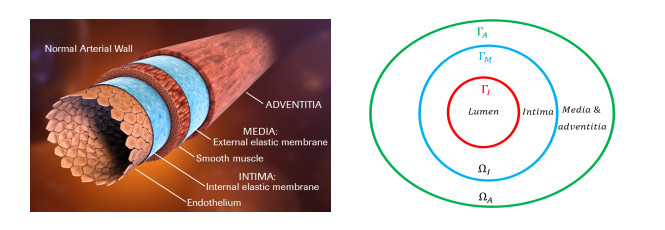
Environmental concern is a determinant in acquiring new green innovation. We aimed to investigate the relationship between environmental concern, consumer attitude, and behavioral intention to switch in the context of drone delivery. The motivation that comes from a green perspective is believed to create a behavior that is also keen on innovative green products. One of the examples is the implementation of drones in delivering parcels, which is believed to cut the carbon footprint. Our purpose was to analyze the direct impact of environmental concern on consumers' behavioral intentions regarding e-commerce drone delivery. Additionally, we aimed to examine the mediating role of consumers' attitudes toward innovation in the relationship between environmental concern and behavioral intention. We sought to provide insights into how environmental awareness and the adoption of innovative delivery technologies like drones can influence consumer behavior, contributing to more sustainable and eco-friendly e-commerce practices. Structured questionnaires were provided to e-commerce users, reliability and validity tests were confirmed, and structural equation modeling (SEM) was used to analyze the relationships among variables. The results of the SEM analysis proved that environmental concern and consumer attitudes have a positive impact on behavioral intention. Customer attitude mediates the relationship between environmental concern and behavioral intention. This research provides a deeper understanding of how environmental concerns influence consumer behavior towards drone delivery innovation within the e-commerce sector. The implications integrate environmental concerns with consumer behavior and innovation adoption, providing a comprehensive view that goes beyond traditional marketing and consumer research.
Citation: Veronica Veronica, Muhtosim Arief, Asnan Furinto, Lim Sanny. E-commerce user's intention to switch toward drone delivery innovation: The role of environmental concern and customers' attitude[J]. AIMS Environmental Science, 2024, 11(5): 847-865. doi: 10.3934/environsci.2024042
[1] | Hengki Tasman, Edy Soewono, Kuntjoro Adji Sidarto, Din Syafruddin, William Oscar Rogers . A model for transmission of partial resistance to anti-malarial drugs. Mathematical Biosciences and Engineering, 2009, 6(3): 649-661. doi: 10.3934/mbe.2009.6.649 |
[2] | Alberto Gambaruto, João Janela, Alexandra Moura, Adélia Sequeira . Shear-thinning effects of hemodynamics in patient-specific cerebral aneurysms. Mathematical Biosciences and Engineering, 2013, 10(3): 649-665. doi: 10.3934/mbe.2013.10.649 |
[3] | Yuyang Xiao, Juan Shen, Xiufen Zou . Mathematical modeling and dynamical analysis of anti-tumor drug dose-response. Mathematical Biosciences and Engineering, 2022, 19(4): 4120-4144. doi: 10.3934/mbe.2022190 |
[4] | Mario Grassi, Giuseppe Pontrelli, Luciano Teresi, Gabriele Grassi, Lorenzo Comel, Alessio Ferluga, Luigi Galasso . Novel design of drug delivery in stented arteries: A numerical comparative study. Mathematical Biosciences and Engineering, 2009, 6(3): 493-508. doi: 10.3934/mbe.2009.6.493 |
[5] | Alberto M. Gambaruto, João Janela, Alexandra Moura, Adélia Sequeira . Sensitivity of hemodynamics in a patient specific cerebral aneurysm to vascular geometry and blood rheology. Mathematical Biosciences and Engineering, 2011, 8(2): 409-423. doi: 10.3934/mbe.2011.8.409 |
[6] | Weirui Lei, Jiwen Hu, Yatao Liu, Wenyi Liu, Xuekun Chen . Numerical evaluation of high-intensity focused ultrasound- induced thermal lesions in atherosclerotic plaques. Mathematical Biosciences and Engineering, 2021, 18(2): 1154-1168. doi: 10.3934/mbe.2021062 |
[7] | Dehua Feng, Xi Chen, Xiaoyu Wang, Xuanqin Mou, Ling Bai, Shu Zhang, Zhiguo Zhou . Predicting effectiveness of anti-VEGF injection through self-supervised learning in OCT images. Mathematical Biosciences and Engineering, 2023, 20(2): 2439-2458. doi: 10.3934/mbe.2023114 |
[8] | Gesham Magombedze, Winston Garira, Eddie Mwenje . Modelling the immunopathogenesis of HIV-1 infection and the effect of multidrug therapy: The role of fusion inhibitors in HAART. Mathematical Biosciences and Engineering, 2008, 5(3): 485-504. doi: 10.3934/mbe.2008.5.485 |
[9] | Avner Friedman, Kang-Ling Liao . The role of the cytokines IL-27 and IL-35 in cancer. Mathematical Biosciences and Engineering, 2015, 12(6): 1203-1217. doi: 10.3934/mbe.2015.12.1203 |
[10] | Xiaoying Wang, Xingfu Zou . Pattern formation of a predator-prey model with the cost of anti-predator behaviors. Mathematical Biosciences and Engineering, 2018, 15(3): 775-805. doi: 10.3934/mbe.2018035 |
Environmental concern is a determinant in acquiring new green innovation. We aimed to investigate the relationship between environmental concern, consumer attitude, and behavioral intention to switch in the context of drone delivery. The motivation that comes from a green perspective is believed to create a behavior that is also keen on innovative green products. One of the examples is the implementation of drones in delivering parcels, which is believed to cut the carbon footprint. Our purpose was to analyze the direct impact of environmental concern on consumers' behavioral intentions regarding e-commerce drone delivery. Additionally, we aimed to examine the mediating role of consumers' attitudes toward innovation in the relationship between environmental concern and behavioral intention. We sought to provide insights into how environmental awareness and the adoption of innovative delivery technologies like drones can influence consumer behavior, contributing to more sustainable and eco-friendly e-commerce practices. Structured questionnaires were provided to e-commerce users, reliability and validity tests were confirmed, and structural equation modeling (SEM) was used to analyze the relationships among variables. The results of the SEM analysis proved that environmental concern and consumer attitudes have a positive impact on behavioral intention. Customer attitude mediates the relationship between environmental concern and behavioral intention. This research provides a deeper understanding of how environmental concerns influence consumer behavior towards drone delivery innovation within the e-commerce sector. The implications integrate environmental concerns with consumer behavior and innovation adoption, providing a comprehensive view that goes beyond traditional marketing and consumer research.
Abdominal aortic aneurysm (AAA) is a leading cause of death globally and involves complex and multi-factorial pathogenesis including factors such as hypertension, dyslipidemia, and tobacco use. Half of patients with untreated aneurysms larger than 5.5 cm will die of rupture within five years, and there is currently no recognized treatment for these AAAs. Patients with AAAs frequently have atherosclerosis, and numerous studies show the association of coronary heart disease and peripheral atherosclerosis with AAA [1]. Whether this association between AAA and atherosclerosis is causal or simply due to common risk factors is unknown. However, the term "atherosclerotic aneurysms" is commonly used and prevalent today. As one of most common causes of AAA, atherosclerosis originates with an accumulation of blood-borne lipids in the inner-most arterial wall (intima), followed by a detailed immune response and the progressive formation of a lesion in the arterial wall [2]. Mature lesions then form atherosclerotic plaques, then arterial walls lose the elasticity via arterial remodeling and develop the AAA. Thus, it is vital to understand the pathophysiology of atherosclerotic aneurysms whose pathology is largely unknown and likely multifactorial [3,4]. Though the pathophysiology is hard to study experimentally due to its network complexity, a computational model could integrate this complex network and include all the possible factors in the pathogenesis. Moreover, computational modeling techniques, such as fluid-structure interactions or computational fluid dynamics, has been successfully implemented in the study of CVD and provides many insightful suggestions for clinical practice [5,6,7,8,9,10,11,12,13,14,15,16,17,18,19,20,21].
In this paper, we develop a mathematical model that accounts not just for the biological networks associated with the pathophysiology of atherosclerotic aneurysms, but also for the multi-layered structure of the arterial wall. Modeling one or two layers would not be sufficient for capturing the progression of the atherosclerotic aneurysm. Therefore our model accounts for the multi-layered structure of the arterial walls, with attention paid to the distinct physical properties of each layer. Biologically speaking, atherosclerosis silently and slowly hardens, narrows, and blocks the arteries, putting blood flow at risk [22]. Then atherosclerotic plaques restrict blood vessels (a process called stenosis) and lead to the destruction of smooth muscle cells (SMCs), depletion of elastin, and disruption of the extracellular matrix (ECM) in the media/adventitia which causes the AAA. Our mathematical model, by including both the mechanism of the arterial wall and the biology of the atherosclerotic aneurysm, can help us to construct the cardiovascular environment virtually and to mimic plaque/aneurysm growth. This is an innovative tool for potential biomarkers' detection, drug efficacy evaluation, and reducing the risk of misdiagnosis due to patient variations. More specifically, we use our model to assess the risk of atherosclerotic aneurysms on a 2D biomarker, the cholesterol ratio and the level of the receptor tyrosine kinase, discoidin domain receptor 1 (DDR1). We further explore the efficacy of three treatments, anti-cholesterol drugs, the anti-DDR1 injection, and the combined therapy. Our simulation results suggest that the dosage of anti-cholesterol drugs is critical for treating atherosclerotic aneurysm patients who can also benefit from additional anti-DDR1 injections.
In order to model the atherosclerotic aneurysm, we present a hybrid computational model which consists of a mechanical model, including the psychical properties and multi-layer structure of arterial wall, and a biological model, including the molecular mechanism of etiology and pathophysiology of atherosclerotic aneurysm.
The arterial wall has a multi-layer structure (see Figure 1), comprising intima, media, and adventitia. [23]. This multi-layered structure is central to the progression atherosclerotic aneurysm which involves changes in all three arterial layers, including changes to their geometry and composition. Modeling the multi-layered structure makes our model possible to render an accurate virtual cardiovascular environment, even recreating and observing the pathophysiological networks of both atherosclerosis (affecting the intima) and aneurysm formation (affecting the media/adventitia).
Porous medium for intima: In light of the intima's high permeability to white cells and platelets [24,25,26], we propose to treat the intima as a porous medium and all the cells in the intima as a low speed flow [27,28] moving with a common velocity, v, which is the result of the movement of macrophages, T cells and SMCs in the intima. The pressure, p, results from the cells' movement and proliferation. This leads to the following Darcy's law and mass conservation equation [27,28]:
{div(v)=f(C,S,M)andv+Kμ∇p=0inΩI(t)p=pB+γκonΓI(t),pn=σn|ΩA+γκnonΓM(t), | (2.1) |
where
f(C,S,M)=λMM+λMCC+λSMMKM+M+λCMM−dMM−dSS−dCCKC+KS+KM, |
which is derived by the biological model for which variables M, S, and C refer to macrophages, SMCs, and collagen, respectively. See next section for more details. Here K is the Darcy permeability of the intima layer, μ is the viscosity of the cell fluid in the intima, pB is the blood pressure (we treat as a constant since we do not include the blood fluid dynamics), γ is a dimensionless surface tension coefficient, κ is the mean curvature of the corresponding free boundaries and σ is the stress tensor determined by the media/adventitia in ΩA(t).
Elasticity for media/adventitia: From the continuum mechanics point of view, mechanical response of the media/adventitia layers of an arterial wall, is a elastic material [29,30]. Specifically, the Cauchy stress tensor is given by
σ=λtr(ε)I+2μsε, | (2.2) |
where μs and λ are material constants, called Lame or elastic moduli coefficients, and ε is the so-called strain stressor defined as
ε=sym(∇u)=12(∇u+∇uT), | (2.3) |
where u is the displacement. Therefore,
σ=λ∇⋅uI+μs(∇u+∇uT). | (2.4) |
The coefficients are directly related to Young's modulus, E, and Poisson's ratio, ν, namely, λ=Eν(1−2ν)(1+ν) and μs=E1+ν. In our model, E=E0−β(SS0−1) where S is the SMCs density and produces the elastic modulus. Then the momentum equation is given by
{dDttu−∇⋅σ=0 in ΩA(t)v=∂tuonΓM(t)σn=0onΓA(t), | (2.5) |
where d is the tissue density. Here we impose the stress-free boundary condition on ΓA and the velocity continuous condition on ΓM(t).
Pathophysiology of atherosclerosis (intima): The atherosclerosis involves a complex series of biological processes with the formation of plaque inside the intima layer. This process begins with a lesion, allowing low-density lipoprotein (LDL), to move from the blood into the intima, where LDL then becomes oxidized (ox-LDL) by free radicals [31]. Because ox-LDL is toxic, macrophages are recruited and differentiated in the intima [32,28]. The ingestion of large amounts of ox-LDL transforms the fatty macrophages into foam cells, which contribute to plaque formation [33,34,35,36]. Moreover, The presence of collagen in the intima also causes activation of macrophages via the DDR1, which is a member of the receptor tyrosine kinase family and binds to several collagen(s) as its ligand, including collagen I, III and IV present in the vasculature [37]. With regard to vascular diseases, it is important to note that DDR1 is expressed in monocytes and macrophages, endothelial cells, SMCs as well as in adventitial fibroblasts. It can thus have a multifaceted role in regulation of vascular diseases such as atherosclerosis and aneurysms. Studies using vessel wall or bone-marrow specific deletion of DDR1 in mice models have elucidated on how DDR1 expression on macrophages mediates their infiltration into the developing plaque, whereas DDR1 expression in resident vessel wall SMCs limits SMC infiltration and lesion fibrosis [38]. The biological network is shown in Figure 2.
Pathophysiology of aneurysm growth (media/adventitia): Aneurysm is due to the destruction of smooth muscle cells (SMCs), depletion of elastin in the media, and disruption of the extracellular matrix (ECM) in the adventitia. Foam cells in the plaque [39,40], degrade elastin and ECM to weaken the pressure of the adventitia layer to withstand blood stress [41]. Foam cells are also known to cause apoptosis in SMCs, which leads to a reduction in elastin and subsequent overall decline of the media's strength [39,40,42].
Equation for Macrophages (M): Activated ECs secrete cytokines like MCP-1 to recruit monocytes to the fatty streak area [43]. The collagens in the intima also causes activation of macrophages. Then the equation of M is written as
{∂M∂t+∇⋅(vM)−DMΔM=λMM+λMCC−dMM in ΩI(t)∂M∂n−α(C)M0=0onΓI(t)∂M∂n=0onΓM(t), | (2.6) |
where λMM is the macrophages proliferation due to the scavenger receptor class A (SR-A) expressed by tissue macrophages [44,45], λMCC accounts for the macrophages activation by the DDR1 [46], dMC represents the natural death of macrophages, α(C)=α0+αCKC+C for which α0 indicates the MCP-1 induced by the oxidized LDL [47] and αCKC+C describes the production of MCP-1 activated by collagens via the DDR1 [48], and M0 is the monocytes density in the blood.
Equation for SMCs (S): Foam cells secrete large amounts of cytokines and growth factors which stimulate the migration of SMCs from the medial layer of the artery into the intima [49]. Then the equation of S is written as
{∂S∂t+∇⋅(∂tuS)−DSΔS=λSχΩA+λSMMKM+MχΩI−dSS in Ω(t)∂S∂n=0onΓIΓA∂S∂n+α(M)S=0onΓM(t), | (2.7) |
where χΩA=1 in ΩA, χΩA=0 in ΩI. S=S0=λSdS represents the SMCs density in health, λSMMKM+M describes the SMCs proliferation due to the stimulation from platelet-derived growth factor (PDGF) produced by activated macrophages [50], and α(M)=αMKM+M accounts for the migration of SMCs due to the foam cells. Since no migration of SMCs occurs on the boundaries ΓI and ΓA, nonflux boundary conditions are specified on them.
Equation for collagen (C): Foam cells form extracellular deposits of lipid material, known as the lipid or necrotic core in the intima while SMCs produce elastin. Thus we have
{∂C∂t+∇⋅(∂tuC)−DCΔC=λCSSχΩA+λCMMχΩI−dCC in Ω(t)[∂C∂n]=α(S0−S)onΓM(t)∂C∂n=0onΓAΓI(t), | (2.8) |
where on ΓM(t), since the 'synthetic' SMCs secrete a matrix rich elastin in the initima [51], we use S0−S account for the 'synthetic' SMCs and model the production through a flux boundary condition.
We cover the healthy case in our model by setting α=0 and α0=0 in Eq (2.6) which means that there is no inflammation in the arterial wall. Moreover, we assume α is proportional to the ratio of the cholesterol levels, LDL/HDL, and refer α=0 in our model to the cholesterol ratio less than 5 [52,53]. Thus we have the density of macrophages in Eq (2.6) be zero. Therefore, there is no migration of SMCs on the interface between the intima and the media in Eq (2.7) which implies that the density of SMCs achieves the steady-state in health, namely, S=S0. Similarly, the density of collagen in Eq (2.8) has steady states C=KC in ΩA and C=0 in ΩI. Thus we have v=0 in Eq (2.1) and u=0 in Eq (2.5). Therefore, there is no plaque formation and no deformation of the arterial wall for the healthy case.
We simulate the hybrid model in the 2D radially symmetric case with finite difference method and moving mesh method [54]. More specifically, for given a function f, we have the following formulas in the polar coordinate with radial symmetry:
∇⋅f=1r∂(rfr)∂r and ∇f=∂fr∂r, |
where fr is the projection of the function f on er. By denoting grid points as r0<r1<⋯≤rN and the numerical approximation of f(ri) as fi, we employ the following numerical schemes to approximate the first and second derivatives with respect to r:
∂f∂r≈−fi(h2i+1−h2i−1)+fi+1h2i−1−fi−1h2i+1hi+1(−h2i−1+hi+1hi−1),∂2f∂r2≈2fi(hi+1−hi−1)+2fi+1hi−1−2fi−1hi+1hi+1(−h2i−1+hi+1hi−1), |
where hi+1=ri+1−ri and hi−1=ri−1−ri. If the grid point is uniformly distributed, these numerical schemes recover the central difference schemes with the second order accuracy by setting hi+1=−hi−1. Due to the free boundaries, we use the moving mesh method to update the grid points [54], namely, ri(t+Δt)=ri(t)+vrΔt. We outline the algorithm of solving the hybrid system with free boundaries in Algorithm 1.
Algorithm 1: The algorithm to solve the hybrid coupled system (Eqs 2.1-2.8) |
![]() |
In order to ensure the numerical stability, we need to satisfy the CFL condition [55], namely, max|v|ΔtΔh≤C, where C is the so-called Courant number between 0 and 1. For simplicity, we used a small fixed Δt in the numerical computation to ensure this stability condition. More specifically, we choose the time step Δt=0.01 day and the initial grid size Δh=0.01 cm, for which the choice has been tested in the simulations to satisfy the CFL condition. The initial conditions have also been specified as:
M=0g/cm−3,S=S0, and C=KC. |
Since the thickness of the abdominal aorta is measured 2.77 cm ± 0.20 cm [56], we set the diameter of lumen, the thickness of intima layer, and the thickness of media layer to be 2 cm, 0.1 cm, and 0.2 cm, respectively, and simulate our model by setting α=1 and α0=0.1 in Eq (2.6) for 2 years. Figure 3 shows the density distributions of macrophages in the intima and SMCs in the media every three months. The macrophage density starts increasing from the boundary ΓI and then spreads to the boundary ΓM in the first six months. Then SMCs start decreasing from month 9-15 and triggers the expansion of the aneurysm after 1 year while the plaque growth starts after 6 months. This is consistent with the pathology of atherosclerotic aneurysm: the inflammation associated with atherosclerosis first leads to destruction and plaque formation then weakening of vascular wall, specifically the tunica media, which leads to aneurysmal dilation of the vessel [57]. In Figure 4 Left, the diameter of the aneurysm grows from 2.60 to 3.48 cm, thus the average aneurysm growth rate is 4.4 mm/year which is in the 95% range of the aneurysm diameter growth rate, 1.0 to 6.1 mm/year [58]. The plaque area is computed by π(R2M−R2I), where RI and RM represents the radius of intima and media, respectively, and grows from 2.72 to 20.37 cm2, thus the growth rate from Y1 to Y2 is around 100%.
Parameter | Description | Value |
DM | diffusion coefficient of cells | 8.64×10−4 cm2 day−1 [27,62,28,63] |
DC | diffusion coefficient of T-cell | 8.64×10−4 cm2 day−1 [27,62,28,63] |
DS | diffusion coefficient of SMCs | 8.64×10−4 cm2 day−1 [27,28] |
λM | production rate of macrophages by SR-A | 1×10−3 day −1 |
λMC | activation rate of macrophages by DDR1 | 1×10−2 day−1 [27,28] |
λS | production rate of SMCs | 5.16×10−1 g/cm−3 day−1 [54] |
λSM | activation rate of SMCs by PDGF | 5×10−3 g/cm−3 day−1 |
λCM | activation rate of ECM due to macrophages | 9×10−2 day−1 [28] |
λCS | activation rate of ECM due to SMCs | 6.17×10−2 day−1 [54] |
dM | death rate of macrophages | 1.5×10−2 day−1 [27,62,28] |
dC | degradation rate of ECM | 3.7×10−1 day−1 [27,62,28] |
dS | death rate of SMCs | 8.6×10−1 day−1 [27,62,28] |
M0 | source/influx of macrophages from blood | 5×10−3 g/cm−3 [28] |
S0 | initial steady state of SMCs | 6×10−1 g/cm−3 [28] |
KC | ECM saturation | 1×10−2 g/cm−3 [28] |
KS | SMCs saturation | 2×10−1 g/cm−3 |
KM | macrophage saturation | 5×10−1 g/cm−3 [28] |
E0 | Young's modulus in health | 5×102 kpa [65,64] |
β | Coefficient of aneurysm tissue material properties | 8×103 kpa [54,66] |
ν | Poisson's ratio | 4.9×10−1 [65] |
Hypertension is a risk factor for the development of atherosclerosis [59] and also has long been suspected to increase the growth rate of abdominal aortic aneurysms [60], although the mechanisms have not been well elucidated. In our model, we studied the effect of blood pressure on the growth of the atherosclerotic aneurysm. The systolic blood pressure corresponds to pB in our model via Eq (2.1) which is taken to be 120 mmHg as the normal blood pressure in Figure 3. We simulate our model for different blood pressure with pB ranging from 90 mmHg (hypotension) to 200 mmHg (hypertensive crisis) [61] and show the results in Figure 4 Right. The effect of blood pressure on the aneurysm diameter varies from 3.46 to 3.51 cm (1.45%), while the effect on the plaque area varies from 20.30 to 20.53 cm2 (1.13%). The effect of blood pressure in our model is not significant due to the early stage of the atherosclerotic aneurysm and also gets larger as the time evolves shown in Figure 4. Moreover, hypertension also induces oxidative stress on the arterial wall [59] and the inflammatory cell infiltration [60]. However, we do not include these mechanisms in the current model so we might underestimate the effect of blood pressure on atherosclerotic aneurysm.
The LDL-cholesterol to HDL-cholesterol (LDL/HDL-cholesterol) ratio is recognized as a good biomarker in atherosclerosis [67]. Although there are no effective biomarkers for aneurysm [68], the level of DDR1 is highly associated with aneurysm growth in mice experiments [69,70]. We will explore the possibility of using the cholesterol ratio and DDR1 as a 2D biomarkers for atherosclerotic aneurysm via our model. As in section 3.1, we refer the cholesterol ratio as the flux rate, α, in Eq (2.6).
The DDR1 is deleted exclusively in vessel wall cells, macrophages can colonize and initiate an inflammatory response in the arterial intima of both Ddr1+/+ and Ddr1-/- hosts. However, vessel-wall specific deletion of DDR1 results in (over 3-fold) increase in the size of aortic sinus plaques due to enhanced collagen accumulation. The cellular composition of the lesions in DDR1-/- hosts shifted toward increased numbers of vessel wall-derived SMCs compared to bone marrow-derived macrophages. In vitro studies confirmed that Ddr1(-/-) SMCs expressed more matrix, proliferated more, and migrated farther than Ddr1(+/+) SMCs. [38]. One plausible cause is that in the absence of DDR1, N-cadherin mediated cell-cell contacts in vSMCs are mislocalised from cell-cell junctions [71], thus facilitating cell invasion. As Ddr1-/- SMCs invade the plaque, their enhanced expression of interstitial matrix genes results in extracellular matrix synthesis and assembly which contributes directly to plaque expansion.
Moreover, DDR1 is also reported to be a negative regulator of collagen(s) in SMCs. The mRNA for collagen type I (and III) was significantly increased in DDR1-/- SMC as compared to DDR1+/+ cells [38,70]. Consistent with these results, overexpression by transient transcription of DDR1 in human SMC was found to induce a significant decrease in collagen type I mRNA, which was accompanied by a similar decrease at the protein level [72]. Besides decreasing the mRNA levels, expression of DDR1 on the cell surface is also reported to inhibit collagen. These multiple effects of DDR1 in suppressing collagen deposition account for increased collagen deposition observed in the adventitial layer of DDR1 KO mice [69]. Therefore we model the level of DDR1 as follows:
● Since DDR1 limits SMC migration [38] and regulates the inflammation [70], α(M) in Eq (2.7) becomes α(M)=αDDR1(MKM+M−dDDR1), where αDDR1 is the SMC migration and dDDR1 accounts for the inflammation regulation;
● Since DDR1 increases SMC-mediated collagen and elastin degradation [73] and is also a central mediator of SMC migration [74], α in Eq (2.8) becomes α=αDDR1.
Thus we define the risk of atherosclerotic aneurysm associated with these two biomarkers as
R(L/H,DDR1)=The outer diameter of the arteryThe inner diameter of the artery, |
where the LDL/HDL ratio, L/H, varies from 5 to 15 [67], the level of DDR1 varies from 5 to 15 μm/ml [70], and the outer/inner diameters of the artery is measured at year 2. The risk, R, combines both the aneurysm growth (the outer diameter) and the plaque size (the inner diameter) and is a good indicator of the progression of the combined disease. The contour plot of the risk map is shown in Figure 5 with two contour curves 1.5 and 2. We have a high-risk region (above 2), a medium-risk region (between 1.5 and 2), and a low-risk region (below 1.5). The DDR1 level contributes significantly to the risk map: if the DDR1 level is low, no matter how large the cholesterol ratio is, the risk remains low; if the DDR1 level is high, even if the cholesterol ratio is low, the risk could be very high. Considering that the DDR1 level remains the same for one patient in the early stage of the aneurysm, thus controlling the cholesterol ratio can still minimize the risk of atherosclerotic aneurysm.
We next test some potential intervention strategies for atherosclerotic aneurysm via our model, e.g. anti-cholesterol drugs, anti-DDR1 injection, and the combined therapy. Statins, only cholesterol-lowering drug class, are recommended for most patients with atherosclerosis to reduce the risk of heart attack or stroke [75]. In our study, we tested the efficacy of Statins on the atherosclerotic aneurysm. Although there are some medications for aneurysm patients whose size is less than 5 cm such as beta blockers and calcium channel blockers [76], these drugs are to lower your blood pressure and relax your blood vessels not related directly to the pathology of aneurysm [77]. Anti-DDR1 has been used in the mice experiments to slow the progression of the aneurysm [78,69]. Therefore, we will test the feasibility of the anti-DDR1 as a treatment for the atherosclerotic aneurysm. More specifically, we will test the three potential intervention strategies as follows:
● Anti-cholesterol drugs, statins, help lower LDL/HDL-cholesterol ratio in our model. We take the flux rate, α, in Eq (2.6) as a control function
α(t)=10−6.5min(t/60,1)10, |
where the initial LDL/HDL-cholesterol ratio is assumed to be 10. One patient starts taking the medications to achieve the ideal cholesterol ratio, 3.5, by 2 months (60 days);
● The anti-DDR1 injection affects the flux rates, α, in Eqs (2.7) and (2.8) and is modeled as follows:
α(t)=10(1−e−t/100), |
where the DDR1 concentration is assumed to be 10 μm/ml initially and returns back to the initial value after one year since the injection;
● The combined therapy of the first two treatments is modeled by changing the flux rates, α, in Eqs (2.6)-(2.8) as the control functions mentioned above.
Figure 6 shows the control functions for both the anti-cholesterol drugs and the anti-DDR1 injection in our study: anti-cholesterol drugs reduces the flux rate consistently after 2 months while the anti-DDR1 injection reduces the flux rate immediately and returns the original flux rate after around 1 year. To explore the three potential treatment strategies, we first let the atherosclerotic aneurysm develop to a certain size, e.g., running our model for 2 years, and then start three treatments for three years. The anit-DDR1 injection is given annually. Figure 7 shows the effects of three different treatments on both the aneurysm diameter and the plaque area. The anti-cholesterol drugs reduce the growth rate of plaque area from 7.19 cm2/year to 2.33 cm2/year and the growth rate of aneurysm diameter from 3.2 mm/year to 1.04 mm/year while the anti-DDR1 injection reduces the growth rates of plaque area and aneurysm diameter to 5.8 cm2/year and 2.56 mm/year, respectively. The combined therapy has the best efficacy by reducing the growth rates of plaque area and aneurysm diameter to 1.42 cm2/year and 0.64 mm/year, respectively. To further explore the efficacy of the combined therapy, we model the dosage of two drugs as follows
α(t)=10−γAmin(t/60,1)10 and α(t)=10(1−γBe−t/100), |
where γA and γB is the dosage of anti-cholesterol drugs and the anti-DDR1 injection, respectively. Then we define the efficacy effectiveness as
E1(γA,γB)=d(γA,γB)d(0,0) and E2(γA,γB)=Area(γA,γB)Area(0,0), |
where d(γA,γB) and Area(γA,γB) are the diameter of aneurysm and the plaque area at Year 5 for given dosage γA and γB, respectively. Thus d(0,0) and Area(0,0) are the control values of no drug treatment. By varying γA from 0.3 to 0.9 with a step size 0.05 and γB from 0.1 to 1 with a stepsize 0.05, we have the efficacy maps for both E1 and E2 plotted in Figure 8 and show that the dosage of anti-cholesterol drugs affects significantly both plaque area and aneurysm diameter. Moreover, for any given dosage of anti-cholesterol drugs, the anti-DDR1 injection can also improve the efficacy of the combined therapy.
More than 80% of aortic aneurysms are associated with atherosclerosis which develops firstly when cholesterol and fat build up inside the arteries. Atherosclerosis accelerates the breakdown of collagen and SMCs, structure and elasticity to the arterial wall. Over time, this causes the arterial walls to weaken and become damaged. As the diameter of the vessel grows, the wall tension increases, leading to even more dilation. The end result is an aneurysm. In this paper, we account for both cardiovascular diseases in the model and develop a comprehensive mathematical model to mimic the progression of the atherosclerotic aneurysm. Our model includes both the multi-layer structure of the arterial wall and the pathophysiology of the atherosclerotic aneurysm. More specifically, we model the plaque formed in the intima layer as a porous medium and use the linear elastic model to describe the media layer; we also include the role of macrophage, SMCs migration, and collagen proliferation into our hybrid model. Then our model is used to predict the progression of atherosclerotic aneurysm, the growth rate of the plaque and the aneurysm diameter. A 2D biomarker, the cholesterol ratio and the level of DDR1, is revealed to evaluate the risk of atherosclerotic aneurysm via our model shown in Figure 5. Then the efficacy of three clinical treatments is also illustrated via our model: the anti-cholesterol treatment is better than the anti-DDR1 injection but the combined therapy is optimal. We accordingly explore the efficacy of the combined therapy with different dosages of the anti-cholesterol treatment and the anti-DDR1 injection. Based on our results shown in Figure 8, we suggest that the anti-cholesterol treatment significantly decreases the risk of the atherosclerotic aneurysm while patients can also benefit from the additional anti-DDR1 injection.
In the current study, we use a simplified circular geometry of the arterial wall with the radially symmetric assumption and do not consider the effect of complex arterial shapes. In the future study, we intend to incorporate the CT scan data of atherosclerotic aneurysm patients into our model and give a personalized prediction for each patient. This would also help us to evaluate the personalized efficacy of different treatments. Another limitation of the current work is the effect of blood pressure via the force balance condition in our model. However, we do not take the biological effects of the blood pressure into consideration, e.g. the inflammatory cell infiltration, therefore, might underestimate the effect of hypertension on the atherosclerotic aneurysm. In the future, we will include a more complex biological network to evaluate the effect of blood pressure. Moreover, the parameters in the current model will be calibrated when clinical data become available. Another future direction is to incorporate a better clinical serum biomarker than DDR1 which is not easily obtainable from a biomarker standpoint. One possible serum biomarker is platelet counts or activated platelet counts since platelets play a significant role in atherosclerosis and have been proved the cross-talk with other inflammatory cells. Furthermore, we plan to validate our suggested treatments to aneurysm patients and quantify the progression of atherosclerosis by using clinical data.
GK and WH carried out all simulations and analyzed the data. GK, CH, GA, KO, MG and WH discussed the project and wrote the article.
Data sharing is not applicable to this article as no new data were created or analyzed in this study.
This work is supported by National Institutes of Health (Grant R01HL124155, CH) and by the American Heart Association (Grant 17SDG33660722, WH).
The authors declare there is no conflict of interest.
[1] | Statista (2023) E-commerce in Indonesia-statistics & Facts. Available from: https://www.statista.com/topics/5742/e-commerce-in-indonesia/#topicOverview. |
[2] | AJ Marketing (2023) Indonesia ecommerce market: Data, trends, top stories. Available from: https://www.indonesia-investments.com/business/business-columns/indonesia-ecommerce-market-data-trends-top-stories/item9630. |
[3] |
Allen J, Piecyk M, Piotrowska M, et al. (2018) Understanding the impact of e-commerce on last-mile light goods vehicle activity in urban areas: The case of London. Transport Res D Tr E 61: 325–338. https://doi.org/10.1016/j.trd.2017.07.020 doi: 10.1016/j.trd.2017.07.020
![]() |
[4] |
Mangiaracina R, Perego A, Seghezzi A, et al. (2019) Innovative solutions to increase last-mile delivery efficiency in B2C e-commerce: A literature review. Int J Phys Distr Log 49: 901–920. https://doi.org/10.1108/IJPDLM-02-2019-0048 doi: 10.1108/IJPDLM-02-2019-0048
![]() |
[5] |
Anvari R (2023) Green, closed loop, and reverse supply chain: A literature review. J Bus Manag 1: 33–57. https://doi.org/10.47747/jbm.v1i1.956 doi: 10.47747/jbm.v1i1.956
![]() |
[6] |
Chen C, Pan S (2016) Using the crowd of taxis to last mile delivery in E-commerce: A methodological research. Serv Orientat Holonic Multi-Ag Manufact 61–70. https://doi.org/10.1007/978-3-319-30337-6_6 doi: 10.1007/978-3-319-30337-6_6
![]() |
[7] |
Anvari R, Askari KOA (2024) Evaluating the efficiency of resource and energy consumption of farmland systems. SSRN Electron J. https://dx.doi.org/10.2139/ssrn.4761505 doi: 10.2139/ssrn.4761505
![]() |
[8] | Lavanya R (2016) The 2015 Paris agreement: Interplay between hard, soft and non-obligations. J Environ law 28: 337–358. https://www.jstor.org/stable/26168923 |
[9] |
Rogelj J, Elzen MD, Hö hne N, et al. (2016) Paris Agreement climate proposals need a boost to keep warming well below 2 ℃. Nature 534: 631–639. https://doi.org/10.1038/nature18307 doi: 10.1038/nature18307
![]() |
[10] |
Maitreyee D, Rangarajan K (2020) Impact of policy initiatives and collaborative synergy on sustainability and business growth of Indian SMEs. Indian Growth Dev Rev 13: 607–627. https://doi.org/10.1108/IGDR-09-2019-0095 doi: 10.1108/IGDR-09-2019-0095
![]() |
[11] |
Muangmee C, Pikiewicz ZD, Meekaewkunchorn N, et al. (2021) Green entrepreneurial orientation and green innovation in small and medium-sized enterprises (Smes). Soc Sci 10: 2021. https://doi.org/10.3390/socsci10040136 doi: 10.3390/socsci10040136
![]() |
[12] |
Raj A, Sah B (2019) Analyzing critical success factors for implementation of drones in the logistics sector using grey-DEMATEL based approach. Comput Ind Eng 138: 106118. https://doi.org/10.1016/j.cie.2019.106118 doi: 10.1016/j.cie.2019.106118
![]() |
[13] |
Kellermann R, Biehle T, Fischer L (2020) Drones for parcel and passenger transportation: A literature review. Transp Res Interdisc 4: 100088. https://doi.org/10.1016/j.trip.2019.100088 doi: 10.1016/j.trip.2019.100088
![]() |
[14] |
Cam LNT (2023) A rising trend in eco-friendly products: A health-conscious approach to green buying. Heliyon 9: e19845. https://doi.org/10.1016/j.heliyon.2023.e19845 doi: 10.1016/j.heliyon.2023.e19845
![]() |
[15] |
Mahesh S, Ramadurai G, Nagendra SMS (2019) Real-world emissions of gaseous pollutants from motorcycles on Indian urban arterials. Transport Res D Tr E 76: 72–84. https://doi.org/10.1016/j.trd.2019.09.010 doi: 10.1016/j.trd.2019.09.010
![]() |
[16] |
Mathew AO, Jha AN, Lingappa AK, et al. (2021) Attitude towards drone food delivery services—role of innovativeness, perceived risk, and green image. J Open Innov Technol Mark Complex 7: 144. https://doi.org/10.3390/joitmc7020144 doi: 10.3390/joitmc7020144
![]() |
[17] | Dileep MR, Navaneeth AV, Ullagaddi S, et al. (2020) A study and analysis on various types of agricultural drones and its applications, in 2020 Fifth International Conference on Research in Computational Intelligence and Communication Networks, 181–185. https://doi.org/10.1109/ICRCICN50933.2020.9296195 |
[18] |
Puri V, Nayyar A, Raja L (2017) Agriculture drones: A modern breakthrough in precision agriculture. J Stat Manag Syst 20: 507–518. https://doi.org/10.1080/09720510.2017.1395171 doi: 10.1080/09720510.2017.1395171
![]() |
[19] |
Nwaogu JM, Yang Y, Chan AP, et al. (2023) Application of drones in the architecture, engineering, and construction (AEC) industry. Automat Constr 150. https://doi.org/10.1016/j.autcon.2023.104827 doi: 10.1016/j.autcon.2023.104827
![]() |
[20] |
Goodchild A, Toy J (2018) Delivery by drone: An evaluation of unmanned aerial vehicle technology in reducing CO2 emissions in the delivery service industry. Transport Res D Tr E 61: 58–67. https://doi.org/10.1016/j.trd.2017.02.017 doi: 10.1016/j.trd.2017.02.017
![]() |
[21] | Clutch (2020) Drone delivery: Benefits and challenges. Available from: https://clutch.co/logistics/resources/drone-delivery-statistics-benefits-challenges. |
[22] |
Hwang J, Choe JY (2019) Exploring perceived risk in building successful drone food delivery services. Int J Contemp Hosp M 31: 3249–3269. https://doi.org/10.1108/IJCHM-07-2018-0558 doi: 10.1108/IJCHM-07-2018-0558
![]() |
[23] |
Yoo W, Yu E, Jung J (2018) Drone delivery: Factors affecting the public's attitude and intention to adopt. Telemat Inform 35: 1687–1700. https://doi.org/10.1016/j.tele.2018.04.014 doi: 10.1016/j.tele.2018.04.014
![]() |
[24] |
Hu ZH, Huang YL, Li YN, et al. (2024) Drone-based instant delivery hub-and-spoke network optimization. Drones 8. https://doi.org/10.3390/drones8060247 doi: 10.3390/drones8060247
![]() |
[25] |
Chiang WC, Li Y, Shang J, et al. (2019) Impact of drone delivery on sustainability and cost: Realizing the UAV potential through vehicle routing optimization. Appl Energy 242: 1164–1175. https://doi.org/10.1016/j.apenergy.2019.03.117 doi: 10.1016/j.apenergy.2019.03.117
![]() |
[26] |
Adnan N, Nordin SM, Rahman I, et al. (2017) A new era of sustainable transport: An experimental examination on forecasting adoption behavior of EVs among Malaysian consumer. Transport Res A-Pol 103: 279–295. https://doi.org/10.1016/j.tra.2017.06.010 doi: 10.1016/j.tra.2017.06.010
![]() |
[27] |
Baeshen Y, Soomro YA, Bhutto MY (2021) Determinants of green innovation to achieve sustainable business performance: Evidence from SMEs. Front Psychol 12: 767968. https://doi.org/10.3389/fpsyg.2021.767968 doi: 10.3389/fpsyg.2021.767968
![]() |
[28] |
Rodrigues TA, Patrikar J, Oliveira NL, et al. (2022) Drone flight data reveal energy and greenhouse gas emissions savings for very small package delivery. Patterns 3: 100569. https://doi.org/10.1016/j.patter.2022.100569 doi: 10.1016/j.patter.2022.100569
![]() |
[29] |
Balderjahn I (1988) Personality variables and environmental attitudes as predictors of ecologically responsible consumption patterns. J Bus Res 17: 51–56. https://doi.org/10.1016/0148-2963(88)90022-7 doi: 10.1016/0148-2963(88)90022-7
![]() |
[30] |
Crosby LA, Taylor JR (1982) Consumer satisfaction with Michigan's container deposit law: An ecological perspective. J Mark 46: 47–60. https://doi.org/10.2307/1251159 doi: 10.2307/1251159
![]() |
[31] |
Thieme J, Royne MB, Jha S, et al. (2015) Factors affecting the relationship between environmental concern and behaviors. Mark Intell Plan 33: 675–690. https://doi.org/10.1108/MIP-08-2014-0149 doi: 10.1108/MIP-08-2014-0149
![]() |
[32] |
Ozaki R, Sevastyanova K (2011) Going hybrid: An analysis of consumer purchase motivations. Energy Policy 39: 2217–2227. https://doi.org/10.1016/j.enpol.2010.04.024 doi: 10.1016/j.enpol.2010.04.024
![]() |
[33] |
Teoh CW, Gaur SS (2019) Environmental concern: An issue for poor or rich. Manag Environ Qual 30: 227–242. https://doi.org/10.1108/MEQ-02-2018-0046 doi: 10.1108/MEQ-02-2018-0046
![]() |
[34] |
Bamberg S (2003) How does environmental concern influence specific environmentally related behaviors? A new answer to an old question. J Environ Psychol 23: 21–32. https://doi.org/10.1016/S0272-4944(02)00078-6 doi: 10.1016/S0272-4944(02)00078-6
![]() |
[35] |
Stafford MR, Stafford TF, Collier JE (2006) The dimensionality of environmental concern: Validation of component measures. Interdiscip Environ Rev 8: 43–61. https://doi.org/10.1504/IER.2006.053946 doi: 10.1504/IER.2006.053946
![]() |
[36] |
Ajzen I (1991) The theory of planned behavior. Organ Behav Hum Dec 50: 179–211. https://doi.org/10.1016/0749-5978(91)90020-T doi: 10.1016/0749-5978(91)90020-T
![]() |
[37] | Schiffman LG, Kanuk LL (2004) Consumer behavior, 8th International Edition, Prentice Hall. |
[38] | Kotler P, (2009) Marketing management, Jakarta: Erlangga. |
[39] | Hawkins DI, Mothersbaugh DL (2013) Consumer behavior: Building marketing strategy, New York: McGraw-Hill Irwin. |
[40] |
Crosby LA, Gill JD, Taylor JR (1981) Consumer voter behavior in the passage of the Michigan container law. J Mark 45: 19–32. https://doi.org/10.1177/002224298104500203 doi: 10.1177/002224298104500203
![]() |
[41] |
Bettencourt LA, Hughner RS, Kuntze RJ, et al. (1999) Lifestyle of the tight and frugal: Theory and measurement. J Consum Res 26: 85–99. https://doi.org/10.1086/209552 doi: 10.1086/209552
![]() |
[42] | Schiffman LG, Wisenblit J (2015) Consumer behavior, England: Pearson Education Limited, 2015. |
[43] |
Agarwal J, Malhotra NK (2005) An integrated model of attitude and affect: Theoretical foundation and an empirical investigation. J Bus Res 58: 483–493. https://doi.org/10.1016/S0148-2963(03)00138-3 doi: 10.1016/S0148-2963(03)00138-3
![]() |
[44] |
Reaños MAT, Meier D, Curtis J, et al. (2023) The role of energy, financial attitudes and environmental concerns on perceived retrofitting benefits and barriers: Evidence from Irish home owners. Energy Buildings 297: 113448. https://doi.org/10.1016/j.enbuild.2023.113448 doi: 10.1016/j.enbuild.2023.113448
![]() |
[45] |
De Groot J, Steg L (2007) General beliefs and the theory of planned behavior: The role of environmental concerns in the TPB. J Appl Soc Psychol 37: 1817–1836. https://doi.org/10.1111/j.1559-1816.2007.00239.x doi: 10.1111/j.1559-1816.2007.00239.x
![]() |
[46] |
Lau JL, Hashim AH (2020) Mediation analysis of the relationship between environmental concern and intention to adopt green concepts. Smart Sustain Built 9: 539–556. https://doi.org/10.1108/SASBE-09-2018-0046 doi: 10.1108/SASBE-09-2018-0046
![]() |
[47] |
Li D, Zhao L, Ma S, et al. (2019) What influences an individual's pro-environmental behavior? A literature review. Resour Conserv Recy 146: 28–34. https://doi.org/10.1016/j.resconrec.2019.03.024 doi: 10.1016/j.resconrec.2019.03.024
![]() |
[48] |
Jaiswal D, Kaushal V, Kant R, et al. (2021) Consumer adoption intention for electric vehicles: Insights and evidence from Indian sustainable transportation. Technol Forecast Soc 173: 121089. https://doi.org/10.1016/j.techfore.2021.121089 doi: 10.1016/j.techfore.2021.121089
![]() |
[49] |
Yue B, Sheng G, She S, et al. (2020) Impact of consumer environmental responsibility on green consumption behavior in China: The role of environmental concern and price sensitivity. Sustainability 12. https://doi.org/10.3390/su12052074 doi: 10.3390/su12052074
![]() |
[50] | SIRCLO (2021) Navigating Indonesia's e-commerce: Omnichannel as the future of retail. Available from: https://www.sirclo.com/research-reports/navigating-indonesia-s-e-commerce-omnichannel-as-the-future-of-retail. |
[51] | Sekaran U, Bougie R (2016) Research methods for business: A skill-building approach, 7 Eds., Chichester, West Sussex, United Kingdom: John Wiley & Sons. |
[52] |
Bentler PM, Chou CP (1987) Practical issues in structural modeling. Sociol Method Res 16: 78–117. https://doi.org/10.1177/0049124187016001004 doi: 10.1177/0049124187016001004
![]() |
[53] | Nunnally JC, Bernstein IH (1967) Psychometric theory, New York: McGraw-Hill. |
1. | Shankar Narayan S, Sunanda Saha, Time-dependent study of blood flow in an aneurysmic stenosed coronary artery with inelastic walls, 2021, 47, 22147853, 4718, 10.1016/j.matpr.2021.05.608 | |
2. | Yatao Liu, Mathematical modeling and simulation of atherosclerotic formation and progress at arterial bifurcations, 2023, 80, 17468094, 104266, 10.1016/j.bspc.2022.104266 | |
3. | Ali A. Rostam-Alilou, Hamid R. Jarrah, Ali Zolfagharian, Mahdi Bodaghi, Fluid–structure interaction (FSI) simulation for studying the impact of atherosclerosis on hemodynamics, arterial tissue remodeling, and initiation risk of intracranial aneurysms, 2022, 21, 1617-7959, 1393, 10.1007/s10237-022-01597-y | |
4. | Shankar Narayan S., Anuradha Bhattacharjee, Sunanda Saha, CFD analysis of the hyper-viscous effects on blood flow across abdominal aortic aneurysm in COVID patients: multiphysics approach, 2023, 1025-5842, 1, 10.1080/10255842.2023.2194474 | |
5. | Egle Kavaliunaite, Thomas Emil Andersen, Jes S. Lindholt, Jane Stubbe, Daily fenugreek intake does not attenuate abdominal aortic aneurysm growth in rats, 2025, 0301-1526, 10.1024/0301-1526/a001185 |
Algorithm 1: The algorithm to solve the hybrid coupled system (Eqs 2.1-2.8) |
![]() |
Parameter | Description | Value |
DM | diffusion coefficient of cells | 8.64×10−4 cm2 day−1 [27,62,28,63] |
DC | diffusion coefficient of T-cell | 8.64×10−4 cm2 day−1 [27,62,28,63] |
DS | diffusion coefficient of SMCs | 8.64×10−4 cm2 day−1 [27,28] |
λM | production rate of macrophages by SR-A | 1×10−3 day −1 |
λMC | activation rate of macrophages by DDR1 | 1×10−2 day−1 [27,28] |
λS | production rate of SMCs | 5.16×10−1 g/cm−3 day−1 [54] |
λSM | activation rate of SMCs by PDGF | 5×10−3 g/cm−3 day−1 |
λCM | activation rate of ECM due to macrophages | 9×10−2 day−1 [28] |
λCS | activation rate of ECM due to SMCs | 6.17×10−2 day−1 [54] |
dM | death rate of macrophages | 1.5×10−2 day−1 [27,62,28] |
dC | degradation rate of ECM | 3.7×10−1 day−1 [27,62,28] |
dS | death rate of SMCs | 8.6×10−1 day−1 [27,62,28] |
M0 | source/influx of macrophages from blood | 5×10−3 g/cm−3 [28] |
S0 | initial steady state of SMCs | 6×10−1 g/cm−3 [28] |
KC | ECM saturation | 1×10−2 g/cm−3 [28] |
KS | SMCs saturation | 2×10−1 g/cm−3 |
KM | macrophage saturation | 5×10−1 g/cm−3 [28] |
E0 | Young's modulus in health | 5×102 kpa [65,64] |
β | Coefficient of aneurysm tissue material properties | 8×103 kpa [54,66] |
ν | Poisson's ratio | 4.9×10−1 [65] |
Algorithm 1: The algorithm to solve the hybrid coupled system (Eqs 2.1-2.8) |
![]() |
Parameter | Description | Value |
DM | diffusion coefficient of cells | 8.64×10−4 cm2 day−1 [27,62,28,63] |
DC | diffusion coefficient of T-cell | 8.64×10−4 cm2 day−1 [27,62,28,63] |
DS | diffusion coefficient of SMCs | 8.64×10−4 cm2 day−1 [27,28] |
λM | production rate of macrophages by SR-A | 1×10−3 day −1 |
λMC | activation rate of macrophages by DDR1 | 1×10−2 day−1 [27,28] |
λS | production rate of SMCs | 5.16×10−1 g/cm−3 day−1 [54] |
λSM | activation rate of SMCs by PDGF | 5×10−3 g/cm−3 day−1 |
λCM | activation rate of ECM due to macrophages | 9×10−2 day−1 [28] |
λCS | activation rate of ECM due to SMCs | 6.17×10−2 day−1 [54] |
dM | death rate of macrophages | 1.5×10−2 day−1 [27,62,28] |
dC | degradation rate of ECM | 3.7×10−1 day−1 [27,62,28] |
dS | death rate of SMCs | 8.6×10−1 day−1 [27,62,28] |
M0 | source/influx of macrophages from blood | 5×10−3 g/cm−3 [28] |
S0 | initial steady state of SMCs | 6×10−1 g/cm−3 [28] |
KC | ECM saturation | 1×10−2 g/cm−3 [28] |
KS | SMCs saturation | 2×10−1 g/cm−3 |
KM | macrophage saturation | 5×10−1 g/cm−3 [28] |
E0 | Young's modulus in health | 5×102 kpa [65,64] |
β | Coefficient of aneurysm tissue material properties | 8×103 kpa [54,66] |
ν | Poisson's ratio | 4.9×10−1 [65] |