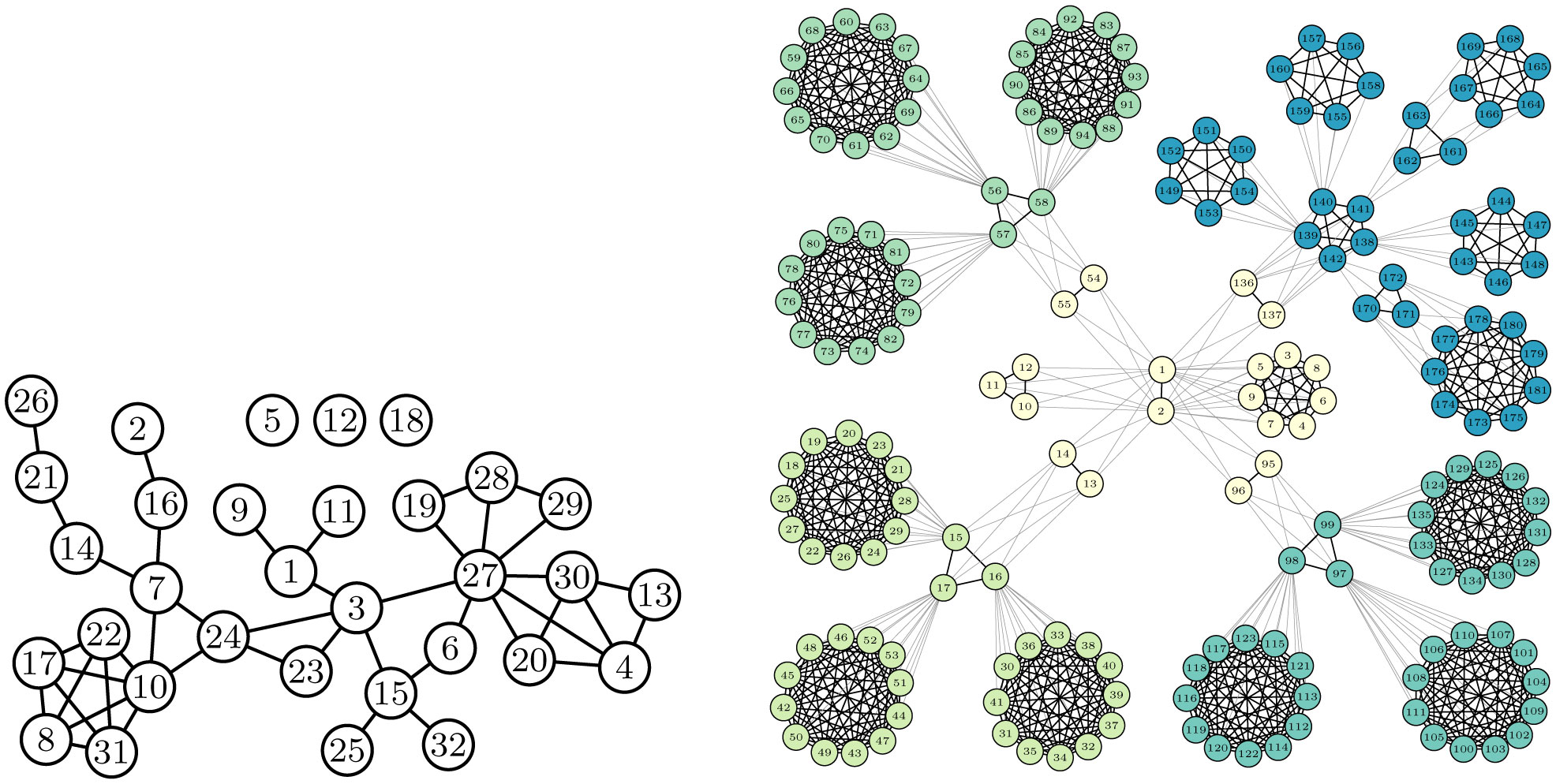
This study evaluated the color stability, bioactive compounds, and antioxidant activity of roselle calyx powders dissolved at different pHs. The results showed that purple roselle calyx powders revealed better color than red roselle, and foam-mat dried powders were better than the conventionally dried powder. The best solution without pH adjustment was found in foam-mat dried purple calyces. pH values significantly affected color stability and quality, bioactive compounds, and antioxidant activity of roselle powder solutions. The lightness, redness, and yellowness of the solutions at different pH values ranged from 27.00 to 74.45, from 0.70 to 52.20, and from 3.9 to 47.50, respectively. The non-adjusted pH solution had a lightness of 33.80–49.05, redness of 19.15–42.45, and yellowness of 7.10–18.35. The color quality, indicated by color intensity at different pHs, ranged from 0.03 to 15.24; the non-adjusted pH solution had a color intensity of 1.37–33.48, revealing that pH decreased color quality. Retention of phenolics and flavonoids was 8.02%–99.69% and 0.00%–81.05%, respectively, indicating the instability of bioactive compounds. Roselle antioxidant activity was observed at different pHs, ranging from 0.00 to 1.11 mg Trolox equivalent (TE)/g for DPPH (2, 2-diphenyl-1-picrylhydrazyl) scavenging activity and 0.46–104.16 mg TE/g for the ABTS [2, 2'-azino-bis(3-ethylbenzothiazoline-6-sulfonic acid)] assay. Acidic pHs preserved better all dependent parameters. In practice, the pH value should be considered when using roselle calyx powders in food products. This study used a solution as a model for a watery food system. Suitable products to use roselle calyx powders are water-based products with an acidic pH, such as syrups, yogurts and yogurt-based products, fermented milk, and sour-taste beverages. Roselle calyx powders are suitable to use as coloring agents or as bioactive compounds to provide healthy functional properties. The use of foam-mat dried powders is suggested due to the better antioxidant activity and color preservation than conventionally dried powder.
Citation: Teti Estiasih, Jatmiko Eko Witoyo, Khofifah Putri Wulandari, Fadhillah Dwi Juniati, Widiastuti Setyaningsih, Hanifah Nuryani Lioe, Miguel Palma, Kgs Ahmadi, Hamidie Ronald Daniel Ray, Elya Mufidah. Stability comparison of conventional and foam-mat red and purple dried roselle calyces powder as a function of pH[J]. AIMS Agriculture and Food, 2025, 10(1): 177-198. doi: 10.3934/agrfood.2025010
[1] | Muhammad Asif Zahoor Raja, Adeeba Haider, Kottakkaran Sooppy Nisar, Muhammad Shoaib . Intelligent computing knacks for infected media and time delay impacts on dynamical behaviors and control measures of rumor-spreading model. AIMS Biophysics, 2024, 11(1): 1-17. doi: 10.3934/biophy.2024001 |
[2] | Yong Zhou, Yiming Ding . Finding travel proportion under COVID-19. AIMS Biophysics, 2022, 9(3): 235-245. doi: 10.3934/biophy.2022020 |
[3] | C. Dal Lin, M. Falanga, E. De Lauro, S. De Martino, G. Vitiello . Biochemical and biophysical mechanisms underlying the heart and the brain dialog. AIMS Biophysics, 2021, 8(1): 1-33. doi: 10.3934/biophy.2021001 |
[4] | Kieran Greer . New ideas for brain modelling 6. AIMS Biophysics, 2020, 7(4): 308-322. doi: 10.3934/biophy.2020022 |
[5] | Mati ur Rahman, Mehmet Yavuz, Muhammad Arfan, Adnan Sami . Theoretical and numerical investigation of a modified ABC fractional operator for the spread of polio under the effect of vaccination. AIMS Biophysics, 2024, 11(1): 97-120. doi: 10.3934/biophy.2024007 |
[6] | Carlo Bianca . Interplay and multiscale modeling of complex biological systems. AIMS Biophysics, 2022, 9(1): 56-60. doi: 10.3934/biophy.2022005 |
[7] | Dafina Xhako, Niko Hyka, Elda Spahiu, Suela Hoxhaj . Medical image analysis using deep learning algorithms (DLA). AIMS Biophysics, 2025, 12(2): 121-143. doi: 10.3934/biophy.2025008 |
[8] | Alexander G. Volkov, Yuri B. Shtessel . Propagation of electrotonic potentials in plants: Experimental study and mathematical modeling. AIMS Biophysics, 2016, 3(3): 358-379. doi: 10.3934/biophy.2016.3.358 |
[9] | Omar El Deeb, Joseph El Khoury Edde . COVID19 vaccines as boosters or first doses: simulating scenarios to minimize infections and deaths. AIMS Biophysics, 2024, 11(2): 239-254. doi: 10.3934/biophy.2024014 |
[10] | Natarajan Mala, Arumugam Vinodkumar, Jehad Alzabut . Passivity analysis for Markovian jumping neutral type neural networks with leakage and mode-dependent delay. AIMS Biophysics, 2023, 10(2): 184-204. doi: 10.3934/biophy.2023012 |
This study evaluated the color stability, bioactive compounds, and antioxidant activity of roselle calyx powders dissolved at different pHs. The results showed that purple roselle calyx powders revealed better color than red roselle, and foam-mat dried powders were better than the conventionally dried powder. The best solution without pH adjustment was found in foam-mat dried purple calyces. pH values significantly affected color stability and quality, bioactive compounds, and antioxidant activity of roselle powder solutions. The lightness, redness, and yellowness of the solutions at different pH values ranged from 27.00 to 74.45, from 0.70 to 52.20, and from 3.9 to 47.50, respectively. The non-adjusted pH solution had a lightness of 33.80–49.05, redness of 19.15–42.45, and yellowness of 7.10–18.35. The color quality, indicated by color intensity at different pHs, ranged from 0.03 to 15.24; the non-adjusted pH solution had a color intensity of 1.37–33.48, revealing that pH decreased color quality. Retention of phenolics and flavonoids was 8.02%–99.69% and 0.00%–81.05%, respectively, indicating the instability of bioactive compounds. Roselle antioxidant activity was observed at different pHs, ranging from 0.00 to 1.11 mg Trolox equivalent (TE)/g for DPPH (2, 2-diphenyl-1-picrylhydrazyl) scavenging activity and 0.46–104.16 mg TE/g for the ABTS [2, 2'-azino-bis(3-ethylbenzothiazoline-6-sulfonic acid)] assay. Acidic pHs preserved better all dependent parameters. In practice, the pH value should be considered when using roselle calyx powders in food products. This study used a solution as a model for a watery food system. Suitable products to use roselle calyx powders are water-based products with an acidic pH, such as syrups, yogurts and yogurt-based products, fermented milk, and sour-taste beverages. Roselle calyx powders are suitable to use as coloring agents or as bioactive compounds to provide healthy functional properties. The use of foam-mat dried powders is suggested due to the better antioxidant activity and color preservation than conventionally dried powder.
Modelling epidemic spreading is a central topic in the research of both contagion spreading and network science [1]. With the gravity of the impact on global health and economy of the recent COVID-19 pandemic [2], the field has gained an increasing amount of attention. Accordingly, models of epidemic spreading have been of great focus with new models constantly being proposed and built upon earlier ones. One approach to such models is compartmental models that divide the population into compartments based on their current role in the spreading process [3]. A simple example of a compartmental model is the SIR model which classifies each individual as either susceptible, infected, or recovered. Once recovered, the infection can no longer be transmitted to the recovered individual. The SIR model is well-studied and has been applied to complex networks in the literature [4]–[6]. Other types of compartmental models include SIS, SIRS, and SEIR, [3] in addition to more complex ones [7]. Models of contagion spreading can be used to quantitatively study effective ways of epidemic prevention [8], [9].
In this article, we highlight two models for different applications introduced in our earlier articles [9]–[11] and explain their equivalent results even when applied to each other's use cases. Both of the models are based on similar foundations for calculating the probabilities of spreading or connections between nodes in a network [12], where methods of probabilistic networks [13] are applied.
As mentioned, the models (referred to as the Spreading model and the Connectivity model) are designed for two separate applications. While the Spreading model is built for epidemic and behaviour spreading [10], the Connectivity model is constructed to simulate the reliability of connectivity in a service network [11]. Since the models are built for different use cases, we will introduce general vocabulary to talk about both models more easily. We call influence spreading the propagation of contagion, information, or a similar phenomenon through nodes of a network. The interpretation of contagion as influence spreading is rather natural: contagion spreads from one individual to another when they are in contact and, through these contacts, continues to spread further. Connectivity in information networks can also be thought of as information spreading through nodes and connections of the network. Where information can be transferred, a connection exists. Neither model simulates the spreading process through time—instead, only the final state of the spreading is calculated.
As the models produce equivalent results, they can both be applied to epidemic spreading. In particular, they are applicable to a situation where individuals gain full immunity to a disease after contagion, as a result of the models' principle to spread influence to a node only once, similarly to the SIR model [3]. In the case of information network connectivity, immunity can be equated to the inactivity of nodes in the network. Diseases resulting in full or near full immunisation include measles, mumps, rubella, and chickenpox [14], though many infections give no or only partial immunity. We will propose a model for partial immunity epidemic spreading in a future study of ours.
The Spreading model is built to be extendable and has the potential for additional parameters that the Connectivity model lacks. For example, parameters such as maximum spreading path length (Lmax) and breakthrough probability can be taken into account. This enables the calculation of novel forms of connectivity, based not only on the structure of the network but also on other possible phenomena arising from the new parameters.
We compare two simulation models built for two use cases: the epidemic spreading simulation model, and the connection reliability model. Both models consider a network as a set of weighted nodes and weighted directed edges between them. Undirected edges are modelled as two identical edges with the endpoints swapped. The output of the models is the two-dimensional probability matrix
The probability matrix C(s, t) produced by both of the models gives the conditional probabilities of influence spreading from node s to node t given that influence starts to spread from node s. If the probabilities, at which the nodes initially start to spread influence are known, the conditional probabilities of the probability matrix can be multiplied by them, producing unconditional probabilities.
The Spreading model works by simulating the spreading of influence from each node to the rest of the network separately. For each node as the source, the simulation is carried out a certain number of times: this is called the number of iterations. The probability of influence spreading to another node is calculated as the number of iterations where the node was influenced divided by the total number of iterations. The simulation itself works in steps, on which all newly influenced nodes (that is, nodes that became influenced on the step right before the current one) attempt to spread influence to all of their neighbouring nodes. This attempt automatically fails if the neighbouring node has been previously influenced. Otherwise, the spreading will succeed with a probability of we · Wt, where we is the weight of the edge connecting the nodes and Wt is the weight of the target node. If the spreading succeeds, the target node will be marked as influenced and will attempt to spread influence in the subsequent step. Another parameter, Lmax, is used to limit the maximum spreading path length: influence will only spread along paths at maximum Lmax edges long. Limiting Lmax can be used to shorten the execution times of the model at the cost of less precise results or to model a situation where spreading paths are limited by some factor, such as cutting spreading chains as a preventive measure against an epidemic. A more detailed description and pseudocode for the Simulation model are provided in our earlier study [10].
The calculation in the Connectivity model works similarly in that it simulates the connectivity multiple times, taking the average results. Instead of simulating the influence spreading from each node separately, for each iteration, the set of active nodes and edges is randomly determined: the probability at which each node and edge is active is its weight. For each active node, the nodes that can be reached by paths consisting of active nodes and edges are considered connected to it. C(s, t) is then the number of iterations where the active node s had an active path to node t divided by the total number of iterations. Unlike the Spreading model, the Connectivity model does not have the Lmax parameter, as the connectivity is not determined by stepping along paths. A more detailed description and pseudocode for the Connectivity model is provided in our earlier study [11].
For both models, the probability of influence spreading through a path with a specified starting node is the product of the weights of each edge and node on the path, excluding that of the starting node. In other words,
where P(ℒ) is the probability of spreading through the path ℒ of m edges and nodes excluding the starting node, and
As the probability of influence spreading through a path is given by the same equation for both of the models, the models' results should theoretically be equivalent. This equivalence only holds, however, when Lmax is not capped to some number in the Spreading model, in order to take into account all possible paths in the network as the Connectivity model does.
The probability matrix that both of the models generate can be used for various applications, as covered in our previous articles [9], [10]. At the core of these applications are different centrality measures that reflect how central each edge and node in the network is. These measures give insight into the structure of the network, helping to understand patterns and phenomena present therein. We give three examples of centrality measures: the in- and out-centralities and the betweenness centrality.
The in- and out-centrality measures are a natural way to approach node centrality [10]. They represent how much influence flows in and out of the node, respectively. We define the in-centrality of node t as
and, similarly, the out-centrality of node s as
where V is the set of nodes in the network [10].
In other words, the in-centrality of a node is the sum of the probabilities of spreading from all other nodes to the node in question, and the out-centrality of a node is the sum of the probabilities of spreading from the node in question to all other nodes. The in- and out-centralities directly translate to physical quantities of the network. As C(s, t) is the probability of influence spreading from node s to node t, the sum can be thought of as an expected value. In the case of in-centrality (Eq. 2.2), the sum represents the expected number of nodes that will spread influence to the specified node, and, for the out-centrality (Eq. 2.3), the sum represents the expected number of nodes that influence from the specified node will spread to. As a concrete example, the out-centrality of a node represents the expected number of infected people in a social network, when a contagious infection begins to spread from the starting node, and the in-centrality of an individual's vulnerability to being infected by different sources.
The betweenness centrality measure is another approach to studying node centrality. It represents the significance of a node in transmitting influence between different parts of the network. Betweenness centrality can be easily defined for a set of nodes S, where the betweenness centrality of a single node s can be expressed as that for the set {s}. We first define the cohesion of a network as
and the partial cohesion of the network without the set of nodes S as
where V is the set of nodes in the network and CS is the probability matrix calculated with only nodes and edges between nodes in S taken into account. The probability matrix for partial cohesion has to be calculated independently from that for total cohesion, as the effects of removing nodes and edges can cut off paths between parts of the network and change the spreading probabilities.
With Eqs. 2.4 and 2.5, we define the betweenness centrality as the relative difference between the total and partial cohesion:
The cohesion portrays the total interconnectivity of the network. As the betweenness centrality is a relative difference in the cohesion (Eq. 2.6), the larger the betweenness centrality, the greater the effect of removing the specified nodes is on the interconnectivity. The betweenness centrality can be used to spot individuals who act in bridging roles between parts of the network, the isolation of which can help to contain the contagion to only a small part of the network.
To compare the models, we run them on two networks, the Student network [15] and the Organisation network [9] (Figure 1a,b). Precise descriptions of how the calculations in the models are carried out are presented in our earlier studies [9], [10]. The Student network is a small, 32-node network composed of empirical data on the relationships of Dutch university students [15]. We consider the network with all edge weights set to 0.5. The Organisation network, on the other hand, is a larger, 181-node network that represents a real-world organisation structure. The network consists of five departments with multiple groups forming each of them as well as hierarchical leadership relations. The Organisation network was first introduced in [9], along with different classes of preventive measures that simulate epidemic prevention by decreasing the weights of certain edges. In this article, we study the case where preventive measures are in use on all edges except on those representing leadership relations, which means that only edges representing leadership and group relations are present. Edges representing group relations have a weight of 0.5, and edges representing leadership relations have a weight of 0.3. Both of the networks are considered to be undirected with node weights equal to 1. The models work and give equivalent results for directed networks with varying node weights as well. Further study into the performance of the models and their application to other networks is presented in our earlier studies [9]–[11].
As both of the networks are real-life social networks, they are well suited for modelling epidemic spreading. Using the two networks, we can compare the models on networks exhibiting different properties: the Student network is small and sparse, whereas the Organisation network is larger and much denser. Together, the networks represent a multitude of different situations for our models to perform on. It is worth noting, however, that as the edges of the networks are undirected, the in- and out-centrality (Eqs. 2.2 and 2.3) for a node will always be equal due to the symmetry of spreading from and to the node. This is not the case for scenarios where a node can be influenced more than once, such as an epidemic of an infectious disease, the contraction of which does not lead to full immunity [2]. In practice, the probability of an infection spreading from one person to another is often different than the probability of spreading in the other direction, represented by different weights in the directed edges between them.
The models calculate the probabilities of spreading between all pairs of nodes given the layout and weight parameters of the network. The results can be used to calculate important quantities, specific to the network and its weights. One example of such quantity is the basic reproduction number for epidemics, which is a measure of contagiousness defined as the number of new infections a single infection on average leads to [16]. We have calculated the basic reproduction number for simulations run on the Organisation network as a function of edge weights in our previous study [9]. It is important to note that quantities such as the basic reproduction number are not specific to the models and must be independently calculated for any network and parameters that the models are run on. As each set of parameters and the input network define a unique spreading scenario, measures such as the basic reproduction number can always be calculated for any combination thereof. An example of such a calculation is provided in [9].
For both networks, we calculate the total cohesion (Eq. 2.4) and mean betweenness centrality (Eq. 2.6) using both models and varying Lmax for the Spreading model. We normalise the total cohesion by the number of values constituting its sum, N(N – 1), where N is the number of nodes in the network, to get the node-wise average in- and out-centrality. This scaling factor is attained from the probability matrix being of shape N × N with the diagonal consisting of N missing values. The sum of the in- and out-centralities (Eqs. 2.2 and 2.3) are equal to the cohesion:
From Eq. 3.1, the averages of the in- and out-centralities are also equal. This means that the normalised cohesion represents both the average node-wise in- and out-centralities.
The mean betweenness centrality is calculated as the average of the node-wise betweenness centralities. The minimum Lmax value, for which the results of the models are ideally equal (assuming arbitrary precision), is the maximum length, for which a self-avoiding path exists in the network, since then the Spreading model is able to simulate spreading throughout the whole network. A self-avoiding path is never longer than the number of nodes in the network, which gives a trivial upper bound. Thus, as Lmax increases, the results of the Spreading model approach those of the Connectivity model. In practice, this happens with Lmax much lower than N (Figures 2 and 3). This is due to the probability of spreading through a path decreasing exponentially with the path length.
The cohesions of the Student and Organisation networks are around 19% and 40%, respectively (Figures 2a and 3a). The Organisation network achieves a higher cohesion due to its much denser nature. The difference in density can also be seen in Figures 2b and 3b, where the Student and Organisation networks' betweenness centralities (Eq. 2.6) are around 0.12 and 0.03, respectively. Nodes in the Student network are connected by much fewer paths than in the Organisation network, and therefore each node has a more prominent role in allowing connections between other nodes.
As the results given by the Spreading model converge already with lower values of Lmax, epidemic simulation can be performed more efficiently by not taking longer spreading paths into account. With a low Lmax, however, the results differ, which allows the simulation of scenarios where spreading paths are limited by factors such as consistent isolation of patients. Since the two models produce identical results with high Lmax, both models can be used for the intended purpose of the other. The equivalence of the results indicates the similarities in the mechanisms of epidemic spreading [9] and information network connectivity [11]: where contagion spreads from node to node with immunity inhibiting it in epidemic spreading, in connectivity, information has to similarly travel from node to node with inactive nodes as a limiting factor. These similarities in the mechanisms might extend to other types of network modelling, which is a subject for future research.
The models can be run on any network with any edge and node weights but are capable of modelling only scenarios where any one node is influenced at most once. In our earlier studies, we have also presented a model where all nodes can get influenced any number of times. We will present a model capable of dealing with situations where getting influenced decreases a node's probability of getting influenced again by a given breakthrough probability in our future studies.
Modelling breakthrough influence is an example of how the models can be applied to the purposes of each other. Namely, many epidemics [9] and influence spreading [10] processes are based on similar spreading mechanisms. Both processes can have significant breakthrough probabilities depending on the specific characteristics of virus species or information types. For example, COVID-19 variants [2] can have different breakthrough probabilities despite being very close in nature. Rumours and propaganda have a higher breakthrough probability, as they circulate between people and change form more effectively than facts and knowledge.
We have compared the calculation methods and results of two models designed for epidemic spreading and connectivity simulation introduced in our earlier research. We call these models the Spreading model and Connectivity model, respectively. Even though the models are designed for very different applications, the manner in which they calculate their results is mathematically equivalent. Accordingly, the two models produce equivalent results with high enough spreading path length, Lmax, for the Spreading model. As the models were independently developed for their different purposes, their equivalence highlights the similarities in the mechanisms of epidemic spreading and information network connectivity. The similar results also enable the application of the models to each others' intended purposes, allowing different parameters to be taken into account.
In this study, we have highlighted the opportunities for using interdisciplinary modelling and simulation methods in the research of epidemic spreading, resilience in communication networks, and influence spreading in social networks.
[1] | El Bayani GF, Marpaung NLE, Simorangkir DAS, et al. (2018) Anti-inflammatory effects of Hibiscus sabdariffa Linn. on the IL-1β/IL-1ra ratio in plasma and hippocampus of overtrained rats and correlation with spatial memory. Kobe J Med Sci 64: E73–E83. |
[2] |
Izquierdo-Vega JA, Arteaga-Badillo DA, Sánchez-Gutiérrez M, et al.(2020) Organic acids from Roselle (Hibiscus sabdariffa L.)-A brief review of its pharmacological effects. Biomedicines 8: 1–16. https://doi.org/10.3390/biomedicines8050100 doi: 10.3390/biomedicines8050100
![]() |
[3] |
Sapian S, Ibrahim Mze AA, Jubaidi FF, et al. (2023) Therapeutic Potential of Hibiscus sabdariffa Linn. in attenuating cardiovascular risk factors. Pharmaceuticals 16: 1–25. https://doi.org/10.3390/ph16060807 doi: 10.3390/ph16060807
![]() |
[4] | Islam A, Jamini TS, Islam M, et al. (2016) Roselle: A functional food with high nutritional and medicinal values. Fundam Appl Agric 1: 44–49. |
[5] |
Tan SL, Sulaiman R (2020) Color and rehydration characteristics of natural red colorant of foam-mat dried Hibiscus sabdariffa L. powder. Int J Fruit Sci 20: 89–105. https://doi.org/10.1080/15538362.2019.1605557 doi: 10.1080/15538362.2019.1605557
![]() |
[6] |
Hamrouni-Sellami I, Rahali FZ, Rebey IB, et al. (2013) Total phenolics, flavonoids, and antioxidant activity of sage (Salvia officinalis L.) plants as affected by different drying methods. Food Bioprocess Technol 6: 806–817. https://doi.org/10.1007/s11947-012-0877-7 doi: 10.1007/s11947-012-0877-7
![]() |
[7] |
Roshanak S, Rahimmalek M, Goli SAH (2016) Evaluation of seven different drying treatments in respect to total flavonoid, phenolic, vitamin C content, chlorophyll, antioxidant activity and color of green tea (Camellia sinensis or C. assamica) leaves. J Food Sci Technol 53: 721–729. https://doi.org/10.1007/s13197-015-2030-x doi: 10.1007/s13197-015-2030-x
![]() |
[8] |
Tajudin NHA, Tasirin SM, Ang WL, et al. (2019) Comparison of drying kinetics and product quality from convective heat pump and solar drying of Roselle calyx. Food Bioprod Process 118: 40–49. https://doi.org/10.1016/j.fbp.2019.08.012 doi: 10.1016/j.fbp.2019.08.012
![]() |
[9] |
Djaeni M, Kumoro AC, Sasongko SB, et al. (2018) Drying rate and product quality evaluation of roselle (Hibiscus sabdariffa L.) calyces extract dried with foaming agent under different temperatures. Int J Food Sci 2018: 9243549. https://doi.org/10.1155/2018/9243549 doi: 10.1155/2018/9243549
![]() |
[10] |
Liu Y, Liu Y, Tao C, et al. (2018) Effect of temperature and pH on stability of anthocyanin obtained from blueberry. J Food Meas Charact 12: 1744–1753. https://doi.org/10.1007/s11694-018-9789-1 doi: 10.1007/s11694-018-9789-1
![]() |
[11] |
Sasongko SB, Djaeni M, Utari FD (2019) Kinetic of anthocyanin degradation in roselle extract dried with foaming agent at different temperatures. Bull Chem React Eng Catal 14: 320–325. https://doi.org/10.9767/bcrec.14.2.2875.320-325 doi: 10.9767/bcrec.14.2.2875.320-325
![]() |
[12] |
Wu HY, Yang KM, Chiang PY (2018) Roselle anthocyanins: antioxidant properties and stability to heat and pH. Molecules 23: 1357. https://doi.org/10.3390/molecules23061357 doi: 10.3390/molecules23061357
![]() |
[13] |
Estiasih T, Witoyo JE, Runtung GKM, et al. (2024) The characteristics of foam-mat dried red and purple roselle calyces powder prepared with commercial emulsifiers as the foaming agents. CYTA-J Food 22: 2356847. https://doi.org/10.1080/19476337.2024.2356847 doi: 10.1080/19476337.2024.2356847
![]() |
[14] |
Enaru B, Drețcanu G, Pop TD, et al. (2021) Anthocyanins: Factors affecting their stability and degradation. Antioxidants 10: 1967. https://doi.org/10.3390/antiox10121967 doi: 10.3390/antiox10121967
![]() |
[15] |
Friedman M, Jürgens HS (2000) Effect of pH on the stability of plant phenolic compounds. J Agric Food Chem 48: 2101–2110. https://doi.org/10.1021/jf990489j doi: 10.1021/jf990489j
![]() |
[16] |
Mattioli R, Francioso A, Mosca L, et al. (2020) Anthocyanins: A comprehensive review of their chemical properties and health effects on cardiovascular and neurodegenerative diseases. Molecules 25: 3809. https://doi.org/10.3390/molecules25173809. doi: 10.3390/molecules25173809
![]() |
[17] |
Maisto M, Marzocchi A, Ciampaglia R, et al. (2024) Effects of food-derived antioxidant compounds on in vitro heavy metal intestinal bioaccessibility. Antioxidants 13: 610. https://doi.org/10.3390/antiox13050610 doi: 10.3390/antiox13050610
![]() |
[18] |
Shruthi VH, Ramachandra CT, Nidoni U, et al. (2017). Physico-chemical, nutritional and functional properties of roselle (Hibiscus sabdariffa L.). Int J Curr Microbiol Appl Sci 6: 1–7. https://doi.org/10.20546/ijcmas.2017.612.347 doi: 10.20546/ijcmas.2017.612.347
![]() |
[19] |
Rocha IDC, Bonnlaender B, Sievers H, et al. (2014). Hibiscus sabdariffa L.—A phytochemical and pharmacological review. Food Chem 165: 424–443. https://doi.org/10.1016/j.foodchem.2014.05.002. doi: 10.1016/j.foodchem.2014.05.002
![]() |
[20] |
Marpaung AM, Paramaputri A (2023) UV-visible light spectra of Clitoria ternatea L. flower extract during aqueous extraction and storage. Int Food Res J. 30: 764–773. https://doi.org/10.47836/ifrj.30.3.18 doi: 10.47836/ifrj.30.3.18
![]() |
[21] |
Samadi S, Fard FR (2020) Phytochemical properties, antioxidant activity and mineral content (Fe, Zn and Cu) in Iranian produced black tea, green tea and roselle calyces. Biocatal Agric Biotechnol 23: 101472. https://doi.org/10.1016/j.bcab.2019.101472 doi: 10.1016/j.bcab.2019.101472
![]() |
[22] |
Huang J, Hu Z, Chin Y, et al. (2023) Improved thermal stability of roselle anthocyanin by co-pigmented with oxalic acid: preparation, characterization and enhancement mechanism. Food Chem 410: 135407. https://doi.org/10.1016/j.foodchem.2023.135407 doi: 10.1016/j.foodchem.2023.135407
![]() |
[23] |
Ifie I, Marshall LJ, Ho P, et al. (2016) Hibiscus sabdariffa (Roselle) extracts and wine: phytochemical profile, physicochemical properties, and carbohydrase inhibition. J Agric Food Chem 64: 4921–4931. https://doi.org/10.1021/acs.jafc.6b01246. doi: 10.1021/acs.jafc.6b01246
![]() |
[24] |
Baliyan S, Mukherjee R, Priyadarshini A, et al. (2022) Determination of antioxidants by DPPH radical scavenging activity and quantitative phytochemical analysis of Ficus religiosa. Molecules 27: 1326. https://doi.org/10.3390/molecules27041326 doi: 10.3390/molecules27041326
![]() |
[25] |
Thaipong K, Boonprakob U, Crosby K, et al. (2006) Comparison of ABTS, DPPH, FRAP, and ORAC Assays for estimating antioxidant activity from guava fruit extracts. J Food Anal 19: 669–675. https://doi.org/10.1016/j.jfca.2006.01.003 doi: 10.1016/j.jfca.2006.01.003
![]() |
[26] | Shruthi VH, Ramachandra CT (2019) Roselle (Hibiscus sabdariffa L.) calyces: A potential source of natural colour and its health benefits. In: Deka SC, Seth D, Hulle VRS (Eds.), Food bioactive: Functionalities and applications in human health, New York: Apple Academic Press, 169–190. https://doi.org/10.1201/9780429242793 |
[27] |
Song W, Yuan Q, Xie Y, et al. (2024) Formulation and characterization of nanocapsules loaded with roselle anthocyanins extract and enhancement of anthocyanins bioaccessibility. Food Chem 459: 140446. https://doi.org/10.1016/j.foodchem.2024.140446 doi: 10.1016/j.foodchem.2024.140446
![]() |
[28] |
Yang D, Xu HX, Guo YX, et al. (2023) Chemical profile of roselle extract and its inhibitory activities on three digestive enzymes in vitro and in vivo. Int J Biol Macromol 253: 126902. https://doi.org/10.1016/j.ijbiomac.2023.126902 doi: 10.1016/j.ijbiomac.2023.126902
![]() |
[29] |
Khoo HE, Azlan A, Tang ST, et al. (2017) Anthocyanidins and anthocyanins: Colored pigments as food, pharmaceutical ingredients, and the potential health benefits. Food Nutr Res 61: 1361779. https://doi.org/10.1080/16546628.2017.1361779 doi: 10.1080/16546628.2017.1361779
![]() |
[30] | Aishah B, Nursabrina M, Noriham A, et al. (2013), Anthocyanins from Hibiscus sabdariffa, Melastoma malabathricum and Ipomoea batatas and its color properties. Int Food Res J 20: 827–834. |
[31] | Marpaung A, Tjahjadi K (2019) The analysis of monomeric anthocyanin by pH differential method is not appropriate for certain anthocyanins. In: Proceedings of the 16th ASEAN Food Conference (16th AFC 2019)—Outlook and Opportunities of Food Technology and Culinary for Tourism Industry, 26–30. https://doi.org/10.5220/0009985400002964 |
[32] |
Dorris MR, Voss DM, Bollom MA, et al. (2018) Browning index of anthocyanin‐rich fruit juice depends on pH and anthocyanin loss more than the gain of soluble polymeric pigments. J Food Sci 83: 911–921. https://doi.org/10.1111/1750-3841.14106 doi: 10.1111/1750-3841.14106
![]() |
[33] |
Gao Q, Li Y, Li Y, et al. (2022) Antioxidant and prooxidant activities of phenolic acids commonly existed in vegetables and their relationship with structures. Food Sci Technol 42: e07622. https://doi.org/10.1590/fst.07622 doi: 10.1590/fst.07622
![]() |
[34] |
Nowak M, Tryniszewski W, Sarniak A, et al. (2022) Concentration dependence of anti-and pro-oxidant activity of polyphenols as evaluated with a light-emitting Fe2+-Egta-H2O2 System. Molecules 27: 3453. https://doi.org/10.3390/molecules27113453 doi: 10.3390/molecules27113453
![]() |
[35] |
Rajashekar CB (2023) Dual role of plant phenolic compounds as antioxidants and prooxidants. Am J Plant Sci 14: 15–28. https://doi.org/10.4236/ajps.2023.141002 doi: 10.4236/ajps.2023.141002
![]() |
[36] |
Wu HY, Yang KM, Chiang PY (2018) Roselle anthocyanins: antioxidant properties and stability to heat and pH. Molecules 23: 1357. https://doi.org/10.3390/molecules23061357 doi: 10.3390/molecules23061357
![]() |
[37] |
Horbowicz M, Kosson R, Grzesiuk A, et al. (2008). Anthocyanins of fruits and vegetables - their occurrence, analysis and role in human nutrition. Veg Crops Res Bull 68: 5–22. https://doi.org/10.2478/v10032-008-0001-8 doi: 10.2478/v10032-008-0001-8
![]() |
[38] |
Punyachareonnon, Deerattrakul V, Luepong K (2021) The Influence of pH, temperature and time on dyeing of silk fabric by black bean anthocyanin-rich extract as colorant. Prog Color Color Coatings 14: 179–186. https://doi.org/10.30509/pccc.2021.81715 doi: 10.30509/pccc.2021.81715
![]() |
[39] |
Millinia BL, Mashithah D, Nawatila R, et al. (2024) Microencapsulation of roselle (Hibiscus sabdariffa L.) anthocyanins: Effects of maltodextrin and trehalose matrix on selected physicochemical properties and antioxidant activities of spray-dried powder. Future Foods 9: 100300. https://doi.org/10.1016/j.fufo.2024.100300 doi: 10.1016/j.fufo.2024.100300
![]() |
[40] |
Idham Z, Putra NR, Aziz AHA, et al. (2022) Improvement of extraction and stability of anthocyanins, the natural red pigment from roselle calyces using supercritical carbon dioxide extraction. J CO2 Util 56: 101839. https://doi.org/10.1016/j.jcou.2021.101839 doi: 10.1016/j.jcou.2021.101839
![]() |
[41] |
Jiang T, Mao Y, Sui L, et al. (2019) Degradation of anthocyanins and polymeric color formation during heat treatment of purple sweet potato extract at different pH. Food Chem 274: 460–470. https://doi.org/10.1016/j.foodchem.2018.07.141 doi: 10.1016/j.foodchem.2018.07.141
![]() |
[42] |
Pasquet PL, Julien-David D, Zhao M, et al. (2024) Stability and preservation of phenolic compounds and related antioxidant activity from agro-food matrix: effect of pH and atmosphere. Food Biosci 57: 103586. https://doi.org/10.1016/j.fbio.2024.103586 doi: 10.1016/j.fbio.2024.103586
![]() |
[43] |
Wang Y, Xing L, Zhang J, et al. (2023) Determination of endogenous phenolic compounds in honey by HPLC-MS/MS. LWT 183: 114951. https://doi.org/10.1016/j.lwt.2023.114951 doi: 10.1016/j.lwt.2023.114951
![]() |
[44] | Krungkri W, Areekul V (2019) Effect of heating condition and pH on stability of total phenolic content and antioxidant activities of samui (Micromelum minutum) extract. In: Proceedings of the 16th ASEAN Food Conference (16th AFC 2019)—Outlook and Opportunities of Food Technology and Culinary for Tourism Industry. Bali, Indonesia, 15–32. https://doi.org/10.5220/0009980801260132 |
[45] |
Chen YT, Kao WT, Lin KW (2008) Effects of pH on the total phenolic compound, antioxidative ability and the stability of dioscorin of various yam cultivars. Food Chem 107: 250–257. https://doi.org/10.1016/j.foodchem.2007.08.017 doi: 10.1016/j.foodchem.2007.08.017
![]() |
[46] |
Rosales-Murillo SS, Sánchez-Bodón J, Hernández Olmos SL, et al. (2024) Anthocyanin-loaded polymers as promising nature-based, responsive, and bioactive materials. Polymers 16: 163. https://doi.org/10.3390/polym16010163 doi: 10.3390/polym16010163
![]() |
[47] |
Cabrita L, Fossen T, Andersen ØM (2000) Colour and stability of the six common anthocyanidin 3-glucosides in aqueous solutions. Food Chem 68: 101–107. https://doi.org/10.1016/S0308-8146(99)00170-3 doi: 10.1016/S0308-8146(99)00170-3
![]() |
[48] |
Rakić V, Ulrih NP (2021) Influence of pH on color variation and stability of cyanidin and cyanidin 3-O-β-glucopyranoside in aqueous solution. CyTA-J Food 19: 174–182. https://doi.org/10.1080/19476337.2021.1874539 doi: 10.1080/19476337.2021.1874539
![]() |
[49] |
Sui X, Dong X, Zhou W (2014) Combined effect of pH and high temperature on the stability and antioxidant activity of two anthocyanins in aqueous solution. Food Chem 163: 163–170. https://doi.org/10.1016/j.foodchem.2014.04.075 doi: 10.1016/j.foodchem.2014.04.075
![]() |
[50] |
Rigolon TCB, Silva RRA, de Oliveira TV, et al. (2024) Exploring anthocyanins-polysaccharide synergies in microcapsule wall materials via spray drying: interaction characterization and evaluation of particle stability. Meas Food 13: 100126. https://doi.org/10.1016/j.meafoo.2023.100126 doi: 10.1016/j.meafoo.2023.100126
![]() |
[51] |
Tena N, Martín J, Asuero AG (2020) State of the art of anthocyanins: antioxidant activity, sources, bioavailability, and therapeutic effect in human health. Antioxidants 9: 451. https://doi.org/10.3390/antiox9050451 doi: 10.3390/antiox9050451
![]() |
[52] |
Floegel A, Kim DO, Chung SJ, et al. (2011) Comparison of ABTS/DPPH assays to measure antioxidant activity in popular antioxidant-rich US foods. J Food Compos Anal 24: 1043–1048. https://doi.org/10.1016/j.jfca.2011.01.008 doi: 10.1016/j.jfca.2011.01.008
![]() |
[53] |
Bayliak MM, Burdyliuk NI, Lushchak VI (2016) Effects of pH on antioxidant and prooxidant properties of common medicinal herbs. Open Life Sci 11: 298–307. https://doi.org/10.1515/biol-2016-0040 doi: 10.1515/biol-2016-0040
![]() |
[54] |
Sun HN, Mu TH, Xi LS (2017) Effect of pH, heat, and light treatments on the antioxidant activity of sweet potato leaf polyphenols. Int J Food Prop 20: 318–332. https://doi.org/10.1080/10942912.2016.1160410 doi: 10.1080/10942912.2016.1160410
![]() |
[55] |
Kungsuwan K, Singh K, Phetkao S, et al. (2014) Effect of pH and anthocycnin concentration on colour and antioxidant activity of Clitorea ternatea extract. Food Appl Biosci J 2: 31–46. https://doi.org/10.14456/fabj.2014.3 doi: 10.14456/fabj.2014.3
![]() |
[56] |
Yu L, Wu Y, Liu D, et al. (2022) The kinetic behavior of antioxidant activity and the stability of aqueous and organic polyphenol extracts from navel orange peel. Food Sci Technol 42 :e90621. https://doi.org/10.1590/fst.90621 doi: 10.1590/fst.90621
![]() |
[57] |
Kamiloglu S, Capanoglu E, Grootaert C, et al. (2015) Anthocyanin absorption and metabolism by human intestinal Caco2-cells—A review. Int J Mol Sci 16: 21555–21574. https://doi.org/10.3390/ijms160921555 doi: 10.3390/ijms160921555
![]() |
[58] |
Kudličková Z, Michalková R, Salayová A, et al. (2023) Design, synthesis, and evaluation of novel indole hybrid chalcones and their antiproliferative and antioxidant activity. Molecules 28: 6583. https://doi.org/10.3390/molecules2818658 doi: 10.3390/molecules2818658
![]() |
1. | Into Almiala, Henrik Aalto, Vesa Kuikka, Influence spreading model for partial breakthrough effects on complex networks, 2023, 630, 03784371, 129244, 10.1016/j.physa.2023.129244 | |
2. | Vesa Kuikka, Detecting Overlapping Communities Based on Influence-Spreading Matrix and Local Maxima of a Quality Function, 2024, 12, 2079-3197, 85, 10.3390/computation12040085 | |
3. | Vesa Kuikka, Kimmo K. Kaski, Detailed-level modelling of influence spreading on complex networks, 2024, 14, 2045-2322, 10.1038/s41598-024-79182-9 | |
4. | Vesa Kuikka, Opinion Formation on Social Networks—The Effects of Recurrent and Circular Influence, 2023, 11, 2079-3197, 103, 10.3390/computation11050103 |