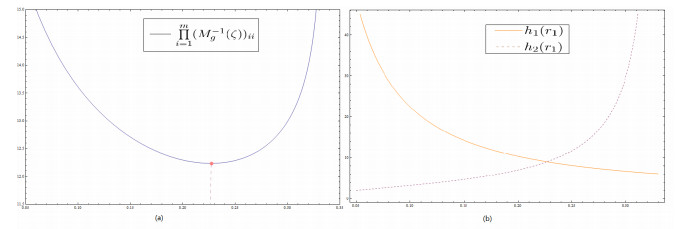
Considering a mixture model with qualitative factors, the R-optimal design problem is investigated when the levels of the qualitative factor interact with the mixture factors. In this paper, the conditions for R-optimality of designs with mixture and qualitative factors are presented. General analytical expressions are also derived for the decision function under the R-optimal designs, in order to verify that the resulting designs satisfy the general equivalence theorem. In addition, the relative efficiency of the R-optimal design is discussed.
Citation: Ling Ling, Guanghui Li, Xiaoyuan Zhu, Chongqi Zhang. R-optimal designs for second-order Scheffé model with qualitative factors[J]. AIMS Mathematics, 2022, 7(3): 4540-4551. doi: 10.3934/math.2022253
[1] | Weiping Zhou, Wan He, Wei Wang, Shigui Huang . Construction of marginally coupled designs with mixed-level qualitative factors. AIMS Mathematics, 2024, 9(12): 33731-33755. doi: 10.3934/math.20241610 |
[2] | Fei Yan, Junpeng Li, Haosheng Jiang, Chongqi Zhang . A-Optimal designs for mixture polynomial models with heteroscedastic errors. AIMS Mathematics, 2023, 8(11): 26745-26757. doi: 10.3934/math.20231369 |
[3] | Wei Li, Guangying Lv . A fully-decoupled energy stable scheme for the phase-field model of non-Newtonian two-phase flows. AIMS Mathematics, 2024, 9(7): 19385-19396. doi: 10.3934/math.2024944 |
[4] | Xiao Liang, Xinran Xie . Key generic technology research and development decision-making in Stackelberg competition. AIMS Mathematics, 2023, 8(12): 28833-28857. doi: 10.3934/math.20231478 |
[5] | Jian-Rong Wu, He Liu . The nearest point problems in fuzzy quasi-normed spaces. AIMS Mathematics, 2024, 9(3): 7610-7626. doi: 10.3934/math.2024369 |
[6] | Youming Guo, Tingting Li . Dynamics and optimal control of an online game addiction model with considering family education. AIMS Mathematics, 2022, 7(3): 3745-3770. doi: 10.3934/math.2022208 |
[7] | Najat Almutairi, Sayed Saber . Chaos control and numerical solution of time-varying fractional Newton-Leipnik system using fractional Atangana-Baleanu derivatives. AIMS Mathematics, 2023, 8(11): 25863-25887. doi: 10.3934/math.20231319 |
[8] | Haishu Lu, Xiaoqiu Liu, Rong Li . Upper semicontinuous selections for fuzzy mappings in noncompact WPH-spaces with applications. AIMS Mathematics, 2022, 7(8): 13994-14028. doi: 10.3934/math.2022773 |
[9] | Ziran Yin, Chongyang Liu, Xiaoyu Chen, Jihong Zhang, Jinlong Yuan . A comprehensive characterization of the robust isolated calmness of Ky Fan k-norm regularized convex matrix optimization problems. AIMS Mathematics, 2025, 10(3): 4955-4969. doi: 10.3934/math.2025227 |
[10] | Xiao Wu, Qi Wang, Yinying Kong . Two-person zero-sum stochastic games with varying discount factors. AIMS Mathematics, 2021, 6(10): 11516-11529. doi: 10.3934/math.2021668 |
Considering a mixture model with qualitative factors, the R-optimal design problem is investigated when the levels of the qualitative factor interact with the mixture factors. In this paper, the conditions for R-optimality of designs with mixture and qualitative factors are presented. General analytical expressions are also derived for the decision function under the R-optimal designs, in order to verify that the resulting designs satisfy the general equivalence theorem. In addition, the relative efficiency of the R-optimal design is discussed.
In recent years, the mixture models have been widely used in sciences, engineering and business applications. Cornell [1] applied models for data from mixture experiments to chick feeding, paper coatings, and many other products. Zijlstra et al. [2] embed mixture models in a panel mixed logit framework to describe preferences and preference heterogeneity for the mobility budget. Goos and Hamidouche [3] applied the mixture models to a real-life cocktail experiment. In mixture experiments, the factors under study are proportions of the ingredients of a mixture. As the application involves the choice of cocktails, the taste of cocktails only depends on the ingredient proportions, and not on the total amount of cocktail.
To obtain the optimal design under certain optimality criteria has always been a hot topic in the research of the mixture design. Available optimality criteria include D-, A-, R-, and I-optimality, etc. (see [4,5]). The D-optimality and A-optimality have been used most frequently. In practice, the efficiency of a specific optimality criterion depends on statistical problem sensitively, and in many cases, the D- and A-optimal designs are reasonable. However, there are also various situations where the application of the D- and A-optimality criteria is not appropriate. The efficiency loss may be considerable when the D-optimal design is used for the construction of rectangular confidence regions, as stated by Dette [6]. For this reason, Dette [6] proposed the R-optimality criterion, which minimizes the volume of the p-dimensional rectangular confidence region for the unknown parameters. Furthermore, the R-optimality criterion not only has an excellent statistical interpretation but also satisfies a beneficial invariance property. It allows an easy calculation of optimal designs on many linearly transformed design spaces. Hao et al. [7] investigated the R-optimal design for the second-order mixture model, while the qualitative factors are not considered.
When an experiment includes qualitative factors, the effects between the quantitative and qualitative factors should be taken into consideration. For example, Lee and Huang [8] demonstrated through an example on chemical study how the D-optimal design may help to design an experiment with both quantitative and qualitative factors more efficiently. Yue et al. [9] investigated the D-optimal designs for multiresponse polynomial regression models with both quantitative and qualitative factors. Kao and Hazar [10] investigated the optimal designs for mixed continuous and binary responses with quantitative and qualitative factors. Zhang et al. [11] proposed a new uniformity criterion for designs with both qualitative and quantitative factors. Although qualitative factors have received increasing attention in the literature, little is known concerning mixture designs with qualitative factors. Following the seminal work of Donev [12], the only published results concerning qualitative factors for mixture models are presented in [13], which generated the A-optimal designs for mixture central polynomial model with qualitative factors. This paper proposes a novel optimal design of mixture model with qualitative factors.
This paper aims at developing a R-optimal design for Scheffé models, when the response depends on the joint influence of both mixture and qualitative factors. The remainder of this paper is organized as follows. Section 2 and Section 3 briefly introduce the R-optimality, and some basic notations of the two kinds of mixture designs. The main results are presented in Section 4. Finally, the conclusion is drawn in Section 5.
A mixture experiment design usually assumes that the response variable y is only related to the proportion of each component x1,⋯,xq, but not to the total. The experimental region determined by the component proportions can be expressed as
X={x=(x1,x2,⋯,xq):q∑i=1xi=1,xi≥0,i=1,2,⋯,q,C′s}, |
in which C′s are some other constraint conditions. When the model does not contain such constraints, it is called a (q−1)-dimensional simplex, denoted as Sq−1. The response at x can be written as
y=βTf(x)+ε, | (2.1) |
where β=(β1,β2,⋯,βm)T is the m-vector of unknown parameters, f(x)=(f1(x),f2(x),⋯, fm(x))T is a given m-vector of regression functions of x=(x1,x2,⋯,xq)T∈X⊆Sq−1. The error ε is assumed to be independent of x and independent identically distributed with a normal distributed with mean 0 and constant variance σ2.
Based on the model (2.1), an approximate design can be expressed as
ξ=(x1x2⋯xkr1r2⋯rk), |
where xi∈X are support points and their weights ξ(xi)=ri satisfy ri>0 and k∑i=1ri=1,i=1,2,⋯,k. For a design ξ, the Fisher information matrix is given by
M(ξ)=∫Xf(x)fT(x)ξ(dx). |
Moreover, let Ξ denote the set of all designs with non-singular information matrix on X.
Definition 1. A design ξ∗∈Ξ is called R-optimal for the model (2.1) if it minimizes
ΦR(ξ)=m∏i=1(M−1(ξ))ii, | (2.2) |
where m is the number of unknown parameters, (M−1(ξ))ii is the element on the main diagonal of M−1(ξ).
For a given design, the equivalence theorem seeks to prove whether the design is the optimal design. Dette [6] investigated the equivalence theorem of R-optimal design.
Lemma 1. For any x∈X, the design ξ∗ is R-optimal if and only if
ψR(x,ξ)=m∑j=1(eTjM−1(ξ)f(x))2eTjM−1(ξ)ej−m≤0, | (2.3) |
where ej is the unit vector whose j-th component is equal to 1 and all others are 0. Moreover, the equality is attained at the support points of the R-optimaldesign ξ∗.
In the mixture experiments, a simplex-lattice design for q ingredients involves all possible mixture formulations, the set of q components n order lattice points can be expressed as
L{q,n}={(α1n,α2n,…,αqn):q∑i=1αi=n,αi∈Z+,i=1,2,…,q}, |
and there are (q+n−1n) points in L{q,n}.
The matrix H(x)={xij}N,qi,j=1 generated by all permutations of the coordinates of x=(x1,x2,⋯,xq)T∈X is called permutation matrix, where i1,i2,⋯,iq is a permutation of the 1,2,⋯,q. Then each row of H(x) is a mixture point and H(x)={x,x1,⋯,xN−1} is called permutation point set about x. The set of q components centroid points can be expressed as C{q}=q⋃i=1H(xi). A simplex-centroid design consists of 2q−1 points: q permutations of (1,0,⋯,0), (q2) permutations of (1/2,1/2,0,⋯,0), ⋯, and one permutation of (1/q,1/q,⋯,1/q). The corresponding model to be fitted to data at the points of the simplex-centroid design is
E(y)=q∑i=1βixi+q−1∑i=1q∑i<jβijxixj+⋯+β12⋯qx1x2⋯xq. |
The Scheffé central polynomial model can reduce the number of trials without reducing the order of the model and maintaining prediction accuracy.
In many practical problems, however, the response is influenced not only by the components' proportion in the mixture, but also by other variables. We can consider the general model which was introduced by Lee and Huang [8], this type of model can be expressed as follows:
E[y(j,x)]=fT1(x)βj+fT2(x)γ,x∈X⊆Sq−1,j=1,2,⋯,s. | (3.1) |
In the model, fT1(x)βj describes the part of the response that is influenced by a qualitative factor, which is generally referred to as the factorial effect, among them, fT1(x)=(f11(x),f12(x), ⋯,f1p1(x)), βj=(βj1,βj2,⋯,βjp1)T, s are the level of qualitative factor, y(j,x) is the value of the response variable at design point x=(x1,x2,⋯,xq)T∈X⊆Sq−1 at the jth level. fT2(x)γ is the part of the response that is not affected by the qualitative factors, generally referred to as fixed effect, where fT2(x)=(f21(x),f22(x),⋯,f2p2(x)), γ=(γ1,γ2,⋯,γp2)T.
Taking the second-order general Scheffé central polynomial model as an example, the model (3.1) can be expressed in the following three forms:
E[y(j,x)]=(fTL1(x),fTL2(x))βj,j=1,2,⋯,s; | (3.2) |
E[y(j,x)]=fTL1(x)β(L1)j+fTL2(x)γ(L2),j=1,2,⋯,s; | (3.3) |
E[y(j,x)]=fTL2(x)β(L2)j+fTL1(x)γ(L1),j=1,2,⋯,s. | (3.4) |
Where fL1(x)=(x1,x2,⋯,xq)T and fL2(x)=(x1x2,x1x3,⋯,xq−1xq)T. Among them, both the primary and interaction terms in model (3.2) are affected by qualitative factors; while these terms in model (3.3) are factorial effects and fixed effects, respectively, and the case is quite the contrary in model (3.4), with the two terms being fixed and factorial effects, respectively.
For example, a 21-herb formula, referred to as the modified Qing Fei Pai Du Tang (mQFPD) for treating COVID-19, is now being tested in a clinical trial sponsored by the University of California, San Diego. The results obtained by these experiments define the efficacy of the drugs. In this case, the difference between the efficacies of the drugs on the gender of the patient can be considered as the first part of models (3.3) and (3.4), while the fixed efficacy of the drugs can be considered as the second part of the two models. Afterwards, the R-optimal design for models (3.3) and (3.4), is presented.
Denote the index set of the qualitative factor as Ds={1,2,⋯,s}, and denote z=(j,x)∈Ω, Ω=Ds×X as the product set formed by the mixture experiment region and the qualitative factor. Then the design on the experimental region Ω can be expressed as a product of two partial designs, i.e., ζ(j,x)=η(j)×ξ(x), where η is the design on Ds and ξ(x) is the design on X. Then ζ has the following form
ζ=((1;x11,⋯,x1n1)(2;x21,⋯,x2n2)⋯(s;xs1,⋯,xsns)(η(1);r11,⋯,r1n1)(η(2);r21,⋯,r2n2)⋯(η(s);rs1,⋯,rsns)). |
First note that the information matrix of the design ξ is
Mf(ξ)=[M11(ξ)M12(ξ)M21(ξ)M22(ξ)], |
which is associated with the quantitative model E[y(x)]=[fT1(x),fT2(x)](βT,γT)T. Let the general model (3.1) be rewritten as
E[y(j,x)]=[eTj⊗fT1(x),fT2(x)](βT1,βT2,⋯,βTs,γT)T=gT(j,x)θ. | (4.1) |
By a result in Lee and Huang [8], the information matrix of the design ζ is
Mg(ζ)=[D⊗M11(ξ)η⊗M12(ξ)ηT⊗M21(ξ)M22(ξ)], |
where D=diag{η(1),η(2),⋯,η(s)},η=[η(1),η(2),⋯,η(s)]T.
According to the R-optimality criterion, the minimization of m∏i=1(M−1g(ζ))ii amounts to minimization of tr[log[m∏i=1(M−1g(ζ))ii]] for all ζ∈Ξ. The following theorem defines the conditions for R-optimality of designs and the proof is given in the Appendix.
Theorem 1. For a design ζ(j,x)=η(j)×ξ(x), where η is the design on Ds={1,2,⋯,s} and ξ is the design on X⊆Sq−1, the following equation holds for model (3.1):
tr[log[m∏i=1(M−1g(ζ))ii]]=s∑j=1tr(log(1η(j)M−111(ξ)+K(1)))+tr{log[D22(ξ)]}, |
where
K(1)=M−111(ξ)M12(ξ)D22(ξ)M21(ξ)M−111(ξ),D22(ξ)=[M22(ξ)−M21(ξ)M−111(ξ)M12(ξ)]−1. |
Moreover, it also follows that, for ζ to be optimal, all the elements of η must be equal, i.e. η(1)=η(2)=⋯=η(s)=1/s.
If the optimal marginal design η is the uniform design on Ds={1,2,⋯,s}, then we have
tr[log[m∏i=1(M−1g(ζ))ii]]=s⋅tr(log(s⋅M−111(ξ)+K(1)))+tr{log[D22(ξ)]}. |
To verify the R-optimality of the designs by the equivalence theorem, the decision function presented in Eq (2.3) can be simplified to
ψR(x,ξ)=fT(x)M−1(ξ)BM−1(ξ)f(x)−m≤0, |
where B=diag(1/M11,1/M22,…,1/Mmm), Mii=(M−1(ξ))ii.
Theorem 2. For a design ζ on Ω, let bii, djj and ckk are the element on the main diagonal of M−111(ξ), D22(ξ) and K(1), respectively, if η is a uniform design on Ds, the decision function of the model (4.1) under the R-optimal design is
ψR−g(j,x;ζ)=gT(j,x)M−1g(ζ)BgM−1g(ζ)g(j,x)=s2u(x;ξ)+s⋅4∑i=1vi(x;ξ)+4∑i=1ωi(x;ξ), |
whereBg=[Is⊗B(s,1)00H],B(u,v)=diag(1/(ub11+vc11),1/(ub22+vc22),⋯, 1/(ubp1p1+vcp1p1)),H=diag(1/d11,1/d22,⋯,1/dp2p2).
In the following, the approximate R-optimal design on the model (3.3) and (3.4) are derived from the results of theorem 2. Suppose ζ∗ is a R-optimal design on the region Ds×Sq−1 and denoted by η∗×ξ∗, where η∗ is the uniform design on Ds. For the model (3.3), the design ξ over the mixture region is arranged by using the set of second-order generalized simplex-centroid points C{q,2}, denoted as
ξ=(H(x1)H(x2)r1r2), |
where x1=(1,0,⋯,0)T and x2=(1/2,1/2,⋯,0)T are the vertices and mid-points of the edges of the simplex, respectively. H(xi)={xi1,xi2,⋯,xini} is permutation point set about xi,i=1,2, and the weights of corresponding point satisfy n1r1+n2r2=1. Then the function ψR−g(j,x;ζ) can be expressed as
ψR−g(j,x;ζ)=(a0+a1b0+(q−1)r22r1(2r1+r2))q∑i=1x2i+(a2b0+r2r1(2r1+r2))∑i<jxixj−(a3b1+2qr22+8r1r2)∑i≠jx2ixj−12r2r1(2r1+r2)∑i<j<kxixjxk+32r21+32r1r2+4qr22r1r2(2r1+r2)∑i<jx2ix2j+32r1+(4q+8)r2r1(2r1+r2)∑i≠j≠kx2ixjxk, |
where
a0=16s2r41+8(2q−3)s2r31r2+(4q2−5q+8)s2r21r22,a1=(q−1)s[8r31r2+(9q−16)r21r22+(q−2)(3q−4)r1r32+(q−2)2(q−1)4r42],a2=s(1−s)[16r31r2+(6q−8)r21r22],a3=8sr21+(6q−8)sr1r2+(q−1)(q−2)sr22,b0=b1[4r21+(3q−4)r1r2+(q−1)(q−2)2r22],b1=4sr31+(2sq+q−3s−1)r21r2+(q−1)(q−2)2r1r22. |
Let q=3, s=2, ΦR(ζ,r1)=m∏i=1(M−1g(ζ))ii, hi(r1)=ψR−g(j,x;ζ),x∈H(xi),i=1,2 is adopted to denote the values of the decision function at the two types of design points, respectively. If ζ∗ is a R-optimal design, on the one hand there should be
r∗1=argminr1∈(0,1/n1)m∏i=1(M−1g(ζ))ii, |
on the other hand
hi(r∗1)=ψR−g(j,x;ζ∗)=sn1+n2,x∈H(xi),i=1,2. |
As r1 transforms, ΦR(ζ,r1) changes as shown in Figure 1(a), we can find the optimal design
ξ∗=(H(x1)H(x2)0.22690.1065). |
Figure 1(b) shows the changes of h1(r1) and h2(r1), which can be seen when r1=r∗1, h1(r1) and h2(r1) intersect at a point, whose coordinates are (r∗1,sn1+n2)=(0.2269,9). The contour map of the function ψR−g(j,x;ζ∗) on the region Ω is shown in Figure 2. It is obvious to see that the maximum of the decision function is no greater than the number of model parameters and the maximum is attained at the support points of ξ∗. According to the Eq (2.3), the design ξ∗ is R-optimal for model (3.3) and satisfies the equivalence theorem.
Table 1 lists the weights of R-optimal designs for model (3.3) and model (3.4) with 3≤q≤6 and 2≤s≤6. The corresponding values of the common logarithm of decision function, lg[ΦR(M(ζ))], are also listed in the fifth and eighth columns of the table. In model (3.3), for any q, the weights of vertices increase with the total number of level combinations. On the contrary, in model (3.4), the weights of vertices decrease with the increase of the total number of level combinations.
Model(3.3) | Model(3.4) | ||||||
q | s | r1(x1) | r2(x2) | lg[ΦR(M(ζ))] | r1(x1) | r2(x2) | lg[ΦR(M(ζ))] |
3 | 2 | 0.2269 | 0.1065 | 12.2362 | 0.1589 | 0.1744 | 16.6097 |
3 | 3 | 0.2412 | 0.0921 | 16.3870 | 0.1333 | 0.2000 | 24.9193 |
3 | 4 | 0.2521 | 0.0812 | 20.9449 | 0.1168 | 0.2165 | 33.5317 |
3 | 5 | 0.2607 | 0.0726 | 25.8066 | 0.1051 | 0.2282 | 42.3861 |
3 | 6 | 0.2677 | 0.0656 | 30.9111 | 0.0963 | 0.2371 | 51.4420 |
4 | 2 | 0.1486 | 0.0676 | 23.3663 | 0.0868 | 0.1088 | 33.1658 |
4 | 3 | 0.1595 | 0.0603 | 29.4999 | 0.0625 | 0.1250 | 48.7669 |
4 | 4 | 0.1684 | 0.0544 | 36.1694 | 0.0560 | 0.1293 | 61.7016 |
4 | 5 | 0.1758 | 0.0495 | 43.2376 | 0.0513 | 0.1325 | 74.8992 |
4 | 6 | 0.1820 | 0.0453 | 50.6236 | 0.0477 | 0.1349 | 88.3275 |
5 | 2 | 0.1064 | 0.0468 | 38.2574 | 0.0522 | 0.0739 | 55.3620 |
5 | 3 | 0.1146 | 0.0427 | 46.5072 | 0.0448 | 0.0776 | 72.5382 |
5 | 4 | 0.1216 | 0.0392 | 55.4224 | 0.0400 | 0.0800 | 90.0000 |
5 | 5 | 0.1277 | 0.0362 | 64.8325 | 0.0365 | 0.0817 | 107.7430 |
5 | 6 | 0.1330 | 0.0335 | 74.6359 | 0.0339 | 0.0830 | 125.7450 |
6 | 2 | 0.0807 | 0.0344 | 57.0488 | 0.0400 | 0.0506 | 78.8374 |
6 | 3 | 0.0871 | 0.0318 | 67.5197 | 0.0342 | 0.0530 | 100.8750 |
6 | 4 | 0.0926 | 0.0296 | 78.7860 | 0.0305 | 0.0545 | 123.1450 |
6 | 5 | 0.0975 | 0.0276 | 90.6445 | 0.0278 | 0.0556 | 145.6890 |
6 | 6 | 0.1019 | 0.0258 | 102.973 | 0.0257 | 0.0564 | 168.5050 |
For any design ζ of the mixture model with qualitative factors, we measure its quality by efficiency, and the efficiency under A-optimality criterion can be expressed as:
Aeff(ζ)=trM−1g(ζA)trM−1g(ζ). |
The relative A-efficiencies of different R-optimal designs in model (3.3) are displayed in Table 2. In model (3.3), for any q, whereas the efficiencies decrease with the increase of the total number of qualitative levels, the R-optimal designs in most cases have relatively high efficiencies. It should be noticed that the R-optimal designs for q=6 and s=2 have an A-efficiency of 98.89 % relative to the A-optimal designs. In other words, the R-optimal designs in model (3.3) perform excellently in terms of the A-optimality criterion.
q | s | Aeff(ζ∗R) | q | s | Aeff(ζ∗R) |
3 | 2 | 0.9666 | 5 | 2 | 0.9852 |
3 | 3 | 0.9616 | 5 | 3 | 0.9807 |
3 | 4 | 0.9601 | 5 | 4 | 0.9773 |
3 | 5 | 0.9607 | 5 | 5 | 0.9749 |
3 | 6 | 0.9623 | 5 | 6 | 0.9733 |
4 | 2 | 0.9788 | 6 | 2 | 0.9889 |
4 | 3 | 0.9735 | 6 | 3 | 0.9852 |
4 | 4 | 0.9702 | 6 | 4 | 0.9821 |
4 | 5 | 0.9685 | 6 | 5 | 0.9797 |
4 | 6 | 0.9679 | 6 | 6 | 0.9778 |
This paper tackles the R-optimal design under the interaction of qualitative factors and mixture components. It considers the continuous R-optimal designs for the second-order Scheffé model with qualitative factors. The obtained results show that the optimal marginal design η is the uniform design under R-optimality criterion. For model (3.3), general analytical expressions for the decision function under the R-optimal designs are derived, and the R-optimality is confirmed by the equivalence theorem. Finally, we remark further that the performance of R-optimal designs in terms of the A-optimality criterion is excellent.
This work was supported by the National Natural Science Foundation of China (Grant No. 12071096, 11901260), Science and Technology Fund for basic research of Guizhou Province ([2020]1Y010).
The authors declare that there is no conflict of interest.
The inverse matrices of Mf(ξ) is
M−1f(ξ)=[M−111(ξ)+K(1)−M−111(ξ)M12(ξ)D22(ξ)−D22(ξ)M21(ξ)M−111(ξ)D22(ξ)], |
we have
M−1g(ζ)=[F11(ζ)F12(ζ)F21(ζ)F22(ζ)], |
where ζ=η×ξ,
F11(ζ)=(D⊗M11(ξ))−1+(D⊗M11(ξ))−1η⊗M12(ξ)[M22(ξ)−ηT⊗M21(ξ)(D⊗M11(ξ))−1η⊗M12(ξ)]−1ηT⊗M21(ξ)[D−1⊗M−111(ξ)]−1=(D−1⊗M−111(ξ))+(D−1⊗M−111(ξ))η⊗M12(ξ)[M22(ξ)−ηTD−1η⊗M21(ξ)M−111(ξ)M12(ξ)]−1ηTD−1⊗M21(ξ)M−111(ξ)=D−1⊗M−111(ξ)+(D−1η⊗M−111(ξ)M12(ξ))D22ηTD−1⊗M21(ξ)M−111(ξ)=D−1⊗M−111(ξ)+D−1ηηTD−1⊗M−111(ξ)M12(ξ)M21(ξ)M−111(ξ)=D−1⊗M−111(ξ)+Js⊗K(1),F12(ζ)=−(D⊗M11(ξ))−1(η⊗M12(ξ))[M22(ξ)−ηT⊗M21(ξ)(D⊗M11(ξ))−1η⊗M12(ξ)]−1=−D−1⊗M−111(ξ)η⊗M12(ξ)D22(ξ)=−D−1η⊗M−111(ξ)M12(ξ)D22(ξ)=−1s⊗M−111(ξ)M12(ξ)D22(ξ)=FT21(ζ),F22(ζ)=[M22(ξ)−M21(ξ)M−111(ξ)M12(ξ)]−1=D22(ξ), |
and 1s is a s×1 vector of all ones, js is the s×s matrix with all elements equal to unity.
As a result, we have
tr[log[m∏i=1(M−1g(ζ))ii]]=tr{log[D−1⊗M−111(ξ)+Js⊗K(1)]}+tr{log[D22(ξ)]}=s∑j=1tr(log(1η(j)M−111(ξ)+K(1)))+tr{log[D22(ξ)]}≥s∏j=1tr(log(1η(j)M−111(ξ)+K(1)))+tr{log[D22(ξ)]}, |
the equality is attained if and only if the elements of η is equal, i.e. η(1)=η(2)=⋯=η(s)=1/s.
ψR−g(j,x;ζ)=gT(j,x)M−1g(ζ)BgM−1g(ζ)g(j,x)=s2u(x;ξ)+s⋅4∑i=1vi(x;ξ)+4∑i=1ωi(x;ξ) |
where
u(x;ξ)=fT1(x)M−111(ξ)B(s,1)M−111(ξ)f1(x),v1(x;ξ)=2fT1(x)M−111(ξ)B(s,1)K(1)f1(x),v2(x;ξ)=fT1(x)K(1)B(s,1)K(1)f1(x),v3(x;ξ)=−fT1(x)M−111(ξ)B(s,1)M−111(ξ)M12(ξ)D22(ξ)f2(x),v4(x;ξ)=−fT1(x)K(1)B(s,1)M−111(ξ)M12(ξ)D22(ξ)f2(x),ω1(x;ξ)=fT1(x)M−111(ξ)M12(ξ)D22(ξ)HD22(ξ)M21(ξ)M−111(ξ)f1(x),ω2(x;ξ)=−fT1(x)M−111(ξ)M12(ξ)D22(ξ)HD22(ξ)f2(x),ω3(x;ξ)=−fT2(x)D22(ξ)HD22(ξ)M21(ξ)M−111(ξ)f1(x),ω4(x;ξ)=fT2(x)D22(ξ)HD22(ξ)f2(x). |
[1] | J. A. Cornell, Experiments with mixtures: designs, models, and the analysis of mixture data, New York: John Wiley & Sons, 2002. |
[2] |
T. Zijlstra, P. Goos, A. Verhetsel, A mixture-amount stated preference study on the mobility budget, Transport. Res. A-Pol., 126 (2019), 230–246. https://doi.org/10.1016/j.tra.2019.06.009 doi: 10.1016/j.tra.2019.06.009
![]() |
[3] |
P. Goos, H. Hamidouche, Choice models with mixtures: An application to a cocktail experiment, Food Qual. Prefer., 77 (2019), 135–146. https://doi.org/10.1016/j.foodqual.2019.04.006 doi: 10.1016/j.foodqual.2019.04.006
![]() |
[4] |
P. Goos, B. Jones, U. Syafitri, Ⅰ-optimal design of mixture experiments, J. Am. Stat. Assoc., 111 (2016), 899–911. https://doi.org/10.1080/01621459.2015.1136632 doi: 10.1080/01621459.2015.1136632
![]() |
[5] |
X. F. Zhang, Z. B. Zhu, C. Q. Zhang, Multi-stage differential evolution algorithm for constrained D-optimal design, AIMS Mathematics, 6 (2021), 2956–2969. https://doi.org/10.3934/math.2021179 doi: 10.3934/math.2021179
![]() |
[6] |
H. Dette, Designing experiments with respect to standardized optimality criteria, J. R. Stat. Soc. B, 59 (1997), 97–110. https://doi.org/10.1111/1467-9868.00056 doi: 10.1111/1467-9868.00056
![]() |
[7] |
H. H. Hao, X. Y. Zhu, X. F. Zhang, C. Q. Zhang, R-optimal design of the second-order scheffé mixture model, Stat. Probabil. Lett., 173 (2021), 109069. https://doi.org/10.1016/j.spl.2021.109069 doi: 10.1016/j.spl.2021.109069
![]() |
[8] |
C. P. Lee, M. N. L. Huang, D-optimal designs for second-order response surface models with qualitative factors, J. Data Sci., 9 (2011), 139–153. https://doi.org/10.6339/JDS.201104_09(2).0001 doi: 10.6339/JDS.201104_09(2).0001
![]() |
[9] |
R. X. Yue, X. Liu, K. Chatterjee, D-optimal designs for multiresponse linear models with a qualitative factor, J. Multivariate Anal., 124 (2014), 57–69. https://doi.org/10.1016/j.jmva.2013.10.011 doi: 10.1016/j.jmva.2013.10.011
![]() |
[10] |
M. H. Kao, K. Hazar, Optimal designs for mixed continuous and binary responses with quantitative and qualitative factors, J. Multivariate Anal., 182 (2021), 104712. https://doi.org/10.1016/j.jmva.2020.104712 doi: 10.1016/j.jmva.2020.104712
![]() |
[11] |
M. Zhang, F. Yang, Y. D. Zhou, Uniformity criterion for designs with both qualitative and quantitative factors, Statistics, 55 (2021), 90–109. https://doi.org/10.1080/02331888.2021.1873993 doi: 10.1080/02331888.2021.1873993
![]() |
[12] |
A. N. Donev, Design of experiments with both mixture and qualitative factors, J. R. Stat. Soc. B, 51 (1989), 297–302. https://doi.org/10.1111/j.2517-6161.1989.tb01767.x doi: 10.1111/j.2517-6161.1989.tb01767.x
![]() |
[13] |
Z. B. Zhu, G. H. Li, C. Q. Zhang, A-optimal designs for mixture central polynomial model with qualitative factors, Commun. Stat. Theor. M., 48 (2019), 2345–2355. https://doi.org/10.1080/03610926.2018.1472783 doi: 10.1080/03610926.2018.1472783
![]() |
1. | Mudthir Bakri, Ibrahim Aldhari, Mohammed Saleh Alfawzan, Qian Chen, Prediction of California Bearing Ratio of Granular Soil by Multivariate Regression and Gene Expression Programming, 2022, 2022, 1687-8094, 1, 10.1155/2022/7426962 | |
2. | Bushra Husain, Fariha Aslam, Weighted Simplex Centroid Mixture Experiments for third order Becker’s models: The R-optimal approach, 2024, 213, 01677152, 110189, 10.1016/j.spl.2024.110189 | |
3. | Fei Yan, Junpeng Li, Haosheng Jiang, Chongqi Zhang, A-Optimal designs for mixture polynomial models with heteroscedastic errors, 2023, 8, 2473-6988, 26745, 10.3934/math.20231369 |
Model(3.3) | Model(3.4) | ||||||
q | s | r1(x1) | r2(x2) | lg[ΦR(M(ζ))] | r1(x1) | r2(x2) | lg[ΦR(M(ζ))] |
3 | 2 | 0.2269 | 0.1065 | 12.2362 | 0.1589 | 0.1744 | 16.6097 |
3 | 3 | 0.2412 | 0.0921 | 16.3870 | 0.1333 | 0.2000 | 24.9193 |
3 | 4 | 0.2521 | 0.0812 | 20.9449 | 0.1168 | 0.2165 | 33.5317 |
3 | 5 | 0.2607 | 0.0726 | 25.8066 | 0.1051 | 0.2282 | 42.3861 |
3 | 6 | 0.2677 | 0.0656 | 30.9111 | 0.0963 | 0.2371 | 51.4420 |
4 | 2 | 0.1486 | 0.0676 | 23.3663 | 0.0868 | 0.1088 | 33.1658 |
4 | 3 | 0.1595 | 0.0603 | 29.4999 | 0.0625 | 0.1250 | 48.7669 |
4 | 4 | 0.1684 | 0.0544 | 36.1694 | 0.0560 | 0.1293 | 61.7016 |
4 | 5 | 0.1758 | 0.0495 | 43.2376 | 0.0513 | 0.1325 | 74.8992 |
4 | 6 | 0.1820 | 0.0453 | 50.6236 | 0.0477 | 0.1349 | 88.3275 |
5 | 2 | 0.1064 | 0.0468 | 38.2574 | 0.0522 | 0.0739 | 55.3620 |
5 | 3 | 0.1146 | 0.0427 | 46.5072 | 0.0448 | 0.0776 | 72.5382 |
5 | 4 | 0.1216 | 0.0392 | 55.4224 | 0.0400 | 0.0800 | 90.0000 |
5 | 5 | 0.1277 | 0.0362 | 64.8325 | 0.0365 | 0.0817 | 107.7430 |
5 | 6 | 0.1330 | 0.0335 | 74.6359 | 0.0339 | 0.0830 | 125.7450 |
6 | 2 | 0.0807 | 0.0344 | 57.0488 | 0.0400 | 0.0506 | 78.8374 |
6 | 3 | 0.0871 | 0.0318 | 67.5197 | 0.0342 | 0.0530 | 100.8750 |
6 | 4 | 0.0926 | 0.0296 | 78.7860 | 0.0305 | 0.0545 | 123.1450 |
6 | 5 | 0.0975 | 0.0276 | 90.6445 | 0.0278 | 0.0556 | 145.6890 |
6 | 6 | 0.1019 | 0.0258 | 102.973 | 0.0257 | 0.0564 | 168.5050 |
q | s | Aeff(ζ∗R) | q | s | Aeff(ζ∗R) |
3 | 2 | 0.9666 | 5 | 2 | 0.9852 |
3 | 3 | 0.9616 | 5 | 3 | 0.9807 |
3 | 4 | 0.9601 | 5 | 4 | 0.9773 |
3 | 5 | 0.9607 | 5 | 5 | 0.9749 |
3 | 6 | 0.9623 | 5 | 6 | 0.9733 |
4 | 2 | 0.9788 | 6 | 2 | 0.9889 |
4 | 3 | 0.9735 | 6 | 3 | 0.9852 |
4 | 4 | 0.9702 | 6 | 4 | 0.9821 |
4 | 5 | 0.9685 | 6 | 5 | 0.9797 |
4 | 6 | 0.9679 | 6 | 6 | 0.9778 |
Model(3.3) | Model(3.4) | ||||||
q | s | r1(x1) | r2(x2) | lg[ΦR(M(ζ))] | r1(x1) | r2(x2) | lg[ΦR(M(ζ))] |
3 | 2 | 0.2269 | 0.1065 | 12.2362 | 0.1589 | 0.1744 | 16.6097 |
3 | 3 | 0.2412 | 0.0921 | 16.3870 | 0.1333 | 0.2000 | 24.9193 |
3 | 4 | 0.2521 | 0.0812 | 20.9449 | 0.1168 | 0.2165 | 33.5317 |
3 | 5 | 0.2607 | 0.0726 | 25.8066 | 0.1051 | 0.2282 | 42.3861 |
3 | 6 | 0.2677 | 0.0656 | 30.9111 | 0.0963 | 0.2371 | 51.4420 |
4 | 2 | 0.1486 | 0.0676 | 23.3663 | 0.0868 | 0.1088 | 33.1658 |
4 | 3 | 0.1595 | 0.0603 | 29.4999 | 0.0625 | 0.1250 | 48.7669 |
4 | 4 | 0.1684 | 0.0544 | 36.1694 | 0.0560 | 0.1293 | 61.7016 |
4 | 5 | 0.1758 | 0.0495 | 43.2376 | 0.0513 | 0.1325 | 74.8992 |
4 | 6 | 0.1820 | 0.0453 | 50.6236 | 0.0477 | 0.1349 | 88.3275 |
5 | 2 | 0.1064 | 0.0468 | 38.2574 | 0.0522 | 0.0739 | 55.3620 |
5 | 3 | 0.1146 | 0.0427 | 46.5072 | 0.0448 | 0.0776 | 72.5382 |
5 | 4 | 0.1216 | 0.0392 | 55.4224 | 0.0400 | 0.0800 | 90.0000 |
5 | 5 | 0.1277 | 0.0362 | 64.8325 | 0.0365 | 0.0817 | 107.7430 |
5 | 6 | 0.1330 | 0.0335 | 74.6359 | 0.0339 | 0.0830 | 125.7450 |
6 | 2 | 0.0807 | 0.0344 | 57.0488 | 0.0400 | 0.0506 | 78.8374 |
6 | 3 | 0.0871 | 0.0318 | 67.5197 | 0.0342 | 0.0530 | 100.8750 |
6 | 4 | 0.0926 | 0.0296 | 78.7860 | 0.0305 | 0.0545 | 123.1450 |
6 | 5 | 0.0975 | 0.0276 | 90.6445 | 0.0278 | 0.0556 | 145.6890 |
6 | 6 | 0.1019 | 0.0258 | 102.973 | 0.0257 | 0.0564 | 168.5050 |
q | s | Aeff(ζ∗R) | q | s | Aeff(ζ∗R) |
3 | 2 | 0.9666 | 5 | 2 | 0.9852 |
3 | 3 | 0.9616 | 5 | 3 | 0.9807 |
3 | 4 | 0.9601 | 5 | 4 | 0.9773 |
3 | 5 | 0.9607 | 5 | 5 | 0.9749 |
3 | 6 | 0.9623 | 5 | 6 | 0.9733 |
4 | 2 | 0.9788 | 6 | 2 | 0.9889 |
4 | 3 | 0.9735 | 6 | 3 | 0.9852 |
4 | 4 | 0.9702 | 6 | 4 | 0.9821 |
4 | 5 | 0.9685 | 6 | 5 | 0.9797 |
4 | 6 | 0.9679 | 6 | 6 | 0.9778 |