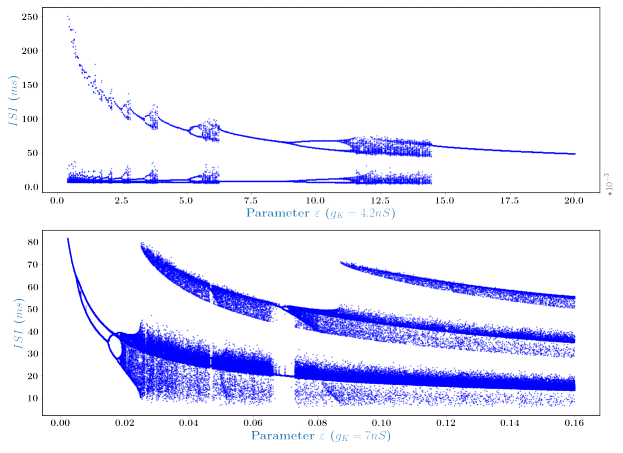
In this paper, we explore the mechanisms of central pattern generators (CPGs), circuits that can generate rhythmic patterns of motor activity without external input. We study the half-center oscillator, a simple form of CPG circuit consisting of neurons connected by reciprocally inhibitory synapses. We examine the role of asymmetric coupling factors in shaping rhythm activity and how different network topologies contribute to network efficiency. We have discovered that neurons with lower synaptic strength are more susceptible to noise that affects rhythm changes. Our research highlights the importance of asymmetric coupling factors, noise, and other synaptic parameters in shaping the broad regimes of CPG rhythm. Finally, we compare three topology types' regular regimes and provide insights on how to locate the rhythm activity.
Citation: Feibiao Zhan, Jian Song, Shenquan Liu. The influence of synaptic strength and noise on the robustness of central pattern generator[J]. Electronic Research Archive, 2024, 32(1): 686-706. doi: 10.3934/era.2024033
[1] | Feibiao Zhan, Jian Song . Complex rhythm and synchronization of half-center oscillators under electromagnetic induction. Electronic Research Archive, 2024, 32(7): 4454-4471. doi: 10.3934/era.2024201 |
[2] | Yuzhi Zhao, Honghui Zhang, Zilu Cao . The important role of astrocytes in activity pattern transition of the subthalamopallidal network related to Parkinson's disease. Electronic Research Archive, 2024, 32(6): 4108-4128. doi: 10.3934/era.2024185 |
[3] | Yunhai Wang, Guodong Huang, Rui Zhu, Shu Zhou, Yuan Chai . Response mechanism of heat-sensitive neurons under combined noise stimulation. Electronic Research Archive, 2024, 32(11): 6405-6423. doi: 10.3934/era.2024298 |
[4] | Hebing Zhang, Xiaojing Zheng . Multi-Local-Worlds economic and management complex adaptive system with agent behavior and local configuration. Electronic Research Archive, 2024, 32(4): 2824-2847. doi: 10.3934/era.2024128 |
[5] | Ariel Leslie, Jianzhong Su . Modeling and simulation of a network of neurons regarding Glucose Transporter Deficiency induced epileptic seizures. Electronic Research Archive, 2022, 30(5): 1813-1835. doi: 10.3934/era.2022092 |
[6] | Rizhao Cai, Liepiao Zhang, Changsheng Chen, Yongjian Hu, Alex Kot . Learning deep forest for face anti-spoofing: An alternative to the neural network against adversarial attacks. Electronic Research Archive, 2024, 32(10): 5592-5614. doi: 10.3934/era.2024259 |
[7] | Zhongsheng Li, Jianchao Huang, Wencheng Wang, Yucai Huang . A new stereo matching algorithm based on improved four-moded census transform and adaptive cross pyramid model. Electronic Research Archive, 2024, 32(7): 4340-4364. doi: 10.3934/era.2024195 |
[8] | Mingtao Cui, Wennan Cui, Wang Li, Xiaobo Wang . A polygonal topology optimization method based on the alternating active-phase algorithm. Electronic Research Archive, 2024, 32(2): 1191-1226. doi: 10.3934/era.2024057 |
[9] | Duhui Chang, Yan Geng . Distributed data-driven iterative learning control for multi-agent systems with unknown input-output coupled parameters. Electronic Research Archive, 2025, 33(2): 867-889. doi: 10.3934/era.2025039 |
[10] | Jieqiong Yang, Panzhu Luo . Study on the spatial correlation network structure of agricultural carbon emission efficiency in China. Electronic Research Archive, 2023, 31(12): 7256-7283. doi: 10.3934/era.2023368 |
In this paper, we explore the mechanisms of central pattern generators (CPGs), circuits that can generate rhythmic patterns of motor activity without external input. We study the half-center oscillator, a simple form of CPG circuit consisting of neurons connected by reciprocally inhibitory synapses. We examine the role of asymmetric coupling factors in shaping rhythm activity and how different network topologies contribute to network efficiency. We have discovered that neurons with lower synaptic strength are more susceptible to noise that affects rhythm changes. Our research highlights the importance of asymmetric coupling factors, noise, and other synaptic parameters in shaping the broad regimes of CPG rhythm. Finally, we compare three topology types' regular regimes and provide insights on how to locate the rhythm activity.
Central pattern generator (CPG) is a microcircuit of neuron network that can generate multi-rhythm pattern sequences spontaneously without any external information input [1,2]. It controls motor behaviors such as swimming [3,4], walking, breathing, heartbeat, and more [2,5,6,7]. Recently, Marder et al. made a well-summarized study about CPG and put forward new insights on small rhythmic circuits [8]. CPG is characterized by the robustness and flexibility of the neuronal network in rhythmic activity.
There have been numerous studies examining the working mechanisms of CPGs from both theoretical and experimental perspectives [9,10,11,12,13,14,15,16]. However, achieving flexibility and robustness in CPGs remains not fully understood. Coordination of motor rhythms can be better achieved through the connection between CPGs, which may form an attractor. Each attractor determines a rhythmic behavior [17,18]. Some researchers have proposed a rhythmic pattern for human arm movement, indicating that humans also possess a higher level of CPG in their central nervous system. Research on the reasons for these characteristics of CPGs is still ongoing, and it remains unclear how CPGs achieve the robustness and flexibility required for the collaborative implementation of essential rhythm patterns [19]. Thus, discussion on the rhythmic activity pattern of CPGs is still active [20,21]. Some models are based on Hodgkin-Huxley (HH) neurons, while others are non-periodic neural oscillation models. Theoretical researchers have revealed the hidden internal mechanisms of CPGs from the perspective of dynamics [13,22]. Collens et al. have revealed a mutually inhibitory tri-neuron neural network that explains the emergence, disappearance, and stability of neuronal rhythm with the change of synaptic parameters [22]. Lu et al. [23,24] have studied the synchronization and resonance of small-world networks based on CPGs and proposed integer and fractional models based on the working mechanisms of CPGs. Recent research by Zang and Marder in PNAS (2023) demonstrates that how the spatial morphology of neurons can significantly impact the firing patterns of the CPG circuit [25]. The firing mechanism of neuronal rhythm is known to vary according to several studies [26,27,28]. However, it is still unclear whether this diversity in neuronal rhythm implies a diversity of CPG circuit function. A crucial question is whether the same CPG circuit can produce multiple motor behaviors, making it multifunctional [29,30]. The generation of motor behavior depends on the modulation of a variety of motor rhythms, making it necessary and important to study rhythm diversity in order to understand the function of CPG.
Studying small circuits is crucial to understanding CPG. Typically, these circuits are half-center oscillators (HCO), the smallest building unit that consists of groups of neurons that inhibit each other [31,32]. HCO circuits are considered the easiest to identify. The firing patterns of HCOs may also be influenced by neuronal phase response curves, as indicated in the work by Zang et al. [33]. The synchronization of two neurons has been explored by researchers [34,35]. They have analyzed how neuronal parameters affect the network activity of HCO, which in turn affects motor control [36]. Researchers have also analyzed the effect of changes in system parameter space on the robustness of the HCO bursting model [37,38]. Noise, the robustness of HCO, and sensory feedback are closely related [39,40]. However, the effect of synaptic conductance on HCO rhythm patterns still needs to be analyzed. Inhibition of connection can improve the stability of CPG, and we will explore how changes in the rhythm pattern of HCO and tri-neuron CPG occur with the system parameter regime. We will also examine the effects of synaptic conductance and noise on CPG rhythm patterns.
In this study, we investigate the origin of the flexibility and robustness of CPGs as well as the impact of synaptic conductance on the network rhythm. We examine three different topological modes where each neuron has at least one connection to the other member of the CPG. Our findings demonstrate that diverse rhythm patterns exist in Patterns B and C, as shown in Figure 3. These results are based on the functional characteristics of CPG network structures, which are classified as "open" and "non-open" and were studied by Huerta et al. These findings are also consistent with certain biological principles [41]. Rhythmic motor patterns are associated with diseases such as spinal cord injury and can be easily studied quantitatively. Therefore, the study of CPG rhythm can significantly contribute to the development of disease diagnosis and the advancement of intelligent science. For example, Mader et al. (2001) elaborated that CPG can contribute to the development of new therapeutic methods in the recovery of spinal cord injury and summarized the mechanism process of CPG [1]. The biological CPG principle and the establishment of spiking neural network [42,43,44,45] inspire the development of intelligent science.
The paper is structured as follows. In Section 2, we describe the materials and methods we used in building CPG, including the HH neuron model. Then, in Section 3.1, we illustrate how the rhythm pattern of HCO is regulated by synaptic strength and noise. In Section 3.2, we investigate CPG rhythms in other topologies. Finally, we present the discussion and conclusion in Section 4.
In our CPG, each interneuron is modeled by a Hodgkin–Huxley (HH) model. This microcircuit captures the dynamics by a single compartment, which is described by the following differential equations [28,46,47]:
CmdVdt=−INa−IK−ILeak+ID,dndt=n∞−nτn,dQdt=ω+√2Dξ(t). | (2.1) |
Here, Cm denotes the neural membrane capacitance density (μF/cm2); V represents the membrane potential (mV); the gating variable n of activated K+ channels, denotes the activation probability of potassium ion channels; τn is the time constant (ms); ξ(t) is Gaussian white noise; ω and D are angular frequency and noise intensity for forcing currents; Q(t) is phase noise; and INa, IK, and ILeak are sodium ion current, potassium ion current, and leakage current. Their expressions are as follows:
INa=gNam∞(V)(1−n)(V−ENa),IK=gKn(V−EK),ILeak=gL(V−EL),ID=Asin(Q(t)), |
where the parameter gNa and gK are the maximal conductances (mS/cm2); ENa, EK, and EL are the reversal potentials (mV); m∞ and n∞ are the steady-state of the ionic gating channels; and A is amplitude for forcing currents. They are modeled using:
m∞(V)=11+exp(−V+355),n∞(V)=11+exp(−V+365). |
Specifically, we add a voltage-dependent linear control current (I) to this model, which is expressed as follows.
dIdt=ε(−80−V). | (2.2) |
Now, ε is the feedback coefficient, and ε=0.001 is used if not otherwise specified.
By adjusting parameters, the HH model can reproduce the firing patterns observed in neurons, which underlie their rhythmic properties in the CPG. Figure 1 displays the sequence diagram of interspike intervals (ISIs) of a single neuron without noise. When gK=4.2nS, the system undergoes period-doubling bifurcation with the change of parameter ε, leading to chaos. At this point, the system has two period-doubling cascade paths, and the range of parameters that produce chaos broadens as ε increases. One period-doubling cascade path disappears around ε=0.0145, while the other path has an interspike interval remaining. As we will see below, the system displays the spiking mode when ε>0.0145. When gK=7nS, the system shows a bifurcation diagram that resembles the period-adding cascade as parameters increase. There is still a bifurcation path from period-doubling to chaos. The ISI diagram clearly shows three layers, which may contain deep neural information not captured by the firing patterns of neurons. In the following section, we will present the firing sequence diagram for the corresponding parameters of Figure 1.
In Figure 2, the left panel displays the time series for gK=4.2nS (above Figure 1). When ε=0.001, the neuron model exhibits bursting, which corresponds to several ISIs shown in Figure 1. For ε=0.005 and 0.0075, there are three and two ISIs respectively, represented by three spikings and two spikings per bursting. When ε=0.01, this extends to four ISIs, and although the firing pattern appears similar to that of ε=0.0075, it has changed. At ε=0.0125, the system discharges chaotically, while at ε=0.015, the firing is a single spiking. The right panel of Figure 2 displays the time series for gK=7nS (below Figure 1). At ε=0.01, the model discharge shows two ISIs. As the parameter varies from 0.02 to 0.014, the discharge pattern of the model exhibits different chaotic discharges. In this manner, by calculating the ISIs, we can observe the hidden information of the firing sequence.
The inhibitory relationship between neurons is considered to be a key point in the generation of rhythms. Our CPG models typically consist of two or three neurons. The most common structure describing firing rhythmic activities consists of two coupled neurons that inhibit each other (Figure 3A). This structure is widely known as half-center oscillator and is symmetrically coupled through both inhibitory connections with g12 and g21. We will start off with the simplest network where two cells next section. On this basis, we extended the number of neurons to three (Figure 3B, C). In three circuits, neurons and inhibitory synapses (small black solid dots) form a hybrid microcircuit. And neurons are labeled with different colors (blue, red and green) which correspond to the colors in the firing activities. The synaptic transmission contained in the CPG structure in Figure 3 are ionotropic synapses. These synaptic are inhibitory synapses and are modeled using a first-order kinetic equations, which are conductance-based type similar to previous studies [48,49,50,51]:
{Ipre→post=gpre→postH∞(Vpre)(Vpre−Epre→post),H∞(Vpre)=11+exp(−Vpre−θσ). | (2.3) |
Here, Vpre is the presynaptic voltage, σ=1mV is the steepness, θ=−60mV sets the value when the function is semi-activated, Epre→post is the reversal potential, and gpre→post is the maximal conductance (gij, i,j=1,2,3 & i≠j in Figure 3).
For numerical integration of network system, the fourth-order Runge–Kutta algorithm was used with a time step of 0.05ms. The total integration time length of each simulation run was 5000ms. Simulations were implemented in Python 3.9.7 on PC with 12th Gen Intel(R) Core(TM) i7-12700H 2.30GHz CPU.
The discussion in this section is based on the reciprocal inhibition of two neurons, which we call half-center oscillators (pattern A in Figure 3). The synchronous firing of neurons contains very important neural information. Here we investigate the synchrony of half-central oscillator rhythm activity. In Figure 4, we show the variation trend of synchronous firing with synaptic parameters of the model, and we can see the roughly synchronous discharge pattern. Fixing the synaptic parameter σ, we can find that the reversal potential Esyn has an intermittent effect on synchrony. The effect of the synaptic parameter σ is more continuous than fixing it. The transversely separated bands are very distinct, indicating that the synaptic reversal potential has a significant effect on synchrony. In the following, we will select three appropriate sets of parameters to investigate the synchronization of half-central oscillation according to the phase difference diagram shown in Figure 4. The three sets of parameters are Esyn=−110mV, σ=1 (phase difference between 100ms and 200ms); Esyn=−89mV, σ=1/2 (phase difference between 200 and 250ms); and Esyn=−61mV, σ=1/6 (phase difference between 0 and 50ms).
From the top of the Figure 5 (i.e., when the synaptic parameters are Esyn=−110mV and σ=1), as the two-parameter synaptic conductance is changed, the phase difference of the neuronal membrane potential exceeds 100 except near the diagonal. The diagonal is shown in dark blue, indicating that the two neurons are perfectly synchronized. By simulating their discharge state, it can also be confirmed that synchronization is achieved when the conductance parameters of the two synapses are equal. In particular, in the small regime of two synaptic conductances close to zero and close to one, the area of perfect synchrony is slightly larger. Dark purple indicates that the phase difference between the two neurons is maximal. One interesting observation is that the bifurcation diagram of the phase difference is symmetric about the diagonal as the two-parameter synaptic conductance change.
When the synaptic parameters were changed to Esyn=−89mV and σ=1/2, we obtained the synchronous firing diagram of the half-center oscillator as shown in the middle of Figure 5. The diagonal synchrony seems to be preserved as we can see from the figure. However, the discharge of the diagonal is richer (The variety of the phase difference indicates the richness in the rhythm activity of the half-central oscillator). The bifurcation diagram of the phase difference is still symmetric and we can get a variety of differences in the phase difference depending on the color distribution of the pattern. A variety of rhythm activities of the half-center oscillator can be realized, which makes it easier to operate and transform the half-center oscillator. Furthermore, we can perform the rhythm control of the half-center oscillator. Eventually, we may be able to achieve synchronous control of a half-central oscillator. Synchronization does not necessarily require the two-parameter synaptic conductance to be equal as can be seen from the bottom of Figure 5. Unlike in the previous two diagrams, the distinct dark blue diagonals are missing, but the symmetry about the synaptic conductance is preserved. Here the dark blue area is spread throughout the parameter bifurcation plane and the phase difference is radially spread around the upper right corner, like a ripple of water. Based on the above analysis, small synaptic conductance parameters may make it easier to achieve the synchronous rhythm of the half-center oscillator, and synaptic parameters Esyn and σ have significant effects on their synchronization.
In the following, we will investigate the ISIs of each neuron in the half-center oscillator model with different potassium conductance gK under the above three sets of parameters. The left panel of Figure 6 depicts the bifurcation diagram under the three groups of parameters when the conductance parameter is 4.2nS. It can be seen from the figure that the rhythm activity in the half-center oscillator of the three bifurcation graphs is not synchronous. The top is like a bifurcation process of inverse period-adding and the discharge of the system is relatively stable. We can see that the discharge is very abundant from the middle, especially when the ε is between 0.01 and 0.015, which can be related to the middle of Figure 5. The bottom shows the complex diversity of ISIs. Like the first two, ISIs are concentrated in two layers and it is in a chaotic state. This corresponds to the bottom of Figure 5 and it is interspersed with large phase differences dotted with dots. When the potassium conductance gK=7nS, the ISIs for the three sets of parameters are shown on the right panel of Figure 6. Compared with the left panel, the ISIs have changed fundamentally. Neuron 1 in the half-center oscillator shows smaller ISIs than neuron 2 shown at the top. In the middle, there is only one ISI in the former stage, and the chaotic ISIs start to appear in the later stage. Therefore, the change in potassium conductance has a great influence on the rhythm activity of the half-center oscillator.
We analyzed the bifurcation patterns and the ISIs patterns of the half-center oscillator to investigate the rhythm activity when the potassium conductance is changed. Our research found that altering the relative size of the two-parameter synaptic conductances could control the rhythm pattern of the half-center oscillator. Different synaptic parameters such as Esyn and σ can trigger very different rhythm patterns. Figure 7 presents an example where we took Esyn=−110mV, σ=1, g12=0.2nS, g21=0.8nS to investigate the HCO rhythm under different potassium conductances. It turns out that a single spike on the left panel has branches that cause neuron 1's ISIs to decrease when noise is added to it. We looked at the bursts rhythm, spiking rhythm, and mixed mode rhythm in HCO. A large number of discharge patterns can be implemented in HCO, especially when gK=7nS, by adjusting the asymmetric coupling factor. By comparing the rhythmic activity, we found that when g12 is larger than g21, neuron 1 fires earlier than neuron 2, and vice versa. In other words, synaptic conductance parameters can control the firing start time of neurons in HCO. Synapse conductance parameters can also synchronize discharge patterns in HCO as shown in Figure 8 under different discharge modes when the synaptic parameters are exactly equal. Different discharge mode synchronization will occur under different conductances such as burst synchronization, spiking synchronization, and subthreshold synchronization. We found that in HCO, the synchronization regime is likely to appear when the synaptic conductance parameters are equal, but the synchronization state will also appear when the synaptic conductance parameters are not equal. Synaptic parameters are required to participate in the mediation of neuron firing in this case. The inequality of the two synaptic parameters will lead to a rich rhythm pattern of HCO. The synchronization state disappears when noise acts on neuron 1. When gK=4.2nS, the rhythmic discharge of neuron 1 changes greatly, while the burster structure of neuron 2 is stable. When gK=7nS, the spiking of neurons is disturbed. In particular, when gK=47.4nS, only neuron 1, which was originally subthreshold began to discharge, while neuron 2 remained subthreshold.
Subthreshold oscillation contains rich expression of neuronal information [52]. Here, subthreshold oscillations of the half-center oscillator are shown in Figure 9. As you can be seen from the right panel, Subthreshold oscillations alternate between neurons 1 and 2 in HCO, and subthreshold oscillations appear accompanied by small oscillations at lower membrane voltage values. The subthreshold oscillations in neuron 2 are consistent with the current in synapse 2. A more detailed subthreshold oscillation process can be found in the left panel, where the membrane voltage value of neuron 2 hovers near the lowest center when subthreshold oscillation of neuron 1 begins. When neuron 1 ends subthreshold oscillation, it continuously changes to hover around smaller membrane voltage values, while neuron 2 begins subthreshold oscillation. This is how subthreshold oscillations of neurons 1 and 2 alternate.
This section discusses the patterns B and C (Figure 3) in a tri-neuron CPG. The time difference in calculating its discharge sequence is significant by comparing the single neuron, half-center oscillator, and tri-neuron CPG. The increase in the number of neurons will greatly reduce the computational efficiency. In contrast, the increase in the number of synapses will also affect the computational efficiency, but it is not as obvious as the increase in the number of neurons. Figures 10 and 11 show the discharge pattern in pattern B (Figure 3) under two different synaptic conductance parameters while fixing the potassium conductance gK=4.2nS and two synaptic parameters Esyn=−110mV, σ=1. Pattern B (Figure 3) is the sequential inhibition of connected neuronal circuits, gij indicates the synaptic conductance that the ith neuron inhibits the jth neuron.
As can be seen from Figure 10, for this set of synaptic conductance parameters, members of CPG exhibit different rhythm patterns than those in single neurons and half-center oscillators, which are caused by synaptic coupling of neurons (left panel). If the discharge of blue neuron 1 is taken as the starting point of the member in CPG, the typical CPG rhythm pattern is shown, that is, the discharge order in CPG members is "blue-green-red" or "neuron 1-neuron 3-neuron 2". When the noise is applied to neuron 1, we find that the firing of neuron 1 changes greatly and the firing of neurons 2 and 3 changes weakly.
When we change the synaptic conductance g12=0.1nS in Figure 10, the rhythm pattern shown in Figure 11 is obtained. At this time, the subtle shift in the discharge of members in CPG can be controlled by adjusting the synaptic conductance g12. That is, the rhythm pattern control of such CPG members can be realized and changes in synaptic conductance regulate the rhythm pattern of members in CPG. When the noise is applied to neuron 1, the firing of neurons 2 and 3 changes weakly.
When the conductance of three synapses is equal, the members in CPG exhibit the same burst discharge in pattern B (Figure 3). In pattern B, we found a small rule that increased synaptic conductance delayed the start time of postsynaptic neuron firing. When g31 is much smaller than g12, neurons 3 and 1 tend to be in sync. Using this property, the firing synchronization of neurons in CPG can be easily regulated and controlled.
In the same way, we also analyze the rhythm pattern of tri-neuron CPG, namely pattern C (Figure 3). This pattern is a tri-neuron circuit composed of pairs of neurons that inhibit each other. Although it has the same two discharge sequences as the members of CPG in pattern B (Figure 3), the discharge mode of members in CPG is completely different from that of pattern B (Figure 3), as shown in Figure 12. At this time, the discharge order of CPG members showed "neuron 1-neuron 2-neuron 3", but the membrane voltage sequence of neuron 1 is different from that of neurons 2 and 3. Unlike the Figure 10, each member of the CPG behaves in the same discharge. That is, the reciprocal inhibition of the CPG loop increases the diversity of the discharge activities of its members. In addition, we found that when noise was stimulated to neurons 1–3 respectively, the stimulation of neuron 2 completely destroyed the original CPG firing rhythm pattern. The reason may be that the synaptic conductance parameters of neuron 2 are very small.
In particular, when the conductance of each synapse in the CPG is different (As shown in Figure 13), the member of CPG named neuron 1 shows an burster firing pattern, while neurons 2 and 3 show a mixed bursting pattern. The discharge activities of such CPG members are caused by completely asymmetric coupling factor regulation. The results show that asymmetric coupling factors can strongly regulate the discharge of neurons. Under these synaptic parameters, the firing rhythm pattern was substantially altered when we stimulated neurons 1–3 with noise, respectively. This may be because CPG at this synaptic parameter is in a critical state. The members of CPG show very rich discharge activities in pattern C (Figure 3), not all of which are shown here. We also investigated the patterns of two other types of synaptic connections in which members also have different discharges, only two of which are shown here.
This paper first introduces the rich discharge properties of the single neuron model, which has been used in our modeling. Second, the influence of asymmetric coupling factor and two synaptic parameters σ and Esyn on the half-center oscillators discharge synchronization is discussed. We find that synaptic parameters and noise together affect the discharge of two neurons in the half-center oscillator. Asymmetric coupling factors modulate the rhythm activity in the half-center oscillator. We find that the firing synchronization occurred not only at the diagonal, but also when the remaining two synaptic parameters σ and Esyn are changed. Small synaptic conductance parameters may make it easier to synchronize the half-center oscillator, and the synaptic conductance may control the discharge order of neurons in the half-center oscillator. The interspike intervals diagram of two neurons in the half-center oscillator is used to verify the firing synchronization under several fixed parameters. In addition to the synaptic conductance parameters, the synaptic parameters σ and Esyn can be adjusted to increase the diversity of rhythm activity of the half-center oscillator. The synaptic conductance and synaptic parameters together control its rhythm activities. The rhythms properties of CPG are regulated by the potassium conductance value. The typical CPG modes and discharge synchronization of three groups of different potassium conductance parameters are shown, which are bursting, spiking, and mixed-mode oscillations (MMOs) respectively. It has been shown that noise can regulate the discharge rhythm and synchronization of HCO. Finally, two typical pattern are discussed, and how the asymmetric coupling factors regulate the discharge of members in CPG is analyzed.
The asymmetric coupling factor can adjust the discharge mode of CPG members, such as bursting mode, spiking, and MMOs, to realize the control of rhythms activity in CPG. The phase diagram explains the alternations between neuronal firing patterns and the effect of synaptic currents on membrane potential. We simulated six connected modes, and only the discharge modes of members in partial CPG of the three types were shown here. We find that the rhythm activity of neurons in patterns B and C (Figure 3) were more abundant than those in single and half-center oscillators neurons. Asymmetric coupling factors (synaptic conductance) can control the start time of postsynaptic neuron firing, and the increase of synaptic conductance will delay the start time of postsynaptic neuron firing. When potassium conductance gK is changed, the discharge of CPG members will be greatly changed. For example, when gK=7nS, the members of CPG show abundant spiking discharge mode in pattern B (Figure 3). In the reciprocal inhibition CPG pattern C (Figure 3), when the potassium conductance gK=4.2nS and the synaptic conductance is equal, the members of CPG do not discharge synchronously, which is different from the performance of pattern B (Figure 3). When the potassium conductance gK=7nS and the synaptic conductance is equal, the discharge of the CPG members does not spiking synchronously, which is a very interesting question but we have not shown the diagram in detail here due to space constraints. That will be one of the things we will look at in the future. In addition, we found that noise and synaptic strength together determine the robustness of CPG, and the critical state of CPG rhythmic discharge is sensitive to noise. Furthermore, we have observed the same noise had a greater effect on the rhythm changes of neurons with lower synaptic strength. That is, high strength inhibition connection will improve the stability of CPG. Of course, the changes in synaptic parameters can also regulate the discharge mode of CPG members, so these factors will jointly determine the discharge mode of CPG members. The influence of comprehensive factors will be the focus and difficulty of our future research, and the rhythm activities of other members will also need to be investigated. Finally, synapse parameters will be integrated to control and realize the rhythm activity of CPG.
Our study focused on the chemical synapses between neurons, while the electrical coupling between them was not considered. However, in future studies, we will analyze the synergistic effects of both electrical and chemical coupling in CPG as it can lead to very different rhythmic patterns. Our current model demonstrates that synaptic conductance can influence the rhythm pattern of the tri-neuron network and a change in the conductance can shift the members of the CPG into typical regular sequential discharge mode. Our findings reveal a unique rhythm pattern in CPG, where neuron 1 exhibits chaotic spiking while neurons 2 and 3 show regular bursting. This rhythmic activity and regular sequential discharge pattern of the CPG is crucial for its flexibility, robustness, and ultimate realization of swimming, walking, or other cognitive functions. The diversity of rhythm activities in CPG is essential for its flexibility and robustness, which can resist interference from external information. Changes in synaptic conductance and noise can produce a large number of different rhythm activities, making the CPG less susceptible to external interference.
CPG rhythm patterns have been discovered in invertebrates (crustacean pyloric or gastric) and leech heartbeats. These patterns are used to simulate the rhythm activity of the human central nervous system, which in turn controls the rhythm of arm movement [17]. Additionally, CPG rhythm can help in the diagnosis of various diseases. For instance, Mader et al. [1] have claimed that CPG can assist in the development of therapeutic methods for the recovery of strong spinal cord injuries. Tassinari et al. reviewed the relationship of central pattern generators with parasomnias and sleep-related epileptic seizures and explained some epileptic seizures and parasomnias using the firing patterns of the CPG [53,54]. Therefore, studying the functioning principle of CPG rhythm activities is essential for understanding related motor behaviors and achieving better motor control as well as improving disease diagnosis.
The authors declare they have not used Artificial Intelligence (AI) tools in the creation of this article.
This work was funded by the National Natural Science Foundation of China (Grant Nos. 12202208 and 11872183), the Basic Science (Natural Science) Research Project of Colleges and Universities of Jiangsu Province (Grant No. 22KJB130009), the Research and Cultivation Project for Young Teachers of Nanjing Audit University (Grant No. 2021QNPY015), 2022 Doctoral program of Entrepreneurship and Innovation in Jiangsu Province (Grant No. JSSCBS20220717), the China Scholarship Council (Grant No. 202206150096).
The authors declare there is no conflict of interest.
[1] |
E. Marder, D. Bucher, Central pattern generators and the control of rhythmic movements, Curr. Biol., 11 (2001), R986–R996. https://doi.org/10.1016/S0960-9822(01)00581-4 doi: 10.1016/S0960-9822(01)00581-4
![]() |
[2] |
E. Marder, R. L. Calabrese, Principles of rhythmic motor pattern generation, Physiol. Rev., 76 (1996), 687–717. https://doi.org/10.1152/physrev.1996.76.3.687 doi: 10.1152/physrev.1996.76.3.687
![]() |
[3] |
A. Sakurai, C. A. Gunaratne, P. S. Katz, Two interconnected kernels of reciprocally inhibitory interneurons underlie alternating left-right swim motor pattern generation in the mollusk Melibe leonina, J. Neurophysiol., 112 (2014), 1317–1328. https://doi.org/10.1152/jn.00261.2014 doi: 10.1152/jn.00261.2014
![]() |
[4] |
D. Alaçam, A. Shilnikov, Making a swim central pattern generator out of latent parabolic bursters, Int. J. Bifurcation Chaos, 25 (2015), 1540003. https://doi.org/10.1142/S0218127415400039 doi: 10.1142/S0218127415400039
![]() |
[5] | A. I. Selverston, Model Neural Networks and Behavior, New York, 1985. https://doi.org/10.1007/978-1-4757-5858-0 |
[6] |
W. N. Frost, P. S. Katz, Single neuron control over a complex motor program, PNAS, 93 (1996), 422–426. https://doi.org/10.1073/pnas.93.1.422 doi: 10.1073/pnas.93.1.422
![]() |
[7] | P. S. Katz, S. L. Hooper, Invertebrate central pattern generators, Cold Spring Harbor Monogr. Ser., 49 (2007), 251. |
[8] |
E. Marder, S. Kedia, E. O. Morozova, New insights from small rhythmic circuits, Curr. Opin. Neurobiol., 76 (2022), 102610. https://doi.org/10.1016/j.conb.2022.102610 doi: 10.1016/j.conb.2022.102610
![]() |
[9] |
E. Marder, Neuromodulation of neuronal circuits: back to the future, Neuron, 76 (2012), 1–11. https://doi.org/10.1016/j.neuron.2012.09.010 doi: 10.1016/j.neuron.2012.09.010
![]() |
[10] |
I. Belykh, A. Shilnikov, When weak inhibition synchronizes strongly desynchronizing networks of bursting neurons, Phys. Rev. Lett., 101 (2008), 078102. https://doi.org/10.1103/PhysRevLett.101.078102 doi: 10.1103/PhysRevLett.101.078102
![]() |
[11] |
T. Nowotny, M. I. Rabinovich, Dynamical origin of independent spiking and bursting activity in neural microcircuits, Phys. Rev. Lett., 98 (2007), 128106. https://doi.org/10.1103/PhysRevLett.98.128106 doi: 10.1103/PhysRevLett.98.128106
![]() |
[12] |
A. I. Selverston, Invertebrate central pattern generator circuits, Phil. Trans. R. Soc. B, 365 (2010), 2329–2345. https://doi.org/10.1098/rstb.2009.0270 doi: 10.1098/rstb.2009.0270
![]() |
[13] |
A. I. Selverston, M. I. Rabinovich, H. D Abarbanel, R. Elson, A. Szücs, R. D. Pinto, et al., Reliable circuits from irregular neurons: a dynamical approach to understanding central pattern generators, J. Physiol.-Paris, 94 (2000), 357–374. https://doi.org/10.1016/S0928-4257(00)01101-3 doi: 10.1016/S0928-4257(00)01101-3
![]() |
[14] |
R. Huerta, M. A. Sánchez-Montañés, F. Corbacho, J. A. Sigüenza, A central pattern generator to control a pyloric-based system, Biol. Cybern., 82 (2000), 85–94. https://doi.org/10.1007/PL00007963 doi: 10.1007/PL00007963
![]() |
[15] |
M. Lodi, A. L. Shilnikov, M. Storace, Design principles for central pattern generators with preset rhythms, IEEE Trans. Neural Networks Learn. Syst., 31 (2019), 3658–3669. https://doi.org/10.1109/TNNLS.2019.2945637 doi: 10.1109/TNNLS.2019.2945637
![]() |
[16] |
S. Chen, Y. Liu, T. Chen, J. Lou, Rhythm motion control in bio-inspired fishtail based on central pattern generator, IET Cyber-Syst. Robot., 3 (2021), 53–67. https://doi.org/10.1049/csy2.12007 doi: 10.1049/csy2.12007
![]() |
[17] |
J. Wojcik, J. Schwabedal, R. Clewley, A. L. Shilnikov, Key bifurcations of bursting polyrhythms in 3-cell central pattern generators, PLoS One, 9 (2014), e92918. https://doi.org/10.1371/journal.pone.0092918 doi: 10.1371/journal.pone.0092918
![]() |
[18] |
J. T. C. Schwabedal, A. B. Neiman, A. L. Shilnikov, Robust design of polyrhythmic neural circuits, Phys. Rev. E, 90 (2014), 022715. https://doi.org/10.1103/PhysRevE.90.022715 doi: 10.1103/PhysRevE.90.022715
![]() |
[19] | R. Azodi-Avval, F. Bahrami, A mathematical model of arm movement during rhythmic motor activity, in 2011 18th Iranian Conference of Biomedical Engineering (ICBME), (2011), 304–308. https://doi.org/10.1109/ICBME.2011.6168578 |
[20] |
M. B. Reyes, P. V. Carelli, J. C. Sartorelli, R. D. Pinto, A modeling approach on why simple central pattern generators are built of irregular neurons, PLoS One, 10 (2015), e0120314. https://doi.org/10.1371/journal.pone.0120314 doi: 10.1371/journal.pone.0120314
![]() |
[21] |
J. Dethier, G. Drion, A. Franci, R. Sepulchre, A positive feedback at the cellular level promotes robustness and modulation at the circuit level, J. Neurophysiol., 114 (2015), 2472–2484. https://doi.org/10.1152/jn.00471.2015 doi: 10.1152/jn.00471.2015
![]() |
[22] |
J. Collens, K. Pusuluri, A. Kelley, D. Knapper, T. Xing, S. Basodi, et al., Dynamics and bifurcations in multistable 3-cell neural networks, Chaos, 30 (2020), 072101. https://doi.org/10.1063/5.0011374 doi: 10.1063/5.0011374
![]() |
[23] |
Q. Lu, X. Wang, J. Tian, A new biological central pattern generator model and its relationship with the motor units, Cognit. Neurodyn., 16 (2022), 135–147. https://doi.org/10.1007/s11571-021-09710-0 doi: 10.1007/s11571-021-09710-0
![]() |
[24] |
Q. Lu, J. Tian, Synchronization and stochastic resonance of the small-world neural network based on the CPG, Cognit. Neurodyn., 8 (2014), 217–226. https://doi.org/10.1007/s11571-013-9275-8 doi: 10.1007/s11571-013-9275-8
![]() |
[25] |
Y. Zang, E. Marder, Neuronal morphology enhances robustness to perturbations of channel densities, PNAS, 120 (2023), e2219049120. https://doi.org/10.1073/pnas.2219049120 doi: 10.1073/pnas.2219049120
![]() |
[26] |
E. M. Izhikevich, Neural excitability, spiking, and bursting, Int. J. Bifurcation Chaos, 10 (2000), 1171–1266. https://doi.org/10.1142/S0218127400000840 doi: 10.1142/S0218127400000840
![]() |
[27] |
B. Lu, X. Jiang, Reduced and bifurcation analysis of intrinsically bursting neuron model, Electron. Res. Arch., 31 (2023), 5928–5945. https://doi.org/10.3934/era.2023301 doi: 10.3934/era.2023301
![]() |
[28] |
F. Zhan, S. Liu, X. Zhang, J. Wang, B. Lu, Mixed-mode oscillations and bifurcation analysis in a pituitary model, Nonlinear Dyn., 94 (2018), 807–826. https://doi.org/10.1007/s11071-018-4395-7 doi: 10.1007/s11071-018-4395-7
![]() |
[29] |
W. B. Kristan, Neuronal decision-making circuits, Curr. Biol., 18 (2008), R928–R932. https://doi.org/10.1016/j.cub.2008.07.081 doi: 10.1016/j.cub.2008.07.081
![]() |
[30] |
K. L. Briggman, W. B. Kristan, Multifunctional pattern-generating circuits, Annu. Rev. Neurosci., 31 (2008), 271–294. https://doi.org/10.1146/annurev.neuro.31.060407.125552 doi: 10.1146/annurev.neuro.31.060407.125552
![]() |
[31] |
A. A. A. Hill, J. Lu, M. A. Masino, O. H. Olsen, R. L. Calabrese, A model of a segmental oscillator in the leech heartbeat neuronal network, J. Comput. Neurosci., 10 (2001), 281–302. https://doi.org/10.1023/A:1011216131638 doi: 10.1023/A:1011216131638
![]() |
[32] | R. L. Calabrese, Half-center oscillators underlying rhythmic movements, in The Handbook of Brain Theory and Neural Networks, (1998), 444–447. |
[33] |
Y. Zang, S. Hong, E. D. Schutter, Firing rate-dependent phase responses of Purkinje cells support transient oscillations, eLife, 9 (2020), e60692. https://doi.org/10.7554/eLife.60692 doi: 10.7554/eLife.60692
![]() |
[34] |
M. Liu, L. Duan, In-phase and anti-phase spikes synchronization within mixed Bursters of the pre-Bözinger complex, Electron. Res. Arch., 30 (2022), 961–977. https://doi.org/10.3934/era.2022050 doi: 10.3934/era.2022050
![]() |
[35] |
S. Li, G. Zhang, J. Wang, Y. Chen, B. Deng, Emergent central pattern generator behavior in chemical coupled two-compartment models with time delay, Physica A, 491 (2018), 177–187. https://doi.org/10.1016/j.physa.2017.08.121 doi: 10.1016/j.physa.2017.08.121
![]() |
[36] |
A. Doloc-Mihu, R. L. Calabrese, A database of computational models of a half-center oscillator for analyzing how neuronal parameters influence network activity, J. Biol. Phys., 37 (2011), 263–283. https://doi.org/10.1007/s10867-011-9215-y doi: 10.1007/s10867-011-9215-y
![]() |
[37] | A. Doloc-Mihu, R. L. Calabrese, Analysis of family structures reveals robustness or sensitivity of bursting activity to parameter variations in a half-center oscillator (HCO) model, eNeuro, 3 (2016). https://doi.org/10.1523/ENEURO.0015-16.2016 |
[38] |
I. Elices, P. Varona, Asymmetry factors shaping regular and irregular bursting rhythms in central pattern generators, Front. Comput. Neurosci., 11 (2017), 9. https://doi.org/10.3389/fncom.2017.00009 doi: 10.3389/fncom.2017.00009
![]() |
[39] |
A. J. White, Sensory feedback expands dynamic complexity and aids in robustness against noise, Biol. Cybern., 116 (2022), 267–269. https://doi.org/10.1007/s00422-021-00917-2 doi: 10.1007/s00422-021-00917-2
![]() |
[40] |
Z. Yu, P. J. Thomas, Dynamical consequences of sensory feedback in a half-center oscillator coupled to a simple motor system, Biol. Cybern., 115 (2021), 135–160. https://doi.org/10.1007/s00422-021-00864-y doi: 10.1007/s00422-021-00864-y
![]() |
[41] |
R. Huerta, P. Varona, M. I. Rabinovich, H. D. I. Abarbanel, Topology selection by chaotic neurons of a pyloric central pattern generator, Biol. Cybern., 84 (2001), L1–L8. https://doi.org/10.1007/PL00007976 doi: 10.1007/PL00007976
![]() |
[42] |
V. In, A. Kho, P. Longhini, J. D. Neff, A. Palacios, P. L. Buono, Meet ANIBOT: the first biologically-inspired animal robot, Int. J. Bifurcation Chaos, 32 (2022), 2230001. https://doi.org/10.1142/S0218127422300014 doi: 10.1142/S0218127422300014
![]() |
[43] |
A. S. Lele, Y. Fang, J. Ting, A. Raychowdhury, Learning to walk: bio-mimetic hexapod locomotion via reinforcement-based spiking central pattern generation, IEEE J. Emerging Sel. Top. Circuits Syst., 10 (2020), 536–545. https://doi.org/10.1109/JETCAS.2020.3033135 doi: 10.1109/JETCAS.2020.3033135
![]() |
[44] |
T. Sun, Z. Dai, P. Manoonpong, Distributed-force-feedback-based reflex with online learning for adaptive quadruped motor control, Neural Networks, 142 (2021), 410–427. https://doi.org/10.1016/j.neunet.2021.06.001 doi: 10.1016/j.neunet.2021.06.001
![]() |
[45] |
A. Espinal, H. Rostro-Gonzalez, M. Carpio, E. I. Guerra-Hernandez, M. Ornelas-Rodriguez, M. Sotelo-Figueroa, Design of spiking central pattern generators for multiple locomotion gaits in hexapod robots by christiansen grammar evolution, Front. Neurorobot., 10 (2016), 6. https://doi.org/10.3389/fnbot.2016.00006 doi: 10.3389/fnbot.2016.00006
![]() |
[46] |
F. Zhan, S. Liu, Response of electrical activity in an improved neuron model under electromagnetic radiation and noise, Front. Comput. Neurosci., 11 (2017), 107. https://doi.org/10.3389/fncom.2017.00107 doi: 10.3389/fncom.2017.00107
![]() |
[47] |
F. Zhan, S. Liu, J. Wang, B. Lu, Bursting patterns and mixed-mode oscillations in reduced Purkinje model, Int. J. Mod. Phys. B, 32 (2018), 1850043. https://doi.org/10.1142/S0217979218500431 doi: 10.1142/S0217979218500431
![]() |
[48] |
D. Terman, J. E. Rubin, A. C. Yew, C. J. Wilson, Activity patterns in a model for the subthalamopallidal network of the basal ganglia, J. Neurosci., 22 (2002), 2963–2976. https://doi.org/10.1523/JNEUROSCI.22-07-02963.2002 doi: 10.1523/JNEUROSCI.22-07-02963.2002
![]() |
[49] |
F. Su, J. Wang, S. Niu, H. Li, B. Deng, C. Liu, et al., Nonlinear predictive control for adaptive adjustments of deep brain stimulation parameters in basal ganglia–thalamic network, Neural Networks, 98 (2018), 283–295. https://doi.org/10.1016/j.neunet.2017.12.001 doi: 10.1016/j.neunet.2017.12.001
![]() |
[50] |
J. Song, S. Liu, H. Lin, Model-based quantitative optimization of deep brain stimulation and prediction of Parkinson's states, Neuroscience, 498 (2022), 105–124. https://doi.org/10.1016/j.neuroscience.2022.05.019 doi: 10.1016/j.neuroscience.2022.05.019
![]() |
[51] |
J. Song, H. Lin, S. Liu, Basal ganglia network dynamics and function: role of direct, indirect and hyper-direct pathways in action selection, Network: Comput. Neural Syst., 34 (2023), 84–121. https://doi.org/10.1080/0954898X.2023.2173816 doi: 10.1080/0954898X.2023.2173816
![]() |
[52] |
M. Valero, I. Zutshi, E. Yoon, G. Buzsáki, Probing subthreshold dynamics of hippocampal neurons by pulsed optogenetics, Science, 375 (2022), 570–574. https://doi.org/10.1126/science.abm1891 doi: 10.1126/science.abm1891
![]() |
[53] |
C. A. Tassinari, G. Cantalupo, B. Hoegl, P. Cortelli, L. Tassi, S. Francione, et al., Neuroethological approach to frontolimbic epileptic seizures and parasomnias: the same central pattern generators for the same behaviours, Rev. Neurol., 165 (2009), 762–768. https://doi.org/10.1016/j.neurol.2009.08.002 doi: 10.1016/j.neurol.2009.08.002
![]() |
[54] |
C. A. Tassinari, E. Gardella, G. Cantalupo, G. Rubboli, Relationship of central pattern generators with parasomnias and sleep-related epileptic seizures, Sleep Med. Clin., 7 (2012), 125–134. https://doi.org/10.1016/j.jsmc.2012.01.003 doi: 10.1016/j.jsmc.2012.01.003
![]() |
1. | Feibiao Zhan, Jian Song, Complex rhythm and synchronization of half-center oscillators under electromagnetic induction, 2024, 32, 2688-1594, 4454, 10.3934/era.2024201 | |
2. | Jian Song, Carlo R. Laing, Shenquan Liu, A dynamical analysis of collective behaviour in a multi-population network with infinite theta neurons, 2024, 01672789, 134468, 10.1016/j.physd.2024.134468 |