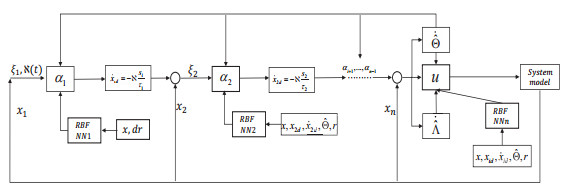
In this paper, the control problem of prescribed-time adaptive neural stabilization for a class of non-strict feedback stochastic high-order nonlinear systems with dynamic uncertainty and unknown time-varying powers is discussed. The parameter separation technique, dynamic surface control technique, and dynamic signals were used to eradicate the influences of unknown time-varying powers together with state and input unmodeled dynamics, and to mitigate the computational intricacy of the backstepping. In a non-strict feedback framework, the radial basis function neural networks (RBFNNs) and Young's inequality were deployed to reconstruct the continuous unknown nonlinear functions. Finally, by establishing a new criterion of stochastic prescribed-time stability and introducing a proper bounded control gain function, an adaptive neural prescribed-time state-feedback controller was designed, ensuring that all signals of the closed-loop system were semi-global practical prescribed-time stable in probability. A numerical example and a practical example successfully validated the productivity and superiority of the control scheme.
Citation: Yihang Kong, Xinghui Zhang, Yaxin Huang, Ancai Zhang, Jianlong Qiu. Prescribed-time adaptive stabilization of high-order stochastic nonlinear systems with unmodeled dynamics and time-varying powers[J]. AIMS Mathematics, 2024, 9(10): 28447-28471. doi: 10.3934/math.20241380
[1] | Bin Hang, Weiwei Deng . Finite-time adaptive prescribed performance DSC for pure feedback nonlinear systems with input quantization and unmodeled dynamics. AIMS Mathematics, 2024, 9(3): 6803-6831. doi: 10.3934/math.2024332 |
[2] | Miao Xiao, Zhe Lin, Qian Jiang, Dingcheng Yang, Xiongfeng Deng . Neural network-based adaptive finite-time tracking control for multiple inputs uncertain nonlinear systems with positive odd integer powers and unknown multiple faults. AIMS Mathematics, 2025, 10(3): 4819-4841. doi: 10.3934/math.2025221 |
[3] | Changgui Wu, Liang Zhao . Finite-time adaptive dynamic surface control for output feedback nonlinear systems with unmodeled dynamics and quantized input delays. AIMS Mathematics, 2024, 9(11): 31553-31580. doi: 10.3934/math.20241518 |
[4] | Yabo Zhao, Huaiqin Wu . Fixed/Prescribed stability criterions of stochastic system with time-delay. AIMS Mathematics, 2024, 9(6): 14425-14453. doi: 10.3934/math.2024701 |
[5] | Yanghe Cao, Junsheng Zhao, Zongyao Sun . State feedback stabilization problem of stochastic high-order and low-order nonlinear systems with time-delay. AIMS Mathematics, 2023, 8(2): 3185-3203. doi: 10.3934/math.2023163 |
[6] | Zaihua Xu, Jian Li . Adaptive prescribed performance control for wave equations with dynamic boundary and multiple parametric uncertainties. AIMS Mathematics, 2024, 9(2): 3019-3034. doi: 10.3934/math.2024148 |
[7] | Liandi Fang, Li Ma, Shihong Ding . Finite-time fuzzy output-feedback control for p-norm stochastic nonlinear systems with output constraints. AIMS Mathematics, 2021, 6(3): 2244-2267. doi: 10.3934/math.2021136 |
[8] | Wajdi Kallel, Noura Allugmani . Finite-time stabilization of stochastic systems with varying parameters. AIMS Mathematics, 2023, 8(8): 17687-17701. doi: 10.3934/math.2023903 |
[9] | Yakufu Kasimu, Gulijiamali Maimaitiaili . Non-fragile H∞ filter design for uncertain neutral Markovian jump systems with time-varying delays. AIMS Mathematics, 2024, 9(6): 15559-15583. doi: 10.3934/math.2024752 |
[10] | Kunting Yu, Yongming Li . Adaptive fuzzy control for nonlinear systems with sampled data and time-varying input delay. AIMS Mathematics, 2020, 5(3): 2307-2325. doi: 10.3934/math.2020153 |
In this paper, the control problem of prescribed-time adaptive neural stabilization for a class of non-strict feedback stochastic high-order nonlinear systems with dynamic uncertainty and unknown time-varying powers is discussed. The parameter separation technique, dynamic surface control technique, and dynamic signals were used to eradicate the influences of unknown time-varying powers together with state and input unmodeled dynamics, and to mitigate the computational intricacy of the backstepping. In a non-strict feedback framework, the radial basis function neural networks (RBFNNs) and Young's inequality were deployed to reconstruct the continuous unknown nonlinear functions. Finally, by establishing a new criterion of stochastic prescribed-time stability and introducing a proper bounded control gain function, an adaptive neural prescribed-time state-feedback controller was designed, ensuring that all signals of the closed-loop system were semi-global practical prescribed-time stable in probability. A numerical example and a practical example successfully validated the productivity and superiority of the control scheme.
This study presents a stochastic nonlinear plant in the form of non-strict feedback, as described below:
dz=q(z,x)dt,dxi=(hi(ˉxi)[xi+1]pi(t)+fi(x)+Γi(z,x))dt+g⊤i(x)dω,dxn=(hn(x)ν+fn(x)+Γn(z,x))dt+g⊤n(x)dω,x(0)=x0,i=1,…,n−1, | (1.1) |
where x=[x1,…,xn]⊤∈Rn, ν∈R, and x0∈R are the system state, input, and initial value, respectively. z∈Rn0 is the stochastic state unmodeled dynamics portion. System power pi(t): R+→R+ is defined as an unknown time-varying function. It is worth mentioning that when pi(t)≥1, we call system (1.1) a high-order system. We denote [⋅]p=sign(⋅)|⋅|p for any real number p>0. ω(t) represents an m-dimensional standard Wiener process. Γi(⋅) represents nonlinear dynamic disturbances. q(⋅), hi(⋅), Γi(⋅), fi(⋅), and gi(⋅) indicate unknown locally Lipschitz continuous functions. The input unmodeled dynamics subsystem is listed as
{dˉz=aΓ(ˉz)+bΓ[u]pn(t),ν=cΓ(ˉz)+dΓ[u]pn(t), | (1.2) |
where ˉz∈Rn1 denotes the input unmodeled dynamics, aΓ indicates a globally Lipschitz smooth function vector, bΓ indicates a constant vector, cΓ(ˉz) indicates a smooth function, dΓ indicates a constant, and u∈R indicates the system input.
Many practical applications require severe time response constraints due to security reasons, or simply to improve productivity. Hence, finite-time and further fixed-time stability problems have been intensively studied, see [1,2,3,4,5,6] and the references therein. In recent decades, due to the widespread application of stochastic nonlinear control in the fields of economics and engineering, the design of stability time for their systems has become a hot topic. Some results have been achieved on the asymptotic stability [7,8,9], finite-time stability [5,11], and fixed-time stability [12,13,14] of stochastic nonlinear systems with pi(t)=1. It must be clarified that the aforementioned control strategies can only determine the upper limit of the stable time through a complex mathematical function composed of multiple control parameters. In many engineering applications, it is necessary not only to achieve the desired control performance within a specified time frame but also without being constrained by initial conditions. In this regard, [15] introduced the concept of prescribed time for normal-form nonlinear systems, which utilizes state scaling methods, allowing users to pre-determine a convergence time independent of other control parameters. Taking into account the frequent occurrence of stochastic disturbances in practical systems, [16] further applied the concept of prescribed-time stability to the inverse optimal control problem of stochastic nonlinear systems. In continuation of previous research in [16], subsequent research in [17] founded a way to ease the burden of control efforts. Additionally, a new non-scaling output-feedback control scheme was developed in [18] for stochastic nonlinear systems with or without sensor uncertainty. Taking a step further, when pi(t)=pi>1, that is, system (1.1) is a stochastic high-order nonlinear system, there poses a challenge to the control design since the presence of high powers impedes the achievement of feedback linearization or controllability through Jacobian linearization, as discussed in [19]. For such systems, the prescribed-time mean-square stability was discussed in [20], addressing both state-feedback control and parameter uncertainty issues.
However, the aforementioned research outcomes are primarily based on an assumption that the powers of system (1.1) are constants and precisely known. In real engineering applications, the powers of many systems may undergo variations influenced by factors such as engineering data and operating conditions. For instance, the power of a boiler turbine unit might be adjusted according to actual demand in [21] and nonlinear springs in unstable mechanical systems could lead to changes in dynamic characteristics in [22]. These situations need to be considered during system modeling. Furthermore, factors such as external disturbances, modeling errors, and model simplifications may negatively impact the stability of controlled systems, thereby degrading control performance. Although some scholars investigated finite-time or fixed-time control of stochastic nonlinear systems with unmodeled dynamics and dynamic disturbances, and proposed some meaningful conclusions in [23,24,25,26], these studies have not yet addressed the issue of prescribed-time control. It is worth emphasizing that the adaptive neural network/fuzzy control method excels in addressing unknown nonlinearities. For example, [27] proposed an event-triggered adaptive fuzzy control scheme that effectively addresses stochastic nonlinear time-delay systems by handling delays using the Lyapunov-Krasovskii function and simplifying uncertainty modeling with fuzzy logic, significantly reducing data transmission and communication burdens. Additionally, [28] introduced an adaptive neural network event-triggered asymptotic tracking control strategy that effectively tackles state constraints and unknown dynamics in multi-input/multi-output (MIMO) nonlinear systems, utilizing barrier Lyapunov functions and neural networks to achieve safe control and dynamic modeling, thereby enhancing control efficiency and reducing data transmission. However, as far as we are aware, there are still some challenges in directly applying this method to achieve prescribed-time stability for stochastic nonlinear systems. Thus, these facts prompt us to raise an interesting question: can we develop an adaptive neural prescribed-time controller to stabilize stochastic nonlinear systems with unmodeled dynamics and unknown time-varying powers pi(t)?
To address this critical issue, this article provides a new constructive control strategy for prescribed-time stabilization in probability. Prior to the controller design, the concept of semi-global practical prescribed-time stability in probability is first introduced. Then, dynamic signal function, normalized signals, dynamic surface control, and the parameter separation technique are used in every phase of the iterative design to solve the issue of differential explosion of the virtual control and to eliminate the effects of multiple unknown uncertainties. Radial basis function neural networks (RBFNNs) are deployed to estimate the uncertainty functions and the backstepping technique is manipulated to design the controller. Based on the bounded control gain function, the virtual controller does not tend to infinity during operation and the suggested control scheme enables the system to persist operation after a specified time, thus ensuring the performance of the closed-loop system. Compared with existing findings, this article incorporates its primary contributions as follows:
(1) The framework of system (1.1) is more prevailing. In comparison with recent literature on prescribed-time control [16,17,18,20], this is the first study of the adaptive neural prescribed-time control problem for stochastic high-order nonlinear systems with unknown time-varying powers.
(2) This paper expands upon previous research on stochastic nonlinear systems with unknown time-varying powers, as discussed in [29,30,31,32,33], by additionally considering unmodeled dynamics in both input and state. These dynamics have the potential to negatively impact control performance and potentially lead to instability in the closed-loop system. On this basis, the control algorithm avoids the repeated differentiation of the virtual controller by dynamic surface control, ensures the system signal will achieve more effective prescribed finite-time stability, and adopts the minimum learning parameter method where only one adaptive parameter needs to be adjusted dynamically to reduce the computation.
(3) Even though stochastic nonlinear systems in [16,17,18,20] can operate stably within the prescribed-time range, the control algorithm relies heavily on the unbounded gain signal Tt−T at t=T, where T is the prescribed stabilization time. This situation presents difficulties for the control system's execution. Considering that any dynamic interference can lead to the controller approaching infinity, which can harm the system response, inspired by [34], this article overcomes the shortcomings of existing prescribed-time control methods when there is multiple interference in the system, and ensures the continuous operation of the closed-loop system.
The aim of this thesis is formulated as to develop an adaptive neural prescribed-time control scheme for system (1.1), which ensures the convergence of all closed-loop system signals to a specified small region after the predetermined moment.
To ensure continuous operation of the control system, we introduce the function ℵ(t) as
ℵ(t)={μ(t),0≤t≤tp,μ(tp),t>tp, | (2.1) |
where tp indicates a specific moment in time that meets the criteria of tp<T, μ(t)=(TT−t)s, s≥1 indicates a constant, and T>0 indicates the liberally predetermined time parameter.
Assumption 1. There exist positive constants ˉp and p_ such that 1≤p_≤pi(t)≤ˉp, i=1,…,n.
Assumption 2. There exist known smooth positive functions h_i(ˉxi), ˉhi(ˉxi) such that h_i(ˉxi)≤|hi(ˉxi)|≤ˉhi(ˉxi), i=1,…,n.
In order to address the nonlinear dynamic disturbance Γi induced by the state unmodeled dynamical system dz=q(z,x)dt and the influence of the input unmodeled dynamical subsystem (1.2), some assumptions need to be outlined.
Assumption 3. The dynamic disturbance Γi of system (1.1) satisfies
|Γi(z,x)|≤ψi1(‖x‖)+ψi2(‖z‖), i=1,…,n, |
where ψi1(⋅) denotes an uncertain non-negative smooth function, and ψi2(⋅) denotes an unknown monotone increasing non-negative smooth function.
Assumption 4. The unmodeled dynamic z is exponentially input-state-practically stable, meaning that for dz=q(z,x), there exists a Lyapunov function V0(z) such that
α1(||z||)≤V0(z)≤α2(||z||),˙V0(z)≤−l0Vχ0(z)+λ(‖x‖)+l1, |
where α1(⋅), α2(⋅), and λ(⋅) are all K∞ functions, l0>0 and l1>0 represent two known constants, and χ∈(0,1).
Assumption 5. If dΓ>0 in (1.2), the following relation holds
|cΓ(ˉz)|≤b0‖ˉz‖, |
where b0 is an unknown non-negative constant.
Assumption 6. There exists a Lyapunov function Vw(ˉz) such that
H1‖ˉz‖2≤Vw(ˉz)≤H2‖ˉz‖2,∂Vw∂ˉzaΓ(ˉz)≤−2e0Vw(ˉz),‖∂Vw∂ˉz‖≤H3‖ˉz‖, |
where H1, H2, and H3 are positive constants and e0 represents a known positive constant.
Consider the stochastic nonlinear system described below:
dx=f(x)dt+g⊤(x)dω, x(0)=x0∈Rn. | (2.2) |
We list some definitions and lemmas below for the following design and analysis process.
Definition 1. For system (2.2), the stochastic differential operator L is listed as
LV(x)=∂V(x)∂xf(x)+12Tr{g(x)⊤∂2V(x)∂2xg(x)}, |
where Tr{gT∂2V∂2xg} represents the Hessian item.
Definition 2. [35] If the solution of the nonlinear stochastic system (2.2) satisfies the following conditions, the system is considered to be semi-global practical prescribed-time stable in probability.
(1) For any stochastic initial value x0, a unique solution is guaranteed.
(2) For initial values x0, there exist a positive scalar ζ and a setting time tp(ζ,x0)<T to make E{‖x(t)‖2}≤ζ, ∀t≥t0+tp<T, where T indicates a predetermined prescribed time independent of the initial value.
Lemma 1. If the continuous function R(t) satisfies
∫thR(ς)dς≤0,∀0≤h≤t, |
then R(t)≤0 for ∀t≥0.
Lemma 2. For system (2.2), if a continuously differentiable (i.e., C2), positive definite, and radially unbounded quadratic function V(x) exists along with K∞ functions ˉα1(⋅) and ˉα2(⋅), constants C>0,0<χ<1, P1>0, and P2>0, such that
ˉα1(‖x‖)≤V(x)≤ˉα2(‖x‖),LV(x)≤−Cℵ(t)Vχ(x)+ℵ(t)P1+P2, |
then system (2.2) is semi-global practical prescribed-time stable in probability, where ℵ(t) is the bounded function defined in (2.1).
Proof. First, since V(x)≥0 and LV(x)≤ℵ(t)P1+P2 hold for all x0, according to the proof of Theorem 4.1 in [1], it can be inferred that a unique strong solution of system (2.2) exists.
According to (2.3), there exists a constant 0<π≤1, and the following inequality holds:
LV(x)≤−CπℵVχ−(1−π)CℵVχ+ℵP, | (2.3) |
where P=P1+P2. Applying the Itˆo formula, one has
EV(x(t))=EV(x(0))+E{∫t0LV(x(ς))dς}=EV(x(0))+∫t0E{LV(x(ς))}dς, |
where E indicates the mathematical expectation.
Let Ω={x|EV(x)≤(P(1−π)C)1χ}, and in the sequel, the following two situations are contemplated.
Situation 1. if the signals do not satisfy Ω, according to (2.3) and applying Jensen's inequality, one has
E{LV(x(t))}≤−CπE{ℵ(t)Vχ(x(t))}≤−Cπℵ(t){EV(x(t))}χ. |
Therefore, it is obvious that
EV(x(t))≤∫t0−Cπℵ(ς){EV(x(ς))}χdς+EV(x(0)). |
Moreover, the following inequality exists:
EV(x(t))−EV(x(0))≤−Cπ∫t0ℵ(ς){EV(x(ς))}χdς. |
Denote EV(x(t))=G(x(t)) and construct the auxiliary function R(x(t)) as
R(x(t))=∫t0{dG(x(ς))dς+Cπℵ(ς)Gχ(x(ς))}dς. | (2.4) |
According to (2.4) and Lemma 1, one has
dG(x(t))dt≤−Cπℵ(t)Gχ(x(t)). |
Furthermore, the following inequality is listed:
11−χG1−χ(x(t))−11−χG1−χ(x(0))≤∫t0−Cπℵ(ς)dς,G(x(t))≤(G1−χ(x(0))−C(1−χ)π∫t0ℵ(ς)d(ς))11−χ. |
According to [34], we define function β(t)=πC(1−χ)∫t0ℵ(ς)d(ς) that is a prescribed-time function. Thus, there exists a time tp=β−1(1Cπ(1−χ)(G1−χ(x(0))−(P(1−π)C)1−χχ))<T such that β(tp)=G1−χ(x(0))−(P(1−π)C)1−χχ. Next, for t≤tp, we get G(x(t))≤(P(1−π)C)1χ. In addition, when t>tp, ℵ(t)=μ(tp) becomes a positive constant such that ℵ(t) is bounded. Then there is a finite time tf=1Cℵ(t)π(1−χ)(G1−χ(x(tp))−(P(1−π)C)1−χχ) such that G(x(t))≤(P(1−π)C)1χ for ∀t≥tp.
Situation 2. if the signals satisfy Ω, according to the Lasalle invariance principle, the track of x(t) remains within the set Ω. In sum, the time to arrive at the set Ω is bounded as tp which is less than T. Therefore, one has EV(x(t))≤(P(1−π)C)1χ.
Remark 1. In comparison with the prescribed-time stable in probability control strategies proposed in [16,17,18,19,20], the selection of a power index χ∈(0,1) in (2.3) ensures the existence of a time 0≤tp<T such that E(x2)≤2(P(1−π)C)12χ for t≥tp, resolving the issue that controllers containing the prescribed time function μ(t) do not involve infinite gain. For system (1.1), a globally stable state within the prescribed settling time T is realized. Moreover, since RBFNNs can infinitely approximate unknown nonlinear functions through online learning, the combination of prescribed-time control with adaptive neural networks allows for its application to stochastic nonlinear systems.
Lemma 3. [37] If (2.2) holds for system dz=q(z,x), then for any constant ˉa∈(0,a0), any initial condition z0=z0(0), and any function ˉλ satisfying ˉλ(‖x‖)≥λ(‖x‖), there exists a finite time T0=max{0,ln(Vw(z0)r0)/(a0−ˉa)}, a non-negative function B(t0,t) given for t≥t0, and the signal depicted by
dr=−ˉar+ˉλ(‖x‖)+c0,r(0)=r0≥0, |
such that B(t0,t)=0 for t≥t0+T0 and V(z(t))≤r(t)+B(t0,t). Without compromising generality, we select ˉλ(‖x‖)=λ(‖x‖).
Lemma 4. [38] If Assumption 6 holds and u belongs to L∞, the first-order system is defined by dℏ=−ℵe0ℏ+|u| for the subsystem (1.2) such that
‖ˉz‖≤q1(‖ˉz(0)‖+|ℏ(0)|)e−e0t+q2|ℏ(t)|, |
where q1>0, and q2>0 are constants.
Lemma 5. [39] Let p≥1, and the relation can be obtained as
n∑i=1|xi|p≤(n∑i=1|xi|)p≤np−1n∑i=1|xi|p,∀xi∈R. |
Lemma 6. [40] Let p≥1, and then
|[x]p−[y]p|≤p(2p−2+1)|x−y|(|x−y|p−1+|y|p−1),∀x,y∈R. |
Lemma 7. [41] Let ℘>0, ρ1>0, and ρ2>0. Then
|x|ρ1|y|ρ2≤℘ρ1ρ1+ρ2|x|ρ1+ρ2+℘−ρ1ρ2ρ2ρ1+ρ2|y|ρ1+ρ2,∀x,y∈R. |
Lemma 8. [42] p(t) is a real-valued function satisfying 0<p_≤p(t)≤ˉp with known constant p_ and ˉp. Then
|x|p(t)≤|x|p_+|x|ˉp,∀x∈R. |
To approximate the successive unknown nonlinear function F(Ψ):Rq→R over a compact set ΩΨ⊂Rq in the design process, we further introduce the RBFNNs defined by
Fnn(Ψ)=W⊤Φ(Ψ) |
in the context of the adaptive control problem, where the input vector Ψ∈ΩΨ⊂Rq is described, ΩΨ is compact, W=[w1,…,wd]⊤∈Rd is the weight vector, d>1 indicates the node number of the hidden layer, the vector of basis function Φ(Ψ)=[ϕ1(Ψ),…,ϕd(Ψ)]⊤∈Rd is denoted, the Gaussian function ϕi(Ψ) is described as
ϕi(Ψ)=e−(Ψ−zi)⊤(Ψ−zi)e21,i=1,…,d, |
where the center vector zi=[zi1,…,ziq]T is represented, and the width of the Gaussian function e1 is represented. If F(Ψ) is a continuous function on a compact set ΩΨ⊂Rq with sufficiently large node number d, then it can be approximated by the RBFNN as
F(Ψ)=W∗⊤Φ(Ψ)+ε(Ψ),∀Ψ∈ΩΨ, |
where ε(Ψ) with |ε(Ψ)|<ε∗ indicates the approximation deviation, and ε∗>0 indicates an unknown constant. The optimal weight vector, denoted as W∗, is expressed as
W∗=argminW∈RdsupΨ∈ΩΨ|F(Ψ)−W⊤Φ(Ψ)|. |
Lemma 9. [43] If the basis function vector of the RBFNNs is expressed by Φ(Ψn)=[ϕ1(Ψn),⋯,ϕd(Ψn)]T with Ψn∈Rn, then ‖Φ(Ψn)‖2≤‖Φ(Ψm)‖2,m≤n.
Remark 2. It is worth noting that the nonlinear functions fi(x) and gi(x) in system (1.1) include all state variables, which is a non-strict-feedback structure. This is also true for some devices in practical applications, such as uncertain robot systems and hyperchaotic inductive capacitor oscillation circuit systems. If the control algorithm adopts the traditional backstepping method, its virtual controller will inevitably contain the state variables of system (1.1), which will lead to algebraic loop problems in simulation. Therefore, based on the definition of ϕi(Ψ) and Φ(Ψ) in RBFNNs, we utilize the properties of Gaussian functions in Lemma 9 and the approach of segregating variables to reduce the number of variables in the controller. Moreover, the restrictive conditions on fi and gi in [29,30,31,32,33] where stochastic disturbances and time-varying powers also exist in the studied system are no longer needed in this paper.
This section introduces a cohesive neural adaptive prescribed-time control strategy. The recursive design process comprises n steps involving dynamic surface control and the backstepping technique. The design of the n-step backstepping is rooted in the following coordinate transformation:
ξ1=x1,ξi=xi−xid,si=xid−αi−1,i=2,...,n, | (3.1) |
where si denotes error surfaces, αi−1 denotes virtual controllers, xid denotes the first-order filter output signal which can be obtained through the input αi−1, and αi−1 will be specified later.
Step 1. Consider the two equations of (1.1) and (3.1), and we have
dξ1(t)=(h1[x2]p1(t)+f1(x)+Γ1)dt+g⊤1(x)dw. | (3.2) |
Take the following Lyapunov function candidate into account:
V1=14ξ41. | (3.3) |
Applying Assumption 3 and Definition 1, it is not difficult to obtain that
LV1=ξ31(h1[x2]p1(t)+f1(x)+Γ1(x,z))+32ξ21g⊤1(x)g1(x)≤ξ31(h1[x2]p1(t)+f1(x))+32ξ21g⊤1(x)g1(x)+ξ31(ψ11(‖x‖)+ψ12(‖z‖)). | (3.4) |
Building upon Young's inequality, Assumption 3, and Lemma 3, it holds that
|ξ31|(ψ11(‖x‖)+ψ12(‖z‖))≤12+34ξ41(ψ4311(‖x‖)+ψ4312(α−11(r+B(t0,t)))),32ξ21g⊤1(x)g1(x)≤34d−21ξ41‖g1(x)‖4+34d21, | (3.5) |
where d1>0 represents a constant.
Then, one has
LV1≤ξ31h1(x1)[x2]p1(t)+ξ31¯f1(X1)+12+34d21, | (3.6) |
where the unknown nonlinear function ¯f1(X1)=f1+34d−21ξ1‖g1(x)‖4+34ξ1(ψ4311(‖x‖)+ψ4312(α−11(r+B(t0,t)))) with X1=(x,r)⊤. Building upon the RBFNNs, a neural network represented as W∗⊤1Φ1(X1) is utilized to approximate ¯f1(X1) with X1∈ΩX1 in a manner that
¯f1(X1)=W∗T1Φ1(X1)+ε1(X1),|ε1(X1)|≤ε∗1, | (3.7) |
with any given constant ε∗1>0.
Using Lemma 9 and Young's inequality, one has
ξ31ˉf1(X1)≤|ξ1|3(‖W∗1‖⋅‖Φ1(Z1)‖+ε∗1)≤33+ˉpϱ3+ˉp31|ξ1|3+ˉp‖W∗1‖3+ˉp3‖Φ1(Z1)‖3+ˉp3+ˉp3+ˉpϱ−ˉp+3ˉp1+33+ˉp|ξ1|ˉp+3+ˉp3+ˉpε∗ˉp+3ˉp1≤|ξ1|ˉp+3((ϱ1‖Φ1(Z1)‖)3+ˉp3Θ∗+1)+ε∗ˉp+3ˉp1+ϱ−ˉp+3ˉp1, | (3.8) |
where Z1=[x1]⊤, Θ∗=max{‖W∗i‖3+ˉp3i=1,…,n}, and ϱ1 indicates a design positive parameter.
Then, the formula (3.6) can be rewritten as
LV1≤ξ31h1([x2]p1(t)−[α1]p1(t))+ξ31h1[α]p1(t)1+|ξ1|3+ˉp((ϱ1||Φ1(Z1)||)3+ˉp3Θ∗+1)+34d21+12+ε∗ˉp+3ˉp1+ϱ−ˉp+3ˉp1. | (3.9) |
The first intermediate control signal is constructed as follows:
α1=−ℵ(ξ1+[ξ1]ˉp)(h_−11+h_−1ˉp1)(k1+1+(ϱ1||Φ1(Z1)||)3+ˉp3ˆΘ), | (3.10) |
where k1>0 is a design parameter. Furthermore, from Lemmas 5 and 8, we get
h1(h_−11+h_−1ˉp1)p1(t)≥h_1(h_−p1(t)1+h_−p1(t)ˉp1)=h_1−p1(t)1+h_1−p1(t)ˉp1≥1,ξ31h1[α1]p1(t)≤−ℵ(|ξ1|p1(t)+3+|ξ1|3+ˉpp1(t))(k1+1+(ϱ1||Φ1(Z1)||)3+ˉp3ˆΘ),|ξ1|3+ˉp≤|ξ1|p1(t)+3+|ξ1|3+ˉpp1(t). | (3.11) |
Thus, LV1 can be obtained as
LV1≤−ℵk1(|ξ1|p1(t)+3+|ξ1|3+ˉpp1(t))+D1+ξ31h1([x2]p1(t)−[α1]p1(t))+˜Θ⊤|ξ1|3+ˉp(ϱ1||Φ1(Z1)||)3+ˉp3, | (3.12) |
where D1=12+34d21+ε∗3+ˉpˉp1+ϱ−3+ˉpˉp1.
According to Assumptions 1 and 2, and Lemmas 6–8, we have
ξ31h1([x2]p1(t)−[α1]p1(t))≤p1(t)(2p1(t)−2+1)¯h1|ξ1|3|ξ2+s2|(|ξ2+s2|p1(t)−1+|α1|p1(t)−1)≤|ξ2|3+ˉpφ21+|s2|4φ22+M0, | (3.13) |
where φ21=2ˉp+2(ˉp(2ˉp−2+1))ˉp+3|¯h1ξ31|3+ˉp(2+|ξ2+s2|(ˉp+3)(ˉp−1)+|α1|(ˉp+3)(ˉp−1)) and φ22 are known C1 functions independent of p1(t), and M0=2+ˉp3+ˉp(3+ˉp)−12+ˉp+344−13.
From Lemma 8 and Assumption 1, it holds that
|ξi|ˉp+3≤|ξi|pi(t)+3+|ξi|3+ˉppi(t), |ξi|pi(t)+3≤|ξi|pi(t)+3+|ξi|3+ˉppi(t),i=1,...,n. | (3.14) |
Substituting (3.13) and (3.14) into (3.12) results in
LV1≤|ξ2|3+ˉpφ21+|s2|4φ22+M0+D1−k1ℵ(|ξ1|p1(t)+3+|ξ1|3+ˉpp1(t))+˜ΘT|ξ1|ˉp+3(ϱ1||Φ1(Z1)||)3+ˉp3. | (3.15) |
Step i (i=2,…,n−1). Based on Itˆo's differentiation rule and (3.1), we have
dξi(t)=(hi[xi+1]pi(t)+fi+Γi−˙xid)dt+g⊤i(x)dw. | (3.16) |
Select the Lyapunov function candidate as
Vi=Vi−1+14ξ4i+14s4i. | (3.17) |
Then, the infinitesimal generator of Vi indicates
LVi=LVi−1+ξ3i(hi[xi+1]pi(t)+fi(x)+Γi(z,x)−˙xid)+32ξ2igi(x)⊤gi(x)+s3idsi. | (3.18) |
In order to circumvent the need for repetitive differentiation of αi−1, the first-order filter can be described as
τi˙xid=ℵ(αi−1−xid),xid(0)=αi−1(0), | (3.19) |
where τi is a positive constant.
By using the definition of si=xid−αi−1, we have ˙xid=−ℵsiτi, and the differential of si is
dsi=(−ℵsiτi−i−1∑j=1∂αi−1∂xj(hj−1[xj]pj−1(t)+fj−1−Γj−1)+∂αi−1∂ˆΘ˙ˆΘ+∂αi−1∂t+12i−1∑k,l=1∂2αi−1∂xk∂xlgTkgl+∂αi−1∂xi−1d˙xi−1d)dt−i−1∑j=1∂αi−1∂xjg⊤j(x)dw. | (3.20) |
From (3.20) and Young's inequality, one concludes
s3idsi=32s2i(i−1∑j=1∂αi−1∂xjgj(x))⊤(i−1∑j=1∂αi−1∂xjgj(x))+s3i(−ℵsiτi−dαi−1)≤32s2i(i−1∑j=1∂αi−1∂xjgj(x))⊤(i−1∑j=1∂αi−1∂xjgj(x))−ℵs4iτi+|si|3|−dαi−1|≤34((i−1∑j=1∂αi−1∂xjgj(x))⊤(i−1∑j=1∂αi−1∂xjgj(x)))2+14(dαi−1)4−ℵs4iτi+32s4i≤−ℵs4iτi+32s4i+ηi(x,ˉsn,ˆΘ,r), | (3.21) |
where dαi−1=∑i−1j=1∂αi−1∂xj(hj−1[xj]pj−1(t)+fj−1−Γj−1)+∂αi−1∂ˆΘ˙ˆΘ+∂αi−1∂t +12∑i−1k,l=1∂2αi−1∂xk∂xlg⊤kgl +∂αi−1∂xi−1d˙xi−1d and ηi≥34((∑i−1j=1∂αi−1∂xjgj(x))⊤(∑i−1j=1∂αi−1∂xjgj(x)))2+14(dαi−1)4 is a non-negative continuous function.
With the help of the same estimation method in step 1, we have
ξ3iΓi≤34ξ4i(ψ43i1(‖x‖)+ψ43i2(α−11(r+B(t0,t))))+12,32ξ2ig⊤igi≤34d−2iξ4i‖gi‖4+34d2i. | (3.22) |
Similar to (3.13), one has
|ξ3i−1hi−1([xi]pi−1(t)−[αi−1]pi−1(t))|≤|ξi|ˉp+3φi1+s4iφi2+M0. | (3.23) |
Then, the dynamic equation of Vi is
LVi≤−i−1∑j=1kjℵ(|ξj|3+pj(t)+|ξj|3+pj(t)ˉp)+M0+ξ3i(hi[xi+1]pi(t)+ˉfi(Xi))+i∑j=2ηj+i−1∑j=1˜ΘT|ξj|ˉp+3(ϱj||Φj(Zj)||)3+ˉp3−ℵi∑j=2s4j(1τj−32−φj2)+34d2i+12, | (3.24) |
where ˉfi(Xi)=fi(x)+34d−2iξi‖gi‖4+34ξi(ψ43i1(‖x‖)+ψ43i2(α−11(r+B(t0,t))))−˙xid+[ξi]ˉpφi1 with Xi=(x,xid,˙xid,ˆΘ,r)⊤ being an unknown nonlinear function. Then, for a given positive constant ε∗i, the neural network W∗⊤iΦi(Xi) with Xi∈ΩXi can be utilized for approximating ˉfi(Xi) in the following manner:
ˉfi(Xi)=W∗⊤iΦi(Xi)+εi(Xi),|εi(Xi)|≤ε∗i. | (3.25) |
Using Young's inequality and Lemma 9 yields
ξ3iˉfi(Xi)≤|ξi|3+ˉp((ϱi‖Φi(Zi)‖)3+ˉp3Θ∗+1)+ε∗3+ˉpˉpi+ϱ−3+ˉpˉpi, | (3.26) |
where Zi=[x1,…,xi,ˆΘ]⊤, and ϱi>0 is a given design parameter.
Then, it can be obtained that
LVi≤−i−1∑j=1kjℵ(|ξj|3+pj(t)+|ξj|3+pj(t)ˉp)+i∑j=1Dj+ξ3ihi([xi+1]pi(t)−[αi]pi(t))+ξ3ihi[αi]pi(t)+˜Θ|ξi|3+ˉp(ϱi‖Φi(Zi)‖)3+ˉp3−ℵi∑j=2s4j(1τj−32−φj2)+|ξi|3+ˉp((ϱi‖Φi(Zi)‖)3+ˉp3ˆΘ+1)+i−1∑j=1˜Θ|ξj|ˉp+3(ϱj||Φj(Zj)||)3+ˉp3+i∑j=2ηj, | (3.27) |
where Di=34d2i+12+ϱ−3+ˉpˉpi+ε∗3+ˉpˉpi+M0.
Next, the virtual controller can be specified as
αi=−ℵ(ξi+[ξi]ˉp)(h_−1i+h_−1ˉpi)(ki+1+ϱ3+ˉp3i‖Φi(Zi)‖3+ˉp3ˆΘ). | (3.28) |
Substituting (3.28) into (3.27), it can be derived that
LVi≤−i∑j=1kjℵ(|ξj|3+pj(t)+|ξj|3+pj(t)ˉp)−ℵi∑j=2s4j(1τj−32−φj2)+i∑j=1Dj+i∑j=2ηj+i∑j=1˜ΘT|ξj|ˉp+3(ϱj||Φj(Zj)||)3+ˉp3+ξ3ihi([xi+1]pi(t)−[αi]pi(t)). | (3.29) |
Step n. In this step, a real control law u will be constructed. Choose the Lyapunov function and compact set Ωn as
Vn(ξ,s,˜Θ,˜Λ,ℏ)=Vn−1+14dΓξ4n+14s4n+12γ˜ΘT˜Θ+12γ1˜Λ2+1γ2ℏ, | (3.30) |
where ξ=[ξ1,…,ξn], s=[s2,…,sn], γ>0, γ1>0, and γ2>0 are the design parameters, and (˜⋅)(t)=(⋅)∗−(ˆ⋅)(t) with (ˆ⋅) being the estimation of (⋅)∗.
From Assumption 5 and Lemma 4, we obtain
|cΓ(ˉz)|≤b0q1(‖ˉz(0)‖+|ℏ(0)|)e−e0t+b0q2|ℏ(t)|≤Λm(1+ℏ(t)), | (3.31) |
where Λm=max{b0q1(‖ˉz(0)‖+|ℏ(0)|),b0q2}.
Thus, we have
1dΓξ3nhncΓ(ˉz)≤ˉp3+ˉp(ˉhn(ˉxn)dΓΛm(1+|ℏ(t)|))ˉp+3ˉp+3ˉp+3|ξn|ˉp+3≤3ˉp+3|ξn|ˉp+3+ξ3+ˉpn(1+|ℏ(t)|)3+ˉpˉpΛ+(1−ξˉp+3nς)(1+|ℏ(t)|)ˉp+3ˉpΛa,1dΓ|ξ3n|Γn(z,x)≤12+34ξ4nd−43Γ(ψ43n1(‖x‖)+ψ43n2(α−11(r+B(t0,t)))),32dΓξ2ng⊤ngn≤34d2Γξ4n‖gn‖4+34d2n, | (3.32) |
where Λ=Λaς, Λa=ˉp3+ˉp(ˉhn(ˉxn)dΓΛm)3+ˉpˉp, and dn>0 and ς>0 are constants.
Furthermore, the expression of LVn is
LVn≤−ℵn−1∑j=1kj(|ξj|3+pj(t)+|ξj|3+pj(t)ˉp)+ξ3n(hn[u]pn(t)+ˉfn(Xn))+n−1∑j=1˜ΘT|ξj|ˉp+3(ϱj||Φj(Zj)||)3+ˉp3+n∑j=2ηj−ℵn−1∑j=2s4j(1τj−32−φj2)+34d2n+12+n−1∑j=1Dj−1γ1˜Λ˙ˆΛ−ℵe0ℏγ2+|u|γ2−1γ˜Θ˙ˆΘ+3ˉp+3|ξn|ˉp+3+ξ3+ˉpn(1+|ℏ(t)|)3+ˉpˉpΛ+(1−ξ3+ˉpnς)(1+|ℏ(t)|)3+ˉpˉpΛa−ℵs4n(1τn−32), | (3.33) |
where ˉfn(Xn)=1dΓ(fn(x)−˙xnd)+34d2Γξn‖gn‖4+34ξnd−43Γ(ψ43n1(‖x‖)+ψ43n2(α−11(r+B(t0,t)))) with Xn=(x,xnd,˙xnd,ˆΘ,r)⊤ being an unknown nonlinear function. Then ˉfn(Xn)=W∗⊤nΦn(Xn)+εn(Xn),|εn(Xn)|≤ε∗n with the neural network W∗⊤nΦn(Xn) and a positive constant ε∗n.
Utilizing Young's inequality and Lemma 9, one has
ξ3nˉfn(Xn)≤|ξn|3+ˉp((ϱn‖Φn(Zn)‖)3+ˉp3Θ∗+1)+ε∗3+ˉpˉpn+ϱ−3+ˉpˉpn, | (3.34) |
where Zn=[x1,…,xn,ˆΘ]T and ϱn indicates a given design positive parameter.
Then, by substituting (3.34) into (3.33), one has
LVn≤−ℵn−1∑j=1kj(|ξj|pj(t)+3+|ξj|3+ˉppj(t))+ξ3nhn[u]pn(t)−ℵn−1∑j=2s4j(1τj−32−φj2)+n∑j=2ηi+n∑j=1Dj+|ξn|3+ˉp(ϱ3+ˉp3n‖Φn(Zn)‖3+ˉp3ˆΘ+1)−1γ1˜Λ˙ˆΛ+ξ3+ˉpn(1+|ℏ(t)|)3+ˉpˉpΛ−ℵe0ℏγ2+|u|γ2+33+ˉp|ξn|3+ˉp+n∑j=1˜ΘTγ(γ|ξj|ˉp+3(ϱj||Φj(Zj)||)3+ˉp3−˙ˆΘ)+(1−ξ3+ˉpnς)(1+|ℏ(t)|)3+ˉpˉpΛa−ℵs4n(1τn−32), | (3.35) |
where D_n = \frac{3}{4}d_n^2+\frac{1}{2} +\varrho_n^{-\frac{\bar p+3}{\bar p}} +\varepsilon_n^{*\frac{\bar p+3}{\bar p}} .
Now, the control law and the parameter update laws are devised as
\begin{eqnarray} u & = & -\aleph(\xi_n+[\xi_n]^{\bar p}) (\underline{h}_n^{-1}+\underline{h}_n^{-\frac{1}{\bar{p}}}) \Big(k_n+1+\varrho_n^{\frac{3+\bar p}{3}}\|\Phi_n(Z_n)\|^{\frac{3+\bar p}{3}}\hat\Theta +(1+|\hbar(t)|)^{\frac{3+\bar p}{\bar p}}\hat{\Lambda} \Big), \cr \dot{\hat{\Theta}} & = & \sum\limits_{j = 1}^{n}\aleph(\gamma|\xi_j|^{3+\bar{p}}(\varrho_j||\Phi_j(Z_j)||)^{\frac{3+\bar{p}}{3}}-\delta\hat{\Theta}), \cr \dot{\hat{\Lambda}} & = & \aleph(\gamma_1\xi_n^{3+\bar p}(1+|\hbar(t)|)^{\frac{3+\bar p}{\bar p}}-\delta_1\hat{\Lambda}), \end{eqnarray} | (3.36) |
where k_n , \delta , and \delta_1 are positive design parameters.
Therefore, we can get
\begin{eqnarray} \mathcal{L} V_n &\leq& -\aleph\Big(k_n-\frac{3}{3+\bar p}\Big)\Big(|\xi_n|^{p_{n}(t)+3}+|\xi_n|^{3+p_{n}(t)\bar p}\Big) -\aleph\sum\limits_{j = 1}^{n-1}k_j\Big(|\xi_j|^{p_{j}(t)+3}+|\xi_j|^{3+p_{j}(t)\bar p}\Big) +\aleph\frac{\delta}{\gamma}\tilde{\Theta}\hat{\Theta} +\sum\limits_{j = 1}^{n}D_j\cr && +\sum\limits_{j = 2}^{n}\eta_j -\aleph\sum\limits_{j = 2}^{n-1}s_j^{4}\Big(\frac{1}{\tau_j}-\frac{3}{2} -\varphi_{j2}\Big) +\Big(1-\frac{\xi_n^{3+\bar p}}{\varsigma}\Big)(1+|\hbar(t)|)^{\frac{3+\bar p}{\bar p}}\Lambda_a -\aleph s_n^{4}\Big(\frac{1}{\tau_n}-\frac{3}{2}\Big)\cr && +\aleph\frac{\delta_1}{\gamma_1}\tilde{\Lambda}\hat{\Lambda} -\frac{\aleph e_0\hbar}{\gamma_2} +\frac{|u|}{\gamma_2}. \end{eqnarray} | (3.37) |
Theorem 1. Under the controllers and parameter adaptive laws (3.10), (3.28), and (3.36), stochastic system (1.1) satisfying Assumptions 1–6 exhibits the following characteristics: (1) All internal signals within the closed-loop system (1.1) remain bounded. (2) The equilibrium point of the system is prescribed-time stable in probability.
Proof. Next, it is evident from the structure of V_n in (3.30) that V_n is a positive definite, radial unbounded, and quadratic continuously differentiable function. Referring to Lemma 4.3 in [44], there exist \mathcal{K}_\infty functions \bar{\alpha}_1(\cdot) and \bar{\alpha}_2(\cdot) such that
\begin{eqnarray} \bar{\alpha}_1(\Delta) \leq V_n(\Delta) \leq \bar{\alpha}_2(\Delta), \ \Delta = (\xi, s, \tilde{\Theta}, \tilde{\Lambda}, \hbar). \end{eqnarray} | (3.38) |
By defining \Theta^\ast and \Lambda^\ast , one obtains
\begin{eqnarray} \frac{\aleph\delta}{\gamma}\tilde{\Theta}^\top\hat{\Theta} & = & \frac{\aleph \delta}{\gamma}\tilde{\Theta}^\top(\Theta^{\ast}-\tilde{\Theta}) \le -\frac{\aleph \delta}{2\gamma}\tilde{\Theta}^\top\tilde{\Theta} +\frac{\aleph \delta}{2\gamma}\Theta^{\ast \top}\Theta^{\ast}, \cr \frac{\aleph\delta_1}{\gamma_1}\tilde{\Lambda}^\top\hat{\Lambda} & = & \frac{\aleph \delta_1}{\gamma_1}\tilde{\Lambda}^\top(\Lambda^{\ast}-\tilde{\Lambda}) \le -\frac{\aleph \delta_1}{2\gamma_1}\tilde{\Lambda}^\top\tilde{\Lambda} +\frac{\aleph \delta_1}{2\gamma_1}\Lambda^{\ast \top}\Lambda^{\ast}. \end{eqnarray} | (3.39) |
With the aid of Lemma 7 and (3.14), one has
\begin{eqnarray} (\xi_i^4)^{\frac{\bar{p}-1}{\bar{p}+1}} \leq |\xi_i|^{\bar{p}+3} -\frac{4(\bar{p}-1)}{(\bar{p}+1)(3+\bar{p})}+ 1. \end{eqnarray} | (3.40) |
From (3.40), choose \pi = \min\{k_1, \ldots, k_{n}, \delta, \delta_1, e_0\} , let \chi = \frac{\bar{p}-1}{\bar{p}+1} and k_n \geq \frac{3}{3+\bar p}+\pi(\frac{1}{d_\Gamma})^\chi , and rewrite (3.37) as
\begin{eqnarray} \mathcal {L} V_n(\Delta) &\le& -\aleph4^\chi\pi \Big(\sum\limits_{j = 1}^{n-1}\Big(\frac{1}{4}\xi_j^{4}\Big)^\chi+\Big(\frac{1}{4d_\Gamma}\xi_n^{4}\Big)^\chi\Big) -\aleph\Big(\frac{\pi}{2\gamma}\tilde{\Theta}^T\tilde{\Theta}\Big)^\chi +\aleph(\frac{\pi }{2\gamma}\tilde{\Theta}^T\tilde{\Theta})^\chi +\aleph\Big(\frac{4(\bar{p}-1)}{(\bar{p}+1)(3+\bar{p})}-1\Big)\cr &&\cdot\Big(\sum\limits_{j = 1}^{n-1}k_j(n-1)+k_n-\frac{3}{3+\bar p}\Big) +\sum\limits_{j = 1}^{n}D_j +\aleph(\frac{\pi}{2\gamma_1}\tilde{\Lambda}^T\tilde{\Lambda})^\chi +\sum\limits_{j = 2}^{n}\eta_j -\aleph\sum\limits_{j = 2}^{n-1}s_j^{4}\Big(\frac{1}{\tau_j}-\frac{3}{2}-\varphi_{j2}\Big)\cr && -\pi\frac{\aleph}{2\gamma}\tilde{\Theta}^T\tilde{\Theta} +\frac{\aleph\delta}{2\gamma}\Theta^{*T}\Theta^{*} -\pi\frac{\aleph}{2\gamma_1}\tilde{\Lambda}^T\tilde{\Lambda} +\frac{\aleph\delta_1}{2\gamma_1}\Lambda^{*T}\Lambda^{*}-\frac{\aleph \pi \hbar}{\gamma_2} +\frac{|u|}{\gamma_2}\cr && +\Big(1-\frac{\xi_n^{3+\bar p}}{\varsigma}\Big)(1+|\hbar(t)|)^{\frac{3+\bar p}{\bar p}}\Lambda_a -\aleph s_n^{4}\Big(\frac{1}{\tau_n}-\frac{3}{2}\Big)-\aleph\Big(\frac{\pi}{2\gamma_1}\tilde{\Lambda}^T\tilde{\Lambda}\Big)^\chi. \end{eqnarray} | (3.41) |
According to Lemma 7, one obtains
\begin{eqnarray} && \aleph\Big(\pi\frac{1}{2\gamma}\tilde{\Theta}^T\tilde{\Theta}\Big)^\chi +\aleph\Big(\frac{\pi}{2\gamma_1}\tilde{\Lambda}^T\tilde{\Lambda}\Big)^\chi -\aleph\Big(\frac{1}{\tau_j}-\frac{3}{2}-\varphi_{j2}\Big)s_j^4 -\frac{\aleph \pi \hbar}{\gamma_2}-\aleph s_n^{4}\Big(\frac{1}{\tau_n}-\frac{3}{2}\Big) \le 5\aleph(1-\chi)\chi^{\frac{\chi}{1-\chi}}\cr && -\aleph\Big(\frac{ \pi \hbar}{\gamma_2}\Big)^\chi +\pi\frac{\aleph}{2\gamma}\tilde{\Theta}^T\tilde{\Theta} +\pi\frac{\aleph}{2\gamma_1}\tilde{\Lambda}^T\tilde{\Lambda}-\aleph\Big(\Big(\frac{1}{\tau_j}-\frac{3}{2}-\varphi_{j2}\Big)s_j^4\Big)^\chi -\aleph\Big(\Big(\frac{1}{\tau_n}-\frac{3}{2}\Big)s_n^4\Big)^\chi.\qquad \end{eqnarray} | (3.42) |
Since \varphi_{j2} , \eta_j , |u| , and |(1-\frac{\xi_n^{3+\bar p}}{\varsigma})(1+|\hbar(t)|)^{\frac{3+\bar p}{\bar p}}\Lambda_a| are non-negative continuous functions, and V_n(\Delta) is bounded, there exist known positive constants \bar{\varphi}_{j2} , \bar{\eta}_j , \bar{H}_1 , and N_1 such that \varphi_{i2} \leq \bar{\varphi}_{i2} , |u| \leq N_1 , \eta_i \leq \bar{\eta}_i , and |(1-\frac{\xi_n^{3+\bar p}}{\varsigma})(1+|\hbar(t)|)^{\frac{3+\bar p}{\bar p}}\Lambda_a| \; \leq \; \bar{H}_1 . Hence, by choosing \frac{1}{\tau_j} \geq \bar{\varphi}_{j2} + \frac{3}{2} and \frac{1}{\tau_n} \geq \frac{3}{2} , (3.41) becomes
\begin{eqnarray} \mathcal{L}V_n(\Delta) &\le& -\aleph4^\chi\pi \Big(\sum\limits_{j = 1}^{n-1}\Big(\frac{\xi_j^{4}}{4}\Big)^\chi+\Big(\frac{\xi_n^{4}}{4d_\Gamma}\Big)^\chi\Big) -\aleph\pi^\chi\Big(\frac{\hbar}{\gamma_2}\Big)^\chi -\aleph\pi^\chi\Big(\frac{1}{2\gamma}\tilde{\Theta}^T\tilde{\Theta}\Big)^\chi -\aleph\pi^\chi\Big(\frac{1}{2\gamma_1}\tilde{\Lambda}^T\tilde{\Lambda}\Big)^\chi +\aleph P_1 +P_2\cr &&-\sum\limits_{j = 2}^{n-1}\aleph 4^\chi\Big(\frac{s_j^{4}}{4}\Big)^\chi\Big(\frac{1}{\tau_j}-\frac{3}{2}-\bar{\varphi}_{j2}\Big)^\chi -\aleph 4^\chi\Big(\frac{1}{4}s_n^{4}\Big)^\chi\Big(\frac{1}{\tau_n}-\frac{3}{2}\Big)^\chi\cr &\leq& -C \aleph V^\chi_n+\aleph P_1+P_2, \end{eqnarray} | (3.43) |
where C = \min\{ 4^\chi\pi, \pi^\chi, (4(\frac{1}{\tau_j}-\frac{3}{2}-\bar{\varphi}_{j2}))^\chi, (4(\frac{1}{\tau_n}-\frac{3}{2}))^\chi \} , P_1 = \delta\frac{1}{2\gamma}\Theta^{*T}\Theta^{*} +\delta_1\frac{1}{2\gamma_1}\Lambda^{*T}\Lambda^{*} +(n+3)(1-\chi)\chi^{\frac{\chi}{1-\chi}} +(1-\frac{4(\bar{p}-1)}{(\bar{p}+1)(3+\bar{p})})(\sum_{j = 1}^{n-1}k_j(n-1)+k_n-\frac{3}{3+\bar p}) , and P_2 = \sum_{j = 1}^{n}D_j+\sum_{j = 2}^{n}\bar{\eta}_i +N_1+\bar{H}_1 . Therefore, we can obtain
\begin{eqnarray} \mathcal{L} V_n \leq -C\aleph V^\chi_n+ \aleph P, \end{eqnarray} | (3.44) |
where P = P_1+P_2 . Consequently, it is deduced from (3.30) and (3.44), in conjunction with Lemma 1, that the \Delta -system attains semi-global practical prescribed-time stability in probability. Taking the proof procedure of Lemma 2 and (3.44) into consideration, it can be concluded that all signals are confined within a compact set \Omega = \{\Delta|EV_n \leq (\frac{P}{(1-\pi)C})^{\frac{1}{\chi}} \} as
\begin{eqnarray} &&[E(\xi_j^2)] \leq [E(\xi_j^4)]^{\frac{1}{2}} \leq (4E(V_n))^{\frac{1}{2}} \leq 2\Big(\frac{P}{(1-\pi)C}\Big)^{\frac{1}{2\chi}}, \cr &&[E(\tilde{\Theta}^2)] = [E(\tilde{\Lambda}^2)] \leq 2\gamma_{\max} EV_n \leq 2\gamma_{\max}\Big(\frac{P}{(1-\pi)C}\Big)^{\frac{1}{\chi}}, \cr &&[E(s_j^2)] \leq [E(s_j^4)]^{\frac{1}{2}} \leq (4E(V_n))^{\frac{1}{2}} \leq 2\Big(\frac{P}{(1-\pi)C}\Big)^{\frac{1}{2\chi}}, \end{eqnarray} | (3.45) |
where \gamma_{\max} = \max\{\gamma, \gamma_1\} .
Remark 3. We further elucidate the challenges presented in this thesis as follows:
(1) In this paper, due to the joint role of unknown time-varying powers, stochastic disturbances, unknown control coefficients, and unmodeled dynamics, to handle the high nonlinearity in the design procedure is not an easy task.
(2) Since the adaptive neural network control is incorporated into the design process to address cases involving an unknown nonlinear function encompassing all state variables, which inherently results in the diversity of variables and the complexity of coefficient functions, therefore, how to overcome this problem to prevent the difficult execution of the controller becomes a challenging task.
(3) How to analyze the semi-global practical prescribed-time stability in probability of system (1.1) with unknown time-varying powers, stochastic disturbances, unknown control coefficients, and unmodeled dynamics is not easy work.
The design procedure of the proposed control scheme can be depicted by Figure 1.
To validate the effectiveness and feasibility of the proposed adaptive control project, this section presents a numerical example and a practical application case.
The following second-order stochastic high-order non-strict-feedback nonlinear system is considered:
\begin{eqnarray} \text{d}z & = & -z+0.5(x_1^2+x_2^2)\sin(x_1)+0.5, \cr \text{d}x_1 & = & ([x_2]^{\frac{7}{6}+\frac{1}{6} \sin t}+x_1\cos (x_2)+0.1z \sin(x_1x_2))\text{d}t+15x_1^2x_2\text{d}w, \cr \text{d}x_2 & = & (\nu+x_1x_2^2+zx_1^2x_2)\text{d}t+16x_2 e^{-x_1^2}\text{d}w, \cr \text{d}\bar{z}_1 & = & -4\bar{z}_1-\bar{z}_1^3+2\bar{z}_2, \text{d}\bar{z}_2 = -2\bar{z}_2+[u]^{\frac{7}{6}+\frac{1}{6}\sin t}, \ \nu = 4\bar{z}_1+\frac{-2\bar{z}_2+3\bar{z}_2^3}{2+\bar{z}_2^2}+[u]^{\frac{7}{6}+\frac{1}{6}\sin t}, \end{eqnarray} | (4.1) |
where p_{1}(t) = p_{2}(t) = \frac{7}{6}+\frac{1}{6}\sin t , \bar p = \frac{4}{3} , \underline p = 1 , \underline{h}_i = \bar{h}_i = 1 , f_1(x) = x_1\cos (x_2) , f_2(x) = x_1x_2^2 , \Gamma_1 = 0.1z \sin(x_1x_2) , \Gamma_2 = zx_1^2x_2 , g_1(x) = 15x_1^2x_2 , and g_2(x) = 16x_2 e^{-x_1^2} .
By adhering to the design methodology elucidated in this thesis, the virtual controller \alpha_1 , the controller u , and adaptive laws \dot{\hat\Theta} and \dot{\hat\Lambda} can be obtained in the following form:
\begin{eqnarray} \alpha_1 & = & -\aleph(\xi_1+[\xi_1]^{\bar p})(\underline{h}_1^{-1}+\underline{h}_1^{-\frac{1}{\bar{p}}}) (k_1+1+\varrho_1^{\frac{3+\bar p}{3}}\|\Phi_1(Z_1)\|^{\frac{3+\bar p}{3}}\hat\Theta), \cr u & = & -\aleph(\xi_2+[\xi_2]^{\bar p})(\underline{h}_2^{-1}+\underline{h}_2^{-\frac{1}{\bar{p}}}) \Big(k_2+1+\varrho_2^{\frac{3+\bar p}{3}}\|\Phi_2(Z_2)\|^{\frac{3+\bar p}{3}}\hat\Theta +(1+|\hbar(t)|)^{\frac{3+\bar p}{\bar p}}\hat{\Lambda}\Big), \cr \dot{\hat{\Theta}} & = & \sum\limits_{j = 1}^{2}\aleph(\gamma|\xi_j|^{3+\bar{p}}(\varrho_j||\Phi_j(Z_j)||)^{\frac{3+\bar{p}}{3}}-\delta\hat{\Theta}), \cr \dot{\hat{\Lambda}} & = & \aleph(\gamma_1\xi_2^{3+\bar p}(1+|\hbar(t)|)^{\frac{3+\bar p}{\bar p}}-\delta_1\hat{\Lambda}). \end{eqnarray} | (4.2) |
In simulation, we choose the prescribed settling time T = 4 and the initial values are designated as [x_1(0), x_2(0)] = [4, −5] , \hat{\Theta}(0) = 0.9 , \hat{\Lambda}(0) = -0.9 , \hbar(0) = 0.9 , z(0) = 1.5 , and \bar{z}(0) = [0.1, 0.1]^T . The design parameters are selected in intermediate control functions as \tau_2 = 0.4 , k_1 = k_2 = 0.5 , \varrho_1 = \varrho_2 = 0.2 , \delta = \delta_1 = 0.3 , \gamma = \gamma_1 = 0.1 , e_0 = 5 , and s = 2 . To establish the basis vector function \Phi_j(Z_j) , for the backstepping steps, we designate the center of the receptive field as z = [-1.5, -1, -0.5, 0, 0.5, 1, 1.5]^T and determine the Gaussian functions width as e = \sqrt{2} .
Thus, the outcomes of the simulation are shown by Figures 2–4. Figure 2 shows the curve of the system states x_1 and x_2 . Figure 3 illustrates the curves of control signal u . Figure 4 depicts that the trajectories of the adaptive parameters \hat{\Theta} and \hat{\Lambda} are bounded. As shown in these figures, we know that the suggested adaptive neural control scheme ensures that the closed-loop system is continuously stable after a preset time T = 4 (s).
In order to verify Assumptions 4 – 6 , we select input unmodeled dynamics \bar{z } = [\bar{z}_1, \bar{z}_2]^T , V_w = \bar{z}^T\bar{z} , and V_0(z) = z^2 . By employing Young's inequality, one obtains
\begin{eqnarray} |c_\Gamma(\bar{z})| \leq |4\bar{z}_1| +|\bar{z}_2\frac{3\bar{z}_2^2-2}{\bar{z}_2^2+2}| \leq4|\bar{z}_1|+3|\bar{z}_2| \leq 4\sqrt{2}\sqrt{\bar{z}_1^2+\bar{z}_2^2} = 4\sqrt{2}\|\bar{z}\|. \end{eqnarray} | (4.3) |
By selecting H_1 = 0.6 , H_2 = 1.7 , and H_3 = 2 , one has
\begin{eqnarray} \frac{\partial V_w (\bar{z})}{\partial \bar{z}}a_\Gamma(\bar{z})& = -2\bar{z}_1^4-8\bar{z}_1^2-4\bar{z}_2^2+4\bar{z}_1\bar{z}_2 \leq -(\bar{z}_1^2+\bar{z}_2^2) = -V_w (\bar{z}), \quad \| \frac{\partial V_w (\bar{z})}{\partial \bar{z}}\|\ \leq 2\|\bar{z}\|. \end{eqnarray} | (4.4) |
Let \alpha_1 = 0.3z^2 and \alpha_2 = 1.5z^2 . Then
\begin{eqnarray} \frac{\partial V_0 (z)}{\partial z}q(z, x) = 2z(-z+0.5(x_1^2+x_2^2)\sin(x_1)+0.5)\leq -(z^2)^\chi+0.5\|x\|^4+(1-\chi)\chi^{\frac{\chi}{1-\chi}}+0.5. \end{eqnarray} | (4.5) |
According to (4.3)–(4.5), it can be observed that input and state unmodeled dynamics satisfy the requirements of Assumptions 4–6.
Remark 4. In order to further study the impact of the control parameters on system response, we choose two groups of different cases for Example 1 in Table 1. In addition, both groups of data is operated with the same other design parameters of \tau_2 = 0.4 , \delta = \delta_1 = 0.3 , \gamma = \gamma_1 = 0.1 , and e_0 = 5 . The final simulation results are shown in Figures 5 and 6. By comparing the responses of the two cases, we can see that the dynamic response of case 1 is better than that of case 2 . On the other hand, by Lemma 2 and (3.44), one can see that the small and convergence rate of system response are affected by the design parameters k_i , \varrho_i , T , and s . When we choose larger prescribed control parameters T and s , then the \aleph(t) in \alpha_1 and controller u are very large. At this time, the values of parameters k_i and \varrho_i in the controller can be selected to be small. Hence, from the perspective of practical application, we need to tradeoff the boundedness of the controller and the convergence time by selecting large k_i , small T , and appropriate \varrho_i and s .
Parameters | Values in case 1 | Values in case 2 |
k_1 , k_2 | 0.5, 0.5 | 4, 4 |
\varrho_1 , \varrho_2 | 0.2, 0.2 | 0.5, 0.5 |
T | 4 | 1.5 |
s | 2 | 2 |
This example is a simplified model representing a boiler-turbine unit in [21] as follows:
\begin{eqnarray} \text{d} z & = & -z+0.5(x_1^2+x_2^2)+0.5, \cr \text{d}x_1 & = & ([x_2]^{p_1(t)}+z\sin(x_1x_2))\text{d}t+0.5x_1^2x_2^2\text{d}w, \cr \text{d}x_2 & = & ((1+x_1^2)\nu+\frac{x_1^2}{1+x_2^2}+0.5zx_1x_2)\text{d}t +0.2x_2 x_1\text{d}w, \cr \text{d}\bar{z}_1 & = & -2\bar{z}_1-\bar{z}_1^3+\bar{z}_2, \ \text{d}\bar{z}_2 = -2\bar{z}_2+[u]^{p_2(t)}, \ \nu = \bar{z}_1+\frac{-\bar{z}_2+4\bar{z}_2^3}{1+\bar{z}_2^2} + [u]^{p_2(t)}, \end{eqnarray} | (4.6) |
where x_1 and x_2 indicate the drum and reheater pressures, respectively. u represents the placement of the control valve, the unknown systems orders are indicated as 1 \leq p_i(t) \leq \frac{7}{5} , i = 1, 2, \; p_1(t) = \frac{8}{7}+\frac{1}{7}\sin t , p_2(t) = \frac{6}{5}+\frac{1}{5}\sin t , \bar{p} = \frac{7}{5} , \underline p = 1 , \underline{h}_1 = \bar{h}_1 = 1 , \underline{h}_2 = 1 , \bar{h}_2 = 1+ x_1^2 , \Gamma_1 = z\sin(x_1x_2) , and \Gamma_2 = 0.5zx_1x_2 .
According to (3.36), the controller, the adaptive law, and the basis functions of NNs are consistent with the structure of Example 1. The design parameters and the initial values are taken as \tau_2 = e^{-t}+0.01 , k_1 = k_2 = 0.7 , \varrho_1 = \varrho_2 = 0.1 , \gamma = \gamma_1 = 0.1 , \delta = \delta_1 = 0.3 , e_0 = 3 , s = 2 , T = 3 , x_1(0) = 0.5 , x_2(0) = 0.2 , \hat{\Theta}(0) = 0.8 , \hat{\Lambda}(0) = -1 , \hbar(0) = 0.2 , z(0) = 1.3 , and \bar{z}(0) = [0.1, 0.1]^T . The control effects are shown by Figures 7 – 9 . Specifically, Figure 7 provides the trajectories of x_1 and x_2 within the prescribed settling time. Figure 8 depicts the actual control input u that can compensate for the effects of the stochastic disturbance and dynamic uncertainties. Figure 9 expresses the curve of adaptive laws \hat{\Theta} and \hat{\Lambda} .
The importance of this thesis lies in its pioneering exploration of the adaptive prescribed-time stabilization issue in stochastic high-order nonlinear systems, where unknown time-varying powers and dynamic uncertainty are prevalent. This study represents the first comprehensive discussion of this challenging problem. A neural predetermined time-varying feedback controller is crafted to avoid the differentiation of virtual controllers and reduce computational burden through the dynamic surface control. The results indicate that all internal signals of the closed-loop system are continuous and bounded, and the equilibrium point is semi-global practical prescribed-time stable in probability. There are still some unresolved issues at present:
(1) We need to find a more comprehensive control scheme that enables the system to achieve global stability without relying on initial values and design parameters, eliminating the impact of unbounded gains.
(2) Note that the dynamic surface control method can generate significant filtering errors and cannot achieve good stability effects. Another future work is to combine command filtering control and eliminate the residual term generated by adaptive control to achieve zero tracking error.
Yihang Kong: Conceptualization, formal analysis, methodology, validation; software, writing original draft, writing review editing. Xinghui Zhang: Conceptualization, formal analysis, methodology, funding acquisition, validation, investigation, writing original draft, writing review editing. Yaxin Huang: Investigation, funding acquisition, validation. Ancai Zhang: Funding acquisition, resources, validation. Jianlong Qiu: Funding acquisition, resources, validation. All authors have read and agreed to the published version of the manuscript.
This work was supported in part by the Taishan Scholar Program of Shandong Province under Grant No. tsqn202211240, the Natural Science Foundation Program of Shandong Province under Grant No. ZR2019YQ28, the Development Plan of Youth Innovation Team of University in Shandong Province under Grant No. 2019KJN007, the Yunnan Provincial Science and Technology Plan Project under Grant No. 202302AD080006, and the China Postdoctoral Science Foundation under Grants No. 2020M682226, 2021T140424.
The authors declare no conflicts of interest.
[1] |
S. Bhat, D. Bernstein, Finite-time stability of continuous autonomous systems, SIAM J. Control Optim., 38 (2000), 751–766. https://doi.org/10.1137/s0363012997321358 doi: 10.1137/s0363012997321358
![]() |
[2] |
A. Polyakov, D. Efimov, W. Perruquetti, Finite-time and fixed-time stabilization: Implicit Lyapunov function approach, Automatica, 51 (2015), 332–340. https://doi.org/10.1016/j.automatica.2014.10.082 doi: 10.1016/j.automatica.2014.10.082
![]() |
[3] |
J. T. Hu, G. X. Sui, X. X. Lu, X. D. Li, Fixed-time control of delayed neural networks with impulsive perturbations, Nonlinear Anal. Model., 23 (2018), 904–920. https://doi.org/10.15388/na.2018.6.6 doi: 10.15388/na.2018.6.6
![]() |
[4] |
D. B. Tong, B. Ma, Q. Y. Chen, Y. B. Wei, P. Shi, Finite-time synchronization and energy consumption prediction for multilayer fractional-order networks, IEEE T. Circuits II, 70 (2023), 2176–2180. https://doi.org/10.1109/tcsii.2022.3233420 doi: 10.1109/tcsii.2022.3233420
![]() |
[5] |
G. Q. Yang, D. B. Tong, Q. Y. Chen, W. N. Zhou, Fixed-time synchronization and energy consumption for Kuramoto-oscillator networks with multilayer distributed control, IEEE T. Circuits II, 70 (2023), 1555–1559. https://doi.org/10.1109/tcsii.2022.3221477 doi: 10.1109/tcsii.2022.3221477
![]() |
[6] |
K. Ding, Q. X. Zhu, T. W. Huang, Prefixed-time local intermittent sampling synchronization of stochastic multicoupling delay reaction-diffusion dynamic networks, IEEE T. Neur. Net. Lear., 35 (2024), 718–732. https://doi.org/10.1109/TNNLS.2022.3176648 doi: 10.1109/TNNLS.2022.3176648
![]() |
[7] |
H. Wang, Q. X. Zhu, Global stabilization of a class of stochastic nonlinear time-delay systems with SISS inverse dynamics, IEEE T. Automat. Contr., 65 (2020), 4448–4455. https://doi.org/10.1109/tac.2020.3005149 doi: 10.1109/tac.2020.3005149
![]() |
[8] |
J. Yu, S. Cheng, P. Shi, C. Lin, Command-filtered neuroadaptive output-feedback control for stochastic nonlinear systems with input constraint, IEEE T. Cybernetics, 53 (2023), 2301–2310. https://doi.org/10.1109/TCYB.2021.3115785 doi: 10.1109/TCYB.2021.3115785
![]() |
[9] |
Z. C. Zhu, Q. X. Zhu, Adaptive event-triggered fuzzy control for stochastic highly nonlinear systems with time delay and nontriangular structure interconnections, IEEE T. Fuzzy Syst., 32 (2024), 27–37. https://doi.org/10.1109/TFUZZ.2023.3287869 doi: 10.1109/TFUZZ.2023.3287869
![]() |
[10] |
F. Wang, B. Chen, Y. Sun, Y. Gao, C. Lin, Finite-time fuzzy control of stochastic nonlinear systems, IEEE T. Cybernetics, 50 (2020), 2617–2626. https://doi.org/10.1109/TCYB.2019.2925573 doi: 10.1109/TCYB.2019.2925573
![]() |
[11] |
H. Min, S. Xu, Z. Zhang, Adaptive finite-time stabilization of stochastic nonlinear systems subject to full-state constraints and input saturation, IEEE T. Automat. Contr., 66 (2021), 1306–1313. https://doi.org/10.1109/TAC.2020.2990173 doi: 10.1109/TAC.2020.2990173
![]() |
[12] |
K. Li, Y. Li, G. Zong, Adaptive fuzzy fixed-time decentralized control for stochastic nonlinear systems, IEEE T. Fuzzy Syst., 29 (2021), 3428–3440. https://doi.org/10.1109/TFUZZ.2020.3022570 doi: 10.1109/TFUZZ.2020.3022570
![]() |
[13] |
Y. G. Yao, J. Q. Tan, J. Wu, X. Zhang, Event-triggered fixed-time adaptive fuzzy control for state-constrained stochastic nonlinear systems without feasibility conditions, Nonlinear Dyn., 105 (2021), 403–416. https://doi.org/10.1007/s11071-021-06633-7 doi: 10.1007/s11071-021-06633-7
![]() |
[14] |
H. Peng, Q. X. Zhu, Fixed time stability of impulsive stochastic nonlinear time-varying systems, Int. J. Robust Nonlin., 33 (2023), 3699–3714. https://doi.org/10.1002/rnc.6589 doi: 10.1002/rnc.6589
![]() |
[15] |
Y. Song, Y. Wang, J. Holloway, M. Krstic, Time-varying feedback for regulation of normal-form nonlinear systems in prescribed finite time, Automatica, 83 (2017), 243–251. https://doi.org/10.1016/j.automatica.2017.06.008 doi: 10.1016/j.automatica.2017.06.008
![]() |
[16] |
W. Q. Li, M. Krstic, Stochastic nonlinear prescribed-time stabilization and inverse optimality, IEEE T. Automat. Contr., 67 (2022), 1179–1193. https://doi.org/10.1109/TAC.2021.3061646 doi: 10.1109/TAC.2021.3061646
![]() |
[17] |
W. Li, M. Krstic, Prescribed-time control of stochastic nonlinear systems with reduced control effort, J. Syst. Sci. Complex, 34 (2021), 1782–1800. https://doi.org/10.1007/s11424-021-1217-7 doi: 10.1007/s11424-021-1217-7
![]() |
[18] |
W. Q. Li, M. Krstic, Prescribed-time output-feedback control of stochastic nonlinear systems, IEEE T. Automat. Contr., 68 (2023), 1431–1446. https://doi.org/10.1109/TAC.2022.3151587 doi: 10.1109/TAC.2022.3151587
![]() |
[19] |
H. Wang, Q. X. Zhu, Finite-time stabilization of high-order stochastic nonlinear systems in strict-feedback form, Automatica, 54 (2015), 284–291. https://doi.org/10.1016/j.automatica.2015.02.016 doi: 10.1016/j.automatica.2015.02.016
![]() |
[20] |
L. Zhang, X. Liu, C. Hua, Prescribed-time control for stochastic high-order nonlinear systems with parameter uncertainty, IEEE T. Circuits II, 70 (2023), 4083–4087. https://doi.org/10.1109/TCSII.2023.3274680 doi: 10.1109/TCSII.2023.3274680
![]() |
[21] |
J. Z. Liu, S. Yan, D. L. Zeng, Y. Hu, Y. Lv, A dynamic model used for controller design of a coal fired once-through boiler-turbine unit, Energy, 93 (2015), 2069–2078. https://doi.org/10.1016/j.energy.2015.10.077 doi: 10.1016/j.energy.2015.10.077
![]() |
[22] |
G. J. Li, X. J. Xie, Adaptive state-feedback stabilization of stochastic high-order nonlinear systems with time-varying powers and stochastic inverse dynamics, IEEE T. Automat. Contr., 65 (2020), 5360–5367. https://doi.org/10.1109/TAC.2020.2969547 doi: 10.1109/TAC.2020.2969547
![]() |
[23] |
F. Shen, X. Wang, X. Yin, Adaptive control based on Barrier Lyapunov function for a class of full-state constrained stochastic nonlinear systems with dead-zone and unmodeled dynamics, T. I. Meas. Control, 43 (2021), 1936–1948. https://doi.org/10.1177/01423312209856 doi: 10.1177/01423312209856
![]() |
[24] |
X. Xia, J. Pan, T. Zhang, Command filter based adaptive DSC of uncertain stochastic nonlinear systems with input delay and state constraints, J. Franklin I., 359 (2022), 9492–9521. https://doi.org/10.1016/j.jfranklin.2022.10.004 doi: 10.1016/j.jfranklin.2022.10.004
![]() |
[25] |
Y. Chen, Y. J. Liu, L. Liu, Event-triggered adaptive stabilization control of stochastic nonlinear systems with unmodeled dynamics, Int. J. Robust Nonlin., 33 (2023), 5322–5336. https://doi.org/10.1002/rnc.6645 doi: 10.1002/rnc.6645
![]() |
[26] |
Y. Shu, Neural dynamic surface control for stochastic nonlinear systems with unknown control directions and unmodelled dynamics, IET Control Theory A., 17 (2023), 649–661. https://doi.org/10.1049/cth2.12221 doi: 10.1049/cth2.12221
![]() |
[27] |
Y. C. Liu, Q. D. Zhu, X. Fan, Event-triggered adaptive fuzzy control for stochastic nonlinear time-delay systems, Fuzzy set. syst., 452 (2023), 42–60. https://doi.org/10.1016/j.fss.2022.07.005 doi: 10.1016/j.fss.2022.07.005
![]() |
[28] |
Y. C. Liu, Q. D. Zhu, Adaptive neural network asymptotic control design for MIMO nonlinear systems based on event-triggered mechanism, Inform. Sciences, 603 (2022), 91–105. https://doi.org/10.1016/j.ins.2022.04.048 doi: 10.1016/j.ins.2022.04.048
![]() |
[29] |
R. H. Cui, X. J. Xie, Finite-time stabilization of stochastic low-order nonlinear systems with time-varying orders and FT-SISS inverse dynamics, Automatica, 125 (2021), 109418. https://doi.org/10.1016/j.automatica.2020.109418 doi: 10.1016/j.automatica.2020.109418
![]() |
[30] |
Y. Liu, Y. Li, W. Li, Output-feedback stabilization of a class of stochastic nonlinear systems with time-varying powers, Int. J. Robust Nonlin., 31 (2021), 9175–9192. https://doi.org/10.1002/rnc.5761 doi: 10.1002/rnc.5761
![]() |
[31] |
H. Li, W. Li, J. Gu, Adaptive tracking control of stochastic nonlinear systems with unknown time-varying powers, J. Intel. Mat. Syst. Str., 43 (2021), 1880–1890. https://doi.org/10.1177/014233122098223 doi: 10.1177/014233122098223
![]() |
[32] |
W. Li, Y. Liu, X. Yao, State-feedback stabilization and inverse optimal control for stochastic high-order nonlinear systems with time-varying powers, Asian J. Control, 23 (2021), 739–750. https://doi.org/10.1002/asjc.2250 doi: 10.1002/asjc.2250
![]() |
[33] |
J. Gu, H. Wang, W. Li, Adaptive state-feedback stabilization for stochastic nonlinear systems with time-varying powers and unknown covariance, Mathematics, 10 (2022), 2873. https://doi.org/10.3390/math10162873 doi: 10.3390/math10162873
![]() |
[34] |
Y. Song, J. Su, A unified Lyapunov characterization for finite time control and prescribed time control, Int. J. Robust Nonlin., 33 (2023), 2930–2949. https://doi.org/10.1002/rnc.6544 doi: 10.1002/rnc.6544
![]() |
[35] |
Y. C. Liu, Q. D. Zhu, Adaptive neural network finite-time tracking control of full state constrained pure feedback stochastic nonlinear systems, J. Franklin I., 357 (2020), 6738–6759. https://doi.org/10.1016/j.jfranklin.2020.04.048 doi: 10.1016/j.jfranklin.2020.04.048
![]() |
[36] | R. Khasminskii, Stochastic stability of differential equations, Berlin: Springer, 2011. https://doi.org/10.1007/978-3-642-23280-0 |
[37] |
Z. P. Jiang, L. Praly, Design of robust adaptive controllers for nonlinear systems with dynamic uncertainties, Automatica, 34 (1998), 825–840. https://doi.org/10.1016/S0005-1098(98)00018-1 doi: 10.1016/S0005-1098(98)00018-1
![]() |
[38] |
M. Arcak, P. Kokotovic, Robust nonlinear control of systems with input unmodeled dynamics, Syst. Control Lett., 41 (2000), 115–122. https://doi.org/10.1016/S0167-6911(00)00044-X doi: 10.1016/S0167-6911(00)00044-X
![]() |
[39] |
F. C. Kong, H. L. Ni, Q. X. Zhu, C. Hu, T. W. Huang, Fixed-time and predefined-time synchronization of discontinuous neutral-type competitive networks via non-chattering adaptive control strategy, IEEE T. Netw. Sci. Eng., 10 (2023), 3644–3657. https://doi.org/10.1109/tnse.2023.3271109 doi: 10.1109/tnse.2023.3271109
![]() |
[40] |
C. C. Chen, C. J. Qian, X. Z. Lin, Z. Y. Sun, Y. W. Liang, Smooth output feedback stabilization for a class of nonlinear systems with time-varying powers, Int. J. Robust Nonlin., 27 (2017), 5113–5128. https://doi.org/10.1002/rnc.3826 doi: 10.1002/rnc.3826
![]() |
[41] |
Q. Chen, D. Tong, W. Zhou, Finite-time stochastic boundedness for Markovian jumping systems via the sliding mode control, J. Franklin I., 359 (2022), 4678–4698. https://doi.org/10.1016/j.jfranklin.2022.05.005 doi: 10.1016/j.jfranklin.2022.05.005
![]() |
[42] |
S. Sui, C. L. P. Chen, S. Tong, Event-trigger-based finite-time fuzzy adaptive control for stochastic nonlinear system with unmodeled dynamics, IEEE T. Fuzzy Syst., 29 (2021), 1914–1926. https://doi.org/10.1109/TFUZZ.2020.2988849 doi: 10.1109/TFUZZ.2020.2988849
![]() |
[43] | R. M. Sanner, J. J. E. Slotine, Gaussian networks for direct adaptive control, In: 1991 American Control Conference, 1991, 2153–2159. https://doi.org/10.23919/ACC.1991.4791778 |
[44] | H. K. Khalil, Nonlinear systems, 2002. |
Parameters | Values in case 1 | Values in case 2 |
k_1 , k_2 | 0.5, 0.5 | 4, 4 |
\varrho_1 , \varrho_2 | 0.2, 0.2 | 0.5, 0.5 |
T | 4 | 1.5 |
s | 2 | 2 |