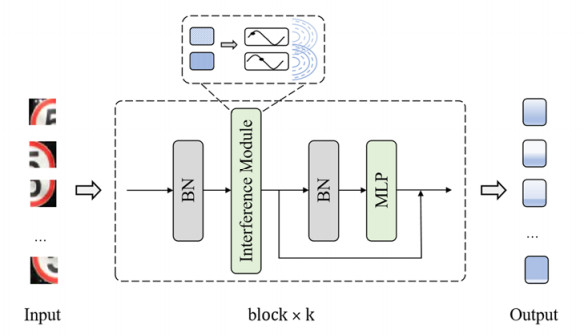
In this paper, we successfully combine convolution with a wave function to build an effective and efficient classifier for traffic signs, named the wave interference network (WiNet). In the WiNet, the feature map extracted by the convolutional filters is refined into many entities from an input image. Each entity is represented as a wave. We utilize Euler's formula to unfold the wave function. Based on the wave-like information representation, the model modulates the relationship between the entities and the fixed weights of convolution adaptively. Experiment results on the Chinese Traffic Sign Recognition Database (CTSRD) and the German Traffic Sign Recognition Benchmark (GTSRB) demonstrate that the performance of the presented model is better than some other models, such as ResMLP, ResNet50, PVT and ViT in the following aspects: 1) WiNet obtains the best accuracy rate with 99.80% on the CTSRD and recognizes all images exactly on the GTSRB; 2) WiNet gains better robustness on the dataset with different noises compared with other models; 3) WiNet has a good generalization on different datasets.
Citation: Qiang Weng, Dewang Chen, Yuandong Chen, Wendi Zhao, Lin Jiao. Wave interference network with a wave function for traffic sign recognition[J]. Mathematical Biosciences and Engineering, 2023, 20(11): 19254-19269. doi: 10.3934/mbe.2023851
[1] | Jing Zhang, Haoliang Zhang, Ding Lang, Yuguang Xu, Hong-an Li, Xuewen Li . Research on rainy day traffic sign recognition algorithm based on PMRNet. Mathematical Biosciences and Engineering, 2023, 20(7): 12240-12262. doi: 10.3934/mbe.2023545 |
[2] | Sung Woong Cho, Sunwoo Hwang, Hyung Ju Hwang . The monotone traveling wave solution of a bistable three-species competition system via unconstrained neural networks. Mathematical Biosciences and Engineering, 2023, 20(4): 7154-7170. doi: 10.3934/mbe.2023309 |
[3] | Shuai Cao, Biao Song . Visual attentional-driven deep learning method for flower recognition. Mathematical Biosciences and Engineering, 2021, 18(3): 1981-1991. doi: 10.3934/mbe.2021103 |
[4] | Weibin Jiang, Xuelin Ye, Ruiqi Chen, Feng Su, Mengru Lin, Yuhanxiao Ma, Yanxiang Zhu, Shizhen Huang . Wearable on-device deep learning system for hand gesture recognition based on FPGA accelerator. Mathematical Biosciences and Engineering, 2021, 18(1): 132-153. doi: 10.3934/mbe.2021007 |
[5] | Boyang Wang, Wenyu Zhang . ACRnet: Adaptive Cross-transfer Residual neural network for chest X-ray images discrimination of the cardiothoracic diseases. Mathematical Biosciences and Engineering, 2022, 19(7): 6841-6859. doi: 10.3934/mbe.2022322 |
[6] | Xiao Ma, Xuemei Luo . Finger vein recognition method based on ant colony optimization and improved EfficientNetV2. Mathematical Biosciences and Engineering, 2023, 20(6): 11081-11100. doi: 10.3934/mbe.2023490 |
[7] | Qingwei Wang, Xiaolong Zhang, Xiaofeng Li . Facial feature point recognition method for human motion image using GNN. Mathematical Biosciences and Engineering, 2022, 19(4): 3803-3819. doi: 10.3934/mbe.2022175 |
[8] | Ting Yao, Farong Gao, Qizhong Zhang, Yuliang Ma . Multi-feature gait recognition with DNN based on sEMG signals. Mathematical Biosciences and Engineering, 2021, 18(4): 3521-3542. doi: 10.3934/mbe.2021177 |
[9] | Jing Wang, Jiaohua Qin, Xuyu Xiang, Yun Tan, Nan Pan . CAPTCHA recognition based on deep convolutional neural network. Mathematical Biosciences and Engineering, 2019, 16(5): 5851-5861. doi: 10.3934/mbe.2019292 |
[10] | Jinhua Zeng, Xiulian Qiu, Shaopei Shi . Image processing effects on the deep face recognition system. Mathematical Biosciences and Engineering, 2021, 18(2): 1187-1200. doi: 10.3934/mbe.2021064 |
In this paper, we successfully combine convolution with a wave function to build an effective and efficient classifier for traffic signs, named the wave interference network (WiNet). In the WiNet, the feature map extracted by the convolutional filters is refined into many entities from an input image. Each entity is represented as a wave. We utilize Euler's formula to unfold the wave function. Based on the wave-like information representation, the model modulates the relationship between the entities and the fixed weights of convolution adaptively. Experiment results on the Chinese Traffic Sign Recognition Database (CTSRD) and the German Traffic Sign Recognition Benchmark (GTSRB) demonstrate that the performance of the presented model is better than some other models, such as ResMLP, ResNet50, PVT and ViT in the following aspects: 1) WiNet obtains the best accuracy rate with 99.80% on the CTSRD and recognizes all images exactly on the GTSRB; 2) WiNet gains better robustness on the dataset with different noises compared with other models; 3) WiNet has a good generalization on different datasets.
Traffic signs give drivers instructions and information that are indispensable in protecting human life and property. Therefore, automatic traffic sign recognition is an important computer vision task [1,2,3]. In recent years, driver assistance systems (DAS) [4,5,6,7] have developed rapidly. Traffic sign recognition is an integrated function of DAS. Normally, professional equipment is mounted on the top of a vehicle to capture traffic sign images. Under real-world conditions, these images are distorted due to various natural and human factors including vehicle speed, weather, destroyed signs, angles, etc. Hence, we apply data augmentation to simulate all kinds of situations. These enhancement techniques refer to rotations, crop, scale, lighting variations and weather conditions changes, which may eventually decrease the traffic sign recognition network performance.
As for the field of traffic sign recognition, it is a famous problem of computer vision tasks. A lot of literature [8,9,10] has studied the topic of traffic sign detection, and some reviews are available in [11,12]. In [13], an algorithm based on Gaussian kernel support vector machines (SVMs) [14] is utilized for traffic sign classification. From experimental results, the proposed algorithm is robust under various conditions including translation, rotation and scale. Ayoub [15] presented a random forest classifier and gave satisfactory results on the Swedish Traffic Signs Dataset. Lu [16] proposed a graph embedding approach that preserved the sparse representation property by using L2, 1-norm. Experiments demonstrate that the proposed approach outperformed previous traffic sign recognition approaches.
In this paper, we introduce the interference module inspired by [17], which dynamically aggregates information by improving the representation way of information according to different semantic contents of an image. In quantum mechanics, a wave function containing both amplitude and phase [18] represents an entity. We describe each entity that is generated by the convolutional filters as a wave to realize the information aggregation procedure dynamically. The amplitude is the real-value feature representing the content of each entity, while the phase term is represented as a complex value. These wave-like entities intervene with each other and close phases tend to enhance each other. The whole framework is constructed by stacking the interference module and channel-mixing multi-layer perceptrons (MLPs). Figure 1 summarizes the architecture. We further conduct ablation experiments and analyze the performance of the proposed model. The final experimental results demonstrate the visible priority of the existing architectures (shown in Table 2).
The contributions of our work are as follows: we propose a novel method (WiNet) for traffic sign recognition. A new convolution structure is introduced for learning multi-scale features and it is successfully combined with a wave function. Additionally, our model achieves the most performance compared with several concurrent works based on ResMLP [19], ResNet50, PVT [20] and ViT [21]. We also test the robustness of all models on datasets with a sufficient number of synthetic samples. Furthermore, we analyze the effects of type of representation on the overall wave interference network that dynamically aggregates information by improving the representation of them according to different semantic contents of an image.
The rest of the paper is organized as follows: Section 2 reviews related methods applied to traffic sign recognition. Section 3 introduces the formulation and architecture of the proposed model. We present experiments and implementation details in Section 4, and further analyze the effectiveness of different modules and activation functions in Section 5. Finally, we draw conclusions in Section 6.
In recent decades, many methods have been proposed for traffic sign recognition. We briefly review related literature [22,23]. It can be divided into traditional methods, methods based convolutional neural networks (CNNs) and methods with attention mechanisms.
Traditional methods for traffic sign recognition: Before CNNs, traffic sign recognition depended on hand-crafted features. In [15], the comparison of different combinations of four features was conducted, including the histogram of oriented gradients (HOG), Gabor, local binary pattern (LBP) and local self-similarity (LSS). The authors tested the proposed method on the Swedish Traffic Signs Data set. Machine learning methods have also been utilized to solve related problems, such as random forests, logistic regression [24] and SVM [25].
CNN for traffic sign recognition: With the rapid development of memory and computation, CNN-based architectures have been the mainstream in the computer vision field. Many works have used CNNs for traffic sign classification. The committee of the CNN-based approach [26] obtains a high recognition rate of 99.15%, which is above the human recognition rate of 98.98%. In the GTSRB competition in 2011, multi-scale CNNs [27] made full use of local and global features and established a new record of an error rate of 1.03%. [28] proposes a novel deep network for traffic sign classification that achieves outstanding performance on GTSRB. The author utilized spatial transformer layers [29] and a modified version of the inception module [30] to build the model. The well-designed inception module allows the network to classify intraclass samples precisely. The spatial transformer layer improves the robustness of the network to deformations such as translation, rotation and scaling of input images.
Attention mechanism for traffic sign recognition: The attention mechanism can adapt to select important information from the input feature. For the outstanding performance, attention mechanism and variants have been applied to a variety of tasks. These improvements include channel attention [31], spatial attention [32] and global attention [33]. This paper [34] proposed an attention-based convolutional pooling neural network (ACPNN). Convolutional pooling replaces max pooling. The ACPNN was validated on the German Traffic Sign Recognition Benchmark (GTSRB). Experiments showed it was robust against external noises and increased recognition accuracy. Based on the ice environment traffic sign recognition benchmark (ITSRB), the author proposed an attention network based on high-resolution traffic sign classification (PFANet) [35] and reached 93.57% accuracy. At the same time, its performance on the GTSRB was as good as the newest and most effective networks.
In this section, we describe our proposed network for traffic-sign recognition in detail. First, we describe the interference module. Then, we describe the whole structure of our proposed network. At last, we describe our data augmentation techniques.
In recent years, many neural network modules based on CNN have been proposed such as [36,37]. In the interference module, the extraction structure of features based on convolution adopts residual-like connections within a single residual block [38]. After the input is normalized, it is split into two subsets which have the same spatial size and number of channels, denoted by X1 and X2, respectively. Each Xi corresponds to a 3×3 convolution, denoted by Ki(), where i∈{1,2}. X2 is added with the output of K1() and then fed into K2(). At last, a 1×1 convolution makes sure the channel number is the same as that of the following module. The output result can be calculated using the following formula:
Ei=Conv1×1(K2(K1(X1)+X2)),i=1,2,...,n. | (1) |
We name the output Ei. The final output Ei can capture feature information from a larger receptive field. Different channels often contain the complete content of different objects in deep neural networks [39]. By using a 1×1 convolution, different channels are assigned to corresponding weights. It is worth noting that the feature extraction structure not only achieves adaptability in the spatial dimension [36] but also adaptability in the channel [36] dimension.
The output Ei is the feature map extracted by the convolutional filters. To describe the feature map concretely, we refer to each feature as an entity. Next, each entity will be converted into a wave. After all, waves have aggregated each other, the new feature map will be generated and fed into the next stage.
An entity is represented as a wave Bj with both amplitude and phase information. A wave can be formulated by
Bj=|Aj|⨂eiθj,j=1,2,...,n,(2) |
where Bj returns the j-th wave, i is the imaginary unit and i2=−1. The |.| denotes the absolute value operation. ⊗ means element-wise multiplication. The amplitude |Aj| represents the information of each entity. A periodic function of the eiθj makes its values distribute over a fixed range. θj is the phase and points out the current location of an entity in a period.
In Eq (2), a wave is represented a complex-value. To embed it in the module, we expand it with Euler's formula. It can be written as
Bj=|Aj|⨂cosθj+i|Aj|⊗sinθj,j=1,2,...,n.(3) |
To get the above equation, we are required to design the form of information expression about both amplitude and phase. The amplitude |Aj| is a real-value feature in the formula. In fact, the absolute operation in the formula is not implemented. A feature map xj=RN×C is taken as the input, and we use a plain channel_FC operation [17] to estimate the amplitude. Specifically, a Tanh activation is adopted to gain the nonlinearity ability. Compared with Relu activation, we found Tanh activation achieves significantly better performance. As can be seen from Figure 3, a wave with a phase has a direction. The value range of Tanh activation is -1–1. Positive and negative numbers can be used to represent different directions. So Tanh activation can help models achieve better performance. Comparisons of Tanh activation with Relu activation are shown in Table 6.
In the above paragraphs, we presented the relevant mathematical expressions. Next, we introduce a concrete evaluation expression about the phase and the result of two waves superimposed.
ChannelFC(xj,Wc)=Wcxj,j=1,2,...,n, | (4) |
where the learnable parameter Wc is the weight. The phase plays an important role in the whole module. It points out the current location of an entity in a period. We take the simplest method to estimate the phase whose parameters are represented with the output value from Tanh activation.
To dynamically adjust the relationship between different entities with fixed parameters, we take the token_FC operation [17] to aggregate information. The token-FC is formulated as:
Token_FC(xj,Wc)=∑KWtjkxk,j=1,2,...,n, | (5) |
where the learnable parameter Wt is the weight. A feature map xk∈RN×C is taken as the input. Here, j means the j-th entity. Finally, the real-value output Oj can be written as:
Oj=∑kWtjkxkcosθk+Wijkxksinθk,j=1,2,...,n, | (6) |
where the learnable parameter Wt and Wi are the weights. As can be seen from Figure 3, when two waves have a similar phase, the output Oj tends to be enhanced. The same semantic content of the input feature map can be extracted in Figure 7 of Section 4.
In the channel dimension, the 1×1 convolution is conducive to fusing information among different channels. To enhance the information fusion ability in the spatial dimension, we use MLPs to exchange information among different entities. Before MLPs, we also apply batch normalization. We adopt residual learning to fusion information from MLPs. As Table 6 illustrates, MLPs significantly improve the performance compared with the network structure not containing MLPs.
In terms of the network architecture, our model is a simple hierarchical structure with 4 stages. Each stage will decrease the output spatial resolution, i.e., H/2×W/2, H/4×W/4, H/8×W/8 and H/16×W/16. Here, H and W represent the height and width of the input image. The number of output channels is increasing with the decrease of resolution. The detailed configuration can be seen in Table 1.
Stage | Output size | Blocks |
1 | H/2×W/2×64 | 2 |
2 | H/4×W/4×128 | 3 |
3 | H/8×W/8×256 | 4 |
4 | H/16×W/16×512 | 2 |
At the beginning of each stage, we downsample the input and control the downsampling rate by using the stride number. In the first stage, we use a 6×6 convolution with stride 2 to embed an input image with the shape H×W×3. In the following three stages, there is a 3×3 convolution with stride 2 to downsample the input data. Note that all other layers in a stage keep the same output size. We use a global average pooling operation for the feature map from the last stage and then adopt a linear classifier to predict the logits.
In general, it is very difficult to estimate which model gives better performance. Many authors evaluate their models on different datasets. To enrich the set of traffic signs, some authors sample images from multiple datasets to perform the evaluation [40,41,42]. On the other hand, lots of authors use their own private datasets [43,44] instead of public datasets [45,46]. In order to be fair, we adopt the ratio of the training set and test set in the CTSRD database that ensures different models are implemented at the same benchmark. We carried out several data augmentation techniques to extend the training set for addressing the challenges from various realistic scenarios.
To demonstrate the generalization of our model, we train our model on another well-known benchmark. The German Traffic Sign Recognition Benchmark (GTSRB) [47] consists of more than 50,000 images in which the classes of traffic signs are more than 40, but we consider only images with a size of at least 30 pixels. All images are resized to 64×64 resolution. The remaining images are ignored on account of the low human visual identification rate. Many models [48,49,50,51] have obtained good results on this dataset, so the comparison between different models is more convincing.
Data augmentation has shown its validity in deep networks. It effectively expands the size of the training set, which is an important factor to consider when training models. A sufficient amount of training data contributes to modulating millions of learnable parameters during the training phase. In real-world situations, traffic signs may be distorted in shape because of human factors. Traffic sign images may contain various appearance distortions such as brightness and contrast. In order to simulate various physical changes in real-world scenarios, we apply image processing techniques to each image randomly and expand the original training dataset as large as five times. Every image is only augmented by one of the available methods in processing, which makes sure that each augmentation operation has the same probability of being implemented. Some samples of original and sample results from these techniques are presented in Figure 4. There are many imaging processing libraries. We apply Albumentations, except for rain, to complete all options. To recapitulate briefly, we perform four classes of augmentations in the data preprocessing stage:
● Random weather. We apply data augmentation to achieve weather effects such as sunny, foggy, rainy and snowy. Synthetic samples keep the size and aspect ratio of input images.
● Random blur. We apply data augmentation to blurred original images. Methods of data augmentation include Blur, MotionBlur, GaussianBlur and MedianBlur in Albumentations.
● Random affine. Geometric transformations for data augmentation are common and effective methods. PiecewiseAffine, Affine and RandomResizedCrop in Albumentations are selected to generate synthetic samples.
● Gause noise. Gauss Noise in Albumentations generates a matrix of random values. Moreno-Barea [52] found adding noise to images can make models more robust on nine datasets from the UCI repository [53].
In this section, we conduct a few analytical experiments to explore our proposed model. To make the comparison fair, we carried out all experiments under the same settings. We report our results on CTSRD, which achieves significant improvements compared with other state-of-the-art models. In addition, we verify the effectiveness of Tanh activation and MLPs. Result visualizations further show the effectiveness of our model.
We implemented our experiments on a PC with an Intel i5-11400H, an NVIDIA GeForce RTX 3050 GPU, and 16 GB RAM. All models are carried out with TensorFlow. During training, we ensure all models use the same parameters for fairness. We train all models using Adam with the same initial learning rate of 10−4. Due to the limitation of the video card, the mini-batch size is set to 64. After considering the resolution of input images, the image is resized to 64 pixels in both width and height. To fuse spatial dimension information, we set the window size to 3 empirically in the interference module. All models are trained for 60 epochs on the original dataset. We train again all models for 30 epochs when using data augmentation and greatly increasing the size of the original dataset.
Table 2 presents the comparison of WiNet with other models, including ResMLP, ResNet50, PVT and ViT. WiNet achieves the best accuracy, which outperforms ResMLP, ResNet50, PVT and ViT by 5.8%, 2.4%, 23.5% and 11%, respectively. This clearly demonstrates that our model has a strong ability to extract features. In comparison to ResNet50 with fewer parameters, our WiNet not only has larger throughputs but also performs better. In particular, WiNet achieves 100% accuracy when images using data augmentation were added to the training set.
Model | Params | Throughput (image/s) | Original dataset | Original dataset including synthetic examples |
Top-1 Accuracy (%) | Top-1 Accuracy (%) | |||
ResMLP | 14.52 M | 13 | 94.0 | 97.2 |
ResNet50 | 23.7 M | 19 | 97.4 | 99.7 |
PVT | 12.3 M | 13 | 76.3 | 89.5 |
ViT | 85.8 M | 11 | 88.8 | 95.7 |
ours | 26.1 M | 14 | 99.8 | 100 |
We give quality histograms in Figure 5, while corresponding statistics (median, mean and standard deviation of all models) are provided in Table 3. It can be seen that the distribution of predicted probability for our model is closer to 1 than for other models trained on CTSRD simultaneously. Statistics further verify the results that WiNet has a higher median value, mean value and a smaller discrete degree.
Model | Median | Mean | Std. Dev. |
ResMLP | 0.99 | 0.96 | 0.109 |
ResNet50 | 1.00 | 0.99 | 0.047 |
PVT | 0.95 | 0.85 | 0.184 |
ViT | 0.99 | 0.94 | 0.121 |
ours | 1.00 | 0.99 | 0.034 |
In order to further analyze the model's performance, we utilize data augmentation including weather, Gauss, blur and affine on the testing set, and then make predictions. Data augmentation has made the distribution of the testing set different from the training set, so it can be used to test the model's robustness. Table 4 lists the predicted results of the model on the testing set. In the above case, our model shows good performance. We use models, which are trained on the training set with synthetic samples, to predict this testing set. As can be seen from Table 5, the performance of WiNet surpasses ResNet50 and has a sharp boost with +2.71, +3.52, +2.21 and +2.76 points for weather, Gauss, blur and affine, respectively. It indicates that our model's effectiveness can be fully exploited on a larger dataset.
Model | Weather | Gauss | Blur | Affine |
ResMLP | 44.08% | 53.01% | 51.86% | 54.71% |
ResNet50 | 70.26% | 65.05% | 64.09% | 65.75% |
PVT | 35.66% | 32.25% | 32.80% | 37.46% |
ViT | 48.14% | 47.34% | 47.09% | 48.35% |
ours | 73.37% | 61.48% | 60.48% | 62.64% |
Model | Weather | Gauss | Blur | Affie |
ResNet50 | 91.22% | 91.57% | 90.97% | 91.27% |
ours | 93.93% | 95.09% | 93.18% | 94.03% |
Except for considering the accuracy of our model, it is crucial to test the generalization of our model by training on different datasets. We divided the dataset from GTSRB into training and validating datasets according to the original proportion. We trained the model with 30 epochs, batch size 64, learning rate 0.0001 and Adam optimizer. The solid curves show the accuracy change, while the dashed curves show the loss change. Here, we can see from Figure 6 that the highest accuracies of both training (21,792 images) and validating datasets (6893 images) are 1.00. The overall trends of accuracies for both training and validating datasets are increasing with epochs. After around 16 epochs, only slight fluctuations can be observed. We observed similar but opposite trends in the loss-changing curves. The losses of both training and testing datasets are very small. In these curves, we can see our proposed model has the advantages of minor loss and high accuracy. This result indicates that our model keeps a good generalization ability rather than only fitting CTSRD.
In this section, we ablate some design components to explore their effectiveness. For a more intuitive feeling, we draw some feature maps with heatmaps in the aggregating process.
To evaluate the performance of design components, we take WiNet with Tanh activation and MLPs as the baseline model. WiNet-Relu is built by replacing Tanh activation with Relu activation on the baseline model. WiNet-noMLP is equal to the baseline model removing MLPs. Ablation results are reported in Table 6. It is obvious that two components play an important part in learning effective parameters. Tanh activation improves the accuracy of WiNet by about 1.2% compared with WiNet-Relu. Without MLPs, the model's performance is down by at least 0.4% (99.8% vs 99.4%).
Model | WiNet-Relu | WiNet-noMLP | WiNet |
Top-1 Accuracy | 98.5% | 99.4% | 99.8% |
Diagrams are an important tool to help people intuitively understand the world. In Section 3.1 (Figure 3 and Eq (6)), we analyze the superposition of two waves with different phases. In order to have a better understanding of the effects of the representation type, we take the visualized feature maps of a traffic sign as an example. From Figure 7, we can clearly see that the visualized feature maps of the first stage with the similar contents are aggregated together. Similar parts in the picture have a closer phase reformulated. Similar parts gradually stick out in the aggregating process, so we get a strong stereo effect from the picture. As the number of network layers deepens, the model extracts more abstract features.
We propose a novel deep learning architecture for traffic sign recognition, whose mechanism for aggregating information is different from the existing transformer, CNN architectures or MLP architectures. In the proposed approach, we combine a new CNN-based module with a wave function successfully. Firstly, we get multi-scale feature representations by the CNN-based module. Then, we utilize channel-FC operations to estimate the amplitude and phase information. Amplitude and phase are key parameters to dynamically modulate relationship entities with similar contents. Extensive experimental evaluations are performed according to different strategies to explore the superiority of the proposed architecture or understand how that works. We will explore further how to use the information representation in different fields or directly use the information representation to preprocess raw data. We also hope our work can encourage people to get new ideas from physical phenomenon.
The authors declare they have not used Artificial Intelligence (AI) tools in the creation of this article.
This work is jointly supported by the National Natural Science Foundation of China under Grant 61976055, the special fund for education and scientific research of Fujian Provincial Department of Finance under Grant GY-Z21001 and Scientific Research Foundation of Fujian University of Technology under Grant GY-Z22071.
The authors declare that they have no known competing financial interests or personal relationships that could have appeared to influence the work reported in this paper.
[1] |
A. Gudigar, S. Chokkadi, U. Raghavendra, U. Rajendra Acharya, An efficient traffic sign recognition based on graph embedding features, Neural Comput. Appl., 31 (2019), 395–407. https://doi.org/10.1007/s00521-017-3063-z doi: 10.1007/s00521-017-3063-z
![]() |
[2] |
Z. Liang, J. Shao, D. Zhang, L. Gao, Traffic sign detection and recognition based on pyramidal convolutional networks, Neural Comput. Appl., 32 (2020), 6533–6543. https://doi.org/10.1007/s00521-019-04086-z doi: 10.1007/s00521-019-04086-z
![]() |
[3] |
R. Abdel-Salam, R. Mostafa, A. H. Abdel-Gawad, RIECNN: real-time image enhanced CNN for traffic sign recognition, Neural Comput. Appl., 34 (2022), 6085–6096. https://doi.org/10.1007/s00521-021-06762-5 doi: 10.1007/s00521-021-06762-5
![]() |
[4] |
M. Lu, K. Wevers, R. V. D. Heijden, Technical feasibility of advanced driver assistance systems (ADAS) for road traffic safety, Transp. Plann. Technol., 28 (2005), 167–187. https://doi.org/10.1080/03081060500120282 doi: 10.1080/03081060500120282
![]() |
[5] | J. C. McCall, M. M. Trivedi, Video-based lane estimation and tracking for driver assistance: survey, system, and evaluation, IEEE Trans. Intell. Transp. Syst., 7 (2006), 20–37. https://doi.org/10.1109/TITS.2006.869595 |
[6] | M. Haloi, D. B. Jayagopi, A robust lane detection and departure warning system, in 2015 IEEE Intelligent Vehicles Symposium (IV), (2015), 126–131. https://doi.org/10.1109/IVS.2015.7225674 |
[7] |
R. Ayachi, Y. Said, A. B. Abdelaali, Pedestrian detection based on light-weighted separable convolution for advanced driver assistance systems, Neural Process. Lett., 52 (2020), 2655–2668. https://doi.org/10.1007/s11063-020-10367-9 doi: 10.1007/s11063-020-10367-9
![]() |
[8] |
Y. Gu, B. Si, A novel lightweight real-time traffic sign detection integration framework based on YOLOv4, Entropy, 24 (2022), 487. https://doi.org/10.3390/e24040487 doi: 10.3390/e24040487
![]() |
[9] | T. Liang, H. Bao, W. Pan, F. Pan, Traffic sign detection via improved sparse R-CNN for autonomous vehicles, J. Adv. Transp., (2022), 1–16. https://doi.org/10.1155/2022/3825532 |
[10] |
J. Wang, Y. Chen, Z. Dong, M. Gao, Improved YOLOv5 network for real-time multi-scale traffic sign detection, Neural Comput. Appl., 35 (2023), 7853–7865. https://doi.org/10.1007/s00521-022-08077-5 doi: 10.1007/s00521-022-08077-5
![]() |
[11] | M Swathi, K. V. Suresh, Automatic traffic sign detection and recognition: A review, in 2017 International Conference on Algorithms, Methodology, Models and Applications in Emerging Technologies (ICAMMAET), (2017), 1–6. https://doi.org/10.1109/ICAMMAET.2017.8186650 |
[12] | C. Liu, S. Li, F. Chang, Y. Wang, Machine vision based traffic sign detection methods: Review, analyses and perspectives, IEEE Access, 7 (2019), 86578–86596. https://doi.org/10.1109/ACCESS.2019.2924947 |
[13] |
S. Maldonado-Bascon, S. Lafuente-Arroyo, P. Gil-Jimenez, H. Gomez-Moreno, F. Lopez-Ferreras, Road-sign detection and recognition based on support vector machines, IEEE Trans. Intell. Transp. Syst., 8 (2007), 264–278. https://doi.org/10.1109/TITS.2007.895311 doi: 10.1109/TITS.2007.895311
![]() |
[14] |
V. Cherkassky, Y. Ma, Practical selection of SVM parameters and noise estimation for SVM regression, Neural Networks, 17 (2004), 113–126. https://doi.org/10.1016/S0893-6080(03)00169-2 doi: 10.1016/S0893-6080(03)00169-2
![]() |
[15] |
A. Ellahyani, M. E. Ansari, I. E. Jaafari, S. Charfi, Traffic sign detection and recognition using features combination and random forests, Int. J. Adv. Comput. Sci. Appl., 7 (2016), 686–693. https://doi.org/10.14569/IJACSA.2016.070193 doi: 10.14569/IJACSA.2016.070193
![]() |
[16] |
K. Lu, Z. Ding, S. Ge, Sparse-representation-based graph embedding for traffic sign recognition, IEEE Trans. Intell. Transp. Syst., 13 (2021), 1515–1524. https://doi.org/10.1109/TITS.2012.2220965 doi: 10.1109/TITS.2012.2220965
![]() |
[17] | Y. Tang, K. Han, J. Guo, C. Xu, Y. Li, C. Xu, et al., An image patch is a wave: Phase-aware vision MLP, in Proceedings of the IEEE/CVF Conference on Computer Vision and Pattern Recognition (CVPR), (2022), 10935–10944. https://doi.org/10.48550/arXiv.2111.12294 |
[18] |
E. J. Heller, M. F. Crommie, C. P. Lutz, D. M. Eigler, Scattering and absorption of surface electron waves in quantum corrals, Nature, 369 (1994), 464–466. https://doi.org/10.1038/369464a0 doi: 10.1038/369464a0
![]() |
[19] |
H. Touvron, P. Bojanowski, M. Caron, M. Cord, A. El-Nouby, E. Grave, et al., ResMLP: Feedforward networks for image classification with data-efficient training, IEEE Trans. Pattern Anal. Mach. Intell., 45 (2023), 5314–5321. https://doi.org/10.1109/TPAMI.2022.3206148 doi: 10.1109/TPAMI.2022.3206148
![]() |
[20] | W. Wang, E. Xie, X. Li, D. Fan, K. Song, D. Liang, et al., Pyramid vision transformer: A versatile backbone for dense prediction without convolutions, in Proceedings of the IEEE/CVF International Conference on Computer Vision (ICCV), (2021), 568–578. https://doi.org/10.48550/arXiv.2102.12122 |
[21] | A. Dosovitskiy, L. Beyer, A. Kolesnikov, D. Weissenborn, X. Zhai, T. Unterthiner, An image is worth 16x16 Words: Transformers for image recognition at scale, preprint, arXiv: 2010.11929. |
[22] | O. N. Manzari, A. Boudesh, S. B. Shokouhi, Pyramid transformer for traffic sign detection, in 2022 12th International Conference on Computer and Knowledge Engineering (ICCKE), (2022), 112–116. https://doi.org/10.1109/ICCKE57176.2022.9960090 |
[23] |
Y. Zheng, W. Jiang, Evaluation of vision transformers for traffic sign classification, Wireless Commun. Mobile Comput., 2022 (2022), 14. https://doi.org/10.1155/2022/3041117 doi: 10.1155/2022/3041117
![]() |
[24] |
D. Pei, F. Sun, H. Liu, Supervised low-rank matrix recovery for traffic sign recognition in image sequences, IEEE Signal Process. Lett., 20 (2013), 241–244. https://doi.org/10.1109/LSP.2013.2241760 doi: 10.1109/LSP.2013.2241760
![]() |
[25] | S. Ardianto, C. Chen, H. Hang, Real-time traffic sign recognition using color segmentation and SVM, in 2017 International Conference on Systems, Signals and Image Processing (IWSSIP), (2017), 1–5. https://doi.org/10.1109/IWSSIP.2017.7965570 |
[26] | D. Cireşan, U. Meier, J. Masci, J. Schmidhuber, A committee of neural networks for traffic sign classification, in the 2011 International Joint Conference on Neural Networks, (2011), 1918–1921. https://doi.org/10.1109/IJCNN.2011.6033458. |
[27] | P. Sermanet, Y. LeCun, Traffic sign recognition with multi-scale convolutional networks, in the 2011 International Joint Conference on Neural Networks, (2011), 2809–2813. https://doi.org/10.1109/IJCNN.2011.6033589 |
[28] | M. Haloi, Traffic sign classification using deep inception based convolutional networks, preprint, arXiv: 1511.02992. |
[29] | M. Jaderberg, K. Simonyan, A. Zisserman, K. kavukcuoglu, Spatial transformer networks, in Advances in Neural Information Processing Systems 28 (NIPS 2015), 28 (2015). |
[30] | C. Szegedy, W. Liu, Y. Jia, P. Sermanet, S. Reed, D. Anguelov, et al., Going deeper with convolutions, in Proceedings of the IEEE Conference on Computer Vision and Pattern Recognition (CVPR), (2015), 1–9. https://doi.org/10.1109/CVPR.2015.7298594 |
[31] | J. Hu, L. Shen, G. Sun, Squeeze-and-excitation networks, in Proceedings of the IEEE Conference on Computer Vision and Pattern Recognition (CVPR), (2018), 7132–7141. https://doi.org/10.48550/arXiv.1709.01507 |
[32] |
Y. Yuan, Z. Xiong, Q. Wang, VSSA-NET: Vertical spatial sequence attention network for traffic sign detection, IEEE Trans. Image Process., 28 (2019), 3423–3434. https://doi.org/10.1109/TIP.2019.2896952 doi: 10.1109/TIP.2019.2896952
![]() |
[33] | Y. Liu, Z. Shao, N. Hoffmann, Global attention mechanism: Retain information to enhance channel-spatial interactions, preprint, arXiv: 2112.05561. |
[34] |
S. Liu, J. Li, C. Hu, W. Wang, Traffic sign recognition based on convolutional neural network and ensemble learning, Comput. Mod., 12 (2019), 67. https://doi.org/10.3969/j.issn.1006-2475.2019.12.013 doi: 10.3969/j.issn.1006-2475.2019.12.013
![]() |
[35] |
K. Zhou, Y. Zhan, D. Fu, Learning region-based attention network for traffic sign recognition, Sensors, 21 (2021), 686. https://doi.org/10.3390/s21030686 doi: 10.3390/s21030686
![]() |
[36] |
M. Guo, C. Lu, Z. Liu, M. Cheng, S. Hu, Visual attention network, Visual Media, 9 (2023), 733–752. https://doi.org/10.1007/s41095-023-0364-2 doi: 10.1007/s41095-023-0364-2
![]() |
[37] |
S. Gao, M. Cheng, K. Zhao, X. Zhang, M. Yang, P. Torr, Res2Net: A new multi-scale backbone architecture, IEEE Trans. Pattern Anal. Mach. Intell., 43 (2019), 652–662. https://doi.org/10.1109/TPAMI.2019.2938758 doi: 10.1109/TPAMI.2019.2938758
![]() |
[38] | K. He, X. Zhang, S. Ren, J. Sun, Deep Residual learning for image recognition, in Proceedings of the IEEE Conference on Computer Vision and Pattern Recognition (CVPR), (2016), 770–778. https://doi.org/10.1109/CVPR.2016.90 |
[39] | L. Chen, H. Zhang, J. Xiao, L. Nie, J. Shao, W. Liu, SCA-CNN: Spatial and channel-wise attention in convolutional networks for image captioning, in Proceedings of the IEEE Conference on Computer Vision and Pattern Recognition (CVPR), (2017), 5659–5667. |
[40] |
Z. Zhu, J. Lu, R. R. Martin, S. Hu, An optimization approach for localization refinement of candidate traffic signs, IEEE Trans. Intell. Transp. Syst., 18 (2017), 3006–016. https://doi.org/10.1109/TITS.2017.2665647 doi: 10.1109/TITS.2017.2665647
![]() |
[41] | S. Mehta, C. Paunwala, B. Vaidya, CNN based traffic sign classification using Adam optimizer, in 2019 International Conference on Intelligent Computing and Control Systems (ICCS), (2019), 1293–1298. https://doi.org/10.1109/ICCS45141.2019.9065537 |
[42] |
A. Staravoitau, Traffic sign classification with a convolutional network, Pattern Recognit. Image Anal., 28 (2018), 155–162. https://doi.org/10.1134/S1054661818010182 doi: 10.1134/S1054661818010182
![]() |
[43] |
J. Greenhalgh, M. Mirmehdi, Real-time detection and recognition of road traffic signs, IEEE Trans. Intell. Transp. Syst., 13 (2012), 1498–1506. https://doi.org/10.1109/TITS.2012.2208909 doi: 10.1109/TITS.2012.2208909
![]() |
[44] | G. Overett, L. Petersson, Large scale sign detection using HOG feature variants, in 2011 IEEE Intelligent Vehicles Symposium (IV), (2011), 326–331. https://doi.org/10.1109/IVS.2011.5940549 |
[45] |
A. Mogelmose, M. M. Trivedi, T. B. Moeslund, Vision-based traffic sign detection and analysis for intelligent driver assistance systems: Perspectives and survey, IEEE Trans. Intell. Transp. Syst., 13 (2012), 1484–1497. https://doi.org/10.1109/TITS.2012.2209421 doi: 10.1109/TITS.2012.2209421
![]() |
[46] | F. Larsson, M. Felsberg, Using fourier descriptors and spatial models for traffic sign recognition, in SCIA 2011: Image Analysis, 6688 (2011), 238–249. https://doi.org/10.1007/978-3-642-21227-7_23 |
[47] |
J. Stallkamp, M. Schlipsing, J. Salmen, C. Igel, Man vs. computer: Benchmarking machine learning algorithms for traffic sign recognition, Neural Networks, 32 (2012), 323–332. https://doi.org/10.1016/j.neunet.2012.02.016 doi: 10.1016/j.neunet.2012.02.016
![]() |
[48] | X. Mao, S. Hijazi, R. Casas, P. Kaul, R. Kumar, C. Rowen, Hierarchical CNN for traffic sign recognition, in 2016 IEEE Intelligent Vehicles Symposium (IV), (2016), 130–135. https://doi.org/10.1109/IVS.2016.7535376 |
[49] | X. Peng, Y. Li, X. Wei, J. Luo, Y. Murphey, Traffic sign recognition with transfer learning, in 2017 IEEE Symposium Series on Computational Intelligence (SSCI), (2017), 1–7. https://doi.org/10.1109/SSCI.2017.8285332 |
[50] |
Y. Jin, Y. Fu, W. Wang, J. Guo. C. Ren, X. Xiang, Multi-feature fusion and enhancement single shot detector for traffic sign recognition, IEEE Access, 8 (2020), 38931–38940. https://doi.org/10.1109/ACCESS.2020.2975828 doi: 10.1109/ACCESS.2020.2975828
![]() |
[51] |
A. Bouti, M. A. Mahraz, J. Riffi, H. Tairi, A robust system for road sign detection and classification using LeNet architecture based on convolutional neural network, Soft Comput., 24 (2020), 6721–6733. https://doi.org/10.1007/s00500-019-04307-6 doi: 10.1007/s00500-019-04307-6
![]() |
[52] | F. J. Moreno-Barea, F. Strazzera, J. M. Jerez, D. Urda, L. Franco, Forward noise adjustment scheme for data augmentation, in 2018 IEEE Symposium Series on Computational Intelligence (SSCI), (2018), 728–734. https://doi.org/10.1109/SSCI.2018.8628917 |
[53] | A. Asuncion, D. Newman, UCI Machine Learning Repository, 2007. Available from: http://archive.ics.uci.edu/ml. |
Stage | Output size | Blocks |
1 | H/2×W/2×64 | 2 |
2 | H/4×W/4×128 | 3 |
3 | H/8×W/8×256 | 4 |
4 | H/16×W/16×512 | 2 |
Model | Params | Throughput (image/s) | Original dataset | Original dataset including synthetic examples |
Top-1 Accuracy (%) | Top-1 Accuracy (%) | |||
ResMLP | 14.52 M | 13 | 94.0 | 97.2 |
ResNet50 | 23.7 M | 19 | 97.4 | 99.7 |
PVT | 12.3 M | 13 | 76.3 | 89.5 |
ViT | 85.8 M | 11 | 88.8 | 95.7 |
ours | 26.1 M | 14 | 99.8 | 100 |
Model | Median | Mean | Std. Dev. |
ResMLP | 0.99 | 0.96 | 0.109 |
ResNet50 | 1.00 | 0.99 | 0.047 |
PVT | 0.95 | 0.85 | 0.184 |
ViT | 0.99 | 0.94 | 0.121 |
ours | 1.00 | 0.99 | 0.034 |
Model | Weather | Gauss | Blur | Affine |
ResMLP | 44.08% | 53.01% | 51.86% | 54.71% |
ResNet50 | 70.26% | 65.05% | 64.09% | 65.75% |
PVT | 35.66% | 32.25% | 32.80% | 37.46% |
ViT | 48.14% | 47.34% | 47.09% | 48.35% |
ours | 73.37% | 61.48% | 60.48% | 62.64% |
Model | Weather | Gauss | Blur | Affie |
ResNet50 | 91.22% | 91.57% | 90.97% | 91.27% |
ours | 93.93% | 95.09% | 93.18% | 94.03% |
Model | WiNet-Relu | WiNet-noMLP | WiNet |
Top-1 Accuracy | 98.5% | 99.4% | 99.8% |
Stage | Output size | Blocks |
1 | H/2×W/2×64 | 2 |
2 | H/4×W/4×128 | 3 |
3 | H/8×W/8×256 | 4 |
4 | H/16×W/16×512 | 2 |
Model | Params | Throughput (image/s) | Original dataset | Original dataset including synthetic examples |
Top-1 Accuracy (%) | Top-1 Accuracy (%) | |||
ResMLP | 14.52 M | 13 | 94.0 | 97.2 |
ResNet50 | 23.7 M | 19 | 97.4 | 99.7 |
PVT | 12.3 M | 13 | 76.3 | 89.5 |
ViT | 85.8 M | 11 | 88.8 | 95.7 |
ours | 26.1 M | 14 | 99.8 | 100 |
Model | Median | Mean | Std. Dev. |
ResMLP | 0.99 | 0.96 | 0.109 |
ResNet50 | 1.00 | 0.99 | 0.047 |
PVT | 0.95 | 0.85 | 0.184 |
ViT | 0.99 | 0.94 | 0.121 |
ours | 1.00 | 0.99 | 0.034 |
Model | Weather | Gauss | Blur | Affine |
ResMLP | 44.08% | 53.01% | 51.86% | 54.71% |
ResNet50 | 70.26% | 65.05% | 64.09% | 65.75% |
PVT | 35.66% | 32.25% | 32.80% | 37.46% |
ViT | 48.14% | 47.34% | 47.09% | 48.35% |
ours | 73.37% | 61.48% | 60.48% | 62.64% |
Model | Weather | Gauss | Blur | Affie |
ResNet50 | 91.22% | 91.57% | 90.97% | 91.27% |
ours | 93.93% | 95.09% | 93.18% | 94.03% |
Model | WiNet-Relu | WiNet-noMLP | WiNet |
Top-1 Accuracy | 98.5% | 99.4% | 99.8% |