is shown with the previous findings. It is hoped that ABC fractional derivative and the projected algorithm will provide new venues in futuristic studies to manipulate and analyze several epidemiological models.
Citation: Saima Rashid, Fahd Jarad, Fatimah S. Bayones. On new computations of the fractional epidemic childhood disease model pertaining to the generalized fractional derivative with nonsingular kernel[J]. AIMS Mathematics, 2022, 7(3): 4552-4573. doi: 10.3934/math.2022254
[1] | Shabir Ahmad, Aman Ullah, Mohammad Partohaghighi, Sayed Saifullah, Ali Akgül, Fahd Jarad . Oscillatory and complex behaviour of Caputo-Fabrizio fractional order HIV-1 infection model. AIMS Mathematics, 2022, 7(3): 4778-4792. doi: 10.3934/math.2022265 |
[2] | Gulalai, Shabir Ahmad, Fathalla Ali Rihan, Aman Ullah, Qasem M. Al-Mdallal, Ali Akgül . Nonlinear analysis of a nonlinear modified KdV equation under Atangana Baleanu Caputo derivative. AIMS Mathematics, 2022, 7(5): 7847-7865. doi: 10.3934/math.2022439 |
[3] | Ihsan Ullah, Aman Ullah, Shabir Ahmad, Hijaz Ahmad, Taher A. Nofal . A survey of KdV-CDG equations via nonsingular fractional operators. AIMS Mathematics, 2023, 8(8): 18964-18981. doi: 10.3934/math.2023966 |
[4] | Bahar Acay, Ramazan Ozarslan, Erdal Bas . Fractional physical models based on falling body problem. AIMS Mathematics, 2020, 5(3): 2608-2628. doi: 10.3934/math.2020170 |
[5] | Saima Rashid, Fahd Jarad, Taghreed M. Jawa . A study of behaviour for fractional order diabetes model via the nonsingular kernel. AIMS Mathematics, 2022, 7(4): 5072-5092. doi: 10.3934/math.2022282 |
[6] | Amir Khan, Abdur Raouf, Rahat Zarin, Abdullahi Yusuf, Usa Wannasingha Humphries . Existence theory and numerical solution of leptospirosis disease model via exponential decay law. AIMS Mathematics, 2022, 7(5): 8822-8846. doi: 10.3934/math.2022492 |
[7] | Muhammad Farman, Ali Akgül, Kottakkaran Sooppy Nisar, Dilshad Ahmad, Aqeel Ahmad, Sarfaraz Kamangar, C Ahamed Saleel . Epidemiological analysis of fractional order COVID-19 model with Mittag-Leffler kernel. AIMS Mathematics, 2022, 7(1): 756-783. doi: 10.3934/math.2022046 |
[8] | Shams A. Ahmed, Tarig M. Elzaki, Abdelgabar Adam Hassan, Husam E. Dargail, Hamdy M. Barakat, M. S. Hijazi . Applications of Elzaki transform to non-conformable fractional derivatives. AIMS Mathematics, 2025, 10(3): 5264-5284. doi: 10.3934/math.2025243 |
[9] | Yudhveer Singh, Devendra Kumar, Kanak Modi, Vinod Gill . A new approach to solve Cattaneo-Hristov diffusion model and fractional diffusion equations with Hilfer-Prabhakar derivative. AIMS Mathematics, 2020, 5(2): 843-855. doi: 10.3934/math.2020057 |
[10] | Eiman, Saowaluck Chasreechai, Thanin Sitthiwirattham, M. A. El-Shorbagy, Muhammad Sohail, Ubaid Ullah, Mati ur Rahman . Qualitative theory and approximate solution to a dynamical system under modified type Caputo-Fabrizio derivative. AIMS Mathematics, 2022, 7(8): 14376-14393. doi: 10.3934/math.2022792 |
The present research investigates the Susceptible-Infected-Recovered (SIR) epidemic model of childhood diseases and its complications with the Atangana-Baleanu fractional derivative operator in the Caputo sense (ABC). With the aid of the Elzaki Adomian decomposition method (EADM), the approximate solutions of the aforesaid model are discussed by exerting the Adomian decomposition method. By employing the fixed point postulates and the Picard–Lindelöf approach, the stability, existence, and uniqueness consequences of the model are demonstrated. Furthermore, we illustrate the essential hypothesis for disease control in order to find the role of unaware infectives in the spread of childhood diseases. Besides that, simulation results and graphical illustrations are presented for various fractional-orders. A comparison analysis is shown with the previous findings. It is hoped that ABC fractional derivative and the projected algorithm will provide new venues in futuristic studies to manipulate and analyze several epidemiological models.
Childhood infections are among the highly prevalent types of infectious diseases. Influenza, hepatitis, chickenpox, malaria, rubella and tetanus are illustrations of infections. Vaccination of children is recommended by healthcare personnel to prevent them from contracting such infections. Vaccination has had a crucial effect in diminishing the prevalence of contagious illnesses in children. On the other hand, preventable diseases constitute a significant global health hazard. Vaccination has a considerable influence on the prevention of infectious illnesses in infants. Therefore, among the most vital aspects of preventative health initiatives is childhood diseases (CHD). Numerous physicians and mathematicians are interested in the dynamics of diseases such as Haq et al. [1], Koca [2], Khan et al. [3], Ullah et al. [4] and Singh et al. [5].
Vaccinations are the more efficient way to prevent illnesses in babies. As a result, developing a framework that anticipates the strongest performance of vaccine effectiveness needed is critical to preventing the development of such illnesses. In 1974, the World Health Organization's (WHO) "Extensive Immunization Program (EPI)" was established to expand vaccine availability for all children. Mathematical simulations are frequently employed to acquire a clearer and more comprehensive knowledge of the mechanisms of illness dissemination in children and to assess preventive approaches [6,7,8].
The scientists employed nonlinear systems to solve real-world challenges in a variety of fields. For physicians, the concept of dynamical problems is well-known. It is gaining popularity in a variety of professions and is exploring various facets of the aforementioned domain [9,10,11,12,13,14,15]. Several experts produced fractional-order differential equations throughout the past three decades. It was widely used to overcome significant challenges in a variety of disciplines; see [16,17,18,19,20,21,22,23,24,25,26,27,28]. Existence, stability analysis, simulation and optimal approaches are some of the noted features that have lately been introduced. The fractional derivative operators were proposed by several researchers, such as Caputo [29], Podlubny [30], Hilfer [31], Baleanu et al. [32], Singh et al. [33], Kumar et al. [34] and henceforth. Atangana-Baleanu [35] recently proposed a new concept of a fractional order derivative incorporating Mittag-Leffler as a non-singular kernel, and the authors [33] highlighted the further attributes. Employing fractional derivatives and implementing them into the CHD model has resulted in a variety of scientific findings.
All of the research aforementioned constructed a mechanistic and chaotic mathematical framework of CHD spread mechanisms, but none of them explored the fractional order feature of the paradigm. Unfortunately, no progress has been made in investigating the fractional-order behaviour of the CHD model, which correlates with the ABC fractional derivative operator up to this point.
Our aim is that we split the disease-affected community into three epidemiological categories in the paradigm described in this article: a susceptible category (S), an infected category (I), and a removed category (R) designating inoculated as well as healed individuals having persistent immunization.
In 2012, Arafa and other academics resurrected the classical childhood illness transmission dynamics [36]. The model is described as follows:
{dSdϱ=(1−P)ν−βSI−νS,dIdϱ=βSI−(γ+ν)I,dRdϱ=Pν+γI−νR. | (1.1) |
where S=s/N,I=ι/N,R=r/N,N=s+ι+r. The vaccine's efficiency is 100 percent in this scenario, and N assumed to be variable. Thus, ν represents the birth rate, β reflects the general contact rate of a vulnerable individual with an infectious person, γ represents the infected individual's recover-ability and readmission into the eliminated class, and P represents the immunized population at birth (with 0<P<1). Recently, Haq et al. [37] established the numerical solutions of fractional CHDs, Baleanu et al. [38] projected the exhibiting of prevalent CHDs via Caputo-Fabrizio in the frame of the Laplace transform, and Singh et al. [39] investigated vaccination of the SIR model.
In 1980, Adomian first proposed the Adomian decomposition method (ADM). This approach is useful for solving systems of differential equations numerically and analytically [40,41]. The Adomian approach handles nonlinear DEs and the system of fractional DEs without requiring a Lagrange multiplier, intricate integrals, or any more variables.
Adopting the above propensity, we use a recently developed arbitrary order derivative in the CHD model. The fundamental objective of this paper is to examine the CHD model by applying a revolutionary ABC fractional derivative operator and to describe the intricacies of the uniqueness and existence of the aforesaid model solution by aiding a fixed point postulate and the Picard–Lindelöf technique.
The following is a summary of the considerations in the design of this article: The ABC derivative of arbitrary order is investigated in Section 2. Furthermore, the existence theory of the mechanism is presented in Section 3. An algorithm is established with the correlation of the Elzaki transform and ADM. By employing the contraction mapping theorem and the Picard–Lindelöf technique, the uniqueness and existence of the solutions to the system are analyzed in Section 4. Some simulation and tabulation consequences are debated in Section 5. Finally, in Section 6, we summarise the concluding remarks and future directions.
The study of hypotheses and the use of derivatives and integrals of contested mappings is known as fractional calculus. In the subsequent ongoing area, we use essential terminology and findings obtained from fractional calculus theory.
Definition 2.1. ([30]) The Caputo fractional derivative structure is stated as
c0Dαϱ={1Γ(n−α)ϱ∫0f(n)(x)(ϱ−x)α+1−ndx,n−1<α<n,dndϱnf(ϱ),α=n. | (2.1) |
Definition 2.2. ([35]) For f∈H1(ˇα,ˇβ),ˇα<ˇβ,α∈[0,1] and The ABC fractional derivative is stated as follows:
ABCa1Dαϱ(f(ϱ))=B(α)1−αϱ∫a1f′(ϱ)Eα[−α(ϱ−x)α1−α]dx, | (2.2) |
where B(α) in (2.2) is the normalization function with B(α)=B(0)=B(1)=1.
Definition 2.3. ([35]) Let α∈[0,1], then the integral of fractional order α of the function f of the ABC-operator is defined as
ABCa1Iαϱ(f(ϱ))=1−αB(α)f(ϱ)+αΓ(α)B(α)ϱ∫a1f(x)(ϱ−x)α−1dx. | (2.3) |
Definition 2.4. ([42]) A set M involving exponential function is defined as
M={f(ϱ):∃z,l1,l2>0,|f(ϱ)|<ze|ϱ|li,ifϱ∈(−1)i×[0,∞)|}. | (2.4) |
where z is a finite number, but l1,l2 may be finite or infinite.
Definition 2.5. ([42,43]) A mapping f(ϱ) having Elzaki transform is described as
E{f(ϱ)}(ϑ)=U(ϑ)=ϑ∞∫0e−ϱϑf(ϱ)dϱ,ϱ≥0,ϑ∈[l1,l2]. | (2.5) |
Theorem 2.6. ([44])(Convolution property). The subsequent consequence holds true for Elzaki transform:
E{f1∗f2}=ω−1E(f1)∗E(f2). | (2.6) |
Definition 2.7. ([44]) The CFD form of Elzaki transform is described as:
E{c0Dαϱ(f(ϱ))}(ω)=ω−αU(ω)−n−1∑κ=0ω2−α+κf(κ)(0),n−1<α<n. | (2.7) |
Definition 2.8. ([45]) The ABC fractional derivative formation of Elzaki transform is described as
E{ABC0Dαϱ(f(ϱ))}(ω)=B(α)αωα+1−α(U(ω)ω−ωf(0)), | (2.8) |
where E{f(ϱ)}(ω)=U(ω).
It is necessary to modify the integer-order form to arbitrary setting in order to numerically investigate the effect of biological components and to approximate the emergence and spread of CHDs. As a result, in this study, we deduce the paradigm (1.1) for the Atangana-Baleanu derivative operator supplied as
ABCDαϱS(ϱ)=Θ1(ϱ,S(ϱ))=(1−P)ν−βS(ϱ)I(ϱ)−νS(ϱ),ABCDαϱI(ϱ)=Θ2(ϱ,I(ϱ))=βS(ϱ)I(ϱ)−(γ+ν)I(ϱ),ABCDαϱR(ϱ)=Θ3(ϱ,R(ϱ))=Pν+γI(ϱ)−νR(ϱ). | (3.1) |
where ABCDαϱ signifies the ABC fractional derivative operator having fractional order α, i.e., 0<α≤1 and the ICs S0(ϱ)=S(0),I0(ϱ)=I(0),R0(ϱ)=R(0).
In what follows, we demonstrate that (3.1) has a fixed point. To do this, we utilize (3.1) as follows:
ABCDαϱS(ϱ)=Θ1(ϱ,S(ϱ)),ABCDαϱI(ϱ)=Θ2(ϱ,I(ϱ)),ABCDαϱR(ϱ)=Θ3(ϱ,R(ϱ)). | (3.2) |
Taking into consideration the Definition 2.3 in the aforesaid system, we have
{S(ϱ)−S(0)=1−αB(α)Θ1(ϱ,S(ϱ))+αB(α)Γ(α)ϱ∫0Θ1(ϱ,S(ϱ))(ϱ−x)α−1dx,I(ϱ)−I(0)=1−αB(α)Θ2(ϱ,S(ϱ))+αB(α)Γ(α)ϱ∫0Θ2(ϱ,S(ϱ))(ϱ−x)α−1dx,R(ϱ)−R(0)=1−αB(α)Θ3(ϱ,S(ϱ))+αB(α)Γ(α)ϱ∫0Θ3(ϱ,S(ϱ))(ϱ−x)α−1dx. | (3.3) |
Furthermore, we show that the kernels Θ1,Θ2,Θ3 satisfy both the Lipschitz and contraction assumptions.
Theorem 3.1. The kernel Θ1(ϱ,S(ϱ)) preserves the Lipschitz assumptions as well as contraction if the inequality
0≤βχ+ν<1 | (3.4) |
is satisfied.
Proof. Assume that Θ1(ϱ,S(ϱ))=(1−P)ν−βS(ϱ)I(ϱ)−νS(ϱ).
For S and ˆS, we attain
‖Θ1(ϱ,S(ϱ))−Θ1(ϱ,ˆS(ϱ))‖=‖((1−P)ν−βS(ϱ)I(ϱ)−νS(ϱ))−((1−P)ν−βˆS(ϱ)I(ϱ)−νˆS(ϱ))‖≤β‖I(ϱ)‖‖S(ϱ)−ˆS(ϱ)‖+ν‖S(ϱ)−ˆS(ϱ)‖≤(β‖I(ϱ)‖+ν)‖S(ϱ)−ˆS(ϱ)‖≤K1‖S(ϱ)−ˆS(ϱ)‖, | (3.5) |
where K1=βχ1+ν, and ‖I(ϱ)‖=maxϱ∈J1‖I(ϱ)‖≤χ1 is a bounded mapping.
Thus, (3.5) holds the Lipschitz assumption for Θ1 and also 0≤βχ+ν<1, shows that Θ1 is a contraction.
Analogously, the Lipschitz assumption for Θ2 and Θ3 are presented as follows:
‖Θ2(ϱ,I(ϱ))−Θ2(ϱ,ˆI(ϱ))‖≤K2‖I(ϱ)−ˆI(ϱ)‖,‖Θ3(ϱ,R(ϱ))−Θ3(ϱ,ˆR(ϱ))‖≤K3‖R(ϱ)−ˆR(ϱ)‖, | (3.6) |
where K2=βχ2+γ+ν and K3=ν and ‖S(ϱ)‖=maxϱ∈J1‖S(ϱ)‖≤χ2 is a bounded mapping. Moreover, if 0≤βχ2+γ+ν<1 and 0≤ν<1, then Θ2 and Θ3 are contraction.
Therefore, in view of kernels, (3.3) can be written in a recursive manner as follows:
Ω1n(ϱ)=Sn(ϱ)−Sn−1(ϱ)=1−αB(α)(Θ1(ϱ,Sn−1)−Θ1(ϱ,Sn−2))+αB(α)Γ(α)ϱ∫0(Θ1(ϱ,Sn−1)−Θ1(ϱ,Sn−2))(ϱ−x)α−1dx,Ω2n(ϱ)=In(ϱ)−In−1(ϱ)=1−αB(α)(Θ2(ϱ,In−1)−Θ2(ϱ,In−2))+αB(α)Γ(α)ϱ∫0(Θ2(ϱ,In−1)−Θ2(ϱ,In−2))(ϱ−x)α−1dx,Ω3n(ϱ)=Rn(ϱ)−Rn−1(ϱ)=1−αB(α)(Θ3(ϱ,Rn−1)−Θ3(ϱ,Rn−2))+αB(α)Γ(α)ϱ∫0(Θ3(ϱ,Rn−1)−Θ3(ϱ,Rn−2))(ϱ−x)α−1dx, | (3.7) |
supplemented by the initial conditions S0(ϱ)=S(0),I0(ϱ)=I(0) and R0(ϱ)=R(0). Applying the norm on (3.7), then we have
‖Ω1n(ϱ)‖=‖Sn(ϱ)−Sn−1(ϱ)‖=‖1−αB(α)(Θ1(ϱ,Sn−1)−Θ1(ϱ,Sn−2))+αB(α)Γ(α)ϱ∫0(Θ1(ϱ,Sn−1)−Θ1(ϱ,Sn−2))(ϱ−x)α−1dx‖≤1−αB(α)‖(Θ1(ϱ,Sn−1)−Θ1(ϱ,Sn−2))‖+αB(α)Γ(α)‖ϱ∫0(Θ1(ϱ,Sn−1)−Θ1(ϱ,Sn−2))(ϱ−x)α−1dx‖(Bytriangularinequality). | (3.8) |
In view of Lipschitz assumption (3.6), we obtain
‖Ω1n(ϱ)‖=‖Sn(ϱ)−Sn−1(ϱ)‖≤K11−αB(α)‖Sn−1(ϱ)−Sn−2(ϱ)‖+K1αB(α)Γ(α)ϱ∫0‖Sn(ϱ)−Sn−1(ϱ)‖(ϱ−x)α−1dx. | (3.9) |
Thus, we attain
‖Ω1n(ϱ)‖≤K11−αB(α)‖Ω1(n−1)(ϱ)‖+K1αB(α)Γ(α)ϱ∫0‖Ω1(n−1)(ϱ)‖(ϱ−x)α−1dx. | (3.10) |
Analogously, we attain
‖Ω2n(ϱ)‖≤K21−αB(α)‖Ω2(n−1)(ϱ)‖+K2αB(α)Γ(α)ϱ∫0‖Ω2(n−1)(ϱ)‖(ϱ−x)α−1dx,‖Ω3n(ϱ)‖≤K31−αB(α)‖Ω3(n−1)(ϱ)‖+K3αB(α)Γ(α)ϱ∫0‖Ω3(n−1)(ϱ)‖(ϱ−x)α−1dx. | (3.11) |
Also, we can express that
Sn(ϱ)=n∑ȷ=1Ω1ȷ(ϱ),In(ϱ)=n∑ȷ=1Ω2ȷ(ϱ),Rn(ϱ)=n∑ȷ=1Ω3ȷ(ϱ). |
Next, to find the existence result, we surmise the subsequent theorem.
Theorem 3.2. The CHDs model (3.1) has a solution, if there exists T∗ such that
1−αB(α)Km+T∗αB(α)Γ(α)Km<1,m=1,2,3,.... | (3.12) |
Proof. Since the mappings S(ϱ),I(ϱ) and R(ϱ) are bounded. Also, the kernels satisfies Lipschitz assumptions. Applying recursive technique on (3.10) and (3.11), we attain
‖Ω1n(ϱ)‖≤‖Sn(0)‖[K11−αB(α)+K1ϱαB(α)Γ(α)]n,‖Ω2n(ϱ)‖≤‖In(0)‖[K21−αB(α)+K2ϱαB(α)Γ(α)]n,‖Ω3n(ϱ)‖≤‖Rn(0)‖[K31−αB(α)+K3ϱαB(α)Γ(α)]n. | (3.13) |
As a result, the mappings Ω1n(ϱ),Ω2n(ϱ) and Ω3n(ϱ) presented in (3.13) have a solution that is continuous. Furthermore, we show that the mappings presented in (3.13) enable the solutions of (3.1), we surmise that
S(ϱ)−S(0)=Sn(ϱ)−Sn(ϱ),I(ϱ)−I(0)=In(ϱ)−In(ϱ),R(ϱ)−R(0)=Rn(ϱ)−Rn(ϱ), | (3.14) |
where Sn(ϱ),In(ϱ) and Rn(ϱ) denotes the remainder terms of the solution.
Therefore, we have
‖Sn(ϱ)‖≤1−αB(α)‖Θ1(ϱ,S(ϱ))−Θ1(ϱ,Sn−1(ϱ))‖+αB(α)Γ(α)ϱ∫0‖Θ1(x,S(x))−Θ1(x,Sn−1(x))‖(ϱ−x)α−1dx≤1−αB(α)K1‖S−Sn−1‖+ϱαB(α)Γ(α)K1‖S−Sn−1‖. | (3.15) |
After a recursive process, we attain
‖Sn(ϱ)‖≤{1−αB(α)+T∗αB(α)Γ(α)}n+1Kn+11α, | (3.16) |
where α=‖S−Sn−1‖. Applying limit on obtaining equation as n tends to ∞, we find ‖Sn(ϱ)‖↦0. In a similar fashion, one can evaluate that ‖In(ϱ)‖↦0 and ‖Rn(ϱ)‖↦0. The existense result is illustrated.
To demonstrate the uniqueness of the solutions, we surmise that the system (3.1) has different solution such that S1(ϱ),I1(ϱ) and R1(ϱ), then we have the following:
S(ϱ)−S1(ϱ)=1−αB(α)(Θ1(ϱ,S(ϱ))−Θ1(ϱ,S1(ϱ)))+αB(α)Γ(α)ϱ∫0(Θ1(ϱ,S(ϱ))−Θ1(ϱ,S1(ϱ)))(ϱ−x)α−1dx. |
Applying norm on above equation, we have
‖S(ϱ)−S1(ϱ)‖=1−αB(α)‖Θ1(ϱ,S(ϱ))−Θ1(ϱ,S1(ϱ))‖+αB(α)Γ(α)ϱ∫0‖Θ1(ϱ,S(ϱ))−Θ1(ϱ,S1(ϱ))‖(ϱ−x)α−1dx. |
In view of Lipschitz assumptions (3.6), we have
‖S(ϱ)−S1(ϱ)‖=1−αB(α)K1‖S(ϱ)−S1(ϱ)‖+ϱαB(α)Γ(α)‖S(ϱ)−S1(ϱ)‖. | (3.17) |
This yields, we have
‖S(ϱ)−S1(ϱ)‖(1−1−αB(α)K1+ϱαB(α)Γ(α))≤0. | (3.18) |
Theorem 3.3. The system of (3.1) has a unique solution if the subsequent assumption satisfy
(1−1−αB(α)K1+ϱαB(α)Γ(α))>0. | (3.19) |
Proof. Assume that the hypothesis of (3.17) satisfy
‖S(ϱ)−S1(ϱ)‖(1−1−αB(α)K1+ϱαB(α)Γ(α))≤0, | (3.20) |
which shows that ‖S(ϱ)−S1(ϱ)‖=0. Hence, we conclude S(ϱ)=S1(ϱ). Analogously, we can continue the same procedure for I and R.
In order to find the solution of the system (3.1), employing the Elzaki transform
{E[ABCDαϱS(ϱ)](ω)=E[(1−P)ν−βS(ϱ)I(ϱ)−νS(ϱ)],E[ABCDαϱI(ϱ)]=E[βS(ϱ)I(ϱ)−(γ+ν)I(ϱ)],E[ABCDαϱR(ϱ)]=E[Pν+γI(ϱ)−νR(ϱ)]. |
It follows that
{B(α)ωα+1−α(E[S(ϱ)]ω−ωS(0))=E[(1−P)ν−βS(ϱ)I(ϱ)−νS(ϱ)],B(α)ωα+1−α(E[I(ϱ)]ω−ωI(0))=E[βS(ϱ)I(ϱ)−(γ+ν)I(ϱ)],B(α)ωα+1−α(E[R(ϱ)]ω−ωR(0))=E[Pν+γI(ϱ)−νR(ϱ)]. |
Now, supplementing the initial conditions and employing the inverse Elzaki transform on the aforesaid system of equations, we have
{S(ϱ)=S(0)+E−1[ωα+1−αB(α)E[(1−P)ν−βS(ϱ)I(ϱ)−νS(ϱ)]],I(ϱ)=I(0)+E−1[ωα+1−αB(α)E[βS(ϱ)I(ϱ)−(γ+ν)I(ϱ)]],R(ϱ)=R(0)+E−1[ωα+1−αB(α)E[Pν+γI(ϱ)−νR(ϱ)]]. | (4.1) |
Suppose that the infinite series formulation of S(ϱ),I(ϱ) and R(ϱ) are represented as
S(ϱ)=∞∑n=0Sn(ϱ),I(ϱ)=∞∑n=0In(ϱ),R(ϱ)=∞∑n=0Rn(ϱ), | (4.2) |
whilst the nonlinear term
S(ϱ)I(ϱ)=∞∑n=0AN(ϱ) | (4.3) |
are dealt by the Adomian polynomial stated as
An(ϱ)=1Γ(n+1)dndαn[n∑κ=0ακSκ(ϱ)n∑κ=0ακIκ(ϱ)]α=0. | (4.4) |
The first three polynomials are presented as
An(ϱ)={S0(ϱ)I0(ϱ),n=0,S0(ϱ)I1(ϱ)+S1(ϱ)I0(ϱ),n=1,2S0(ϱ)I2(ϱ)+2S1(ϱ)I1(ϱ)+2S2(ϱ)I0(ϱ),n=2. |
plugging (4.2)–(4.4) into (4.1), we attain
{E[∞∑n=0Sn(ϱ)]=ω2S(0)+ωα+1−αB(α)E[(1−P)ν−β∞∑n=0Sn(ϱ)∞∑n=0I(ϱ)−ν∞∑n=0Sn(ϱ)],E[∞∑n=0In(ϱ)]=ω2I(0)+ωα+1−αB(α)E[β∞∑n=0Sn(ϱ)∞∑n=0In(ϱ)−(γ+ν)∞∑n=0In(ϱ)],E[∞∑n=0Rn(ϱ)]=ω2R(0)+ωα+1−αB(α)E[Pν+γ∞∑n=0In−ν∞∑n=0Rn(ϱ)]. | (4.5) |
Comparing both sides of (4.5) presents the subsequent recursive algorithm, we have
E[S0]=ω2S(0),E[S1]=ωα+1−αB(α)[ω2(1−P)ν−βE[A0(ϱ)]−νE[S0(ϱ)]],E[S2]=ωα+1−αB(α)[ω2(1−P)ν−βE[A1(ϱ)]−νE[S(ϱ)]],⋮E[Sn+1]=ωα+1−αB(α)[ω2(1−P)ν−βE[An(ϱ)]−νE[Sn(ϱ)]],E[I0]=ω2I(0),E[I1]=ωα+1−αB(α)[βE[A0(ϱ)]−(γ+ν)E[I0(ϱ)]],E[I2]=ωα+1−αB(α)[βE[A1(ϱ)]−(γ+ν)E[I1(ϱ)]],⋮E[In+1]=ωα+1−αB(α)[βE[An(ϱ)]−(γ+ν)E[In(ϱ)]],E[R0]=ω2R(0),E[R1]=ωα+1−αB(α)[ω2Pν+γE[I0(ϱ)]−νE[R0(ϱ)]],E[R2]=ωα+1−αB(α)[ω2Pν+γE[I1(ϱ)]−νE[R1(ϱ)]],⋮E[Rn+1]=ωα+1−αB(α)[ω2Pν+γE[In(ϱ)]−νE[Rn(ϱ)]]. | (4.6) |
By employing the inverse Elzaki transform, we attain the iterative terms as follows:
S0=S0,S1=(1−P)νB(α)[αϱαΓ(α+1)+(1−α)]−β(S0I0+νI0)B(α)[αϱαΓ(α+1)+(1−α)]−(1−P)ν2B2(α)[α2ϱ2αΓ(2α+1)+2α(1−α)ϱαΓ(α+1)+(1−α)2],S2=(1−P)νB(α)[αϱαΓ(α+1)+(1−α)]−β2(S20I0+β(γ+ν)S0I0)B2(α)×[α2ϱ2αΓ(2α+1)+2α(1−α)ϱαΓ(α+1)+(1−α)2]−β(S0I0+S0ν)B2(α)[α2ϱ2αΓ(2α+1)+2α(1−α)ϱαΓ(α+1)+(1−α)2]−(1−P)ν2(βI0+ν)B3(α)×[α3ϱ3αΓ(3α+1)+3α2(1−α)ϱ2αΓ(2α+1)+3α(1−α)2ϱαΓ(α+1)+(1−α)3],I0=I0,I1=βS0I0B(α)[αϱαΓ(α+1)+(1−α)]−(γ+ν)B(α)[αϱαΓ(α+1)+(1−α)],I2=βS0I0B2(α)(βS0I0−(γ+ν)−(γ+ν)βS0I0−(γ+ν)I0)×[α2ϱ2αΓ(2α+1)+2α(1−α)ϱαΓ(α+1)+(1−α)2]−βI0(βS0I0+S0ν)B2(α)[α2ϱ2αΓ(2α+1)+2α(1−α)ϱαΓ(α+1)+(1−α)2]−(1−P)ν2−(βI0+ν)B3(α)×[α3ϱ3αΓ(3α+1)+3α2(1−α)ϱ2αΓ(2α+1)+3α(1−α)2ϱαΓ(α+1)+(1−α)3],R0=R0,R1=Pν−γI0−γR0B(α)[αϱαΓ(α+1)+(1−α)]−PνB2(α)[α2ϱ2αΓ(2α+1)+2α(1−α)ϱαΓ(α+1)+(1−α)2],R2=PνB(α)[αϱαΓ(α+1)+(1−α)]+γ(βS0I0−(γ+ν))+(νγI0−ν2R0)B2(α)×[α2ϱ2αΓ(2α+1)+2α(1−α)ϱαΓ(α+1)+(1−α)2]−Pν2B3(α)×[α3ϱ3αΓ(3α+1)+3α2(1−α)ϱ2αΓ(2α+1)+3α(1−α)2ϱαΓ(α+1)+(1−α)3]. | (4.7) |
Analogously, one can achieve the additional components. Hence, the infinite series solution of (3.1) is presented as:
{S(ϱ)=S0(ϱ)+S1(ϱ)+S2(ϱ)+...,I(ϱ)=I0(ϱ)+I1(ϱ)+I2(ϱ)+...,R(ϱ)=R0(ϱ)+R1(ϱ)+R2(ϱ)+.... | (4.8) |
In this analysis, we utilize S0=1,I0=0.5,R0=0,ν=0.4,β=0.8,γ=0.03,P1=0.9 and the EADM yields an approximate solution in the form of an infinite series. Thus, we compute the first four terms of (4.8) as follows:
S(ϱ)=1−0.75520B(α)(αϱαΓ(α+1)+(1−α))−0.446080B2(α)(α2ϱ2αΓ(2α+1)+2α(1−α)ϱαΓ(α+1)+(1−α)2)−0.01280B3(α)(α3ϱ3αΓ(3α+1)+3α2(1−α)ϱ2αΓ(2α+1)+3α(1−α)2ϱαΓ(α+1)+(1−α)3),I(ϱ)=0.5+0.185B(α)(αϱαΓ(α+1)+(1−α))−0.48680B2(α)(α2ϱ2αΓ(2α+1)+2α(1−α)ϱαΓ(α+1)+(1−α)2)+0.784B3(α)(α3ϱ3αΓ(3α+1)+3α2(1−α)ϱ2αΓ(2α+1)+3α(1−α)2ϱαΓ(α+1)+(1−α)3),R(ϱ)=0.345B(α)(αϱαΓ(α+1)+(1−α))−0.360720B2(α)(α2ϱ2αΓ(2α+1)+2α(1−α)ϱαΓ(α+1)+(1−α)2)−0.14400B3(α)(α3ϱ3αΓ(3α+1)+3α2(1−α)ϱ2αΓ(2α+1)+3α(1−α)2ϱαΓ(α+1)+(1−α)3). | (4.9) |
In Figure 1, the estimated solutions of several segments versus the provided data and pertaining to various fractional orders have been shown. Furthermore, the outcomes reveal that the response is constantly contingent on the time-fractional derivative and parametric settings. According to inoculation, the prevalence of the cured group progressively improves as the number of the vulnerable group diminishes. As shown in Figure 1, for a short period of time, responsiveness diminishes, resulting in a reduction in infection, as illustrated in Figure 2. Figure 3 depicts a typical case of glucose intolerance. This can be seen in the graphs for excessive plasma concentrations, where despite considerable increases in adjusted glucose metabolism concentrations in the liver, glycogen synthesis generation could be completely inhibited. The fractional-order derivative corresponds to the growth and contraction of distinct components. For a short period of time, the involved procedure is rapid on a small fractional order, but subsequently it reverses and remains sluggish on the identical lesser fractional order. As a result, in biological models, the fractional calculus depicts systemic mechanisms of deterioration and regeneration. The diffraction peaks and their associated vibrational frequencies are identical across scenarios; however, the timeframe of these peaks varies. Within fractional orders, the investigated model's transitory vibrations are considerably more spread out than those of its integer-order counterpart, whereby responses have prolonged interepidemic intervals. As a consequence, we arrived at the conclusion that, relying on the attribute values, the fractional-order framework simultaneously cumulates to the classical model and matches statistics equally, or incorporates data more accurately and excels the conventional paradigm.
Tables 1–3 exhibit the findings of the model of epidemic CHDs using Caputo, Caputo-Fabrizio, and ABC fractional derivatives for the susceptible class(S), infected class(I), and removed class(R), respectively. The summary statistics of the local stability investigations are supported by exisiting results provided by Haq et al. [37] and Baleanu et al. [38]. We proceeded by presenting several standard stability conclusions for a simplistic formula, as well as their application to a scenario in which the framework was reinforced substantially feasible by including a category of incubating persons and a group of biologically protective children.
ϱ | CDα(α=0.7) | CFDα(α=0.7) | ABCDα(α=0.7) | CDα(α=0.9) | CFDα(α=0.9) | ABCDα(α=0.9) |
0.1 | 5.3254×10−1 | 4.9673×10−1 | 4.6457×10−1 | 9.5907×10−1 | 8.9987×10−1 | 8.0459×10−1 |
0.2 | 4.6534×10−1 | 3.9846×10−1 | 3.2426×10−1 | 8.2342×10−2 | 7.8945×10−2 | 7.0285×10−1 |
0.3 | 7.7789×10−1 | 6.7789×10−1 | 6.0087×10−1 | 2.5673×10−1 | 1.9999×10−1 | 1.9807×10−1 |
0.4 | 5.0000×10−1 | 4.9999×10−1 | 4.9727×10−1 | 8.0099×10−2 | 8.0000×10−2 | 7.9055×10−2 |
0.5 | −4.0060×10−1 | −3.9889×10−1 | −3.5590×10−2 | 4.0000×10−1 | 3.9999×10−1 | 3.9146×10−1 |
0.6 | −2.3452×10−1 | −1.9445×10−1 | −1.4732×10−1 | 3.2378×10−2 | 2.9889×10−2 | 2.8317×10−1 |
0.7 | −3.8809×10−1 | −2.8998×10−1 | −2.5703×10−1 | 2.3334×10−1 | 1.9344×10−2 | 1.7227×10−1 |
0.8 | −4.0092×10−1 | −3.9987×10−1 | −3.6520×10−1 | 6.0001×10−2 | 5.9991×10−2 | 5.8529×10−2 |
0.9 | −5.3423×10−1 | −4.9924×10−1 | −4.7263×10−1 | −6.0022×10−2 | −5.9992×10−2 | −5.8002×10−2 |
1.0 | −6.8870×10−1 | −5.9991×10−1 | −5.7886×10−1 | −2.0090×10−1 | −1.9939×10−1 | −1.7730×10−1 |
ϱ | CDα(α=0.7) | CFDα(α=0.7) | ABCDα(α=0.7) | CDα(α=0.9) | CFDα(α=0.9) | ABCDα(α=0.9) |
0.1 | 6.0023×10−1 | 5.8871×10−1 | 5.2585×10−1 | 6.3465×10−1 | 5.8912×10−1 | 5.5334×10−1 |
0.2 | 6.1200×10−1 | 5.9233×10−1 | 5.3392×10−1 | 7.1022×10−1 | 5.9909×10−1 | 5.7221×10−1 |
0.3 | 6.9982×10−1 | 5.9833×10−1 | 5.4100×10−1 | 6.1122×10−1 | 5.9999×10−1 | 5.9330×10−1 |
0.4 | 6.0100×10−1 | 5.8842×10−1 | 5.4820×10−1 | 7.8881×10−1 | 6.7723×10−1 | 6.1708×10−1 |
0.5 | 6.3889×10−1 | 5.9844×10−1 | 5.5639×10−1 | 7.0821×10−1 | 6.8891×10−1 | 6.4370×10−1 |
0.6 | 6.3241×10−1 | 5.9999×10−1 | 5.6613×10−1 | 7.2312×10−1 | 6.7778×10−1 | 6.7328×10−1 |
0.7 | 6.1188×10−1 | 5.8800×10−1 | 5.7821×10−1 | 8.1817×10−1 | 7.7623×10−1 | 7.0587×10−1 |
0.8 | 6.0000×10−1 | 5.9999×10−1 | 5.9318×10−1 | 8.0023×10−1 | 7.9988×10−1 | 7.4152×10−1 |
0.9 | 7.7478×10−1 | 6.9999×10−1 | 6.1157×10−1 | 8.8000×10−1 | 8.0001×10−1 | 7.8025×10−1 |
1.0 | 7.4458×10−1 | 6.9901×10−1 | 6.3394×10−1 | 9.3114×10−1 | 8.8891×10−1 | 8.2213×10−1 |
ϱ | CDα(α=0.7) | CFDα(α=0.7) | ABCDα(α=0.7) | CDα(α=0.9) | CFDα(α=0.9) | ABCDα(α=0.9) |
0.1 | 2.6611×10−1 | 1.9911×10−1 | 1.7711×10−1 | 2.0001×10−1 | 1.9590×10−1 | 1.4498×10−1 |
0.2 | 3.0012×10−1 | 2.9345×10−1 | 2.0212×10−1 | 3.0079×10−1 | 2.9988×10−1 | 2.0347×10−1 |
0.3 | 3.9345×10−1 | 2.9999×10−1 | 2.1549×10−1 | 3.2000×10−1 | 2.9563×10−1 | 2.5365×10−1 |
0.4 | 3.0012×10−1 | 2.8921×10−1 | 2.2101×10−1 | 3.5663×10−1 | 2.9912×10−1 | 2.9702×10−1 |
0.5 | 3.0003×10−1 | 2.9900×10−1 | 2.2038×10−1 | 4.0000×10−1 | 3.9982×10−1 | 3.3429×10−1 |
0.6 | 3.1101×10−1 | 3.9999×10−1 | 2.1461×10−1 | 4.0044×10−1 | 3.9899×10−1 | 3.6584×10−1 |
0.7 | 3.0081×10−1 | 3.9900×10−1 | 2.0431×10−1 | 5.0000×10−1 | 4.1231×10−1 | 3.9192×10−1 |
0.8 | 2.5622×10−1 | 2.0077×10−1 | 1.8996×10−1 | 5.0012×10−1 | 4.8912×10−1 | 4.1267×10−1 |
0.9 | 2.0001×10−1 | 3.4491×10−1 | 1.7189×10−1 | 5.0099×10−1 | 4.9888×10−1 | 4.2818×10−1 |
1.0 | 2.6702×10−1 | 2.5556×10−1 | 1.5031×10−1 | 5.6540×10−1 | 5.0034×10−1 | 4.3856×10−1 |
Theorem 5.1. System of Eq (3.1) has a solutions by employing the Picard–Lindelöf technique.
Proof. By means of kernels defined in (3.2), we have
Θ1(ϱ,S(ϱ))=(1−P)ν−βS(ϱ)I(ϱ)−νS(ϱ),Θ2(ϱ,I(ϱ))=βS(ϱ)I(ϱ)−(γ+ν)I(ϱ),Θ3(ϱ,R(ϱ))=Pν+γI(ϱ)−νR(ϱ), | (5.1) |
where Θ1(ϱ,S(ϱ)),Θ2(ϱ,I(ϱ)) and Θ3(ϱ,R(ϱ)) are contraction mappings according to (3.6), respectively.
Assume that
Υ1=supQa1,B|Θ1(ϱ,S(ϱ))|,Υ2=supQa1,b2|Θ1(ϱ,S(ϱ))|,Υ3=supQa1,b3|Θ1(ϱ,S(ϱ))| | (5.2) |
where
Qa1,b1=|ϱ−a1,ϱ+a1|×[Θ1−b1,Θ1+b1]=W1×Y1, |
Qa1,b2=|ϱ−a1,ϱ+a1|×[Θ2−b2,Θ2+b2]=W1×Y2, |
Qa1,b3=|ϱ−a1,ϱ+a1|×[Θ2−b3,Θ2+b3]=W1×Y3. |
Taking the Picard operator, we have
Φ:Q(W1,Y1,Y2,Y3)↦Q(W1,Y1,Y2,Y3), | (5.3) |
presented as follows:
ΦΞ(ϱ)=Ξ0(ϱ)+Δ(ϱ,Θ(ϱ))1−αB(α)+αB(α)Γ(α)ϱ∫0(ϱ−x)α−1Δ(x,Θ(x))dx, | (5.4) |
where Ξ(ϱ)={S(ϱ),I(ϱ),R(ϱ)},Ξ0(ϱ)={ζ1,ζ2,ζ3} and Δ(ϱ,Θ(ϱ))={Θ1(ϱ,S(ϱ)),Θ2(ϱ,I(ϱ)),Θ3(ϱ,R(ϱ))}.
Next, we surmise that the solutions of the fractional CHD model are bounded in a specified time domain,
‖Ξ(ϱ)‖∞≤max{Θ1,Θ2,Θ3}‖Ξ(ϱ)−Ξ0(ϱ)‖=‖Δ(ϱ,Θ(ϱ))1−αB(α)+αB(α)Γ(α)ϱ∫0(ϱ−x)α−1Δ(x,Θ(x))dx‖≤1−αB(α)‖Δ(ϱ,Θ(ϱ))‖+αB(α)Γ(α)ϱ∫0(ϱ−x)α−1‖Δ(x,Θ(x))‖dx≤(1−αB(α)+ζϱαB(α)Γ(α))max{Y1,Y2,Y3}≤ρζ≤Y, | (5.5) |
then we have
ρ<Yζ. |
Taking into account the fixed point postulate via the Banach space with the metric, we get
‖ΦΞ1−ΦΞ2‖∞=supϱ↦W1|Ξ1−Ξ2|. | (5.6) |
Now we have
‖ΦΞ1−ΦΞ2‖=‖[Δ(ϱ,Ξ1(ϱ))−Δ(ϱ,Ξ2(ϱ))]1−αB(α)+αB(α)Γ(α)ϱ∫0(ϱ−x)α−1[Δ(x,Ξ1(x))−Δ(x,Ξ2(x))]‖dx≤1−αB(α)‖Δ(ϱ,Ξ1(ϱ))−Δ(ϱ,Ξ2(ϱ))‖+αB(α)Γ(α)ϱ∫0(ϱ−x)α−1‖Δ(x,Ξ1(x))−Δ(x,Ξ2(x))‖dx≤1−αB(α)ζ‖Ξ1(ϱ)−Ξ2(ϱ)‖+ϱαζB(α)Γ(α)‖Ξ(x)−Ξ2(x)‖≤(1−αB(α)ζ+ϱαζB(α)Γ(α))‖Ξ1(x)−Ξ2(x)‖≤ρζ‖Ξ1(x)−Ξ2(x)‖,ζ<1. | (5.7) |
Since Ξ is a contraction and ρζ<1. Also, the introduced operator, Φ is contraction. Thus, the CHD model concerning the ABC derivative presented in (3.1) has a fixed point.
Assume that (φ,‖.‖) denotes a Banach space and U signifies a self-map of φ. Also, suppose that ℏn+1=Ψ(U,ℏn) be a recursive scheme. Surmise that a fixed point set ℵ(U) of U has at least one element. Suppose that ℏn is convergent and tends to z1∈ℵ(U). If {ℏn}⊆φ and introducing an=‖ℏn+1−Ψ(U,ℏn)‖ and if limn↦∞an1=0 gives the outcome limn↦∞ℏn=z1, then the recursive approach ℏn+1=Ψ(U,ℏn) is said to be U-stable.
Theorem 5.2. ([46]) Assume that a Banach space (φ,‖.‖) and U be a self-map of the Banach space φ yields the outcome
‖Ux−Uy‖≤˜H‖x−Ux‖+ℏ‖x−y‖,∀x,y∈φ, | (5.8) |
where ˜H and ℏ such that ℏ∈[0,1],˜H≥0. Also, surmise that U is Picard U-stable.
We surmise that the following recursive formulae have an association with the fractional CHD model (3.1)
{Sn+1(ϱ)=S(ϱ)+E−1[ωα+1−αB(α)E[(1−P)ν−βSn(ϱ)In(ϱ)−νSn(ϱ)]],In+1(ϱ)=I(ϱ)+E−1[ωα+1−αB(α)E[βSn(ϱ)In(ϱ)−(γ+ν)In(ϱ)]],Rn+1(ϱ)=R(ϱ)+E−1[ωα+1−αB(α)E[Pν+γIn(ϱ)−νRn(ϱ)]]. |
The term ωα+1−αB(α) considered to be the Lagrange multiplier in the aforesaid system.
In the next result, we present the following theorem.
Theorem 5.3. Assume that the self-map G is represented as follows:
{G(Sn(ϱ))=Sn+1(ϱ)=S(ϱ)+E−1[ωα+1−αB(α)E[(1−P)ν−βSn(ϱ)In(ϱ)−νSn(ϱ)]],G(In(ϱ))=In+1(ϱ)=I(ϱ)+E−1[ωα+1−αB(α)E[βSn(ϱ)In(ϱ)−(γ+ν)In(ϱ)]],G(Rn(ϱ))=Rn+1(ϱ)=R(ϱ)+E−1[ωα+1−αB(α)E[Pν+γIn(ϱ)−νRn(ϱ)]], |
then the recursive system are G stable in L2∈(a1,B) if (1−P)ν−βφ4(ϱ)−νφ1(ϱ)<1,βφ4(ϱ)−(γ+ν)φ2(ϱ)<1 and Pν+γφ2(ϱ)−νφ3(ϱ)<1.
Proof. Initially, we prove that G has a fixed point. To prove the above consequence, we obtain the outcome presented below for (n,m)∈B×B.
‖G(Sn(ϱ))−G(Sm(ϱ))‖≤‖Sn(ϱ)−Sm(ϱ)‖+‖E−1[ωα+1−αB(α)E[(1−P)ν−β(Sn(ϱ)In(ϱ)−Sm(ϱ)Im(ϱ))−ν(Sn(ϱ)−Sm(ϱ))]]‖,‖G(In(ϱ))−G(Im(ϱ))‖≤‖In(ϱ)−Im(ϱ)‖+‖E−1[ωα+1−αB(α)E[β(Sn(ϱ)In(ϱ)−Sm(ϱ)Im(ϱ))−(γ+ν)(In(ϱ)−Im(ϱ))]]‖ | (5.9) |
and
‖G(Rn(ϱ))−G(Rm(ϱ))‖≤‖Rn(ϱ)−Rm(ϱ)‖+‖E−1[ωα+1−αB(α)E[Pν+γ(In(ϱ)−Im(ϱ))−ν(Rn(ϱ)−Rm(ϱ))]]‖. | (5.10) |
Also, we have that
‖Sn(ϱ)−Sm(ϱ)‖≊‖In(ϱ)−Im(ϱ)‖≊‖Rn(ϱ)−Rm(ϱ)‖≊‖Sn(ϱ)In(ϱ)−Sm(ϱ)Im(ϱ)‖. | (5.11) |
By plugging (5.11) in (5.9) and (5.10), we have
‖G(Sn(ϱ))−G(Sm(ϱ))‖≤((1−P)ν−βφ4(ϱ)−νφ1(ϱ))‖Sn(ϱ)−Sm(ϱ)‖,‖G(In(ϱ))−G(Im(ϱ))‖≤(βφ4(ϱ)−(γ+ν)φ2(ϱ))‖In(ϱ)−Im(ϱ)‖ | (5.12) |
and
‖G(Rn(ϱ))−G(Rm(ϱ))‖≤(Pν+γφ2(ϱ)−νφ3(ϱ))‖Rn(ϱ)−Rm(ϱ)‖, | (5.13) |
where φi,i=1,....,4, are mappings arising from E−1[ωα+1−αB(α)E(˙)] along with ((1−P)ν−βφ4(ϱ)−νφ1(ϱ))<1,(βφ4(ϱ)−(γ+ν)φ2(ϱ))<1 and (Pν+γφ2(ϱ)−νφ3(ϱ))<1.
As a result, the self-map G has a fixed point. Moreover, the assumptions connected with the Theorem 5.2 are satisfied by G. Now, suppose that (5.12) and (5.13) hold, thus we have
˜H={((1−P)ν−βφ4(ϱ)−νφ1(ϱ)),(βφ4(ϱ)−(γ+ν)φ2(ϱ)),(Pν+γφ2(ϱ)−νφ3(ϱ)). | (5.14) |
It is noteworthy that in map G the supposition concerned with Theorem 5.2 satisfied. Thus, for the supposed map, G and the hypothesis relating to Theorem 5.2 are satisfied, hence G is Picard-stable. This completes the proof.
In this study, the generalized fractional derivative is implemented to examine the mathematical model of CHDs. Also, with the assistance of the contraction mapping theorem, we demonstrated that the response of the proposed model (3.1) exists and has at least one solution. The Picard–Lindelöf approach with the self-map U used to validate the solution's stability. Furthermore, using the EADM algorithm, we comprehended the approximate results for various fractional-orders using the Matlab/Maple application, and we concluded that the results of the projected model (3.1) approach the classical solution when α↦1. As a result, it can be concluded that EADM is a controllable, simplistic, and more powerful analytical process for analyzing linear/non-linear phenomena. The findings of this research are extremely beneficial to healthcare professionals who interact with peditric and associated concerns. Consequently, we deduce that the ABC derivative can be used to investigate the physical, pharmacological, physiological, medicinal, sociological, and economic systems.
This research was supported by Taif University Research Supporting Project Number (TURSP-2020/164), Taif University, Taif, Saudi Arabia.
The authors declare that they have no competing interests.
[1] | F. Haq, K. Shah, G. Rahman, M. Shahzad, Numerical analysis of fractional order model of HIV-1 infection of CD4+ T-cells, Comput. Methods Differ. Eq., 5 (2017), 1–11. |
[2] |
I. Koca, Analysis of rubella disease model with non-local and non-singular fractional derivatives, IJOCTA, 8 (2018), 17–25. http://dx.doi.org/10.11121/ijocta.01.2018.00532 doi: 10.11121/ijocta.01.2018.00532
![]() |
[3] |
A. A. Khan, Z. Hammouch, D. Baleanu, Modeling the dynamics of hepatitis E via the Caputo-Fabrizio derivative, Math. Model. Nat. Phenom., 14 (2019), 311. http://dx.doi.org/10.1051/mmnp/2018074 doi: 10.1051/mmnp/2018074
![]() |
[4] |
S. Ullah, M. A. Khan, M. Farooq, Z. Hammouch, D. Baleanu, A fractional model for the dynamics of tuberculosis infection using Caputo-Fabrizio derivative, Discrete Cont. Dyn. Syst., 13 (2020), 975–993. http://dx.doi.org/10.3934/dcdss.2020057 doi: 10.3934/dcdss.2020057
![]() |
[5] |
J. Singh, D. Kumar, Z. Hammouch, A. Atangana, A fractional epidemiological model for computer viruses pertaining to a new fractional derivative, Appl. Math. Comput., 316 (2018), 504–515. http://dx.doi.org/10.1016/j.amc.2017.08.048 doi: 10.1016/j.amc.2017.08.048
![]() |
[6] |
X.-P. Li, N. Gul, M. A. Khan, R. Bilal, A. Ali, M. Y. Alshahrani, et al., A new Hepatitis B model in light of asymptomatic carriers and vaccination study through Atangana-Baleanu derivative, Results Phys., 29 (2021), 104603. http://dx.doi.org/10.1016/j.rinp.2021.104603 doi: 10.1016/j.rinp.2021.104603
![]() |
[7] |
X.-P. Li, Y. Wang, M. A. Khan, M. Y. Alshahrani, T. Muhammad, A dynamical study of SARS-COV-2: A study of third wave, Results Phys., 29 (2021), 104705. http://dx.doi.org/10.1016/j.rinp.2021.104705 doi: 10.1016/j.rinp.2021.104705
![]() |
[8] |
X.-P. Li, H. Al Bayatti, A. Din, A. Zeb, A vigorous study of fractional order COVID-19 model via ABC derivatives, Results Phys., 29 (2021), 104737. http://dx.doi.org/10.1016/j.rinp.2021.104737 doi: 10.1016/j.rinp.2021.104737
![]() |
[9] |
S.-S. Zhou, M. I. Khan, S. Qayyum, B. C. Prasannakumara, R. N. Kumar, S. U. Khan, et al., Nonlinear mixed convective Williamson nanofluid flow with the suspension of gyrotactic microorganisms, Int. J. Mod. Phys. B, 35 (2021), 2150145. http://dx.doi.org/10.1142/S0217979221501459 doi: 10.1142/S0217979221501459
![]() |
[10] |
Y.-Q. Song, H. Waqas, K. Al-Khaled, U. Farooq, S. U. Khan, M. I. Khan, et al., Bioconvection analysis for Sutterby nanofluid over an axially stretched cylinder with melting heat transfer and variable thermal features: A Marangoni and solutal model, Alex. Eng. J., 60 (2021), 4663–4675. http://dx.doi.org/10.1016/j.aej.2021.03.056 doi: 10.1016/j.aej.2021.03.056
![]() |
[11] |
Y.-Q. Song, S. A. Khan, M. Imran, H. Waqas, S. U. Khan, M. I. Khan, et al., Applications of modified Darcy law and nonlinear thermal radiation in bioconvection flow of micropolar nanofluid over an off centered rotating disk, Alex. Eng. J., 60 (2021), 4607–4618. http://dx.doi.org/10.1016/j.aej.2021.03.053 doi: 10.1016/j.aej.2021.03.053
![]() |
[12] |
Y.-Q. Song, M. Hassan, S. U. Khan, M. I. Khan, S. Qayyum, Y.-M. Chu, et al., Thermal and boundary layer flow analysis for MWCNT-SiO2 hybrid nanoparticles: an experimental thermal model, Mod. Phys. Lett. B, 35 (2021), 2150303. http://dx.doi.org/10.1142/S0217984921503036 doi: 10.1142/S0217984921503036
![]() |
[13] |
J. F. Li, H. Jahanshahi, S. Kacar, Y.-M. Chu, J. F. Gómez-Aguilar, N. D. Alotaibi, et al., On the variable-order fractional memristor oscillator: data security applications and synchronization using a type-2 fuzzy disturbance observer-based robust control, Chaos Soliton. Fract., 145 (2021), 110681. http://dx.doi.org/10.1016/j.chaos.2021.110681 doi: 10.1016/j.chaos.2021.110681
![]() |
[14] |
P.-Y. Xiong, A. Almarashi, H. A. Dhahad, W. H. Alawee, A. Issakhov, Y.-M. Chu, Nanoparticles for phase change process of water utilizing FEM, J. Mol. Liq., 334 (2021), 116096. http://dx.doi.org/10.1016/j.molliq.2021.116096 doi: 10.1016/j.molliq.2021.116096
![]() |
[15] |
P.-Y. Xiong, A. Hamid, Y.-M. Chu, M. I. Khan, R. J. P. Gowda, R. N. Kumar, et al., Dynamics of multiple solutions of Darcy-Forchheimer saturated flow of Cross nanofluid by a vertical thin needle point, Eur. Phys. J. Plus, 136 (2021), 315. http://dx.doi.org/10.1140/epjp/s13360-021-01294-2 doi: 10.1140/epjp/s13360-021-01294-2
![]() |
[16] |
M. A. Dokuyucu, E. Celik, H. Bulut, H. M. Baskonus, Cancer treatment model with the Caputo-Fabrizio fractional derivative, Eur. Phys. J. Plus, 133 (2018), 92. http://dx.doi.org/10.1140/epjp/i2018-11950-y doi: 10.1140/epjp/i2018-11950-y
![]() |
[17] |
H. Kang, X. Fu, Epidemic spreading and global stability of an SIS model with an infective vector on complex networks, Commun. Nonlinear Sci. Numer. Simul., 27 (2015), 30–39. http://dx.doi.org/10.1016/j.cnsns.2015.02.018 doi: 10.1016/j.cnsns.2015.02.018
![]() |
[18] |
X. Liu, Y. Liu, Y. Zhang, Z. Chen, Z. Tang, Q. Xu, et al., Pre-existing immunity with high neutralizing activity to 2009 pandemic H1N1 influenza virus in shanghai population, PloS ONE, 8 (2013), e58810. http://dx.doi.org/10.1371/journal.pone.0058810 doi: 10.1371/journal.pone.0058810
![]() |
[19] | K. Karthikeyan, P. Karthikeyan, H. M. Baskonus, K. Venkatachalam, Y. -M. Chu, Almost sectorial operators on Ψ-Hilfer derivative fractional impulsive integro-differential equations, Math. Method. Appl. Sci, 2021, in press. http://dx.doi.org/10.1002/mma.7954 |
[20] |
R. K. Upadhyay, P. Roy, Spread of a disease and its effect on population dynamics in an Eco-epidemiological system, Commun. Nonlinear Sci. Numer. Simul., 19 (2014), 4170–4184. http://dx.doi.org/10.1016/j.cnsns.2014.04.016 doi: 10.1016/j.cnsns.2014.04.016
![]() |
[21] |
E. Ucar, N. Ozdemir, E. Altun, Fractional order model of immune cells influenced by cancer cells, Math. Model. Nat. Phenom., 14 (2019), 308. http://dx.doi.org/10.1051/mmnp/2019002 doi: 10.1051/mmnp/2019002
![]() |
[22] |
T. Zhang, X. Meng, Y. Song, T. Zhang, A stage-structured predator-prey si model with disease in the prey and impulsive effects, Math. Model. Anal., 18 (2013), 505–528. http://dx.doi.org/10.3846/13926292.2013.840866 doi: 10.3846/13926292.2013.840866
![]() |
[23] |
A. Atangana, B. S. T. Alkahtani, Analysis of the keller-segel model with a fractional derivative without singular kernel, Entropy, 17 (2015), 4439–4453. http://dx.doi.org/10.3390/e17064439 doi: 10.3390/e17064439
![]() |
[24] |
S.-B. Chen, S. Rashid, M. A. Noor, Z. Hammouch, Y.-M. Chu, New fractional approaches for n-polynomial P-convexity with applications in special function theory, Adv. Differ. Equ., 2020 (2020), 543. http://dx.doi.org/10.1186/s13662-020-03000-5 doi: 10.1186/s13662-020-03000-5
![]() |
[25] |
S.-B. Chen, S. Rashid, M. A. Noor, R. Ashraf, Y.-M. Chu, A new approach on fractional calculus and probability density function, AIMS Mathematics, 5 (2020), 7041–7054. http://dx.doi.org/10.3934/math.2020451 doi: 10.3934/math.2020451
![]() |
[26] |
S.-B. Chen, S. Rashid, Z. Hammouch, M. A. Noor, R. Ashraf, Y.-M. Chu, Integral inequalities via Raina's fractional integrals operator with respect to a monotone function, Adv. Differ. Equ., 2020 (2020), 647. http://dx.doi.org/10.1186/s13662-020-03108-8 doi: 10.1186/s13662-020-03108-8
![]() |
[27] |
S.-B. Chen, S. Saleem, M. N. Alghamdi, K. S. Nisar, A. Arsalanloo, A. Issakhov, et al., Combined effect of using porous media and nano-particle on melting performance of PCM filled enclosure with triangular double fins, Case Stud. Therm. Eng., 25 (2021), 100939. http://dx.doi.org/10.1016/j.csite.2021.100939 doi: 10.1016/j.csite.2021.100939
![]() |
[28] |
S.-B. Chen, S. Soradi-Zeid, H. Jahanshahi, R. Alcaraz, J. F. Gómez-Aguilar, S. Bekiros, et al., Optimal control of time-delay fractional equations via a joint application of radial basis functions and collocation method, Entropy, 22 (2020), 1213. http://dx.doi.org/10.3390/e22111213 doi: 10.3390/e22111213
![]() |
[29] | M. Caputo, Elasticita e Dissipazione, Bologna: Zanichelli, 1969. |
[30] | I. Podlubny, Fractional differential equations, San Diego: Academic Press, 1999. |
[31] | R. Hilfer, Applications of fractional calculus in physics, Singapore: Word Scientific, 2000. |
[32] | D. Baleanu, Z. B. Guvenc, J. A. Tenreiro Machado, New trends in nanotechnology and fractional calculus applications, Dordrecht: Springer, 2010. http://dx.doi.org/10.1007/978-90-481-3293-5 |
[33] |
J. Singh, D. Kumar, D. Baleanu, On the analysis of chemical kinetics system pertaining to a fractional derivative with Mittag–Leffler type kernel, Chaos, 27 (2017), 103113. http://dx.doi.org/10.1063/1.4995032 doi: 10.1063/1.4995032
![]() |
[34] |
D. Kumar, J. Singh, D. Baleanu, Sushila, Analysis of regularized long-wave equation associated with a new fractional operator with Mittag–Leffler type kernel, Physica A, 492 (2018), 155–167. http://dx.doi.org/10.1016/j.physa.2017.10.002 doi: 10.1016/j.physa.2017.10.002
![]() |
[35] | A. Atangana, D. Baleanu, New fractional derivatives with non-local and non-singular kernel theory and application to heat transfer model, Therm. Sci., 20 (2016), 763–769. |
[36] |
A. A. M. Arafa, S. Z. Rida, M. Khalil, Solutions of fractional order model of childhood disease dynamics with constant vaccination strategy, Math. Sci. Lett., 1 (2012), 17–23. http://dx.doi.org/10.12785/msl/010103 doi: 10.12785/msl/010103
![]() |
[37] |
F. Haq, M. Shahzad, S. Muhammad, H. A. Wahab, G. U. Rahman, Numerical analysis of fractional order epidemic model of childhood diseases, Discrete Dyn. Nat. Soc., 2017 (2017), 4057089. http://dx.doi.org/10.1155/2017/4057089 doi: 10.1155/2017/4057089
![]() |
[38] |
D. Baleanu, S. M. Aydogn, H. Mohammadi, S. Rezapour, On modelling of epidemic childhood diseases with the Caputo-Fabrizio derivative by using the Laplace Adomian decomposition method, Alex. Eng.. J., 59 (2020), 3029–3039. http://dx.doi.org/10.1016/j.aej.2020.05.007 doi: 10.1016/j.aej.2020.05.007
![]() |
[39] |
H. Singh, J. Dhar, H. S. Bhatti, S. Chandok, An epidemic model of childhood disease dynamics with maturation delay and latent period of infection, Model. Earth Syst. Environ., 2 (2016), 79. http://dx.doi.org/10.1007/s40808-016-0131-9 doi: 10.1007/s40808-016-0131-9
![]() |
[40] |
S. Rashid, Z. Hammouch, H. Aydi, A. G. Ahmad, A. M. Alsharif, Novel computations of the time-fractional Fisher's model via generalized fractional integral operators by means of the Elzaki transform, Fractal Fract., 5 (2021), 94. http://dx.doi.org/10.3390/fractalfract5030094 doi: 10.3390/fractalfract5030094
![]() |
[41] |
S. Rashid, K. T. Kubra, J. L. G. Guirao, Construction of an approximate analytical solution for multi-dimensional fractional Zakharov–Kuznetsov equation via Aboodh Adomian decomposition method, Symmetry, 13 (2021), 1542. http://dx.doi.org/10.3390/sym13081542 doi: 10.3390/sym13081542
![]() |
[42] | T. M. Elzaki, The new integral transform Elzaki transform, Global Journal of Pure and Applied Mathematics, 7 (2011), 57–64. |
[43] |
A. A. Alderremy, T. M. Elzaki, M. Chamekh, New transform iterative method for solving some Klein-Gordon equations, Results Phys., 10 (2018), 655–659. http://dx.doi.org/10.1016/j.rinp.2018.07.004 doi: 10.1016/j.rinp.2018.07.004
![]() |
[44] | A. H. Sedeeg, A coupling Elzaki transform and homotopy perturbation method for solving nonlinear fractional heat-like equations, American Journal of Mathematical and Computer Modelling, 1 (2016), 15–20. |
[45] |
M. Yavuz, T. Abdeljawad, Nonlinear regularized long-wave models with a new integral transformation applied to the fractional derivative with power and Mittag-Leffler kernel, Adv. Differ. Equ., 2020 (2020), 367. http://dx.doi.org/10.1186/s13662-020-02828-1 doi: 10.1186/s13662-020-02828-1
![]() |
[46] |
Y. Qing, B. E. Rhoades, T-stability of Picard iteration in metric space, Fixed Point Theory Appl., 2008 (2008), 418971. http://dx.doi.org/10.1155/2008/418971 doi: 10.1155/2008/418971
![]() |
1. | Saima Rashid, Rehana Ashraf, Fahd Jarad, Strong interaction of Jafari decomposition method with nonlinear fractional-order partial differential equations arising in plasma via the singular and nonsingular kernels, 2022, 7, 2473-6988, 7936, 10.3934/math.2022444 | |
2. | Fouad Mohammad Salama, Nur Nadiah Abd Hamid, Umair Ali, Norhashidah Hj. Mohd Ali, Fast hybrid explicit group methods for solving 2D fractional advection-diffusion equation, 2022, 7, 2473-6988, 15854, 10.3934/math.2022868 | |
3. | Fouad Mohammad Salama, On Numerical Simulations of Variable-Order Fractional Cable Equation Arising in Neuronal Dynamics, 2024, 8, 2504-3110, 282, 10.3390/fractalfract8050282 |
ϱ | CDα(α=0.7) | CFDα(α=0.7) | ABCDα(α=0.7) | CDα(α=0.9) | CFDα(α=0.9) | ABCDα(α=0.9) |
0.1 | 5.3254×10−1 | 4.9673×10−1 | 4.6457×10−1 | 9.5907×10−1 | 8.9987×10−1 | 8.0459×10−1 |
0.2 | 4.6534×10−1 | 3.9846×10−1 | 3.2426×10−1 | 8.2342×10−2 | 7.8945×10−2 | 7.0285×10−1 |
0.3 | 7.7789×10−1 | 6.7789×10−1 | 6.0087×10−1 | 2.5673×10−1 | 1.9999×10−1 | 1.9807×10−1 |
0.4 | 5.0000×10−1 | 4.9999×10−1 | 4.9727×10−1 | 8.0099×10−2 | 8.0000×10−2 | 7.9055×10−2 |
0.5 | −4.0060×10−1 | −3.9889×10−1 | −3.5590×10−2 | 4.0000×10−1 | 3.9999×10−1 | 3.9146×10−1 |
0.6 | −2.3452×10−1 | −1.9445×10−1 | −1.4732×10−1 | 3.2378×10−2 | 2.9889×10−2 | 2.8317×10−1 |
0.7 | −3.8809×10−1 | −2.8998×10−1 | −2.5703×10−1 | 2.3334×10−1 | 1.9344×10−2 | 1.7227×10−1 |
0.8 | −4.0092×10−1 | −3.9987×10−1 | −3.6520×10−1 | 6.0001×10−2 | 5.9991×10−2 | 5.8529×10−2 |
0.9 | −5.3423×10−1 | −4.9924×10−1 | −4.7263×10−1 | −6.0022×10−2 | −5.9992×10−2 | −5.8002×10−2 |
1.0 | −6.8870×10−1 | −5.9991×10−1 | −5.7886×10−1 | −2.0090×10−1 | −1.9939×10−1 | −1.7730×10−1 |
ϱ | CDα(α=0.7) | CFDα(α=0.7) | ABCDα(α=0.7) | CDα(α=0.9) | CFDα(α=0.9) | ABCDα(α=0.9) |
0.1 | 6.0023×10−1 | 5.8871×10−1 | 5.2585×10−1 | 6.3465×10−1 | 5.8912×10−1 | 5.5334×10−1 |
0.2 | 6.1200×10−1 | 5.9233×10−1 | 5.3392×10−1 | 7.1022×10−1 | 5.9909×10−1 | 5.7221×10−1 |
0.3 | 6.9982×10−1 | 5.9833×10−1 | 5.4100×10−1 | 6.1122×10−1 | 5.9999×10−1 | 5.9330×10−1 |
0.4 | 6.0100×10−1 | 5.8842×10−1 | 5.4820×10−1 | 7.8881×10−1 | 6.7723×10−1 | 6.1708×10−1 |
0.5 | 6.3889×10−1 | 5.9844×10−1 | 5.5639×10−1 | 7.0821×10−1 | 6.8891×10−1 | 6.4370×10−1 |
0.6 | 6.3241×10−1 | 5.9999×10−1 | 5.6613×10−1 | 7.2312×10−1 | 6.7778×10−1 | 6.7328×10−1 |
0.7 | 6.1188×10−1 | 5.8800×10−1 | 5.7821×10−1 | 8.1817×10−1 | 7.7623×10−1 | 7.0587×10−1 |
0.8 | 6.0000×10−1 | 5.9999×10−1 | 5.9318×10−1 | 8.0023×10−1 | 7.9988×10−1 | 7.4152×10−1 |
0.9 | 7.7478×10−1 | 6.9999×10−1 | 6.1157×10−1 | 8.8000×10−1 | 8.0001×10−1 | 7.8025×10−1 |
1.0 | 7.4458×10−1 | 6.9901×10−1 | 6.3394×10−1 | 9.3114×10−1 | 8.8891×10−1 | 8.2213×10−1 |
ϱ | CDα(α=0.7) | CFDα(α=0.7) | ABCDα(α=0.7) | CDα(α=0.9) | CFDα(α=0.9) | ABCDα(α=0.9) |
0.1 | 2.6611×10−1 | 1.9911×10−1 | 1.7711×10−1 | 2.0001×10−1 | 1.9590×10−1 | 1.4498×10−1 |
0.2 | 3.0012×10−1 | 2.9345×10−1 | 2.0212×10−1 | 3.0079×10−1 | 2.9988×10−1 | 2.0347×10−1 |
0.3 | 3.9345×10−1 | 2.9999×10−1 | 2.1549×10−1 | 3.2000×10−1 | 2.9563×10−1 | 2.5365×10−1 |
0.4 | 3.0012×10−1 | 2.8921×10−1 | 2.2101×10−1 | 3.5663×10−1 | 2.9912×10−1 | 2.9702×10−1 |
0.5 | 3.0003×10−1 | 2.9900×10−1 | 2.2038×10−1 | 4.0000×10−1 | 3.9982×10−1 | 3.3429×10−1 |
0.6 | 3.1101×10−1 | 3.9999×10−1 | 2.1461×10−1 | 4.0044×10−1 | 3.9899×10−1 | 3.6584×10−1 |
0.7 | 3.0081×10−1 | 3.9900×10−1 | 2.0431×10−1 | 5.0000×10−1 | 4.1231×10−1 | 3.9192×10−1 |
0.8 | 2.5622×10−1 | 2.0077×10−1 | 1.8996×10−1 | 5.0012×10−1 | 4.8912×10−1 | 4.1267×10−1 |
0.9 | 2.0001×10−1 | 3.4491×10−1 | 1.7189×10−1 | 5.0099×10−1 | 4.9888×10−1 | 4.2818×10−1 |
1.0 | 2.6702×10−1 | 2.5556×10−1 | 1.5031×10−1 | 5.6540×10−1 | 5.0034×10−1 | 4.3856×10−1 |
ϱ | CDα(α=0.7) | CFDα(α=0.7) | ABCDα(α=0.7) | CDα(α=0.9) | CFDα(α=0.9) | ABCDα(α=0.9) |
0.1 | 5.3254×10−1 | 4.9673×10−1 | 4.6457×10−1 | 9.5907×10−1 | 8.9987×10−1 | 8.0459×10−1 |
0.2 | 4.6534×10−1 | 3.9846×10−1 | 3.2426×10−1 | 8.2342×10−2 | 7.8945×10−2 | 7.0285×10−1 |
0.3 | 7.7789×10−1 | 6.7789×10−1 | 6.0087×10−1 | 2.5673×10−1 | 1.9999×10−1 | 1.9807×10−1 |
0.4 | 5.0000×10−1 | 4.9999×10−1 | 4.9727×10−1 | 8.0099×10−2 | 8.0000×10−2 | 7.9055×10−2 |
0.5 | −4.0060×10−1 | −3.9889×10−1 | −3.5590×10−2 | 4.0000×10−1 | 3.9999×10−1 | 3.9146×10−1 |
0.6 | −2.3452×10−1 | −1.9445×10−1 | −1.4732×10−1 | 3.2378×10−2 | 2.9889×10−2 | 2.8317×10−1 |
0.7 | −3.8809×10−1 | −2.8998×10−1 | −2.5703×10−1 | 2.3334×10−1 | 1.9344×10−2 | 1.7227×10−1 |
0.8 | −4.0092×10−1 | −3.9987×10−1 | −3.6520×10−1 | 6.0001×10−2 | 5.9991×10−2 | 5.8529×10−2 |
0.9 | −5.3423×10−1 | −4.9924×10−1 | −4.7263×10−1 | −6.0022×10−2 | −5.9992×10−2 | −5.8002×10−2 |
1.0 | −6.8870×10−1 | −5.9991×10−1 | −5.7886×10−1 | −2.0090×10−1 | −1.9939×10−1 | −1.7730×10−1 |
ϱ | CDα(α=0.7) | CFDα(α=0.7) | ABCDα(α=0.7) | CDα(α=0.9) | CFDα(α=0.9) | ABCDα(α=0.9) |
0.1 | 6.0023×10−1 | 5.8871×10−1 | 5.2585×10−1 | 6.3465×10−1 | 5.8912×10−1 | 5.5334×10−1 |
0.2 | 6.1200×10−1 | 5.9233×10−1 | 5.3392×10−1 | 7.1022×10−1 | 5.9909×10−1 | 5.7221×10−1 |
0.3 | 6.9982×10−1 | 5.9833×10−1 | 5.4100×10−1 | 6.1122×10−1 | 5.9999×10−1 | 5.9330×10−1 |
0.4 | 6.0100×10−1 | 5.8842×10−1 | 5.4820×10−1 | 7.8881×10−1 | 6.7723×10−1 | 6.1708×10−1 |
0.5 | 6.3889×10−1 | 5.9844×10−1 | 5.5639×10−1 | 7.0821×10−1 | 6.8891×10−1 | 6.4370×10−1 |
0.6 | 6.3241×10−1 | 5.9999×10−1 | 5.6613×10−1 | 7.2312×10−1 | 6.7778×10−1 | 6.7328×10−1 |
0.7 | 6.1188×10−1 | 5.8800×10−1 | 5.7821×10−1 | 8.1817×10−1 | 7.7623×10−1 | 7.0587×10−1 |
0.8 | 6.0000×10−1 | 5.9999×10−1 | 5.9318×10−1 | 8.0023×10−1 | 7.9988×10−1 | 7.4152×10−1 |
0.9 | 7.7478×10−1 | 6.9999×10−1 | 6.1157×10−1 | 8.8000×10−1 | 8.0001×10−1 | 7.8025×10−1 |
1.0 | 7.4458×10−1 | 6.9901×10−1 | 6.3394×10−1 | 9.3114×10−1 | 8.8891×10−1 | 8.2213×10−1 |
ϱ | CDα(α=0.7) | CFDα(α=0.7) | ABCDα(α=0.7) | CDα(α=0.9) | CFDα(α=0.9) | ABCDα(α=0.9) |
0.1 | 2.6611×10−1 | 1.9911×10−1 | 1.7711×10−1 | 2.0001×10−1 | 1.9590×10−1 | 1.4498×10−1 |
0.2 | 3.0012×10−1 | 2.9345×10−1 | 2.0212×10−1 | 3.0079×10−1 | 2.9988×10−1 | 2.0347×10−1 |
0.3 | 3.9345×10−1 | 2.9999×10−1 | 2.1549×10−1 | 3.2000×10−1 | 2.9563×10−1 | 2.5365×10−1 |
0.4 | 3.0012×10−1 | 2.8921×10−1 | 2.2101×10−1 | 3.5663×10−1 | 2.9912×10−1 | 2.9702×10−1 |
0.5 | 3.0003×10−1 | 2.9900×10−1 | 2.2038×10−1 | 4.0000×10−1 | 3.9982×10−1 | 3.3429×10−1 |
0.6 | 3.1101×10−1 | 3.9999×10−1 | 2.1461×10−1 | 4.0044×10−1 | 3.9899×10−1 | 3.6584×10−1 |
0.7 | 3.0081×10−1 | 3.9900×10−1 | 2.0431×10−1 | 5.0000×10−1 | 4.1231×10−1 | 3.9192×10−1 |
0.8 | 2.5622×10−1 | 2.0077×10−1 | 1.8996×10−1 | 5.0012×10−1 | 4.8912×10−1 | 4.1267×10−1 |
0.9 | 2.0001×10−1 | 3.4491×10−1 | 1.7189×10−1 | 5.0099×10−1 | 4.9888×10−1 | 4.2818×10−1 |
1.0 | 2.6702×10−1 | 2.5556×10−1 | 1.5031×10−1 | 5.6540×10−1 | 5.0034×10−1 | 4.3856×10−1 |