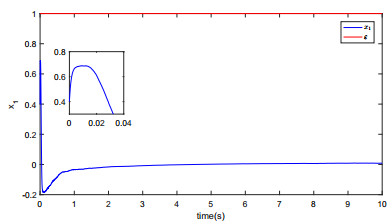
This paper investigates the finite-time control problem of p-norm stochastic nonlinear systems subject to output constraint. Combining a tan-type barrier Lyapunov function (BLF) with the adding a power integrator technique, a fuzzy state-feedback controller is constructed. Then, an output-feedback controller design scheme is developed by the constructed state-feedback controller and a reduce-order observer. Finally, both the rigorous analysis and the simulation results demonstrate that the designed output-feedback controller not only guarantees that the output constraint is not violated, but also ensures that the system is semi-global finite-time stable in probability (SGFSP).
Citation: Liandi Fang, Li Ma, Shihong Ding. Finite-time fuzzy output-feedback control for p-norm stochastic nonlinear systems with output constraints[J]. AIMS Mathematics, 2021, 6(3): 2244-2267. doi: 10.3934/math.2021136
[1] | Lu Zhi, Jinxia Wu . Adaptive constraint control for nonlinear multi-agent systems with undirected graphs. AIMS Mathematics, 2021, 6(11): 12051-12064. doi: 10.3934/math.2021698 |
[2] | Jingjing Yang, Jianqiu Lu . Stabilization in distribution of hybrid stochastic differential delay equations with Lévy noise by discrete-time state feedback controls. AIMS Mathematics, 2025, 10(2): 3457-3483. doi: 10.3934/math.2025160 |
[3] | Wei Zhao, Lei Liu, Yan-Jun Liu . Adaptive neural network control for nonlinear state constrained systems with unknown dead-zones input. AIMS Mathematics, 2020, 5(5): 4065-4084. doi: 10.3934/math.2020261 |
[4] | Changgui Wu, Liang Zhao . Finite-time adaptive dynamic surface control for output feedback nonlinear systems with unmodeled dynamics and quantized input delays. AIMS Mathematics, 2024, 9(11): 31553-31580. doi: 10.3934/math.20241518 |
[5] | Zhengqi Zhang, Huaiqin Wu . Cluster synchronization in finite/fixed time for semi-Markovian switching T-S fuzzy complex dynamical networks with discontinuous dynamic nodes. AIMS Mathematics, 2022, 7(7): 11942-11971. doi: 10.3934/math.2022666 |
[6] | Chuang Liu, Jinxia Wu, Weidong Yang . Robust $ {H}_{\infty} $ output feedback finite-time control for interval type-2 fuzzy systems with actuator saturation. AIMS Mathematics, 2022, 7(3): 4614-4635. doi: 10.3934/math.2022257 |
[7] | Yebin Li, Dongshu Wang, Zuowei Cai . On asymptotic fixed-time controller design for uncertain nonlinear systems with pure state constraints. AIMS Mathematics, 2023, 8(11): 27151-27174. doi: 10.3934/math.20231389 |
[8] | Bin Hang, Weiwei Deng . Finite-time adaptive prescribed performance DSC for pure feedback nonlinear systems with input quantization and unmodeled dynamics. AIMS Mathematics, 2024, 9(3): 6803-6831. doi: 10.3934/math.2024332 |
[9] | Tan Zhang, Pianpian Yan . Asymmetric integral barrier function-based tracking control of constrained robots. AIMS Mathematics, 2024, 9(1): 319-339. doi: 10.3934/math.2024019 |
[10] | Wajdi Kallel, Noura Allugmani . Finite-time stabilization of stochastic systems with varying parameters. AIMS Mathematics, 2023, 8(8): 17687-17701. doi: 10.3934/math.2023903 |
This paper investigates the finite-time control problem of p-norm stochastic nonlinear systems subject to output constraint. Combining a tan-type barrier Lyapunov function (BLF) with the adding a power integrator technique, a fuzzy state-feedback controller is constructed. Then, an output-feedback controller design scheme is developed by the constructed state-feedback controller and a reduce-order observer. Finally, both the rigorous analysis and the simulation results demonstrate that the designed output-feedback controller not only guarantees that the output constraint is not violated, but also ensures that the system is semi-global finite-time stable in probability (SGFSP).
Over the past decades, a variety of control design strategies have been proposed for different nonlinear systems [1,2,3,4,5,6,7]. Especially, many approximated-based control schemes have been developed for uncertain nonlinear systems by using neural networks (NNs) or the fuzzy logic systems (FLSs) [8,9,10,11,12,13,14,15,16,17,18,19]. Among these studies, the research of stochastic systems is much more attracted (see, e.g. [16,17,18,19] and the references therein), due to their wide application. It is worth noting that the aforementioned NNs-based or FLSs-based control strategies haven't taken output constraint into account. In fact, many practical systems are usually required to satisfy an output constraint in the operation for considering the performance specifications or safety [20,21]. It is well known that, the BLF-based approaches are useful tools to settle controller design problems of output-constrained nonlinear systems, see references [22,23,24,25,26,27] for instances. In the latest research progress of constrained control, many kinds of adaptive neural or fuzzy control design methods have been presented by combining the different BLFs with NNs or FLSs approximators for various stochastic nonlinear systems subject to output constraint and unknown nonlinearities in [28,29,30,31,32]. Nevertheless, the above-mentioned works have mainly considered strict-feedback stochastic systems whose fractional powers are all equal to one, rather than p-norm stochastic nonlinear systems in which the fractional powers are the positive odd rational numbers and at least one of the fractional powers is greater than one.
As an important class of nonlinear systems, the p-norm stochastic nonlinear systems which are more general and complex, have received increased attention in recent years. For such a kind of systems, many control design problems have been well solved by utilizing the adding a power integrator technique under some strong or weaker growth conditions [33,34,35,36,37]. Subsequently, references [38,39,40] have taken these growth conditions away and considered the adaptive NNs control for switched and large-scale stochastic high-order nonlinear systems. Meanwhile, the control problems have been investigated for p-norm nonlinear systems with output/states constraints under some nonlinear growth conditions in a few literatures, such as [41,42,43,44]. In these works, different state-feedback controllers have been mainly constructed and finite-time stability has been obtained. Worth noting that an output-feedback controller has been designed for high-order planar deterministic nonlinear systems in [44]. On these basics, references [45,46,47,48] have further taken both unknown nonlinearities and constraints into accounts, and designed some fuzzy controllers for p-norm stochastic nonlinear systems with output/states constraints. However, it should be pointed out that the above-mentioned results of p-norm stochastic nonlinear systems have mainly focused on addressing the asymptotical convergence rather than the finite-time convergence in the case of unknown nonlinearities. As a matter of fact, the finite-time convergence has only been investigated for p-norm stochastic nonlinear systems with known nonlinearities satisfying some nonlinear growth conditions. On the other hand, it can be observed that the existing constrained controllers of p-norm stochastic nonlinear systems are mainly based on the assumption that full-state measurements are available. In other words, when only the system output can be accurately measured, the problem of finite-time output-feedback control for p-norm stochastic nonlinear systems with unknown nonlinearities and output constraints, to our best knowledge, has never been considered in the literature.
Motivated by above discussions, this paper will investigate how to design the finite-time output-feedback controller for a kind of p-norm stochastic nonlinear systems subject to output constraint. The main contributions can be summarized as follows: 1) It is first time to consider p-norm stochastic nonlinear systems with output constraints and unmeasurable states. Note that the existing studies have mainly addressed the constrained control problems under the the assumption that all the states are measurable (e.g., [33,34,35,36,37,41,43]), while this paper investigates the constrained control design in the case of that the states are all unmeasurable except the system output. What's more, the system nonlinearities are completely unknown. Thus, this work will extend and develop the existing control design theory for p-norm stochastic nonlinear systems; 2) A finite-time controller is designed. The developed scheme not only ensures the system output is constrained in a given compact set, but also enables the closed-loop system is semi-global finite-time stable in probability (SGFSP).
In this paper, we consider the following class of p-norm stochastic nonlinear systems
dxi=xpi+1dt+ϕi(ˉxi)dt+gTi(ˉxi)dω,i=1,⋯,n−1,dxn=updt+ϕn(x)dt+gTn(x)dω,y=x1, | (2.1) |
where ω is a r-dimension standard Wiener process; x=(x1,⋯,xn)T∈Rn is system state vector; u∈Randy∈R are respectively control input and output; the fractional power p∈R≥1odd:={m/k|m≥k,mandkarepositiveoddintegers}; for i=1,⋯,n, ˉxi=(x1,⋯,xi)T∈Ri; ϕi:Ri→R and gi:Ri→Rr are unknown continuous functions satisfying ϕi(0)=0,gi(0)=0. The system output y=x1 is measurable and constrained in Π1={y(t)∈R,|y(t)|<ε} with a constant ε>0, while the other states x2,⋯,xn are all unmeasurable.
The objective of this paper is to design a finite-time fuzzy output-feedback controller for system (2.1) such that: 1) the output don't violate the given constrained boundary; 2) all the signals of the closed-loop system converge to a small compact of the original point in finite-time in probability in presence of unknown nonlinearities and unmeasured states xi(i=2,⋯,n).
Firstly, some concepts and lemmas are presented for preliminaries. Consider the following stochastic system
dx=ϕ(x)dt+g(x)dω, | (2.2) |
where ϕ(x) and g(x) are continuous functions with satisfying ϕ(0)=g(0)=0.
Definition 1. [1] For any given V(x)∈C2(Rn), associated with system (2.2), the second-order differential operator ℓ is defined as follows:
ℓV=∂V∂xϕ(x)+12tr{gT(x)∂2V∂x2g(x)}. | (2.3) |
Definition 2. [17] The equilibrium x=0 of stochastic nonlinear system (2.2) is semi-global finite-time stable in probability (SGFSP) if for all x(t0,ω)=x(0), there exist a constant c>0 and a settling time T∗(c,x0,ω)<∞ to make E[‖x(t,ω)‖]<c, for all t≥t0+T∗.
Lemma 1. [17] Consider the stochastic system (2.2) and assume that f(0), h(0) are bounded uniformly in t. If there exist a C2 Lyapunov function V:Rn→R+, functions ϱ1,ϱ2∈K∞, and constants ˉμ0>0, 0<ˉς0<1 and ˉν0>0 such that
{ϱ1(|x|)≤V(x)≤ϱ2(|x|)ℓV(x)≤−ˉμ0V(x)ˉς0+ˉν0,∀x∈Rn, | (2.4) |
Then, the stochastic nonlinear system (2.2) is SGFSP.
Remark 1. As stated in [17], the Eq (2.4) implies that there exists the stochastic setting time function T∗(x,ω)=1l0ˉμ0(1−ˉς0)[E[V1−ˉς0(x(0))]−(ˉν0(1−l0)ˉμ0)ˉς01−ˉς0], such that E[Vˉς0(x)]≤ˉν0(1−l0)ˉμ0 for all t≥t0+T∗(x,ω), where 0<l0≤1 is an arbitrary constant.
Lemma 2. [7] Let a,b∈R+witha≥1. For any ζ,η∈R, the following inequalities hold:
(i) |ζa−ηa|≤a(2a−2+2)|ζ−η|(|ζ−η|a−1+ηa−1),
(ii) ∣ζba−ηba∣≤21−1a∣⌈ζ⌉b−⌈η⌉b∣1a,
(iii) (|ζ|+|η|)1a≤|ζ|1a+|η|1a≤21−1a(|ζ|+|η|)1a.
Lemma 3. [42] For any constants k1,k2,ϑ,ς∈R+ and any variables ζ1,ζ2∈R, we have the following inequality
ϑ|ζ1|k1|ζ2|k2≤ςk1k1+k2|ζ1|k1+k2+k1k1+k2ϑk1+k2k2ς−k1k2|ζ2|k1+k2. |
Lemma 4. [8] Let p∈(0,∞), for any ζi∈R,i=1,⋯,n, one has
(|ζ1|+⋯+|ζn|)p≤b(|ζ1|p+⋯+|ζn|p), |
where b=max{np−1,1}.
Lemma 5. [36] If ζ,η∈R and p>1 is an odd number, then
−(ζ−η)(ζp−ηp)≤−12p−1(ζ−η)p+1. |
Lemma 6. [12] With any a0>0,c0>0, and ζ(t)>0, for ˙η(t)=a0ζ(t)−c0η(t), if η(0)≥0 can be satisfied, then one has η(t)≥0 for ∀t≥0.
In this paper, the nonlinear functions ϕi(⋅) and gi(⋅) are all unknown. The unknown functions will be approximated by the FLSs based on the following presented lemma.
Lemma 7. [49] Let F(X) be a continuous function defined on a compact set Π0. Then, for a given desired level of accuracy δ>0, there exists a fuzzy logic system ΥTΨ(X) such that
supX∈Π0|F(X)−ΥTΨ(X)|≤δ, |
Υ=(υ1,⋯,υN)T is the ideal constant weight vector, and Ψ(X)=(ψ1(X),⋯,ψN(X))T∑Nj=1ψj(X) is the basis function vector, with N>1 being the number of the fuzzy rules and ψj(X) being chosen as Gaussian functions, i.e., for j=1,⋯,N
ψj(X)=exp[−(X−λj)T(X−λj)ϑ2j] |
where λj=(λj1,⋯,λjn)T and ϑj respectively denote the center vector and the width of the Gaussian function.
Remark 2. In view of Lemma 7, any function F(X) which is defined and continuous on a compact set Π0, can be approximated by
F(X)=ΥTΨ(X)+ϵ(X), |
where ϵ(X) is the FLS approximation error satisfying |ϵ(X)|<δ.
In this section, a fuzzy state-feedback controller will be explicitly designed for system (2.1) by combining a tan-type BLF and the FLSs into the adding a power integrator technique.
First of all, we introduce a coordinate transformation as follow
χ1=x1,χi=xiHqi,i=2,⋯,n,ν=uHqn+1, | (3.1) |
where q1=0, qj=qj−1+1p(j=2,⋯,n+1), and H>1 is a constant to be determined later. Based on (3.1), system (2.1) turns into
dχi=Hχpi+1dt+fi(ˉχi)dt+hTi(ˉχi)dω,i=1,⋯,n−1,dχn=Hνpdt+fn(χ)dt+hTn(χ)dω,y=χ1. | (3.2) |
where ˉχi=(χ1,⋯,χi), f1=ϕ1, h1=g1, fj=ϕjHqj, and hj=gjHqj,(j=2,⋯,n).
In what follows, a fuzzy state-feedback controller will be designed through n steps based on the equivalent system (3.2).
Define
ξ1=χ1,ξi=χi−βi−1,i=2,…,n, | (3.3) |
where βi's are the virtual signals being constructed later.
Step 1. From (3.1), we can get
dξ1=dχ1=(Hχp2+f1)dt+hT1dω. | (3.4) |
Choose the first Lyapunov function
V1=ε42πtan(πξ412ε4)+12b1˜α21≜VB(ξ1)+12b1˜α21, |
where b1>0 is an adjustment parameter, ˜α1=α1−ˆα1 is the estimate error and ˆα1 is the estimator of the parameter α1.
Remark 3. Clearly, VB(ξ1)=ε42πtan(πξ412ε4) is a tan-type BLF adopted to deal with the system output constraint. Compared to the log-type BLF, VB(ξ1) possesses the following characteristic:
limε→∞VB(ξ1)=ε42πtan(πξ412ε4)=ξ414, |
which implies that the proposed method is also applicable to the system without output constraints.
Then, one can easily get from the definition of VB(ξ1) that
∂VB∂ξ1=S1(ξ1)ξ31, | (3.5) |
∂2VB∂ξ21=3S1(ξ1)ξ21+4πε4tan(πξ412ε4)S1(ξ1)ξ61. | (3.6) |
where S1(ξ1)=sec2(πξ412ε4).
In view of (2.3), (3.5) and (3.6), it is not hard to gain
ℓV1=∂VB∂ξ1(Hχp2+f1)+12∂2VB∂ξ21hT1h1−1b1˜α1˙ˆα1=S1(ξ1)ξ31(Hχp2+f1)−1b1˜α1˙ˆα1+32S1(ξ1)‖h1‖2ξ21+2πε4tan(πξ412ε4)S1(ξ1)‖h1‖2ξ61. |
From Lemma 3, one obtains
32S1(ξ1)‖h1‖2ξ21≤34S1(ξ1)2‖h1‖4ξ41+34. |
Then, we have
ℓV1≤HS1(ξ1)ξ31χp2+HS1(ξ1)ξ31F1(Z1)−ϱ1ε42πtan(πξ412ε4)−1b1˜α1˙ˆα1+34, | (3.7) |
where Z1=χ1,
F1(Z1)=1H[f1+34S1(ξ1)2‖h1‖4ξ1]+1H2πε4tan(πξ412ε4)S1(ξ1)‖h1‖2ξ31+ϱ1ε4sin(πξ412ε4)2Hπξ31cos(πξ412ε4). |
and ϱ1>0 is an adjustment parameter.
Thus, by Lemma 7, one can approximate F1(Z1) by
F1(Z1)=ΥT1Ψ1(Z1)+ϵ1(Z1), | (3.8) |
where |ϵ1(Z1)|≤δ1 and δ1>0 is a given constant.
Since ΨT1(⋅)Ψ1(⋅)≤1, it is easily obtained from Lemma 3 that
S1(ξ1)ξ31F1(Z1)≤S1(ξ1)|ξ1|3(‖Υ1‖‖Ψ1‖+δ1)≤3σ11α1p+3(S1(ξ1))p+33ξp+31+3p+3(S1(ξ1))p+33ξp+31+pp+3σ−3p11+pp+3δp+3p1, | (3.9) |
where α1=‖Υ1‖p+33 and σ11>0 is an adjustment parameter.
Substituting (3.9) into (3.7) gets
ℓV1≤HS1(ξ1)ξ31(xp2−βp1)+HS1(ξ1)ξ31βp1−ϱ1ε42πtan(πξ412ε4)+3Hp+3S1(ξ1)p+33[σ11ˆα1+1]ξp+31+pHp+3σ−3p11+H˜α1[3σ11p+3(S1(ξ1))p+33ξp+31−1b1H˙ˆα1]+34+pHp+3δp+3p1. | (3.10) |
Then, one could design
β1=−M1p1ξ1≜−φ1ξ1 | (3.11) |
and
˙ˆα1=3Hb1σ11p+3(S1(ξ1))p+33ξp+31−d1ˆα1, | (3.12) |
where M1≥3p+3(S1(ξ1))p3[σ11ˆα1+1]+ρ1S1(ξ1)+ϱ1>0; ϱ1,d1>0 are adjustment parameters; and the value of ρ1>0 will be given in the next step.
Substituting (3.11) and (3.12) into (3.10), gets
ℓV1≤−ϱ1ε42πtan(πξ412ε4)−H(ρ1+ϱ1)ξp+31+HS1(ξ1)ξ31(χp2−βp1)+d1b1˜α1ˆα1+34+pHp+3σ−3p11+pHp+3δp+3p1. |
In addition, it is easily obtained that
d1b1˜α1ˆα1=d1b1(α1−˜α1)˜α1≤−d1˜α212b1+d1α212b1. |
Therefore, we can get
ℓV1−ϱ1ε42πtan(πξ412ε4)−d1˜α212b1−H(ρ1+ϱ1)ξp+31+Q1+HS1(ξ1)ξ31(xp2−βp1), | (3.13) |
where Q1=34+pHp+3σ−3p11+pHp+3δp+3p1+d1α212b1.
Remark 4. Notice that
limξ1→0ε4sin(πξ412ε4)cos(πξ412ε4)2πξ31=limξ1→0ε4πξ412ε4cos(πξ412ε4)2πξ31=0, |
which means that the continuity of F1(Z1) is ensured.
Remark 5. According to Lemma 6, one gets ˆα1≥0, for ∀t≥0. In each design step, this characteristic will be always applied.
Step 2. From (3.3) and Itˆo's formula, we have
dξ2=(Hχp3+f2−ℓβ1)dt+(h2−∂β1∂χ1h1)Tdω, | (3.14) |
where ℓβ1=∂β1∂χ1(Hχp2+f1)+∂β1∂ˆα1˙ˆα1+12∂2β1∂χ21hT1h1. Combining the definition of β1 with the properties of f1(χ1) and h1(χ1), implies that ℓβ1 is valid and continuous.
Choose the second Lyapunov function as
V2=V1+Λ2 | (3.15) |
with
Λ2=14ξ42+12b2˜α22, | (3.16) |
where b2>0 is an adjustment parameter, ˜α2=α2−ˆα2 is the estimate error and ˆα2 is the estimator of the parameter α2.
Applying (2.3), (3.14) and (3.16), it can be gotten that
ℓΛ2=ξ32(Hχp3+f2−ℓβ1)−1b2˜α2˙ˆα2+32‖h2−∂β1∂χ1h1‖2ξ22. | (3.17) |
Besides, applying Lemma 3 renders
32‖h2−∂β1∂χ1h1‖2ξ22≤34‖h2−∂β1∂χ1h1‖4ξ42+34. |
On the other hand, we gets
S1(ξ1)ξ31(χp2−βp1)≤S1(ξ1)|ξ1|3|χp2−βp1|≤DS1(ξ1)|ξ1|3(|ξ2|p+φp1|ξ1|p−1⋅|ξ2|)≤ρ1ξp+31+τ2ξp+32+pσ12p+3ξp+32, | (3.18) |
where D=(2p−2+2)p; ρ1=1p+3[3(DS1(ξ1))p+33σ−p312+(p+2)S1(ξ1)], τ2=S1(ξ1)φp(p+3)1p+3, and σ12>0 is an adjustment parameter.
Thus, from (3.13), (3.17) and (3.18), we have
ℓV2=ℓV1+ℓΛ2≤−ϱ1ε42πtan(πξ412ε4)−d12b1˜α21−Hϱ1ξp+31−1b2˜α2˙ˆα2+Hξ32(χp3−βp2)+Hξ32βp2+Hξ32F2(Z2)+Hpσ12p+3ξp+32+Q1+34, | (3.19) |
where Z2=(ˉx2,ˆα1)T,
F2(Z2)=1H[f2−ℓβ1]+τ2ξp2+34H‖h2−∂β1∂χ1h1‖4ξ2. |
Obviously, the continuity of F2(Z2) can be directly proved by the fact that the functions f2(ˉχ2), h2(ˉχ2) and ℓβ1 are all continuous. Thus, F2(Z2) can be approximated as
F2(Z2)=ΥT2Ψ2(Z2)+ϵ2(Z2), | (3.20) |
where |ϵ2(Z2)|≤δ2 and δ2>0 is a given constant.
In view of the fact ΨT2(⋅)Ψ2(⋅)≤1 and Lemma 3, we obtain
ξ32F2(Z2)≤|ξ2|3(‖Υ2‖‖Ψ2‖+δ2)≤3σ21α2p+3ξp+32+pp+3σ−3p21+3p+3ξp+32+pp+3δp+3p2, | (3.21) |
where α2=‖Υ2‖p+33 and σ21>0 is an adjustment parameter.
Substituting (3.21) into (3.19) yields
ℓV2≤−ϱ1ε42πtan(πξ412ε4)−d12b1˜α21−Hϱ1ξp+31+Hξ32(χp3−βp2)+Hξ32βp2+3Hp+3[σ21ˆα2+1]ξp+32+Hpσ12p+3ξp+32+H˜α2[3σ21p+3ξp+32−1b2H˙ˆα2]+Q1+34+pHp+3σ−3p21+pHp+3δp+3p2. | (3.22) |
Then, one can design
β2=−M1p2ξ2≜−φ2ξ2 | (3.23) |
and
˙ˆα2=3Hb2σ21p+3ξp+32−d2ˆα2, | (3.24) |
where M2≥3p+3[σ21ˆα2+1]+pσ12p+3+ρ2+ϱ2>0; ϱ2>0,d2>0 are adjustment parameters. The value of ρ2>0 will be given in the next step.
In addition, it is evident that
d2b2ˆα2˜α2≤−d2˜α222b2+d22b2ˆα22. | (3.25) |
Substituting (3.25) into (3.22), one gets
ℓV2≤−ϱ1ε42πtan(πξ412ε4)−2∑j=1dj2bj˜α21−H2∑j=1ϱjξp+3j+Hξ32(χp3−βp2)+2∑j=1Qj, | (3.26) |
where Q2=34+pHp+3σ−3p12+pHp+3δp+3p2+d2α222b2.
Inductive Step (3≤k≤n). In view of above two steps, we can deduce the following similar property whose proof can be found in the Appendix.
Proposition 1. For the kth Lyapunov function Vk:Πk→R+ as
Vk=Vk−1+Λk | (3.27) |
with
Λk=14ξ4k+12bk˜α2k, | (3.28) |
there exists a virtual controller βk and the adaptive law of ˆαk of the following forms
βk=−M1pkξk≜−φkξk, | (3.29) |
˙ˆαk=3Hbkσk1p+3ξp+3k−dkˆαk, | (3.30) |
such that
ℓVk≤−ϱ1ε42πtan(πξ412ε4)−k∑j=1dj˜α2j2bj−Hρkξp+3k−Hk∑j=1ϱjξp+3j+k∑j=1Qj+Hξ3k(χpk+1−βpk), | (3.31) |
where Mk≥3p+3[σk1ˆαk+1]+pσk−12p+3+ρk+ϱk>0; bk,ϱk,dk>0 are adjustment parameters; and the value of ρk>0 will be given in the next step.
Step n According to above steps, there exist a series of virtual controllers and adaptive parameter laws (βk,ˆαk)(k=1,⋯,n) make that Eq (3.31) holds when k=n with χn+1=ν. Therefore, the fuzzy adaptive control law can be designed as
βn=−M1pnξn≜−φnξn, | (3.32) |
˙ˆαn=3Hbnσn1p+3ξp+3n−dnˆαn. | (3.33) |
Apparently, one can further get
ℓVn≤−ϱ1ε42πtan(πξ412ε4)−n∑j=1dj˜α2j2bj−Hn∑j=1ϱjξp+3j+n∑j=1Qj+Hξ3n(νp−βpn). | (3.34) |
By the definitions of ξk(k=1,⋯,n), one gets the fuzzy state-feedback controller
βn=−(ˉφ1χ1+⋯+ˉφnχn), | (3.35) |
where ˉφk=∏nj=kφj for k=1,…,n.
In this subsection, a fuzzy output-feedback controller will be designed by combining the above-constructed state-feedback controller with the state-observer constructed later. Since χ2,⋯,χn are unmeasurable, a reduced-order observer is required. Firstly, define a series of new variables as follows:
zi=χi−γi…γ2χ1,i=2,⋯,n. |
Then, it directly gets
dzi=H(χpi+1−γi…γ2χp2)dt+(fi−γi…γ2f1)dt+[hi−γi…γ2h1]Tdω,i=2,⋯,n−1,dzn=H(νp−γn…γ2χp2)dt+(fn−γn…γ2f1)dt+[hn−γn…γ2h]Tdω, |
where γi≥1(i=2,⋯,n) are gain parameters to be determined. Hence, the (n−1)-dimensional observer can be constructed as [36]
˙ˆzi=H(ˆzi+γi…γ2χ1)p−Hγi…γ2(ˆz2+γ2χ1)p,i=2,⋯,n−1,˙ˆzn=Hνp−Hγn…γ2(ˆz2+γ2χ1)p. | (3.36) |
According to (3.36), the estimate ˆχi of χi can be gotten by
ˆχi=ˆzi+γi…γ2χ1,i=2,⋯,n. | (3.37) |
Thus, one constructs the implementable controller of system (2.4) by using Eq (3.35) and the certainty equivalence principle as below:
ν=−(ˉφ1ˆχ1+⋯+ˉφnˆχn). | (3.38) |
Therefore, the output-feedback controller of origin system (2.1) is
u=Hqn+1ν=−Hqn+1(ˉφ1ˆχ1+⋯+ˉφnˆχn). | (3.39) |
In this section, we will analyze the appropriate values of the gains γi(i=2,⋯,n) and some constant parameters in output-feedback controller.
To determine the observer gains γ2,⋯,γn, we first define the error dynamics
ei≜χi−ˆχi,i=2,⋯,n. | (3.40) |
Further, the following coordinate transformation is introduced
˜e2=e2,˜e3=e3−γ3e2,⋯,˜en=en−γnen−1. | (3.41) |
It can easily infer from (3.40) and (3.41) that
d˜ei=H[(χpi+1−ˆχpi+1)−γi(χpi−ˆχpi)]dt+[fi−γifi−1]dt+[hi−γihi−1]Tdω,i=2,⋯,n−1d˜en=−Hγn(χpn−ˆχpn)dt+[fn−γnfn−1]dt+[hn−γnhn−1]Tdω. | (3.42) |
Now, a proposition is provided for helping to determine gain constants, whose proof will be given in Appendix.
Proposition 2. For the Lyapunov function
Un=14γn∑i=2˜e4i, |
by utilizing Lemmas 2-5, Eqs (2.3) and (3.42), it is not difficult to obtain
ℓUn≤−H[n∑i=2γγi2p−1˜ep+3i+n∑i=2ˉci˜ep+3i+n∑i=26γp+33(p+3)Hp+33˜ep+3i]+(p−1)Hp+3+˜F1(χ), | (3.43) |
where γ>0 is an adjustment constant; ˉci=ˉci(γi+1,⋯,γn)>0,(i=2,⋯,n−1) are constants independent of H; and ˉcn is a constant independent of H and γi(i=2,⋯,n); and ˜F1(χ)=H∑ni=2[14(fi−γifi−1)4+3γ234H23‖hi−γihi−1‖4+2p−1p+3χp+3i] is an unknown continuous function.
Besides, we can obtain from Lemmas 2-4 that
|ξ3n(νp−βpn)|≤D|ξn|3|ν−βn|[|ν−βn|p+|βn|p]≤D(n−1)p|ξn|3⋅n∑i=2ˉφpi|ei|p+D|ξn|3⋅(n∑i=2ˉφi|ei|)⋅φp−1n|ξn|p−1≤p+1p+3n∑i=2ep+3i+τnξp+3n≤˜ιin∑i=2˜ep+3i+˜F2(ξn), | (3.44) |
where ˜F2(ξn)=τnξp+3n; τn=3p+3∑ni=2[D(n−1)pˉφpi]p+33+p+2p+3∑ni=23[D(n−1)pφp−1nˉφi]p+3p+2; ˜ιn=np+2p+1p+3 and ˜ιi=˜ιi(γi+1,⋯,γn)(i=2,⋯,n−1) are positive constants.
Then, substituting (3.44) into (3.34) yields
ℓVn≤−ϱ1ε42πtan(πξ412ε4)−n∑j=1dj˜α2j2bj−Hn∑j=1ϱjξp+3j+n∑j=1Qj+Hn∑i=2˜ιi˜ep+3i+˜F2(ξn). | (3.45) |
Then, define the entire Lyapunov function V=Vn+Un. Apparently, it directly infers from Proposition 2 and Eq (3.45) that
ℓV=ℓVn+ℓUn≤−ϱ1ε42πtan(πξ412ε4)−n∑j=1dj˜α2j2bj−Hn∑j=1ϱjξp+3j+˜F(⋅)+n∑j=1Qj−Hn∑i=2[γγi2p−1−ˉci−˜ιi]˜ep+3i+Hn∑i=26γp+33(p+3)Hp+33˜ep+3i+(p−1)Hp+3, | (3.46) |
where ˜F(⋅)=˜F1(⋅)+˜F2(⋅).
Using FLS to deal with the unknown function ˜F(⋅), one deduces from Lemma 7 that ˜F(⋅)=ΥT0Ψ0+ϵ0(⋅)≤‖Υ0‖‖Ψ0‖+δ0≤3p+3α0+pp+3+δ0 where α0=‖Υ0‖p+33.
Therefore, the observer gains γ2,⋯,γn and constant H can be chosen in the following recursive manner
γn≥max{2p−1γ(ˉcn+˜ιn+1+θn),1},γn−1≥max{2p−1γ(ˉcn−1(γn)+˜ιn−1(γn)+1+θn−1),1},⋮γ2≥max{2p−1γ(ˉc2(γn,⋯,γ3)+˜ι2(γn,⋯,γ3)+1+θ2),1},H≥max{1,(6p+3)3p+3γ}. | (3.47) |
Then, Eq (3.46) turns into
ℓV≤−ϱ1ε42πtan(πξ412ε4)−n∑j=1dj˜α2j2bj−Hn∑i=1ϱiξp+3i−Hn∑i=2θi˜ep+3i+Q, | (3.48) |
where θi(i=2,⋯,n) are positive constants, and Q=∑ni=1Qj+(p−1)Hp+3+3p+3α0+pp+3+δ0.
On the other hand, it can be verified from Lemma 3 that
˜e4i≤4p+3˜ep+3i+p−1p+3,ξ4i≤4p+3ξp+3i+p−1p+3, | (3.49) |
which renders
−Hn∑i=2θi˜ep+3i≤−n∑i=2ˉθi4˜e4i+p−14Hn∑i=2θi,−Hn∑i=2ϱiξp+3i≤−n∑i=2ˉϱi4ξ4i+p−14Hn∑i=2ϱi, | (3.50) |
where i=2,⋯,n, ˉθi=(p+3)Hθi and ˉϱi=(p+3)Hϱi. Further, we get
ℓV≤−ϱ1ε42πtan(πξ412ε4)−n∑j=1dj˜α2j2bj−n∑i=2ˉϱi4ξ4i−n∑i=2ˉθi4˜e4i+ˉQ, | (3.51) |
where ˉQ=Q+p−14H∑ni=2θi+p−14H∑ni=2ϱi.
To state the main result, the following theorem is presented.
Theorem 1. For the p-norm stochastic nonlinear system (2.1) and a given constant, there exists a finite-time fuzzy output-feedback controller (3.39) together with the parameter adaptive laws (3.12), (3.24), (3.30), and (3.33) such that
i) the system output isn't violated in the sense of probability, i.e., P{|y(t)|<ε}=1.
ii) all the signals in the closed-loop stochastic nonlinear system (2.1) are SGFSP.
Proof. ⅰ) Let μ0=min{ϱ1,d1,⋯,dn,ˉϱ2,⋯ˉϱn,ˉθ2γ,⋯ˉθnγ} and π0=ˉQ. Then, Eq (3.51) can be expressed as
ℓV≤−μ0V+π0. | (3.52) |
We can easily get from Eq (3.52) that
EV(t)≤V(t0)e−μ0t+π0μ0. | (3.53) |
For x(0)=(x1(t0),⋯,xn(t0))T satisfying x1(t0)∈Π1, it easily obtains that the mean of V(t) is bounded, which implies that V is bounded in probability. It can be directly deduced from the definition of V that
P{VB(ξ1)<∞}=1. | (3.54) |
Consequently, it is clear that P{|y(t)|<ε}=P{|ξ1(t)|<ε}=1, which demonstrates that the output constraint of system (2.1) is not violated in the sense of probability.
ⅱ) For ∀0<ˉς0<1, it is easy to get from Lemma 3 that
Vˉς0≤ˉς0V+(1−ˉς0). |
Further, one has
−μ0V≤−μ0ˉς0Vˉς0+(1−ˉς0)μ0ˉς0. | (3.55) |
Then, substituting (3.55) into (3.52) drives
ℓV≤−ˉμ0Vˉς0+ˉπ0, | (3.56) |
where ˉμ0=μ0ˉς0 and ˉπ0=(1−ˉς0)μ0ˉς0+π0.
Let T∗=1l0ˉμ0(1−ˉς0)[E(V1−ˉς0(χ(0),˜e(0),ˆα(0)))−(ˉπ0ˉμ0(1−ˉς0))1−ˉς0ˉς0] where χ(0)=(χ1(t0),⋯,χn(t0))T, ˜e(0)=(˜e2(t0),⋯,˜en(t0))T, ˆα(0)=(ˆα1(t0),⋯,ˆαn(t0)), 0<l0<1 is a constant. Then it follows from Lemma 1 that for ∀t≥t0+T∗, E(V1−ς(χ,˜e,ˆα))≤ˉπ0ˉμ0(1−ˉς0), which means that all the signals in the closed-loop systems are semi-global finite-time stable in probability.
Remark 6. In this paper, we construct an output-feedback controller rather than the designed state-feedback controllers in existing results about output constraints. On the other hand, it should be pointed out the considered constraint is symmetric rather than asymmetric, which leads that the proposed scheme can not be directly employed or further extended to the case of asymmetric constraints. However, a control scheme based on a new BLF can be developed for asymmetric output constraints in a similar way to this paper. In addition, another limitation is that all of the fractional powers are equal to p. If pi's are taken different values, the proposed strategy seems not applicable. In the future, we will address the two issues.
The validation of the proposed strategy will be testified by the following system.
{dx1=x1352dt+2ln(1+x21)dt+4x21dω,dx2=u135dt+x1x22dt+x22dω,y=χ1, | (4.1) |
where the output y=x1 is measurable and constrained by Π1={y(t)∈R,|y(t)|<1}, and the state x2 is unmeasurable.
According to the controller design procedure, we can respectively design the finite-time output-feedback controller, the adaptive laws and the observer as follows:
u=−H90169(M5131x1+M5132ˆχ2),˙ˆα1=15Hb1σ1128(S1(x1))2815x2851−d1ˆα1,˙ˆα2=15Hb2σ2128ξ2852−d2ˆα2,˙ˆz2=Hν135−Hγ2(ˆz2+γ2x1)135,ˆχ2=ˆz2+γ2x1, | (4.2) |
where M1=1528(S1(x1))1315[σ11ˆα1+1]+ρ1S1(x1)+ϱ1 and M2=1528[σ21ˆα2+1]+13σ1228+ρ2+ϱ2.
Now, we choose the related parameters as γ=1.5,σ11=22,σ21=20,σ12=1, θ2=3, b1=b2=40, d1=d2=2, ϱ1=ϱ2=1 and infer H=1.6,γ2=8.1. Next, the initial states and adaptive parameters are selected as [x1(0),x2(0),ˆx2(0),ˆα1,ˆα2]T=[0.4,8,8,10,10]T the simulation results are displayed in Figures 1–4.
Figure 1 provides the trajectory of x1(t), which indicates that the system output constraint is not violated under controller (4.2). Meanwhile, the trajectories of x2(t) and ˆx2(t) are given in Figure 2, which shows that x2(t) is well estimated by ˆx2(t). Moreover, the trajectory of the controller u is displayed in Figure 3. Finally, Figure 4 expresses the curves of the adaptive parameter vector under the developed strategy. Also, one could evidently observe from these figures that all the signals of system (4.1) are semi-global finite-time stable in probability under controller (4.2).
In this paper, the output-feedback controller design problem is investigated for a class of p-norm stochastic nonlinear systems with output constraints. Through using a tan-type BLF, an adaptive fuzzy state-feedback controller is proposed by the adding a power integrator technique. Then, a finite-time fuzzy output-feedback controller is constructed by combining the proposed state-feedback controller and a reduced-order observer. Both rigorous proof and the simulation example verify that the designed controller can ensure the achievement of the system output constraint and semi-global finite-time stability of all the signals in probability. In the future, we will consider the situations of asymmetric constraints, different fractional powers, or multi-input multi-output stochastic nonlinear systems.
This work was supported by supported by the National Science Foundation of China under Grant 61973142, the Jiangsu Natural Science Foundation for Distinguished Young Scholars under Grant BK20180045, the Priority Academic Program Development of Jiangsu Higher Education Institutions, and the Project of Anhui Province Outstanding Young Talent Support Program under Grant gxyq2018089.
The authors declare that there are no conflicts of interest.
Proof of Proposition 1. Firstly, suppose there exist βk−1(3≤k≤n) such that
ℓVk−1≤−ϱ1ε42πtan(πξ412ε4)−k−1∑j=1dj˜α2j2bj−Hρk−1ξp+3k−1−Hk−1∑j=1ϱjξp+3j+k−1∑j=1Qj+Hξ3k−1(χpk−βpk−1). | (A.1) |
At the same time, from Itˆo's formula and (3.1), one gets
dξk=(Hχpk−1+fk−ℓβk−1)dt+(hk−k−1∑j=1∂βk−1∂χjhj)Tdω, | (A.2) |
where ℓβk−1=∑k−1j=1∂βk−1∂χj(Hχpj+1+fj)+Σk−1j=1∂βk−1∂ˆαj˙ˆαj+12∑k−1j,l=1∂2βk−1∂χj∂χlhTjhl. Clearly, ℓβk−1 is valid and continuous, which can be illustrated in a similar way by following the lines to obtain ℓβ1.
We choose the Lyapunov function as
Vk=Vk−1+Λk | (A.3) |
with
Λk=14ξ4k+12bk˜α2k, | (A.4) |
where bk>0 is an adjustment parameter, ˜αk=αk−ˆαk is the parameter error, and ˆαk is the estimation of the unknown parameter αk.
Applying (2.3), (A.2) and (A.4), one can obtain
ℓΛk=ξ3k(Hχpk+1+fk−ℓβk−1)−1bk˜αk˙ˆαk+32‖hk−k−1∑j=1∂βk−1∂χjhj‖2ξ2k. | (A.5) |
It is not difficult to get from Lemma 3 that
32‖hk−k−1∑j=1∂βk−1∂χjhj‖2ξ2k≤34‖hk−k−1∑j=1∂βk−1∂χjhj‖4ξ4k+34. | (A.6) |
On the other hand, from Lemmas 2 and 3, one can verify
ξ3k−1(χpk−βpk−1)≤D|ξk−1|3[|ξk|p+φp−1k−1|ξk−1|p−1⋅|ξk|]≤ρk−1ξp+3k−1+τkξp+3k+pσk−12p+3ξp+3k, | (A.7) |
where ρk−1=1p+3[3Dp+33σ−p312+p+2], τk=φ(p−1)(p+3)1p+3, and σk−12>0 is an adjustment parameter.
From (A.1)-(A.7), it can be deduced that
ℓVk=ℓVk−1+ℓΛk≤−ϱ1ε42πtan(πξ412ε4)−k−1∑j=1dj˜α2j2bj−Hk−1∑j=1ϱjξp+3j+k−1∑j=1Qj+34+Hpσk−12p+3ξp+3k+Hξ3kFk(Zk)+Hξ3k(χpk+1−βpk)+Hξ3kβpk | (A.8) |
where Zk=(ˉχTk,ˉˆαTk−1)T, ˉˆαk−1=(ˆα1,⋯,ˆαk−1)T and
Fk(Zk)=1H(fk−ℓβk−1)+τkξpk+34‖hk−k−1∑j=1∂βk−1∂χjhj‖4ξk. |
Similar to the first two steps, Fk(Zk) can also be approximated as
Fk(Zk)=‖ΥTkΨk(Zk)‖+ϵk(Zk), | (A.9) |
where |ϵk(Zk)|≤δk and δk>0 is a given constant.
One directly obtains from Eq (A.9) and Lemma 3 that
ξ3kFk(Zk)≤|ξk|3(‖Υk‖‖Ψk‖+δk)≤3σk1αkp+3ξp+3k+pp+3σ−3pk1+3p+3ξp+3k+pp+3δp+3pk, | (A.10) |
where αk=‖Υk‖p+33 and σk1>0 is an adjustment parameter.
Substituting (A.10) into (A.8) renders
ℓVk≤−ϱ1ε42πtan(πξ412ε4)−k−1∑j=1dj˜α2j2bj−Hk−1∑j=1ϱjξp+3j+Hξ3k(χpk+1−βpk)+3Hp+3[σk1ˆαk+1]ξp+3k+Hpσk−12p+3ξp+3k+Hξ3kβpk+k−1∑j=1Qj+H˜αk[3σk1p+3ξp+3k−1bkH˙ˆαk]+34+pHp+3σ−3pk1+pHp+3δp+3pk. | (A.11) |
Then, we can design
βk=−M1pkξk≜−φkξk,˙ˆαk=3Hbkσk1p+3ξp+3k−dkˆαk, | (A.12) |
where Mk≥3p+3[σk1ˆαk+1]+pσk−12p+3+ρk+ϱk; ϱk,dk>0 are adjustment parameters; and the value of ρk>0 will be given in (k+1)th step.
Moreover, one has
dkbkˆαk˜αk≤−dk2bk˜α2k+dk2bkˆα2k. | (A.13) |
From (A.1)-(A.13), it can be deduced that
ℓVk≤−ϱ1ε42πtan(πξ412ε4)−k∑j=1dj˜α2j2bj−Hk∑j=1ϱjξp+3j−Hτkξp+3k+k∑j=1Qj+Hξ3k(χpk+1−βpk), | (A.14) |
where Qk=34+pHp+3σ−3pk1+pHp+3δp+3pk+dkα2k2bk. The proof of Proposition 1 is completed.
Proof of Proposition 2. By the definition of Un, one has
ℓUn=Hγn−1∑i=2˜e3i(χpi+1−ˆχpi+1)−Hγn∑i=2˜e3iγi(χpi−ˆχpi)+γn∑i=2˜e3i(fi−γifi−1)+32γn∑i=2˜e2i‖hi−γihi−1‖2=−Hn∑i=2γγi˜e3i[(ˆχi+˜ei)p−ˆχpi]−Hn∑i=2γγi˜e3i[χpi−(ˆχi+˜ei)p]+Hn∑i=3γ˜e3i−1(χpi−ˆχpi)+Hn∑i=2γH˜e3i(fi−γifi−1)+Hn∑i=23γ2H˜e2i‖hi−γihi−1‖2. | (A.15) |
By Lemma 5, one can infer
−γγi˜e3i[(ˆχi+˜ei)p−ˆχpi]=−γγi˜e2i(ˆχi+˜ei−ˆχi)[(ˆχi+˜ei)p−ˆχpi]≤−γγi2p−1˜ep+3i. | (A.16) |
Since χi−ˆχi−˜ei=∑i−1j=2γi⋯γj+1˜ej, we can get that through applying Lemmas 2-5
|−γγi˜e3i[χpi−(ˆχi+˜ei)p]|≤γγi|˜ei|3|χi−ˆχi−˜ei||(χi−ˆχi−˜ei)p−1+χp−1i|≤γi|˜ei|3(i−1∑j=2γi⋯γj+1|˜ej|)p+γi|˜ei|3[1p(i−1∑j=2γi⋯γj+2|˜ej|)p+p−1p|χi|p]≤3γγip+3˜ep+3i+pγγip+3(i−1∑j=2γi⋯γj+1|˜ej|)p+3+3γγip(p+3)˜ep+3i+γγip+3(i−1∑j=2γi⋯γj+2|˜ej|)p+3+3(p−1)(γγi)p+33p(p+3)˜ep+3i+p−1p+3χp+3i≤i∑j=2˜aij˜ep+3j+p−1p+3χp+3i, | (A.17) |
where ˜aij=˜aij(γi,⋯,γj+1) is a constant independent of H.
Noting that ei=∑i−1j=2γi⋯γj+1˜ej+˜ei, one has
|γ˜e3i−1(χpi−ˆχpi)|=γ|˜ei−1|3|χpi−(χi−ei)p|≤2pγ|˜ei−1|3(|χi|p+|ei|p)≤3(2pγ)p+33p+3˜ep+3i−1+pp+3χp+3i+3(2pγ)p+33p+3˜ep+3i−1+pp+3ep+3i≤6(2pγ)p+33p+3˜ep+3i−1+p2p+2p+3[ξp+3i+φp+3i−1ξp+3i−1]+pip+2p+3[˜ep+3i+i−1∑j=2(γi⋯γj+1)p+3˜ep+3j]≤i∑j=2˜cij(γi,⋯,γj+1)˜ep+3j+pp+3χp+3i, | (A.18) |
where ˜cij=˜cij(γi,⋯,γj+1) is a constant independent of H.
In addition, we have
γH˜e3i(fi−γifi−1)+32γH˜e2i‖hi−γihi−1‖2≤3γ432H43˜e4i+14(fi−γifi−1)4+γ234H23‖hi−γihi−1‖4≤6γp+33(p+3)Hp+33˜ep+3i+p−1p+3+14(fi−γifi−1)4+3γ234H23‖hi−γihi−1‖4. | (A.19) |
Substituting (A.16)-(A.19) into (A.15) yields
ℓUn≤−H[n∑i=2γγi2p−1˜ep+3i−n∑i=2ˉci˜ep+3i−6γp+33(p+3)Hp+33˜ep+3i]+(p−1)Hp+3+n∑i=2(2p−1)Hp+3χp+3i+n∑i=2H[14(fi−γifi−1)4+3γ234H23‖hi−γihi−1‖4], |
where ˉci=∑ij=2(˜aji+˜cji). The proof of Proposition 2 is completed.
[1] | S. Y. Khoo, J. L. Yin, Z. Man, X. Yu, Finite-time stabilization of stochastic nonlinear systems in strict-feedback form, Automatica, 47 (2013), 1403–1410. |
[2] | S. H. Ding, W. H. Chen, K. Q. Mei, D. Murray-Smith, Disturbance observer design for nonlinear systems represented by input-output models, IEEE Trans. Ind. Electron, 67 (2020), 1222–1232. |
[3] | X. D. Li, X. Y. Yang, T. W. Huang, Persistence of delayed cooperative models: Impulsive control method, Appl. Math. Comput., 342 (2019), 130–146. |
[4] | H. Shen, M. S. Chen, Z. G. Wu, J. D. Cao, J. H. Park, Reliable event-triggered asynchronous passive control for semi-Markov jump fuzzy systems and its application, IEEE T. Fuzzy Syst., 28 (2020), 1708–1722. |
[5] |
S. H. Ding, A. Levant, S. H. Li, Simple homogeneous sliding-mode controller, Automatica, 67 (2016), 22–32. doi: 10.1016/j.automatica.2016.01.017
![]() |
[6] |
X. Y. Yang, X. D. Li, Q. Xi, P. Y. Duan, Review of stability and stabilization for impulsive delayed systems, Math. Biosci. Eng., 15 (2018), 1495–1515. doi: 10.3934/mbe.2018069
![]() |
[7] |
S. H. Ding, S. H. Li, Second-order sliding mode controller design subject to mismatched term, Automatica, 77 (2017), 388–392. doi: 10.1016/j.automatica.2016.07.038
![]() |
[8] | K. Q. Mei, S. H. Ding, Second-order sliding mode controller design subject to an upper-triangular structure, IEEE T. Syst. Man Cybern. Syst., (2018), 1–11. |
[9] | S. C. Tong, Y. M. Li, Observer-based adaptive fuzzy backstepping control of uncertain nonlinear pure-feedback systems, Sci. China Inform. Sci., 57 (2014), 1–14. |
[10] |
J. T. Hu, G. X. Sui, X. X. Lv, X. D. Li, Fixed-time control of delayed neural networks with impulsive perturbations, Nonlinear Anal. Model. Control, 23 (2018), 904–920. doi: 10.15388/NA.2018.6.6
![]() |
[11] |
X. D. Zhao, X. Y. Wang, L. Ma, G. D. Zong, Fuzzy approximation based asymptotic tracking control for a class of uncertain switched nonlinear systems, IEEE T. Fuzzy Syst., 28 (2020), 632–644. doi: 10.1109/TFUZZ.2019.2912138
![]() |
[12] | F. Wang, B. Chen, Y. Sun, Y. Gao, C. Lin, Finite-time fuzzy control of stochastic nonlinear systems, IEEE T. Syst. Man Cybern., 50 (2020), 2617–2626. |
[13] | L. Liu, W. Zheng, S. H. Ding, An adaptive SOSM controller design by using a sliding-mode-based filter and its application to buck converter, IEEE T. Circ. Syst. I., 67 (2020), 2409–2418. |
[14] | H. Y. Li, Y. Wu, M. Chen, Adaptive fault-tolerant tracking control for discrete-time multi-agent systems via reinforcement learning algorithm, IEEE T. Syst. Man Cybern., (2020), 1–12. |
[15] | Q. Zhou, W. Wang, H. Liang, M. Basin, B. Wang, Observer-based event-triggered fuzzy adaptive bipartite containment control of multi-agent systems with input quantization, IEEE T. Fuzzy Syst., (2019), 1–1. |
[16] |
Z. F. Li, T. S. Li, G. Feng, R. Zhao, Q. H. Shan, Neural network-based adaptive control for purefeedback stochastic nonlinear systems with time-varying delays and dead-zone input, IEEE T. Syst. Man Cybern. Syst., 50 (2020), 5317–5329. doi: 10.1109/TSMC.2018.2872421
![]() |
[17] |
S. Sui, C. L. P. Chen, S. C. Tong, Fuzzy adaptive finite-time control design for non-triangular stochastic nonlinear systems, IEEE T. Fuzzy Syst., 27 (2019), 172–184. doi: 10.1109/TFUZZ.2018.2882167
![]() |
[18] |
B. Niu, Y. J. Liu, W. L. Zhou, H. T. Li, P. Y. Duan, J. Q. Li, Multiple Lyapunov functions for adaptive neural tracking control of switched nonlinear nonlower-triangular systems, IEEE T. Cybernetics, 50 (2020), 1877–1886. doi: 10.1109/TCYB.2019.2906372
![]() |
[19] |
Y. M. Li, S. C. Tong, T. S. Li, Observer-based adaptive fuzzy tracking control of MIMO stochastic nonlinear systems with unknown control directions and unknown dead zones, IEEE T. Fuzzy Syst., 23 (2015), 1228–1241. doi: 10.1109/TFUZZ.2014.2348017
![]() |
[20] | X. D. Li, J. H. Shen, R. Rakkiyappan, Persistent impulsive effects on stability of functional differential equations with finite or infinite delay, Appl. Math. Comput., 329 (2018), 14–22. |
[21] | J. H. Park, H. Shen, X. H. Chang, T. H. Lee, Recent advances in control and filtering of dynamic systems with constrained signals, Switzerland: Springer, 2019. |
[22] | L. D. Fang, L. Ma, S. H. Ding, J. H. Park, Finite-time stabilization of high-order stochastic nonlinear systems with asymmetric output constraints, IEEE T. Syst. Man Cybern. Syst., (2020), 1–13. |
[23] |
C. C. Chen, A unified approach to finite-time stabilization of high-order nonlinear systems with and without an output constraint, Int. J. Robust Nonlin. Control, 29 (2019), 393–407. doi: 10.1002/rnc.4393
![]() |
[24] |
S. H. Ding, J. H. Park, C. C. Chen, Second-order sliding mode controller design with output constraint, Automatica, 112 (2020), 108704. doi: 10.1016/j.automatica.2019.108704
![]() |
[25] |
L. B. Wu, J. H. Park, Adaptive fault-tolerant control of uncertain switched nonaffine nonlinear systems with actuator faults and time delays, IEEE T. Syst., Man, Cybern., Syst., 50 (2020), 3470–3480. doi: 10.1109/TSMC.2019.2894750
![]() |
[26] |
X. Jin, Adaptive fault tolerant tracking control for a class of stochastic nonlinear systems with output constraint and actuator faults, Syst. Control Lett., 107 (2017), 100–109. doi: 10.1016/j.sysconle.2017.07.007
![]() |
[27] |
S. H. Ding, K. Q. Mei, S. H. Li, A new second-order sliding mode and its application to nonlinear constrained systems, IEEE T. Automat. Contr., 64 (2019), 2545–2552. doi: 10.1109/TAC.2018.2867163
![]() |
[28] | Y. M. Li, S. C. Tong, Adaptive fuzzy output constrained control design for multi-input multioutput stochastic nonstrict-feedback nonlinear systems, IEEE T. Syst. Man Cybern., 47 (2017), 4086– 4095. |
[29] | B. Niu, W. Ding, H. Li, X. Xie, A novel neural-network-based adaptive control scheme for outputconstrained stochastic switched nonlinear systems, IEEE T. Syst. Man Cybern. Syst., 49 (2017), 418–432. |
[30] | S. Yin, H. Yu, R. Shahnazi, A. Haghani, Fuzzy adaptive tracking control of constrained nonlinear switched stochastic pure-feedback systems, IEEE T. Syst. Man Cybern., 47 (2017), 579–588. |
[31] | Q. K. Hou, S. H. Ding, X. H. Yu, Composite super-twisting sliding mode control design for PMSM speed regulation problem based on a novel disturbance observer, IEEE T. Energy Conver., (2020), 1–1. |
[32] |
D. Yang, X. D. Li, J. L. Qiu, Output tracking control of delayed switched systems via statedependent switching and dynamic output feedback, Nonlinear Anal. Hybri. Syst., 32 (2019), 294–305. doi: 10.1016/j.nahs.2019.01.006
![]() |
[33] |
H. Wang, Q. Zhu, Finite-time stabilization of high-order stochastic nonlinear systems in strictfeedback form, Automatica, 54 (2015), 284–291. doi: 10.1016/j.automatica.2015.02.016
![]() |
[34] | W. T. Zha, J. Y. Zhai, S. M. Fei, Output feedback control for a class of stochastic high-order nonlinear systems with time-varying delays, Int. J. Robust Nonlin. Control, 24 (2015), 2243–2260. |
[35] |
H. Wang, Q. Zhu, Global stabilization of stochastic nonlinear systems via C1 and C∞ controllers, IEEE T. Automat. Contr., 62 (2017), 5880–5887. doi: 10.1109/TAC.2016.2644379
![]() |
[36] |
W. Q. Li, X. J. Xie, S. Y. Zhang, Output-feedback stabilization of stochastic high-order nonlinear systems under weaker conditions, SIAM J. Control Optim., 49 (2011), 1262–1282. doi: 10.1137/100798259
![]() |
[37] |
M. Jiang, X. Xie, K. Zhang, Finite-time stabilization of stochastic high-order nonlinear systems with FT-SISS inverse dynamics, IEEE T. Automat. Contr., 64 (2019), 313–320. doi: 10.1109/TAC.2018.2827993
![]() |
[38] |
W. J. Si, X. D. Dong, F. F. Yang, Decentralized adaptive neural control for high-order interconnected stochastic nonlinear time-delay systems with unknown system dynamics, Neural Netw., 99 (2018), 123–133. doi: 10.1016/j.neunet.2017.12.013
![]() |
[39] |
N. Duan, H. F. Min, Decentralized adaptive NN state-feedback control for large-scale stochastic high-order nonlinear systems, Neurocomputing, 173 (2016), 1412–1421. doi: 10.1016/j.neucom.2015.09.013
![]() |
[40] | X. D. Zhao, X. Y. Wang, G. D. Zong, X. L. Zheng, Adaptive neural tracking control for switched high-order stochastic nonlinear systems, IEEE T. Syst. Man Cybern., 47 (2017), 3088–3099. |
[41] | L. D. Fang, L. Ma, S. H. Ding, D. A. Zhao, Finite-time stabilization for a class of high-order stochastic nonlinear systems with an output constraint, Appl. Math. Comput., 358 (2019), 63–79. |
[42] |
C. C. Chen, Z. Y. Sun, A unified approach to finite-time stabilization of high-order nonlinear systems with an asymmetric output constraint, Automatica, 111 (2020), 108581. doi: 10.1016/j.automatica.2019.108581
![]() |
[43] |
L. D. Fang, L. Ma, S. H. Ding, D. A. Zhao, Robust finit-time stabilization of a class of high-order stochastic nonlinear systems subject to output constraint and disturbances, Int. J. Robust Nonlin. Control, 29 (2019), 5550–5573. doi: 10.1002/rnc.4685
![]() |
[44] |
C. C. Chen, Z. Y. Sun, Output feedback finite-time stabilization for high-order planar systems with an output constraint, Automatica, 114 (2020), 108843. doi: 10.1016/j.automatica.2020.108843
![]() |
[45] |
Y. Wu, X. J. Xie, Adaptive fuzzy control for high-order nonlinear time-delay systems with full-state constraints and input saturation, IEEE T. Fuzzy Syst., 28 (2020), 1652–1663. doi: 10.1109/TFUZZ.2019.2920808
![]() |
[46] | L. D. Fang, S. H. Ding, J. H. Park, L. Ma, Adaptive fuzzy control for stochastic high-order nonlinear systems with output constraints, IEEE T. Fuzzy Syst., (2020), 1–1. |
[47] | W. Sun, S. F. Su, G. W. Dong, W. W. Bai, Reduced adaptive fuzzy tracking control for highorder stochastic nonstrict feedback nonlinear system with full-state constraints, IEEE T. Syst. Man Cybern. Syst., (2019), 1–11. |
[48] | L. D. Fang, H. S. Ding, J. H. Park, L. Ma, Adaptive Fuzzy Control for Nontriangular Stochastic High-Order Nonlinear Systems Subject to Asymmetric Output Constraints, IEEE T. Cybernetics, (2020), 1–12. |
[49] | L. X. Wang, Adaptive fuzzy systems and control, Englewood Cliffs, NJ: PTR Prentice Hall, 1994. |
1. | Rong-Heng Cui, Xue-Jun Xie, Output feedback stabilization of stochastic planar nonlinear systems with output constraint, 2022, 143, 00051098, 110471, 10.1016/j.automatica.2022.110471 | |
2. | Chen Ding, Li Ma, Shihong Ding, Keqi Mei, 2021, Finite-time Stabilization for SOSM Dynamics Subject to Time-varying Output Constraint, 978-1-6654-2423-3, 1076, 10.1109/DDCLS52934.2021.9455703 | |
3. | Ruiming Xie, Shengyuan Xu, Output feedback stabilization of stochastic high-order planar nonlinear systems with stochastic inverse dynamics and output-constraint, 2024, 67, 1674-733X, 10.1007/s11432-023-3875-5 | |
4. | Changgui Wu, Liang Zhao, Finite-time adaptive dynamic surface control for output feedback nonlinear systems with unmodeled dynamics and quantized input delays, 2024, 9, 2473-6988, 31553, 10.3934/math.20241518 | |
5. | Mojtaba Hosseini Toodeshki, Qijia Yao, Fuzzy adaptive fixed-time control for output-constrained uncertain nonstrict-feedback time-delay systems, 2024, 361, 00160032, 107302, 10.1016/j.jfranklin.2024.107302 | |
6. | Kemei Zhang, You Wu, Xue-Jun Xie, Output-feedback stabilisation of output-constrained high-order nonlinear systems with high-order and low-order nonlinearities, 2025, 0020-7721, 1, 10.1080/00207721.2025.2482004 |