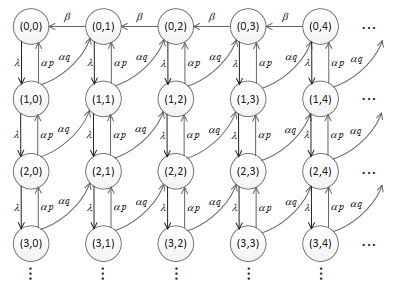
The economic improvements of a queueing system with two types of customers achieved by service decomposition are examined. The service process for a Type 2 customer can be split into two phases: a basic service and an additional service. The basic service rate is equal to that of the Type 1 customer. Additional services can be viewed as orders stored in inventory and processed when the server is idle. Once a new customer arrives during idle time, the server will provide a basic service to the customer upon his/her arrival. That is, customers have preemptive priority for orders stored in inventory. We obtain a two-dimensional unbounded Markov process and apply the multivariate generating function to derive the stationary probability of the proposed model as well as some performance measures. The condition under which performing service decomposition can improve economic efficiency is also obtained. Both the optimal inventory capacity and the minimum system cost are obtained numerically. Numerical experiments demonstrate the impact of optimal inventory setting on economic improvement efficiency. Finally, simulation experiments prove the correctness of our theoretical analysis.
Citation: Linhong Li, Wei Xu, Zhen Wang, Liwei Liu. Improving efficiency of the queueing system with two types of customers by service decomposition[J]. AIMS Mathematics, 2023, 8(11): 25382-25408. doi: 10.3934/math.20231295
[1] | K. Jeganathan, S. Selvakumar, N. Anbazhagan, S. Amutha, Porpattama Hammachukiattikul . Stochastic modeling on M/M/1/N inventory system with queue-dependent service rate and retrial facility. AIMS Mathematics, 2021, 6(7): 7386-7420. doi: 10.3934/math.2021433 |
[2] | Yuejiao Wang, Chenguang Cai . Equilibrium strategies of customers and optimal inventory levels in a make-to-stock retrial queueing system. AIMS Mathematics, 2024, 9(5): 12211-12224. doi: 10.3934/math.2024596 |
[3] | Zhen Wang, Liwei Liu, Yuanfu Shao, Yiqiang Q. Zhao . Joining strategies under two kinds of games for a multiple vacations retrial queue with N-policy and breakdowns. AIMS Mathematics, 2021, 6(8): 9075-9099. doi: 10.3934/math.2021527 |
[4] | Rani Rajendiran, Indhira Kandaiyan . Transient scrutiny of MX/G(a,b)/1 queueing system with feedback, balking and two phase of service subject to server failure under Bernoulli vacation. AIMS Mathematics, 2023, 8(3): 5391-5412. doi: 10.3934/math.2023271 |
[5] | Shaojun Lan, Yinghui Tang . An unreliable discrete-time retrial queue with probabilistic preemptive priority, balking customers and replacements of repair times. AIMS Mathematics, 2020, 5(5): 4322-4344. doi: 10.3934/math.2020276 |
[6] | Bharathy Shanmugam, Mookkaiyah Chandran Saravanarajan . Unreliable retrial queueing system with working vacation. AIMS Mathematics, 2023, 8(10): 24196-24224. doi: 10.3934/math.20231234 |
[7] | Ciro D'Apice, Alexander Dudin, Sergei Dudin, Rosanna Manzo . Study of a semi-open queueing network with hysteresis control of service regimes. AIMS Mathematics, 2025, 10(2): 3095-3123. doi: 10.3934/math.2025144 |
[8] | Xiaolong Li . Asymptotic optimality of a joint scheduling–control policy for parallel server queues with multiclass jobs in heavy traffic. AIMS Mathematics, 2025, 10(2): 4226-4267. doi: 10.3934/math.2025196 |
[9] | Alexander Dudin, Sergey Dudin, Rosanna Manzo, Luigi Rarità . Queueing system with batch arrival of heterogeneous orders, flexible limited processor sharing and dynamical change of priorities. AIMS Mathematics, 2024, 9(5): 12144-12169. doi: 10.3934/math.2024593 |
[10] | Jiaqi Qu, Yunlan Wei, Yanpeng Zheng, Zhaolin Jiang . Fast algorithms for a linear system with infinitesimal generator structure of a Markovian queueing model. AIMS Mathematics, 2025, 10(3): 6546-6559. doi: 10.3934/math.2025299 |
The economic improvements of a queueing system with two types of customers achieved by service decomposition are examined. The service process for a Type 2 customer can be split into two phases: a basic service and an additional service. The basic service rate is equal to that of the Type 1 customer. Additional services can be viewed as orders stored in inventory and processed when the server is idle. Once a new customer arrives during idle time, the server will provide a basic service to the customer upon his/her arrival. That is, customers have preemptive priority for orders stored in inventory. We obtain a two-dimensional unbounded Markov process and apply the multivariate generating function to derive the stationary probability of the proposed model as well as some performance measures. The condition under which performing service decomposition can improve economic efficiency is also obtained. Both the optimal inventory capacity and the minimum system cost are obtained numerically. Numerical experiments demonstrate the impact of optimal inventory setting on economic improvement efficiency. Finally, simulation experiments prove the correctness of our theoretical analysis.
Classical queueing-inventory systems often assume that when customer service is complete, the system inventory level decreases accordingly. When inventory is depleted, replenishment orders are then triggered. This replenishment is provided by an external supplier. We recommend Marand et al. [1] to readers interested in a comprehensive look at the queueing-inventory system. Furthermore, the service system can also produce the products by itself. We want to highlight the preliminary service (PS) that is performed by servers during idle periods to generate stockable inventory items and consumed as the customer's service is completed. PS is first introduced in Hanukov et al. [2], which is executed when the server is empty for the customer. The authors gave several cases where executing PS during the server's idle time can increase the idle time fraction of the system. Hanukov et al. [3] extended the research of Hanukov et al. [2] and analyzed the performance improvement of the PS on the service system in which the inventory produced by PS deteriorates while in storage, creating spoilage costs. The other one is a complementary service that can be conducted without customers. Complementary service is presented for the first time in Hanukov [4]. The service is divided into two phases: the opening service and the complementary service. The customer's complementary service is stored in the inventory as a pending order that is processed when the server is dormant. They compared the proposed model with the status quo model under different cost scenarios regarding efficiency improvement. The above two services can reduce the overall sojourn time of the customer by performing a part of the service when the server is dormant.
Malachowski and Simonini [5] pointed out that time wasted by employees at work was still costing companies billions, which further emphasized the importance of improving the efficiency of service systems. Using the system' idle time to perform other services is prevalent for service systems to improve efficiency. Many authors proposed "vacation" models to address the service system issue. When the system is empty, the server does not remain dormant but handles service-related or other auxiliary tasks (e.g., maintenance, repair, or organization). Doshi [6] gave some methods and decomposition results of the queueing systems with vacation. Ke [7] investigated an M/G/1 queueing system in which the authors analyzed the optimal setting of thresholds for the server to take vacations. Zhang et al. [8] studied a queueing-inventory system with server vacations. The authors assumed that the server goes for multiple vacations once the system inventory is depleted. Due to vacations, the servers are not immediately available to serve customers who arrive during vacations. To reduce the impact of vacations on the system's primary services, many authors have addressed this by limiting the number of vacations (Meena et al. [9] and Ke [10]) or introducing working vacations to queueing systems (Laxmi et al. [11] and Tian [12]). The above articles all assumed that the tasks performed by the server during the vacation periods are not related to the primary task. Hanukov et al. [2,3,4] also studied the utilization of the idle time to improve the efficiency of service systems. In contrast, the work done by the server during idle periods is related to the primary task and can reduce the customer's overall sojourn time.
In this article, we study queueing systems with two types of customers. There are already well-established theoretical results for queueing systems with multiple classes of customers. Blanc et al. [13] described an M/M/c queueing system with two arrival streams. One type of customer can be rejected for entry. They also obtained a stationary admission policy to maximize the discounted cost function. Turhan et al.[14] also studied the optimal admission policy for a queueing system with two types of customers. When the system is full, the arrival of a Type 1 customer terminates the service of a Type 2 customer. The authors gave the admission policy for Type 2 customers in a threshold style. Kim and Kim [15] investigated a retrial queueing system with two classes of customers in which class-2 customers enter the retrial orbit when the system is busy, and class-1 customers form an infinite queue in the system. Both the waiting time distributions of class-1 and class-2 customers are obtained. We place particular emphasis on Hanukov[16]. The author studied a queueing-inventory model with two types of customers: skeptical and trusting customers. In this article, the trusting customer's service is divided into two phases: opening service and complementary service. A complementary service is stored in the system to be processed when the server is idle. Customers who insist on receiving the complete service are defined as skeptical customers. The system stores a finite number of complementary services. Once the system is fully stocked, both types of customers receive full services with the same service rate. The author compared the proposed model with a classical M/M/1 model regarding economic efficiency.
In this work, we investigate improving the efficiency of a service system with two types of customers by service decomposition. Hanukov[16] also studied a queueing system with two types of customers and finite inventory capacity. The author assumed that skeptical customers refuse to decompose their services when the inventory is not full. Once the inventory is full, all customers are considered skeptical and served at the same rate. In this article, we also investigate a queueing model with limited inventory capacity and two types of customers, Model 3. We assume that the complete service rate differs for the two customer types. Before the system inventory is full, all customers are served at the same rate. After a Type 2 customer leaves, the system inventory increases by one. Once the inventory is full, the service process of Type 2 customers is no longer decomposed. Both types of customers leave the system after receiving the complete service with different service rates. The whole model becomes a queueing-inventory model. Our model is mostly motivated by the following examples. Customers choose the necessary products in a furniture company and settle at the cashier. Customers who only purchase small items leave the system after checkout (Type 1 customers); customers who purchase large items need the merchant to provide delivery service after checkout (Type 2 customers). The service of Type 2 customers can be divided into two phases: one is the checkout process and the other is delivery. Another example of service decomposition is ultrasound in hospitals. The whole ultrasound process includes the examination and the report's writing afterward. In some primary hospitals, the doctor writes a report immediately after examining the patient. The patient can leave the hospital at once with the report. In some tertiary hospitals, however, the doctor will finish the examination for all waiting patients and then write the report at leisure.
The major scientific contributions made in this paper can be summarized in the following:
(1) We study a queueing system with two types of customers. In this case, the service for Type 2 customers can be decomposed into two phases. We obtain closed-form expressions for the stationary distribution of the queueing system with service decomposition applied and explicit expressions to the system performance measures.
(2) Model 1 is a two-unbounded queueing system, which is rare in the literature. Combing the probability generating function method with the multivariable L′Hˆopital rule, we derive the explicit expression of the mean number of stored orders in the system. This approach has been introduced for the first time in [4].
(3) The performance measures for the queueing model without service decomposition are derived by the probability generating function. After constructing an appropriate cost function, we obtain the condition for determining the adoption of the service decomposition. Under this condition, service decomposition should be provided for all Type 2 customers. In addition, the fraction of system idle time can become larger under a specific condition by service decomposition.
(4) We propose a queueing system with service decomposition and finite inventory capacity. Taking the inventory capacity as the independent variable and using an optimization algorithm, we can obtain the optimal inventory capacities and the minimum system cost under different parameter settings. Moreover, we also show the effect of each parameter on the optimal values through numerical examples. The economic improvements of the queueing system with service decomposition and optimal capacity are also analyzed numerically.
The rest of this paper is organized as follows. In Section 2, we give a brief description of the proposed model and obtain the closed-form expressions for the stationary probabilities and some system performance measures. In Section 3, we obtain the performance measures of the original system. An analysis of model selection in extreme cases is obtained by employing the constructed cost function. Section 4 includes a steady-state analysis of the limited capacity queueing model and an economic comparison with the previous two models. In order to validate our analysis results, comparisons of simulation and theoretical results are presented in Section 5. Finally, a summary is given in Section 6.
In this section, we thoroughly examine the Model 1 obtained by performing the service decomposition for all Type 2 customers. Furthermore, we obtain the system performance measures explicitly.
We consider an M/M/1 queueing system with two types of customers. The customers' arrival process is a Poisson process with rate λ. The service time of Type 1 customers is exponentially distributed with mean 1/α. The full service time of Type 2 customers follows an exponential distribution with mean 1/μ. In the previous literature, customer service is continuous. Even with staged service, customers are served on a global first-come, first-served order. However, we split the service of Type 2 customers into two phases: basic service and additional service. The basic service rate is equal to the service rate of Type 1 customers α. Additional services are stored in inventory as pending orders, waiting to be processed when the server is idle. After performing service decomposition for all Type 2 customers, a Type 2 customer receives a basic server and leave the system (so effectively acting as a Type 1 customers). The difference is that the departure of a Type 1 customer does not result in an increase in the number of additional services stored in inventory. Therefore, all customers can be seen as leaving the system immediately after receiving the basic service. Additionally, we assume that the proportion of Type 2 customers among all customers is q. Thus, after the customer's basic service is completed, the system inventory is increased by 1 with probability q. The additional service time is assumed to be exponentially distributed with rate β. We do not assume that the total mean duration of a decomposed service 1/α+1/β equals to the average full service time for a Type 2 customer 1/μ. See Section 3.1.2. The stored orders are executed only when no customers are in the system. Otherwise the server delays additional services and holds the orders in the inventory. In addition, when a customer arrives at a busy server processing the additional services, the undergoing service is interrupted immediately and the newly arriving customer receives service instead. In other words, customers have preemptive priority over stored orders. Therefore, the queueing behavior of customers in the system is consistent with the conventional M/M/1 queueing model with the traffic intensity λ/α.
Denote the number of customers and the stored orders in the system at time t by N1(t) and I1(t), respectively. Then, X1(t)={(N1(t),I1(t)),t≥0} is a two-dimensional continuous-time Markov process with the state space Ω1={(n,i),n,i∈N}. The transition situations between the states are shown in Figure 1. When the traffic intensity ρ=λα+λqβ=λβ+λαqαβ<1, the two-unbonded Markov process {X1(t),t≥0} is irreducible and recurrent with invariant probability p1n,i,(n,i)∈Ω1.
The steady-state probability p1n,i is defined as
p1n,i=limt→∞Pr(N1(t)=n,I1(t)=i),(n,i)∈Ω1. | (2.1) |
The balance equations between the states are as follows:
λp10,0=βp10,1+pαp11,0, | (2.2) |
(α+λ)p1n,0=pαp1n+1,0+λp1n−1,0,n≥1, | (2.3) |
(λ+β)p10,i=βp10,i+1+pαp11,i+qαp11,i−1,i≥1, | (2.4) |
(λ+α)p1n,i=λp1n−1,i+pαp1n+1,i+qαp1n+1,i−1,n≥1,i≥1. | (2.5) |
The probability generating function is defined as H(z,w)=∑∞n=0∑∞i=0p1n,iznwi, which is a multivariate function. The corresponding partial probability generating function is defined as Hi(z)=∑∞n=0p1n,izn,i≥0. We can obtain a set of equations in the following by multiplying the Eqs (2.2)–(2.5) by zn and summing over all n.
[(λ+α−λz)z−pα]H0(z)=(αz−pα)p10,0+βzp10,1, | (2.6) |
[(α+λ−λz)z−pα]Hi(z)−qαHi−1(z)=(αz−βz−pα)p10,i−qαp10,i−1+βzp10,i+1,i≥1. | (2.7) |
We also define a generating function of boundary probabilities p10,i,i≥0 as B0(w)=∑∞i=0p10,iwi. Same as before, we multiply the Eq (2.6) by w0 and Eq (2.7) by wi. Then, after arranging, we get the probability generating function has the following form:
H(z,w)=[(αz−βz−pα−qαw)w+βz]B0(w)+βz(w−1)p10,0w[(α+λ(1−z))z−pα−qαw]. | (2.8) |
Note that there are two unknowns in Eq (2.8): B0(w) and p10,0. In order to calculate p10,0, we should first point out that when the system is stable, the inflow and outflow between the two state sets are balanced. Then, we focus on the transitions between rows in Figure 1. The balance equations between rows can be summarized as follows:
λp1n,.=qαp1n+1,.+pαp1n+1,.=αp1n+1,.,n=0,1,2,⋯, | (2.9) |
where p1n,.=∑∞i=0p1n,i. Summing the Eq (2.9) over all n, we obtain p10,.=1−λα. Then, we focus on the transitions between volumes. Same as the previous operation, the balance equations between volumes can be summarized as follows:
qα(p1.,i−p10,i)=βp10,i+1,i=0,1,2,⋯, | (2.10) |
Summing the Eq (2.10) over all i, we obtain
p10,0=(α−λ)β−qαλαβ. | (2.11) |
It should be reminded that we cannot get the explicit expression of B0(w). However, we will prove that any order partial derivatives of B0(w) can be derived in a particular procedure which will be shown later. Although B0(w) is an unknown function, we still give the expression for H(z,w) as follows by substituting Eq (2.11) into Eq (2.8):
H(z,w)=[(αz−βz−pα−qαw)w+βz]αB0(w)+z(w−1)((α−λ)β−λqα)αw[(α+λ(1−z))z−pα−qαw]. | (2.12) |
Under the stability condition, we can derive several performance measures in following.
Theorem 2.1. The mean number of customers in the system E(N1) is
E(N1)=λα−λ. | (2.13) |
Proof. The probability generating function is defined as H(z,w)=∑∞n=0∑∞i=0p1n,iznwi. The mean number of customers in the system can be calculated by ∂H(z,w)∂z|z=1,w=1. It leads to 00. We apply the multivariable L′Hˆopital rule developed by Lawlor [17] to it at the fixed direction v=(1,0). The result is obvious.
Remark 1. Actually, as the server stop processing the stored orders when the customers arrive at the system, the queueing behavior of customers in this system is no different from the conventional M/M/1 queueing system with service intensity λ/α. Therefore, E(N1) is equal to the mean queue length of conventional M/M/1 queueing system with traffic intensity λα .
Theorem 2.2. The mean number of stored orders in the system E(I) is
E(I1)=∂H(z,w)∂w|z=1,w=1=λq(λβ+α2−λαp)(α−λ)(αβ−λ(β+qα)). | (2.14) |
Proof. According to the properties of multivariate probability generating function, the mean number of stored orders in the system E(I1)=∂H(z,w)∂w|z=1,w=1. Obviously, the partial derivative of the numerator with respect to w needs to use the expression of B′0(w). Since B0(w) is not an explicit expression, we first need to obtain the expression of B′0(w).
As mentioned before, we use the multivariable L′Hˆopital rule to calculate E(N1) by taking derivations with respect to a fixed direction →v1=(1,0). We can also apply the multivariable L′Hˆopital to obtain E(N1) via a different direction →v2=(0,1). The following symbols are used to simplify the expression: H(z,w)=B+CA, where
A=αw[(α+λ(1−z))z−pα−qαw], | (2.15) |
B=[(αz−βz−pα−qαw)w+βz]αB0(w), | (2.16) |
C=z(w−1)((α−λ)β−λqα). | (2.17) |
Then, we apply the multivariable L′Hˆopital rule twice to ∂(B+C)∂zA−∂A∂z(B+C)A2 via the fixed direction →v2=(0,1), which is the partial derivative of the above numerator and denominator with respect to w. After substituting z=1,w=1 into the result, the mean number of customers in the system E(N1) also has the following expression:
E(N1)=(αβ−λβ−λαq)B′0(w=1)+λq(λ−αp)q2α2. | (2.18) |
By comparing the Eqs (2.13) and (2.18), we can derive that
B′0(w=1)=λq[(α−λ)2+λqα](α−λ)[αβ−λ(β+qα)]. | (2.19) |
Then, E(I1)=∂H(z,w)∂w|z=1,w=1 also leads to 00. Applying the multivariable L′Hˆopital rule twice to the equation in direction →v2=(0,1), the mean number of stored orders in the system E(I1) is given by
E(I1)=(β+qα)B′0(w=1)−λqqα. | (2.20) |
Finally, by substituting the Eq (2.19) into Eq (2.20), the proof is concluded.
Denote the mean sojourn time of a customer in the system by E(S1). Using the Little's law, we can obtain E(S1) as: E(S1)=E(N1)/λ. Similarly, we can obtain the mean sojourn time of a stored order in the system E(So)=E(I1)/αe, where αe represents the actual arrival rate of the stored orders. Obviously, αe=qα(1−p10,.)=λq is also the arrival rate of the Type 2 customers when the system is stable. As for the steady-state probabilities p1n,i,(n,i)∈Ω1, we can calculate them according to the properties of the multivariate probability generating function H(z,w) as follows:
p1n,i=∂n+iH(z,w)∂nz∂iw|z=0,w=0⋅(n!i!)−1. | (2.21) |
It should be noted that the values of derivatives of B0(w) at w=0: B(k)0(w=0),k=0,1,2,⋯,i must be known before calculating p1n,i. We have obtained B0(w=0)=p10,0. In Theorem 2.2, we apply the multivariable L′Hˆopital rule to calculate B′0(w=1). Similarly, we can obtain B(k)0(w=0), k=0,1,2,⋯,i by applying the multivariable L′Hˆopital rule. Although this method is cumbersome, it is feasible.
Now, we focus on the conditional expected sojourn time T(k,j) for a stored order when it enters the inventory with k customers in the system and another j−1 orders queueing before it. We denote this order as a tagged order. Based on the transition diagram, we can obtain the following theorem.
Theorem 2.3. If the system state is (k,j−1) when this tagged order is created, its conditional expected sojourn time T(k,j) can be obtained as
T(k,j)=kα−λ+j⋅αβ(α−λ),k≥0,j≥1. | (2.22) |
Proof. Before proving this theorem, we first introduce the concept of k-order busy period Mk for a classical M/M/1 queueing system. Mk refers to the duration from when there are K customers in the system to when there are no customers in the system. From Cohen [18], the Mk has the following expression: Mk=kα−λ,k=1,2,3,⋯. The conditional sojourn time of an order, given that it sees the state (0,j−1) when it enters the inventory, can be calculated in the following. For j=1, this order will be processed at first when the server is idle. Since the customer's basic service has preemptive priority over the additional service of the orders stored in inventory, additional service is interrupted by the arrival of a customer. Customers arriving during this basic service time will also be served. The time period from an order is interrupted until the server resumes additional service for the order can be viewed as the 1-order busy period M1 of the M/M/1 queueing system. We obtain the T(0,1) as follows:
T(0,1)=1λ+β+λλ+β(M1+T(0,1))=1β+λβM1=αβ(α−λ). |
If arrival occurs before service completion with probability λλ+β, the order must wait an entire busy period M1 before being served again. If no customer arrives while the order is being processed, then the order is directly served without interruption.
For j≥2, the average sojourn time T(0,j) is obtained as
T(0,j)=1λ+β+λλ+β(M1+T(0,j))+βλ+βT(0,j−1)=j⋅αβ(α−λ). | (2.23) |
When the tagged order is created, there are k customers present in the system. Then, these k customers, as well as new arrivals during the service period, will be served before the server can process the inventory orders. This period can be regarded as the k−order busy period Mk of the classical M/M/1 queueing model. We can use the same method to obtain T(k,j), k≥1, j≥1.
T(k,j)=Mk+T(0,j)=kα−λ+j⋅αβ(α−λ). | (2.24) |
The proof is completed.
Remark 2. The conditional expected sojourn time for an order can help Type 2 customers understand the status of the order. Type 2 customers are homogeneous and risk neutral. A risk neutral customer will maximize his/her revenue by considering the waiting costs of additional services. Excessive inventory orders will delay the completion of additional service for Type 2 customers. Therefore, the inventory capacity of the system is limited when Type 2 customers can choose whether to accept the service decomposition or not.
In order to determine whether performing service decomposition for Type 2 customers improves the economic efficiency of the system, we further investigate the original model without service decomposition. If we do not split the service of Type 2 customers into two phases, the system is simplified to a classical M/M/1 queueing system with two types of customers in which all customers join a single waiting queue. Finally, we will compare the cost functions of the two models and obtain the condition for performing the service decomposition. Unlike Model 1, the customers' service in this system is continuous. The service time of the Type 1 customer follows an exponential distribution with parameter α. The service time of the Type 2 customer is exponentially distributed with mean 1/μ. Since all customers join a single waiting queue, the server can only recognize the type of the customer at the head of the queue. The probability that the customer at the head of the queue is a Type 1 customer is p. Denote the number of customers in the system at time t by N2(t). Denote the state of server at time t by I2(t), where
I2(t)={0,the server is idle at timet,1,the server is busy with a Type 1 customer at timet,2,the server is busy with a Type 2 customer at timet. | (3.1) |
The process X2(t)={(N2(t),I2(t)),t≥0} is also a continuous-time Markov process with the state space Ω2=(0,0)∪{(n,i),n=0,1,2,⋯,i=1,2}. The transitions between states are shown in Figure 2.
The steady-state probability p2n,i is defined by
p2n,i=limt→∞Pr(N2(t)=n,I2(t)=i),(n,i)∈Ω2. | (3.2) |
Arranging the Markov process in lexicography sequence, we obtain the following infinitesimal generator:
G=(M0N0L0MNLMN⋱⋱⋱), | (3.3) |
where
L=(αpαqμpμq),M=(−(λ+α)p00−(λ+μ)),N=(λ00λ), | (3.4) |
M0=−λ,N0=(λpλq),L0=(αμ)′. | (3.5) |
Applying the mean drift method, we can obtain the steady state condition for Model 2 as: λ<μααq+μp. The steady condition can be rewritten as 1λ>qμ+pα, which can be interpreted as the arrival interval (1λ) is greater than the average service time (qμ+pα). The balance equations between the above states can be written as follows:
λp20,0=μp21,2+αp21,1, | (3.6) |
(λ+α)p21,1=λpp20,0+μpp22,2+αpp22,1, | (3.7) |
(λ+μ)p21,2=λqp20,0+μqp22,2+αqp22,1, | (3.8) |
(λ+α)p2n,1=λp2n−1,1+μpp2n+1,2+αpp2n+1,1,n≥2, | (3.9) |
(λ+μ)p2n,2=λp2n−1,2+μqp2n+1,2+αqp2n+1,1,n≥2. | (3.10) |
Define the partial generating function of the states as: Gi(z)=∑∞n=1p2n,izn,z∈(0,1)i=1,2. By simple algebraic manipulation, the partial generating function Gi(z) have the following expression:
G1(z)=−p20,0⋅λp(1−z)z((λ+μ)z−λz2)(−λz2+(λ+μ)z−μq)(−λz2+(λ+α)z−αp)−αμpq, | (3.11) |
G2(z)=−p20,0⋅λq(1−z)z((λ+α)z−λz2)(−λz2+(λ+μ)z−μq)(−λz2+(λ+α)z−αp)−αμpq. | (3.12) |
Combining with the nominal condition G1(1)+G2(1)+p20,0=1, the probability that the system is idle without customers can be obtained as
p20,0=μα−λ(αq+μp)μα. | (3.13) |
In order to compare the original system with Model 1, it is also necessary to give the expression of the expected queue length. According to the properties of the generating function, the number of customers in the system can be expressed as
E(N2)=2∑i=1∞∑n=1np2n,i=G′1(1)+G′2(1)=λ[λpq(α−μ)2+αμ(αq+μp)]αμ(αμ−λ(αq+μp)). | (3.14) |
In this part, we mainly focus on analyzing the effect of service decomposition among Models 1 & 2. We should emphasize that both the stationary condition of the two models should be satisfied λ<min(αβqα+β,αμqα+pμ). Another condition that should be provided is α>μ. Otherwise, service decomposition has only negative effects. Under the above two conditions, we compare the cost function of Models 1 & 2 and give the condition under which the manager prefers to adopt the idea of service decomposition.
Two cost components are considered: the waiting cost rate of customers in the system, c and the inventory holding cost rate, h. It is evident that the Model 2 does not have the inventory holding cost. The total expected cost of the queueing-inventory system (Model 1) and the queueing system (Model 2) are denoted by C1 and C2
C1=cE(N1)+hE(I1), | (3.15) |
C2=cE(N2). | (3.16) |
Proposition 3.1. Considering the above two cost components, management with Model 1 is more profitable if and only if the following condition is satisfied
κ1≡hc<E(N2)−E(N1)E(I1)=(α−μ)((α−λ)(α−μ)λp+α2μ)(αβ−λ(αq+β))αμ(αμ−λ(αq+μp))(λβ+α2−λαp)≡Δ1. | (3.17) |
From Proposition 3.1, if Δ1>0, we can conclude that applying service decomposition to Type 2 customers reduces the queue length but simultaneously increases the holding cost of inventory orders. Moreover, whether to adopt the service decomposition strategy proposed in Model 1 depends only on Eq (3.17). We further examine the improvement achieved by Model 1 compared with Model 2. The improvement is evaluated in terms of the percentage reduction in the total expected cost and is given by
ϵ1=C2−C1C2=cE(N2)−cE(N1)−hE(I1)cE(N2). | (3.18) |
Proposition 3.2. The percentage reduction ϵ1 in the total cost of Model 1 can be written in the following form:
ϵ1=(1−αμ[αμ−λ(qα+pμ)](α−λ)[λpq(α−μ)2+αμ(αq+μp)])(1−κ1Δ1). |
Proof.
ϵ1=E(N2)−E(N1)E(N2)−hcE(I1)E(N2)=E(N2)−E(N1)E(I1)E(I1)E(N2)−κ1E(I1)E(N2)=E(I1)E(N2)(Δ1−κ1)=Δ1E(I1)E(N2)(1−κ1Δ1)=E(N2)−E(N1)E(N2)(1−κ1Δ1)=(1−αμ[αμ−λ(qα+pμ)](α−λ)[λpq(α−μ)2+αμ(αq+μp)])(1−κ1Δ1) |
To investigate the influence of the system's parameters on model selection, we give the trend of Δ1 in some extreme cases.
Corollary 3.1. (1) as λ→0, Δ1→β(α−μ)αμ; (2) when 1μ<1α+1β, as λ→αβαq+β−, Δ1→0; (3) when 1μ>1α+1β, as λ→αμαq+μp−, Δ1→∞.
Proof. Under the assumption α>max(μ,λ), (α−μ), [(α−λ)(α−μ)λ+α2μ] and (λβ+α2−λαp) in the expression of Δ1 are always positive.
(1) This conclusion is obvious when we substitute λ→0 into the expression for Δ1 in Eq (3.17). It can be verified in Figure 3(a) and 3(b).
(2) When 1μ<1α+1β, we can conclude that αβαq+β<αμαq+μp. Then if λ→αβαq+β, (αμ−λ(αq+μp)) is positive and Δ1→0.
(3) When 1μ>1α+1β, we can conclude that αβαq+β>αμαq+μp. Then if λ→αμαq+μp, (αβ−λ(αq+β)) is positive and Δ1→∞.
Remark 3. Corollary 3.1 gives the values of Δ1 for the three extreme cases. (1)When the customer arrival rate λ is low(λ→0+), Δ1 tends to a constant with fixed α, β and μ. This suggests that the manager's decision, in this case, depends mainly on the relationship between hc and Δ1. If Model 1 is more profitable, the percentage reduction in the total cost is given by ϵ1λ→0+⟶q(β(α−μ)−κ1αμ)β(αq+μp). (2) When 1μ<1α+1β, the average full service time for Type 2 customers is smaller than the total average decomposed service time. Model 2 is beneficial as λ→αβαq+β− regardless of the ratio of c, h; see Figure 3(a). This is in line with our intuitive guess. In Model 1, when the customer arrival rate is high (λ→αβαq+β−), there are two queues in the system. One is the customers' waiting queue, and the other is for stored orders. Service decomposition reduces customers' waiting time while increasing the cost of maintaining the stored orders. Conversely, the increased sojourn time of Type 2 customers is less than the processing time of stored orders. Then Model 2 is preferable even for a low but positive h. See Figure 3(a). (3) When 1μ>1α+1β, the total average decomposed service time is shorter than the average continuous service time. If the customer arrival rate is high (λ→αμαq+μp−), the customer average sojourn time is high. Service decomposition can largely reduce the queue length and lower costs even for a large but finite h. In this case, the economic improvement is ϵ1λ→αμαq+μp−⟶1. See Figure 3(b).
Corollary 3.2. (1) As β→λαqα−λ+, Δ1→0; (2) As β→∞, Δ1→(α−μ)((α−λ)(α−μ)λp+α2μ)(α−λ)αμλ(αμ−λ(αq+μp)).
Proof. Straightforward by substituting in Eq (3.17) and rearranging items.
Remark 4. Corollary 3.2 indicates that Δ1 tends to zeros for a low additional service rate (β→λαqα−λ+). This can help managers make quick decisions on model selection: if the additional service β rate is less than λαqα−λ, retain the full service for Type 2 customer instead of service decomposition. See Figure 3(c). When the system processes pending orders in inventory at a high rate (β→∞), a new arrival can preempt the current service. In this case, Δ1 tends to be a positive constant. This implies that the manager may retain the full service of Type 2 customers (Model 2) even for a high additional service rate. See Figure 3(c).
Corollary 3.3. (1) As α→max(μ,λββ−λq)+, Δ1→0; (2) As α→λμpμ−λq, Δ1→∞; (3) As α→∞, Δ1→(λp+μ)(β−λq)μ(μ−λq).
Proof. Straightforward by substituting in Equation (3.17) and rearranging items.
Remark 5. Corollary 3.3 summarizes the values of Δ1 for extreme values of the basic service rate α. In the first case, Δ1 tends to zeros when the basic service rate α is small (α→max(μ,λββ−λq)+). This situation is consistent with our intuitive inference. If the basic service rate α gradually approaches the full service rate μ for Type 2 customers, the system will process pending orders in inventory during idle time. This situation does not reduce the customer's waiting cost while increasing the system's inventory holding cost. Therefore Model 2 is more profitable than Model 1; see Figure 3(e). In the second case, Δ1 tends to be infinite when the basic service rate is small α→λμpμ−λq. It seems that Model 1 is more appropriate in this case. See Figure 3(e). However, this occurs only when the basic service rate is smaller than the full service rate of Type 2 customers (α<μ). This contradicts our previous assumptions (α>μ), so we exclude this extreme case in the following discussion. In the last case, for a large basic service rate (α→∞), Δ1 tends to a finite constant. This means that Model 2 is preferable regardless of the ratio of h and c. If the basic service rate α is large, only the pending orders queue in the inventory. Compared to Model 2, Model 1 is more profitable when the cost of Model 1 to hold inventory is less than the customers' waiting cost in Model 2. Also known as κ1<Δ1=(λp+μ)(β−λq)μ(μ−λq). Regarding economic improvement, the percentage reduction in the total cost of this case can be obtained by ϵ1=1−κ1μ(μ−λq)(λp+μ)(β−λq). Figure 3(d) illustrates the trend of Δ1.
Corollary 3.4. (1) As {α,β}→{μ+,∞}, Δ1→0; (2) As {α,β}→{∞,λq+}, Δ1→0; (3) As {α,β}→{∞,∞}, Δ1→∞.
Proof. Straightforward by substituting in Eq (3.17) and rearranging items.
Remark 6. Corollary 3.4 shows that Model 2 is preferable if the basic service rate α is small (α→μ+), even for a high additional service rate (β→∞). It is easy to explain that as α→μ, the service rates of the two types of customers gradually equalize. It would only increase the cost of the system to split the Type 2 customer's service into two phases, even if the additional service rate is infinite. The same outcome holds when the additional service rate β is low (β→λq+), even for a large basic service rate (α→∞). This result is intuitive. When the additional service rate is low, many pending orders accumulate in the inventory, significantly increasing the system holding cost. Conversely, when the basic service rate α and the additional service rate β are significant, Δ1 tends to infinity. At this point, Model 1 is beneficial for almost all values of c and h. The economic improvement for the case with high values of the basic service rate and the additional service rate ({α,β}→{∞,∞}) is given by ϵ1→1. Figure 3(f) shows the trends of the values of Δ1.
We now examine the impact of service decomposition on system idle time. The idle probability can be used to represent the average idle fraction. In Model 1, the server is dormant only when there are no customers in the system and no stored orders in the inventory. Thus, the idle probability of Model 1 is p10,0. The idle probability of Model 2 is p20,0. Obviously, we can obtain the following proposition.
Proposition 3.3. The idle fraction of Model 1 to be larger than Model 2, if 1μ>1α+1β is satisfied.
Proof. Directly substitute the two probability expressions to complete the proof.
p10,0=(α−λ)β−λαqαβ>αμ−λ(αq+μp)αμ=p20,0μ((α−λ)β−λαq)>β(αμ−λ(αq+μp))1μ>1α+1β |
In Remark 2, we mentioned that a Type 2 customer might request a full service due to the excessive waiting time for a stored order. The system inventory level would then be a finite value, which is also more in line with the actual situation. In this subsection, we focus on another queueing-inventory model in which the service of a Type 2 customer is split into two parts only when the inventory level is less than N. That means the inventory capacity is limited and only allows a maximum of N orders to be stored. If the inventory level is less than N, the system operates as Model 1. Conversely, if the inventory capacity is N, a Type 2 customer will receive a full service. At this point, the system operates in line with Model 2. Therefore, Model 3 can be seen as a mixture of Models 1 & 2 .
Denote the number of customers in the system, the server's state and the inventory level at time t by N3(t), S(t) and I3(t), respectively, where
S(t)={0,the server is idle,1,the server is busy with a Type 1 customer,2,the server is busy with a Type 2 customer,3,the server is in the basic service,4,the server is in the additional service. | (4.1) |
The state space Ω3=∪∞n=0{Hn}, where
H0={(0,0,0),(0,4,1),(0,4,2),⋯,(0,4,N)},{Hn}n≥1={(n,3,0),(n,3,1),(n,3,2),⋯,(n,3,N−1),(n,1,N),(n,2,N)}. |
The transitions between all the states can be illustrated in Figure 4.
Arranging the Markov process X3(t)={(N3(t),S(t),I3(t)),t≥0} in lexicography sequence, we can obtain the following infinitesimal generator:
Q=(B00C01A10BCABCABC⋱⋱⋱), | (4.2) |
where
B00=(−λβ−(λ+β)β−(λ+β)⋱⋱β−(λ+β))(N+1)×(N+1),C01=(λλ⋱λλpλq)(N+1)×(N+2), | (4.3) |
A10=(αpαqαpαq⋱⋱αpαqαμ)(N+2)×(N+1),A=(αpαqαpαq⋱⋱αpαqαpαpqαq2αpαqμpμq)(N+2)×(N+2), | (4.4) |
and
B=diag(−(λ+α),−(λ+α),⋯,−(λ+α),−(λ+μ)),C=diag(λ,λ,⋯,λ). | (4.5) |
B and C are both square arrays of order N+2. The steady condition for Model 3 can be obtained by the mean drift method. Let ξ=(ξ0,ξ1,⋯,ξN−1,ξN,1,ξN,2) be te invariant probability vector of the matrix:
D=A+B+C=(−αqαq−αqαq⋱⋱−αqαq−αqαpqαq2−αqαqμp−μp). | (4.6) |
We can obtain vector ξ from ξD=0 and ξe=1 (e represents the all-1 vector of the corresponding order) as:
{ξk=0,k=0,1,⋯,N−1,ξN,1=μpαq+μp,ξN,2=αqαq+μp. | (4.7) |
According to the mean-drift method, the stationary condition of Model 3 is ξAe>ξCe, which can be rewritten as λ<αμαq+μp. When the number of customers in the system accumulates to a certain number, the inventory level will reach the maximum capacity N. After that, the service rules of system customers are the same as Model 2, so the steady-state conditions are also the same.
Next, we analyze this queueing-inventory system with limited capacity under the stability condition. The Matrix-Geometric method developed by Neuts [19] is applied to derive the stationary distribution of Model 3. First, we define the stationary joint probability p3n,s,i as
p3n,s,i=limt→∞Pr[N3(t)=n,S(t)=s,I3(t)=i],(n,s,i)∈Ω3,p30=(p30,0,0,p30,4,1,⋯,p30,4,N)p3n=(p3n,3,0,p3n,3,1,⋯,p3n,3,N−1,p3n,1,N,p3n,2,N),n≥1p3=(p30,p31,;⋯,p3n,⋯) | (4.8) |
We should emphasize that the stationary joint probability p3 must satisfy the following equations: p3Q=0 and p3e=1, which equal to
p30B00+p31A01=0, | (4.9) |
p30C01+p31B+p32A=0, | (4.10) |
p3n−1C+p3nB+p3n+1A=0,n≥2. | (4.11) |
If we assume that the stationary p3n have the following expression:
p3n=p31Rn−1,n≥2, | (4.12) |
where R is a matrix of order (N+2)×(N+2) satisfying C+RB+R2A=0. The boundary probability vectors p30, p31 can be determined by Eqs (4.9) and (4.10) and the normalizing condition as follows:
{p30B00+p31A01=0,p30C01+p31(B+RA)=0,p30e+p31(I−R)−1e=1. | (4.13) |
Since R cannot be computed explicitly, we can obtain a numerical result for R with the algorithms devised by Latouche and Ramaswami [20]. Then, we list two performance measures of Model 3.
(1) The average number of customers in the system is denoted by L:
L=∞∑n=0np3ne=p31[I−R]−2e; | (4.14) |
(2) Let Iq denote the mean number of stored orders in inventory. We have
Iq=p30v+∞∑i=1p3iw=p30v+p31(∞∑i=1Ri−1)w=p30v+p31[I−R]−1w, | (4.15) |
where v=(0,0,1,2,⋯,N−1)T and w=(0,1,2,3,⋯,N−1,N,N)T.
In this subsection, we consider determining the optimal inventory capacity N to reduce system costs under different parameter settings. The performance measures can be expressed as a function of the maximum inventory capacity N, i.e., L(N) and Iq(N). First, we should establish a total cost function for the queueing-inventory system with limited capacity as
Tc(N)=cL(N)+hIq(N), | (4.16) |
where c and h are defined as Section 2. Without loss of generality, we set the customer's sojourn time cost rate to 1, i.e., c=1. Also, set h to 0.6. This means that the unit cost of maintaining an inventory order costs is less than that of making a customer wait for service.
Example 1. According to the previous discussion in Section 2, the relationship between 1μ and 1α+1β will affect the numerical results, so we discuss the effect of N on the total cost function Tc(N) in two cases. The curves of case 1 :1μ<1α+1β and case 2: 1μ>1α+1β are displayed in Figure 5. The figure indicates that when case 2 holds, the total cost function decreases as N increases. This implies that performing service decomposition for all Type 2 customers is profitable. According to our intuitive conjecture, the queue length L(N) decreases as N increases, which is consistent with the numerical results in Figure 6. However, the trend of the average inventory level Iq(N) against N in Figure 6 is opposite to our conjecture, which can be explained as follows. When N tends to infinity, the system can store sufficient orders. Therefore, the average queue length L(N) gradually tends to a constant value (queue length of the classical M/M/1 queueing system λα−λ). However, the system inventory level does not keep growing but tends to stabilize. According to Proposition 3.3, the idle fraction is increased due to service decomposition in case 2. The customer leaves the system immediately after receiving the basic service. Thus, the pending orders in the inventory can be processed during the idle time, so the system inventory level does not keep growing. Therefore, in case 2, performing service decomposition for all Type 2 customers is beneficial. As for case 1, the blue curve in Fig. 5 demonstrates the variation of the cost function as N increases. The point (4, 2.1146) is the optimal value point, indicating that the minimum total cost can be obtained when the maximum inventory capacity of the system is set to 4 at this parameter setting. According to the previous analysis, in case 1, an optimal solution of the cost function Tc(N) with respect to N exists. A simple algorithm can be used to search the optimal values over a small set of integer values of the maximum inventory level N. This suggests that, in some cases, providing service decomposition for all Type 2 customers is not optimal. Managers can set an optimal inventory capacity to obtain the optimal cost.
Example 2. In this numerical example, we focus on the effect of some parameters on the optimal values N∗ and Tc∗(N).
(1) Figure 7(a) shows that the system optimal inventory capacity N∗ decreases as λ increases. This result contradicts our intuitive guesses, which can be explained as follows. An increase in λ leads to an increase in the number of customers arriving in the system. After the system inventory reaches a set level (maximum inventory capacity), it operates in line with Model 2. This results in the inventory level not decreasing while the queue length increases. As a result, the system cost will increase rapidly, so that N∗ is reduced to reduce the inventory maintenance cost. As for the system, total cost Tc∗(N) increases with the increase of λ. When λ>8, the growth rate of Tc∗(N) also becomes larger. This is because the system is gradually saturated if λ>8. A slight increase in λ leads to a significant increase in the queue length and the total cost Tc∗(N).
(2) Figure 7(b) displays the curves of N∗ and Tc∗(N) with respect to α. As we can see from the blue curve, the optimal inventory capacity N∗ increases as α increases. When α is small, the customer queue length increases, resulting in an increase in customer waiting costs. As the service rate α increases, the number of customers waiting in the system decreases. What is more, the customer waiting cost decreases, and the system idle time increases. The orders stored in the inventory can be processed during the idle time. An increase in N∗ allows the system to provide service decomposition for more Type 2 customers, thus reducing system costs which can be confirmed by the orange curve in Figure 7b. In the previous discussion, we have assumed that the ratio of h to c is 0.6. When α increases, Tc∗(N) decreases even though N∗ is increasing. This implies that cL(N) outperforms hIq(N).
(3) Figure 7(c) illustrates the effect of β on the optimal values (N∗,Tc∗(N)). The customer waiting cost is constant since β does not affect the system queue length. Managers need only consider the cost of maintaining inventory in this case. When β takes a smaller value, the average time to execute the additional service is longer. During the idle period, the additional service is more likely to be interrupted by the arrival of a new customer. Therefore, providing service decomposition for Type 2 customers is not recommended. Conversely, when β>18, providing service decomposition to more Type 2 customers is recommended. This is because the additional service rate β is much greater than the customer arrival rate λ. During the customer arrival interval, the number of stored orders in inventory decreases even though N∗ is large. The change in β only directly affects the system's inventory level. An increase in N∗ means that more orders can be stored but does not represent an increase in the system's average inventory level since the inventory is not always full. Instead, the optimal inventory capacity N∗ increases due to the additional service rate β increases. More Type 2 customers can leave the system immediately after receiving basic services, which reduces the waiting time for all customers. Thus, the optimal total cost Tc∗(N) decreases as β increases even though N∗ is increasing, implying that cL(N) is outperforming hIq(N).
(4) Figure 7(d) describes the trends of N∗ and Tc∗(N) with respect to q. The increase in q represents an increase in the number of Type 2 customers arriving in the system. The blue curve indicates that the optimal inventory capacity N∗ decreases as q increases. This result may be considered counter intuitive. Due to the increase in q, the system inventory level will reach the maximum inventory capacity more quickly. After this point, the system no longer provides service decomposition for Type 2 customers. Since the full service rate for Type 2 customers is less than that of Type 1 customers (μ<α), the waiting cost of Type 1 customers increases. In this way, the system inventory is kept at a high level while the queue length increases, which leads to a higher total cost. Thus, providing service decomposition for more Type 2 customers is not beneficial, and vice versa; when q is small, if the inventory capacity is set smaller, more Type 2 customers will need to receive full service. This will increase the waiting cost for Type 1 customers. Therefore, in this case, providing service decomposition for more Type 2 customers is recommended, which means setting the inventory capacity N higher.
Example 3. In this example, we investigate the economic improvement of the optimal capacity setting with respect to the two extreme capacities (n=∞ for Model 1 and n=0 for Model 2). First, we define the improvement achieved by Model 3 as evaluated in terms of the percentage reduction in the total expected cost of Model 1 as
γ=C1−Tc∗(N)C1. | (4.17) |
The economic improvement achieved by Model 3 is evaluated as the percentage reduction in the total average cost of Model 2, which is defined as
η=C2−Tc∗(N)C2. | (4.18) |
Figure 8 presents the effect of system parameters on the economic percentage improvements γ and η.
(1) From Figure 8(a), we can observe that both the optimal decision N∗ and the monetary improvement η (compared with Model 2) decrease with λ. As N∗ decreases, Model 3 gradually converges to Model 2. So η has the same trend as N∗. γ decreases as λ increases when λ<7. However, once λ>7, there is a rapid increase in γ. The former result may be the opposite of our conjecture. Nevertheless, it can be explained as follows. γ can be rewritten as 1−Tc∗(N)C1. When λ<7, Tc∗(N) increases at a greater rate than C1, so γ shows a decreasing trend.
(2) Figure 8(b) displays the curves of (N∗,γ,η) against α. It can be observed that N∗ increases rapidly, and η increases with α. As for γ, it shows a trend of decreasing first and then increasing with the increase of α. The increasing trend of γ can be explained as the rate at which Tc∗(N) approaches C1 is smaller than the rate at which C1 grows, for a large α, although the N∗ is large (N∗>80).
(3) Figure 8(c) indicates that both the optimal decision N∗ and the monetary improvement η increase with β, which are consistent with our conjectures. We notice that the economic improvement γ decreases with β. What is more, when β<13, the percentage reduction γ is large, especially for β=10. This result is consistent with the first case in Corollary 3.2. Therefore, managers are advised to provide only service decomposition for some Type 2 customers in this case.
(4) Figure 8(d) presents the effect of q on (γ,η,N∗). Intuitively, as q increases, γ increases and N∗ decreases. The latter has been explained in Example 2(4). For a large q, Model 1 will store many orders in the inventory, accompanied by high inventory maintenance costs. Since the economic improvement γ increases at this point, providing service decomposition for fewer Type 2 customers is more beneficial. When q>0.3, η has the same trend as N∗, which is understandable. Conversely, γ increases with q for q<0.3 implying that Tc∗(N) is more affected by q than C2.
In this section, we compare the theoretical results with the simulation results to verify the correctness of the analysis. We consider the case of Model 1 and Model 2 for different values of p. Some results of the simulations are given in Figure 9, in which, we assume that λ=2, μ=2, α=4, β=6 and p=0.7. We summarize in Table 1 the comparison between the theoretical and simulation values of the performance measures for Model 1 and Model 2. The parameters are set as follows: λ=10, μ=10, α=20 and β=25. The notations of the performance measures in Table 1 are described as follows. S denotes the average sojourn time of a stored additional service. I denotes the average number of additional services stored in inventory. W represents the average waiting time of customers. N represents the queue length of customers. We can see in Table 1, the relative differences in the system performances are less than 8%. These numerical results imply the reliability of our proposed model and the derived performance measures.
Performance measure | Theoretical result | Simulation result | Relative diff. | ||
Model 1 | p=0.2 | S | 0.6778 | 0.7191 | 0.059130933 |
I | 5.4222 | 5.3724 | 0.009226836 | ||
W | 0.05 | 0.0469 | 0.063983488 | ||
N | 1 | 0.9955 | 0.004510148 | ||
p=0.4 | S | 0.4385 | 0.4186 | 0.046435655 | |
I | 2.6308 | 2.4767 | 0.060342633 | ||
W | 0.05 | 0.0477 | 0.047082907 | ||
N | 1 | 1.0196 | 0.019409784 | ||
p=0.6 | S | 1.2471 | 1.3405 | 0.072190447 | |
I | 0.3118 | 0.3190 | 0.022828155 | ||
W | 0.05 | 0.0465 | 0.07253886 | ||
N | 1 | 1.0072 | 0.007174173 | ||
Model 2 | p=0.2 | W | 0.1357 | 0.1338 | 0.014100186 |
N | 2.0071 | 1.9890 | 0.009058832 | ||
p=0.4 | W | 0.35 | 0.3512 | 0.003422704 | |
N | 4.3 | 4.3407 | 0.009420533 | ||
p=0.6 | W | 0.35 | 0.3435 | 0.018745494 | |
N | 4.3 | 4.2573 | 0.009979783 |
In this article, we investigated the efficiency improvement problem of a queueing system with two types of customers through service decomposition. The service of Type 2 customers can be split into two parts. One part is the basic service with the same service rate as the Type 1 customers. When no customers are waiting, additional services are executed after the last customer leaves the system. An additional service can be seen as a pending order stored in the system inventory. The matrix geometric method and the probability generating function were used to perform stationary analysis.
Due to the existence of explicit expressions for the performance measures of Model 1 and Model 2, the impact of service decomposition was also discussed categorically. We demonstrated that the proposed service decomposition is more favorable when Eq (3.7) holds. The idle fraction of the server in Model 1 is shown to be larger than that in Model 2 when the total average time of the split service is less than the average full service time of Type 2 customers. This yields a paradox, where performing additional services during server idle time will increase the fraction of time the server is idle. As for some cases (the total average time of the split service is longer than the average continuous service time of Type 2 customers), the proposed decomposition of services is not beneficial. Therefore, we considered limiting the number of pending orders stored in the system. Similarly, we numerically investigated the improvement of Model 3 versus Models 1 & 2 in terms of economic lift. The numerical examples indicated that when the total average time of the split service is less than the average continuous service time of Type 2 customers, the total cost function decreases as N increases. This means that managers should provide split services for more Type 2 customers. An intelligent algorithm is used to obtain the optimal inventory capacity and the minimum system cost. The economic improvement achieved by applying the optimal capacity to Model 3 was also graphically presented.
In the future, many interesting directions can be investigated. An interesting direction is that the customer has non-preemption priority to the stored orders. Another direction for future investigation is taking the vacation or working vacation into account when the system is empty. In addition, considering the online shopping model in the context of today's developments, Type 3 customers can be defined as those who place their orders online without basic services.
The authors declare they have not used Artificial Intelligence (AI) tools in the creation of this article.
This research was supported in partial by the Guangxi Natural Science Foundation (No. 2023GXNSFBA026033), the Guangxi Young Teachers Basic Ability Improvement Project (No. 2022KY0191), the National Natural Science Foundation of China (No. 61773014).
The authors declare that none of the authors have any competing interests in the manuscript.
[1] |
A. Marand, H. Li, A. Thorstenson, Joint inventory control and pricing in a service-inventory system, Int. J. Prod. Econ., 209 (2019), 78–91. http://dx.doi.org/10.1016/j.ijpe.2017.07.008 doi: 10.1016/j.ijpe.2017.07.008
![]() |
[2] |
G. Hanukov, T. Avinadav, T. Chernonog, U. Spiegel, U. Yechiali, A queueing system with decomposed service and inventoried preliminary services, Appl. Math. Model., 47 (2017), 276–293. http://dx.doi.org/10.1016/j.apm.2017.03.008 doi: 10.1016/j.apm.2017.03.008
![]() |
[3] |
G. Hanukov, T. Avinadav, T. Chernonog, U. Yechiali, Performance improvement of a service system via stocking perishable preliminary services, Eur. J. Oper. Res., 274 (2019), 1000–1011. http://dx.doi.org/10.1016/j.ejor.2018.10.027 doi: 10.1016/j.ejor.2018.10.027
![]() |
[4] |
G. Hanukov, Improving efficiency of service systems by performing a part of the service without the customer's presence, Eur. J. Oper. Res., 302 (2022), 606–620. http://dx.doi.org/10.1016/j.ejor.2022.01.045 doi: 10.1016/j.ejor.2022.01.045
![]() |
[5] | Wasted time at work costing companies billions in 2006, Salary.com Staff, 2012. Available form: http://www.salary.com/wasted-time-at-work-still-costing-companies-billions-in-2006/. |
[6] |
B. Doshi, Queueing systems with vacations-a survey, Queueing Syst., 1 (1986), 29–66. http://dx.doi.org/10.1007/BF01149327 doi: 10.1007/BF01149327
![]() |
[7] |
J. Ke, The optimal control of an M/G/1 queueing system with server startup and two vacation types, Appl. Math. Model., 27 (2003), 437–450. http://dx.doi.org/10.1016/S0307-904X(03)00047-7 doi: 10.1016/S0307-904X(03)00047-7
![]() |
[8] |
Y. Zhang, D. Yue, W. Yue, A queueing-inventory system with random order size policy and server vacations, Ann. Oper. Res., 310 (2022), 595–620. http://dx.doi.org/10.1007/s10479-020-03859-3 doi: 10.1007/s10479-020-03859-3
![]() |
[9] |
R. Meena, M. Jain, A. Assad, R. Sethi, D. Garg, Performance and cost comparative analysis for M/G/1 repairable machining system with N-policy vacation, Math. Comput. Simulat., 200 (2022), 315–328. http://dx.doi.org/10.1016/j.matcom.2022.04.012 doi: 10.1016/j.matcom.2022.04.012
![]() |
[10] |
J. Ke, Modified T vacation policy for an M/G/1 queueing system with an unreliable server and startup, Math. Comput. Model., 41 (2005), 1267–1277. http://dx.doi.org/10.1016/j.mcm.2004.08.009 doi: 10.1016/j.mcm.2004.08.009
![]() |
[11] |
P. Vijaya Laxmi, P. Rajesh, T. Kassahun, Analysis of a variant working vacation queue with customer impatience and server breakdowns, International Journal of Operational Research, 40 (2021), 437–459. http://dx.doi.org/10.1504/IJOR.2021.114839 doi: 10.1504/IJOR.2021.114839
![]() |
[12] |
J. Li, N. Tian, The M/M/1 queue with working vacations and vacation interruptions, J. Syst. Sci. Syst. Eng., 16 (2007), 121–127. http://dx.doi.org/10.1007/s11518-006-5030-6 doi: 10.1007/s11518-006-5030-6
![]() |
[13] |
J. Blanc, P. Waal, P. Nain, D. Towsley, Optimal control of admission to a multiserver queue with two arrival streams, IEEE T. Automat. Contr., 37 (1992), 785–797. http://dx.doi.org/10.1109/9.256332 doi: 10.1109/9.256332
![]() |
[14] |
A. Turhan, M. Alanyali, D. Starobinski, Optimal admission control in two-class preemptive loss systems, Oper. Res. Lett., 40 (2012), 510–515. http://dx.doi.org/10.1016/j.orl.2012.08.012 doi: 10.1016/j.orl.2012.08.012
![]() |
[15] |
B. Kim, J. Kim, Waiting time distributions in an M/G/1 retrial queue with two classes of customers, Ann. Oper. Res., 252 (2017), 121–134. http://dx.doi.org/10.1007/s10479-015-1979-1 doi: 10.1007/s10479-015-1979-1
![]() |
[16] | G. Hanukov, A queueing-inventory model with skeptical and trusting customers, Ann. Oper. Res., in press. http://dx.doi.org/10.1007/s10479-022-04936-5 |
[17] |
G. Lawlor, I'Hôpital's rule for multivariable functions, The American Mathematical Monthly, 127 (2020), 717–725. http://dx.doi.org/10.1080/00029890.2020.1793635 doi: 10.1080/00029890.2020.1793635
![]() |
[18] | J. Cohen, The single server queue, In: North-Holland series in applied mathematics and mechanics, Amsterdam: Elsevier, 1982. |
[19] | M. Neuts, Matrix-geometric solutions in stochastic models: an algorithmic approach, New York: Dover Publications, 1994. |
[20] |
G. Latouche, V. Ramaswami, A logarithmic reduction algorithm for quasi-birth-and-death processes, J. Appl. Probab., 30 (1993), 650–674. http://dx.doi.org/10.2307/3214773 doi: 10.2307/3214773
![]() |
Performance measure | Theoretical result | Simulation result | Relative diff. | ||
Model 1 | p=0.2 | S | 0.6778 | 0.7191 | 0.059130933 |
I | 5.4222 | 5.3724 | 0.009226836 | ||
W | 0.05 | 0.0469 | 0.063983488 | ||
N | 1 | 0.9955 | 0.004510148 | ||
p=0.4 | S | 0.4385 | 0.4186 | 0.046435655 | |
I | 2.6308 | 2.4767 | 0.060342633 | ||
W | 0.05 | 0.0477 | 0.047082907 | ||
N | 1 | 1.0196 | 0.019409784 | ||
p=0.6 | S | 1.2471 | 1.3405 | 0.072190447 | |
I | 0.3118 | 0.3190 | 0.022828155 | ||
W | 0.05 | 0.0465 | 0.07253886 | ||
N | 1 | 1.0072 | 0.007174173 | ||
Model 2 | p=0.2 | W | 0.1357 | 0.1338 | 0.014100186 |
N | 2.0071 | 1.9890 | 0.009058832 | ||
p=0.4 | W | 0.35 | 0.3512 | 0.003422704 | |
N | 4.3 | 4.3407 | 0.009420533 | ||
p=0.6 | W | 0.35 | 0.3435 | 0.018745494 | |
N | 4.3 | 4.2573 | 0.009979783 |
Performance measure | Theoretical result | Simulation result | Relative diff. | ||
Model 1 | p=0.2 | S | 0.6778 | 0.7191 | 0.059130933 |
I | 5.4222 | 5.3724 | 0.009226836 | ||
W | 0.05 | 0.0469 | 0.063983488 | ||
N | 1 | 0.9955 | 0.004510148 | ||
p=0.4 | S | 0.4385 | 0.4186 | 0.046435655 | |
I | 2.6308 | 2.4767 | 0.060342633 | ||
W | 0.05 | 0.0477 | 0.047082907 | ||
N | 1 | 1.0196 | 0.019409784 | ||
p=0.6 | S | 1.2471 | 1.3405 | 0.072190447 | |
I | 0.3118 | 0.3190 | 0.022828155 | ||
W | 0.05 | 0.0465 | 0.07253886 | ||
N | 1 | 1.0072 | 0.007174173 | ||
Model 2 | p=0.2 | W | 0.1357 | 0.1338 | 0.014100186 |
N | 2.0071 | 1.9890 | 0.009058832 | ||
p=0.4 | W | 0.35 | 0.3512 | 0.003422704 | |
N | 4.3 | 4.3407 | 0.009420533 | ||
p=0.6 | W | 0.35 | 0.3435 | 0.018745494 | |
N | 4.3 | 4.2573 | 0.009979783 |