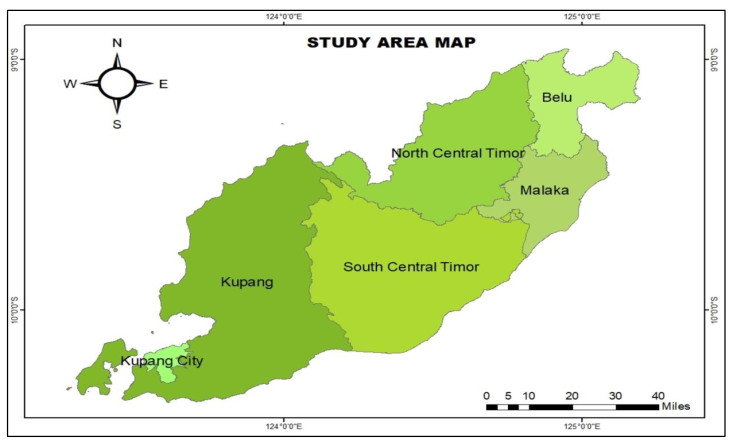
Deep learning, particularly generative models, has inspired controllable image synthesis methods and applications. These approaches aim to generate specific visual content using latent prompts. To explore low-level controllable image synthesis for precise rendering and editing tasks, we present a survey of recent works in this field using deep learning. We begin by discussing data sets and evaluation indicators for low-level controllable image synthesis. Then, we review the state-of-the-art research on geometrically controllable image synthesis, focusing on viewpoint/pose and structure/shape controllability. Additionally, we cover photometrically controllable image synthesis methods for 3D re-lighting studies. While our focus is on algorithms, we also provide a brief overview of related applications, products and resources for practitioners.
Citation: Shixiong Zhang, Jiao Li, Lu Yang. Survey on low-level controllable image synthesis with deep learning[J]. Electronic Research Archive, 2023, 31(12): 7385-7426. doi: 10.3934/era.2023374
[1] | Kaoutar MOUNIR, Isabelle LA JEUNESSE, Haykel SELLAMI, Abdessalam ELKHANCHOUFI . Spatiotemporal analysis of drought occurrence in the Ouergha catchment, Morocco. AIMS Environmental Science, 2023, 10(3): 398-423. doi: 10.3934/environsci.2023023 |
[2] | Zinabu A. Alemu, Emmanuel C. Dioha, Michael O. Dioha . Hydro-meteorological drought in Addis Ababa: A characterization study. AIMS Environmental Science, 2021, 8(2): 148-168. doi: 10.3934/environsci.2021011 |
[3] | Carolyn Payus, Siti Irbah Anuar, Fuei Pien Chee, Muhammad Izzuddin Rumaling, Agoes Soegianto . 2019 Southeast Asia Transboundary Haze and its Influence on Particulate Matter Variations: A Case Study in Kota Kinabalu, Sabah. AIMS Environmental Science, 2023, 10(4): 547-558. doi: 10.3934/environsci.2023031 |
[4] | Joseph A. Kazery, Dayakar P. Nittala, H. Anwar Ahmad . Meteorological effects on trace element solubility in Mississippi coastal wetlands. AIMS Environmental Science, 2019, 6(1): 1-13. doi: 10.3934/environsci.2019.1.1 |
[5] | Suhail Mahmud, Md Al Masum Bhuiyan, Nusrat Sarmin, Sanjida Elahee . Study of wind speed and relative humidity using stochastic technique in a semi-arid climate region. AIMS Environmental Science, 2020, 7(2): 156-173. doi: 10.3934/environsci.2020010 |
[6] | Tuan Syaripah Najihah, Mohd Hafiz Ibrahim, Nurul Amalina Mohd Zain, Rosimah Nulit, Puteri Edaroyati Megat Wahab . Activity of the oil palm seedlings exposed to a different rate of potassium fertilizer under water stress condition. AIMS Environmental Science, 2020, 7(1): 46-68. doi: 10.3934/environsci.2020004 |
[7] | Abebe Kebede Habtegebreal, Abebaw Bizuneh Alemu, U. Jaya Prakash Raju . Examining the Role of Quasi-biennial Oscillation on Rainfall patterns over Upper Blue Nile Basin of Ethiopia. AIMS Environmental Science, 2021, 8(3): 190-203. doi: 10.3934/environsci.2021013 |
[8] | John R. Christy . Examination of Extreme Rainfall Events in Two Regions of the United States since the 19th Century. AIMS Environmental Science, 2019, 6(2): 109-126. doi: 10.3934/environsci.2019.2.109 |
[9] | Khaled Hazaymeh, Quazi K. Hassan . Remote sensing of agricultural drought monitoring: A state of art review. AIMS Environmental Science, 2016, 3(4): 604-630. doi: 10.3934/environsci.2016.4.604 |
[10] | Volodymyr Ivanov, Viktor Stabnikov, Chen Hong Guo, Olena Stabnikova, Zubair Ahmed, In S. Kim, and Eng-Ban Shuy . Wastewater engineering applications of BioIronTech process based on the biogeochemical cycle of iron bioreduction and (bio)oxidation. AIMS Environmental Science, 2014, 1(2): 53-66. doi: 10.3934/environsci.2014.2.53 |
Deep learning, particularly generative models, has inspired controllable image synthesis methods and applications. These approaches aim to generate specific visual content using latent prompts. To explore low-level controllable image synthesis for precise rendering and editing tasks, we present a survey of recent works in this field using deep learning. We begin by discussing data sets and evaluation indicators for low-level controllable image synthesis. Then, we review the state-of-the-art research on geometrically controllable image synthesis, focusing on viewpoint/pose and structure/shape controllability. Additionally, we cover photometrically controllable image synthesis methods for 3D re-lighting studies. While our focus is on algorithms, we also provide a brief overview of related applications, products and resources for practitioners.
The recent abnormal behavior of rainfall and air temperature has caused great impacts on the environment and human life. One of the most significant impacts is the unpredictable changes in seasonal patterns. In some areas, high rainfall will cause flooding; in others, low rainfall with high air temperatures will result in drought. Drought prediction is one of the biggest challenges for scientists and hydrologists, mainly due to its complex nature: these events are random and can fluctuate over time [1]. In predicting drought, the accuracy of rainfall data is crucial because rainfall is the main factor determining water availability in a region [2]. In addition, air temperature, which affects the rate of evaporation, also plays an important role in modeling and predicting drought phenomena [3,4,5].
Drought is one of the most devastating natural disasters, impacting water supply, agriculture, energy production, ecosystems, and society [6]. Drought has affected many parts of the world over the past few decades, such as in the Southeast United States [7], China [8,9], Brazil [10], and Pakistan [11]. Drought can be classified into four categories: Meteorological drought, which is the lack of rainfall to below-normal levels in a certain period of time, hydrological drought, the lack of water availability in and on the surface of the soil, agricultural drought, the reduced yield or agricultural production due to reduced water supply, and socioeconomic drought, related to demand and supply in a market for goods of economic value [12].
The measurement tool for drought is called the drought index, a single value that can describe the severity of drought. Meteorological drought indices that can be used to monitor drought conditions include the Palmer drought severity index (PDSI), which uses the water balance equation in the soil [13], and the standardized precipitation index (SPI) using the rainfall probability approach [14]. In recent years, new drought indices have been developed to improve the effectiveness of existing ones. One of these developments is the standardized precipitation evapotranspiration index (SPEI). The SPEI is a development of the SPI that only considers rainfall. SPEI adds potential evapotranspiration parameters in its calculation to describe drought better than relying on rainfall alone. This is a response to climate change and its effect on drought [15]. The time scales of SPEI calculation are the same as the SPI: The 1-month period is used for short-term drought recognition, the 3- and 6-month periods are used for seasonal drought recognition, the 12-month period is used for medium-term drought, and the 24- and 48-month periods are used for long-term drought assessment [14].
Drought monitoring using the 1-month SPEI drought index in Timor Island, East Nusa Tenggara, has shown that drought events in Kupang City spanned 94 months, with different intensity classifications: 63 months experienced "moderately dry" levels, 25 months had "severely dry" levels, and 6 months reached "extremely dry" levels. Meanwhile, in Kupang Regency, there were 93 months of drought, 62 months at the "moderately dry" level, 26 months at the "severely dry" level, and 5 months reaching the "extremely dry" level. South Central Timor Regency recorded 90 months of drought intensity, with 59 months at the "moderately dry" level, 25 months at the "severely dry" level, and 6 months reaching the "extremely dry" level. In North Central Timor Regency, 88 months of drought occurred, with 62 months at the "moderately dry" level, 20 months at the "severely dry" level, and 6 months at the "extremely dry" level. Malaka Regency recorded 95 months of drought, with 66 months at the "moderately dry" level, 25 months at the "severely dry" level, and 4 months at the "extremely dry" level. In Belu Regency, there were 87 months of drought, with 59 months at the "moderately dry" level, 23 months at the "severely dry" level, and 5 months at the "extremely dry" level.
The distribution of drought intensity on Timor Island, classified based on the 1-month SPEI drought level in each observation area, shows a very significant variation. In addition, there is a natural trend where more severe drought events, namely "extremely dry" and "very dry" events, tend to occur less frequently than less severe events, such as normal drought. In a stochastic process, this trend can be interpreted as a power law where the intensity of drought events decreases as their severity increases. The power law reflects that very severe drought events have a lower probability of occurrence than milder drought events. The large fluctuations in the tail of the power law distribution, which includes extreme but rare events, indicate that extreme events have a very low probability but can occur with very large intensity [16]. The power law process is one of the case models of the non-homogeneous Poisson process with the intensity function of the form (βγ)(tγ)(β−1) [17].
The non-homogeneous Poisson process is a commonly used model to model the number of events as a function of time [18]. Special case models of the non-homogeneous Poisson process have been widely used in various disciplines, including hydrometeorology, as shown in the study of Achcar et al. [19], where non-homogeneous Poisson process models, namely Weibull and Goel Okumoto with multiple variable points, were used to estimate the number of ozone levels exceeding the standard limit in Mexico City. Another study by Achar et al. [20] used the non-homogeneous Poisson process at the change point with the power law process model to analyze the drought period based on the SPI in Brazil. Ellahi et al. [21] used the non-homogeneous Poisson process model with a linear intensity function to assess the number of hydrological drought events using the SPI in Pakistan.
In addition, research on drought prediction using the SPEI index has also been carried out in many parts of the world. Ghasemi et al. [22] and Karbasi et al. [23] forecasted the SPEI 12 drought index in Iran; Dikshit et al. [24] predicted the size of drought using the SPEI on two different time scales (SPEI 1 and SPEI 3) in the New South Wales region, Australia; Affandy et al. [25] modeled and predicted meteorological drought measured by the SPEI with a time range of 1, 3, 6, and 12 months in Lamongan Regency, Indonesia.
The power law process can occur in various natural and artificial phenomena, covering several fields of science such as biology, economics, physics, chemistry, and computer science [26,27,28,29,30]. Statistical inference for the power law process is generally based on the maximum likelihood estimator (MLE) and its asymptotic properties. The MLE is used to find the parameter values in the power law process model that are most likely to yield the observed data [31]. A special characteristic of the power law process is the estimated value of the shape parameter (β), which can describe how an intensity can increase or decrease. If β>1, the intensity of an event will increase; if β<1, the intensity of an event will decrease; and if β=1, the power law process reduces to a homogeneous Poisson process [17]. Two categories of data cases can be applied for parameter estimation in the power law process model: the time interval between events and the number of events observed in the specified interval [32]. In this study, the estimation method is applied to the second category, where the number of drought events based on 1-month SPEI in Timor Island is considered a random variable with a predetermined observed time interval. The fit test results using the acute Cramér-von Mises test showed that the intensity of drought events based on the 1-month SPEI on Timor Island fits the power law process model. The parameter estimation of the power law process using MLE in each observation area shows (β)>1, with Kupang City having a value of 1.063; Kupang Regency 1.174; South Central Timor Regency 1.095; North Central Timor Regency 1.049; Malaka Regency 1.034, and Belu Regency 1.112. This indicates a possible increase in the intensity of drought events in these areas. Therefore, as a mitigation effort and early planning in the face of future drought events, this study aims to analyze short-term meteorological drought periods using the power law process to obtain an estimate of the duration of future drought events.
Figure 1 shows the area of study was conducted in the Timor Island region of East Nusa Tenggara (NTT), Indonesia, covering six regency/cities, namely Kupang City, Kupang Regency, South Central Timor Regency, North Central Timor Regency, Malaka Regency, and Belu Regency. Geographically, the six locations are located in the western part of the Timor Archipelago with coordinates of 9°14' N and 124°56' E. The data used are secondary in the form of monthly rainfall amounts and monthly average air temperatures obtained from NASA Power through the website https://power.larc.nasa.gov/data-access. Data were used as input data for the SPEI 1-month drought index calculation parameters These results were classified based on drought severity to obtain the intensity of drought events in each observation area. The intensity of drought events was measured based on the frequency of drought periods within a time span. The observation period was from January 1981 to December 2023, with a record length of 516 months.
The SPEI was designed to consider rainfall and potential evapotranspiration (PET) in determining drought. The SPEI drought index calculation is based on the deficit value between rainfall and PET [15]. PET can be calculated by the Thornthwaite method using average air temperature based on the following equation [33]:
PET=16K(10TI)m | (1) |
K is a correction factor based on the latitude position of the observation area, T is the monthly average air temperature (∘C), and I is the annual internal heat index obtained from the sum of i for 12 months in the following equation:
i=(T5)1,514andI=∑121i | (2) |
The m is a coefficient that is a variable tied to I with the equation given by:
m=6,75×10−7I3−7,71×10−5I2+1,792×10−2I+0.492 | (3) |
The deficit between rainfall and PET or climate water balance can be determined by the following equation:
Di=CHi−PETi | (4) |
Di is the value of climate water balance in month i, CHi is the amount of rainfall in month i, and PETi is PET in month i in mm. Next, the value of Di is standardized based on the probability density function of the log-logistic distribution with three parameters to capture the deficit value, since it is likely that the moisture deficit in arid and semi-arid areas may be negative. For the two-parameter distribution as used in SPI, the variable D has a lower limit of zero (0>D<∞), which means D can only take positive values, while for the three-parameter distribution used in SPEI, D can take values in the range (γ>D<∞), which means D can also take negative values [15]. The probability density function of the log-logistic distribution is given as:
f(D)=βα(D−γα)β−1[1+(D−γα)β]−2 | (5) |
The parameters α, β, γ in the log-logistic distribution are calculated using the L-moment procedure. L-moment calculation of Pearson Ⅲ distribution parameters can be obtained through the following equation [34]:
β=2W1−W06W1−W0−6W2 | (6) |
α=(W0−2W1)βГ(1+1β)Г(1−1β) | (7) |
γ=W0−αГ(1+1β)Г(1−1β) | (8) |
Г(β) is the gamma distribution function of β. W is the probability weighted moments (PWMs) obtained from the following equation:
Ws=1N∑Ni=1(1−Fi)sDi | (9) |
s is the number of PWMs, and Fi is a frequency estimator that can be calculated using the equation given by:
Fi=i−0,35N | (10) |
i is the range of observations arranged in ascending order and N is the number of data used. The probability function of the distribution D over various time scales can be calculated using the following equation:
F(D)=[1+(αD−γ)β]−1 | (11) |
Based on the probability function, the SPEI can be calculated using the following equation [35]:
SPEI=(t−c0+c1W+c2W21+d1W+d2W2+d3W3) | (12) |
W=√−2ln(P) for P ≤0.5 | (13) |
W=√−2ln(1−P) for P ≥0.5 | (14) |
P is the probability of exceeding the value of D, which is determined by the following equation:
P=1−F(x) | (15) |
The coefficient value of McKee is as follows:
c0=2.515517d1=1.432788c1=0.802853d2=0.189269c2=0.010328d3=0.001308 |
Drought occurs when SPEI reaches drought intensity with SPEI value ≤ –1. The classification of SPEI drought index values is based on Table 1 [36].
SPEI value | Classification |
≥2.00 | Extremely wet |
1.50–1.99 | Very wet |
1.00–1.49 | Moderately wet |
(–0.99)–0.99 | Normal |
(–1.00)–(–1.49) | Moderately dry |
(–1.50)–(–1.99) | Severely dry |
≤ –2.00 | Extremely dry |
The non-homogeneous Poisson process is a stochastic process used to count the number of events in a given time interval, where the rate of events is not constant but depends on time. A process counting {N(t),t≥0} is said [37] to be a non-homogeneous Poisson process with intensity function λ(t), t≥0, if:
a. N(0)=0,
b. {N(t),t≥0} has independent increment,
c. P{N(t+h)−N(t)=1}=λ(t)+o(h), and
d. P{N(t+h)−N(t)≥2}=o(h), where h > 0 and o(h) is a small number satisfying the condition limh→0o(h)h=0.
The expected value, also known as the cumulative function of the non-homogeneous Poisson process {N(t),t≥0} with intensity function λ(t) is defined as:
m(t)=∫t0λ(t)dt | (16) |
Based on Eq 16, the average estimate of N(t) is given by the equation:
ˆm(t)=E(N(t))=∫t0ˆλ(t)dt | (17) |
where E(.) is the expectation value. {N(t),t≥0}, modeled as a non-homogeneous Poisson process, is expressed as:
P(N(t)=n)=[∫t0λ(t)dt]n!exp−∫t0λ(t)dtn=0,1,2…n | (18) |
Based on Eq 16, for t,s>0,N(t+s)−N(t) has the following expected value function:
m(t+s)−m(t)=∫t+s0λ(t)dt | (19) |
Thus, if {N(t+s),t,s≥0}, based on Eq 18, it can be modeled as a non-homogeneous Poisson process as follows:
P(N(t+s)−N(t)=n)=(m(t+s)−m(t))nn!exp(−(m(t+s)−m(t)) | (20) |
A power law process is a special case of non-homogeneous Poisson process with intensity function given by [17]:
λ(t)=(βγ)(tγ)β−1,γ>0,β>1,t>0 | (21) |
Meanwhile, the expectation value based on Eq 16 is given by:
m(t)=(tγ)β,γ>0,β>1,t>0 | (22) |
The intensity function of the power law process can be used to estimate the event rate at a given time. This is because the shape parameter (β) can describe how an intensity can increase or decrease. If β > 1, the intensity of an event will increase: if β<1, the intensity of the event will decrease; and if β=1, the power law process reduces to a homogeneous Poisson process [17].
There are several goodness-of-fit test procedures that can be used to test the suitability of the power law process model, including Kuiper's V Test, Watson's U2 Test, Anderson-Darling A2 Test, Shapiro-Wilk Test, and Cramér-von Mises Test. The Cramér-von Mises test uses the following hypothesis:
H0: Event intensity fits the power law process model.
H1: Event intensity does not fit the power law process model.
The Cramér-von Mises test statistic is expressed based on the following equation:
C2R=112(n)+n∑i=1(¯R−2i−12n)2 | (23) |
¯R is the ratio power transformation given by Eq 29.
¯R=(tit)¯β | (24) |
¯β is the unbiased estimator given by Eq 27.
¯β=(n−2)∑ni=1ln(tti) | (25) |
The H0 decision is accepted if the calculated value of the C2R test statistic is smaller than the critical value for the Cramér-von Mises test, which means that the power law process model is appropriate. If the value of the C2R test statistic is greater than the critical value for the Cramér-von Mises test, then H0 is rejected, which means that the model is not suitable, and a more suitable model needs to be used [17].
Suppose t1,t2,t3,…,tn are mutually independent random samples from a distribution with a joint probability density function f(t1,t2,t3,…,tn;β,γ) with n representing the number of events occurring until time ti for 0<t1<t2<t3<⋯<tn. If the joint likelihood function is expressed as a function of β and γ, then the likelihood function is denoted as L(t1,t2,t3,…,tn;β,γ). The likelihood function for the parameters β and γ is given as follows [17]:
L(t1,t2,t3,…,tn;β,γ)=(n∏i=1λ(ti;β,γ))exp(−∫tn0λ(ti;β,γ)dt) | (26) |
Based on Eq 21, the likelihood function with intensity function λ(ti;β,γ) in Eq 23 is:
L(ti;β,γ)=(n∏i=1(βγ)(tiγ)β−1)exp(−∫tn0(βγ)(tγ)β−1dt) | (27) |
Based on Eq 24, the logarithmic likelihood function L(t;β,γ)=ln(L(t;β,γ)) is:
L(t;β,γ)=nln(β)−nβln(γ)+(β−1)n∑i=1ln(ti)−(tγ)β | (28) |
Furthermore, Eq 25 is derived with respect to β and γ so that the maximum likelihood estimator is obtained as follows:
ˆβ=n∑ni=1ln(tti) | (29) |
ˆγ=tn1ˆβ | (30) |
The SPEI is a drought index used to analyze meteorological drought conditions by considering the standardization of rainfall deficits with potential evapotranspiration (PET) or climate water balance. In this study, the SPEI calculation time scale used a 1-month period, which is adjusted to the needs of researchers to evaluate drought in the short term. SPEI defines a drought event as occurring when the SPEI value is below or equal to the -1 threshold, and the drought event ends when the SPEI value returns to positive. The index classifies drought levels into three main categories: dry, very dry, and extremely dry. The classification of drought levels by SPEI is based on the SPI classification table as follows [14,15,36]:
1) Moderately dry: Occurs when the SPEI value is between –1 and –1.49. This indicates mild drought that may affect water availability.
2) Severely dry: Occurs when the SPEI value is between –1.5 and –1.99. This indicates a more serious drought that can significantly impact agriculture, clean water, and ecosystems.
3) Extremely dry: Occurs when the SPEI value is below –2. This category represents the worst drought index and can cause major losses to agriculture, water availability, and the environment.
The SPEI calculation process uses Eqs 1–15. A time series plot of the calculated values of the 1-month SPEI for each observation area on Timor Island is shown in Figure 2.
Figure 2 shows that SPEI values close to 0 in each observation area indicate near-normal conditions, while positive or negative values indicate above or below-normal conditions. There are many negative SPEI values lower than or equal to –1, indicating that there are frequent droughts at dry, very dry, and extremely dry levels in each observation area of Timor Island. This result aligns with the research of Kuswanto et al. [38], which shows that very dry events are more common in the eastern region of NTT; in this case, Timor Island is the eastern region of NTT.
Furthermore, SPEI values lower than or equal to –1 were characterized to obtain drought intensity, duration, and severity during the observation period [14]. The characterization of 1-month SPEI values in each region of Timor Island can be seen in Table 2.
Observation area | Extremely dry index | Longest drought duration | Intensity of drought months by drought level | ||||
Value | Month of incident | Moderately dry | Severely dry | Extremely dry | Total | ||
Kupang City | –2.47 | August 1988 | 4 months | 63 months | 25 months | 6 months | 94 months |
Kupang | –2.74 | April 2016 | 4 months | 62 months | 26 months | 5 months | 93 months |
South Central Timor | –3.86 | August 1998 | 4 months | 59 months | 25 months | 6 months | 90 months |
North Central Timor | –2.61 | August 1998 | 4 months | 62 months | 20 months | 6 months | 88 months |
Malaka | –2.82 | August 2010 | 5 months | 66 months | 25 months | 4 months | 95 months |
Belu | –3.07 | August 2010 | 4 months | 59 months | 23 months | 5 months | 87 months |
The worst SPEI indices, with values lower than or equal to –2 (extremely dry conditions), have occurred throughout Timor Island. On average, the most severe SPEI drought indices occurred in April, except in Kupang Regency. The most extreme short-term meteorological drought ever recorded on Timor Island occurred in South Central Timor Regency, with a drought index of –3.86 in August 1998.
The longest droughts were as follows: In Kupang City for 4 consecutive months from August to November 1988; in Kupang Regency for 4 consecutive months, occurring from June to September 1998; in South Central Timor Regency for 4 consecutive months from May to September 1998 and again from February to March 2018; in North Central Timor Regency for 4 consecutive months, also in two different periods, namely from June to September 1996 and June to September 1998; in Malaka Regency for 5 consecutive months, occurring from August to November 2020; and in Belu Regency for 4 consecutive months, occurring from November 1997 to February 1998 and again from June to September 1998. In addition, the intensity of drought events in each region varies greatly. The distribution of the intensity of drought events can be seen in Figure 3.
Figure 3 illustrates the pattern of variance in the intensity of drought events, which is that extremely dry events tend to occur less frequently than severely dry and moderately dry ones. This phenomenon demonstrates the complexity of drought as a natural phenomenon that involves factors such as time distribution, scale, and varying intensity of occurrence. An appropriate and effective method is needed to understand and describe drought dynamics.
In the analysis of short-term meteorological drought using the 1-month SPEI on Timor Island, the natural trend where more severe events tend to be less frequent than weaker events can be interpreted with a stochastic process model, the power law process. This process can explain how the event's intensity is inversely proportional to its magnitude [16,18]. The power law process helps researchers understand the pattern of drought intensity and can be used to predict the likelihood of future drought events. Therefore, to better understand the spatial distribution of SPEI values on Timor Island, the SPEI index was mapped every month in 2023, as shown in Figure 4.
The 2023 drought distribution map shows that all areas of Timor Island were affected by drought, as seen from the orange and red colors. In 2023, there were 3 months of drought in Kupang City, Kupang, South Central Timor, and Malaka, and 2 months in North Central Timor and Belu. In January, all observed areas experienced drought at a moderately dry level; in October, almost all observed areas experienced drought at a severely dry level, except for Malaka Regency, which experienced a moderately dry level. In November, a moderately dry drought occurred in Malaka Regency, while other regions had returned to normal. In December, an extremely dry drought occurred in South Central Timor Regency, and a severely dry drought was observed in Kupang City and Kupang Regency.
The presentation of the drought distribution map only for 2023 is based on the need to provide up-to-date information on drought conditions on Timor Island. Although the range of observations covers the years 1981–2023, 2023 was chosen due to the relevance of the current information desired in this study. By focusing on that year, a more in-depth understanding of the current spatial distribution of SPEI values in the Timor Island region can be obtained.
We tested the suitability of the power law process model using the Cramér-von Mises test [17]. The hypothesis used is as follows:
H0: The intensity of drought events based on the 1-month SPEI fits the power law process model.
H1: The intensity of drought events based on the 1-month SPEI does not fit the power law process model.
The Cramér-von Mises statistic (C2R) is obtained using Eqs 23–25. The results are presented in Table 3.
Observation area | C2R | Critical value | Decision |
Kupang City | 0.082 | 0.22 | H0 accepted |
Kupang | 0.062 | 0.22 | H0 accepted |
South Central Timor | 0.089 | 0.22 | H0 accepted |
North Central Timor | 0.171 | 0.22 | H0 accepted |
Malaka | 0.043 | 0.22 | H0 accepted |
Belu | 0.022 | 0.22 | H0 accepted |
Based on Table 3, the C2R value of all observation areas is below the critical value determined based on the frequency of drought events, and H0 is accepted. These results indicate that the intensity of drought events based on 1-month SPEI in each region of Timor Island fits the power law process model.
The estimated values of the shape (β) and scale (γ) parameters in the power law process intensity function were obtained using the MLE method based on Eqs 29 and 30 using the time truncated estimate of the power law process [17]. Data used are drought frequency, time of occurrence, and observation time span. The parameter estimation results are presented in Table 4.
Observation area | Parameter | |
ˆβ | ˆγ | |
Kupang City | 1.063 | 7.170 |
Kupang | 1.174 | 10.859 |
South Central Timor | 1.095 | 8.481 |
North Central Timor | 1.049 | 7.245 |
Malaka | 1.034 | 6.296 |
Belu | 1.112 | 9.282 |
Table 4 shows that the ˆβ parameter in each observation area was higher than 1. Based on the characteristics of the power law process, if the intensity function is greater than 1, the intensity of an event will increase [13]. Therefore, it can be concluded that the intensity of drought events in each region of Timor Island will increase, so it is necessary to estimate the frequency of future drought events.
Suppose the time value of drought occurrence in each observation area is ti<t2<⋯<tn<t so that N(t)={N,ti<t2<⋯<tn;(0,t]} with N expressing the frequency of months with drought occurrence in the time interval (0, t]. It is known that the total months observed from 1981–2023 are t = 516 months. The period of months to be estimated is the following 12 months, so if t + s, then 516 + 12 = 528, meaning that the last month of estimation is the 528th month. The initial time of estimation starts from the time after the last time of observation, so the estimation time interval becomes [517,528]. The number of months to be estimated is 12, so the possible value of n is n = 1, 2, 3, ..., 12. The interpretation of the observation time based on the range of 1981–2023 is as follows: The first month is January 2023, and the 516th month is December 2023. For the estimation months, the 517th month is January 2024, and the 528th month is December 2024.
The expected frequency m(t) of drought occurrence in the observation time [1,516] can be obtained by substituting the estimated values of parameters ˆβ and ˆγ in each region of Timor Island into Eq 22. A comparison between expected values and real monthly frequency values is presented in Figure 5.
Based on Figure 4, the expected value shows a very good level of agreement with the actual value. This indicates that the power law process model accurately predicts the number of months with drought events. Furthermore, the estimation of the expected value (m(t+s)) of drought frequency in the future 12 months or in observations [517,528] is presented in Table 5.
Months | Kupang City | Kupang | South Central Timor | Nort Central Timor | Malaka | Belu |
517 (Jan 2024) | 94.207 | 93.244 | 90.195 | 87.957 | 95.393 | 87.374 |
518 (Feb 2024) | 94.400 | 93.456 | 90.386 | 88.136 | 95.584 | 87.562 |
519 (Mar 2024) | 94.594 | 93.668 | 90.577 | 88.314 | 95.775 | 87.750 |
520 (Apr 2024) | 94.788 | 93.879 | 90.768 | 88.493 | 95.965 | 87.938 |
521 (May 2024) | 94.981 | 94.091 | 90.959 | 88.671 | 96.156 | 88.126 |
522 (Jun 2024) | 95.175 | 94.304 | 91.150 | 88.850 | 96.347 | 88.315 |
523 (Jul 2024) | 95.369 | 94.516 | 91.342 | 89.028 | 96.538 | 88.503 |
524 (Aug 2024) | 95.563 | 94.728 | 91.533 | 89.207 | 96.729 | 88.691 |
525 (Sep 2024 | 95.756 | 94.940 | 91.724 | 89.385 | 96.920 | 88.879 |
526 (Oct 2024) | 95.950 | 95.152 | 91.916 | 89.564 | 97.111 | 89.067 |
527 (Nov 2024) | 96.144 | 95.365 | 92.107 | 89.743 | 97.301 | 89.256 |
528 (Dec 2024) | 96.338 | 95.577 | 92.299 | 89.921 | 97.492 | 89.444 |
The predicted results show that, in the next 12 months, the drought frequency in each region of Timor Island will increase by 2 months from the initial observation. For example, in Kupang City, the drought frequency in the 516-month observation is 94 months, and in the 528-month observation is 96 months. The same is true for every other observation area, where in the next 12 months, the frequency of drought events will increase by 2 months. Therefore, it is imperative to make early preparations and implement effective mitigation strategies to reduce the possible impacts of more frequent droughts in the future.
Using the homogeneous Poisson process, the probability value of the expected future frequency of drought can be obtained by substituting the expected value m(t+s) into the non-homogeneous Poisson process based on Eq 20, where m(t) is the expected value at the last time of observation. As mentioned, the power law process is a special case of the non-expected frequency of drought occurrence in the future, as presented in Tables 6–11. For example, if the expected frequency of drought in the next 12 months is 2 months, the probability values based on Tables 6–11 for each region of Timor Island are 0.264 for Kupang City, 0.254 for Kupang, 0.265 for South Central Timor, 0.269 for North Central Timor, 0.266 for Malaka, and 0.267 for Belu. In addition, the probability value of the expected frequency is 1, 3, and 12 months. For example, in Table 7, it is shown that the probability of drought frequency being 3 months in the future 12 months in Kupang City is 0.205. Meanwhile, in Kupang Regency, that probability value is 0.216. This interpretation also applies to each of the following estimates.
Months | n | |||||||||||
1 | 2 | 3 | 4 | 5 | 6 | 7 | 8 | 9 | 10 | 11 | 12 | |
517 (Jan 2024) | 0.160 | |||||||||||
518 (Feb 2024) | 0.263 | 0.051 | ||||||||||
519 (Mar 2024) | 0.325 | 0.094 | 0.018 | |||||||||
520 (Apr 2024) | 0.357 | 0.138 | 0.036 | 0.007 | ||||||||
521 (May 2024) | 0.368 | 0.178 | 0.057 | 0.014 | 0.003 | |||||||
522 (Jun 2024) | 0.364 | 0.211 | 0.082 | 0.024 | 0.006 | 0.001 | ||||||
523 (Jul 2024) | 0.349 | 0.237 | 0.107 | 0.036 | 0.010 | 0.002 | 0.000 | |||||
524 (Aug 2024) | 0.329 | 0.255 | 0.132 | 0.051 | 0.016 | 0.004 | 0.001 | 0.000 | ||||
525 (Sep 2024 | 0.305 | 0.266 | 0.154 | 0.067 | 0.023 | 0.007 | 0.002 | 0.000 | 0.000 | |||
526 (Oct 2024) | 0.279 | 0.270 | 0.175 | 0.085 | 0.033 | 0.011 | 0.003 | 0.001 | 0.000 | 0.000 | ||
527 (Nov 2024) | 0.253 | 0.270 | 0.191 | 0.102 | 0.043 | 0.015 | 0.005 | 0.001 | 0.000 | 0.000 | 0.000 | |
528 (Dec 2024) | 0.227 | 0.264 | 0.205 | 0.119 | 0.055 | 0.021 | 0.007 | 0.002 | 0.001 | 0.000 | 0.000 | 0.000 |
Months | n | |||||||||||
1 | 2 | 3 | 4 | 5 | 6 | 7 | 8 | 9 | 10 | 11 | 12 | |
517 (Jan 2024) | 0.172 | |||||||||||
518 (Feb 2024) | 0.277 | 0.059 | ||||||||||
519 (Mar 2024) | 0.337 | 0.107 | 0.023 | |||||||||
520 (Apr 2024) | 0.363 | 0.154 | 0.043 | 0.009 | ||||||||
521 (May 2024) | 0.367 | 0.194 | 0.069 | 0.018 | 0.004 | |||||||
522 (Jun 2024) | 0.357 | 0.227 | 0.096 | 0.031 | 0.008 | 0.002 | ||||||
523 (Jul 2024) | 0.336 | 0.250 | 0.123 | 0.046 | 0.014 | 0.003 | 0.001 | |||||
524 (Aug 2024) | 0.311 | 0.264 | 0.149 | 0.063 | 0.021 | 0.006 | 0.001 | 0.000 | ||||
525 (Sep 2024 | 0.283 | 0.270 | 0.172 | 0.082 | 0.031 | 0.010 | 0.003 | 0.001 | 0.000 | |||
526 (Oct 2024) | 0.254 | 0.270 | 0.191 | 0.101 | 0.043 | 0.015 | 0.005 | 0.001 | 0.000 | 0.000 | ||
527 (Nov 2024) | 0.226 | 0.264 | 0.205 | 0.120 | 0.056 | 0.022 | 0.007 | 0.002 | 0.001 | 0.000 | 0.000 | |
528 (Dec 2024) | 0.200 | 0.254 | 0.216 | 0.137 | 0.070 | 0.030 | 0.011 | 0.003 | 0.001 | 0.000 | 0.000 | 0.000 |
Months | n | |||||||||||
1 | 2 | 3 | 4 | 5 | 6 | 7 | 8 | 9 | 10 | 11 | 12 | |
517 (Jan 2024) | 0.158 | |||||||||||
518 (Feb 2024) | 0.261 | 0.050 | ||||||||||
519 (Mar 2024) | 0.323 | 0.093 | 0.018 | |||||||||
520 (Apr 2024) | 0.356 | 0.136 | 0.035 | 0.007 | ||||||||
521 (May 2024) | 0.367 | 0.175 | 0.056 | 0.013 | 0.003 | |||||||
522 (Jun 2024) | 0.364 | 0.209 | 0.080 | 0.023 | 0.005 | 0.001 | ||||||
523 (Jul 2024) | 0.351 | 0.235 | 0.105 | 0.035 | 0.009 | 0.002 | 0.000 | |||||
524 (Aug 2024) | 0.331 | 0.253 | 0.129 | 0.049 | 0.015 | 0.004 | 0.001 | 0.000 | ||||
525 (Sep 2024 | 0.308 | 0.265 | 0.152 | 0.065 | 0.022 | 0.006 | 0.002 | 0.000 | 0.000 | |||
526 (Oct 2024) | 0.283 | 0.270 | 0.172 | 0.082 | 0.031 | 0.010 | 0.003 | 0.001 | 0.000 | 0.000 | ||
527 (Nov 2024) | 0.257 | 0.270 | 0.189 | 0.100 | 0.042 | 0.015 | 0.004 | 0.001 | 0.000 | 0.000 | 0.000 | |
528 (Dec 2024) | 0.231 | 0.265 | 0.203 | 0.116 | 0.053 | 0.020 | 0.007 | 0.002 | 0.000 | 0.000 | 0.000 | 0.000 |
Months | n | |||||||||||
1 | 2 | 3 | 4 | 5 | 6 | 7 | 8 | 9 | 10 | 11 | 12 | |
517 (Jan 2024) | 0.149 | |||||||||||
518 (Feb 2024) | 0.250 | 0.045 | ||||||||||
519 (Mar 2024) | 0.313 | 0.084 | 0.015 | |||||||||
520 (Apr 2024) | 0.350 | 0.125 | 0.030 | 0.005 | ||||||||
521 (May 2024) | 0.366 | 0.163 | 0.049 | 0.011 | 0.002 | |||||||
522 (Jun 2024) | 0.367 | 0.197 | 0.070 | 0.019 | 0.004 | 0.001 | ||||||
523 (Jul 2024) | 0.358 | 0.224 | 0.093 | 0.029 | 0.007 | 0.002 | 0.000 | |||||
524 (Aug 2024) | 0.342 | 0.244 | 0.116 | 0.042 | 0.012 | 0.003 | 0.001 | 0.000 | ||||
525 (Sep 2024 | 0.322 | 0.259 | 0.139 | 0.056 | 0.018 | 0.005 | 0.001 | 0.000 | 0.000 | |||
526 (Oct 2024) | 0.299 | 0.267 | 0.159 | 0.071 | 0.025 | 0.008 | 0.002 | 0.000 | 0.000 | 0.000 | ||
527 (Nov 2024) | 0.276 | 0.271 | 0.177 | 0.087 | 0.034 | 0.011 | 0.003 | 0.001 | 0.000 | 0.000 | 0.000 | |
528 (Dec 2024) | 0.251 | 0.269 | 0.192 | 0.103 | 0.044 | 0.016 | 0.005 | 0.001 | 0.000 | 0.000 | 0.000 | 0.000 |
Months | n | |||||||||||
1 | 2 | 3 | 4 | 5 | 6 | 7 | 8 | 9 | 10 | 11 | 12 | |
517 (Jan 2024) | 0.158 | |||||||||||
518 (Feb 2024) | 0.261 | 0.050 | ||||||||||
519 (Mar 2024) | 0.323 | 0.092 | 0.018 | |||||||||
520 (Apr 2024) | 0.356 | 0.136 | 0.035 | 0.007 | ||||||||
521 (May 2024) | 0.367 | 0.175 | 0.056 | 0.013 | 0.003 | |||||||
522 (Jun 2024) | 0.364 | 0.209 | 0.080 | 0.023 | 0.005 | 0.001 | ||||||
523 (Jul 2024) | 0.351 | 0.235 | 0.104 | 0.035 | 0.009 | 0.002 | 0.000 | |||||
524 (Aug 2024) | 0.332 | 0.253 | 0.129 | 0.049 | 0.015 | 0.004 | 0.001 | 0.000 | ||||
525 (Sep 2024 | 0.308 | 0.265 | 0.152 | 0.065 | 0.022 | 0.006 | 0.002 | 0.000 | 0.000 | |||
526 (Oct 2024) | 0.283 | 0.270 | 0.172 | 0.082 | 0.031 | 0.010 | 0.003 | 0.001 | 0.000 | 0.000 | ||
527 (Nov 2024) | 0.257 | 0.270 | 0.189 | 0.099 | 0.042 | 0.015 | 0.004 | 0.001 | 0.000 | 0.000 | 0.000 | |
528 (Dec 2024) | 0.232 | 0.266 | 0.203 | 0.116 | 0.053 | 0.020 | 0.007 | 0.002 | 0.000 | 0.000 | 0.000 | 0.000 |
Months | n | |||||||||||
1 | 2 | 3 | 4 | 5 | 6 | 7 | 8 | 9 | 10 | 11 | 12 | |
517 (Jan 2024) | 0.156 | |||||||||||
518 (Feb 2024) | 0.258 | 0.049 | ||||||||||
519 (Mar 2024) | 0.321 | 0.090 | 0.017 | |||||||||
520 (Apr 2024) | 0.354 | 0.133 | 0.033 | 0.006 | ||||||||
521 (May 2024) | 0.367 | 0.173 | 0.054 | 0.013 | 0.002 | |||||||
522 (Jun 2024) | 0.365 | 0.206 | 0.077 | 0.022 | 0.005 | 0.001 | ||||||
523 (Jul 2024) | 0.353 | 0.232 | 0.102 | 0.034 | 0.009 | 0.002 | 0.000 | |||||
524 (Aug 2024) | 0.334 | 0.251 | 0.126 | 0.047 | 0.014 | 0.004 | 0.001 | 0.000 | ||||
525 (Sep 2024 | 0.311 | 0.264 | 0.149 | 0.063 | 0.021 | 0.006 | 0.001 | 0.000 | ||||
526 (Oct 2024) | 0.287 | 0.270 | 0.169 | 0.080 | 0.030 | 0.009 | 0.003 | 0.001 | 0.000 | |||
527 (Nov 2024) | 0.261 | 0.270 | 0.186 | 0.096 | 0.040 | 0.014 | 0.004 | 0.001 | 0.000 | 0.000 | ||
528 (Dec 2024) | 0.236 | 0.267 | 0.201 | 0.113 | 0.051 | 0.019 | 0.006 | 0.002 | 0.000 | 0.000 | 0.000 | 0.000 |
Note that a limitation of this study lies in the estimation of the power law process model, where the researchers stipulated that the value of the shape parameter (β) should be greater than 1 to indicate an increase in event intensity, as described in Rigdon and Basu’s study [17]. In addition, it should be noted that other modeling approaches or the use of different drought index datasets can be considered. For example, Ghasemi et al. used a Gaussian process regression model to forecast the SPEI drought index [22]; other drought indices, such as the Palmer drought severity index (PDSI) or the Z-Index, may also be used. However, it is important to highlight that future research could focus on modeling and comparing different results. Furthermore, the model used to analyze the SPEI drought index in Timor Island has a wide potential application in cases where extreme events are rare compared to common weaker events, such as in the study of earthquakes, extreme weather, temperature changes, and others.
The analysis of short-term meteorological drought events using SPEI for a 1-month period on Timor Island shows that extremely dry events are less frequent than very dry and dry events. The results of the power law process parameter estimation show a β>1 value in all regions of Timor Island, specifically 1.063 for Kupang City, 1.174 for Kupang Regency, 1.095 for South Central Timor Regency, 1.049 for North Central Timor Regency, 1.034 for Malaka Regency, and 1.112 for Belu Regency. This indicates an increase in drought events in the future. In the next 12 months, the estimated duration of short-term meteorological droughts in all regions is 2 months, with the following probability values: 0.264 for Kupang City, 0.25 for Kupang, 0.265 for South Central Timor, 0.269 for North Central Timor, 0.265 for Malaka, and 0.266 for Belu.
The authors declare they have not used Artificial Intelligence (AI) tools in the creation of this article.
The authors are grateful for financial support from the Directorate General of Higher Education, Ministry of Education and Culture, Research and Technology of Indonesia.
The authors stated that there is no conflict of interest for the study.
[1] | R. Rombach, A. Blattmann, D. Lorenz, P. Esser, B. Ommer, High-resolution image synthesis with latent diffusion models, in Proceedings of the IEEE/CVF Conference on Computer Vision and Pattern Recognition (CVPR), IEEE, (2022), 10684–10695. |
[2] | Y. Cao, S. Li, Y. Liu, Z. Yan, Y. Dai, P. S. Yu, et al., A comprehensive survey of AI-generated content (aigc): A history of generative AI from GAN to ChatGPT, preprint, arXiv: 2303.04226. |
[3] | R. Bommasani, D. A. Hudson, E. Adeli, R. Altman, S. Arora, S. V. Arx, et al., On the opportunities and risks of foundation models, preprint, arXiv: 2108.07258. |
[4] | L. Zhang, A. Rao, M. Agrawala, Adding conditional control to text-to-image diffusion models, in Proceedings of the IEEE/CVF International Conference on Computer Vision (ICCV), IEEE, (2023), 3836–3847. |
[5] | X. Wang, L. Xie, C. Dong, Y. Shan, Real-ESRGAN: Training real-world blind super-resolution with pure synthetic data, in Proceedings of the IEEE/CVF International Conference on Computer Vision Workshops (ICCVW), IEEE, (2021), 1905–1914. |
[6] | H. Jonathan, J. Ajay, A. Pieter, Denoising diffusion probabilistic models, in Advances in Neural Information Processing Systems, Curran Associates, Inc., 33 (2020), 6840–6851. |
[7] | I. J. Goodfellow, J. Pouget-Abadie, M. Mirza, B. Xu, D. Warde-Farley, S. Ozair, et al., Generative adversarial nets, in Advances in Neural Information Processing Systems, Curran Associates, Inc., 27 (2014), 1–9. |
[8] | Y. LeCun, S. Chopra, R. Hadsell, M. Ranzato, F. Huang, A tutorial on energy-based learning, Predict. Struct. Data, 1 (2006), 1–59. |
[9] |
J. Zhou, Z. Wu, Z. Jiang, K. Huang, K. Guo, S. Zhao, Background selection schema on deep learning-based classification of dermatological disease, Comput. Biol. Med., 149 (2022), 105966. https://doi.org/10.1016/j.compbiomed.2022.105966 doi: 10.1016/j.compbiomed.2022.105966
![]() |
[10] |
Q. Su, F. Wang, D. Chen, G. Chen, C. Li, L. Wei, Deep convolutional neural networks with ensemble learning and transfer learning for automated detection of gastrointestinal diseases, Comput. Biol. Med., 150 (2022), 106054. https://doi.org/10.1016/j.compbiomed.2022.106054 doi: 10.1016/j.compbiomed.2022.106054
![]() |
[11] |
G. Liu, Q. Ding, H. Luo, M. Sha, X. Li, M. Ju, Cx22: A new publicly available dataset for deep learning-based segmentation of cervical cytology images, Comput. Biol. Med., 150 (2022), 106194. https://doi.org/10.1016/j.compbiomed.2022.106194 doi: 10.1016/j.compbiomed.2022.106194
![]() |
[12] |
L. Xu, R. Magar, A. B. Farimani, Forecasting COVID-19 new cases using deep learning methods, Comput. Biol. Med., 144 (2022), 105342. https://doi.org/10.1016/j.compbiomed.2022.105342 doi: 10.1016/j.compbiomed.2022.105342
![]() |
[13] | D. P. Kingma, M. Welling, Auto-encoding variational bayes, preprint, arXiv: 1312.6114. |
[14] | A. Radford, J. Wu, R. Child, D. Luan, D. Amodei, I. Sutskever, Language models are unsupervised multitask learners, OpenAI Blog, 1 (2019), 9. |
[15] | H. Huang, P. S. Yu, C. Wang, An introduction to image synthesis with generative adversarial nets, preprint, arXiv: 1803.04469. |
[16] | M. Mirza, S. Osindero, Conditional generative adversarial nets, preprint, arXiv: 1411.1784. |
[17] | L. A. Gatys, A. S. Ecker, M. Bethge, Image style transfer using convolutional neural networks, in 2016 IEEE Conference on Computer Vision and Pattern Recognition (CVPR), IEEE, (2016), 2414–2423. https://doi.org/10.1109/CVPR.2016.265 |
[18] | S. Agarwal, N. Snavely, I. Simon, S. M. Seitz, R. Szeliski, Building rome in a day, in 2009 IEEE 12th International Conference on Computer Vision, IEEE, (2009), 72–79. https://doi.org/10.1109/ICCV.2009.5459148 |
[19] | L. Yang, T. Yendo, M. P. Tehrani, T. Fujii, M. Tanimoto, Probabilistic reliability based view synthesis for FTV, in 2010 IEEE International Conference on Image Processing, IEEE, (2010), 1785–1788. https://doi.org/10.1109/ICIP.2010.5650222 |
[20] |
Y. Zheng, G. Zeng, H. Li, Q. Cai, J. Du, Colorful 3D reconstruction at high resolution using multi-view representation, J. Visual Commun. Image Represent., 85 (2022), 103486. https://doi.org/10.1016/j.jvcir.2022.103486 doi: 10.1016/j.jvcir.2022.103486
![]() |
[21] | J. Deng, W. Dong, R. Socher, L. Li, L. Kai, F. Li, ImageNet: A large-scale hierarchical image database, in 2009 IEEE Conference on Computer Vision and Pattern Recognition, IEEE, (2009), 248–255. https://doi.org/10.1109/CVPR.2009.5206848 |
[22] | S. Christoph, B. Romain, V. Richard, G. Cade, W. Ross, C. Mehdi, et al., Laion-5b: An open large-scale dataset for training next generation image-text models, in Advances in Neural Information Processing Systems, Curran Associates, Inc., 35 (2022), 25278–25294. |
[23] | S. M. Mohammad, S. Kiritchenko, Wikiart emotions: An annotated dataset of emotions evoked by art, in Proceedings of the 11th Edition of the Language Resources and Evaluation Conference (LREC-2018), (2018), 1–14. |
[24] | M. Ben, P. P. Srinivasan, M. Tancik, J. T. Barron, R. Ramamoorthi, R. Ng, NeRF: Representing scenes as Neural Radiance Fields for view synthesis, in European Conference on Computer Vision, Springer, (2020), 405–421. https://doi.org/10.1007/978-3-030-58452-8_24 |
[25] | S. Huang, Q. Li, J. Liao, L. Liu, L. Li, An overview of controllable image synthesis: Current challenges and future trends, SSRN, 2022. |
[26] |
A. Tsirikoglou, G. Eilertsen, J. Unger, A survey of image synthesis methods for visual machine learning, Comput. Graphics Forum, 39 (2020), 426–451. https://doi.org/10.1111/cgf.14047 doi: 10.1111/cgf.14047
![]() |
[27] | H. Ren, G. Stella, B. S. Sami, Controllable GAN synthesis using non-rigid structure-from-motion, in Proceedings of the IEEE/CVF Conference on Computer Vision and Pattern Recognition, IEEE, (2023), 678–687. |
[28] | J. Zhang, A. Siarohin, Y. Liu, H. Tang, N. Sebe, W. Wang, Training and tuning generative neural radiance fields for attribute-conditional 3D-aware face generation, preprint, arXiv: 2208.12550. |
[29] | J. Ko, K. Cho, D. Choi, K. Ryoo, S. Kim, 3D GAN inversion with pose optimization, in Proceedings of the IEEE/CVF Winter Conference on Applications of Computer Vision (WACV), IEEE, (2023), 2967–2976. |
[30] | S. Yang, W. Wang, B. Peng, J. Dong, Designing a 3D-aware StyleNeRF encoder for face editing, in ICASSP 2023–2023 IEEE International Conference on Acoustics, Speech and Signal Processing (ICASSP), IEEE, (2023), 1–5. https://doi.org/10.1109/ICASSP49357.2023.10094932 |
[31] | J. Collins, S. Goel, K. Deng, A. Luthra, L. Xu, E. Gundogdu, et al., ABO: Dataset and benchmarks for real-world 3D object understanding, in Proceedings of the IEEE/CVF Conference on Computer Vision and Pattern Recognition, IEEE, (2022), 21126–21136. |
[32] | B. Yang, Y. Zhang, Y. Xu, Y. Li, H. Zhou, H. Bao, et al., Learning object-compositional Neural Radiance Field for editable scene rendering, in 2021 IEEE/CVF International Conference on Computer Vision (ICCV), IEEE, (2021), 13759–13768. https://doi.org/10.1109/ICCV48922.2021.01352 |
[33] | M. Niemeyer, A. Geiger, GIRAFFE: Representing scenes as compositional generative neural feature fields, in Proceedings of the IEEE/CVF Conference on Computer Vision and Pattern Recognition, IEEE, (2021), 11453–11464. |
[34] | J. Zhu, C. Yang, Y. Shen, Z. Shi, B. Dai, D. Zhao, et al., LinkGAN: Linking GAN latents to pixels for controllable image synthesis, in Proceedings of the IEEE/CVF International Conference on Computer Vision (ICCV), IEEE, (2023), 7656–7666. |
[35] | R. Gross, I. Matthews, J. Cohn, T. Kanade, S. Baker, Multi-PIE, in 2008 8th IEEE International Conference on Automatic Face and Gesture Recognition, IEEE, (2008), 1–8. https://doi.org/10.1109/AFGR.2008.4813399 |
[36] | M. Boss, R. Braun, V. Jampani, J. T. Barron, C. Liu, H. P. A. Lensch, NeRD: Neural reflectance decomposition from image collections, in 2021 IEEE/CVF International Conference on Computer Vision (ICCV), IEEE, (2021), 12664–12674. https://doi.org/10.1109/ICCV48922.2021.01245 |
[37] | X. Yan, Z. Yuan, Y. Du, Y. Liao, Y. Guo, Z. Li, et al., CLEVR3D: Compositional language and elementary visual reasoning for question answering in 3D real-world scenes, preprint, arXiv: 2112.11691. |
[38] | A. Dai, A. X. Chang, M. Savva, M. Halber, T. Funkhouser, M. Nießner, ScanNet: Richly-annotated 3D reconstructions of indoor scenes, in Proceedings of the IEEE/CVF Conference on Computer Vision and Pattern Recognition, IEEE, (2017), 5828–5839. |
[39] |
T. Zhou, T. Richard, F. John, F. Graham, S. Noah, Stereo magnification: Learning view synthesis using multiplane images, ACM Trans. Graphics, 37 (2018), 1–12. https://doi.org/10.1145/3197517.3201323 doi: 10.1145/3197517.3201323
![]() |
[40] | A. X. Chang, T. A. Funkhouser, L. J. Guibas, P. Hanrahan, Q. Huang, Z. Li, et al., ShapeNet: An information-rich 3D model repository, preprint, arXiv: 1512.03012. |
[41] | A. Geiger, P. Lenz, R. Urtasun, Are we ready for autonomous driving? the KITTI vision benchmark suite, in 2012 IEEE Conference on Computer Vision and Pattern Recognition, IEEE, (2012), 3354–3361. https://doi.org/10.1109/CVPR.2012.6248074 |
[42] | H. Caesar, V. Bankiti, A. H. Lang, S. Vora, V. E. Liong, Q. Xu, et al., NuScenes: A multimodal dataset for autonomous driving, in Proceedings of the IEEE/CVF Conference on Computer Vision and Pattern Recognition (CVPR), IEEE, (2020), 11621–11631. |
[43] | S. K. Ramakrishnan, A. Gokaslan, E. Wijmans, O. Maksymets, A. Clegg, J. M. Turner, et al., Habitat-Matterport 3D dataset (HM3D): 1000 large-scale 3D environments for embodied AI, in Thirty-fifth Conference on Neural Information Processing Systems Datasets and Benchmarks Track (Round 2), (2021), 1–12. |
[44] |
D. Scharstein, R. Szeliski, A taxonomy and evaluation of dense two-frame stereo correspondence algorithms, Int. J. Comput. Vision, 47 (2002), 7–42. https://doi.org/10.1023/A:1014573219977 doi: 10.1023/A:1014573219977
![]() |
[45] | D. Scharstein, R. Szeliski, High-accuracy stereo depth maps using structured light, in 2003 IEEE Computer Society Conference on Computer Vision and Pattern Recognition, IEEE, (2003), 1. https://doi.org/10.1109/CVPR.2003.1211354 |
[46] | D. Scharstein, C. Pal, Learning conditional random fields for stereo, in 2007 IEEE Conference on Computer Vision and Pattern Recognition, IEEE, (2007), 1–8. https://doi.org/10.1109/CVPR.2007.383191 |
[47] | H. Hirschmuller, D. Scharstein, Evaluation of cost functions for stereo matching, in 2007 IEEE Conference on Computer Vision and Pattern Recognition, IEEE, (2007), 1–8. https://doi.org/10.1109/CVPR.2007.383248 |
[48] | D. Scharstein, H. Hirschmüller, Y. Kitajima, G. Krathwohl, N. Nesic, X. Wang, et al., High-resolution stereo datasets with subpixel-accurate ground truth, in 36th German Conference on Pattern Recognition, Springer, (2014), 31–42. https://doi.org/10.1007/978-3-319-11752-2_3 |
[49] | N. Silberman, D. Hoiem, K. Pushmeet, R. Fergus, Indoor segmentation and support inference from rgbd images, in Computer Vision–ECCV 2012: 12th European Conference on Computer Vision, Springer, (2012), 746–760. https://doi.org/https://doi.org/10.1007/978-3-642-33715-4_54 |
[50] |
K. Guo, P. Lincoln, P. Davidson, J. Busch, X. Yu, M. Whalen, et al., The Relightables: Volumetric performance capture of humans with realistic relighting, ACM Trans. Graphics, 38 (2019), 1–19. https://doi.org/10.1145/3355089.3356571 doi: 10.1145/3355089.3356571
![]() |
[51] | A. Horé, D. Ziou, Image quality metrics: PSNR vs. SSIM, in 2010 20th International Conference on Pattern Recognition, IEEE, (2010), 2366–2369. https://doi.org/10.1109/ICPR.2010.579 |
[52] |
Z. Wang, A. C. Bovik, H. R. Sheikh, E. P. Simoncelli, Image quality assessment: From error visibility to structural similarity, IEEE Trans. Image Process., 13 (2004), 600–612. https://doi.org/10.1109/TIP.2003.819861 doi: 10.1109/TIP.2003.819861
![]() |
[53] | R. Zhang, P. Isola, A. A. Efros, E. Shechtman, O. Wang, The unreasonable effectiveness of deep features as a perceptual metric, in Proceedings of the IEEE conference on computer vision and pattern recognition, IEEE, (2018), 586–595. |
[54] | T. Salimans, I. Goodfellow, W. Zaremba, V. Cheung, A. Radford, X. Chen, Improved techniques for training GANs, in Advances in Neural Information Processing Systems, Curran Associates, Inc., 29 (2016), 1–9. |
[55] | M. Heusel, H. Ramsauer, T. Unterthiner, B. Nessler, S. Hochreiter, GANs trained by a two time-scale update rule converge to a local nash equilibrium, in Advances in Neural Information Processing Systems, Curran Associates Inc., 30 (2017), 1–12. |
[56] | M. Bińkowski, D. J. Sutherland, M. Arbel, A. Gretton, Demystifying MMD GANs, in International Conference on Learning Representations, 2018. |
[57] | Z. Shi, S. Peng, Y. Xu, Y. Liao, Y. Shen, Deep generative models on 3D representations: A survey, preprint, arXiv: 2210.15663. |
[58] | R. Huang, S. Zhang, T. Li, R. He, Beyond face rotation: Global and local perception GAN for photorealistic and identity preserving frontal view synthesis, in Proceedings of the IEEE International Conference on Computer Vision (ICCV), IEEE, (2017), 2439–2448. |
[59] | B. Zhao, X. Wu, Z. Cheng, H. Liu, Z. Jie, J. Feng, Multi-view image generation from a single-view, in Proceedings of the 26th ACM International Conference on Multimedia, ACM, (2018), 383–391. https://doi.org/10.1145/3240508.3240536 |
[60] | K. Regmi, A. Borji, Cross-view image synthesis using conditional GANs, in 2018 IEEE/CVF Conference on Computer Vision and Pattern Recognition, IEEE, (2018), 3501–3510. https://doi.org/10.1109/CVPR.2018.00369 |
[61] |
K. Regmi, A. Borji, Cross-view image synthesis using geometry-guided conditional GANs, Comput. Vision Image Understanding, 187 (2019), 102788. https://doi.org/10.1016/j.cviu.2019.07.008 doi: 10.1016/j.cviu.2019.07.008
![]() |
[62] | F. Mokhayeri, K. Kamali, E. Granger, Cross-domain face synthesis using a controllable GAN, in 2020 IEEE Winter Conference on Applications of Computer Vision (WACV), IEEE, (2020), 241–249. https://doi.org/10.1109/WACV45572.2020.9093275 |
[63] | X. Zhu, Z. Yin, J. Shi, H. Li, D. Lin, Generative adversarial frontal view to bird view synthesis, in 2018 International Conference on 3D Vision (3DV), IEEE, (2018), 454–463. https://doi.org/10.1109/3DV.2018.00059 |
[64] | H. Ding, S. Wu, H. Tang, F. Wu, G. Gao, X. Jing, Cross-view image synthesis with deformable convolution and attention mechanism, in Pattern Recognition and Computer Vision, Springer, (2020), 386–397. https://doi.org/10.1007/978-3-030-60633-6_32 |
[65] | B. Ren, H. Tang, N. Sebe, Cascaded cross MLP-Mixer GANs for cross-view image translation, in British Machine Vision Conference, (2021), 1–14. |
[66] | J. Zhu, T. Park, P. Isola, A. A. Efros, Unpaired image-to-image translation using cycle-consistent adversarial networks, in 2017 IEEE International Conference on Computer Vision (ICCV), IEEE, (2017), 2242–2251. https://doi.org/10.1109/ICCV.2017.244 |
[67] | M. Yin, L. Sun, Q. Li, Novel view synthesis on unpaired data by conditional deformable variational auto-encoder, in Computer Vision–ECCV 2020, Springer, (2020), 87–103. https://doi.org/10.1007/978-3-030-58604-1_6 |
[68] | X. Shen, J. Plested, Y. Yao, T. Gedeon, Pairwise-GAN: Pose-based view synthesis through pair-wise training, in Neural Information Processing, Springer, (2020), 507–515. https://doi.org/10.1007/978-3-030-63820-7_58 |
[69] | E. R. Chan, M. Monteiro, P. Kellnhofer, J. Wu, G. Wetzstein, pi-GAN: Periodic implicit generative adversarial networks for 3D-aware image synthesis, in 2021 IEEE/CVF Conference on Computer Vision and Pattern Recognition (CVPR), IEEE, (2021), 5795–5805. https://doi.org/10.1109/CVPR46437.2021.00574 |
[70] | S. Cai, A. Obukhov, D. Dai, L. V. Gool, Pix2NeRF: Unsupervised conditional π-GAN for single image to Neural Radiance Fields translation, in 2022 IEEE/CVF Conference on Computer Vision and Pattern Recognition (CVPR), IEEE, (2022), 3971–3980. https://doi.org/10.1109/CVPR52688.2022.00395 |
[71] |
T. Leimkhler, G. Drettakis, FreeStyleGAN, ACM Trans. Graphics, 40 (2021), 1–15. https://doi.org/10.1145/3478513.3480538 doi: 10.1145/3478513.3480538
![]() |
[72] | S. C. Medin, B. Egger, A. Cherian, Y. Wang, J. B. Tenenbaum, X. Liu, et al., MOST-GAN: 3D morphable StyleGAN for disentangled face image manipulation, in Proceedings of the AAAI Conference on Artificial Intelligence, AAAI Press, 36 (2022), 1962–1971. https://doi.org/10.1609/aaai.v36i2.20091 |
[73] | R. Or-El, X. Luo, M. Shan, E. Shechtman, J. J. Park, I. Kemelmacher-Shlizerman, StyleSDF: High-resolution 3D-consistent image and geometry generation, in Proceedings of the IEEE/CVF Conference on Computer Vision and Pattern Recognition (CVPR), IEEE, (2022), 13503–13513. |
[74] |
X. Zheng, Y. Liu, P. Wang, X. Tong, SDF-StyleGAN: Implicit SDF-based StyleGAN for 3D shape generation, Comput. Graphics Forum, 41 (2022), 52–63. https://doi.org/10.1111/cgf.14602 doi: 10.1111/cgf.14602
![]() |
[75] | Y. Deng, J. Yang, J. Xiang, X. Tong, GRAM: Generative radiance manifolds for 3D-aware image generation, in 2022 IEEE/CVF Conference on Computer Vision and Pattern Recognition, IEEE, (2022), 10663–10673. https://doi.org/10.1109/CVPR52688.2022.01041 |
[76] | J. Xiang, J. Yang, Y. Deng, X. Tong, GRAM-HD: 3D-consistent image generation at high resolution with generative radiance manifolds, in Proceedings of the IEEE/CVF International Conference on Computer Vision (ICCV), IEEE, (2023), 2195–2205. |
[77] | E. R. Chan, C. Z. Lin, M. A. Chan, K. Nagano, B. Pan, S. D. Mello, et al., Efficient geometry-aware 3D generative adversarial networks, in Proceedings of the IEEE/CVF Conference on Computer Vision and Pattern Recognition (CVPR), IEEE, (2022), 16123–16133. |
[78] | X. Zhao, F. Ma, D. Güera, Z. Ren, A. G. Schwing, A. Colburn, Generative multiplane images: Making a 2D GAN 3D-aware, in Computer Vision–ECCV 2022, Springer, (2022), 18–35. https://doi.org/10.1007/978-3-031-20065-6_2 |
[79] | H. A. Alhaija, A. Dirik, A. Knrig, S. Fidler, M. Shugrina, XDGAN: Multi-modal 3D shape generation in 2D space, in British Machine Vision Conference, (2022), 1–14. |
[80] | K. Zhang, G. Riegler, N. Snavely, V. Koltun, NeRF++: Analyzing and improving Neural Radiance Fields, preprint, arXiv: 2010.07492. |
[81] | D. Rebain, W. Jiang, S. Yazdani, K. Li, K. M. Yi, A. Tagliasacchi, DeRF: Decomposed radiance fields, in 2021 IEEE/CVF Conference on Computer Vision and Pattern Recognition (CVPR), IEEE, (2021), 14148–14156. https://doi.org/10.1109/CVPR46437.2021.01393 |
[82] | K. Park, U. Sinha, J. T. Barron, S. Bouaziz, D. B. Goldman, S. M. Seitz, et al., Nerfies: Deformable Neural Radiance Fields, in 2021 IEEE/CVF International Conference on Computer Vision (ICCV), IEEE, (2021), 5845–5854. https://doi.org/10.1109/ICCV48922.2021.00581 |
[83] | J. Li, Z. Feng, Q. She, H. Ding, C. Wang, G. H. Lee, MINE: Towards continuous depth MPI with NeRF for novel view synthesis, in 2021 IEEE/CVF International Conference on Computer Vision (ICCV), IEEE, (2021), 12558–12568. https://doi.org/10.1109/ICCV48922.2021.01235 |
[84] |
K. Park, U. Sinha, P. Hedman, J. T. Barron, S. Bouaziz, D. B. Goldman, et al., HyperNeRF: A higher-dimensional representation for topologically varying Neural Radiance Fields, ACM Trans. Graphics, 40 (2021), 1–12. https://doi.org/10.1145/3478513.3480487 doi: 10.1145/3478513.3480487
![]() |
[85] | T. Chen, P. Wang, Z. Fan, Z. Wang, Aug-NeRF: Training stronger Neural Radiance Fields with triple-level physically-grounded augmentations, in 2022 IEEE/CVF Conference on Computer Vision and Pattern Recognition (CVPR), IEEE, (2022), 15170–15181. https://doi.org/10.1109/CVPR52688.2022.01476 |
[86] | T. Kaneko, AR-NeRF: Unsupervised learning of depth and defocus effects from natural images with aperture rendering Neural Radiance Fields, in Proceedings of the IEEE/CVF Conference on Computer Vision and Pattern Recognition (CVPR), IEEE, (2022), 18387–18397. |
[87] | X. Li, C. Hong, Y. Wang, Z. Cao, K. Xian, G. Lin, SymmNeRF: Learning to explore symmetry prior for single-view view synthesis, in Proceedings of the Asian Conference on Computer Vision (ACCV), (2022), 1726–1742. |
[88] | K. Zhou, W. Li, Y. Wang, T. Hu, N. Jiang, X. Han, et al., NeRFLix: High-quality neural view synthesis by learning a degradation-driven inter-viewpoint mixer, in Proceedings of the IEEE/CVF Conference on Computer Vision and Pattern Recognition (CVPR), IEEE, (2023), 12363–12374. |
[89] | Z. Wang, S. Wu, W. Xie, M. Chen, V. A. Prisacariu, NeRF–: Neural Radiance Fields without known camera parameters, preprint, arXiv: 2102.07064. |
[90] |
B. Mildenhall, P. P. Srinivasan, R. Ortiz-Cayon, N. K. Kalantari, R. Ramamoorthi, R. Ng, et al., Local light field fusion: Practical view synthesis with prescriptive sampling guidelines, ACM Trans. Graphics, 38 (2019), 1–14. https://doi.org/10.1145/3306346.3322980 doi: 10.1145/3306346.3322980
![]() |
[91] | Q. Meng, A. Chen, H. Luo, M. Wu, H. Su, L. Xu, et al., GNeRF: GAN-based Neural Radiance Field without posed camera, in Proceedings of the IEEE/CVF International Conference on Computer Vision (ICCV), IEEE, (2021), 6351–6361. |
[92] | R. Jensen, A. Dahl, G. Vogiatzis, E. Tola, H. Aanæs, Large scale multi-view stereopsis evaluation, in Proceedings of the 2014 IEEE Conference on Computer Vision and Pattern Recognition, IEEE, (2014), 406–413. https://doi.org/10.1109/CVPR.2014.59 |
[93] | Y. Jeong, S. Ahn, C. Choy, A. Anandkumar, M. Cho, J. Park, Self-calibrating Neural Radiance Fields, in 2021 IEEE/CVF International Conference on Computer Vision (ICCV), IEEE, (2021), 5826–5834. https://doi.org/10.1109/ICCV48922.2021.00579 |
[94] |
A. Knapitsch, J. Park, Q. Zhou, V. Koltun, Tanks and temples: Benchmarking large-scale scene reconstruction, ACM Trans. Graphics, 36 (2017), 1–13. https://doi.org/10.1145/3072959.3073599 doi: 10.1145/3072959.3073599
![]() |
[95] | W. Bian, Z. Wang, K. Li, J. Bian, V. A. Prisacariu, NoPe-NeRF: Optimising Neural Radiance Field with no pose prior, in Proceedings of the IEEE/CVF Conference on Computer Vision and Pattern Recognition (CVPR), IEEE, (2023), 4160–4169. |
[96] | P. Truong, M. Rakotosaona, F. Manhardt, F. Tombari, SPARF: Neural Radiance Fields from sparse and noisy poses, in Proceedings of the IEEE/CVF Conference on Computer Vision and Pattern Recognition (CVPR), IEEE, (2023), 4190–4200. |
[97] | J. Straub, T. Whelan, L. Ma, Y. Chen, E. Wijmans, S. Green, et al., The replica dataset: A digital replica of indoor spaces, preprint, arXiv: 1906.05797. |
[98] | J. Y. Zhang, G. Yang, S. Tulsiani, D. Ramanan, NeRS: Neural reflectance surfaces for sparse-view 3D reconstruction in the wild, in Conference on Neural Information Processing Systems, Curran Associates, Inc., 34 (2021), 29835–29847. |
[99] | S. Seo, D. Han, Y. Chang, N. Kwak, MixNeRF: Modeling a ray with mixture density for novel view synthesis from sparse inputs, in Proceedings of the IEEE/CVF Conference on Computer Vision and Pattern Recognition (CVPR), IEEE, (2023), 20659–20668. |
[100] | A. Cao, R. D. Charette, SceneRF: Self-supervised monocular 3D scene reconstruction with radiance fields, in Proceedings of the IEEE/CVF International Conference on Computer Vision (ICCV), IEEE, (2023), 9387–9398. |
[101] | J. Behley, M. Garbade, A. Milioto, J. Quenzel, S. Behnke, C. Stachniss, et al., SemanticKITTI: A dataset for semantic scene understanding of lidar sequences, in 2019 IEEE/CVF International Conference on Computer Vision (ICCV), IEEE, (2019), 9296–9306. https://doi.org/10.1109/ICCV.2019.00939 |
[102] | J. Chen, W. Yi, L. Ma, X. Jia, H. Lu, GM-NeRF: Learning generalizable model-based Neural Radiance Fields from multi-view images, in Proceedings of the IEEE/CVF Conference on Computer Vision and Pattern Recognition (CVPR), IEEE, (2023), 20648–20658. |
[103] | T. Yu, Z. Zheng, K. Guo, P. Liu, Q. Dai, Y. Liu, Function4D: Real-time human volumetric capture from very sparse consumer rgbd sensors, in 2021 IEEE/CVF Conference on Computer Vision and Pattern Recognition (CVPR), IEEE, (2021), 5742–5752. https://doi.org/10.1109/CVPR46437.2021.00569 |
[104] | B. Bhatnagar, G. Tiwari, C. Theobalt, G. Pons-Moll, Multi-Garment net: Learning to dress 3D people from images, in 2019 IEEE/CVF International Conference on Computer Vision (ICCV), IEEE, (2019), 5419–5429. https://doi.org/10.1109/ICCV.2019.00552 |
[105] | W. Cheng, S. Xu, J. Piao, C. Qian, W. Wu, K. Lin, et al., Generalizable neural performer: Learning robust radiance fields for human novel view synthesis, preprint, arXiv: 2204.11798. |
[106] | S. Peng, Y. Zhang, Y. Xu, Q. Wang, Q. Shuai, H. Bao, et al., Neural Body: Implicit neural representations with structured latent codes for novel view synthesis of dynamic humans, in 2021 IEEE/CVF Conference on Computer Vision and Pattern Recognition (CVPR), IEEE, (2021), 9050–9059. https://doi.org/10.1109/CVPR46437.2021.00894 |
[107] | B. Mildenhall, P. Hedman, R. Martin-Brualla, P. P. Srinivasan, J. T. Barron, NeRF in the dark: High dynamic range view synthesis from noisy raw images, in Proceedings of the IEEE/CVF Conference on Computer Vision and Pattern Recognition (CVPR), IEEE, (2022), 16190–16199. |
[108] | L. Ma, X. Li, J. Liao, Q. Zhang, X. Wang, J. Wang, et al., Deblur-NeRF: Neural Radiance Fields from blurry images, in Proceedings of the IEEE/CVF Conference on Computer Vision and Pattern Recognition (CVPR), IEEE, (2022), 12861–12870. |
[109] | X. Huang, Q. Zhang, Y. Feng, H. Li, X. Wang, Q. Wang, Hdr-NeRF: High dynamic range Neural Radiance Fields, in Proceedings of the IEEE/CVF Conference on Computer Vision and Pattern Recognition, IEEE, (2022), 18398–18408. |
[110] | P. Naama, T. Tali, K. Simon, NAN: Noise-aware NeRFs for burst-denoising, in Proceedings of the IEEE/CVF Conference on Computer Vision and Pattern Recognition (CVPR), IEEE, (2022), 12672–12681. |
[111] | J. T. Barron, B. Mildenhall, D. Verbin, P. P. Srinivasan, P. Hedman, Mip-NeRF 360: Unbounded anti-aliased Neural Radiance Fields, in Proceedings of the IEEE/CVF Conference on Computer Vision and Pattern Recognition (CVPR), IEEE, (2022), 5470–5479. |
[112] | Y. Xiangli, L. Xu, X. Pan, N. Zhao, A. Rao, C. Theobalt, et al., BungeeNeRF: Progressive Neural Radiance Field for extreme multi-scale scene rendering, in Computer Vision–ECCV 2022, Springer, (2022), 106–122. https://doi.org/10.1007/978-3-031-19824-3_7 |
[113] | Google, Google earth studio, 2018. Available from: https://www.google.com/earth/studio/. |
[114] | M. Tancik, V. Casser, X. Yan, S. Pradhan, B. P. Mildenhall, P. Srinivasan, et al., Block-NeRF: Scalable large scene neural view synthesis, in 2022 IEEE/CVF Conference on Computer Vision and Pattern Recognition (CVPR), IEEE, (2022), 8238–8248. https://doi.org/10.1109/CVPR52688.2022.00807 |
[115] | L. Xu, Y. Xiangli, S. Peng, X. Pan, N. Zhao, C. Theobalt, et al., Grid-guided Neural Radiance Fields for large urban scenes, in Proceedings of the IEEE/CVF Conference on Computer Vision and Pattern Recognition (CVPR), IEEE, (2023), 8296–8306. |
[116] | T. Haithem, R. Deva, S. Mahadev, Mega-NERF: Scalable construction of large-scale NeRFs for virtual fly-throughs, in Proceedings of the IEEE/CVF Conference on Computer Vision and Pattern Recognition (CVPR), IEEE, (2022), 12922–12931. |
[117] | C. Choi, S. M. Kim, Y. M. Kim, Balanced spherical grid for egocentric view synthesis, in Proceedings of the IEEE/CVF Conference on Computer Vision and Pattern Recognition (CVPR), IEEE, (2022), 16590–16599. |
[118] | A. Yu, R. Li, M. Tancik, H. Li, R. Ng, A. Kanazawa, PlenOctrees for real-time rendering of Neural Radiance Fields, in 2021 IEEE/CVF International Conference on Computer Vision (ICCV), IEEE, (2021), 5732–5741. https://doi.org/10.1109/ICCV48922.2021.00570 |
[119] | C. Sun, M. Sun, H. Chen, Direct voxel grid optimization: Super-fast convergence for radiance fields reconstruction, in 2022 IEEE/CVF Conference on Computer Vision and Pattern Recognition (CVPR), IEEE, (2022), 5449–5459. https://doi.org/10.1109/CVPR52688.2022.00538 |
[120] | L. Liu, J. Gu, K. Z. Lin, T. Chua, C. Theobalt, Neural sparse voxel fields, in Advances in Neural Information Processing Systems, Curran Associates, Inc., 33 (2020), 15651–15663. |
[121] | Y. Yao, Z. Luo, S. Li, J. Zhang, Y. Ren, L. Zhou, et al., BlendedMVS: A large-scale dataset for generalized multi-view stereo networks, in Proceedings of the IEEE/CVF Conference on Computer Vision and Pattern Recognition (CVPR), IEEE, (2020), 1790–1799. |
[122] | V. Sitzmann, J. Thies, F. Heide, M. Nießner, G. Wetzstein, M. Zollhöfer, DeepVoxels: Learning persistent 3D feature embeddings, in Proceedings of the IEEE/CVF Conference on Computer Vision and Pattern Recognition (CVPR), IEEE, (2019), 2437–2446. |
[123] | H. Wang, J. Ren, Z. Huang, K. Olszewski, M. Chai, Y. Fu, et al., R2L: Distilling Neural Radiance Field to neural light field for efficient novel view synthesis, in Computer Vision–ECCV 2022, Springer, (2022), 612–629. https://doi.org/10.1007/978-3-031-19821-2_35 |
[124] |
T. Neff, P. Stadlbauer, M. Parger, A. Kurz, J. H. Mueller, C. R. A. Chaitanya, et al., DONeRF: Towards real-time rendering of compact Neural Radiance Fields using depth oracle networks, Comput. Graphics Forum, 40 (2021), 45–59. https://doi.org/10.1111/cgf.14340 doi: 10.1111/cgf.14340
![]() |
[125] | K. Wadhwani, T. Kojima, SqueezeNeRF: Further factorized FastNeRF for memory-efficient inference, in 2022 IEEE/CVF Conference on Computer Vision and Pattern Recognition Workshops (CVPRW), IEEE, (2022), 2716–2724. https://doi.org/10.1109/CVPRW56347.2022.00307 |
[126] | Z. Chen, T. Funkhouser, P. Hedman, A. Tagliasacchi, MobileNeRF: Exploiting the polygon rasterization pipeline for efficient neural field rendering on mobile architectures, in Proceedings of the IEEE/CVF Conference on Computer Vision and Pattern Recognition, IEEE, (2023), 16569–16578. |
[127] | Y. Chen, X. Chen, X. Wang, Q. Zhang, Y. Guo, Y. Shan, et al., Local-to-global registration for bundle-adjusting Neural Radiance Fields, in Proceedings of the IEEE/CVF Conference on Computer Vision and Pattern Recognition, IEEE, (2023), 8264–8273. |
[128] | C. Sbrolli, P. Cudrano, M. Frosi, M. Matteucci, IC3D: Image-conditioned 3D diffusion for shape generation, preprint, arXiv: 2211.10865. |
[129] | J. Gu, Q. Gao, S. Zhai, B. Chen, L. Liu, J. Susskind, Learning controllable 3D diffusion models from single-view images, preprint, arXiv: 2304.06700. |
[130] | T. Anciukevičius, Z. Xu, M. Fisher, P. Henderson, H. Bilen, N. J. Mitra, et al., RenderDiffusion: Image diffusion for 3D reconstruction, inpainting and generation, in Proceedings of the IEEE/CVF Conference on Computer Vision and Pattern Recognition (CVPR), IEEE, (2023), 12608–12618. |
[131] | J. Xiang, J. Yang, B. Huang, X. Tong, 3D-aware image generation using 2D diffusion models, in Proceedings of the IEEE/CVF International Conference on Computer Vision (ICCV), IEEE, (2023), 2383–2393. |
[132] | R. Liu, R. Wu, B. V. Hoorick, P. Tokmakov, S. Zakharov, C. Vondrick, Zero-1-to-3: Zero-shot one image to 3D object, preprint, arXiv: 2303.11328. |
[133] | E. R. Chan, K. Nagano, M. A. Chan, A. W. Bergman, J. J. Park, A. Levy, et al., Generative novel view synthesis with 3D-aware diffusion models, in Proceedings of the IEEE/CVF International Conference on Computer Vision (ICCV), IEEE, (2023), 4217–4229. |
[134] | A. Dosovitskiy, L. Beyer, A. Kolesnikov, D. Weissenborn, X. Zhai, T. Unterthiner, et al., An image is worth 16 x 16 words: Transformers for image recognition at scale, in International Conference on Learning Representations, 2021. |
[135] | A. Vaswani, N. Shazeer, N. Parmar, J. Uszkoreit, L. Jones, A. N. Gomez, et al., Attention is all you need, in Proceedings of the 31st International Conference on Neural Information Processing Systems, Curran Associates Inc., (2017), 6000–6010. |
[136] | P. Nguyen-Ha, L. Huynh, E. Rahtu, J. Heikkila, Sequential view synthesis with transformer, in Proceedings of the Asian Conference on Computer Vision (ACCV), 2020. |
[137] |
J. Yang, Y. Li, L. Yang, Shape transformer nets: Generating viewpoint-invariant 3D shapes from a single image, J. Visual Commun. Image Represent., 81 (2021), 103345. https://doi.org/10.1016/j.jvcir.2021.103345 doi: 10.1016/j.jvcir.2021.103345
![]() |
[138] | J. Kulhánek, E. Derner, T. Sattler, R. Babuška, ViewFormer: NeRF-free neural rendering from few images using transformers, in Computer Vision–ECCV 2022, Springer, (2022), 198–216. https://doi.org/10.1007/978-3-031-19784-0_12 |
[139] | P. Zhou, L. Xie, B. Ni, Q. Tian, CIPS-3D: A 3D-aware generator of GANs based on conditionally-independent pixel synthesis, preprint, arXiv: 2110.09788. |
[140] | X. Xu, X. Pan, D. Lin, B. Dai, Generative occupancy fields for 3D surface-aware image synthesis, in Advances in Neural Information Processing Systems, Curran Associates, Inc., 34 (2021), 20683–20695. |
[141] | Y. Lan, X. Meng, S. Yang, C. C. Loy, B. Dai, Self-supervised geometry-aware encoder for style-based 3D GAN inversion, in Proceedings of the IEEE/CVF Conference on Computer Vision and Pattern Recognition (CVPR), IEEE, (2023), 20940–20949. |
[142] | S. Li, J. van de Weijer, Y. Wang, F. S. Khan, M. Liu, J. Yang, 3D-aware multi-class image-to-image translation with NeRFs, in Proceedings of the IEEE/CVF Conference on Computer Vision and Pattern Recognition (CVPR), IEEE, (2023), 12652–12662. |
[143] | M. Shahbazi, E. Ntavelis, A. Tonioni, E. Collins, D. P. Paudel, M. Danelljan, et al., NeRF-GAN distillation for efficient 3D-aware generation with convolutions, in Proceedings of the IEEE/CVF International Conference on Computer Vision (ICCV) Workshops, IEEE, (2023), 2888–2898. |
[144] | A. Kania, A. Kasymov, M. Ziba, P. Spurek, HyperNeRFGAN: Hypernetwork approach to 3D NeRF GAN, preprint, arXiv: 2301.11631. |
[145] | A. R. Bhattarai, M. Nießner, A. Sevastopolsky, TriPlaneNet: An encoder for EG3D inversion, preprint, arXiv: 2303.13497. |
[146] | N. Müller, Y. Siddiqui, L. Porzi, S. R. Bulo, P. Kontschieder, M. Nießner, Diffrf: Rendering-guided 3D radiance field diffusion, in Proceedings of the IEEE/CVF Conference on Computer Vision and Pattern Recognition, IEEE, (2023), 4328–4338. |
[147] | D. Xu, Y. Jiang, P. Wang, Z. Fan, Y. Wang, Z. Wang, NeuralLift-360: Lifting an in-the-wild 2D photo to a 3D object with 360deg views, in Proceedings of the IEEE/CVF Conference on Computer Vision and Pattern Recognition (CVPR), IEEE, (2023), 4479–4489. |
[148] | H. Chen, J. Gu, A. Chen, W. Tian, Z. Tu, L. Liu, et al., Single-stage diffusion NeRF: A unified approach to 3D generation and reconstruction, in Proceedings of the IEEE/CVF International Conference on Computer Vision (ICCV), IEEE, (2023), 2416–2425. |
[149] | J. Gu, A. Trevithick, K. Lin, J. Susskind, C. Theobalt, L. Liu, et al., NerfDiff: Single-image view synthesis with NeRF-guided distillation from 3D-aware diffusion, in International Conference on Machine Learning, PMLR, (2023), 11808–11826. |
[150] | D. Wang, X. Cui, S. Salcudean, Z. J. Wang, Generalizable Neural Radiance Fields for novel view synthesis with transformer, preprint, arXiv: 2206.05375. |
[151] | K. Lin, L. Yen-Chen, W. Lai, T. Lin, Y. Shih, R. Ramamoorthi, Vision transformer for NeRF-based view synthesis from a single input image, in 2023 IEEE/CVF Winter Conference on Applications of Computer Vision (WACV), IEEE, (2023), 806–815. https://doi.org/10.1109/WACV56688.2023.00087 |
[152] | J. Liu, Q. Nie, Y. Liu, C. Wang, NeRF-Loc: Visual localization with conditional Neural Radiance Field, preprint, arXiv: 2304.07979. |
[153] | Y. Liao, K. Schwarz, L. Mescheder, A. Geiger, Towards unsupervised learning of generative models for 3D controllable image synthesis, in Proceedings of the IEEE/CVF Conference on Computer Vision and Pattern Recognition, IEEE, (2020), 5871–5880. |
[154] | T. Nguyen-Phuoc, C. Richardt, L. Mai, Y. Yang, N. Mitra, BlockGAN: Learning 3D object-aware scene representations from unlabelled images, in Advances in Neural Information Processing Systems, Curran Associates, Inc., 33 (2020), 6767–6778. |
[155] | X. Pan, B. Dai, Z. Liu, C. C. Loy, P. Luo, Do 2D GANs know 3D shape? Unsupervised 3D shape reconstruction from 2D image GANs, in International Conference on Learning Representations, 2021. |
[156] | A. Tewari, M. B. R, X. Pan, O. Fried, M. Agrawala, C. Theobalt, Disentangled3D: Learning a 3D generative model with disentangled geometry and appearance from monocular images, in 2022 IEEE/CVF Conference on Computer Vision and Pattern Recognition (CVPR), IEEE, (2022), 1506–1515. https://doi.org/10.1109/CVPR52688.2022.00157 |
[157] | S. Kobayashi, E. Matsumoto, V. Sitzmann, Decomposing NeRF for editing via feature field distillation, in Advances in Neural Information Processing Systems, Curran Associates, Inc., 35 (2022), 23311–23330. |
[158] | X. Zhang, K. Abhijit, F. Thomas, G. Leonidas, S. Hao, G. Kyle, Nerflets: Local radiance fields for efficient structure-aware 3D scene representation from 2D supervision, in Proceedings of the IEEE/CVF Conference on Computer Vision and Pattern Recognition (CVPR), IEEE, (2023), 8274–8284. |
[159] | C. Zheng, W. Lin, F. Xu, EditableNeRF: Editing topologically varying Neural Radiance Fields by key points, in Proceedings of the IEEE/CVF Conference on Computer Vision and Pattern Recognition (CVPR), IEEE, (2023), 8317–8327. |
[160] |
J. Zhang, L. Yang, MonodepthPlus: Self-supervised monocular depth estimation using soft-attention and learnable outlier-masking, J. Electron. Imaging, 30 (2021), 023017. https://doi.org/10.1117/1.JEI.30.2.023017 doi: 10.1117/1.JEI.30.2.023017
![]() |
[161] | R. Liang, J. Zhang, H. Li, C. Yang, Y. Guan, N. Vijaykumar, SPIDR: SDF-based neural point fields for illumination and deformation, preprint, arXiv: 2210.08398. |
[162] | Y. Zhang, X. Huang, B. Ni, T. Li, W. Zhang, Frequency-modulated point cloud rendering with easy editing, in Proceedings of the IEEE/CVF Conference on Computer Vision and Pattern Recognition (CVPR), IEEE, (2023), 119–129. |
[163] | J. Chen, J. Lyu, Y. Wang, NeuralEditor: Editing Neural Radiance Fields via manipulating point clouds, in Proceedings of the IEEE/CVF Conference on Computer Vision and Pattern Recognition, IEEE, (2023), 12439–12448. |
[164] | J. Zhu, Z. Zhang, C. Zhang, J. Wu, A. Torralba, J. B. Tenenbaum, et al., Visual object networks: Image generation with disentangled 3D representations, in Advances in Neural Information Processing Systems, Curran Associates, Inc., 31 (2018). |
[165] | A. Mirzaei, T. Aumentado-Armstrong, M. A. Brubaker, J. Kelly, A. Levinshtein, K. G. Derpanis, et al., Reference-guided controllable inpainting of Neural Radiance Fields, in Proceedings of the IEEE/CVF International Conference on Computer Vision (ICCV), IEEE, (2023), 17815–17825. |
[166] | Y. Yin, Z. Fu, F. Yang, G. Lin, OR-NeRF: Object removing from 3D scenes guided by multiview segmentation with Neural Radiance Fields, preprint, arXiv: 2305.10503. |
[167] | H. G. Kim, M. Park, S. Lee, S. Kim, Y. M. Ro, Visual comfort aware-reinforcement learning for depth adjustment of stereoscopic 3D images, in Proceedings of the AAAI Conference on Artificial Intelligence, AAAI Press, 35 (2021), 1762–1770. https://doi.org/10.1609/aaai.v35i2.16270 |
[168] | R. Jheng, T. Wu, J. Yeh, W. H. Hsu, Free-form 3D scene inpainting with dual-stream GAN, in British Machine Vision Conference, 2022. |
[169] | Q. Wang, Y. Wang, M. Birsak, P. Wonka, BlobGAN-3D: A spatially-disentangled 3D-aware generative model for indoor scenes, preprint, arXiv: 2303.14706. |
[170] | J. Gu, L. Liu, P. Wang, C. Theobalt, StyleNeRF: A style-based 3D aware generator for high-resolution image synthesis, in Tenth International Conference on Learning Representations, (2022), 1–25. |
[171] | C. Wang, M. Chai, M. He, D. Chen, J. Liao, CLIP-NeRF: Text-and-image driven manipulation of Neural Radiance Fields, in 2022 IEEE/CVF Conference on Computer Vision and Pattern Recognition (CVPR), IEEE, (2022), 3825–3834. https://doi.org/10.1109/CVPR52688.2022.00381 |
[172] | K. Kania, K. M. Yi, M. Kowalski, T. Trzciński, A. Tagliasacchi, CoNeRF: Controllable Neural Radiance Fields, in Proceedings of the IEEE Conference on Computer Vision and Pattern Recognition, IEEE, (2022), 18623–18632. |
[173] | V. Lazova, V. Guzov, K. Olszewski, S. Tulyakov, G. Pons-Moll, Control-NeRF: Editable feature volumes for scene rendering and manipulation, in 2023 IEEE/CVF Winter Conference on Applications of Computer Vision (WACV), IEEE, (2023), 4329–4339. https://doi.org/10.1109/WACV56688.2023.00432 |
[174] | Y. Yuan, Y. Sun, Y. La, Y. Ma, R. Jia, L. Gao, NeRF-Editing: Geometry editing of Neural Radiance Fields, in 2022 IEEE/CVF Conference on Computer Vision and Pattern Recognition (CVPR), IEEE, (2022), 18332–18343. https://doi.org/10.1109/CVPR52688.2022.01781 |
[175] | C. Sun, Y. Liu, J. Han, S. Gould, NeRFEditor: Differentiable style decomposition for full 3D scene editing, preprint, arXiv: 2212.03848. |
[176] |
Z. Wang, Y. Deng, J. Yang, J. Yu, X. Tong, Generative deformable radiance fields for disentangled image synthesis of topology-varying objects, Comput. Graphics Forum, 41 (2022), 431–442. https://doi.org/10.1111/cgf.14689 doi: 10.1111/cgf.14689
![]() |
[177] | K. Tertikas, D. Paschalidou, B. Pan, J. J. Park, M. A. Uy, I. Emiris, et al., PartNeRF: Generating part-aware editable 3D shapes without 3D supervision, preprint, arXiv: 2303.09554. |
[178] | C. Bao, Y. Zhang, B. Yang, T. Fan, Z. Yang, H. Bao, et al., SINE: Semantic-driven image-based NeRF editing with prior-guided editing field, in Proceedings of the IEEE/CVF Conference on Computer Vision and Pattern Recognition, IEEE, (2023), 20919–20929. |
[179] | D. Cohen-Bar, E. Richardson, G. Metzer, R. Giryes, D. Cohen-Or, Set-the-Scene: Global-local training for generating controllable NeRF scenes, in Proceedings of the IEEE/CVF International Conference on Computer Vision (ICCV) Workshops, IEEE, (2023), 2920–2929. |
[180] | A. Mirzaei, T. Aumentado-Armstrong, K. G. Derpanis, J. Kelly, M. A. Brubaker, I. Gilitschenski, et al., SPIn-NeRF: Multiview segmentation and perceptual inpainting with Neural Radiance Fields, in Proceedings of the IEEE/CVF Conference on Computer Vision and Pattern Recognition, IEEE, (2023), 20669–20679. |
[181] | O. Avrahami, D. Lischinski, O. Fried, Blended diffusion for text-driven editing of natural images, in Proceedings of the IEEE/CVF Conference on Computer Vision and Pattern Recognition (CVPR), IEEE, (2022), 18208–18218. |
[182] | A. Nichol, P. Dhariwal, A. Ramesh, P. Shyam, P. Mishkin, B. McGrew, et al., GLIDE: Towards photorealistic image generation and editing with text-guided diffusion models, in Proceedings of the 39th International Conference on Machine Learning, PMLR, (2022), 16784–16804. |
[183] | G. Couairon, J. Verbeek, H. Schwenk, M. Cord, DiffEdit: Diffusion-based semantic image editing with mask guidance, in the Eleventh International Conference on Learning Representations, 2023. |
[184] | E. Sella, G. Fiebelman, P. Hedman, H. Averbuch-Elor, Vox-E: Text-guided voxel editing of 3D objects, in Proceedings of the IEEE/CVF International Conference on Computer Vision (ICCV), IEEE, (2023), 430–440. |
[185] | A. Haque, M. Tancik, A. A. Efros, A. Holynski, A. Kanazawa, Instruct-NeRF2NeRF: Editing 3D scenes with instructions, in Proceedings of the IEEE/CVF International Conference on Computer Vision (ICCV), IEEE, (2023), 19740–19750. |
[186] | Y. Lin, H. Bai, S. Li, H. Lu, X. Lin, H. Xiong, et al., CompoNeRF: Text-guided multi-object compositional NeRF with editable 3D scene layout, preprint, arXiv: 2303.13843. |
[187] | R. Martin-Brualla, N. Radwan, M. S. M. Sajjadi, J. T. Barron, A. Dosovitskiy, D. Duckworth, NeRF in the wild: Neural Radiance Fields for unconstrained photo collections, in 2021 IEEE/CVF Conference on Computer Vision and Pattern Recognition (CVPR), IEEE, (2021), 7206–7215. https://doi.org/10.1109/CVPR46437.2021.00713 |
[188] | M. Boss, A. Engelhardt, A. Kar, Y. Li, D. Sun, J. T. Barron, et al., SAMURAI: Shape and material from unconstrained real-world arbitrary image collections, in Advances in Neural Information Processing Systems, Curran Associates, Inc., 35 (2022), 26389–26403. |
[189] | C. Choi, J. Kim, Y. M. Kim, IBL-NeRF: Image-based lighting formulation of Neural Radiance Fields, preprint, arXiv: 2210.08202. |
[190] | Z. Yan, C. Li, G. H. Lee, NeRF-DS: Neural Radiance Fields for dynamic specular objects, in Proceedings of the IEEE/CVF Conference on Computer Vision and Pattern Recognition (CVPR), IEEE, (2023), 8285–8295. |
[191] |
D. Guo, L. Zhu, S. Ling, T. Li, G. Zhang, Q. Yang, et al., Face illumination normalization based on Generative Adversarial Network, Nat. Comput., 22 (2022), 105–117. https://doi.org/10.1007/s11047-022-09892-4 doi: 10.1007/s11047-022-09892-4
![]() |
[192] | Z. Cui, L. Gu, X. Sun, Y. Qiao, T. Harada, Aleth-NeRF: Low-light condition view synthesis with concealing fields, preprint, arXiv: 2303.05807. |
[193] |
A. R. Nandhini, V. P. D. Raj, Low-light image enhancement based on generative adversarial network, Front. Genet., 12 (2021), 799777. https://doi.org/10.3389/fgene.2021.799777 doi: 10.3389/fgene.2021.799777
![]() |
[194] |
W. Kim, R. Lee, M. Park, S. Lee, Low-light image enhancement based on maximal diffusion values, IEEE Access, 7 (2019), 129150–129163. https://doi.org/10.1109/ACCESS.2019.2940452 doi: 10.1109/ACCESS.2019.2940452
![]() |
[195] | P. Ponglertnapakorn, N. Tritrong, S. Suwajanakorn, DiFaReli: Diffusion face relighting, preprint, arXiv: 2304.09479. |
[196] | M. Guo, A. Fathi, J. Wu, T. Funkhouser, Object-centric neural scene rendering, preprint, arXiv: 2012.08503. |
[197] | Y. Wang, W. Zhou, Z. Lu, H. Li, UDoc-GAN: Unpaired document illumination correction with background light prior, in Proceedings of the 30th ACM International Conference on Multimedia, ACM, (2022), 5074–5082. https://doi.org/10.1145/3503161.3547916 |
[198] | J. Ling, Z. Wang, F. Xu, ShadowNeuS: Neural SDF reconstruction by shadow ray supervision, in Proceedings of the IEEE/CVF Conference on Computer Vision and Pattern Recognition (CVPR), IEEE, (2023), 175–185. |
[199] | V. Rudnev, M. Elgharib, W. Smith, L. Liu, V. Golyanik, C. Theobalt, NeRF for outdoor scene relighting, in Computer Vision–ECCV 2022, Springer, (2022), 615–631. https://doi.org/10.1007/978-3-031-19787-1_35 |
[200] | C. Higuera, B. Boots, M. Mukadam, Learning to read braille: Bridging the tactile reality gap with diffusion models, preprint, arXiv: 2304.01182. |
[201] | T. Guo, D. Kang, L. Bao, Y. He, S. Zhang, NeRFReN: Neural Radiance Fields with reflections, in Proceedings of the IEEE/CVF Conference on Computer Vision and Pattern Recognition (CVPR), IEEE, (2022), 18409–18418. |
[202] | C. LeGendre, W. Ma, G. Fyffe, J. Flynn, L. Charbonnel, J. Busch, et al., DeepLight: Learning illumination for unconstrained mobile mixed reality, in 2019 IEEE/CVF Conference on Computer Vision and Pattern Recognition (CVPR), IEEE, (2019), 5911–5921. https://doi.org/10.1109/CVPR.2019.00607 |
[203] | W. Ye, S. Chen, C. Bao, H. Bao, M. Pollefeys, Z. Cui, et al., IntrinsicNeRF: Learning intrinsic Neural Radiance Fields for editable novel view synthesis, in Proceedings of the IEEE/CVF International Conference on Computer Vision (ICCV), IEEE, (2023), 339–351. |
[204] | M. Boss, V. Jampani, R. Braun, C. Liu, J. T. Barron, H. P. A. Lensch, Neural-PIL: Neural pre-integrated lighting for reflectance decomposition, in Advances in Neural Information Processing Systems, Curran Associates, Inc., 34 (2021), 10691–10704. |
[205] | S. Saito, T. Simon, J. Saragih, H. Joo, PIFuHD: Multi-level pixel-aligned implicit function for high-resolution 3D human digitization, in 2020 IEEE/CVF Conference on Computer Vision and Pattern Recognition (CVPR), IEEE, (2020), 81–90. https://doi.org/10.1109/CVPR42600.2020.00016 |
[206] | H. Tang, S. Bai, L. Zhang, P. H. Torr, N. Sebe, XingGAN for person image generation, in Computer Vision–ECCV 2020, Springer, (2020), 717–734. https://doi.org/10.1007/978-3-030-58595-2_43 |
[207] | Y. Ren, X. Yu, J. Chen, T. H. Li, G. Li, Deep image spatial transformation for person image generation, in 2020 IEEE/CVF Conference on Computer Vision and Pattern Recognition (CVPR), IEEE, (2020), 7687–7696. https://doi.org/10.1109/CVPR42600.2020.00771 |
[208] |
Y. Liu, Z. Qin, T. Wan, Z. Luo, Auto-painter: Cartoon image generation from sketch by using conditional wasserstein generative adversarial networks, Neurocomputing, 311 (2018), 78–87. https://doi.org/10.1016/j.neucom.2018.05.045 doi: 10.1016/j.neucom.2018.05.045
![]() |
[209] | H. Li, AI synthesis for the metaverse: From avatars to 3D scenes, Stanford University, Stanford Talks, 2022. Available from: https://talks.stanford.edu/hao-li-pinscreen-on-ai-synthesis-for-the-metaverse-from-avatars-to-3d-scenes/. |
[210] | S. Murray, A. Tallon, Mapping gothic france, Columbia University, Media Center for Art History, 2023. Available from: https://mcid.mcah.columbia.edu/art-atlas/mapping-gothic. |
[211] | Y. Xiang, C. Lv, Q. Liu, X. Yang, B. Liu, M. Ju, A creative industry image generation dataset based on captions, preprint, arXiv: 2211.09035. |
[212] | C. Tatsch, J. A. Bredu, D. Covell, I. B. Tulu, Y. Gu, Rhino: An autonomous robot for mapping underground mine environments, in 2023 IEEE/ASME International Conference on Advanced Intelligent Mechatronics (AIM), IEEE, (2023), 1166–1173. https://doi.org/10.1109/AIM46323.2023.10196202 |
[213] | Y. Tian, L. Li, A. Fumagalli, Y. Tadesse, B. Prabhakaran, Haptic-enabled mixed reality system for mixed-initiative remote robot control, preprint, arXiv: 2102.03521. |
[214] |
G. Pu, Y. Men, Y. Mao, Y. Jiang, W. Ma, Z. Lian, Controllable image synthesis with attribute-decomposed GAN, IEEE Trans. Pattern Anal. Mach. Intell., 45 (2023), 1514–1532. https://doi.org/10.1109/TPAMI.2022.3161985 doi: 10.1109/TPAMI.2022.3161985
![]() |
[215] |
X. Wu, Y. Zhang, Q. Li, Y. Qi, J. Wang, Y. Guo, Face aging with pixel-level alignment GAN, Appl. Intell., 52 (2022), 14665–14678. https://doi.org/10.1007/s10489-022-03541-0 doi: 10.1007/s10489-022-03541-0
![]() |
[216] |
D. Sero, A. Zaidi, J. Li, J. D. White, T. B. G. Zarzar, M. L. Marazita, et al., Facial recognition from dna using face-to-dna classifiers, Nat. Commun., 10 (2019), 1. https://doi.org/10.1038/s41467-018-07882-8 doi: 10.1038/s41467-018-07882-8
![]() |
[217] | M. Nicolae, M. Sinn, M. Tran, B. Buesser, A. Rawat, M. Wistuba, et al., Adversarial robustness toolbox v1.0.0, 2018. Available from: https://github.com/Trusted-AI/adversarial-robustness-toolbox. |
1. | Nurtiti Sunusi, Nur Hikmah Auliana, Assessing SPI and SPEI for Drought Forecasting through the Power Law Process: A Case Study in South Sulawesi, Indonesia, 2025, 22150161, 103235, 10.1016/j.mex.2025.103235 |
SPEI value | Classification |
≥2.00 | Extremely wet |
1.50–1.99 | Very wet |
1.00–1.49 | Moderately wet |
(–0.99)–0.99 | Normal |
(–1.00)–(–1.49) | Moderately dry |
(–1.50)–(–1.99) | Severely dry |
≤ –2.00 | Extremely dry |
Observation area | Extremely dry index | Longest drought duration | Intensity of drought months by drought level | ||||
Value | Month of incident | Moderately dry | Severely dry | Extremely dry | Total | ||
Kupang City | –2.47 | August 1988 | 4 months | 63 months | 25 months | 6 months | 94 months |
Kupang | –2.74 | April 2016 | 4 months | 62 months | 26 months | 5 months | 93 months |
South Central Timor | –3.86 | August 1998 | 4 months | 59 months | 25 months | 6 months | 90 months |
North Central Timor | –2.61 | August 1998 | 4 months | 62 months | 20 months | 6 months | 88 months |
Malaka | –2.82 | August 2010 | 5 months | 66 months | 25 months | 4 months | 95 months |
Belu | –3.07 | August 2010 | 4 months | 59 months | 23 months | 5 months | 87 months |
Observation area | C2R | Critical value | Decision |
Kupang City | 0.082 | 0.22 | H0 accepted |
Kupang | 0.062 | 0.22 | H0 accepted |
South Central Timor | 0.089 | 0.22 | H0 accepted |
North Central Timor | 0.171 | 0.22 | H0 accepted |
Malaka | 0.043 | 0.22 | H0 accepted |
Belu | 0.022 | 0.22 | H0 accepted |
Observation area | Parameter | |
ˆβ | ˆγ | |
Kupang City | 1.063 | 7.170 |
Kupang | 1.174 | 10.859 |
South Central Timor | 1.095 | 8.481 |
North Central Timor | 1.049 | 7.245 |
Malaka | 1.034 | 6.296 |
Belu | 1.112 | 9.282 |
Months | Kupang City | Kupang | South Central Timor | Nort Central Timor | Malaka | Belu |
517 (Jan 2024) | 94.207 | 93.244 | 90.195 | 87.957 | 95.393 | 87.374 |
518 (Feb 2024) | 94.400 | 93.456 | 90.386 | 88.136 | 95.584 | 87.562 |
519 (Mar 2024) | 94.594 | 93.668 | 90.577 | 88.314 | 95.775 | 87.750 |
520 (Apr 2024) | 94.788 | 93.879 | 90.768 | 88.493 | 95.965 | 87.938 |
521 (May 2024) | 94.981 | 94.091 | 90.959 | 88.671 | 96.156 | 88.126 |
522 (Jun 2024) | 95.175 | 94.304 | 91.150 | 88.850 | 96.347 | 88.315 |
523 (Jul 2024) | 95.369 | 94.516 | 91.342 | 89.028 | 96.538 | 88.503 |
524 (Aug 2024) | 95.563 | 94.728 | 91.533 | 89.207 | 96.729 | 88.691 |
525 (Sep 2024 | 95.756 | 94.940 | 91.724 | 89.385 | 96.920 | 88.879 |
526 (Oct 2024) | 95.950 | 95.152 | 91.916 | 89.564 | 97.111 | 89.067 |
527 (Nov 2024) | 96.144 | 95.365 | 92.107 | 89.743 | 97.301 | 89.256 |
528 (Dec 2024) | 96.338 | 95.577 | 92.299 | 89.921 | 97.492 | 89.444 |
Months | n | |||||||||||
1 | 2 | 3 | 4 | 5 | 6 | 7 | 8 | 9 | 10 | 11 | 12 | |
517 (Jan 2024) | 0.160 | |||||||||||
518 (Feb 2024) | 0.263 | 0.051 | ||||||||||
519 (Mar 2024) | 0.325 | 0.094 | 0.018 | |||||||||
520 (Apr 2024) | 0.357 | 0.138 | 0.036 | 0.007 | ||||||||
521 (May 2024) | 0.368 | 0.178 | 0.057 | 0.014 | 0.003 | |||||||
522 (Jun 2024) | 0.364 | 0.211 | 0.082 | 0.024 | 0.006 | 0.001 | ||||||
523 (Jul 2024) | 0.349 | 0.237 | 0.107 | 0.036 | 0.010 | 0.002 | 0.000 | |||||
524 (Aug 2024) | 0.329 | 0.255 | 0.132 | 0.051 | 0.016 | 0.004 | 0.001 | 0.000 | ||||
525 (Sep 2024 | 0.305 | 0.266 | 0.154 | 0.067 | 0.023 | 0.007 | 0.002 | 0.000 | 0.000 | |||
526 (Oct 2024) | 0.279 | 0.270 | 0.175 | 0.085 | 0.033 | 0.011 | 0.003 | 0.001 | 0.000 | 0.000 | ||
527 (Nov 2024) | 0.253 | 0.270 | 0.191 | 0.102 | 0.043 | 0.015 | 0.005 | 0.001 | 0.000 | 0.000 | 0.000 | |
528 (Dec 2024) | 0.227 | 0.264 | 0.205 | 0.119 | 0.055 | 0.021 | 0.007 | 0.002 | 0.001 | 0.000 | 0.000 | 0.000 |
Months | n | |||||||||||
1 | 2 | 3 | 4 | 5 | 6 | 7 | 8 | 9 | 10 | 11 | 12 | |
517 (Jan 2024) | 0.172 | |||||||||||
518 (Feb 2024) | 0.277 | 0.059 | ||||||||||
519 (Mar 2024) | 0.337 | 0.107 | 0.023 | |||||||||
520 (Apr 2024) | 0.363 | 0.154 | 0.043 | 0.009 | ||||||||
521 (May 2024) | 0.367 | 0.194 | 0.069 | 0.018 | 0.004 | |||||||
522 (Jun 2024) | 0.357 | 0.227 | 0.096 | 0.031 | 0.008 | 0.002 | ||||||
523 (Jul 2024) | 0.336 | 0.250 | 0.123 | 0.046 | 0.014 | 0.003 | 0.001 | |||||
524 (Aug 2024) | 0.311 | 0.264 | 0.149 | 0.063 | 0.021 | 0.006 | 0.001 | 0.000 | ||||
525 (Sep 2024 | 0.283 | 0.270 | 0.172 | 0.082 | 0.031 | 0.010 | 0.003 | 0.001 | 0.000 | |||
526 (Oct 2024) | 0.254 | 0.270 | 0.191 | 0.101 | 0.043 | 0.015 | 0.005 | 0.001 | 0.000 | 0.000 | ||
527 (Nov 2024) | 0.226 | 0.264 | 0.205 | 0.120 | 0.056 | 0.022 | 0.007 | 0.002 | 0.001 | 0.000 | 0.000 | |
528 (Dec 2024) | 0.200 | 0.254 | 0.216 | 0.137 | 0.070 | 0.030 | 0.011 | 0.003 | 0.001 | 0.000 | 0.000 | 0.000 |
Months | n | |||||||||||
1 | 2 | 3 | 4 | 5 | 6 | 7 | 8 | 9 | 10 | 11 | 12 | |
517 (Jan 2024) | 0.158 | |||||||||||
518 (Feb 2024) | 0.261 | 0.050 | ||||||||||
519 (Mar 2024) | 0.323 | 0.093 | 0.018 | |||||||||
520 (Apr 2024) | 0.356 | 0.136 | 0.035 | 0.007 | ||||||||
521 (May 2024) | 0.367 | 0.175 | 0.056 | 0.013 | 0.003 | |||||||
522 (Jun 2024) | 0.364 | 0.209 | 0.080 | 0.023 | 0.005 | 0.001 | ||||||
523 (Jul 2024) | 0.351 | 0.235 | 0.105 | 0.035 | 0.009 | 0.002 | 0.000 | |||||
524 (Aug 2024) | 0.331 | 0.253 | 0.129 | 0.049 | 0.015 | 0.004 | 0.001 | 0.000 | ||||
525 (Sep 2024 | 0.308 | 0.265 | 0.152 | 0.065 | 0.022 | 0.006 | 0.002 | 0.000 | 0.000 | |||
526 (Oct 2024) | 0.283 | 0.270 | 0.172 | 0.082 | 0.031 | 0.010 | 0.003 | 0.001 | 0.000 | 0.000 | ||
527 (Nov 2024) | 0.257 | 0.270 | 0.189 | 0.100 | 0.042 | 0.015 | 0.004 | 0.001 | 0.000 | 0.000 | 0.000 | |
528 (Dec 2024) | 0.231 | 0.265 | 0.203 | 0.116 | 0.053 | 0.020 | 0.007 | 0.002 | 0.000 | 0.000 | 0.000 | 0.000 |
Months | n | |||||||||||
1 | 2 | 3 | 4 | 5 | 6 | 7 | 8 | 9 | 10 | 11 | 12 | |
517 (Jan 2024) | 0.149 | |||||||||||
518 (Feb 2024) | 0.250 | 0.045 | ||||||||||
519 (Mar 2024) | 0.313 | 0.084 | 0.015 | |||||||||
520 (Apr 2024) | 0.350 | 0.125 | 0.030 | 0.005 | ||||||||
521 (May 2024) | 0.366 | 0.163 | 0.049 | 0.011 | 0.002 | |||||||
522 (Jun 2024) | 0.367 | 0.197 | 0.070 | 0.019 | 0.004 | 0.001 | ||||||
523 (Jul 2024) | 0.358 | 0.224 | 0.093 | 0.029 | 0.007 | 0.002 | 0.000 | |||||
524 (Aug 2024) | 0.342 | 0.244 | 0.116 | 0.042 | 0.012 | 0.003 | 0.001 | 0.000 | ||||
525 (Sep 2024 | 0.322 | 0.259 | 0.139 | 0.056 | 0.018 | 0.005 | 0.001 | 0.000 | 0.000 | |||
526 (Oct 2024) | 0.299 | 0.267 | 0.159 | 0.071 | 0.025 | 0.008 | 0.002 | 0.000 | 0.000 | 0.000 | ||
527 (Nov 2024) | 0.276 | 0.271 | 0.177 | 0.087 | 0.034 | 0.011 | 0.003 | 0.001 | 0.000 | 0.000 | 0.000 | |
528 (Dec 2024) | 0.251 | 0.269 | 0.192 | 0.103 | 0.044 | 0.016 | 0.005 | 0.001 | 0.000 | 0.000 | 0.000 | 0.000 |
Months | n | |||||||||||
1 | 2 | 3 | 4 | 5 | 6 | 7 | 8 | 9 | 10 | 11 | 12 | |
517 (Jan 2024) | 0.158 | |||||||||||
518 (Feb 2024) | 0.261 | 0.050 | ||||||||||
519 (Mar 2024) | 0.323 | 0.092 | 0.018 | |||||||||
520 (Apr 2024) | 0.356 | 0.136 | 0.035 | 0.007 | ||||||||
521 (May 2024) | 0.367 | 0.175 | 0.056 | 0.013 | 0.003 | |||||||
522 (Jun 2024) | 0.364 | 0.209 | 0.080 | 0.023 | 0.005 | 0.001 | ||||||
523 (Jul 2024) | 0.351 | 0.235 | 0.104 | 0.035 | 0.009 | 0.002 | 0.000 | |||||
524 (Aug 2024) | 0.332 | 0.253 | 0.129 | 0.049 | 0.015 | 0.004 | 0.001 | 0.000 | ||||
525 (Sep 2024 | 0.308 | 0.265 | 0.152 | 0.065 | 0.022 | 0.006 | 0.002 | 0.000 | 0.000 | |||
526 (Oct 2024) | 0.283 | 0.270 | 0.172 | 0.082 | 0.031 | 0.010 | 0.003 | 0.001 | 0.000 | 0.000 | ||
527 (Nov 2024) | 0.257 | 0.270 | 0.189 | 0.099 | 0.042 | 0.015 | 0.004 | 0.001 | 0.000 | 0.000 | 0.000 | |
528 (Dec 2024) | 0.232 | 0.266 | 0.203 | 0.116 | 0.053 | 0.020 | 0.007 | 0.002 | 0.000 | 0.000 | 0.000 | 0.000 |
Months | n | |||||||||||
1 | 2 | 3 | 4 | 5 | 6 | 7 | 8 | 9 | 10 | 11 | 12 | |
517 (Jan 2024) | 0.156 | |||||||||||
518 (Feb 2024) | 0.258 | 0.049 | ||||||||||
519 (Mar 2024) | 0.321 | 0.090 | 0.017 | |||||||||
520 (Apr 2024) | 0.354 | 0.133 | 0.033 | 0.006 | ||||||||
521 (May 2024) | 0.367 | 0.173 | 0.054 | 0.013 | 0.002 | |||||||
522 (Jun 2024) | 0.365 | 0.206 | 0.077 | 0.022 | 0.005 | 0.001 | ||||||
523 (Jul 2024) | 0.353 | 0.232 | 0.102 | 0.034 | 0.009 | 0.002 | 0.000 | |||||
524 (Aug 2024) | 0.334 | 0.251 | 0.126 | 0.047 | 0.014 | 0.004 | 0.001 | 0.000 | ||||
525 (Sep 2024 | 0.311 | 0.264 | 0.149 | 0.063 | 0.021 | 0.006 | 0.001 | 0.000 | ||||
526 (Oct 2024) | 0.287 | 0.270 | 0.169 | 0.080 | 0.030 | 0.009 | 0.003 | 0.001 | 0.000 | |||
527 (Nov 2024) | 0.261 | 0.270 | 0.186 | 0.096 | 0.040 | 0.014 | 0.004 | 0.001 | 0.000 | 0.000 | ||
528 (Dec 2024) | 0.236 | 0.267 | 0.201 | 0.113 | 0.051 | 0.019 | 0.006 | 0.002 | 0.000 | 0.000 | 0.000 | 0.000 |
SPEI value | Classification |
≥2.00 | Extremely wet |
1.50–1.99 | Very wet |
1.00–1.49 | Moderately wet |
(–0.99)–0.99 | Normal |
(–1.00)–(–1.49) | Moderately dry |
(–1.50)–(–1.99) | Severely dry |
≤ –2.00 | Extremely dry |
Observation area | Extremely dry index | Longest drought duration | Intensity of drought months by drought level | ||||
Value | Month of incident | Moderately dry | Severely dry | Extremely dry | Total | ||
Kupang City | –2.47 | August 1988 | 4 months | 63 months | 25 months | 6 months | 94 months |
Kupang | –2.74 | April 2016 | 4 months | 62 months | 26 months | 5 months | 93 months |
South Central Timor | –3.86 | August 1998 | 4 months | 59 months | 25 months | 6 months | 90 months |
North Central Timor | –2.61 | August 1998 | 4 months | 62 months | 20 months | 6 months | 88 months |
Malaka | –2.82 | August 2010 | 5 months | 66 months | 25 months | 4 months | 95 months |
Belu | –3.07 | August 2010 | 4 months | 59 months | 23 months | 5 months | 87 months |
Observation area | C2R | Critical value | Decision |
Kupang City | 0.082 | 0.22 | H0 accepted |
Kupang | 0.062 | 0.22 | H0 accepted |
South Central Timor | 0.089 | 0.22 | H0 accepted |
North Central Timor | 0.171 | 0.22 | H0 accepted |
Malaka | 0.043 | 0.22 | H0 accepted |
Belu | 0.022 | 0.22 | H0 accepted |
Observation area | Parameter | |
ˆβ | ˆγ | |
Kupang City | 1.063 | 7.170 |
Kupang | 1.174 | 10.859 |
South Central Timor | 1.095 | 8.481 |
North Central Timor | 1.049 | 7.245 |
Malaka | 1.034 | 6.296 |
Belu | 1.112 | 9.282 |
Months | Kupang City | Kupang | South Central Timor | Nort Central Timor | Malaka | Belu |
517 (Jan 2024) | 94.207 | 93.244 | 90.195 | 87.957 | 95.393 | 87.374 |
518 (Feb 2024) | 94.400 | 93.456 | 90.386 | 88.136 | 95.584 | 87.562 |
519 (Mar 2024) | 94.594 | 93.668 | 90.577 | 88.314 | 95.775 | 87.750 |
520 (Apr 2024) | 94.788 | 93.879 | 90.768 | 88.493 | 95.965 | 87.938 |
521 (May 2024) | 94.981 | 94.091 | 90.959 | 88.671 | 96.156 | 88.126 |
522 (Jun 2024) | 95.175 | 94.304 | 91.150 | 88.850 | 96.347 | 88.315 |
523 (Jul 2024) | 95.369 | 94.516 | 91.342 | 89.028 | 96.538 | 88.503 |
524 (Aug 2024) | 95.563 | 94.728 | 91.533 | 89.207 | 96.729 | 88.691 |
525 (Sep 2024 | 95.756 | 94.940 | 91.724 | 89.385 | 96.920 | 88.879 |
526 (Oct 2024) | 95.950 | 95.152 | 91.916 | 89.564 | 97.111 | 89.067 |
527 (Nov 2024) | 96.144 | 95.365 | 92.107 | 89.743 | 97.301 | 89.256 |
528 (Dec 2024) | 96.338 | 95.577 | 92.299 | 89.921 | 97.492 | 89.444 |
Months | n | |||||||||||
1 | 2 | 3 | 4 | 5 | 6 | 7 | 8 | 9 | 10 | 11 | 12 | |
517 (Jan 2024) | 0.160 | |||||||||||
518 (Feb 2024) | 0.263 | 0.051 | ||||||||||
519 (Mar 2024) | 0.325 | 0.094 | 0.018 | |||||||||
520 (Apr 2024) | 0.357 | 0.138 | 0.036 | 0.007 | ||||||||
521 (May 2024) | 0.368 | 0.178 | 0.057 | 0.014 | 0.003 | |||||||
522 (Jun 2024) | 0.364 | 0.211 | 0.082 | 0.024 | 0.006 | 0.001 | ||||||
523 (Jul 2024) | 0.349 | 0.237 | 0.107 | 0.036 | 0.010 | 0.002 | 0.000 | |||||
524 (Aug 2024) | 0.329 | 0.255 | 0.132 | 0.051 | 0.016 | 0.004 | 0.001 | 0.000 | ||||
525 (Sep 2024 | 0.305 | 0.266 | 0.154 | 0.067 | 0.023 | 0.007 | 0.002 | 0.000 | 0.000 | |||
526 (Oct 2024) | 0.279 | 0.270 | 0.175 | 0.085 | 0.033 | 0.011 | 0.003 | 0.001 | 0.000 | 0.000 | ||
527 (Nov 2024) | 0.253 | 0.270 | 0.191 | 0.102 | 0.043 | 0.015 | 0.005 | 0.001 | 0.000 | 0.000 | 0.000 | |
528 (Dec 2024) | 0.227 | 0.264 | 0.205 | 0.119 | 0.055 | 0.021 | 0.007 | 0.002 | 0.001 | 0.000 | 0.000 | 0.000 |
Months | n | |||||||||||
1 | 2 | 3 | 4 | 5 | 6 | 7 | 8 | 9 | 10 | 11 | 12 | |
517 (Jan 2024) | 0.172 | |||||||||||
518 (Feb 2024) | 0.277 | 0.059 | ||||||||||
519 (Mar 2024) | 0.337 | 0.107 | 0.023 | |||||||||
520 (Apr 2024) | 0.363 | 0.154 | 0.043 | 0.009 | ||||||||
521 (May 2024) | 0.367 | 0.194 | 0.069 | 0.018 | 0.004 | |||||||
522 (Jun 2024) | 0.357 | 0.227 | 0.096 | 0.031 | 0.008 | 0.002 | ||||||
523 (Jul 2024) | 0.336 | 0.250 | 0.123 | 0.046 | 0.014 | 0.003 | 0.001 | |||||
524 (Aug 2024) | 0.311 | 0.264 | 0.149 | 0.063 | 0.021 | 0.006 | 0.001 | 0.000 | ||||
525 (Sep 2024 | 0.283 | 0.270 | 0.172 | 0.082 | 0.031 | 0.010 | 0.003 | 0.001 | 0.000 | |||
526 (Oct 2024) | 0.254 | 0.270 | 0.191 | 0.101 | 0.043 | 0.015 | 0.005 | 0.001 | 0.000 | 0.000 | ||
527 (Nov 2024) | 0.226 | 0.264 | 0.205 | 0.120 | 0.056 | 0.022 | 0.007 | 0.002 | 0.001 | 0.000 | 0.000 | |
528 (Dec 2024) | 0.200 | 0.254 | 0.216 | 0.137 | 0.070 | 0.030 | 0.011 | 0.003 | 0.001 | 0.000 | 0.000 | 0.000 |
Months | n | |||||||||||
1 | 2 | 3 | 4 | 5 | 6 | 7 | 8 | 9 | 10 | 11 | 12 | |
517 (Jan 2024) | 0.158 | |||||||||||
518 (Feb 2024) | 0.261 | 0.050 | ||||||||||
519 (Mar 2024) | 0.323 | 0.093 | 0.018 | |||||||||
520 (Apr 2024) | 0.356 | 0.136 | 0.035 | 0.007 | ||||||||
521 (May 2024) | 0.367 | 0.175 | 0.056 | 0.013 | 0.003 | |||||||
522 (Jun 2024) | 0.364 | 0.209 | 0.080 | 0.023 | 0.005 | 0.001 | ||||||
523 (Jul 2024) | 0.351 | 0.235 | 0.105 | 0.035 | 0.009 | 0.002 | 0.000 | |||||
524 (Aug 2024) | 0.331 | 0.253 | 0.129 | 0.049 | 0.015 | 0.004 | 0.001 | 0.000 | ||||
525 (Sep 2024 | 0.308 | 0.265 | 0.152 | 0.065 | 0.022 | 0.006 | 0.002 | 0.000 | 0.000 | |||
526 (Oct 2024) | 0.283 | 0.270 | 0.172 | 0.082 | 0.031 | 0.010 | 0.003 | 0.001 | 0.000 | 0.000 | ||
527 (Nov 2024) | 0.257 | 0.270 | 0.189 | 0.100 | 0.042 | 0.015 | 0.004 | 0.001 | 0.000 | 0.000 | 0.000 | |
528 (Dec 2024) | 0.231 | 0.265 | 0.203 | 0.116 | 0.053 | 0.020 | 0.007 | 0.002 | 0.000 | 0.000 | 0.000 | 0.000 |
Months | n | |||||||||||
1 | 2 | 3 | 4 | 5 | 6 | 7 | 8 | 9 | 10 | 11 | 12 | |
517 (Jan 2024) | 0.149 | |||||||||||
518 (Feb 2024) | 0.250 | 0.045 | ||||||||||
519 (Mar 2024) | 0.313 | 0.084 | 0.015 | |||||||||
520 (Apr 2024) | 0.350 | 0.125 | 0.030 | 0.005 | ||||||||
521 (May 2024) | 0.366 | 0.163 | 0.049 | 0.011 | 0.002 | |||||||
522 (Jun 2024) | 0.367 | 0.197 | 0.070 | 0.019 | 0.004 | 0.001 | ||||||
523 (Jul 2024) | 0.358 | 0.224 | 0.093 | 0.029 | 0.007 | 0.002 | 0.000 | |||||
524 (Aug 2024) | 0.342 | 0.244 | 0.116 | 0.042 | 0.012 | 0.003 | 0.001 | 0.000 | ||||
525 (Sep 2024 | 0.322 | 0.259 | 0.139 | 0.056 | 0.018 | 0.005 | 0.001 | 0.000 | 0.000 | |||
526 (Oct 2024) | 0.299 | 0.267 | 0.159 | 0.071 | 0.025 | 0.008 | 0.002 | 0.000 | 0.000 | 0.000 | ||
527 (Nov 2024) | 0.276 | 0.271 | 0.177 | 0.087 | 0.034 | 0.011 | 0.003 | 0.001 | 0.000 | 0.000 | 0.000 | |
528 (Dec 2024) | 0.251 | 0.269 | 0.192 | 0.103 | 0.044 | 0.016 | 0.005 | 0.001 | 0.000 | 0.000 | 0.000 | 0.000 |
Months | n | |||||||||||
1 | 2 | 3 | 4 | 5 | 6 | 7 | 8 | 9 | 10 | 11 | 12 | |
517 (Jan 2024) | 0.158 | |||||||||||
518 (Feb 2024) | 0.261 | 0.050 | ||||||||||
519 (Mar 2024) | 0.323 | 0.092 | 0.018 | |||||||||
520 (Apr 2024) | 0.356 | 0.136 | 0.035 | 0.007 | ||||||||
521 (May 2024) | 0.367 | 0.175 | 0.056 | 0.013 | 0.003 | |||||||
522 (Jun 2024) | 0.364 | 0.209 | 0.080 | 0.023 | 0.005 | 0.001 | ||||||
523 (Jul 2024) | 0.351 | 0.235 | 0.104 | 0.035 | 0.009 | 0.002 | 0.000 | |||||
524 (Aug 2024) | 0.332 | 0.253 | 0.129 | 0.049 | 0.015 | 0.004 | 0.001 | 0.000 | ||||
525 (Sep 2024 | 0.308 | 0.265 | 0.152 | 0.065 | 0.022 | 0.006 | 0.002 | 0.000 | 0.000 | |||
526 (Oct 2024) | 0.283 | 0.270 | 0.172 | 0.082 | 0.031 | 0.010 | 0.003 | 0.001 | 0.000 | 0.000 | ||
527 (Nov 2024) | 0.257 | 0.270 | 0.189 | 0.099 | 0.042 | 0.015 | 0.004 | 0.001 | 0.000 | 0.000 | 0.000 | |
528 (Dec 2024) | 0.232 | 0.266 | 0.203 | 0.116 | 0.053 | 0.020 | 0.007 | 0.002 | 0.000 | 0.000 | 0.000 | 0.000 |
Months | n | |||||||||||
1 | 2 | 3 | 4 | 5 | 6 | 7 | 8 | 9 | 10 | 11 | 12 | |
517 (Jan 2024) | 0.156 | |||||||||||
518 (Feb 2024) | 0.258 | 0.049 | ||||||||||
519 (Mar 2024) | 0.321 | 0.090 | 0.017 | |||||||||
520 (Apr 2024) | 0.354 | 0.133 | 0.033 | 0.006 | ||||||||
521 (May 2024) | 0.367 | 0.173 | 0.054 | 0.013 | 0.002 | |||||||
522 (Jun 2024) | 0.365 | 0.206 | 0.077 | 0.022 | 0.005 | 0.001 | ||||||
523 (Jul 2024) | 0.353 | 0.232 | 0.102 | 0.034 | 0.009 | 0.002 | 0.000 | |||||
524 (Aug 2024) | 0.334 | 0.251 | 0.126 | 0.047 | 0.014 | 0.004 | 0.001 | 0.000 | ||||
525 (Sep 2024 | 0.311 | 0.264 | 0.149 | 0.063 | 0.021 | 0.006 | 0.001 | 0.000 | ||||
526 (Oct 2024) | 0.287 | 0.270 | 0.169 | 0.080 | 0.030 | 0.009 | 0.003 | 0.001 | 0.000 | |||
527 (Nov 2024) | 0.261 | 0.270 | 0.186 | 0.096 | 0.040 | 0.014 | 0.004 | 0.001 | 0.000 | 0.000 | ||
528 (Dec 2024) | 0.236 | 0.267 | 0.201 | 0.113 | 0.051 | 0.019 | 0.006 | 0.002 | 0.000 | 0.000 | 0.000 | 0.000 |