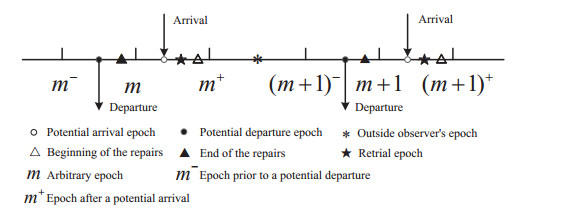
Citation: Shaojun Lan, Yinghui Tang. An unreliable discrete-time retrial queue with probabilistic preemptive priority, balking customers and replacements of repair times[J]. AIMS Mathematics, 2020, 5(5): 4322-4344. doi: 10.3934/math.2020276
[1] | Bharathy Shanmugam, Mookkaiyah Chandran Saravanarajan . Unreliable retrial queueing system with working vacation. AIMS Mathematics, 2023, 8(10): 24196-24224. doi: 10.3934/math.20231234 |
[2] | Yuejiao Wang, Chenguang Cai . Equilibrium strategies of customers and optimal inventory levels in a make-to-stock retrial queueing system. AIMS Mathematics, 2024, 9(5): 12211-12224. doi: 10.3934/math.2024596 |
[3] | Rani Rajendiran, Indhira Kandaiyan . Transient scrutiny of $ M^X/G(a, b)/1 $ queueing system with feedback, balking and two phase of service subject to server failure under Bernoulli vacation. AIMS Mathematics, 2023, 8(3): 5391-5412. doi: 10.3934/math.2023271 |
[4] | Mengrao Ma, Linmin Hu, Yuyu Wang, Fang Luo . Discrete-time stochastic modeling and optimization for reliability systems with retrial and cold standbys. AIMS Mathematics, 2024, 9(7): 19692-19717. doi: 10.3934/math.2024961 |
[5] | Linhong Li, Wei Xu, Zhen Wang, Liwei Liu . Improving efficiency of the queueing system with two types of customers by service decomposition. AIMS Mathematics, 2023, 8(11): 25382-25408. doi: 10.3934/math.20231295 |
[6] | K. Jeganathan, S. Selvakumar, N. Anbazhagan, S. Amutha, Porpattama Hammachukiattikul . Stochastic modeling on M/M/1/N inventory system with queue-dependent service rate and retrial facility. AIMS Mathematics, 2021, 6(7): 7386-7420. doi: 10.3934/math.2021433 |
[7] | Alexander Dudin, Sergey Dudin, Rosanna Manzo, Luigi Rarità . Queueing system with batch arrival of heterogeneous orders, flexible limited processor sharing and dynamical change of priorities. AIMS Mathematics, 2024, 9(5): 12144-12169. doi: 10.3934/math.2024593 |
[8] | Zhen Wang, Liwei Liu, Yuanfu Shao, Yiqiang Q. Zhao . Joining strategies under two kinds of games for a multiple vacations retrial queue with $ N $-policy and breakdowns. AIMS Mathematics, 2021, 6(8): 9075-9099. doi: 10.3934/math.2021527 |
[9] | S. Sundarapandiyan, S. Nandhini . Sensitivity analysis of a non-Markovian feedback retrial queue, reneging, delayed repair with working vacation subject to server breakdown. AIMS Mathematics, 2024, 9(8): 21025-21052. doi: 10.3934/math.20241022 |
[10] | YanLing Li, GenQi Xu, Hao Chen . Analysis of two components parallel repairable degenerate system with vacation. AIMS Mathematics, 2021, 6(10): 10602-10619. doi: 10.3934/math.2021616 |
In the study of the classical queueing theory, it is always assumed that when an arriving customer finds the server temporarily unavailable (e.g., the server may be busy, on vacation or under repair), the customer either joins the waiting line to get service or leaves the service system forever. However, in day-to-day queueing activities, we often meet some congestion situations where the arriving customers who cannot be served in time leave the service zone and join a retrial group (called orbit) to approach the server for their requests after some random length of time. In literature such queueing phenomena are referred to as retrial queues which arise naturally in the real world. Nowadays, retrial queues have been widely and successfully used to model many realistic congestion scenarios such as telephone switching systems, computer and communication systems, packet switching networks and collision-avoidance star local area networks. Taking call center as an example, when a caller finds the line busy, he/she may decide to enter a virtual retrial group and can retry for service again and again after a random amount of time until the line is found available. During the last few decades, a considerable amount of work has been devoted to investigating retrial queueing model. For comprehensive details on retrial queues we refer the reader to the outstanding survey papers by Yang and Templeton [1], Falin [2], Artalejo [3,4], and Kim and Kim [5], and the monographs by Falin and Templeton [6], and Artalejo and Gómez-Corral [7].
In the retrial queueing literature, the previous studies were focused mainly on continuous time setting. However, in recent years, there has been an increasing interest in addressing discrete-time retrial queues. The research of discrete-time retrial queueing systems does have important practical significance in daily life. In modern communication technology, there are numerous systems, such as broadband integrated services digital network (BISDN), time division multiple access (TDMA) and asynchronous transfer mode (ATM), operating in discrete-time environment where the events (e.g., arrival of packets and their forward transmissions) can only occur at regularly spaced epochs. This fact indicates that discrete-time queues are more suitable than their continuous-time counterparts in characterizing the behaviors of data communication and computer networks. The pioneer work concerning discrete-time queue can be traced to Meisling [8]. Detailed analysis and applications of discrete-time queues can be found in the books by Hunter [9], Bruneel and Kim [10], and Woodward [11]. Since there are repeated requests for establishing a communication link among various nodes and repeated transmissions of unsuccessfully received packets, retrial phenomenon is inherited in network communication system which operates in discrete time regime. Yang and Li [12] pioneered the study of discrete-time retrial queue. They investigated a Geo/G/1 retrial queueing system with geometric retrial times by using generating function technique. Based on the fundamental work of Yang and Li [12], many researchers have concentrated on the study of discrete-time retrial queueing model, see e.g., [13,14,15,16,17,18,19], and references therein.
In many real life scenarios such as computer and communication networks, flexible manufacturing system, transportation system and production system, we often encounter the case that the service station may be subject to breakdowns (e.g., arrival of virus to the CPU, hardware breakdowns) when rendering service to customers. The breakdowns of service facilities will result in a temporarily unavailable period of the systems and therefore the performance and the efficiency of these systems will be heavily affected. As a consequence, the managers or manufacturers not only have to face the disruption of production and the loss of products, but also afford the additional repair expense due to the broken machine. Under such circumstances, the research of repairable queueing system is well worth doing from the viewpoint of queueing and reliability theory. Atencia and Moreno [20] considered a discrete-time Geo/G/1 retrial queue where the server may fail during busy period (called active breakdowns). Also, Atencia and Moreno [21] applied the supplementary variables method to examine a discrete-time Geo/G/1 retrial queueing system associated with starting failures (called passive breakdown) and repairs, in which an arriving customer who finds the server is idle must turn on the server and the server may fail to be activated. Wang and Zhao [22] dealt with a discrete-time Geo/G/1 retrial queueing model where all the arriving customers require a first essential service while only some of them may opt for a second optional service. Several other papers on the discrete-time retrial queues with server breakdowns can be referred to [23,24,25].
It is observed from the existing literature that in almost all the models of repairable discrete-time retrial queues (including the above-mentioned models), the authors always assumed that during repair period the repair times can last, without any interruption, from the beginning of the repair until the completion of the repair. But in real-world situations, the repair of the broken server may be interrupted by some casual random factors such as the limited ability of repairman, the failures of repair facility and the delayed supplies of the required device components. On the basis of this fact, in the present study, we introduce the repair replacement discipline into our model. More specifically speaking, when the repair is subject to interruption, a replacement occurs in the remaining repair times and a new repair whose length is independent of the previous repair times commences from the beginning. The introduction of repair replacements makes the considered system more realistic and flexible. Up to now, the study regarding the repair replacement discipline in discrete-time queue can only be found in [26], but it does not take retrial phenomenon into consideration.
A very important class of queues in congestion situations is retrial queues with priorities. The priority queue has received remarkable attention in the queueing literature because different kinds of customers need different quality of service (QoS). Queues with priority subscribers can be used in a wide range of stochastic service systems like manufacturing and production systems, transportation systems, telecommunication industry, computer and communication systems, etc. In recent years, many fruitful theoretical results have been reported in the area of retrial queues with priorities. Atencia [27] analyzed a Geo/G/1 retrial queueing system with priority services in which the arriving customers can decide to go directly to the server expelling out of the system the customer that is currently being served, if any, or to join the retrial orbit. Lan and Tang [28] considered a discrete-time Geo/G/1 retrial queueing system with non-preemptive priority, working vacations and vacation interruption, and derived various crucial performance measures in steady state. More studies on retrial queues with priorities can be referred to [29,30,31,32,33].
Another significant feature in queueing literature is queue with balking behavior proposed by Haight [34]. The balking means that when the arriving customers find that the server is unavailable or the queue is long, they may be discouraged and decide to abandon the system without service. Queueing models with balking phenomenon arise in many real-life congestion problems such as customers' impatience at a telephone switchboard, hospital emergency rooms handling critical patients, machine repair models and perishable goods for storage in inventory systems. Additionally, since a customer who balks is definitely considered to be lost, the balking of customers can greatly lead to the economic loss, which is what the system managers do not want to see. Therefore, it is worth investigating the queues with balking customers. Although the incorporation of balking customers in queueing systems is rather significant, there is only little work concerning discrete-time queues with balking customers in the literature, see [35,36,37,38].
In this paper, we are interested in analyzing a single-server discrete-time Geo/G/1 retrial queueing system with probabilistic preemptive priority and balking customers taking into account starting failures and replacements of repair times. To the best of our knowledge, the present investigation is the first one that deals with such system, and there are some significant differences between our research and the existing works. The contributions and advantages of this paper are stated as follows.
(1) Model. A novel discrete-time Geo/G/1 retrial queueing system is considered. By incorporating probabilistic preemptive priority, balking customers, starting failures and replacements of repair times, this model has much greater flexibility in characterizing some complicated stochastic phenomena.
(2) Methodology and Results. Employing the supplementary variable method and the generating function technique, the probability generating functions of the queue size under different server states are obtained. Various performance measures in steady state are also derived. Some corresponding results for special discrete-time queues are directly obtained by our results.
(3) Numerical Illustrations. Sensitivity analysis is carried out to illustrate the impact of different system parameters on the performance characteristics, which can provide insight to the system managers so as to supervise the operation status of this system and reduce the congestion problem. In addition, we establish a cost function to determine the optimal value of replacement probability η so as to help the system managers or decision-makers regulate the system economically.
A practical justification of the considered model is the following flexible manufacturing system that operates in discrete-time environment where the events (e.g., arrival of components and their processing time) can only occur at regularly spaced epochs. Since diverse components have different processing requirements, the situation with priority levels is often encountered in practice. Assume that there are two types of components (the urgent components and the regular components). The raw components arrive at the system according to a Bernoulli process. If the system is busy processing component upon an arrival, the newly arriving component either interrupts the component in service and occupies the system to begin its own processing immediately with probability p (corresponding to the urgent components), or leaves the service zone and enters a group of blocked components with complementary probability ˉp (corresponding to the regular components). In order to save energy, the system will be turned off once there is no component needing to be processed, and the new arrival must turn on the server to commence its service. Also, the system may be subjected to starting failures. When the system breaks down, it will be fixed immediately. During a repair period, replacements may take place due to the limited ability of repairman (or the failures of repair facility), and the new arrivals may balk with some probability. In this scenario, the flexible manufacturing system, the raw components, the failures of system, and a group of blocked components correspond to the server, the customers, unreliable server, and retrial customers in the queueing terminology, respectively.
The rest of this paper is as follows. In the next section, a detailed description of the mathematical model under consideration is given. Section 3 is dedicated to the steady-state analysis by the supplementary variable method and the generating function technique. We obtain the closed-form expressions for the generating functions of the stationary distribution of the considered system. In Section 4, various important performance indices of our model are given. Further, some special cases of the proposed model are deduced in Section 5. In Section 6, some numerical results for sensitivity analysis are provided. Section 7 focuses on a cost optimization problem. At last, concluding remarks and suggestions for future work are given in Section 8.
Consider a single-server discrete-time Geo/G/1 retrial queueing system with probabilistic preemptive priority, starting failures, balking rule and replacements of repair times. It is well known that the probability of two or more queueing activities occurring simultaneously is zero in continuous-time queueing systems while it is not so in discrete-time queueing systems. In discrete-time queues, the time axis is divided into equal time intervals (called slots) and is marked with 0,1,2,⋯,m,⋯. All the queueing events (arrivals, departures, beginning and ending of repairs, and retrials) are nonnegative integer-valued random variables and only occur around the slot boundaries. As a result, these queueing activities may take place at the same time and the order of occurrence of these events must be stipulated. In this study, it is assumed that the arrivals, the retrials and the beginning of the repairs occur in time interval (m,m+), and the departures and the completion of the repairs occur in time interval (m−,m). That is, we consider an early arrival system (EAS). More details on the EAS policy and related concepts can be referred to [9]. To make it clearer, the various time epochs at which queueing events occur are displayed in Figure 1.
The detailed formulation of our model is described as follows. Throughout this paper, we have ˉx=1−x for any real number x∈[0,1].
Customers arrive at the system according to a Bernoulli process with parameter λ. There is no waiting space in front of the service station. If the server is busy rendering service to a customer upon an arrival, the newly arriving customer either interrupts the customer in service and occupies the server to begin its own service immediately with probability p or leaves the service zone and enters a group of blocked customers (called retrial orbit) with complementary probability ˉp. The interrupted customer joins the orbit and its service resumes from the beginning after some random length of time. It is supposed that the customers in the orbit form a waiting line in accordance with first-come-first-served (FCFS) regime, that is, only the customer (if any) at the head of the orbit is allowed to make retrials to get service. Successive inter-retrial times of any customer are governed by a general distribution with probability distribution function (pdf) {ai}∞i=0 and probability generating function (pgf) A(z)=∑∞i=0aizi. When the server is free and both a new external arrival and a retrial take place at the same time, the customer making retrials gives up the attempt for service and the newly arriving customer has access to the server. In this case, the retrial customer goes back to its position in the retrial queue.
If an arriving customer (external or repeated) finds the server free, he/she must turn on the server to commence his/her service. If the server is switched on successfully (with probability β), the customer is served immediately and leaves the system forever after service completion. Otherwise, if the server is activated unsuccessfully (with complementary probability ˉβ), the server immediately undergoes a repair process and the customer must enter the orbit. The repair times of the broken server are independent and identically distributed with pdf {ri}∞i=1, pgf R(z)=∑∞i=1rizi and n-th factorial moments μ2,n. During a repair period, replacements in the repair times may take place, that is, in every slot of a repair period, a replacement in the remaining repair time occurs with probability η, and does not occur with probability ˉη. The replacement time is assumed to be negligible. After repair completion, the server is as good as new. Moreover, if an external arrival finds that the server is down (under repair), he/she decides either to join the orbit with probability q or leaves the system completely (balking) with probability ˉq.
The service times follow an arbitrary distribution with pdf {si}∞i=1, pgf S(z)=∑∞i=1sizi, and n-th factorial moments μ1,n. It is further assumed that all the random variables involved in our model are independent of each other. Finally, in order to avoid trivial cases and make our system more realistic, we suppose that 0<λ<1, 0<p≤1, 0<β≤1, 0≤q≤1, and 0≤η<1.
The state of the system at time m+ (the epoch immediately after time epoch m) can be designated by the process
{Xm,m∈N}={(Jm,ξJm,m,Nm),m∈N}, | (3.1) |
where Nm denotes the number of repeated customers in the orbit, and Jm represents the state of the server as
Jm={0,the server is free at time m+, 1,the server is busy at time m+, 2,the server is under repair at time m+. | (3.2) |
If Jm=0 and Nm>0, then ξ0,m denotes the remaining retrial time. If Jm=1, then ξ1,m corresponds to the remaining service time of the customer currently being served. If Jm=2, then ξ2,m represents the residual repair time.
After introducing the supplementary variables corresponding to the remaining retrial time, the remaining service time and the remaining repair time, the next state of the system is independent of the past state and only depends on the present state. Therefore, it can be readily shown that {Xm,m∈N} is the Markov chain of our queueing model with state space
Ω={(0,0)}∪{(0,i,k):i≥1,k≥1}∪{(1,i,k):i≥1,k≥0}∪{(2,i,k):i≥1,k≥1}. | (3.3) |
The steady-state probability distributions of the Markov chain {Xm,m∈N} are defined as follows:
π0,0=limm→∞Pr[Jm=0,Nm=0], | (3.4) |
π0,i,k=limm→∞Pr[Jm=0,ξ0,m=i,Nm=k],i≥1,k≥1, | (3.5) |
π1,i,k=limm→∞Pr[Jm=1,ξ1,m=i,Nm=k],i≥1,k≥0, | (3.6) |
π2,i,k=limm→∞Pr[Jm=2,ξ2,m=i,Nm=k],i≥1,k≥1. | (3.7) |
By using the supplementary variable method, we establish the Kolmogorov equations for the stationary distribution of the system under investigation as follows
π0,0=ˉλπ0,0+ˉλπ1,1,0, | (3.8) |
π0,i,k=ˉλπ0,i+1,k+ˉλaiπ1,1,k+ˉλaiπ2,1,k,i≥1,k≥1, | (3.9) |
π1,i,k=δ0,kλβsiπ0,0+ˉλβsiπ0,1,k+1+(1−δ0,k)λβsi∞∑j=1π0,j,k+λβsiπ1,1,k+ˉλβsia0π1,1,k+1+(1−δ0,k)λpsi∞∑j=2π1,j,k−1+(1−δ0,k)λˉpπ1,i+1,k−1+ˉλπ1,i+1,k+(1−δ0,k)λβsiπ2,1,k+ˉλβa0siπ2,1,k+1,i≥1,k≥0, | (3.10) |
π2,i,k=δ1,kλˉβriπ0,0+ˉλˉβriπ0,1,k+(1−δ1,k)λˉβri∞∑j=1π0,j,k−1+λˉβriπ1,1,k−1+ˉλˉβria0π1,1,k+(1−δ1,k)λˉβriπ2,1,k−1+ˉλˉβria0π2,1,k+(1−δ1,k)λqˉηπ2,i+1,k−1+(1−δ1,k)λqηri∞∑j=2π2,j,k−1+(ˉλ+λˉq)ˉηπ2,i+1,k+(ˉλ+λˉq)ηri∞∑j=2π2,j,k,i≥1,k≥1, | (3.11) |
where δi,j is the Kronecker delta, i.e., δi,j={1,i=j,0,i≠j,, and the normalization condition is
π0,0+∞∑i=1∞∑k=1π0,i,k+∞∑i=1∞∑k=0π1,i,k+∞∑i=1∞∑k=1π2,i,k=1. | (3.12) |
In order to derive the solutions of (3.8)–(3.11), we introduce the following generating functions
φ0(x,z)=∞∑i=1∞∑k=1π0,i,kxizk, | (3.13) |
φ1(x,z)=∞∑i=1∞∑k=0π1,i,kxizk, | (3.14) |
φ2(x,z)=∞∑i=1∞∑k=1π2,i,kxizk, | (3.15) |
and the auxiliary generating functions
φ0,i(z)=∞∑k=1π0,i,kzk,i≥1, | (3.16) |
φ1,i(z)=∞∑k=0π1,i,kzk,i≥1, | (3.17) |
φ2,i(z)=∞∑k=1π2,i,kzk,i≥1. | (3.18) |
Multiplying Eq (3.9)–(3.11) by zk, summing over k, and taking into account (3.8), it follows that
φ0,i(z)=ˉλφ0,i+1(z)+ˉλaiφ1,1(z)+ˉλaiφ2,1(z)−λaiπ0,0,i≥1, | (3.19) |
φ1,i(z)=ˉλβzsiφ0,1(z)+λβsiφ0(1,z)+(λz+ˉλa0zβ−λpz)siφ1,1(z)+τ1(z)φ1,i+1(z)+λpzsiφ1(1,z)+λz+ˉλa0zβsiφ2,1(z)+z−a0zλβsiπ0,0,i≥1, | (3.20) |
φ2,i(z)=ˉλˉβriφ0,1(z)+λˉβzriφ0(1,z)+(λz+ˉλa0)ˉβriφ1,1(z)+τ2(z)(ˉηφ2,i+1(z)+ηriφ2(1,z))+(z−a0)λˉβriπ0,0+[(λz+ˉλa0)ˉβ−τ2(z)η]riφ2,1(z),i≥1, | (3.21) |
where τ1(z)=ˉλ+λˉpz, τ2(z)=ˉλ+λˉq+λqz.
Multiplying (3.19)–(3.21) by xi and summing over i gives
x−ˉλxφ0(x,z)=ˉλ(A(x)−a0)(φ1,1(z)+φ2,1(z)−λˉλπ0,0)−ˉλφ0,1(z), | (3.22) |
x−τ1(z)xφ1(x,z)=ˉλβzS(x)φ0,1(z)+λβS(x)φ0(1,z)+λpzS(x)φ1(1,z)+[(λz+ˉλa0)β−λpz2zS(x)−τ1(z)]φ1,1(z)+λz+ˉλa0zβS(x)φ2,1(z)+z−a0zλβS(x)π0,0, | (3.23) |
x−τ2(z)ˉηxφ2(x,z)=ˉλˉβR(x)φ0,1(z)+λˉβR(x)φ0(1,z)+(λz+ˉλa0)ˉβR(x)φ1,1(z)+{[(λz+ˉλa0)ˉβ−τ2(z)η]R(x)−τ2(z)ˉη}φ2,1(z)+τ2(z)ηR(x)φ2(1,z)+(z−a0)λˉβR(x)π0,0. | (3.24) |
Setting x=1 in (3.22)–(3.24) and solving for φ0(1,z), φ1(1,z) and φ2(1,z), we have
λφ0(1,z)=ˉλ(1−a0)(φ1,1(z)+φ2,1(z)−λˉλπ0,0)−ˉλφ0,1(z), | (3.25) |
λz(1−z)φ1(1,z)=ˉλβ(1−z)φ0,1(z)+[τ3(z)β−z(ˉλ+λz)]φ1,1(z)+τ3(z)βφ2,1(z)−(1−z)λβa0π0,0, | (3.26) |
λq(1−z)φ2(1,z)=ˉλˉβ(1−z)φ0,1(z)+τ3(z)ˉβφ1,1(z)+[τ3(z)ˉβ−τ2(z)]φ2,1(z)−(1−z)λˉβa0π0,0, | (3.27) |
where τ3(z)=z+ˉλa0(1−z).
Substituting Eq (3.25)–(3.27) into (3.23) and (3.24), after some tedious algebraic manipulation, we obtain
z(1−z)x−τ1(z)xφ1(x,z)=ˉλβ(1−z)(1−ˉpz)S(x)φ0,1(z)+{[β(1−ˉpz)τ3(z)−pz2]S(x)−z(1−z)τ1(z)}φ1,1(z)+β(1−ˉpz)τ3(z)S(x)φ2,1(z)−(1−z)(1−ˉpz)λβa0S(x)π0,0, | (3.28) |
λq(1−z)x−τ2(z)ˉηxφ2(x,z)=ˉλˉβ(1−z)τ4(z)R(x)φ0,1(z)+ˉβτ3(z)τ4(z)R(x)φ1,1(z)+{[ˉβτ3(z)τ4(z)−τ2(z)η]R(x)−λq(1−z)τ2(z)ˉη}φ2,1(z)−λˉβ(1−z)τ4(z)a0R(x)π0,0, | (3.29) |
where τ4(z)=λq(1−z)+τ2(z)η.
Letting x=ˉλ in (3.22), x=τ1(z) in (3.28) and x=τ2(z)ˉη in (3.29) respectively, it finally leads to
λ(A(ˉλ)−a0)π0,0=−ˉλφ0,1(z)+ˉλ(A(ˉλ)−a0)φ1,1(z)+ˉλ(A(ˉλ)−a0)φ2,1(z), | (3.30) |
(1−z)(1−ˉpz)λβa0S(τ1(z))π0,0=ˉλβ(1−z)(1−ˉpz)S(τ1(z))φ0,1(z)+{[β(1−ˉpz)τ3(z)−pz2]S(τ1(z))−z(1−z)τ1(z)}φ1,1(z)+β(1−ˉpz)τ3(z)S(τ1(z))φ2,1(z), | (3.31) |
λˉβ(1−z)τ4(z)a0R(τ2(z)ˉη)π0,0=ˉλˉβ(1−z)τ4(z)R(τ2(z)ˉη)φ0,1(z)+ˉβτ3(z)τ4(z)R(τ2(z)ˉη)φ1,1(z)+{[ˉβτ3(z)τ4(z)−τ2(z)η]R(τ2(z)ˉη)−λq(1−z)τ2(z)ˉη}φ2,1(z), | (3.32) |
Solving the above system for φ0,1(z), φ1,1(z) and φ2,1(z), we get
φ0,1(z)=(A(ˉλ)−a0)zD0(z)D(z)λˉλπ0,0, | (3.33) |
φ1,1(z)=λβA(ˉλ)(1−ˉpz)S(τ1(z))τ2(z)D1(z)D(z)π0,0, | (3.34) |
φ2,1(z)=λˉβA(ˉλ)zR(τ2(z)ˉη)τ4(z)D2(z)D(z)π0,0, | (3.35) |
where
D(z)=S(τ1(z)){R(τ2(z)ˉη)[pz2ˉβ[λqz+ˉλA(ˉλ)τ4(z)]+Δ1(z)]+λqτ2(z)ˉη[β(1−ˉpz)Δ2(z)−pz2]}+zτ1(z){R(τ2(z)ˉη)[ˉβτ4(z)Δ2(z)−τ2(z)η]−λqˉη(1−z)τ2(z)}, | (3.36) |
D0(z)=S(τ1(z)){λqτ2(z)ˉη[pz−(1−ˉpz)β]−R(τ2(z)ˉη)[λqpz2ˉβ+(β−pzˉβ)τ2(z)η]}+τ1(z){R(τ2(z)ˉη)[τ2(z)η−ˉβzτ4(z)]+λqˉη(1−z)τ2(z)}, | (3.37) |
D1(z)=ηR(τ2(z)ˉη)+λqˉη(1−z), | (3.38) |
D2(z)=pzS(τ1(z))+(1−z)τ1(z), | (3.39) |
Δ1(z)=[(β−pzˉβ)z+(1−ˉpz)βˉλA(ˉλ)]τ2(z)η, | (3.40) |
Δ2(z)=z+ˉλA(ˉλ)(1−z). | (3.41) |
Inserting (3.33)–(3.35) into (3.22), (3.28) and (3.29) gives
φ0(x,z)=A(x)−A(ˉλ)x−ˉλλxzD0(z)D(z)π0,0, | (3.42) |
φ1(x,z)=S(x)−S(τ1(z))x−τ1(z)λβxA(ˉλ)(1−ˉpz)τ1(z)τ2(z)D1(z)D(z)π0,0, | (3.43) |
φ2(x,z)=R(x)−R(τ2(z)ˉη)x−τ2(z)ˉηλˉβˉηxA(ˉλ)zτ2(z)τ4(z)D2(z)D(z)π0,0. | (3.44) |
At this point, the only unknown is π0,0 and it can be determined by the normalization condition π0,0+φ0(1,1)+φ1(1,1)+φ2(1,1)=1. Taking x=z=1 in (3.42)–(3.44) and using L'Hospital rule, we can get
π0,0=D(1)pA(ˉλ)S(ˉλ+λˉp)[βηR(ˉη)+λˉβˉq(ˉη−R(ˉη))], | (3.45) |
where D(1)=S(ˉλ+λˉp)[R(ˉη)[λpqˉβ+ˉλpηA(ˉλ)+(β−pˉβ)η]−λpqˉβˉη]−(ˉλ+λˉp)βηR(ˉη).
From the representation of π0,0, as π0,0>0, we have that D(1)>0 is a necessary condition for the system to be stable. Also, employing Foster's criterion (see [39]), we can show that D(1)>0 is also a sufficient condition for the stability of the system.
We summarize the above results in the following theorem.
Theorem 1. If D(1)>0, the stationary distribution of the Markov chain {Xm,m∈N} has the following generating functions
φ0(x,z)=A(x)−A(ˉλ)x−ˉλλxzD0(z)D(z)π0,0, | (3.46) |
φ1(x,z)=S(x)−S(τ1(z))x−τ1(z)λβxA(ˉλ)(1−ˉpz)τ1(z)τ2(z)D1(z)D(z)π0,0, | (3.47) |
φ2(x,z)=R(x)−R(τ2(z)ˉη)x−τ2(z)ˉηλˉβˉηxA(ˉλ)zτ2(z)τ4(z)D2(z)D(z)π0,0, | (3.48) |
where π0,0 is given by (3.45).
Based on Theorem 1, we can directly derive the marginal generating functions for the number of customers in the orbit under different server states and the generating functions for the number of customers in the orbit and in the system.
Corollary 1. (1) The marginal generating function for the number of customers in the orbit when the server is idle is given by
π0,0+φ0(1,z)=A(ˉλ)K(z)D(z)π0,0, | (3.49) |
where
K(z)=S(τ1(z)){R(τ2(z)ˉη)[pz2ˉβ[λqz+ˉλτ4(z)]+[(β−pzˉβ)z+(1−ˉpz)βˉλ]τ2(z)η]+λqτ2(z)ˉη[β(1−ˉpz)(ˉλ+λz)−pz2]}+zτ1(z){R(τ2(z)ˉη)[ˉβτ4(z)(ˉλ+λz)−τ2(z)η]−λqˉη(1−z)τ2(z)}. | (3.50) |
(2) The marginal generating function for the number of customers in the orbit when the server is busy is given by
φ1(1,z)=(1−S(τ1(z)))βA(ˉλ)τ1(z)τ2(z)D1(z)D(z)π0,0. | (3.51) |
(3) The marginal generating function for the number of customers in the orbit when the server is down (under repair) is given by
φ2(1,z)=1−R(τ2(z)ˉη)1−τ2(z)ˉηλˉβˉηA(ˉλ)zτ2(z)τ4(z)D2(z)D(z)π0,0. | (3.52) |
(4) The probability generating function of the number of customers in the orbit is given by
ϕ(z)=π0,0+φ0(1,z)+φ1(1,z)+φ2(1,z). | (3.53) |
(5) The probability generating function of the number of customers in the system is given by
ψ(z)=π0,0+φ0(1,z)+zφ1(1,z)+φ2(1,z). | (3.54) |
In the previous discussion, the analytical results for the probability generating functions of various queue size distributions for different system states are established. Now some important performance measures of the system in steady state are obtained as follows.
(1) The probability that the system is empty is given by
π0,0=D(1)pA(ˉλ)S(ˉλ+λˉp)[βηR(ˉη)+λˉβˉq(ˉη−R(ˉη))], | (4.1) |
where
D(1)=S(ˉλ+λˉp)[R(ˉη)[λpqˉβ+ˉλpηA(ˉλ)+(β−pˉβ)η]−λpqˉβˉη]−(ˉλ+λˉp)βηR(ˉη). | (4.2) |
(2) The probability that the system is non-empty (occupied) is given by
φ0(1,1)+φ1(1,1)+φ2(1,1)=1−π0,0. | (4.3) |
(3) The probability that the server is idle is given by
π0,0+φ0(1,1)=K(1)pS(ˉλ+λˉp)[βηR(ˉη)+λˉβˉq(ˉη−R(ˉη))], | (4.4) |
where
K(1)=S(ˉλ+λˉp)[R(ˉη)[λpqˉβ+ˉλpη+(β−pˉβ)η]−λpqˉβˉη]−(ˉλ+λˉp)βηR(ˉη). | (4.5) |
(4) The probability that the server is busy is given by
φ1(1,1)=(ˉλ+λˉp)[1−S(ˉλ+λˉp)]βηA(ˉλ)R(ˉη)D(1)π0,0. | (4.6) |
(5) The probability that the server is down (under repair) is given by
φ2(1,1)=λpˉβˉηA(ˉλ)S(ˉλ+λˉp)(1−R(ˉη))D(1)π0,0. | (4.7) |
(6) The expected number of customers in the orbit, denoted by E[W], is given by
E[N]=ϕ′(z)|z=1. | (4.8) |
(7) The expected number of customers in the system, denoted by E[L], is given by
E[L]=ψ′(z)|z=1=E[N]+φ1(1,1). | (4.9) |
(8) The arrival rate to the orbit is given by
POrbit=λˉpφ1(1,1)+λqφ2(1,1). | (4.10) |
(9) The arrival rate to the system is given by
PSystem=λ(π0,0+φ0(1,1)+φ1(1,1))+λqφ2(1,1)=λ(1−ˉqφ2(1,1)). | (4.11) |
(10) The loss probability of a customer due to server breakdowns
PLoss=λˉqφ2(1,1). | (4.12) |
(11) The equilibrium interruption frequency of the service due to preemptive resume is given by
PInterruption=λpφ1(1,1). | (4.13) |
(12) The steady-state availability of the server is given by
PA=1−φ2(1,1). | (4.14) |
(13) The steady-state replacement frequency is given by
PReplacement=ηφ2(1,1). | (4.15) |
(14) According to the well-known Little's formula, the expected sojourn time of a customer in the system, denoted by E[W], is given by
E[W]=E[L]PSystem. | (4.16) |
In this section, some special cases are directly deduced by choosing appropriate values of some critical parameters in our results.
(1) If a0=1 (i.e., without retrials), then A(ˉλ)=1 and our model becomes the discrete-time Geo/G/1 queue with preemptive resume, starting failures, balking customers and replacements of repair times. In this case, the probability generating function of the number of customers in the system is
ψ(z)=[K(z)+(1−S(τ1(z)))βzτ1(z)τ2(z)D1(z)K(z)+λˉβˉηzτ2(z)τ4(z)D2(z)(1−R(τ2(z)ˉη))(1−τ2(z)ˉη)K(z)]π0,0, | (5.1) |
where τ1(z), τ2(z), τ4(z), K(z), K(1), D1(z) and D2(z) are given as previously defined, and
π0,0=K(1)pS(ˉλ+λˉp)[βηR(ˉη)+λˉβˉq(ˉη−R(ˉη))]. | (5.2) |
(2) When p→0, q=1 and η=0 in our model, the queueing system under consideration reduces to the discrete-time Geo/G/1 retrial queue with starting failures. In this case, the probability generating function of the number of customers in the system is
ψ(z)=βA(ˉλ)(1−z)(ˉλ+λz)S(ˉλ+λz)(βS(ˉλ+λz)+ˉβzR(ˉλ+λz))[z+ˉλA(ˉλ)(1−z)]−z(ˉλ+λz)π0,0, | (5.3) |
where π0,0=λ+ˉλA(ˉλ)−ˉβ−λβμ1,1−λˉβμ2,1βA(ˉλ). This result coincides with the corresponding formula presented by Corollary 1(5) of [23] with θ=0.
(3) If q=0 and β=1, then the proposed model becomes the discrete-time Geo/G/1 retrial queue with FCFS preemptive resume discipline. In this case, the probability generating function of the number of customers in the system is
ψ(z)=A(ˉλ)(1−ˉpz)(ˉλ+λz)S(ˉλ+λˉpz)S(ˉλ+λˉpz)[z+ˉλA(ˉλ)(1−ˉpz)]−z(ˉλ+λˉpz)π0,0, | (5.4) |
where π0,0=S(ˉλ+λˉp)(1+ˉλpA(ˉλ))−(ˉλ+λˉp)pA(ˉλ)S(ˉλ+λˉp).
(4) If p=1, q=0 and β=1, then the proposed model is reduced to the discrete-time Geo/G/1 retrial queue with LCFS preemptive resume discipline. In this case, the probability generating function of the number of customers in the system is
ψ(z)=(ˉλ+λz)[S(ˉλ)(1+ˉλA(ˉλ))−ˉλ]S(ˉλ)(z+ˉλA(ˉλ))−ˉλz. | (5.5) |
(5) If p→0, q=0 and β=1, then our system can be reduced to the Geo/G/1 retrial queue with general retrial times. In this case, the probability generating function of the number of customers in the system is
ψ(z)=(1−z)S(ˉλ+λz)(ˉλ+λz)(λ+ˉλA(ˉλ)−λμ1,1)[z+(1−z)ˉλA(ˉλ)]S(ˉλ+λz)−z(ˉλ+λz), | (5.6) |
which is in agreement with the corresponding result given by Corollary 1(4) of [13].
(6) If a0=1, p→0, q=0 and β=1, our system becomes the classical discrete-time Geo/G/1 queue. In this case, the probability generating function of the number of customers in the system is
ψ(z)=(1−λμ1,1)(1−z)S(ˉλ+λz)S(ˉλ+λz)−z, | (5.7) |
which matches with the result obtained by Hunter [9].
In this section, some numerical results are presented to illustrate the effect of various system parameters on the performance measures of our queueing model, such as the probability that the system is empty π0,0, the probability that the server is down φ2(1,1), the loss probability of a customer due to server breakdowns PLoss, and the expected number of customers in the system E[L]. All the computations are done by developing program in MATLAB software and the values of the parameters involved in these numerical examples are selected to satisfy the stability condition D(1)>0. Throughout this section, it is assumed that the service time is governed by a geometric distribution with parameter χ and the inter-retrial times are geometrically distributed with parameter ˉα=1−α, where α is the probability that a repeated customer does not make a retrial in a slot.
In Figures 2–7, we assume that the repair time follows a geometric distribution with parameter σ=0.4. Figures 2 and 3 are depicted to explore the impact of p on π0,0 and E[L] for different values of α. We set default parameters for Figures 2 and 3 as λ=0.2, χ=0.6, σ=0.4, q=0.2, η=0.15, and β=0.7. It can be observed from Figure 2 that for fixed α, π0,0 is insensitive to the change of p, which is due to the Markovian property of geometric service time distribution. On the other hand, as α increases from 0.15 to 0.75, π0,0 shows a decreasing trend. The reason is that as α increases (i.e., the probability that customer does not make retrial increases), the number of customers in the orbit gradually increases, which undoubtedly leads to the decrease of π0,0. From Figure 3, one can see that the mean system size E[L] decreases with the increase of p while increases as α increases, which is in accordance with our expectation. In fact, when α increases, more and more customers accumulate in the retrial orbit, which certainly causes the increase of the mean queue length of system E[L].
Figures 4–7 describe the influence of q on π0,0, E[L], φ2(1,1) and PLoss for different values of β. We set default parameters for Figures 4–7 as λ=0.2, χ=0.6, σ=0.4, p=0.35, η=0.15, and α=0.15. Figure 4 shows that π0,0 slightly decreases with the increment of q for β=0.6 and β=0.8. Actually, as the values of q become larger, the newly arriving customers are more likely to join the system, which in turn reduces the probability that the system is empty. However, when β=1, we observe that π0,0 keeps unchanged with the increase of q. This is due to the fact that for β=1, the idle server is activated successfully with probability 1 and our model is reduced to the corresponding queue without starting failures. Therefore, all arrivals must enter the system regardless of the value of q. Additionally, for a fixed q, π0,0 shows an increasing trend as β increases. This is intuitively true. Actually, as the value of β (i.e., the probability that the idle server is activated successfully) becomes larger, the server can serve more customers, which can obviously lead to the increase of the probability that the system is empty. The reverse effect of q and β on E[L] is plotted in Figure 5. From Figure 6, there is a gradual growth in φ2(1,1) with the increase of q. This could be due to the fact that the increasing q will lead to the excessive accumulation of customers in the orbit, and the system operates under an overload condition, which makes the idle server be prone to breakdowns. Also, it is clear from Figure 6 that φ2(1,1) decreases with the increasing values of β, which matches with the reality. In particular, it is noted that φ2(1,1) is equal to zero when β=1, which matches with our intuition. Moreover, as it is expected in Figure 7, PLoss shows a decreasing trend with the increasing values of q or β. In deed, as q increases, the probability that an arrival joins the queue increases, and hence the probability of loss of customer decreases. Similarly, when β (i.e., the probability that the idle server is activated successfully) increases, the probability that the arrivals enter the system increases, which evidently results in the decrease of the probability of loss of customer PLoss.
In Figure 8, φ2(1,1) is plotted against the parameter η with three types of repair time distributions, i.e., geometric (σ=1/3), deterministic (r3=1), arbitrary (r1=0.2, r3=0.6, r5=0.2). It is easy to be seen that the three distributions have the same mean value 3. The other default parameters for Figure 8 are taken as λ=0.2, χ=0.6, p=0.35, q=0.2, α=0.15, β=0.7. We can observe from Figure 8 that when the repair time follows geometric distribution, the value of φ2(1,1) is the lowest and remains constant with the increase of η, which is due to the Markovian property of geometric distribution. In addition, it is remarkable that η has a significant impact on φ2(1,1) when the repair time is governed by a deterministic distribution. That is, as the replacement probability η increases, the probability that the system is under repair becomes bigger and bigger. This observation suggests that system designers should pay attention to choosing appropriate repair time distribution to avoid the practical congestion situation.
The above sensitivity analysis is highly consistent with the practical situation and our expectation, which not only demonstrates the validity of our queueing model and analytical results, but also can provide managerial insight to the system designers and decision makers so as to reduce the congestion problem encountered in their respective discrete-time queueing systems.
In practice, the operating cost of the system plays a key role in the analysis of economic revenue. Therefore, in order to make the system more profitable, system designers or system managers are usually interested in minimizing the operating cost of unit time. To demonstrate the applicability of the results obtained in the previous discussion, in this section, we develop an expected operating cost function per unit time for the queueing model under investigation in this paper, in which the replacement probability η is a decision variable. Our objective is to determine the optimum value of η, say η∗, so as to minimize the long-run expected operating cost per unit time.
To this end, we first discuss the expected length of a busy cycle period C. Denote by E[B] the expected length of the period that begins at the epoch at which a new arrival finds the system is empty and the server is free and ends at a service completion epoch at which the server becomes idle and the system is empty again. Let E[I] be the expected length of the period that starts at the instant at which the system becomes empty and ends at the instant when the first customer arrives at the empty system. Thus, the expected length of a busy cycle period is given by E[C]=E[B]+E[I]. Since the inter-arrival times follow a geometric distribution with parameter λ, E[I] can be expressed as E[I]=1λ.
Furthermore, by using the argument of an alternating renewal process, we get
π0,0=E[I]E[C], | (7.1) |
which gives
E[C]=E[I]π0,0=1λπ0,0, | (7.2) |
where π0,0 is determined by (3.45).
We now begin to study the cost optimization problem. Let us define the cost elements as follows.
Ch≡ per unit time cost of every customer present in the system;
Ci≡ per unit time cost for keeping the server idle;
Cb≡ per unit time cost for keeping the server busy;
Cr≡ per unit time fixed repair cost for broken server.;
Cs≡ fixed setup cost per busy cycle;
Co≡ fixed cost for the every replacement of repair times.
Utilizing the above cost elements and the corresponding performance measures obtained previously, the total expected cost function per unit time is given by
TC(η)=ChE[L]+Ci(π0,0+φ0(1,1))+Cbφ1(1,1)+Crφ2(1,1)+Cs1E[C]+Coηφ2(1,1). | (7.3) |
One may note that it would be a hard task to solve the cost minimization problem (7.3) by using analytic method because TC(η) is highly non-linear and complex. Here, we use the parabolic method to find the optimum value η∗. The details about the parabolic method can be referred to [40]. According to the polynomial approximation theory, the unique optimum of the quadratic function agreeing with the objective function g(x) at 3-point pattern {x(l),x(m),x(r)} occurs at
x(q)=g(x(l))((x(m))2−(x(r))2)+g(x(m))((x(r))2−(x(l))2)+g(x(r))((x(l))2−(x(m))2)2[g(x(l))(x(m)−x(r))+g(x(m))(x(r)−x(l))+g(x(r))(x(l)−x(m))]. | (7.4) |
The parabolic method uses this approximation to improve the current 3-point pattern by replacing one of its points with an approximate optimum x(q). For the purpose of clarity, the procedures of the parabolic method are described as follows.
Step 1 (Initialization). Choose a starting 3-point pattern {x(l),x(m),x(r)} along with a stopping tolerance ε=10−6, and initialize the iteration counter i=0.
Step 2 (Stopping). If |x(q)−x(m)|≤ε, stop and report approximate optimum solution x(m).
Step 3 (Quadratic fit). Compute a quadratic fit optimum x(q) according to (7.3) and (7.4). If x(q)≤x(m), go to Step 4. If x(q)>x(m), go to Step 5.
Step 4 (Left). If g(x(m)) is less than g(x(q)), then update x(q)→x(l). Otherwise, replace x(m)→x(r), x(q)→x(m). Either way, advance i=i+1, and return to Step 2.
Step 5 (Right). If g(x(m)) is less than g(x(q)), then update x(q)→x(r). Otherwise, replace x(m)→x(l), x(q)→x(m). Either way, advance i=i+1, and return to Step 2.
In the following numerical experiment, we employ the parabolic method to determine the optimum replacement probability η∗.
Example 1. We consider a practical problem concerning the flexible manufacturing system mentioned in Introduction (Section 1). It is assumed that the raw components arrive at the system according to a Bernoulli process with parameter λ. The processing times per component, the inter-retrial times and the repair times are geometrically distributed with parameter χ, ˉα and σ, respectively. The default values of the system parameters and the cost elements are taken as λ=0.2, χ=0.6, σ=0.4, p=0.35, q=0.2, α=0.25, β=0.7, Ch=$35, Ci=$20, Cb=$55, Cr=$15, Cs=$10 and Co=$25. The effect of η on the system operating cost is illustrated in Figure 9. From the information of Figure 9, we observe that there is an optimum value of η to minimize the system cost and we choose the initial 3-point pattern η(l)=0.2, η(m)=0.25 and η(r)=0.3. Applying the parabolic method as mentioned above with the stopping tolerance ε=10−6, after six iterations, one can see from Table 1 that the minimum expected operating cost per unit time converges to the solution η∗=0.259841 with value $102.899274.
No. of iterations | η(l) | η(m) | η(r) | TC(η(l)) | TC(η(m)) | TC(η(r)) | η∗ | TC(η∗) | Tolerance |
0 | 0.200000 | 0.250000 | 0.300000 | 102.986247 | 102.901156 | 102.925387 | 0.263918 | 102.899580 | 0.013918 |
1 | 0.250000 | 0.263918 | 0.300000 | 102.901156 | 102.899580 | 102.925387 | 0.260376 | 102.899279 | 0.003542 |
2 | 0.250000 | 0.260376 | 0.263918 | 102.901156 | 102.899279 | 102.899580 | 0.259925 | 102.899274 | 4.513242×10−4 |
3 | 0.250000 | 0.259925 | 0.260376 | 102.901156 | 102.899274 | 102.899279 | 0.259853 | 102.899274 | 7.166558×10−5 |
4 | 0.250000 | 0.259853 | 0.259925 | 102.901156 | 102.899274 | 102.899274 | 0.259843 | 102.899274 | 9.823264×10−6 |
5 | 0.250000 | 0.259843 | 0.259853 | 102.901156 | 102.899274 | 102.899274 | 0.259842 | 102.899274 | 1.541255×10−6 |
6 | 0.250000 | 0.259842 | 0.259843 | 102.901156 | 102.899274 | 102.899274 | 0.259841 | 102.899274 | 2.152343×10−7 |
In the foregoing study, we considered a discrete-time Geo/G/1 retrial queue with probabilistic preemptive priority, balking customers, starting failures and replacements of repair times. The introduction of replacements in the repair times is an interesting novelty of this investigation. By employing the supplementary variable method and the generating function technique, the closed-form expressions for the probability generating functions of the stationary distributions of different system states, orbit size and system size were obtained. Some performance measures such as the probabilities that the server is free, busy period or down, the mean steady-state system queue length, the mean sojourn time were also derived. Furthermore, sensitivity analysis was carried out by some numerical examples, which can help the system managers better understand the operating characteristics of the system. Finally, we established a cost structure to search for optimal replacement probability for minimizing the system cost. The incorporation of retrial policy, preemptive resume priority, balking behavior, starting failures and replacements of repair times makes our system closer to real-life congestion scenarios and the analysis of this paper can provide potentially practical application in telecommunication systems, flexible manufacturing systems, transportation system, inventory problems, etc.
For future research, one could extend the present work by considering server vacations, multi-optional services, Markovian arrival process (MAP) of customers. It is also interesting to consider the case that the replacement time cannot be negligible.
The authors would like to sincerely thank two anonymous reviewers for their valuable comments and constructive suggestions which are very helpful in improving the presentation of this paper. This research is supported by the Scientific Research Project of Sichuan University of Science and Engineering (No. 2017RCL56), the Opening Project of Sichuan Province University Key Laboratory of Bridge Non-destruction Detecting and Engineering Computing (No. 2019QYJ03), and the National Natural Science Foundation of China (No. 71571127).
The authors declare no conflicts of interest in this paper.
[1] |
T. Yang, J. G. C. Templeton, A survey on retrial queues, Queueing Syst., 2 (1987), 201-233. doi: 10.1007/BF01158899
![]() |
[2] |
G. I. Falin, A survey of retrial queues, Queueing Syst., 7 (1990), 127-167. doi: 10.1007/BF01158472
![]() |
[3] |
J. R. Artalejo, A classified bibliography of research on retrial queues: Progress in 1990-1999, Top, 7 (1999), 187-211. doi: 10.1007/BF02564721
![]() |
[4] |
J. R. Artalejo, Accessible bibliography on retrial queues: Progress in 2000-2009, Math. Comput. Modell., 51 (2010), 1071-1081. doi: 10.1016/j.mcm.2009.12.011
![]() |
[5] |
J. Kim, B. Kim, A survey of retrial queueing systems, Ann. Oper. Res., 247 (2016), 3-36. doi: 10.1007/s10479-015-2038-7
![]() |
[6] | G. I. Falin, J. G. C. Templeton, Retrial Queues, London: Chapman & Hall, 1997. |
[7] | J. R. Artalejo, A. Gómez-Corral, Retrial Queueing Systems: A Computational Approach, Berlin: Springer, 2008. |
[8] | T. Meisling, Discrete-time queueing theory, Oper. Res., 6 (1956), 96-105. |
[9] | J. J. Hunter, Mathematical Techniques of Applied Probability, Vol. 2, Discrete Time Models: Techniques and Applications, New York: Academic Press, 1983. |
[10] | H. Bruneel, B. G. Kim, Discrete-Time Models for Communication Systems Including ATM, Boston: Kluwer Academic Publishers, 1993. |
[11] | M. E. Woodward, Communication and Computer Networks: Modelling with Discrete-Time Queues, California: IEEE Computer Society Press, 1994. |
[12] |
T. Yang, H. Li, On the steady-state queue size distribution of the discrete-time Geo/G/1 queue with repeated customers, Queueing Syst., 21 (1995), 199-215. doi: 10.1007/BF01158581
![]() |
[13] |
I. Atencia, P. Moreno, A discrete-time Geo/G/1 retrial queue with general retrial times, Queueing Syst., 48 (2004), 5-21. doi: 10.1023/B:QUES.0000039885.12490.02
![]() |
[14] |
J. R. Artalejo, I. Atencia, P. Moreno, A discrete-time Geo[X]/G/1 retrial queue with control of admission, Appl. Math. Model., 29 (2005), 1100-1120. doi: 10.1016/j.apm.2005.02.005
![]() |
[15] |
A. K. Aboul-Hassan, S. I. Rabia, F. A. Taboly, Performance evaluation of a discrete-time Geo[X]/G/1 retrial queue with general retrial times, Comput. Math. Appl., 58 (2009), 548-557. doi: 10.1016/j.camwa.2009.03.101
![]() |
[16] |
I. Atencia, I. Fortes, S. Nishimura, et al. A discrete-time retrial queueing system with recurrent customers, Comput. Oper. Res., 37 (2010), 1167-1173. doi: 10.1016/j.cor.2009.03.029
![]() |
[17] |
J. Wang, Discrete-time Geo/G/1 retrial queues with general retrial time and Bernoulli vacation, J. Syst. Sci. Complex., 25 (2012), 504-513. doi: 10.1007/s11424-012-0254-7
![]() |
[18] | I. Atencia-Mc.Killop, J. L. Galán-García, G. Aguilera-Venegas, et al. A Geo[X]/G[X]/1 retrial queueing system with removal work and total renewal discipline, Appl. Math. Comput., 319 (2018), 245-253. |
[19] | S. Gao, X. Wang, Analysis of a single server retrial queue with server vacation and two waiting buffers based on ATM networks, Math. Probl. Eng., 2019 (2019), 1-14. |
[20] |
I. Atencia, P. Moreno, A discrete-time Geo/G/1 retrial queue with server breakdowns, Asia Pac. J. Oper. Res., 23 (2006), 247-271. doi: 10.1142/S0217595906000929
![]() |
[21] |
I. Atencia, P. Moreno, A discrete-time Geo/G/1 retrial queue with the server subject to starting failures, Ann. Oper. Res., 141 (2006), 85-107. doi: 10.1007/s10479-006-5295-7
![]() |
[22] |
J. Wang, Q. Zhao, A discrete-time Geo/G/1 retrial queue with starting failures and second optional service, Comput. Math. Appl., 53 (2007), 115-127. doi: 10.1016/j.camwa.2006.10.024
![]() |
[23] |
I. Atencia, I. Fortes, S. Sánchez, A discrete-time retrial queueing system with starting failures, Bernoulli feedback and general retrial times, Comput. Ind. Eng., 57 (2009), 1291-1299. doi: 10.1016/j.cie.2009.06.011
![]() |
[24] |
J. Wang, P. Zhang, A discrete-time retrial queue with negative customers and unreliable server, Comput. Ind. Eng., 56 (2009), 1216-1222. doi: 10.1016/j.cie.2008.07.010
![]() |
[25] |
S. Gao, Z. Liu, A repairable GeoX/G/1 retrial queue with Bernoulli feedback and impatient customers, Acta Math. Appl. Sin. E., 30 (2014), 205-222. doi: 10.1007/s10255-014-0278-y
![]() |
[26] |
I. Atencia, A discrete-time queueing system with server breakdowns and changes in the repair times, Ann. Oper. Res., 235 (2015), 37-49. doi: 10.1007/s10479-015-1940-3
![]() |
[27] |
I. Atencia, A Geo/G/1 retrial queueing system with priority services, Eur. J. Oper. Res., 256 (2017), 178-186. doi: 10.1016/j.ejor.2016.07.011
![]() |
[28] | S. Lan, Y. Tang, Performance analysis of a discrete-time Geo/G/1 retrial queue with nonpreemptive priority, working vacations and vacation interruption, J. Ind. Manag. Optim., 15 (2019), 1421-1446. |
[29] |
H. Li, T. Yang, Geo/G/1 discrete time retrial queue with Bernoulli schedule, Eur. J. Oper. Res., 111 (1998), 629-649. doi: 10.1016/S0377-2217(97)90357-X
![]() |
[30] | B. D. Choi, J. W. Kim, Discrete-time Geo1,Geo2/G/1 retrial queueing systems with two types of calls, Comput. Math. Appl., 33 (1997), 79-88. |
[31] |
J. Wu, Z. Liu, Y. Peng, A discrete-time Geo/G/1 retrial queue with preemptive resume and collisions, Appl. Math. Model., 35 (2011), 837-847. doi: 10.1016/j.apm.2010.07.039
![]() |
[32] | M. Jain, A. Bhagat, C. Shekhar, Double orbit finite retrial queues with priority customers and service interruptions, Appl. Math. Comput., 253 (2015), 324-344. |
[33] | S. Upadhyaya, Performance prediction of a discrete-time batch arrival retrial queue with Bernoulli feedback, Appl. Math. Comput., 283 (2016), 108-119. |
[34] |
F. A. Haight, Queueing with balking, Biometrika, 44 (1957), 360-369. doi: 10.1093/biomet/44.3-4.360
![]() |
[35] | A. K. Aboul-Hassan, S. I. Rabia, A. Kadry, Analytical study of a discrete-time retrial queue with balking customers and early arrival scheme, Alex. Eng. J., 44 (2005), 911-917. |
[36] |
A. K. Aboul-Hassan, S. I. Rabia, F. A. Taboly, A discrete time Geo/G/1 retrial queue with general retrial times and balking customers, J. Korean Stat. Soc., 37 (2008), 335-348. doi: 10.1016/j.jkss.2008.04.006
![]() |
[37] |
M. Lozano, P. Moreno, A discrete time single-server queue with balking: economic applications, Appl. Econ., 40 (2008), 735-748. doi: 10.1080/00036840600749607
![]() |
[38] |
V. Laxmi, V. Goswami, K. Jyothsna, Analysis of discrete-time single server queue with balking and multiple working vacations, Qual. Technol. Quant. Manag., 10 (2013), 443-456. doi: 10.1080/16843703.2013.11673424
![]() |
[39] |
A. G. Pakes, Some conditions for ergodicity and recurrence of Markov chains, Oper. Res., 17 (1969), 1058-1061. doi: 10.1287/opre.17.6.1058
![]() |
[40] | R. L. Rardin, Optimization in Operations Research, New Jersey: Prentice Hall, 1998. |
1. | Rani Rajendiran, Indhira Kandaiyan, Transient scrutiny of $ M^X/G(a, b)/1 $ queueing system with feedback, balking and two phase of service subject to server failure under Bernoulli vacation, 2022, 8, 2473-6988, 5391, 10.3934/math.2023271 | |
2. | Mridula Jain, Anamika Jain, Genetic algorithm in retrial queueing system with server breakdown and caller intolerance with voluntary service, 2022, 13, 0975-6809, 582, 10.1007/s13198-021-01364-9 | |
3. | Radhika Agarwal, Divya Agarwal, Shweta Upadhyaya, Izhar Ahmad, Optimization of a stochastic model having erratic server with immediate or delayed repair, 2022, 0254-5330, 10.1007/s10479-022-04804-2 | |
4. | Bharathy Shanmugam, Mookkaiyah Chandran Saravanarajan, Unreliable retrial queueing system with working vacation, 2023, 8, 2473-6988, 24196, 10.3934/math.20231234 | |
5. | Bharathi J., Nandhini S., A single server Non-Markovian with non-compulsory re-service and balking under Modified Bernoulli Vacation, 2024, 36, 10183647, 103007, 10.1016/j.jksus.2023.103007 | |
6. | Vaishnawi Shree, Shweta Upadhyaya, Rakhee Kulshrestha, Cost scrutiny of discrete-time priority queue with cluster arrival and Bernoulli feedback, 2024, 61, 0030-3887, 2312, 10.1007/s12597-024-00742-8 | |
7. | K. Vasanthamani, S. Pavai Madheswari, Analysis of a GEO/G/1 queueing system with repeated attempts pre-emptive priority and replacement in repair times, 2024, 46, 10641246, 9249, 10.3233/JIFS-233406 | |
8. | Mengrao Ma, Linmin Hu, Yuyu Wang, Fang Luo, Discrete-time stochastic modeling and optimization for reliability systems with retrial and cold standbys, 2024, 9, 2473-6988, 19692, 10.3934/math.2024961 | |
9. | R. Harini, K. Indhira, Dynamical behaviour and meta heuristic optimization of a hospital management software system, 2024, 10, 24058440, e35937, 10.1016/j.heliyon.2024.e35937 | |
10. | Mridula Jain, Indeewar Kumar, Comparison of (s, S) and (s, Q) type discrete time queueing inventory model and cost optimization using genetic algorithm with some fuzzy interpretation using PFNs, 2024, 16, 2511-2104, 2965, 10.1007/s41870-024-01793-2 | |
11. | Shweta Upadhyaya, Shree Vaishnawi, Divya Agarwal, Izhar Ahmad, Performance Forecasting of Discrete‐Time Priority Retrial Queue With Its Application in Cognitive Radio Networks, 2025, 38, 1074-5351, 10.1002/dac.6136 |
No. of iterations | η(l) | η(m) | η(r) | TC(η(l)) | TC(η(m)) | TC(η(r)) | η∗ | TC(η∗) | Tolerance |
0 | 0.200000 | 0.250000 | 0.300000 | 102.986247 | 102.901156 | 102.925387 | 0.263918 | 102.899580 | 0.013918 |
1 | 0.250000 | 0.263918 | 0.300000 | 102.901156 | 102.899580 | 102.925387 | 0.260376 | 102.899279 | 0.003542 |
2 | 0.250000 | 0.260376 | 0.263918 | 102.901156 | 102.899279 | 102.899580 | 0.259925 | 102.899274 | 4.513242×10−4 |
3 | 0.250000 | 0.259925 | 0.260376 | 102.901156 | 102.899274 | 102.899279 | 0.259853 | 102.899274 | 7.166558×10−5 |
4 | 0.250000 | 0.259853 | 0.259925 | 102.901156 | 102.899274 | 102.899274 | 0.259843 | 102.899274 | 9.823264×10−6 |
5 | 0.250000 | 0.259843 | 0.259853 | 102.901156 | 102.899274 | 102.899274 | 0.259842 | 102.899274 | 1.541255×10−6 |
6 | 0.250000 | 0.259842 | 0.259843 | 102.901156 | 102.899274 | 102.899274 | 0.259841 | 102.899274 | 2.152343×10−7 |
No. of iterations | η(l) | η(m) | η(r) | TC(η(l)) | TC(η(m)) | TC(η(r)) | η∗ | TC(η∗) | Tolerance |
0 | 0.200000 | 0.250000 | 0.300000 | 102.986247 | 102.901156 | 102.925387 | 0.263918 | 102.899580 | 0.013918 |
1 | 0.250000 | 0.263918 | 0.300000 | 102.901156 | 102.899580 | 102.925387 | 0.260376 | 102.899279 | 0.003542 |
2 | 0.250000 | 0.260376 | 0.263918 | 102.901156 | 102.899279 | 102.899580 | 0.259925 | 102.899274 | 4.513242×10−4 |
3 | 0.250000 | 0.259925 | 0.260376 | 102.901156 | 102.899274 | 102.899279 | 0.259853 | 102.899274 | 7.166558×10−5 |
4 | 0.250000 | 0.259853 | 0.259925 | 102.901156 | 102.899274 | 102.899274 | 0.259843 | 102.899274 | 9.823264×10−6 |
5 | 0.250000 | 0.259843 | 0.259853 | 102.901156 | 102.899274 | 102.899274 | 0.259842 | 102.899274 | 1.541255×10−6 |
6 | 0.250000 | 0.259842 | 0.259843 | 102.901156 | 102.899274 | 102.899274 | 0.259841 | 102.899274 | 2.152343×10−7 |