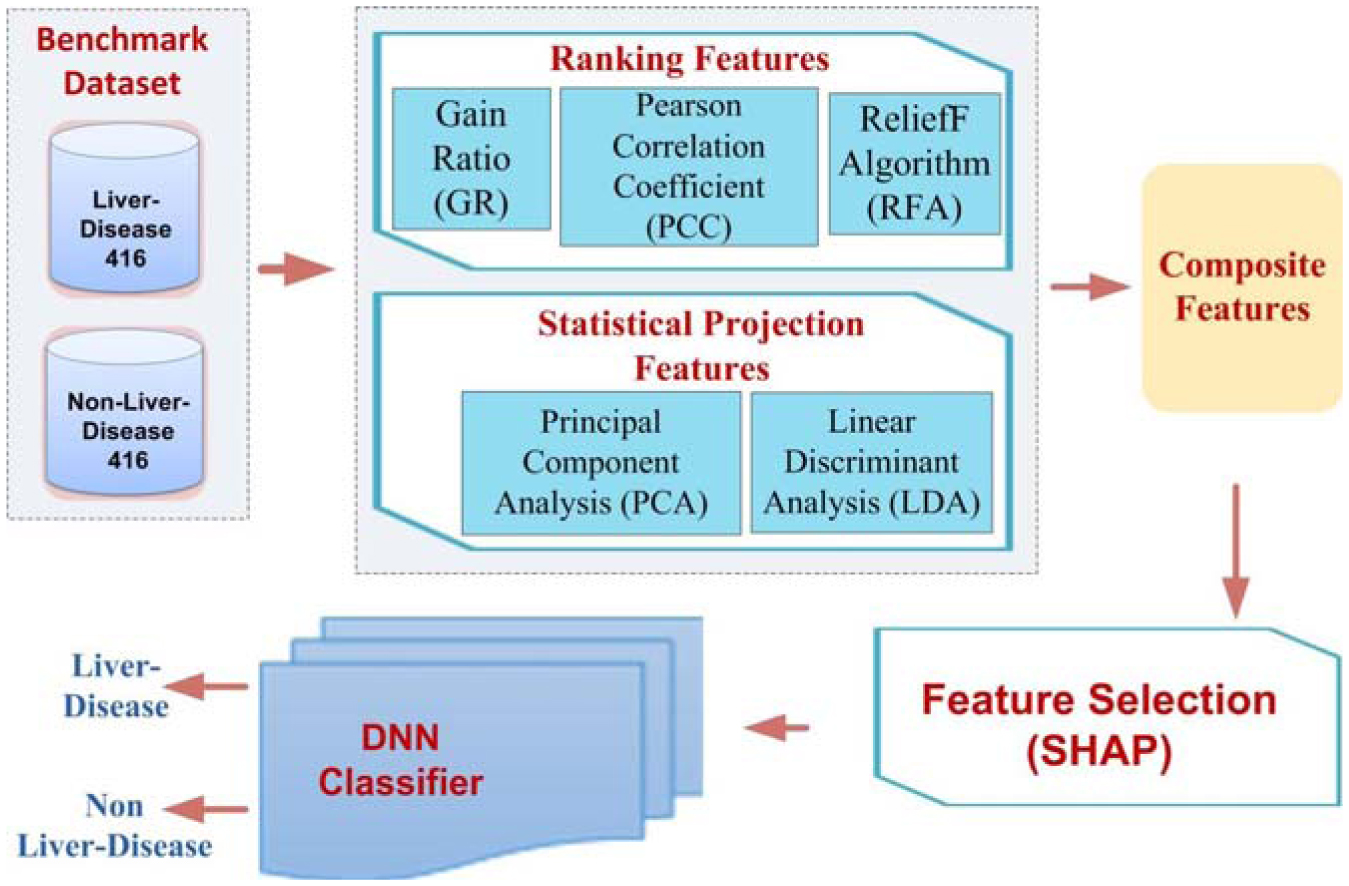
Submersible vehicles may be regarded as complex systems because of their complex interaction with the surrounding fluid. This paper presents a mathematical model of a submersible vehicle formulated in a coordinate-free manner through the language of Lie groups and Lie algebras. The d'Alembert virtual-work principle was applied in conjunction with the minimal-action principle for a rigid body in order to incorporate into the mathematical model external influences such as fluid-current-induced deflection and control inputs. Such a method from mathematical physics can also take into consideration how a vehicle interacts with the fluid it is immersed in under the form of added (or virtual) mass. The resulting equations of motion were given over the Lie group of three-dimensional rotations as (non-pure) Euler-Poincaré relations. A numerical simulation technique based on Lie-group integrators was also briefly recalled and deployed to simulate the behavior of such mathematical model of an existing, academic-design-type submersible vehicle.
Citation: Simone Fiori. Coordinate-free Lie-group-based modeling and simulation of a submersible vehicle[J]. AIMS Mathematics, 2024, 9(4): 10157-10184. doi: 10.3934/math.2024497
[1] | Islam Uddin, Salman A. AlQahtani, Sumaiya Noor, Salman Khan . Deep-m6Am: a deep learning model for identifying N6, 2′-O-Dimethyladenosine (m6Am) sites using hybrid features. AIMS Bioengineering, 2025, 12(1): 145-161. doi: 10.3934/bioeng.2025006 |
[2] | Shital Hajare, Rajendra Rewatkar, K.T.V. Reddy . Design of an iterative method for enhanced early prediction of acute coronary syndrome using XAI analysis. AIMS Bioengineering, 2024, 11(3): 301-322. doi: 10.3934/bioeng.2024016 |
[3] | Wallace Camacho Carlos, Alessandro Copetti, Luciano Bertini, Leonard Barreto Moreira, Otávio de Souza Martins Gomes . Human activity recognition: an approach 2D CNN-LSTM to sequential image representation and processing of inertial sensor data. AIMS Bioengineering, 2024, 11(4): 527-560. doi: 10.3934/bioeng.2024024 |
[4] | Rabia Aziz, C.K. Verma, Namita Srivastava . Dimension reduction methods for microarray data: a review. AIMS Bioengineering, 2017, 4(1): 179-197. doi: 10.3934/bioeng.2017.1.179 |
[5] | Kayode Oshinubi, Augustina Amakor, Olumuyiwa James Peter, Mustapha Rachdi, Jacques Demongeot . Approach to COVID-19 time series data using deep learning and spectral analysis methods. AIMS Bioengineering, 2022, 9(1): 1-21. doi: 10.3934/bioeng.2022001 |
[6] | Marianthi Logotheti, Eleftherios Pilalis, Nikolaos Venizelos, Fragiskos Kolisis, Aristotelis Chatziioannou . Development and validation of a skin fibroblast biomarker profile for schizophrenic patients. AIMS Bioengineering, 2016, 3(4): 552-565. doi: 10.3934/bioeng.2016.4.552 |
[7] | Abdulmajeed Alsufyani . Performance comparison of deep learning models for MRI-based brain tumor detection. AIMS Bioengineering, 2025, 12(1): 1-21. doi: 10.3934/bioeng.2025001 |
[8] | Lamia Fatiha KAZI TANI, Mohammed Yassine KAZI TANI, Benamar KADRI . Gas-Net: A deep neural network for gastric tumor semantic segmentation. AIMS Bioengineering, 2022, 9(3): 266-282. doi: 10.3934/bioeng.2022018 |
[9] | Kuna Dhananjay Rao, Mudunuru Satya Dev Kumar, Paidi Pavani, Darapureddy Akshitha, Kagitha Nagamaleswara Rao, Hafiz Tayyab Rauf, Mohamed Sharaf . Cardiovascular disease prediction using hyperparameters-tuned LSTM considering COVID-19 with experimental validation. AIMS Bioengineering, 2023, 10(3): 265-282. doi: 10.3934/bioeng.2023017 |
[10] | Xiao-Li Gu, Li Yu, Yu Du, Xiu-Peng Yang, Yong-Gang Xu . Identification and validation of aging-related genes and their classification models based on myelodysplastic syndromes. AIMS Bioengineering, 2023, 10(4): 440-465. doi: 10.3934/bioeng.2023026 |
Submersible vehicles may be regarded as complex systems because of their complex interaction with the surrounding fluid. This paper presents a mathematical model of a submersible vehicle formulated in a coordinate-free manner through the language of Lie groups and Lie algebras. The d'Alembert virtual-work principle was applied in conjunction with the minimal-action principle for a rigid body in order to incorporate into the mathematical model external influences such as fluid-current-induced deflection and control inputs. Such a method from mathematical physics can also take into consideration how a vehicle interacts with the fluid it is immersed in under the form of added (or virtual) mass. The resulting equations of motion were given over the Lie group of three-dimensional rotations as (non-pure) Euler-Poincaré relations. A numerical simulation technique based on Lie-group integrators was also briefly recalled and deployed to simulate the behavior of such mathematical model of an existing, academic-design-type submersible vehicle.
The liver is a vital organ in the human body that maintains basic physiological activities necessary for health. The liver is essential for digestion, metabolism, detoxification, and immunity, highlighting its critical role in maintaining bodily equilibrium. Despite being one of the most diverse and intricate organs, the liver is susceptible to infections, traumas, and genetic disorders, jeopardizing human life and longevity [1],[2]. These disorders will adversely affect the liver and lead to severe consequences; thus, preventative actions and cures for general liver issues are essential. However, persistent injury to this organ may disrupt its activities and overall health despite its significant ability for renewal and adaptation. Consequently, understanding the factors that lead to liver function deterioration, inflammation, and jaundice, alongside pursuing timely diagnosis and prevention, are two essential steps toward enhancing the quality of life and mitigating mortality risks associated with liver diseases (LD).
The healthcare industry has seen significant transformations in its delivery systems and organizations as a result of data processing, primarily via the use of machine learning (ML) and artificial intelligence (AI) [3]. These innovations have transformed data collection, storage, and analysis methods, allowing clinical practitioners to integrate cutting-edge decision-making models into traditional healthcare procedures. This integration has significantly enhanced inspection and informed decision-making in the healthcare industry. It is important to note that, because of the progressive advancement of AI and ML applications, early illness prediction is feasible for conditions such as diabetes, hypertension, COPD, and cardiovascular diseases [4]. These technologies use big data to provide significant insights into a specific diagnosis, aiding illness prognosis management and treatment. Although closely linked with technology, we must recognize that preventative efforts promoting healthy behavior have enhanced the positive transformation this innovation brings. Integrating AI and ML into healthcare procedures is already underway, with potential advancements in precision medicine, medication discovery, and diagnostic tools. The following sections illustrate that these technological advancements include enhancements in healthcare delivery, including improved diagnostics, more focused medication, and overall population health outcomes.
Researchers have proposed many machine learning techniques in the literature for accurately and quickly classifying liver patients. In 2016, Babu et al. [5] introduced a K-means clustering methodology for identifying liver illness, using several classification models to assess its effectiveness. Their study evaluated the efficacy of the Naive Bayes (NB), C4.5 decision tree, and k-nearest neighbors (KNN) classifiers. The results indicated that NBC obtained an accuracy of 56%, KNN reached 64%, and C4.5 attained 69%. This approach illustrates the differential efficacy of several classifiers in conjunction with k-means clustering for identifying liver patients. Likewise, Gan et al. [6] investigated several categorization methods for predicting liver illness. They suggested that the AdaC-TANBN approach had an accuracy of 69.03%. The integrated strategy, which merges AdaBoost with a modified tree-augmented Naive Bayes (TANBN) model, outperformed other classification techniques evaluated in their research. The result highlights the efficacy of integrating boosting methods with probabilistic models to improve prediction accuracy in liver disease categorization. Subsequently, Amare et al. [7] proposed an innovative predictor for identifying LD via sophisticated machine-learning techniques. They created a classification model that combines the complement Naive Bayes (CNB) technique with other classifiers to improve predictive accuracy, comparing it to traditional Naive Bayes classifiers and other models. Their results demonstrated that the CNB approach achieved an accuracy of 71.36%, exceeding the performance of many classifiers analyzed in the literature. This method underscores the efficacy of the CNB technique in improving the accuracy of liver disease categorization. Sreejith and colleagues [8] evaluated classification performance using the ILPD, Pima Indian Diabetes (PID), and Thoracic Surgery (TSD) datasets. They used chaotic multi-verse optimization (CMVO) for feature selection and the synthetic minority oversampling technique (SMOTE), achieving an accuracy of 82.62% on the ILPD dataset with enhanced methodologies. P. Kumar et al. improved liver disease classification accuracy with the ILPD dataset. They used 10-fold cross-validation and utilized variable neighbor-weighted fuzzy KNN, neighbor-weighted K-NN (NWKNN), and fuzzy-neighbor-weighted K-NN classifiers. To address dataset imbalance, they used Tomek connections and redundancy-based under-sampling (TR-RUS), attaining accuracies of 72.31% with NWKNN, 76.61% with fuzzy NWKNN, and 87.71% with their developed variable-NWFKNN approach, which surpassed existing classifiers. Kuzhippallil et al. [9] enhanced the categorization of chronic liver disease by several data preparation and feature selection techniques. Their methodologies improved classification efficacy, achieving a maximum accuracy of 88%. Ruhul et al. [10] recently presented a sophisticated feature extraction and classification technique for predicting LD with the ILPD dataset. Their study integrated projection-based statistical methods to enhance feature selection with relevant machine learning approaches, including support vector machine, logistic regression, and random forest. The suggested technique improves the accuracy of liver disease diagnosis to 88.10%, facilitating early identification and treatment alternatives.
This research introduces a precise and robust learning approach to successfully differentiate liver disease. We first use the random oversampling strategy to address overfitting and class imbalance during model building. Subsequently, we employ two feature methodologies: ranking and statistical projection-based strategies. The Pearson correlation coefficient (PCC), gain ratio (GR), and ReliefF algorithm (RFA) are utilized to rank and evaluate each feature's contribution to the target class (liver illness). We use principal component analysis (PCA) and linear discriminant analysis (LDA) for statistical projection-based techniques. A hybrid feature vector is generated by combining these five feature vectors. We use a feature selection method, Shapley additive explanations (SHAP) [11], to improve computational efficiency, identifying the most significant characteristics by analyzing their impact on model predictions. Diverse hypothesis learners, such as logistic regression (LR), k-nearest neighbor (KNN), random forest (RF), support vector machine (SVM), and Naive Bayes (NB), are used to assess prediction rates. A thorough performance evaluation using measures such as Matthew's correlation coefficient, accuracy, sensitivity, specificity, and area under the curve (AUC) illustrates the enhanced efficacy of our proposed DNN model in predicting liver disease. This methodology offers significant insights into predicting early liver disease, improving diagnostic accuracy, and enabling prompt medical treatments.
This section delivers a detailed, illustrated representation of data transfers via the prototype and architecture of a suggested predictor model. Figure 1 illustrates essential design components to be entirely explored in subsequent sections.
The dataset under study, the ILPD dataset [10], comprises 583 observations and encompasses 10 distinct features. A comprehensive summary of the dataset ILPD is presented in Table 1. The dataset under consideration comprises gender distribution revealing 439 male participants, constituting approximately 75.30%, and 144 female participants, accounting for 24.70% of the total cohort. The dataset's primary target class revolves around diagnosing liver disease, distinguishing participants based on whether they have been diagnosed with this condition.
Feature | Description |
Gender | Participant's gender |
Albumin (ALB) | Albumin level |
Direct bilirubin (DB) | Direct bilirubin level |
Age (years) | Age of participants (4–90 years) |
Total bilirubin (TB) | Total bilirubin level |
Aspartate aminotransferase (SGOT) | Aspartate aminotransferase level |
Total protein (TP) | Total protein level |
Alkaline phosphatase (ALP) | Alkaline phosphatase level |
Alanine aminotransferase (SGPT) | Alanine aminotransferase level |
Albumin and globulin ratio (AGR) | Albumin and globulin ratio |
The long-term liver disease risk is addressed as a classification challenge with Liver-Disease (LD) or Non-Liver-Disease (non-LD) classes. The total dataset comprises 416 positive cases of liver disease and 167 controlled cases. Considering the significance of balanced datasets in machine learning analysis, we applied a data balancing technique, i.e., random oversampling, resulting in a dataset of 832 cases, evenly distributed between instances of liver disease and non-disease cases (i.e., LD = 416, non-LD = 416). Finally, Table 2 outlines the balanced dataset statistical properties of the features, highlighting essential measurements, i.e., standard deviation, mean, minimum, and maximum, for each variable. This analysis provides a complete analysis of the dataset's numerical distributions. Table 2 summarizes the statistical properties of features, including min, max, mean, and standard deviation, providing numerical distribution analysis.
Features | Min | Mean | Max | ± Stdv |
TB | 0.4 | 2.65 | 75 | ± 5.32 |
SGOT | 10 | 88.78 | 4929 | ± 245.07 |
Age | 4 | 43.55 | 90 | ± 16.28 |
ALP | 63 | 267.26 | 2110 | ± 212.62 |
SGPT | 10 | 66.78 | 2000 | ± 155.16 |
AGR | 0.3 | 0.98 | 2.8 | ± 0.30 |
DB | 0.1 | 1.16 | 19.7 | ± 2.42 |
ALB | 0.9 | 3.19 | 5.5 | ± 0.76 |
TP | 2.7 | 6.5 | 9.6 | ± 1.02 |
ML algorithms often face the obscenity of dimensionality, which arises when there are many data points but relatively few meaningful features or when the feature space contains irrelevant information. To address this challenge, we first implement three ranking strategies [12],[13], i.e., Pearson correlation coefficient (PCC), gain ratio (GR), and ReliefF algorithm (RFA), and statistical projection-based strategies such as principal component analysis (PCA) and linear discriminant analysis (LDA) to examine the involvement of each feature to the target class (i.e., liver disease). Subsequently, we use Shapley additive explanations (SHAP) to determine how much each feature matters. These techniques help simplify the dataset by focusing only on the most important parts, making it easier to analyze and reducing the computing power needed. These methods are crucial to finding the most valuable features when predicting liver disease, leading to more accurate and understandable diagnostic and prediction models [14],[15].
In this section, three ranking methods, i.e., PCC, GR, and RFA, are nominated to calculate the involvement of a feature (i.e., LD class). Table 3 provides an evaluation of feature contributions to predicting the target class.
Feature | PCC | GR | RFA |
SGPT | 0.2141 | 0.0701 | 0.2613 |
TB | 0.2874 | 0.1373 | 0.2848 |
Gender | 0.0857 | 0.0065 | 0.025 |
SGOT | 0.2017 | 0.1005 | 0.2393 |
AGR | 0.2046 | 0.0822 | 0.2599 |
DB | 0.3205 | 0.1421 | 0.2895 |
ALB | 0.1836 | 0.0408 | 0.2883 |
Age | 0.1596 | 0.0372 | 0.2625 |
ALP | 0.246 | 0.0867 | 0.1936 |
TP | 0.0443 | 0.00 | 0.2161 |
First, using PCC analysis, some significant relationships were detected, including highly significant ones, for example, 0.88, indicating a relationship between TB and DB. The percentage for this association was comparatively low, at 0.32. DB remained the most frequent feature with 32 DDR, followed by task-interdependent, task-supportive, and task-contrast with 14 DDR, 13 DDR, 11 DDR, and 5 DDR, respectively. Second, the GR method used in the “screening variable selection” highlighted DB, TB, and SGOT as prominent ones. Third, using the Gini impurity ReliefF algorithm suggested that gender had the least priority, whereas DB, ALP, and TB were the least essential features. These methods all emphasize the importance of all features in screening for liver diseases.
PCA reduces data dimensions using covariance matrices [16] with minimum loss of discriminative features. Let us consider a feature vector S with the dimension of i*j, where i represents the number of extracted features and j represents the number of samples. Let k represent the number of desired features. The value of k must be less than i. Let us consider the following input feature vector S using Eq. (1):
Through the PCA algorithm, we implemented the following steps to reduce the dimensionality of the feature vector:
Step 1: Compute the mean
Step 2: Subtract the mean
Step 3: Compute the covariance
Where
Step 4: Calculate the eigenvalues in ascending order. The first eigenvalue should be greater than the second and fourth.
Step 5: Compute the corresponding eigenvectors:
Last step: Select k eigenvectors corresponding to the largest eigenvalues to reconstruct a new set of feature vectors in the space. This selection process helps avoid dimensionality problems, keeping the most essential data. It also improves the computational speed and interpretability of various other tasks being performed in the analytical methods of the dataset, which is the main aim of PCA in the case of data preprocessing and data analysis.
Linear discriminant analysis (LDA) is a supervised dimensionality reduction technique that projects data onto a lower-dimensional space while maximizing class separability. The main goal of LDA is to find a transformation matrix W that maximizes the ratio of between-class variance to within-class variance, ensuring that different classes are well-separated in the projected space. Mathematically, the objective function of LDA is to maximize the following criterion.
Here, Sb is the between-class scatter matrix, Sw is the within-class scatter matrix, and W is the transformation matrix to be optimized.
The methodology proposed in this paper consists of five feature extraction mechanisms described in section 2.2. To develop a hybrid feature vector, we combine the five feature vectors as given in Eq. (9). Mathematically, the hybrid feature vector is also called the hybrid feature vector. Table 4 shows the overview of features contributed by each technique and the total number of features created by the hybrid vector.
Method | Number of features (dimensionality) |
Principal component analysis (PCA) | 10 |
Linear discriminant analysis (LDA) | 10 |
Gain ratio (GR) | 10 |
Pearson correlation coefficient (PCC) | 10 |
ReliefF algorithm (RFA) | 10 |
Hybrid features | 50 |
Feature selection is vital in the machine learning pipeline to identify the most relevant features to improve model performance, reduce overfitting, and lower computational costs [17]. This paper employs Shapley additive explanations (SHAP) for feature selection, which leverages cooperative game theory to assign importance to input features based on their contribution to predictions. SHAP quantifies each feature's impact, providing insights into its influence on model outcomes, as detailed in Eq. (10). Understanding the structure and size of the dataset is equally essential, as it informs preprocessing steps like data splitting, regularization, and feature selection. Properly shaping data ensures efficient processing and supports informed decision-making throughout the data science process.
Where φi, represents the SHAP value for the feature i, N is the set of all features, S is a subset of features excluding i, f(S) is the model's prediction given features in S, and
In this paper, we selected the vital features shown in Figure 2. Each row represents a feature, with dots indicating their corresponding SHAP values. Blue dots signify low values, while red dots denote high values. Positive SHAP values indicate a higher likelihood of liver disease, whereas negative values suggest a lower risk. Testing different feature groups revealed that the top 25 features significantly improved the proposed method's performance.
Deep neural networks (DNNs) mimic the human brain's functioning, consisting of an input layer for receiving data, an output layer for producing results, and multiple hidden layers that process information through interconnected tiers [18]. These layers transform input data, enabling the model to identify intricate patterns and representations. The deep structure of DNNs makes them highly effective in tasks such as image recognition and natural language processing, as each layer extracts increasingly complex features. However, while additional hidden layers improve the model's ability to learn intricate patterns, they also raise computational costs, increase the risk of overfitting, and complicate the model. DNNs excel in feature extraction from unstructured or unlabeled data and have proven valuable in biotechnology, natural language processing, image analysis, audio processing, and engineering [19],[20].
This study focuses on the early prediction of liver illness using benchmark data and a deep neural network model with four hidden layers, as illustrated in Figure 3. The DNN architecture comprises multiple neurons that process the input feature vector to produce output, as detailed in Eqs (11) and (12). The neurons' weights in each layer are initialized using the Xavier function to ensure equal variance across layers [14],[15]. A backpropagation method adjusts the weight matrices to minimize the discrepancies between predicted and target classes. The ReLU activation function is applied in the input and hidden layers to capture nonlinearity and patterns in the data, while the logistic sigmoid activation function is used in the output layer. This mechanism determines whether a neuron is activated or remains inactive based on its output. Additionally, the Softmax activation function in the output layer converts the generated values into probabilities between 0 and 1, representing the likelihood of the data belonging to a specific class.
To thoroughly evaluate the performance of the proposed models, five essential evaluation metrics were employed, i.e., sensitivity (SN), specificity (SP), overall accuracy (ACC), Matthew's correlation coefficient (MCC), and the area under the curve (AUC) [21]. These critical indicators provide insights into the predictor's effectiveness from multiple perspectives. The ACC represents the proportion of correct predictions relative to the total number of samples. The SN measures the proportion of actual positives correctly identified, while SP reflects the proportion of actual negatives accurately detected. The AUC assesses the model's classification ability across multiple classes, with the receiver operating characteristic (ROC) curve plotting the true positive rate against the false positive rate. The MCC evaluates the classifier's performance by accounting for all four confusion matrix elements. The corresponding calculation formulas are provided below.
Here, TP and FN represent the true positive and negative samples (i.e., liver and non-liver diseases). Similarly, TN and FP are errors as the model inaccurately predicted the samples to be true or false.
This section provides a detailed evaluation and discussion of the proposed model's effectiveness. The experiments were conducted using machine learning tests implemented with Python and TensorFlow, both open-source tools that facilitated efficient setup and testing of the prediction models. The tests were executed on a system equipped with an 11th Gen Intel Core i7-1165G7 processor (2.80 GHz), 16 GB of RAM, running Windows 11 Home on a 64-bit architecture.
This section aims to identify the optimal configuration for hyperparameters in DNN topology. To analyze the performance of the DNN on different hyperparameters, we used a grid search technique that applies different combinations of parameters. We considered only those hyperparameters that primarily affect the DNN model's performance. These parameters include activation function, learning rate, and the number of iterations [15],[18],[20],[22].
Parameters list | Optimize values |
Hidden layers | 4 |
Updater | ADAGRAD function |
Hidden layers neurons | 23-17-14-6 |
Seed | 12345L |
Epoch | 10 |
Momentum | 0.9 |
LR | 0.01 |
Dropout | 0.25 |
Regularization l2 | 0.001 |
Activation functions | ReLU & Softmax |
Optimizer | SGD Method |
Weight initialization function | XAVIER function |
First, experiments were conducted to evaluate the impact of activation functions and learning rates on the model's performance. As shown in Table 6, the DNN classifier achieved its highest accuracy of 90.12% while using the ReLU activation function and a learning rate of 0.01. Furthermore, a gradual reduction in the learning rate improved the model's performance; however, further decreases beyond this point did not result in substantial accuracy gains. Thus, a learning rate of 0.01 and the ReLU activation function were identified as optimal parameters for achieving high accuracy in the DNN model.
LR | ReLU | Tanh | Sigmoid |
0.01 | 90.12 | 88.10 | 89.44 |
0.02 | 89.91 | 88.09 | 89.23 |
0.03 | 89.45 | 87.71 | 89.01 |
0.04 | 89.16 | 87.58 | 88.80 |
0.05 | 88.49 | 87.38 | 88.17 |
0.06 | 87.82 | 87.19 | 87.54 |
0.07 | 87.55 | 86.99 | 86.91 |
0.08 | 87.09 | 86.80 | 86.28 |
0.09 | 87.05 | 86.60 | 85.65 |
0.10 | 87.03 | 86.41 | 85.02 |
Next, we conducted numerous experiments to evaluate the performance of the DNN model by varying the number of training epochs. Figure 4 illustrates the error loss observed when using the ReLU activation function. The results indicate a consistent decrease in the error rate with increased training epochs. For example, the error loss began at 0.892 during the initial epoch and progressively declined to 0.002 by the 50th epoch. These findings suggest that 50 epochs are optimal, as the error rates stabilize beyond this point. The optimal configuration determined from this analysis is detailed in Table 5.
This section examines the prediction results of the deep neural network (DNN) model using individual and hybrid feature extraction methods. Machine learning techniques such as k-fold cross-validation (CV) have been widely used in the literature to evaluate model performance. A 10-fold cross-validation approach was adopted in this study, offering an optimal balance between computational efficiency and comprehensive performance evaluation while minimizing the influence of data variability. Table 7 presents the DNN model's predicted outcomes on the balanced dataset using various feature vectors.
Methods | ACC (%) | SP (%) | SN (%) | MCC |
PCA | 72.37 | 70.61 | 74.13 | 0.481 |
LDA | 84.88 | 81.22 | 88.54 | 0.703 |
GR | 77.85 | 76.38 | 79.32 | 0.561 |
PCC | 82.38 | 79.12 | 85.64 | 0.662 |
RFA | 81.32 | 78.18 | 84.46 | 0.665 |
Hybrid feature (before SHAP) | 89.36 | 88.21 | 90.44 | 0.797 |
Hybrid feature (after SHAP) | 90.12 | 88.17 | 92.05 | 0.825 |
Table 7 demonstrates that the DNN model attained superior performance with a hybrid feature vector compared to the individual feature extraction technique. The DNN model achieved an average accuracy of 89.36%, sensitivity of 90.44%, specificity of 88.21%, and an MCC of 0.797 utilizing a hybrid feature vector. In order to further enhance the performance of the proposed DNN model, we apply the feature selection method, i.e., SHAP. SHAP-selected features attained an accuracy of 90.12%, sensitivity of 92.05%, specificity of 88.17%, and a Matthew's correlation coefficient of 0.825. The result demonstrates the effectiveness of feature selection in enhancing prediction accuracy and target result identification in deep neural networks (DNNs).
In this section, we compare the performance of various classification methods based on accuracy (ACC), sensitivity (SN), specificity (SP), and Matthew's correlation coefficient (MCC). The classifiers being assessed are random forest (RF) [23], support vector machine (SVM) [24], and k-nearest neighbor (KNN) [25]. RF is a popular ensemble learning method for classification and prediction, building multiple decision trees from random samples. SVM is widely used in life sciences for linear and nonlinear classification, as it finds the best boundary to separate classes [26]. KNN, commonly used in image processing, is a distance-based method that classifies by comparing examples. Table 8 shows the overall performance comparison of the different classifiers on the benchmark dataset.
Method | ACC (%) | SN (%) | SP (%) | MCC |
Proposed DNN | 90.12 | 92.05 | 88.17 | 0.825 |
SVM | 89.66 | 87.75 | 91.67 | 0.794 |
RF | 87.86 | 84.98 | 90.91 | 0.759 |
KNN | 85.96 | 84.98 | 86.96 | 0.719 |
The proposed DNN model achieved the highest accuracy of 90.12%, with a sensitivity of 92.05% and specificity of 88.17%, resulting in an MCC of 0.825. Comparatively, among the traditional machine learning models, the SVM demonstrated an accuracy of 89.66%, a sensitivity of 87.75%, a specificity of 91.67%, and an MCC of 0.794. Similarly, the RF and KNN models achieved lower accuracy, at 87.86% and 85.96%, respectively. Overall, the proposed DNN demonstrates the most balanced and robust performance, improving average accuracy by 2.29%. The superior performance of the DNN model compared to traditional machine learning algorithms can be attributed to its use of multi-stack processing layers. These layers enable DNN to effectively handle complex datasets with high nonlinearity, a task that traditional ML algorithms struggle with due to their reliance on single-stack processing layers, which are insufficient for such intricate data. These findings confirm that the DNN model outperforms conventional learning methods in predicting liver and non-liver diseases.
Furthermore, we also compared the performance of the DNN model in terms of AUC (area under the ROC curve) [27] with traditional learning algorithms, as shown in Figure 5. The figure shows that the DNN model achieved the highest AUC value compared to the other algorithms. Specifically, the DNN model had an AUC of 0.921, while the SVM, RF, and KNN algorithms had AUC values of 0.901, 0.889, and 0.872, respectively. The main reason for the DNN model's superior performance is its multi-layer structure (hidden layers) with backpropagation, which effectively optimizes weights. This allows the DNN to handle complex and highly nonlinear datasets better than other models, which use a single-layer processing approach and struggle with such data. Additionally, the confusion matrix analysis in Figure 6 shows that our proposed DNN model achieves balanced recognition of both positive and negative. This strongly confirms the model's accuracy and reliability in distinguishing between positive and negative samples, making it particularly suitable for predictive tasks in the specific problem domain
In this section, we compare the performance of the proposed model with the existing state-of-the-art models, as mentioned in [10],[28]–[30]. Table 9 shows the comparative findings. Moreover, we provide the proposed models from published research works based on the same dataset. Table 9 shows that the proposed model provided the best prediction accuracy among four previously published predictors. For example, K. Gupta et al. [29] achieved an accuracy level of 63%, whereas the recently published predictor, R. Amin et al. [10], achieved an accuracy of 88.10%. Similarly, D. Elias et al. [30] achieved 80.10%. These results confirm that the proposed model performed better than the existing predictors, with an average accuracy improvement of 13.93%.
Method | Classifiers | ACC (%) |
K. Gupta et al. [29] | Light GB | 63.00 |
Altaf et al. [28] | Voting | 73.56 |
D. Elias et al. [30] | Voting | 80.10 |
R. Amin et al. [10] | Random Forest | 88.10 |
Proposed DNN | DNN | 90.12 |
Liver disease (LD) is a severe condition that poses significant risks to human health and requires timely medical intervention. Healthcare professionals rely on neurological techniques for evaluating and diagnosing affected individuals. In this paper, we explored the prediction of chronic LD using a deep neural network algorithm enhanced with integrated and optimized features. Our model used several techniques to assess feature importance, including PCA, LDA, gain ratio, Pearson correlation coefficient, and ReliefF algorithm. We also employed SHAP analysis to interpret complex features and select the most relevant ones for accurate LD prediction. Our novel approach demonstrated superior performance compared to existing models, which can be attributed to the implementation of efficient feature extraction methods, advanced compression techniques, and an optimized feature selection process. The results show that our model enhances discriminative ability, offering a more reliable tool for the early detection of chronic liver disease. Integrating AI and machine learning into clinical settings holds great potential for advancing disease detection and improving patient outcomes.
In the future, we plan to create an accessible web platform for biologists to use this model. Additionally, we aim to expand the dataset, explore new features, and implement more advanced algorithms to refine further and validate our model's predictive capabilities.
[1] | A. F. Molland, Chapter 10 - Underwater vehicles, In: The Maritime Engineering Reference Book – A Guide to Ship Design, Construction and Operation, 2008, 728–783. |
[2] |
B. Allotta, R. Costanzi, L. Pugi, A. Ridolfi, Identification of the main hydrodynamic parameters of Typhoon AUV from a reduced experimental dataset, Ocean Eng., 147 (2018), 77–88. https://doi.org/10.1016/j.oceaneng.2017.10.032 doi: 10.1016/j.oceaneng.2017.10.032
![]() |
[3] |
L. D. L. Barker, M. V. Jakuba, A. D. Bowen, C. R. German, T. Maksym, L. Mayer, et al., Scientific challenges and present capabilities in underwater robotic vehicle design and navigation for oceanographic exploration under-ice, Remote Sens., 12 (2020), 2588. https://doi.org/10.3390/rs12162588 doi: 10.3390/rs12162588
![]() |
[4] | Y. Bestaoui, A Lagrangian approach to modeling of an airship with wind and varying mass effects, In: 48th AIAA Aerospace Sciences Meeting Including the New Horizons Forum and Aerospace Exposition, Orlando, Florida, USA, 2010. https://doi.org/10.2514/6.2010-40 |
[5] |
L. Bevilacqua, W. Kleczka, E. Kreuzer, On the mathematical modeling of ROVs, IFAC Proc. Volumes, 24 (1991), 51–54. https://doi.org/10.1016/S1474-6670(17)51031-9 doi: 10.1016/S1474-6670(17)51031-9
![]() |
[6] | E. Borhaug, A. Pavlov, K. Y. Pettersen, Integral LOS control for path following of underactuated marine surface vessels in the presence of constant ocean currents, In: 2008 47th IEEE Conference on Decision and Control, 2008, 4984–4991. https://doi.org/10.1109/CDC.2008.4739352 |
[7] |
E. Celledoni, E. Çokaj, A. Leone, D. Murari, B. Owren, Lie group integrators for mechanical systems, Int. J. Comput. Math., 99 (2022), 58–88. https://doi.org/10.1080/00207160.2021.1966772 doi: 10.1080/00207160.2021.1966772
![]() |
[8] | C. W. Chen, N. M. Yan, Prediction of added mass for an autonomous underwater vehicle moving near sea bottom using panel method, In: 2017 4th International Conference on Information Science and Control Engineering (ICISCE), 2017, 1094–1098. https://doi.org/10.1109/ICISCE.2017.228 |
[9] |
M. Chyba, T. Haberkorn, R. N. Smith, G.Wilkens, A geometric analysis of trajectory design for underwater vehicles, Discrete Cont. Dynam. Sys. B, 11 (2009), 233–262. https://doi.org/10.3934/dcdsb.2009.11.233 doi: 10.3934/dcdsb.2009.11.233
![]() |
[10] |
S. Fiori, Model formulation over Lie groups and numerical methods to simulate the motion of gyrostats and quadrotors, Mathematics, 7 (2019), 935. https://doi.org/10.3390/math7100935 doi: 10.3390/math7100935
![]() |
[11] |
S. Fiori, Manifold calculus in system theory and control - Fundamentals and first-order systems, Symmetry, 13 (2021), 2092. https://doi.org/10.3390/sym13112092 doi: 10.3390/sym13112092
![]() |
[12] |
T. I. Fossen, O. E. Fjellstad, Nonlinear modelling of marine vehicles in 6 degrees of freedom, Math. Model. Syst., 1 (1995), 17–27. https://doi.org/10.1080/13873959508837004 doi: 10.1080/13873959508837004
![]() |
[13] | T. I. Fossen, K. Y. Pettersen, Modeling of Underwater Vehicles, Berlin: Springer Berlin Heidelberg, 2018. https://doi.org/10.1007/978-3-642-41610-1_12-1 |
[14] |
T. I. Fossen, A nonlinear unified state-space model for ship maneuvering and control in a seaway, Int. J. Bifurc. Chaos, 15 (2005), 2717–2746. https://doi.org/10.1142/S0218127405013691 doi: 10.1142/S0218127405013691
![]() |
[15] |
J. González-García, A. Gómez-Espinosa, E. Cuan-Urquizo, L. G. García-Valdovinos, T. Salgado-Jiménez, J. A. E. Cabello, Autonomous underwater vehicles: Localization, navigation, and communication for collaborative missions, Appl. Sci., 10 (2020), 1256. https://doi.org/10.3390/app10041256 doi: 10.3390/app10041256
![]() |
[16] | E. Y. Hong, H. G. Soon, M. Chitre, Depth control of an autonomous underwater vehicle, starfish, In: Oceans'10 IEEE Sydney, 2010. https://doi.org/10.1109/OCEANSSYD.2010.5603566 |
[17] |
P. Jagtap, P. Raut, P. Kumar, A. Gupta, N. Singh, F. Kazi, Control of autonomous underwater vehicle using reduced order model predictive control in three dimensional space, IFAC Papers OnLine, 49 (2016), 772–777. https://doi.org/10.1016/j.ifacol.2016.03.150 doi: 10.1016/j.ifacol.2016.03.150
![]() |
[18] |
A. Krishnan, J. Kadiyam, S. Mohan, Robust motion control of fully/over-actuated underwater vehicle using sliding surfaces, J. Intell. Robot. Syst., 108 (2023), 60. https://doi.org/10.1007/s10846-023-01918-y doi: 10.1007/s10846-023-01918-y
![]() |
[19] |
S. K. Lee, T. H. Joung, S. J. Cheo, T. S. Jang, J. H. Lee, Evaluation of the added mass for a spheroid-type unmanned underwater vehicle by vertical planar motion mechanism test, Int. J. Naval Archit. Ocean Eng., 3 (2011), 174–180. https://doi.org/10.2478/IJNAOE-2013-0060 doi: 10.2478/IJNAOE-2013-0060
![]() |
[20] |
N. E. Leonard, Stability of a bottom-heavy underwater vehicle, Automatica, 33 (1997), 331–346. https://doi.org/10.1016/S0005-1098(96)00176-8 doi: 10.1016/S0005-1098(96)00176-8
![]() |
[21] |
C. C. Liang, T. L. Teng, W. H. Lai, A study of diving depth on deep-diving submersible vehicles, Int. J. Pres. Ves. Pip., 75 (1998), 447–457. https://doi.org/10.1016/S0308-0161(98)00041-6 doi: 10.1016/S0308-0161(98)00041-6
![]() |
[22] | X. Liang, Y. Pang, L. Wan, B. Wang, Dynamic Modelling and Motion Control for Underwater Vehicles with Fins, Rijeka: Intech Open Access Publisher, 2009. https://doi.org/10.5772/6720 |
[23] | N. Nordkvist, A. K. Sanyal, A Lie group variational integrator for rigid body motion in SE(3) with applications to underwater vehicle dynamics, In: 49th IEEE Conference on Decision and Control (CDC), 2010, 5414–5419. https://doi.org/10.1109/CDC.2010.5717622 |
[24] |
J. P. Panda, A. Mitra, H. V. Warrior, A review on the hydrodynamic characteristics of autonomous underwater vehicles, Proc. Institut. Mech. Eng. M J. Eng. Marit. Environ., 235 (2021), 15–29. https://doi.org/10.1177/1475090220936896 doi: 10.1177/1475090220936896
![]() |
[25] |
M. Saghafi, R. Lavimi, Optimal design of nose and tail of an autonomous underwater vehicle hull to reduce drag force using numerical simulation, Proc. Institut. Mech. Eng. M J. Eng. Marit. Environ., 234 (2020), 76–88. https://doi.org/10.1177/1475090219863191 doi: 10.1177/1475090219863191
![]() |
[26] |
C. Shen, Y. Shi, B. Buckham, Trajectory tracking control of an autonomous underwater vehicle using Lyapunov-based model predictive control, IEEE T. Industrial Elect., 65 (2018), 5796–5805. https://doi.org/10.1109/TIE.2017.2779442 doi: 10.1109/TIE.2017.2779442
![]() |
[27] | Ø. N. Smogeli, T. Pérez, T. I. Fossen, A. J. Sørensen, The marine systems simulator statespace model representation for dynamically positioned surface vessels, In: International Maritime Association of the Mediterranean IMAM Conference, Lisbon, 2005. |
[28] | SNAME, Nomenclature for treating the motion of a submerged body through a fluid, Soc. Naval Archit. Marine Eng. Tech. Res. Bull., 1950 (1950), 1–5. |
[29] | Y. Sun, X. Ran, J. Cao, Y. Li, Deep submergence rescue vehicle docking based on parameter adaptive control with acoustic and visual guidance, Int. J. Adv. Robot. Syst., 17 (2020). https://doi.org/10.1177/1729881420919955 |
[30] |
N. Syahroni, Y. B. Seo, J. W. Choi, Depth control of autonomous underwater vehicle based on open control platform, IFAC Proc. Volumes, 41 (2008), 3707–3712. https://doi.org/10.3182/20080706-5-KR-1001.00626 doi: 10.3182/20080706-5-KR-1001.00626
![]() |
[31] |
A. S. Tijjani, A. Chemori, V. Creuze, Robust adaptive tracking control of underwater vehicles: Design, stability analysis, and experiments, IEEE/ASME Transact. Mechat., 26 (2021), 897–907. https://doi.org/10.1109/TMECH.2020.3012502 doi: 10.1109/TMECH.2020.3012502
![]() |
[32] |
F. Udwadia, R. Kalaba, On the foundations of analytical dynamics, Int. J. Non-Linear Mech., 37 (2002), 1079–1090. https://doi.org/10.1016/S0020-7462(01)00033-6 doi: 10.1016/S0020-7462(01)00033-6
![]() |
[33] |
C. Woolsey, N. Leonard, Stabilizing underwater vehicle motion using internal rotors, Automatica, 38 (2002), 2053–2062. https://doi.org/10.1016/S0005-1098(02)00136-X doi: 10.1016/S0005-1098(02)00136-X
![]() |
[34] |
Y. Zhang, J. Che, Y. Hu, J. Cui, J. Cui, Real-time ocean current compensation for AUV trajectory tracking control using a meta-learning and self-adaptation hybrid approach, Sensors, 23 (2023), 6417. https://doi.org/10.3390/s23146417 doi: 10.3390/s23146417
![]() |
[35] |
J. Zhou, Y. Si, Y. Chen, A review of subsea AUV technology, J. Mar. Sci. Eng., 11 (2023), 1119. https://doi.org/10.3390/jmse11061119 doi: 10.3390/jmse11061119
![]() |
[36] | K. Zhu, L. Gu, A MIMO nonlinear robust controller for work-class ROVs positioning and trajectory tracking control, In: 2011 Chinese Control and Decision Conference (CCDC), 2011, 2565–2570. https://doi.org/10.1109/CCDC.2011.5968643 |
1. | Max C. W. Engelen, River Betting, Christos Strydis, SimHH: A Versatile, Multi-GPU Simulator for Extended Hodgkin-Huxley Networks, 2025, 13, 2169-3536, 46865, 10.1109/ACCESS.2025.3550444 |
Feature | Description |
Gender | Participant's gender |
Albumin (ALB) | Albumin level |
Direct bilirubin (DB) | Direct bilirubin level |
Age (years) | Age of participants (4–90 years) |
Total bilirubin (TB) | Total bilirubin level |
Aspartate aminotransferase (SGOT) | Aspartate aminotransferase level |
Total protein (TP) | Total protein level |
Alkaline phosphatase (ALP) | Alkaline phosphatase level |
Alanine aminotransferase (SGPT) | Alanine aminotransferase level |
Albumin and globulin ratio (AGR) | Albumin and globulin ratio |
Features | Min | Mean | Max | ± Stdv |
TB | 0.4 | 2.65 | 75 | ± 5.32 |
SGOT | 10 | 88.78 | 4929 | ± 245.07 |
Age | 4 | 43.55 | 90 | ± 16.28 |
ALP | 63 | 267.26 | 2110 | ± 212.62 |
SGPT | 10 | 66.78 | 2000 | ± 155.16 |
AGR | 0.3 | 0.98 | 2.8 | ± 0.30 |
DB | 0.1 | 1.16 | 19.7 | ± 2.42 |
ALB | 0.9 | 3.19 | 5.5 | ± 0.76 |
TP | 2.7 | 6.5 | 9.6 | ± 1.02 |
Feature | PCC | GR | RFA |
SGPT | 0.2141 | 0.0701 | 0.2613 |
TB | 0.2874 | 0.1373 | 0.2848 |
Gender | 0.0857 | 0.0065 | 0.025 |
SGOT | 0.2017 | 0.1005 | 0.2393 |
AGR | 0.2046 | 0.0822 | 0.2599 |
DB | 0.3205 | 0.1421 | 0.2895 |
ALB | 0.1836 | 0.0408 | 0.2883 |
Age | 0.1596 | 0.0372 | 0.2625 |
ALP | 0.246 | 0.0867 | 0.1936 |
TP | 0.0443 | 0.00 | 0.2161 |
Method | Number of features (dimensionality) |
Principal component analysis (PCA) | 10 |
Linear discriminant analysis (LDA) | 10 |
Gain ratio (GR) | 10 |
Pearson correlation coefficient (PCC) | 10 |
ReliefF algorithm (RFA) | 10 |
Hybrid features | 50 |
Parameters list | Optimize values |
Hidden layers | 4 |
Updater | ADAGRAD function |
Hidden layers neurons | 23-17-14-6 |
Seed | 12345L |
Epoch | 10 |
Momentum | 0.9 |
LR | 0.01 |
Dropout | 0.25 |
Regularization l2 | 0.001 |
Activation functions | ReLU & Softmax |
Optimizer | SGD Method |
Weight initialization function | XAVIER function |
LR | ReLU | Tanh | Sigmoid |
0.01 | 90.12 | 88.10 | 89.44 |
0.02 | 89.91 | 88.09 | 89.23 |
0.03 | 89.45 | 87.71 | 89.01 |
0.04 | 89.16 | 87.58 | 88.80 |
0.05 | 88.49 | 87.38 | 88.17 |
0.06 | 87.82 | 87.19 | 87.54 |
0.07 | 87.55 | 86.99 | 86.91 |
0.08 | 87.09 | 86.80 | 86.28 |
0.09 | 87.05 | 86.60 | 85.65 |
0.10 | 87.03 | 86.41 | 85.02 |
Methods | ACC (%) | SP (%) | SN (%) | MCC |
PCA | 72.37 | 70.61 | 74.13 | 0.481 |
LDA | 84.88 | 81.22 | 88.54 | 0.703 |
GR | 77.85 | 76.38 | 79.32 | 0.561 |
PCC | 82.38 | 79.12 | 85.64 | 0.662 |
RFA | 81.32 | 78.18 | 84.46 | 0.665 |
Hybrid feature (before SHAP) | 89.36 | 88.21 | 90.44 | 0.797 |
Hybrid feature (after SHAP) | 90.12 | 88.17 | 92.05 | 0.825 |
Method | ACC (%) | SN (%) | SP (%) | MCC |
Proposed DNN | 90.12 | 92.05 | 88.17 | 0.825 |
SVM | 89.66 | 87.75 | 91.67 | 0.794 |
RF | 87.86 | 84.98 | 90.91 | 0.759 |
KNN | 85.96 | 84.98 | 86.96 | 0.719 |
Feature | Description |
Gender | Participant's gender |
Albumin (ALB) | Albumin level |
Direct bilirubin (DB) | Direct bilirubin level |
Age (years) | Age of participants (4–90 years) |
Total bilirubin (TB) | Total bilirubin level |
Aspartate aminotransferase (SGOT) | Aspartate aminotransferase level |
Total protein (TP) | Total protein level |
Alkaline phosphatase (ALP) | Alkaline phosphatase level |
Alanine aminotransferase (SGPT) | Alanine aminotransferase level |
Albumin and globulin ratio (AGR) | Albumin and globulin ratio |
Features | Min | Mean | Max | ± Stdv |
TB | 0.4 | 2.65 | 75 | ± 5.32 |
SGOT | 10 | 88.78 | 4929 | ± 245.07 |
Age | 4 | 43.55 | 90 | ± 16.28 |
ALP | 63 | 267.26 | 2110 | ± 212.62 |
SGPT | 10 | 66.78 | 2000 | ± 155.16 |
AGR | 0.3 | 0.98 | 2.8 | ± 0.30 |
DB | 0.1 | 1.16 | 19.7 | ± 2.42 |
ALB | 0.9 | 3.19 | 5.5 | ± 0.76 |
TP | 2.7 | 6.5 | 9.6 | ± 1.02 |
Feature | PCC | GR | RFA |
SGPT | 0.2141 | 0.0701 | 0.2613 |
TB | 0.2874 | 0.1373 | 0.2848 |
Gender | 0.0857 | 0.0065 | 0.025 |
SGOT | 0.2017 | 0.1005 | 0.2393 |
AGR | 0.2046 | 0.0822 | 0.2599 |
DB | 0.3205 | 0.1421 | 0.2895 |
ALB | 0.1836 | 0.0408 | 0.2883 |
Age | 0.1596 | 0.0372 | 0.2625 |
ALP | 0.246 | 0.0867 | 0.1936 |
TP | 0.0443 | 0.00 | 0.2161 |
Method | Number of features (dimensionality) |
Principal component analysis (PCA) | 10 |
Linear discriminant analysis (LDA) | 10 |
Gain ratio (GR) | 10 |
Pearson correlation coefficient (PCC) | 10 |
ReliefF algorithm (RFA) | 10 |
Hybrid features | 50 |
Parameters list | Optimize values |
Hidden layers | 4 |
Updater | ADAGRAD function |
Hidden layers neurons | 23-17-14-6 |
Seed | 12345L |
Epoch | 10 |
Momentum | 0.9 |
LR | 0.01 |
Dropout | 0.25 |
Regularization l2 | 0.001 |
Activation functions | ReLU & Softmax |
Optimizer | SGD Method |
Weight initialization function | XAVIER function |
LR | ReLU | Tanh | Sigmoid |
0.01 | 90.12 | 88.10 | 89.44 |
0.02 | 89.91 | 88.09 | 89.23 |
0.03 | 89.45 | 87.71 | 89.01 |
0.04 | 89.16 | 87.58 | 88.80 |
0.05 | 88.49 | 87.38 | 88.17 |
0.06 | 87.82 | 87.19 | 87.54 |
0.07 | 87.55 | 86.99 | 86.91 |
0.08 | 87.09 | 86.80 | 86.28 |
0.09 | 87.05 | 86.60 | 85.65 |
0.10 | 87.03 | 86.41 | 85.02 |
Methods | ACC (%) | SP (%) | SN (%) | MCC |
PCA | 72.37 | 70.61 | 74.13 | 0.481 |
LDA | 84.88 | 81.22 | 88.54 | 0.703 |
GR | 77.85 | 76.38 | 79.32 | 0.561 |
PCC | 82.38 | 79.12 | 85.64 | 0.662 |
RFA | 81.32 | 78.18 | 84.46 | 0.665 |
Hybrid feature (before SHAP) | 89.36 | 88.21 | 90.44 | 0.797 |
Hybrid feature (after SHAP) | 90.12 | 88.17 | 92.05 | 0.825 |
Method | ACC (%) | SN (%) | SP (%) | MCC |
Proposed DNN | 90.12 | 92.05 | 88.17 | 0.825 |
SVM | 89.66 | 87.75 | 91.67 | 0.794 |
RF | 87.86 | 84.98 | 90.91 | 0.759 |
KNN | 85.96 | 84.98 | 86.96 | 0.719 |
Method | Classifiers | ACC (%) |
K. Gupta et al. [29] | Light GB | 63.00 |
Altaf et al. [28] | Voting | 73.56 |
D. Elias et al. [30] | Voting | 80.10 |
R. Amin et al. [10] | Random Forest | 88.10 |
Proposed DNN | DNN | 90.12 |