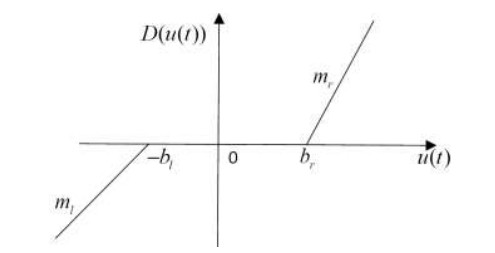
Citation: Wei Zhao, Lei Liu, Yan-Jun Liu. Adaptive neural network control for nonlinear state constrained systems with unknown dead-zones input[J]. AIMS Mathematics, 2020, 5(5): 4065-4084. doi: 10.3934/math.2020261
[1] | Yanxin Li, Shangkun Liu, Jia Li, Weimin Zheng . Congestion tracking control of multi-bottleneck TCP networks with input-saturation and dead-zone. AIMS Mathematics, 2024, 9(5): 10935-10954. doi: 10.3934/math.2024535 |
[2] | Hadil Alhazmi, Mohamed Kharrat . Echo state network-based adaptive control for nonstrict-feedback nonlinear systems with input dead-zone and external disturbance. AIMS Mathematics, 2024, 9(8): 20742-20762. doi: 10.3934/math.20241008 |
[3] | Shihua Zhang, Xiaohui Qi, Sen Yang . A cascade dead-zone extended state observer for a class of systems with measurement noise. AIMS Mathematics, 2023, 8(6): 14300-14320. doi: 10.3934/math.2023732 |
[4] | Xiaoling Liang, Chen Xu, Duansong Wang . Adaptive neural network control for marine surface vehicles platoon with input saturation and output constraints. AIMS Mathematics, 2020, 5(1): 587-602. doi: 10.3934/math.2020039 |
[5] | Mohamed Kharrat, Moez Krichen, Loay Alkhalifa, Karim Gasmi . Neural networks-based adaptive command filter control for nonlinear systems with unknown backlash-like hysteresis and its application to single link robot manipulator. AIMS Mathematics, 2024, 9(1): 959-973. doi: 10.3934/math.2024048 |
[6] | Miao Xiao, Zhe Lin, Qian Jiang, Dingcheng Yang, Xiongfeng Deng . Neural network-based adaptive finite-time tracking control for multiple inputs uncertain nonlinear systems with positive odd integer powers and unknown multiple faults. AIMS Mathematics, 2025, 10(3): 4819-4841. doi: 10.3934/math.2025221 |
[7] | Xiaohang Su, Peng Liu, Haoran Jiang, Xinyu Yu . Neighbor event-triggered adaptive distributed control for multiagent systems with dead-zone inputs. AIMS Mathematics, 2024, 9(4): 10031-10049. doi: 10.3934/math.2024491 |
[8] | Kairui Chen, Yongping Du, Shuyan Xia . Adaptive state observer event-triggered consensus control for multi-agent systems with actuator failures. AIMS Mathematics, 2024, 9(9): 25752-25775. doi: 10.3934/math.20241258 |
[9] | Yihang Kong, Xinghui Zhang, Yaxin Huang, Ancai Zhang, Jianlong Qiu . Prescribed-time adaptive stabilization of high-order stochastic nonlinear systems with unmodeled dynamics and time-varying powers. AIMS Mathematics, 2024, 9(10): 28447-28471. doi: 10.3934/math.20241380 |
[10] | Mohamed Kharrat, Hadil Alhazmi . Neural networks-based adaptive fault-tolerant control for a class of nonstrict-feedback nonlinear systems with actuator faults and input delay. AIMS Mathematics, 2024, 9(6): 13689-13711. doi: 10.3934/math.2024668 |
In the last few years, the uncertainties general consist in the nonlinear systems, the most important is that it is also widely applied in constraint. By taking adaptive control [1], the parametric uncertainty of nonlinear system is presented in [2]. In the actual systems, out of the realistic need as well as the difficulty of operations, the unknown continuous functions are approximated, which is based on fuzzy logic systems (FLSs) or neural networks (NNs) in [3,4,5,6,7]. On the basis of NNs or FLSs, adaptive control algorithm comes up for nonlinear single-input single-output (SISO) systems [8]. As same as for MIMO nonlinear systems [9] with unknown functions and discrete-time systems, the above method also applies. Besides, a multilayer NNs estimator was first developed in [10] to improve the compensation accuracy of model-based feedforward control terms. However, the aforementioned results do not refer to constraint.
As one of the most important factors restricting the system performance, constraints extensive exist in actual systems, such as robotic manipulator system, nonuniform gantry crane and so on. Constraint cannot be omitted, otherwise it may have an impact on the equipment and something unexpected, thus, constraint control has become a significant portion of nonlinear control. As we all know, the Barrier Lyapunov function (BLF) is the significant tool to dispose constrained problem. So far it widespread application in nonlinear systems with output constraint in [11] and full state constraints in [12,13], and its effectiveness is also verified. The NNs or FLSs is used to design adaptive controllers for nonlinear systems using neural networks with constant constraint in [5]. Nevertheless, none of the above methods mention time-varying constraints in [14]. In this article, the time-varying with full state constraints are further studied. Adaptive controllers are designed for time-varying output constraints and time-varying full-state constraints [15], respectively. However, the above backstepping recursion method ignore the feasibility conditions of virtual controllers, namely, the virtual controller is within the given constraint bounded. In [16], a new coordinate transformation is introduced to completely remove this limitation.
The constraints of the above research are all direct constraints on the states, whether full state constraints or other types of constraints. Subsequent some studies are not limited to state constraints. At present, the state transfer function is introduced for coordinate transformation in [17] indirect processing constraint such as constrain the error. Compared with the above-mentioned research methods, the advantage of this approach is not only independent of initial tracking condition, but also suitable for asymmetric time-varying constraints, which is studied in [15]. However, in any of these cases, the effect of dead-zone on constraint is omitted.
Mention nonlinear input, the most common are dead-zones, saturation, time-delay, and so on. An innovative approach is to propose a dead-zone compensation in motion control systems using adaptive fuzzy logic control. In this paper, the dead-zone nonlinear input is our focus. The existing dead-zones prevent us from getting the desired control results, and the problem caused by it is serious. For instance, if the robot servo system has nonlinear links such as friction and unknown dead zone, it not only reduces the efficiency of the control system, but also lead to the instability of the system. In recent years, the study of dead-zone has become the focus of control research. In [18], for discrete-time plants with unknown dead-zone, a new control structure with adaptive dead region inverse is put forward. To this purpose, some adaptive control method is proposed, such as neural network control and adaptive fuzzy sliding mode control. Adaptive tracking of asymmetric dead-zone input nonlinear systems with uncertain parameters is proposed. As is well-known, for dead-zone in multi-input multi-output nonlinear system, which has lower triangular structure and asymmetric structure, a new control method is proposed. To eliminate dead-zone effects, the dead-zones compensation control is implemented for precision instrument control.
In this paper, the adaptive neural network control method is proposed, it is the realization of the control target. The design of the controller is beneficial. After comprehension of above achievements, the control scheme has the following advantages.
1) Compared with previous adaptive neural network control methods, this article takes into account more complex case. The effects of delay constraints and dead-zones on system performance are considered, which is more practical in line with the needs of the actual system.
2) State transition function is introduced; the appropriate time node is selected to ensure that the states are in the constraint bounds. Namely, the initial state is out of bounds, and then in the bounds. There is no mention of delay constraints in [16], the problem of delay constraints is considered in this paper. It provides convenience for error tracking that not widely involved in the previous research for nonlinear adaptive control.
3) In this paper, based on the introduction of BLF, potential dead-zones in the system is resolved, which affects the stability of the system and increases steady state error. Manipulative know m and unknown d, the difficult problem of controller design is solved.
Consider a class of nonlinear strict-feedback systems with dead-zones as following:
{˙xi=gi(ˉxi)xi+1+fi(ˉxi),i=1,...,n−1˙xn=gn(ˉxn)D(u(t))+fn(ˉxn)y=x1 | (2.1) |
where x=[x1,…,xn]T∈R with ˉxi=[x1,…,xj]T j=1,…,n, xi∈R, D(u(t))∈R and y∈R are the state variables, the input and the output of the systems, respectively, gi(ˉxi) are unknown control coefficients and fi(ˉxi) are unknown smooth functions. xi∈(−k_ci(t),ˉkci(t)), xi is unconstrained when t∈[0,T]. Nevertheless, when t∈[T,∞), xi are within the given bound. So as to ensure the validity of the constraint, the value of T is crucial. Let −k_ci(t)<xi(t)<ˉkci(t), where k_ci(t) and ˉkci(t) are given.
Remark 1: There are many factors that affect system performance, but one of the most significant is constraint. In order to ensure that constraint is not violated in the control process, the work that needs attention in the adaptive control strategy is proposed in [19,20,21,22,23,24,25,26,27,28,29,30]. In this paper, to make sure constraints are not violated, the transfer function is introduced for coordinate transformation. Constraints appear some time later, the system stability is improved.
The delay constraint means that the constraint occurs over a period of time, which does not have to constrain the signal all the time. By designing the appropriate controller, the signal satisfies the constraint condition after a certain time.
All of state variables in the system (2.1) are constrained in the compact set. D(u(t)) is a dead-zone defined as:
D(u(t))={mr(u(t)−br),u(t)⩾br0,−bl<u(t)<bml(u(t)+bl),u(t)⩽−bl | (2.2) |
where u(t) is the dead-zone input, mr and ml respectively stand for dead-zone right slope and left slope, br and bl represent the right and left cut point of the input nonlinearity. The detailed structure diagram of dead zone is given in Figure 1.
The aforementioned model can be transformed into the following form:
D(u(t))=m(t)u(t)+b(t) | (2.3) |
where
m(t)={mr,u(t)>0ml,u(t)⩽0 |
and
b(t)={−mrbr,u(t)⩾br−m(t)u(t),−bl<u(t)<brmlbl,u(t)⩽−bl |
Assumption 1. The function m(t) is known, b(t) is unknown and its up bound is ˉb(t), namely, |b(t)|⩽ˉb(t).
The task is to design an adaptive controller u, such that the system output y tracks a desired trajectory yd(t). All the signals in the closed-loop system are bounded, meanwhile the full state constraints are not violated. It holds that −y_d(t)⩽yd(t)⩽ˉyd(t), where y_d(t) and ˉyd(t) are continuous positive functions, with k_c1(t)⩾y_d(t) and ˉkc1(t)>ˉyd(t).
In this paper, on account of radial basis function neural networks (RBFNNs) approximate ability, it is chosen to approximate unknown and continuous function.
Consider a continuous function h(z): Rq→R, the following form can be obtained:
hnn(z)=θ∗TS(z) | (3.1) |
where the input variable z∈Ωz⊂Rq, desired weight matrix θ∗=[θ1,θ2,…θl]T∈Rl, l>1 is the NN node number. In addition, S(z)=[s1(z),…,sl(z)]T, it is often expressed by Gaussian function, which has the following form:
si(z)=exp[−(z−μi)T(z−μi)η2i],i=1,2,…,l |
where μi=[μi1,μi2,…,μiq] is the center of NNs and ηi is the width of the Gaussian function.
According to the character of NNs, with regard to any continuous unknown function, it can be represented as
h(z)=θ∗TS(z)+ε(z) | (3.2) |
where ε(z) is alluded to as the least approximate error and |ε(z)|⩽ε with ε>0 for any z∈Ωz. The weight matrix θ∗ has the following representation
θ∗≜argminθ∈Rl{supZ∈Ωz|h(z)−θTS(z)|} |
Assumption 2. The function gi(ˉxi) is unknown, time-varying, and bounded away from zero, respectively. Its upper bound and lower bound are ˉgi and g_i, They are also unknown continuous function, such that 0<g_i⩽|gi(ˉxi)|⩽ˉgi, ∀ˉxi∈Ω⊂Rn.
A new asymmetric Barrier Lyapunov function is introduced
V=ζ2(t)(F1(t)+ζ(t))(F2(t)−ζ(t)) | (3.3) |
where F1(t) and F2(t) are positive functions, ζ(t) will be defined later. Note that V is valid in the interval −F1(t)<ζ(t)<F2(t).
Then, the transfer function is introduced to better constrain the states. The function is defined as
ζi(t)={0,τ(t)zi(t),zi(t),t=00<t<T(i=1,...,n)t⩾T | (3.4) |
where
τ(t)={1−(T−tT)n+2,0⩽t<T1,t⩾T | (3.5) |
The effect of (3.4) is to solve the problem of uncertain initial conditions. In the meantime, τ(t) is a continuous and differentiable function.
Remark 2: The T is a time node. There exist two crucial features for τ(t), τ(0)=0 and τ(t)=1 for t⩾T. These two properties have important applications in the conversion of initial values, that is to transform a non-zero initial value into a zero initial value. In the end, their values will converge to a finite bounded.
In the following study, adaptive control method and backstepping recursive are used to design virtual controllers αi, actual controller u and adaptive laws ˆwi. Adaptive control process has a total of n steps, the coordinate transformation is introduced as
zi=xi−αi−1(i=2,...,n) | (4.1) |
In the step 1, virtual controller α1 and adaptive law ˆw1 are defined as follows:
α1=−k1z1−ψ1−ˆw1P1 | (4.2) |
˙ˆw1=λ1G1ζ1τP1−δ1ˆw1 | (4.3) |
where k1 is a positive constant, δ1>0 is a constant, λ1>0 is a constant, ˆw1 stands for adaptive parameter. In addition, ψ1, P1, and G1 will be defined later.
In the step i(2⩽i⩽n−1), virtual controller αi and adaptive law ˆwi are defined as follows:
αi=−kizi−ψi−ˆwiPi | (4.4) |
˙ˆwi=λiGiζiτPi−δiˆwi | (4.5) |
where ki is a positive constant, δi>0 is a constant, λi>0 is a constant, ˆwi stands for adaptive parameter. In addition, ψi, Pi, and Gi will be defined later.
In the last step, actual controller u and adaptive law for ˆwn are defined as follows:
u=1m(−knzn−ψn−ˆwnPn) | (4.6) |
˙ˆwn=λnGnζnτPn−δnˆwn | (4.7) |
where kn is a positive constant, δn>0 is a constant, λn>0 is a constant, ˆwn stands for adaptive parameter. In addition, ψn, Pn, and Gn will be defined later.
Let us consider tracking error z1=x1−yd. From the first Eq (2.1), we get the derivation of z1
˙z1=g1x2+f1−˙yd=g1(z2+α1)+f1−˙yd | (4.8) |
From (3.4), we obtain
˙ζ1=˙τz1+τg1(z2+α1)+τ(f1−˙yd)=g1τα1+g1ζ2+˙τz1+τ(f1−˙yd) |
Remark 3: We just think about 0<t<T, so let ζi(t)=τ(t)zi(t). States are constrained indirectly through constraints on transition functions, this will be discussed below.
From zi=xi−αi−1(i=1,...,n), then we get
˙zi=gixi+1+fi−˙αi−1=gixi+1+fi−i−1∑j=1∂αi−1∂xj(gjxj+1+fj)−Δαi−1=gi(zi+1+αi)+fi−i−1∑j=1∂αi−1∂xj(gjxj+1+fj)−Δαi−1 | (4.9) |
where
˙αi−1=i−1∑j=1∂αi−1∂xj(gjxj+1+fj)+Δαi−1 | (4.10) |
with
Δαi−1=∑i−1j=0∂αi−1∂y(j)dy(j+1)d+∑i−1j=1∑i−jk=0∂αi−1∂F(k)j2F(k+1)j2+∑i−1j=0∂αi−1∂τ(j)τ(j+1)+∑i−1j=1∑i−jk=0∂αi−1∂F(k)j1F(k+1)j1 |
From (3.4), we obtain the following dynamics
˙ζi=˙τzi+τgi(zi+1+αi)+τ(fi−i−1∑j=1∂αi−1∂xj(gjxj+1+fj)−Δαi−1)=˙τzi+giζi+1+giταi+τ(fi−i−1∑j=1∂αi−1∂xj(gjxj+1+fj)−Δαi−1) |
Substituting (2.3) into zn=xn−αn−1, one deduces that
˙zn=gn(mu+b)+fn−n−1∑j=1∂αn−1∂xj(gjxj+1+fj)−Δαn−1 | (4.11) |
where
˙αn−1=n−1∑j=1∂αn−1∂xj(gjxj+1+fj)+Δαn−1 | (4.12) |
with
Δαn−1=∑n−1j=0∂αn−1∂y(j)dy(j+1)d+∑n−1j=1∑n−jk=0∂αn−1∂F(k)j2F(k+1)j2+∑n−1j=1∑n−jk=0∂αn−1∂F(k)j1F(k+1)j1+∑n−1j=0∂αn−1∂τ(j)τ(j+1) |
then, we obtain the following dynamics
˙ζn=˙τzn+τ(fn−n−1∑j=1∂αn−1∂xj(gjxj+1+fj)−Δαn−1)+τgn(mu+b) |
Please see the appendix for the specific derivation process
Theorem 1: Consider a class of nonlinear system (2.1) with a dead-zone (2.2) under Assumptions 1–2, virtual controllers are designed in (4.2) and (4.4), and the actual controller is designed in (4.6). Meanwhile the adaption laws are constructed in (4.3), (4.5) and (4.7). If the design parameters are chosen appropriately, it ensures that all signals in the closed-loop system are UUB and bounded. The system output y tracks a desired trajectory yd(t).
Proof. It is obvious that ˜wjˆwj=˜wj(wj−˜wj)≤−˜w2j2+w2j2 we can derive that
2Fj1Fj2−Fj1ξj+Fj2ξj⩾Fj1Fj2−Fj1ξj+Fj2ξj−ξ2j=(Fj1+ξj)(Fj2−ξj)>0 | (4.13) |
Then, we get
Gjξ2j=2Fj1Fj2−Fj1ξj+Fj2ξj[(Fj1+ξj)(Fj2−ξj)]2ξ2j⩾ξ2j(Fj1+ξj)(Fj2−ξj) | (4.14) |
2Fj1Fj2−Fj1ξj+Fj2ξj/(Fj1+ξj)(Fj2−ξj)⩾1 | (4.15) |
Hence, we have the following inequality:
˙Vn⩽−n∑j=1kjg_jξ2j(Fj1+ξj)(Fj2−ξj)−n∑j=1g_jδj2λj˜w2j+n∑j=1g_jδj2λjw2j+n∑j=1Ξj⩽−ρVn+c | (4.16) |
where ρ=min{kjg_j,δj}>0, c=n∑j=1g_jδj2λjw2j+n∑j=1Ξj
Besides, from (4.16), we get
0⩽Vn(t)⩽c/cρρ+(Vn(0)−c/cρρ)e−ρt | (4.17) |
Hence, we obtain that Vn(t)∈l∞, it implies that ˆw1∈l∞ and Vi0(t)∈l∞. The upper of ζi(t) is −Fi1(t) and lower is Fi2(t), so −Fi1(t)<ζi(t)<Fi2(t). Based on the shifting function τ(t), when t∈[0,T), τ(t) is strictly increasing. And τ(0)=0, when and only when t=0, then, ζi(0)=τ(0)zi(0)=0. We get −Fi1(0)<ζi(0)<Fi2(0). When 0<t<T, τ(t) is not zero. From (4.8), zi(t)=ζi(t)/τ(t), zi(t) is bounded because ζi(t) is bounded. When t⩾T, τ(t)=1, then we can get zi(t)=ζi(t), so −Fi1(t)<zi(t)<Fi2(t). In conclusion, zi(t) is bounded anyway. According to the definition of F11 and F12, thus F11, F12, ˙F11 and ˙F12 are bounded. From z1=x1−yd, z1 is bounded and x1 is also bounded. Because F11, F12, ˙F11, ˙F12, ˆw1, yd, ˙yd, τ and ˙τ are bounded, it follows that virtual controller α1 and adaptive law ˙ˆw1 are bounded. Then follow the definition of F21 and F22, thus F21, F22, ˙F21 and ˙F22 are bounded. From z2=x2−α1, z2 is bounded and x2 is also bounded. In the similar way, α2 and ˙ˆw2 can also be bounded. Continue this derivation, we can obtain that xi(i=3,⋯,n), αi(i=3,⋯,n−1), ˙ˆwi(i=3,⋯,n) and control input u are bounded. In a word, all signals in closed-loop systems are bounded. The proof is completed.
In this paper, to prove the effectiveness of this method, the simulation experiment is given. Consider the following nonlinear systems
{˙x1=x2˙x2=−mglsin(x1)2M−Bx2My=x1+D(u(t))M | (5.1) |
where y and u are the outputs and inputs of the system. Meanwhile, D(u(t)) is the output of the dead zone, described as
D(u(t))={0.2(u−0.3),u>0.30,−0.3⩽u⩽0.30.3(u+0.3),u<−0.3 | (5.2) |
and the states x1 and x2 are constrained by ˉkc1(t)<x1(t)<−k_c1(t) and ˉkc2(t)<x2(t)<−k_c2(t) with ˉkc1(t)=0.07+0.01sin(2t), k_c1(t)=0.11+0.05sin(4t), ˉkc2(t)=0.3sin(3t)+0.6, k_c2(t)=0.4sin(2t)+0.72. The ideal trajectory yd is chosen as yd=0.05cos(6t). The initial states are chosen as x1(0)=0.16, x2(0)=0.001, ˆw1(0)=0.6, ˆw2(0)=0.279. The controllers and adaptive laws of simulation system are chosen as follow:
α1=−k1z1−G1ζ1τ1−ˆw1G1ζ1τST1(z1)S1(z1)u=1m(−k2z2−G2ζ2τ−ˆw2G2ζ2τST2(z2)S2(z2))˙ˆw1=λ1G1ζ1τP1−δ1ˆw1˙ˆw2=λ2G2ζ2τP2−δ2ˆw2 |
In the simulation, the first NN includes 10 nodes with the center μ1 evenly spaced on [−1,1]×[−1,1]×[−1,1] with width η1=1, the second NN includes 16 nodes with the center μ2 evenly spaced on [−3,3]×[−3,3]×[−3,3]×[−3,3]×[−3,3] with width η2=1. The parameters in the simulation system are given as m=1kg, g=9.8m/s2, l=1m, M=0.5kg.m2, B=1N.m.s. The control parameters are selected as k1=29, k2=44, λ1=0.01, λ2=0.01, δ1=0.1, δ2=0.5. Delay constraint time T=1, x1 and x2 are out of bounds, when t∈(0,1) and while T⩾1, x1 and x2 are expected completely within the bounds. The selection of the delay constraint time is based on the minimum tracking error and the best tracking performance. Besides, according to the desired trajectory, we obtain that F11=0.01sin(2t)−1.93 and F12=0.05sin(4t)−1.89, F21=0.222+0.1sin(5t) and F22=0.25+0.05sin(10t).
The simulation results are given in Figures 2–7. Figure 2 shows the trajectory yd, output y and constrained intervals with a good tracking performance. Figure 3 shows the trajectory of error z1. The trajectory of the dead-zone D(u) is given in Figure 4. The trajectory of state x2 is displayed in Figure 5. The trajectory of error z2 and adaptive laws are shown in Figure 6 and Figure 7, respectively. Simulation results prove that all signals are bounded.
An adaptive neural network tracking control method is introduced for the strict feedback nonlinear systems with unknown dead-zones and full state constraints. The parameters of the dead-zones are unknown but bounded. Combining backsteping technique with neural network, it ensures that the state constraints are not violated. The feasibility of the control algorithm is proved. In the meantime, all signals in the closed-loop system are UUB. In this paper, delay constraint and BLF are combined. In other words, the constraint occurs after a period of time, it is not constrained from the beginning. Furthermore, the tracking error converges to a small area away from zero. In the end, simulation results verify the effectiveness of the design. We will further investigate the control performance by setting different delay times such as time-varying delay, which will enrich the applied range of the proposed control method. Besides, since the reliability is an interested topic and has been well discussed in [23,24,25,26,27], it will be taken into account in our future work.
Step 1: In order to achieve the desired control objective, the following Barrier Lyapunov function is chosen
V10=ζ21(t)(F11(t)+ζ1(t))(F12(t)−ζ1(t)) |
where F11(t) and F12(t) are time varying continuous functions. Its definition will be given later. For ζ1(t), its scope is F11(t)<ζ1(t)<F12(t). The time-varying functions F11(t) and F12(t) are chosen such that
F11(t)=k_c1(t)−ny_d,F12(t)=ˉkc1(t)−nˉyd |
where n is a positive constant.
Consider the Barrier Lyapunov function as
V=V10+g_12λ1˜w21 | (7.1) |
where λ1>0 is a constant, w1=maxk∈K{‖θ∗K1‖2}, ˜w1=w1−ˆw1 stands for the estimation error, with ˆw1 being the estimation of w1. Based on (3.4), we get the time derivative of V1
˙V1=G1ζ1(˙ζ1+N1ζ1)−g_1λ1˜w1˙ˆw1=G1ζ1[g1τα1+g1ζ2+˙τz1+τ(f1−˙yd)+ζ1N1]−g_1λ1˜w1˙ˆw1 | (7.2) |
where
G1=2F11F12−F11ζ1+F12ζ1[(F11+ζ1)(F12−ζ1)]2 |
and
N1=−˙F11F12−F11˙F12+(˙F11−˙F12)ζ12F11F12−F11ζ1+F12ζ1. |
In this way, the design of controller and adaptive law are simplified.
Combining the Assumption 2, on the basis of Young’s inequality, we get
G1g1ζ1ζ2⩽g_2G21ζ21ζ22+g214g_2 | (7.3) |
G1ζ1˙τz1⩽g_1G21ζ21˙τ2z21+14g_1 | (7.4) |
G1ζ21N1⩽g_1G21ζ41N21+14g_1 | (7.5) |
Substituting (7.3), (7.4) and (7.5) into (7.2) leads to
˙V1⩽G1ζ1τ(g1α1+g_1G1˙τ2z31+g_1G1ζ21N21z1+f1−˙yd)+g_2G21ζ21ζ22−g_1λ1˜w1˙ˆw1+g214g_2+12g_1 | (7.6) |
Let
h1(z1)=g_1G1˙τ2z31+g_1G1ζ21N21z1+f1−˙yd | (7.7) |
The unknown continuous function h1(z1) can be approximated by an RBF NN as
h1(z1)=θ1∗TS1(z1)+ε1(z1) | (7.8) |
Substituting (7.7) and (7.8) into (7.6) and using Young’s inequality, one gets
G1ζ1τε1(z1)⩽g_1G21ζ21τ2+ε214g_1 | (7.9) |
G1ζ1τθ1∗TS1(z1)⩽g_1G21ζ21τ2w1ST1(z1)S1(z1)+14g_1 | (7.10) |
Then, we obtain
˙V1⩽G1ζ1τ(g1α1+g_1ψ1+g_1w1P1)+g_2G12ζ21ζ22−g_1λ1˜w1˙ˆw1+Ξ1 | (7.11) |
with
P1=G1ζ1τST1(z1)S1(z1) |
ψ1=G1ζ1τ |
Ξ1=g214g_2+34g_1+ε214g_1 |
From Assumption 2, we get
g1G1ζ1τα1=−k1g1G1ζ12−g1G1ζ1τψ1−g1G1ζ1τˆw1P1⩽−k1g_1G1ζ12−g_1G1ζ1τψ1−g_1G1ζ1τˆw1P1 |
then
˙V1⩽−k1g_1G1ζ21+g_2G21ζ21ζ22+g_1λ1δ1˜w1ˆw1+Ξ1 | (7.12) |
Step i (i=2,...,n−1): The following Barrier Lyapunov function is chosen as
Vi0=ζ2i(t)(Fi1(t)+ζi(t))(Fi2(t)−ζi(t)) |
Consider the BLF as
Vi=Vi−1+Vi0+g_i2λi˜w2i | (7.13) |
where λi>0 is a constant, wi=maxk∈K{‖θ∗Ki‖2}, ˜wi=wi−ˆwi stands for the estimation error, with ˆwi being the estimating of wi, Based on (3.4), we get the time derivative of Vi
˙Vi⩽−i−1∑j=1kjg_jGjζ2j+g_iG2i−1ζ2i−1ζ2i+i−1∑j=1g_jλjδj˜wjˆwj+i−1∑j=1Ξj+Giζi(˙ζi+Niζi)−g_iλi˜wi˙ˆwi⩽−i−1∑j=1kjg_jGjζ2j+i−1∑j=1g_jλjδj˜wjˆwj+i−1∑j=1Ξj−g_iλi˜wi˙ˆwi+Giζi(Niζi+giταi+giζi+1+˙τzi+g_iG2i−1ζ2i−1ζiGi)+Giζiτ(fi−i−1∑j=1∂αi−1∂xj(gjxj+1+fj)−Δαi−1) | (7.14) |
where
Gi=2Fi1Fi2−Fi1ζi+Fi2ζi[(Fi1+ζi)(Fi2−ζi)]2 |
and
Ni=−˙Fi1Fi2−Fi1˙Fi2+(˙Fi1−˙Fi2)ζi2Fi1Fi2−Fi1ζi+Fi2ζi |
According to the Young’s inequality, it yields
Gigiζiζi+1⩽g_i+1G2iζ2iζ2i+1+g2i4g_i+1 | (7.15) |
Giζi˙τzi⩽g_iG2iζ2i˙τ2z2i+14g_i | (7.16) |
Giζ2iNi⩽g_iG2iζ4iN2i+14g_i | (7.17) |
Let hi(zi)=fi−i−1∑j=1∂αi−1∂xj(gjxj+1+fj)−Δαi−1+g_iGi˙τ2z3i+g_iGiζ2iN2izi+g_iG2i−1ζ2i−1ziGi | (7.18) |
The unknown continuous function hi(zi) can be approximated by an RBF NN as
hi(zi)=θi∗TSi(zi)+εi(zi) | (7.19) |
Substituting (7.18) and (7.19) into (7.14) and using Young’s inequality, one gets
Giζiτεi⩽g_iG2iζ2iτ2+ε2i4g_i | (7.20) |
Giζiτθi∗TSi(zi)⩽g_iG2iζ2iτ2wiSTi(zi)Si(zi)+14g_i | (7.21) |
Then, we obtain
˙Vi⩽−i−1∑j=1kjg_jGjζ2j+i−1∑j=1g_jλjδj˜wjˆwj+g_i+1G2iζ2iζ2i+1+Giζiτ(giαi+g_iψi+g_iwiPi)−g_iλi˜wi˙ˆwi+i−1∑j=1Ξj | (7.22) |
with
Pi=GiζiτSTi(zi)Si(zi) |
ψi=Giζiτ |
Ξi=g2i4g_i+1+34g_i+ε2i4g_i |
Let
giGiζiταi=−gikiGiζi2−giGiζiτψi−giGiζiτˆwiPi⩽g_ikiGiζi2−g_iGiζiτψi−g_iGiζiτˆwiPi |
Then, we get
˙Vi⩽−i∑j=1kjg_jGjζ2j+i∑j=1g_jλjδj˜wjˆwj+i∑j=1Ξj+g_i+1G2iζ2iζ2i+1 | (7.23) |
Step n: Because ˙Vn is similar to ˙Vi, we can figure out the following formula
˙Vn⩽Gnζn(g_nG2n−1ζ2n−1ζnGn+τgn(mu+b)+˙τzn)+GnNnζn2−g_nλn˜wn˙ˆwn+n−1∑j=1Ξj+n−1∑j=1g_jλjδj˜wjˆwj+Gnζnτ(fn−n−1∑j=1∂αn−1∂xj(gjxj+1+fj)−Δαn−1)−n−1∑j=1kjg_jGjζ2j | (7.24) |
Since b is bounded, one obtains b/m⩽ˉb/m≜η where η is a constant. Using Young’s inequality get the following formula:
Gnξnτgn(mu+b)=Gnξnτgnmu+Gnξnτgnmbm⩽Gnξnτgnmu+G2nξ2nτ2m+mη2g2n4 | (7.25) |
According to the Young’s inequality, it causes
Gnζn˙τzn⩽g_nG2nζ2n˙τ2z2n+14g_n | (7.26) |
Gnζ2nNn⩽g_nG2nζ4nN2n+14g_n | (7.27) |
Denote
hn(zn)=fn−n−1∑j=1∂αn−1∂xj(gjxj+1+fj)−Δαn−1+Gnζnτm+g_nGn˙τ2z3n+g_nGnζ2nN2nzn+g_nG2n−1ζ2n−1znGn | (7.28) |
The unknown continuous function hn(zn) can be approximated by an RBF NN as
hn(zn)=θn∗TSn(zn)+εn(zn) | (7.29) |
Substituting (7.28) and (7.29) into Error! Reference source not found., and using the Young’s inequality, it yields
Gnζnτεn⩽g_nG2nζ2nτ2+ε2n4g_n | (7.30) |
Gnζnτθn∗TSn(zn)⩽g_nG2nζ2nτ2wnSTn(zn)Sn(zn)+14g_n | (7.31) |
Then, using the above inequalities we get
˙Vn⩽−n−1∑j=1kjg_jGjζ2j+n−1∑j=1g_jλjδj˜wjˆwj+n−1∑j=1Ξj+Gnζnτ(gnmu+g_nψn+g_nwnPn)−g_nλn˜wn˙ˆwn | (7.32) |
where
Pn=GnζnτSTn(zn)Sn(zn) |
ψn=Gnζnτ |
Ξn=34g_n+ε2n4g_n+mη2g2n4 |
with
Gnζnτgnmu=−kngnGnζn2−gnGnζnτψn−gnGnζnτˆwnPn⩽−kng_nGnζn2−g_nGnζnτψn−g_nGnζnτˆwnPn |
Then, we get
˙Vn⩽−n∑j=1kjg_jGjζ2j+n∑j=1g_jλjδj˜wjˆwj+n∑j=1Ξj | (7.33) |
All authors declare no conflicts of interest.
[1] | S. C. Tong, X. Min, Y. X. Li, Observer-based adaptive fuzzy tracking control for strict-feedback nonlinear systems with unknown control gain functions, IEEE Trans. Cybernetics, DOI:10.1109/TCYB.2020.2977175, 2020. |
[2] | M. Krstic, I. Kanellakopoulos, P. V. Kokotovic, Nonlinear and Adaptive Control Design. Hoboken, NJ, USA: Wiley, 1995. |
[3] |
D. P. Li, Y. J. Liu, S. C. Tong, et al. Neural networks-based adaptive control for nonlinear state constrained systems with input delay, IEEE Trans. Cybernetics, 49 (2019), 1249-1258. doi: 10.1109/TCYB.2018.2799683
![]() |
[4] | C. L. P. Chen, Y. H. Pao, An integration of neural network and rule-based systems for design and planning of mechanical assemblies, IEEE Trans. Syst. Man, Cybernetics, 23 (1993), 1359-1371. |
[5] |
D. P. Li, L. Liu, Y. J. Liu, et al. Adaptive NN control without feasibility conditions for nonlinear state constrained stochastic systems with unknown time delays, IEEE Trans. Cybernetics, 49 (2019), 4485-4494. doi: 10.1109/TCYB.2019.2903869
![]() |
[6] |
S. C. Tong, Y. Li, Y. M. Li, et al. Observer-based adaptive fuzzy backstepping control for a class of stochastic nonlinear strict-feedback systems, IEEE Trans. Syst., Man Cybernetics, Part B, 41 (2011), 1693-1704. doi: 10.1109/TSMCB.2011.2159264
![]() |
[7] |
Y. J. Liu, Q. Zeng, S. C. Tong, et al. Adaptive neural network control for active suspension systems with time-varying vertical displacement and speed constraints, IEEE Trans. Ind. Electron., 66 (2019), 9458-9466. doi: 10.1109/TIE.2019.2893847
![]() |
[8] | L. Liu, Y. J. Liu, S. C. Tong, et al. Integral Barrier Lyapunov function based adaptive fuzzy control for switched nonlinear systems, Sci. China Infor. Sci., DOI: 10.1007/s11432-019-2714-7, 2019. |
[9] |
S. C. Tong, Y. M. Li, G. Feng, et al. Observer-based adaptive fuzzy backstepping dynamic surface control for a class of MIMO nonlinear systems, IEEE Trans. Syst., Man Cybernetics, Part B, 41 (2011), 1124-1135. doi: 10.1109/TSMCB.2011.2108283
![]() |
[10] | Z. Yao, J. Yao, W. Sun, Adaptive RISE control of hydraulic systems with multilayer neural networks. IEEE Trans. Ind. Electron., 66 (2018), 8638-8647. |
[11] |
Z. Liu, G. Lai, Y. Zhang, et al. Adaptive neural output feedback control of output-constrained nonlinear systems with unknown output nonlinearity, IEEE Trans. Neural Networks Learn. Syst., 26 (2015), 1789-1802. doi: 10.1109/TNNLS.2015.2420661
![]() |
[12] |
T. Zhang, M. Xia, Y. Yi, Adaptive neural dynamic surface control of strict-feedback nonlinear systems with full state constraints and unmodeled dynamics, Automatica, 81 (2017), 232-239. doi: 10.1016/j.automatica.2017.03.033
![]() |
[13] |
T. T. Gao, Y. J. Liu, L Liu, et al. Adaptive neural network-based control for a class of nonlinear pure-feedback systems with time-varying full state constraints, IEEE/CAA J. Automatica Sinica, 5 (2018), 923-933. doi: 10.1109/JAS.2018.7511195
![]() |
[14] | D. P. Li, L. Liu, Y. J. Liu, et al. Fuzzy approximation-based adaptive control of nonlinear uncertain state constrained systems with time-varying delays, IEEE Trans. Fuzzy Systems, DOI: 10.1109/TFUZZ.2019.2919490, 2019. |
[15] |
Y. D. Song, S. Zhou, Tracking control of uncertain nonlinear systems with deferred asymmetric time-varying full state constraints, Automatica, 98 (2018), 314-322. doi: 10.1016/j.automatica.2018.09.032
![]() |
[16] | K. Zhao, Y. D. Song, Removing the feasibility conditions imposed on tracking control designs for state-constrained strict-feedback systems, IEEE Trans. Automatic Control, 64 (2018), 1265-1272. |
[17] |
Y. Li, C. Yang, S. S. Ge, et al. Adaptive output feedback NN control of a class of discrete-time MIMO nonlinear systems with unknown control directions, IEEE Trans. Syst., Man Cybernetics, Part B (Cybernetics), 41 (2011), 507-517. doi: 10.1109/TSMCB.2010.2065223
![]() |
[18] |
M. Roopaei, M. Z. Jahromi, R. John, et al. Unknown nonlinear chaotic gyros synchronization using adaptive fuzzy sliding mode control with unknown dead-zone input, Comm. Nonlinear Sci. Numer. Simul., 15 (2010), 2536-2545. doi: 10.1016/j.cnsns.2009.09.022
![]() |
[19] | L. Liu, Y. J. Liu, D. P. Li, et al. Barrier Lyapunov function based adaptive fuzzy FTC for switched systems and its applications to resistance inductance capacitance circuit system, IEEE Trans. Cybern., DOI: 10.1109/TCYB.2019. 2931770, 2019. |
[20] |
C. L. P. Chen, L. Liu, L. Chen, et al. Weighted couple sparse representation with classified regularization for impulse noise removal, IEEE Trans. Image Process., 24 (2015), 4014-4026. doi: 10.1109/TIP.2015.2456432
![]() |
[21] |
S. C. Tong, C. L. Liu, Y. M. Li, Fuzzy-adaptive decentralized output-feedback control for large-scale nonlinear systems with dynamical uncertainties, IEEE Trans. Fuzzy Syst., 18 (2010), 845-861. doi: 10.1109/TFUZZ.2010.2050326
![]() |
[22] |
D. Yang, X. D. Li, J. L. Qiu, Output tracking control of delayed switched systems via state-dependent switching and dynamic output feedback, Nonlinear Anal.: Hybrid Syst., 32 (2019), 294-305. doi: 10.1016/j.nahs.2019.01.006
![]() |
[23] | S. J. Dyke, B. F. Spencer, P. Quast, et al. Role of control-structure interaction in protective system, Design. J. Eng. Mech., 121 (1995), 322-338. |
[24] | A. P. Darby, M. S. Williams, A. Blakeborough, Stability and delay compensation for real-time substructure testing. J. Eng. Mech., 128 (2002), 1276-1284. |
[25] | X. D. Li, X. Y. Yang, T. W. Huang, Persistence of delayed cooperative models: Impulsive control method, Appl. Math. Comput., 342 (2019), 130-146. |
[26] |
Y. Qian, G. Ou, A. Maghareh, et al. Parametric identification of a servohydraulic actuator for real-time hybrid simulation, Mech. Syst. Signal Process., 48 (2014), 260-273. doi: 10.1016/j.ymssp.2014.03.001
![]() |
[27] | Y. Ouyang, W. Shi, J. Shan, et al. Backstepping adaptive control for realtime hybrid simulation including servo-hydraulic dynamics. Mech. Syst. Signal Process., 130 (2019), 732-754. |
[28] |
K. P. Tee, B. Ren, S. S. Ge, Control of nonlinear systems with time-varying output constraints, Automatica, 47 (2011), 2511-2516. doi: 10.1016/j.automatica.2011.08.044
![]() |
[29] |
T. M. Athira1, S. J. John, H. Garg, A novel entropy measure of Pythagorean fuzzy soft sets, AIMS Mathematics, 5 (2020), 1050-1061. doi: 10.3934/math.20200073
![]() |
[30] | T. Godoy, A. Guerin, Multiple finite-energy positive weak solutions to singular elliptic problems with a parameter. AIMS Math., 3 (2018), 233-252. |
1. | Yanxin Li, Shangkun Liu, Jia Li, Weimin Zheng, Congestion tracking control of multi-bottleneck TCP networks with input-saturation and dead-zone, 2024, 9, 2473-6988, 10935, 10.3934/math.2024535 | |
2. | Xinyu Lei, Fazhan Tao, Nan Wang, Zhumu Fu, Haoxiang Ma, Finite‐time prescribed performance adaptive fuzzy output feedback control for stochastic nonlinear systems with dead‐zone input, 2023, 1049-8923, 10.1002/rnc.7116 |