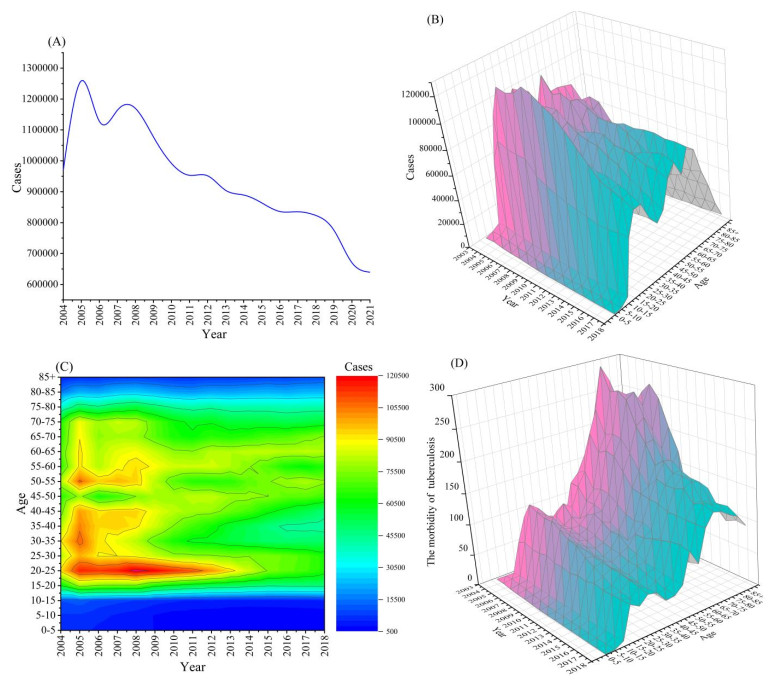
Tuberculosis (TB) is an infectious disease transmitted through the respiratory system. China is one of the countries with a high burden of TB. Since 2004, an average of more than 800,000 cases of active TB has been reported each year in China. Analyzing the case data from 2004 to 2018, we found significant differences in TB incidence by age group. A model of TB is put forward to explore the effect of age heterogeneity on TB transmission. The nonlinear least squares method is used to obtain the key parameters in the model, and the basic reproduction number Rv = 0.8017 is calculated and the sensitivity analysis of Rv to the parameters is given. The simulation results show that reducing the number of new infections in the elderly population and increasing the recovery rate of elderly patients with the disease could significantly reduce the transmission of TB. Furthermore, the feasibility of achieving the goals of the World Health Organization (WHO) End TB Strategy in China is assessed, and we obtained that with existing TB control measures it will take another 30 years for China to reach the WHO goal to reduce 90% of the number of new cases by the year 2049. However, in theory it is feasible to reach the WHO strategic goal of ending TB by 2035 if the group contact rate in the elderly population can be reduced, though it is difficult to reduce the contact rate.
Citation: Chuanqing Xu, Kedeng Cheng, Yu Wang, Maoxing Liu, Xiaojing Wang, Zhen Yang, Songbai Guo. Analysis of the current status of TB transmission in China based on an age heterogeneity model[J]. Mathematical Biosciences and Engineering, 2023, 20(11): 19232-19253. doi: 10.3934/mbe.2023850
[1] | Tao-Li Kang, Hai-Feng Huo, Hong Xiang . Dynamics and optimal control of tuberculosis model with the combined effects of vaccination, treatment and contaminated environments. Mathematical Biosciences and Engineering, 2024, 21(4): 5308-5334. doi: 10.3934/mbe.2024234 |
[2] | Benjamin H. Singer, Denise E. Kirschner . Influence of backward bifurcation on interpretation of $R_0$ in a model of epidemic tuberculosis with reinfection. Mathematical Biosciences and Engineering, 2004, 1(1): 81-93. doi: 10.3934/mbe.2004.1.81 |
[3] | Siyu Liu, Yong Li, Yingjie Bi, Qingdao Huang . Mixed vaccination strategy for the control of tuberculosis: A case study in China. Mathematical Biosciences and Engineering, 2017, 14(3): 695-708. doi: 10.3934/mbe.2017039 |
[4] | Silvia Martorano Raimundo, Hyun Mo Yang, Ezio Venturino . Theoretical assessment of the relative incidences of sensitive andresistant tuberculosis epidemic in presence of drug treatment. Mathematical Biosciences and Engineering, 2014, 11(4): 971-993. doi: 10.3934/mbe.2014.11.971 |
[5] | Luju Liu, Jianhong Wu, Xiao-Qiang Zhao . The impact of migrant workers on the tuberculosis transmission: General models and a case study for China. Mathematical Biosciences and Engineering, 2012, 9(4): 785-807. doi: 10.3934/mbe.2012.9.785 |
[6] | Azizeh Jabbari, Carlos Castillo-Chavez, Fereshteh Nazari, Baojun Song, Hossein Kheiri . A two-strain TB model with multiplelatent stages. Mathematical Biosciences and Engineering, 2016, 13(4): 741-785. doi: 10.3934/mbe.2016017 |
[7] | Zuqin Ding, Yaxiao Li, Xiaomeng Wang, Huling Li, Yongli Cai, Bingxian Wang, Kai Wang, Weiming Wang . The impact of air pollution on the transmission of pulmonary tuberculosis. Mathematical Biosciences and Engineering, 2020, 17(4): 4317-4327. doi: 10.3934/mbe.2020238 |
[8] | Lih-Ing W. Roeger, Z. Feng, Carlos Castillo-Chávez . Modeling TB and HIV co-infections. Mathematical Biosciences and Engineering, 2009, 6(4): 815-837. doi: 10.3934/mbe.2009.6.815 |
[9] | Carlos Castillo-Chavez, Baojun Song . Dynamical Models of Tuberculosis and Their Applications. Mathematical Biosciences and Engineering, 2004, 1(2): 361-404. doi: 10.3934/mbe.2004.1.361 |
[10] | Abba B. Gumel, Baojun Song . Existence of multiple-stable equilibria for a multi-drug-resistant model of mycobacterium tuberculosis. Mathematical Biosciences and Engineering, 2008, 5(3): 437-455. doi: 10.3934/mbe.2008.5.437 |
Tuberculosis (TB) is an infectious disease transmitted through the respiratory system. China is one of the countries with a high burden of TB. Since 2004, an average of more than 800,000 cases of active TB has been reported each year in China. Analyzing the case data from 2004 to 2018, we found significant differences in TB incidence by age group. A model of TB is put forward to explore the effect of age heterogeneity on TB transmission. The nonlinear least squares method is used to obtain the key parameters in the model, and the basic reproduction number Rv = 0.8017 is calculated and the sensitivity analysis of Rv to the parameters is given. The simulation results show that reducing the number of new infections in the elderly population and increasing the recovery rate of elderly patients with the disease could significantly reduce the transmission of TB. Furthermore, the feasibility of achieving the goals of the World Health Organization (WHO) End TB Strategy in China is assessed, and we obtained that with existing TB control measures it will take another 30 years for China to reach the WHO goal to reduce 90% of the number of new cases by the year 2049. However, in theory it is feasible to reach the WHO strategic goal of ending TB by 2035 if the group contact rate in the elderly population can be reduced, though it is difficult to reduce the contact rate.
Tuberculosis (TB) is an ancient disease with a worldwide distribution and is the leading cause of death from bacterial infections. Mycobacterium TB, is the causative agent of TB. It was discovered and proved to be the causative agent of human TB by the German bacteriologist Koch in 1882, and it can invade all organs of the body, but is most common in causing pulmonary TB. TB is mainly transmitted through the respiratory tract, and the source of infection is through contact with TB patients who have excreted the bacteria [1]. In the 19th century, TB became a major epidemic in Europe and elsewhere, spreading to all levels of society and causing one out of every seven deaths, known as the "Great White Plague" [2]. Due to the low effectiveness of drugs used to treat TB, the disease is still uncontrollable and remains widespread worldwide. Between 1993 and 1996, the number of TB cases worldwide increased by 13% and TB killed more people than AIDS and malaria combined. In late 1995, the World Health Organization (WHO) established March 24 as World TB Day to further promote global awareness of TB prevention and control [3]. Approximately 80% of new TB cases worldwide occur in 22 high-burden countries, with the largest number of global cases accounted to India with 26% and China with 12%, respectively [4]. To this day, TB remains the leading cause of disease and death in most high-incidence countries [3]. To end the global TB epidemic, WHO proposed in 2014 a post-2015 global end-TB strategy target of a 50% reduction in TB incidence by 2025 (compared to 2015) and a 90% reduction in new cases by 2035.
Mathematical models have become a powerful tool for analyzing epidemiological characteristics [5]. Many scholars developed mathematical models reflecting the characteristics of TB based on its transmission mechanism, principles of biology, seasonal characteristics and social influences. In 1962, Waaler developed the first model of TB transmission kinetics [6]. In 1967 Brogger further refined Waaler's model. He not only introduced heterogeneity but also changed the method of calculating the incidence rate; however, he did not give the relationship between infection and incidence rates [7]. ReVelle developed the first nonlinear differential equation model for TB using Brogger and Waaler's model as a template [8]. E. Ziv et al. studied the effect of early treatment on the incidence of TB and found that early treatment reduced the incidence of TB if the treatment rate for active TB was increased from 50% to 60%. Carlos Castillo-Chavez et al. studied the role of mobility and health disparities on the transmission dynamics of TB [9]. In addition, medical studies have shown that anti-TB drugs can reduce the length of treatment for TB [10]. Meanwhile, many studies have considered the effects of drug-resistant cases [11], time lag [12] and age structure [13]. However, few studies use models of TB with different age groupings. Therefore, based on the collected data and the observed data characteristics (Figure 2), a susceptible-exposed-infectious-recovered (SEIR) model with different age groups is developed, and the feature of heterogeneity is considered in the model to assess the effect of age as a factor on TB transmission.
The main research of this paper is as follows: in section two, the detailed data collected is given and the data in relation is analyzed. A kinetic transmission model is developed, the main parameters in the model are fitted and the value of the basic reproduction number Rv is calculated. In section three, a sensitivity analysis of the basic reproduction number Rv is performed, considering the effect of the proportion of preferential contact within the group on Rv. Finally, a feasibility assessment of the WHO strategic objective of ending TB is presented. Section four contains the discussion section.
China is one of the high TB burden countries and faces a serious TB epidemic. The burden of TB in China has increased in the last two decades due to the emergence of drug-resistant strains of Mycobacterium TB [14], with an average of more than 800,000 new infections per year. The number of new cases from 2004 to 2021 is presented in Figure 1(A), which shows that the number of new infections per year is decreasing each year. A three-dimensional plot of the incidence by age group is given, and it is shows that the incidence of TB in different age groups is significantly different. The incidence rate was lowest in the 0–15 age group and much higher in the 60+ age group compared to other age groups. Overall, the number of TB cases was the highest in the 20–25 and 60–65 age groups, the results of which are shown in Figure 1(B), (C). Statistical data on the morbidity of TB in each age group is shown in Figure 1(D).
According to the data of the United Nations [15], the rate of aging in China is gradually accelerating. The incidence of TB in different age groups was counted, mainly including the mean number of TB incidence, the mean incidence rate and its 95% confidence interval (CI) for each age group in the past fifteen years and the results of statistical analysis, which are shown in Table 1 with the incidence rate in units of 1/100,000. The results show that with the gradual increase of age, the number of TB incidence showed a trend of rising and then decreasing, while the incidence of TB showed a trend of increasing.
Age grouping | Mean number of incidences | 95%CI | Mean incidence rate | 95%CI |
0–5 | 2692 | 1645–3738 | 3.4073 | 2.0316–4.7830 |
5–10 | 3005 | 1619–4391 | 3.9197 | 2.1201–5.7192 |
10–15 | 6666 | 5284–8047 | 7.8952 | 7.1978–8.5925 |
15–20 | 58,335 | 51,904–64,766 | 56.2213 | 54.8779–57.5647 |
20–25 | 97,491 | 86,176–108,805 | 83.3704 | 69.7977–96.9431 |
25–30 | 79,469 | 74,453–84,486 | 80.3324 | 71.4972–89.1677 |
30–35 | 67,689 | 56,899–78,479 | 68.0293 | 61.3837–74.6749 |
35–40 | 69,529 | 57,746–81,312 | 59.7398 | 51.3281–68.1515 |
40–45 | 76,188 | 67,365–85,011 | 66.8514 | 57.3751–76.3276 |
45–50 | 74,451 | 71,112–77,790 | 73.0433 | 64.3025–81.7842 |
50–55 | 80,333 | 73,185–87,480 | 95.4184 | 87.3205–103.5163 |
55–60 | 78,587 | 72,486–84,689 | 101.3584 | 88.3025–114.4143 |
60–65 | 81,710 | 78,840–84,580 | 146.2743 | 131.4336–161.1151 |
65–70 | 71,538 | 67,286–75,789 | 170.6339 | 154.6441–186.6238 |
70–75 | 65,196 | 58,412–71,981 | 201.5498 | 172.0765–231.0232 |
75–80 | 44,653 | 41,831–47,474 | 195.5469 | 168.0045–223.0892 |
80–85 | 21,985 | 20,706–23,264 | 175.2472 | 151.4695–199.0249 |
85+ | 8164 | 7339–8988 | 163.7941 | 126.7235–200.8647 |
Based on the statistical data from 2004 to 2018, a cluster analysis of the data on the incidence of TB in different age groups is given. The complete classification process for all data is shown in Figure 2 and their complete clustering results are shown in Table 2, where distance refers to a distance between a member of each class and the center of that class, and center, in this case, refers to a concept similar to the mean value within group. The cluster column in the table shows the final clustering results. We obtained the age group 0–15 years is the category with the lowest incidence of TB, the age group 15–60 years is the category with higher incidence and the group over 60 years is the category with the highest incidence.
Case Number | Age Group | Cluster | Distance | Case Number | Age Group | Cluster | Distance |
1 | 0–5 | 1 | 6.846 | 10 | 45–50 | 2 | 30.071 |
2 | 5–10 | 1 | 5.865 | 11 | 50–55 | 2 | 93.997 |
3 | 10–15 | 1 | 11.941 | 12 | 55–60 | 2 | 106.104 |
4 | 15–20 | 2 | 90.778 | 13 | 60–65 | 3 | 133.339 |
5 | 20–25 | 2 | 51.670 | 14 | 65–70 | 3 | 58.259 |
6 | 25–30 | 2 | 32.120 | 15 | 70–75 | 3 | 106.789 |
7 | 30–35 | 2 | 33.865 | 16 | 75–80 | 3 | 80.648 |
8 | 35–40 | 2 | 63.602 | 17 | 80–85 | 3 | 32.058 |
9 | 40–45 | 2 | 40.032 |
We analyzed the influence of age as a factor in TB infection in Figure 3, which shows the important role of age as a factor in the transmission of TB. Therefore, in this paper we develop a TB epidemic model that includes age-group heterogeneity, and through qualitative analysis and numerical simulation we predict the future incidence of TB in China. We assess whether China could meet the WHO strategic target by 2035 with the current control measures, as well as explore measures to more effectively prevent and control TB outbreaks.
The annual number of TB cases reported in mainland China from 2004 to 2021 was obtained from the Public Health Sciences Data Center [1]. Over a period of nearly 20 years, the number of reported cases exceeded 16 million. Among them, the number of cases between the ages of 15–60 years is the highest, with an average of 680,000 new infections per year, or 69%. The number of cases between the ages of 0–15 years is the lowest, with an average of 12,000 new infections per year, or 1.3%, as shown in Table A1 (Appendix A). Looking at the overall TB data in China, the number of new infections per year is gradually decreasing from the initial 970,279 cases in 2004 to 639,548 cases in 2021.
In this section we develop a model of TB dynamics that includes age heterogeneity and vaccination and divides the entire population into three groups according to TB incidence, with 0–15 years as the first group, 15–60 years as the second group and over 60 years as the third group. Each group is also divided into susceptible (S), exposed (E), infectious (I) and recovered (R). The number of natural deaths and the natural death rates are different in each age group. The age transition from the previous age group to the next age group is considered. Exposed TB cases refers to individuals who have been infected with TB bacteria but are asymptomatic, and the exposed patients and reinfection of recovered individuals has no place in the model. The dynamic process of TB transmission is shown in Figure 4.
The model is:
{dS1/dt=(1−ρω)A−(λ1+μ1+θ1)S1,dE1/dt=λ1S1−(σ1+μ1+θ1)E1,dI1/dt=σ1E1−(γ1+d1+μ1+θ1)I1,dR1/dt=ρωA+γ1I1−(μ1+θ1)R1,dS2/dt=θ1S1−(λ2+μ2+θ2)S2,dE2/dt=λ2S2+θ1E1−(σ2+μ2+θ2)E2,dI2/dt=σ2E2+θ1I1−(γ2+d2+μ2+θ2)I2,dR2/dt=γ2I2+θ1R1−(μ2+θ2)R2,dS3/dt=θ2S2−(λ3+μ3)S3,dE3/dt=λ3S3+θ2E2−(σ3+μ3)E3,dI3/dt=σ3E3+θ2I2−(γ3+d3+μ3)I3,dR3/dt=γ3I3+θ2R2−μ3R3. | (1) |
A is the annual number of births in the population and ρ is the Bacillus Calmette-Guerin (BCG) vaccination rate at birth. ω is the effective rate of BCG and μi is the natural mortality rate of members of group i. θi is the transfer rate of members of age group i into age group i+1(i=1,2). σi is the activation rate (conversion rate) of latent TB patients. γi is the recovery rate of TB patients.di is the mortality rate due to TB infection in group i, and λi is the hazard rates of infection of infected persons to susceptible persons among members of group i. The parameter λi is related to the average number of contacts of group i members ai, which is the probability that a member of group i is infected after each contact with an infected person βi. cij was proposed by Jacquez et al [16] in 1988 to represent the proportion of contacts between members of group i and members of group j, as shown in expression (3), which is preferentially contacted to members within the same group [16]. The Kronecker function δij with a value of one when i = j and zero otherwise. fj is the proportional mixing fraction, as in the expression (4).
λi=aiβi3∑j=1cijIjNj, | (2) |
cij=εiδij+(1−εi)fj, | (3) |
fj=(1−εj)ajNj/(1−εj)ajNj3∑k=1(1−εk)akNk3∑k=1(1−εk)akNk, | (4) |
Here, Nj=Sj+Ej+Ij+Rj.
The outbreak of severe acute respiratory syndrome (SARS) in 2003 posed a challenge to the public health system in China, and the government, in an effort to better address public health issues, increased public health funding, revised laws regarding infectious disease control, implemented an internet-based disease reporting system and initiated a program to rebuild local public health facilities. These measures have facilitated TB control [17]. Complete data on TB cases is available on the official website of the Chinese Center for Disease Control and Prevention from after 2004. When numerical fitting was performed, data from 2004 was used to calculate the initial values and data from 2005–2021 was used for parameter fitting.
We first estimate the parameters in the model, and the results are shown in Table 3.
Parameter | Value | Source | Parameter | Value | Source |
A | 1.644×107 | [18] | θ2 | 0.0067 | [19] |
ρ | 1 | [20] | a1 | 12 × 365 | [23] |
ω | 0.728 | Fitting | a2 | 10 × 365 | [23] |
μ1 | 0.0017 | [19] | a3 | 8 × 365 | [23] |
μ2 | 0.0023 | [19] | β1 | 1.325 × 10-4 | Fitting |
μ3 | 0.0367 | [19] | β2 | 7.402 × 10-5 | Fitting |
di | 0.0025 | [4] | β3 | 4.690 × 10-4 | Fitting |
γi | 0.496 | [22] | ε1 | 0.4 | Assumption |
σi | 6 | [21] | ε2 | 0.3 | Assumption |
θ1 | 0.079 | [19] | ε3 | 0.3 | Assumption |
(a) From the data published in the China Population Statistical Yearbook 2005–2018 [18], the annual number of births of the population is A≈16,440,000/year. The natural mortality rates of the three age groups were μ1≈0.0017/year, μ2≈0.0023/year and μ3≈0.0367/year [19]. China started its immunization planning policy in 1992, and newborn infants must be vaccinated within 24 hours of birth with the BCG vaccine [20], thus, ρ=1.
(b) The average incubation period of TB is about two months [21], so the conversion rate of patients with latent TB is taken as σi=6. The mortality rate is di = 0.0025/year according to the WHO Global TB Report 2013 [4]. The recovery rate of infected cases is γi = 0.496/year [22]. Each person will have contact with an average of 10–12 people per day [23], and we calculate the value of a1 = 4380/year, a2 = 3650/year, a3 = 2920/year, θ1 = 0.079/year and θ2 = 0.0067/year [19].
(c) Based on the proportion of each age group in 2005, the initial values S1(0) = 264,991,621, besides S2(0) = 940,454,335 and S3(0) = 99,961,110 are calculated. Based on the number of people infected with TB in 2005 [1], the initial values I1(0) = 26048, I2(0) = 881,944 and I3(0) = 351,316 were obtained. The percent of people with a TB bacteria infection but asymptomatic and those successfully treated for TB are 12.1% and 80%, respectively. Based on the number of people infected with TB in each age group in 2004, E1(0) = 2934, E2(0) = 83,257, E3(0) = 31,212 R1(0) = 19,397, R2(0) = 550,464 and R3(0) = 206,362 were calculated.
(d) The number of cases of the TB infection from 2005 to 2021 is fitted according to model (1).
China has taken various control measures to control TB transmission, including a five-year national plan in the 1980s, a 10-year national plan in the 1990s and the modern TB control strategy (Directed Observed Treatment Short-Course) introduced in the 20th century [24]. After the implementation of these short-term and long-term plans, TB was effectively controlled in China. The model takes into account the heterogeneity of age subgroups and exposure between age groups. After 2004, China began to provide detailed data on TB cases, so we selected the data from 2005 to 2021 and used the model to fit the number of TB cases in China from 2005 to 2018 [25]. The values of the parameters obtained from the fitting at this time are shown in Table 3 and the fitting effect is shown in Figure 5. It can be seen from the figure that the fitting results are very good, and the gap between the fitting curve and the actual data is very small. We performed a goodness-of-fit test on the fit results and the goodness-of-fit coefficient was 0.954 (the calculation of the goodness-of-fit coefficient can be found in Appendix B). The better fitting effect shows that the considered model is very trustworthy and can reflect the variation of the actual data well, even when considering more realistic situations.
In epidemiological studies, the reproduction number (denoted as Rv) indicates the average number of infections in an infected person during the period of infection [26], and is one of the most important indicators to assess the risk of an infectious disease. The reproduction number Rv is also considered a key epidemiological parameter in determining whether the disease can spread in an area, with Rv > 1 often implying that the disease will persist and Rv < 1 implying that the disease will become extinct. The reproduction number for model (1) was calculated using the next generation matrix method [27], and the complete calculation process is presented in Appendix C.
Based on the values of the parameters obtained from the fit, the basic reproduction number Rv = 0.8017 is calculated for model (1). The strength of the correlation between each parameter in the model and the basic reproduction number Rv is judged as a way to find the most sensitive epidemiological parameter that should be prioritized when controlling infectious diseases [28]. The sensitivity analysis of Rv is performed using the partial rank correlation coefficient (PRCC) [29] of each parameter in the model, and the results are shown in Figure 6. The basic reproduction number Rv, A(annual population births), β3(probability of exposure to infection in people over 60 years of age) and γ3(recovery rate in elderly people over 60 years of age) are the most sensitive parameters, and |PRCC(A)|>|PRCC(γ3)|>|PRCC(β3)|. Furthermore, reducing new infections in the elderly population and improving recovery rate in older patients with the disease can significantly reduce the transmission of TB.
Transmission of TB occurs mainly through close human-to-human contact, and the concept of contact is quantified in the model. The hazard rate of infection λi is defined by the function of parameters of the average number of contacts ai, the proportion of preferential contacts within the group εi and the probability of infection βi. Among these parameters, the most important parameter is εi, which indicates the extent to which each individual prioritizes contacts with members of the same group. A larger εi means that an individual has more frequent contacts with members of the same group.
We considered the effect of εi on Rv by changing the value of εi (i=1,2,3) to observe the change in the basic reproduction number Rv. The effect of εi on Rv is considered by fixing one of the values of parameters εi and changing the value of the others, as seen in Figure 7. The results show that increasing the values of ε1, ε2 and ε3, respectively will cause an increase in the basic reproduction number Rv. The value of ε3 has the greatest effect on Rv and ε1 has the least effect on Rv. The oldest groups' frequent contact with each other can increase the spread of TB. Therefore, efforts to protect the elderly population should be strengthened by calling on them to increase their nutrition, exercise themselves and have regular health checks. We also appeal more young people to give care to the elderly. It is believed that TB in China will be better controlled if there are fewer TB infections in the elderly group, though this is not easy to do.
In the nearly two decades between 2004 and 2021, China has taken various control measures against TB and achieved very significant results, with the number of new TB cases in China decreasing from a peak of 1.25 million in 2005 to 630,000 in 2021, and significant progress has been made in controlling TB. However, TB still remains a huge challenge and in order to end the TB epidemic globally, WHO proposed a post-2015 global TB endgame strategy in 2014 with the strategic goal of reducing TB incidence by 50% by 2025 (compared to 2015) and new cases by 90% by 2035 [30]. The number of new TB cases in China in 2015 was 864,015, and the WHO target expects China to reduce the number of new TB cases to 86,402 in 2035.
In the previous analysis, we calculated that the reproduction number of TB Rv = 0.8017. The value of Rv is less than one, but without considering the increase of other control measures, by 2035 China will still have nearly 300,000 new infections of TB. This will not reach the desired target of WHO. According to the simulation results shown in Figure 8, China will be in 2049; that is, it will take nearly 30 years to reach the desired target of WHO.
The effectiveness of BCG vaccine ω, average number of contacts ai and the proportion of preferential contacts εi are the most important factors in the TB control process. To investigate the impact of parameters ω, ai and εi on the number of new cases of TB based on current control strategies, we tried to find the feasibility of achieving WHO's end-TB strategy goals. The parameter values listed in Table 3 are used to compare the control effects.
First, considering an intervention scenario that increases the parameter ω, the results are shown in Figure 9(A) and the number of TB cases for different values of ω is given in Figure 9(B). It was found that increasing the effectiveness of BCG was of limited help in reducing the number of TB cases in the short term. Increasing ω by 10% would reduce the number of TB cases by an average of 3000 per year (Appendix D). Even if the effectiveness of BCG was increased from 72.8% to 95%, China would still have more than 230,000 new infections in 2035 (Appendix D), but the number of new cases per year would already be significantly reduced. Therefore, increasing the effectiveness of the vaccine would be a good option, although it would not meet the WHO strategic goal of 2035. The reason for this is that BCG is more effective in younger children, but less effective in the older age groups. The number of new cases in younger TB-infected patients has been low, so the impact of increasing the effectiveness of BCG is not significant.
We consider an intervention scenario that reduces the average number ai of contacts, and the simulation results are shown in Figure 10. Reducing the average number of contacts a1, a2 and a3 in each group can reduce the number of TB new cases. However, by reducing a1 and a2 by 20%, China would still have more than 200,000 new infections in 2035 and would not meet the WHO TB strategic targets. Reducing a1 and a2 would have a lesser effect, reducing the average number of contacts in the third group a3 would have a larger effect and reducing a3 by 25% would result in a rapid decline in the number of TB infections in China. The number of new infections in 2035 is only 60,000, and the WHO target can be reached by 2035.
Considering the impact of the proportion of preferential contacts εi within the group, the results are given in Figure 11. Figure 11(A) illustrates that the impact caused by ε3 is the largest, while Figure 11(B) can show that reducing only ε1 or ε2 has a smaller impact. It shows that reducing the proportion of preferential contact ε1, ε2 and ε3 within a group can help reduce the number of new cases of TB. However, the effect of reducing the parameter values ε1 and ε2 on the number of new cases is not very significant. Reducing ε1 and ε2 by 25% and 33%, respectively, would still leave China with more than 200,000 new infections in 2035 and would not meet the WHO TB strategy target. However, reducing the proportion of preferential contact ε3 of group three would have a dramatic effect. By reducing the value parameter ε3 by 50%, the number of TB infections in China decreased rapidly. With only 70,000 new TB infections per year by 2035, the WHO strategic target for 2035 can be reached.
In summary, for all intervention scenarios it will be difficult for China to reach the ultimate WHO target by 2035 by using the existing TB control measures. However, if the average contact rate a3 can be reduced by 25% or the preferential contact rate ε3 within the group can be reduced by 50%, the WHO strategic target for TB control by 2035 can be reached. China still has a long way to go on the road to eliminating TB by strengthening the implementation of control measures, achieving early detection and treatment and improving the effectiveness of anti-TB drugs.
With TB control measures, the number of TB cases reported each year in China is gradually decreasing, which means that China is reducing the number of new cases of TB each year. However, there is still a long way to go to eliminate TB and the TB epidemic may remain a serious problem in the future. According to the data of the China Population Statistical Yearbook [18] and China's population structure, the proportion of elderly people is increasing year by year. China considers its post-2015 end-TB strategy [31], and an aging population poses a great challenge to TB control in China. More importantly, our study shows in Figure 3 that there were more significant differences in TB incidence between different age groups. Using TB data reported in China from 2005 to 2021, we developed a SEIR infectious disease model that includes three age groups, juvenile (0–15 years), middle-aged (15–60 years) and elderly (60 years or older), to investigate the role of age in the transmission of TB in China. The parameters in the model were fitted using the least squares method and numerical simulations were performed using the fitted parameters. The fitted data was compared with the reported real data in high agreement with the annual data reported for TB in China. All of our fits were obtained from the number of reported TB cases in China, but we have no way of knowing whether the number of reported cases equals the actual number of cases. If the number of reported cases is less than the actual number of cases, this will have an impact on the estimation of parameters and model predictions. On this basis, the current basic reproduction number of TB transmission in China is Rv = 0.8017. Even if the value of the basic reproduction number of TB transmission in China, Rv, is less than one, it would still take 45 years for China to eliminate TB (to reduce the number of new infections to less than 10,000 per year). The feasibility of achieving the WHO strategic goal of ending TB by 2035 under the current TB control initiatives adopted in China was assessed. With the current control measures, it would take nearly 30 years for China to reach the expected WHO goal. How to shorten this process is one of the issues to be considered in China.
According to the model, we evaluated the effect of different intervention options and the effect of increasing the effectiveness of BCG vaccine exists, but it was limited. Even if BCG effectiveness was increased to 95%, China would not reach the WHO strategic goal of ending TB by 2035. However, if there was a 25% reduction in a3, a 50% reduction in ε3 or a reduction in overall contact in the elderly population, China could reach the WHO strategic goal by 2035. In order to eliminate TB as soon as possible, China needs to continue to strengthen the implementation of TB control measures, improve the effectiveness of TB drugs and further explore other effective control measures.
It is reasonable to consider age grouping and contact heterogeneity in the TB model, which would be more realistic and help us to improve control strategies for TB in China [19]. Interventions such as increased nutrition for the elderly and early detection and treatment for specific groups of the elderly can be a very effective epidemic control measure [32]. Thus, our age grouping model provides a valuable foresight. For example, BCG is highly effective in young adults but less effective in middle-aged and older adults, with effectiveness in the second and third age groups being only about 50% [33]. However, with the increased aging of the Chinese population and the fact that the elderly population has a high incidence of TB, a similar BCG control strategy should be implemented for the potentially high-risk elderly sub-population, which may significantly reduce the incidence in this group. According to reports on TB [34], approximately 0.5% to 7.2% of TB cases in developed countries was caused by Mycobacterium bovis, while in many developing countries, the severity of human infection with bovine TB was much higher than in developed countries [35]. In China, there are several regions that depend on animal husbandry, such as the pastoral areas of Xinjiang, Tibet and Inner Mongolia where cows are mostly raised on a small scale or free-range, which can greatly facilitate the transmission of bovine TB between humans and cattle [36]. Therefore, it is reasonable to believe that a proportion of the TB patients in China are bovine TB patients, and that bovine TB patients are more capable of transmitting the virus. If measures can be taken to control the number of infections in this group, it is believed that this will help to reduce the overall number of TB cases in China. This is an issue that we intend to continue to study. Studying the role of age in TB transmission may help to predict long-term health risks and, thus, suggest targeted TB control strategies, more rational programming and more efficient use of limited resources [37], which is still of great significance for TB control. Also, when focusing on the elderly population, improving their healthy living standards, increasing their nutrition and calling for their greater participation in exercise all have a positive impact on TB control.
The limitation of the findings in this paper is that only Chinese yearly TB cases data were fitted, with less analysis and simulation of different age groups. We will improve this in future studies.
The authors declare they have not used Artificial Intelligence (AI) tools in the creation of this article.
This study was funded by the National Natural Science Foundation of China (No. 11901027), the China Postdoctoral Science Foundation (No. 2021M703426), the Pyramid Talent Training Project of BUCEA (JDYC20200327), the Undergraduate Teaching Practice Program (J1703) and the Post Graduate Innovation Project of BUCEA (PG2022139). We thank all the individuals who generously shared their time and materials for this study.
The authors declare there is no conflict of interest.
Year | 2004 | 2005 | 2006 | 2007 | 2008 | 2009 | 2010 | 2011 | 2012 |
0–15 | 24,247 | 26,048 | 20,735 | 17,972 | 16,011 | 12,320 | 9751 | 8275 | 8058 |
15–60 | 688,080 | 881,944 | 795,790 | 811,150 | 814,952 | 759,035 | 705,680 | 679,621 | 662,458 |
60+ | 257,952 | 351,316 | 311,046 | 334,837 | 338,577 | 305,583 | 275,919 | 265,379 | 280,992 |
Sum | 970,279 | 1,259,308 | 1,127,571 | 1,163,959 | 116, 9540 | 1,076,938 | 991,350 | 953,275 | 951,508 |
Year | 2013 | 2014 | 2015 | 2016 | 2017 | 2018 | 2019 | 2020 | 2021 |
0–15 | 7070 | 6695 | 6861 | 6769 | 7037 | 7591 | 8116 | ||
15–60 | 626,042 | 606,179 | 575,854 | 553,732 | 543,950 | 526,626 | 499,140 | ||
60+ | 271,322 | 276,507 | 281,300 | 275,735 | 284,206 | 289,125 | 268,508 | ||
Sum | 904,434 | 889,381 | 864,015 | 836,236 | 835,193 | 823,342 | 775,764 | 670,538 | 639,548 |
We use R2 to denote the goodness-of-fit coefficient, and R2 is defined by the expression (5).
R2 = 1−RSSTSS | (5) |
where RSS means the residual sum of squares, which represents the sum of squares of the deviations between the actual and simulated values. TSS means total sum of squares, which represents the sum of squares of the deviations between the actual and expected values.
We use the magnitude of the goodness-of-fit coefficient to judge the effectiveness of the fit. The closer the goodness-of-fit coefficient is to one, the better the fit is.
The disease-free equilibrium point of model (1) is P0=(S01,0,0,R01,S02,0,0,R02,S03,0,0,R03), where S01=(1−ρω)Aμ1+θ1, R01=ρωAμ1+θ1, S02=θ1S01μ2+θ2, R02=θ1R01μ2+θ2, S03=θ2S02μ3, R03=θ2R02μ3.
The reproduction number of model (1) is calculated using the next generation matrix approach,
F = (a1β1S1(c11I1N1+c12I2N2+c13I3N3)0a2β2S2(c21I1N1+c22I2N2+c23I3N3)0a3β3S3(c31I1N1+c32I2N2+c33I3N3)0),V = ((μ1+σ1+θ1)E1(μ1+d1+γ1+θ1)I1−σ1E1(μ2+σ2+θ2)E2−θ1E1(μ2+d2+γ2+θ2)I2−σ2E2−θ1I1(μ3+σ3)E3−θ2E2(μ3+d3+γ3)I3−σ3E3−θ2I2) |
The partial derivatives of Ei and Ii are obtained,
F=(0a1β1c11S011N100a1β1c12S011N200a1β1c13S011N300000000a2β2c21S021N100a2β2c22S021N200a2β2c23S021N300000000a3β3c31S031N100a3β3c32S031N200a3β3c33S031N30000000) |
The partial derivatives of Ei and Ii are obtained,
V=(μ1+σ1+θ100000−σ1μ1+d1+γ1+θ10000−θ10μ2+σ2+θ20000−θ1−σ2μ2+d2+γ2+θ20000−θ20μ3+σ30000−θ2−σ3μ3+d3+γ3) |
and
V−1=(1A100000σ1A1A21A20000θ1A1A301A3000B1θ1A2A4σ2A3A41A400θ1θ2A1A3A50θ2A3A501A50B2θ1θ2A2A4A6B3θ2A4A6σ3A5A61A6) |
Among them,
A1=μ1+σ1+θ1,A2=μ1+d1+γ1+θ1,A3=μ2+σ2+θ2,A4=μ2+d2+γ2+θ2, |
A5=μ3+σ3,A6=μ3+d3+γ3,B1=σ1θ1A1A2A4+σ2θ1A1A3A4, |
B2=σ1θ1θ2A1A2A4A6+σ2θ1θ2A1A3A4A6+σ3θ1θ2A1A3A5A6,B3=σ2θ2A3A4A6+σ3θ2A3A5A6 |
Therefore, at the disease-free equilibrium point P0, there is
FV−1=(HKOT), |
Among them,
H=(a1β1S01(c11σ1N10A1A2+c12B1N20+c13B2N30)a1β1S01(c11N10A2+c12θ1N20A2A4+c13θ1θ2N30A2A4A6)a1β1S01(c12σ2N20A3A4+c13B3N30)000a2β2S02(c21σ1N10A1A2+c22B1N20+c23B2N30)a2β2S02(c21N10A2+c22θ1N20A2A4+c23θ1θ2N30A2A4A6)a2β2S02(c22σ2N20A3A4+c23B3N30))K=(a1β1c12S01N20A4+a1β1c13θ2S01N30A4A6a1β1c13σ3S01N30A5A6a1β1c13S01N30A6000a2β2c22S02N20A4+a2β2c23θ2S02N30A4A6a2β2c23σ3S02N30A5A6a2β2c23S02N30A6)O=(000a3β3S03(c31σ1N10A1A2+c32B1N20+c33B2N30)a3β3S03(c31N10A2+c32θ1N20A2A4+c33θ1θ2N30A2A4A6)a3β3S03(c32σ2N20A3A4+c33B3N30)000)T=(000a3β3c32S03N20A4+a3β3c33θ2S03N30A4A6a3β3c33σ3S03N30A5A6a3β3c33S03N30A6000) |
The basic reproduction number is the spectral radius of the matrix FV−1[38], i.e., Rv=ρ(FV−1).
Year | 2022 | 2023 | 2024 | 2025 | 2026 | 2027 | 2028 | |
Cases | ω = 0.8 | 595,736 | 552,311 | 514,403 | 480,536 | 449,676 | 421,138 | 394,438 |
ω = 0.9 | 595,663 | 551,990 | 513,698 | 479,352 | 447,964 | 418,866 | 391,609 | |
ω = 0.95 | 595,626 | 551,830 | 513,347 | 478,765 | 447,116 | 417,747 | 390,221 | |
Year | 2029 | 2030 | 2031 | 2032 | 2033 | 2034 | 2035 | |
Cases | ω = 0.8 | 369,259 | 345,381 | 322,660 | 300,998 | 280,332 | 260,619 | 241,832 |
ω = 0.9 | 365,888 | 341,499 | 318,303 | 296,209 | 275,157 | 255,106 | 236,028 | |
ω = 0.95 | 364,242 | 339,610 | 316,192 | 293,899 | 272,671 | 252,468 | 233,262 |
[1] | Public Health Sciences Data Center. Available from: http://www.phsciencedata.cn. |
[2] |
H. M. Zhao, Health education in the prevention and treatment of TB, World Latest Med. Inf., 18 (2018), 197–199. https://doi.org/10.19613/j.cnki.1671-3141.2018.51.110 doi: 10.19613/j.cnki.1671-3141.2018.51.110
![]() |
[3] | Chinese Center for Disease Control and Prevention. Available from: http://www.chinatb.org. |
[4] | World Health Organization, Global TB Report (2013). Available from: http://www.who.int/tb/publications/globalreport/en/. |
[5] |
R. M. G. J. Houben, N. A. Menzies, T. Sumner, G. H. Huynh, N. Arinaminpathy, J. D. Goldhaber-Fiebert, et al., Feasibility of achieving the 2025 WHO global TB target in South Africa, China, and India: a combined analysis of 11 mathematical models, Lancet Global Health, 4 (2016), e806–e815. https://doi.org/10.1016/S2214-109X(16)30199-1 doi: 10.1016/S2214-109X(16)30199-1
![]() |
[6] |
H. Waaler, A. Geser, S. Andersen. The use of mathematical models in the study of the epidemiology of TB, Am. J. Public Health, 52 (1962), 1002–1013. https://doi.org/10.2105/ajph.52.6.1002 doi: 10.2105/AJPH.52.6.1002
![]() |
[7] |
S. Brogger, Systems analysis in TB control: a model, Am. Rev. Respir. Dis., 95 (1967), 419–434. https://doi.org/10.1164/arrd.1967.95.3.419 doi: 10.1164/arrd.1967.95.3.419
![]() |
[8] |
C. S. Revelle, W. R. Lynn, F. Feldmann, Mathematical models for the economic allocation of TB control activities in developing nations, Am. Rev. Respir. Dis., 96 (1967), 893–909. https://doi.org/10.1164/arrd.1967.96.5.893 doi: 10.1164/arrd.1967.96.5.893
![]() |
[9] |
V. Moreno, B. Espinoza, K. Barley, M. Paredes, D. Bichara, A. Mubayi, et al., The role of mobility and health disparities on the transmission dynamics of TB, Theor. Biol. Med. Modell., 14 (2017). https://doi.org/10.1186/s12976-017-0049-6 doi: 10.1186/s12976-017-0049-6
![]() |
[10] |
A. Koch, H. Cox, V. Mizrahi, Drug-resistant TB: challenges and opportunities for diagnosis and treatment, Curr. Opin. Pharmacol., 42 (2018), 7–15. https://doi.org/10.1016/j.coph.2018.05.013 doi: 10.1016/j.coph.2018.05.013
![]() |
[11] |
Y. J. Lin, C. M. Liao, Seasonal dynamics of TB epidemics and implications for multidrug-resistant infection risk assessment, Epidemiol. Infect., 142 (2014), 358–370. https://doi.org/10.1017/S0950268813001040 doi: 10.1017/S0950268813001040
![]() |
[12] |
H. X. Wang, L. Q. Jiang, G. H. Wang, Stability of a TB model with a time delay in transmission, J. North Univ. China, 35 (2014), 238–242. https://doi.org/10.3969/j.issn.1673-3193.2014.03.003 doi: 10.3969/j.issn.1673-3193.2014.03.003
![]() |
[13] |
T. Cohen, M. Lipsitch, R. P. Walensky, M. Murray, Beneficial and perverse effects of isoniazid preventive therapy for latent TB infection in HIV-TB co-infected populations, PNAS, 103 (2006), 7042–7047. https://doi.org/10.1073/pnas.0600349103 doi: 10.1073/pnas.0600349103
![]() |
[14] | Y. Wang, The fifth national TB epidemiological survey in 2010, Chin. J. Antituberc., 8 (2012), 485–508. |
[15] |
J. P. Smith, S. Strauss, Y. H. Zhao, Healthy aging in China, J. Econ. Ageing, 4 (2014), 37–43. https://doi.org/10.1016/j.jeoa.2014.08.006 doi: 10.1016/j.jeoa.2014.08.006
![]() |
[16] |
J. A. Jacquez, C. P. Simon, J. Koopman, L. Sattenspiel, T. Perry, Modeling and analyzing HIV transmission: the effect of contact patterns, Math. Biosci., 92 (1988), 119–199. https://doi.org/10.1016/0025-5564(88)90031-4 doi: 10.1016/0025-5564(88)90031-4
![]() |
[17] |
J. P. Millet, E. Shaw, À. Orcau, M. Casals, J. M. Miró, J. A. Caylà, et al., TB recurrence after completion treatment in a European city: reinfection or relapse, PLoS One, 8 (2013), e64898. https://doi.org/10.1371/journal.pone.0064898 doi: 10.1371/journal.pone.0064898
![]() |
[18] | China Population Statistic Yearbook (2021). Available from: http://www.stats.gov.cn/tjsj/ndsj/. |
[19] |
Y. Zhao, M. Li, S. Yuan, Analysis of transmission and control of TB in mainland China, 2005–2016, based on the age-structure mathematical model, Int. J. Environ. Res. Public Health, 14 (2017), 1192. https://doi.org/10.3390/ijerph14101192 doi: 10.3390/ijerph14101192
![]() |
[20] | J. Wang, Can BCG vaccine protect you for life, Fam. Med., 3 (2015), 14. |
[21] | National Scientific Data Sharing Platform for Population and Health (2021). Available from: https://www.ncmi.cn. |
[22] |
S. M. Blower, A. R. Mclean, T. C. Porco, P. M. Small, P. C. Hopewell, M. A. Sanchez, et al., The intrinsic transmission dynamics of TB epidemics, Nat. Med., 1 (1995) 815–821. https://doi.org/10.1038/nm0895-815 doi: 10.1038/nm0895-815
![]() |
[23] |
J. M. Read, J. Lessler, S. Riley, S. Wang, L. J. Tan, K. O. Kwok, et al., Social mixing patterns in rural and urban areas of southern China, Proc. Biol. Sci., 1785 (2014), 281. https://doi.org/10.1098/rspb.2014.0268 doi: 10.1098/rspb.2014.0268
![]() |
[24] | S. Cheng, E. Liu, F. Wang, Y. Ma, L. Zhou, L. Wang, et al., Systematic review and implemention progress of DOTS strategy, Chin. J. Antituberc., 34 (2012), 585–591. |
[25] |
Z. L. Feng, J. W. Glasser, A. N. Hill, M. A. Franko, R. Carlsson, H. Hallander, et al., Modeling rates of infection with transient maternal antibodies and waning active immunity: application to Bordetella pertussis in Sweden, J. Theor. Biol., 356 (2014), 123–132. https://doi.org/10.1016/j.jtbi.2014.04.020 doi: 10.1016/j.jtbi.2014.04.020
![]() |
[26] |
C. Fraser, C. A. Donnelly, S. Cauchemez, W. P. Hanage, M. D. Van Kerkhove, T. Déirdre Hollingsworth, et al., Pandemic potential of a strain of Influenza A (H1N1): early findings, Science, 324 (2009), 1557–1561. https://doi.org/10.1126/science.1176062 doi: 10.1126/science.1176062
![]() |
[27] |
P. V. D Driessche, J. Watmough, Reproduction numbers and sub-threshold endemic equilibria for compartmental models of disease transmission, Math. Biosci., 180 (2002), 29–48. https://doi.org/10.1016/s0025-5564(02)00108-6 doi: 10.1016/S0025-5564(02)00108-6
![]() |
[28] |
D. Gao, Y. Lou, D. He, T. C. Porco, Y. Kuang, G. Chowell, et al., Prevention and control of Zika as a mosquito-borne and sexually transmitted disease: a mathematical modeling analysis, Sci. Rep., 6 (2016), 28070. https://doi.org/10.1038/srep28070 doi: 10.1038/srep28070
![]() |
[29] |
L. K. Yan, Application of correlation coefficient and biased correlation coefficient in related analysis, J. Yunnan Univ. Finance Econ., 3 (2003), 78–80. https://doi.org/10.16537/j.cnki.jynufe.2003.03.018 doi: 10.16537/j.cnki.jynufe.2003.03.018
![]() |
[30] | World Health Organization, WHO End TB Strategy (2014). Available from: https://www.who.int/teams/global-tuberculosis-programme/the-end-tb-strategy. |
[31] |
G. H. Huynh, D. J. Klein, D. P. Chin, B. G. Wagner, P. A. Eckhoff, R. Liu, et al. TB control strategies to reach the 2035 global targets in China: the role of changing demographics and reactivation disease, BMC Med., 13 (2015), 88. https://doi.org/10.1186/s12916-015-0341-4 doi: 10.1186/s12916-015-0341-4
![]() |
[32] |
E. Ziv, C. L. Daley, S. M. Blower, Early therapy for latent TB infection, Am. J. Epidemiol., 153 (2001), 381–385. https://doi.org/10.1093/aje/153.4.381 doi: 10.1093/aje/153.4.381
![]() |
[33] |
E. Ziv, C. L. Daley, S. M. Blower, Potential public health impact of new TB vaccines, Emerging Infect. Dis., 10 (2004), 1529–1535. https://doi.org/10.3201/eid1009.030921 doi: 10.3201/eid1009.030921
![]() |
[34] | A. Z. Guo, Control and decontamination of TB in animals, in 2019 International Symposium on Human-Veterinary Diseases and China Rabies Annual Meeting, Hefei, China, |
[35] | X. Y. Zhang, M. J. Sun, W. X. Fan, The spread and control of bovine TB, China Anim. Health Inspection, 34 (2017), 70–74. |
[36] | Y. Y. Chen, H. C. Chen, A. Z. Guo, Epidemiological characteristics of bovine TB and its prevention and control, China Animal Health, 14 (2012), 7–9. |
[37] |
L. J. Liu, X. Q. Zhao, Y. C. Zhou, A TB model with seasonality, Bull. Math. Biol., 72 (2010), 931–952. https://doi.org/10.1007/s11538-009-9477-8 doi: 10.1007/s11538-009-9477-8
![]() |
[38] |
O. Diekmann, J. A. Heesterbeek, J. A. Metz, On the definition and the computation of the basic reproduction ratio R0 in models for infectious diseases in heterogeneous populations, J. Math. Biol., 28 (1990), 365–382. https://doi.org/10.1007/BF00178324 doi: 10.1007/BF00178324
![]() |
1. | Yiwen Tao, Jiaxin Zhao, Hao Cui, Lili Liu, Long He, Exploring the impact of socioeconomic and natural factors on pulmonary tuberculosis incidence in China (2013–2019) using explainable machine learning: A nationwide study, 2024, 253, 0001706X, 107176, 10.1016/j.actatropica.2024.107176 | |
2. | Yudi Ari Adi, , An investigation of Susceptible–Exposed–Infectious–Recovered (SEIR) tuberculosis model dynamics with pseudo-recovery and psychological effect, 2024, 6, 27724425, 100361, 10.1016/j.health.2024.100361 | |
3. | Jianling Xiong, Lingming Kong, Yangling Shen, Shilu Yao, Liuxia Wei, Jiangyan Zhao, Liang Chen, Zhen Wang, Guanghu Zhu, Model-informed evaluation of interventions to eliminate tuberculosis transmission in China, 2025, 241, 00333506, 33, 10.1016/j.puhe.2025.02.007 |
Age grouping | Mean number of incidences | 95%CI | Mean incidence rate | 95%CI |
0–5 | 2692 | 1645–3738 | 3.4073 | 2.0316–4.7830 |
5–10 | 3005 | 1619–4391 | 3.9197 | 2.1201–5.7192 |
10–15 | 6666 | 5284–8047 | 7.8952 | 7.1978–8.5925 |
15–20 | 58,335 | 51,904–64,766 | 56.2213 | 54.8779–57.5647 |
20–25 | 97,491 | 86,176–108,805 | 83.3704 | 69.7977–96.9431 |
25–30 | 79,469 | 74,453–84,486 | 80.3324 | 71.4972–89.1677 |
30–35 | 67,689 | 56,899–78,479 | 68.0293 | 61.3837–74.6749 |
35–40 | 69,529 | 57,746–81,312 | 59.7398 | 51.3281–68.1515 |
40–45 | 76,188 | 67,365–85,011 | 66.8514 | 57.3751–76.3276 |
45–50 | 74,451 | 71,112–77,790 | 73.0433 | 64.3025–81.7842 |
50–55 | 80,333 | 73,185–87,480 | 95.4184 | 87.3205–103.5163 |
55–60 | 78,587 | 72,486–84,689 | 101.3584 | 88.3025–114.4143 |
60–65 | 81,710 | 78,840–84,580 | 146.2743 | 131.4336–161.1151 |
65–70 | 71,538 | 67,286–75,789 | 170.6339 | 154.6441–186.6238 |
70–75 | 65,196 | 58,412–71,981 | 201.5498 | 172.0765–231.0232 |
75–80 | 44,653 | 41,831–47,474 | 195.5469 | 168.0045–223.0892 |
80–85 | 21,985 | 20,706–23,264 | 175.2472 | 151.4695–199.0249 |
85+ | 8164 | 7339–8988 | 163.7941 | 126.7235–200.8647 |
Case Number | Age Group | Cluster | Distance | Case Number | Age Group | Cluster | Distance |
1 | 0–5 | 1 | 6.846 | 10 | 45–50 | 2 | 30.071 |
2 | 5–10 | 1 | 5.865 | 11 | 50–55 | 2 | 93.997 |
3 | 10–15 | 1 | 11.941 | 12 | 55–60 | 2 | 106.104 |
4 | 15–20 | 2 | 90.778 | 13 | 60–65 | 3 | 133.339 |
5 | 20–25 | 2 | 51.670 | 14 | 65–70 | 3 | 58.259 |
6 | 25–30 | 2 | 32.120 | 15 | 70–75 | 3 | 106.789 |
7 | 30–35 | 2 | 33.865 | 16 | 75–80 | 3 | 80.648 |
8 | 35–40 | 2 | 63.602 | 17 | 80–85 | 3 | 32.058 |
9 | 40–45 | 2 | 40.032 |
Parameter | Value | Source | Parameter | Value | Source |
A | 1.644×107 | [18] | θ2 | 0.0067 | [19] |
ρ | 1 | [20] | a1 | 12 × 365 | [23] |
ω | 0.728 | Fitting | a2 | 10 × 365 | [23] |
μ1 | 0.0017 | [19] | a3 | 8 × 365 | [23] |
μ2 | 0.0023 | [19] | β1 | 1.325 × 10-4 | Fitting |
μ3 | 0.0367 | [19] | β2 | 7.402 × 10-5 | Fitting |
di | 0.0025 | [4] | β3 | 4.690 × 10-4 | Fitting |
γi | 0.496 | [22] | ε1 | 0.4 | Assumption |
σi | 6 | [21] | ε2 | 0.3 | Assumption |
θ1 | 0.079 | [19] | ε3 | 0.3 | Assumption |
Year | 2004 | 2005 | 2006 | 2007 | 2008 | 2009 | 2010 | 2011 | 2012 |
0–15 | 24,247 | 26,048 | 20,735 | 17,972 | 16,011 | 12,320 | 9751 | 8275 | 8058 |
15–60 | 688,080 | 881,944 | 795,790 | 811,150 | 814,952 | 759,035 | 705,680 | 679,621 | 662,458 |
60+ | 257,952 | 351,316 | 311,046 | 334,837 | 338,577 | 305,583 | 275,919 | 265,379 | 280,992 |
Sum | 970,279 | 1,259,308 | 1,127,571 | 1,163,959 | 116, 9540 | 1,076,938 | 991,350 | 953,275 | 951,508 |
Year | 2013 | 2014 | 2015 | 2016 | 2017 | 2018 | 2019 | 2020 | 2021 |
0–15 | 7070 | 6695 | 6861 | 6769 | 7037 | 7591 | 8116 | ||
15–60 | 626,042 | 606,179 | 575,854 | 553,732 | 543,950 | 526,626 | 499,140 | ||
60+ | 271,322 | 276,507 | 281,300 | 275,735 | 284,206 | 289,125 | 268,508 | ||
Sum | 904,434 | 889,381 | 864,015 | 836,236 | 835,193 | 823,342 | 775,764 | 670,538 | 639,548 |
Year | 2022 | 2023 | 2024 | 2025 | 2026 | 2027 | 2028 | |
Cases | ω = 0.8 | 595,736 | 552,311 | 514,403 | 480,536 | 449,676 | 421,138 | 394,438 |
ω = 0.9 | 595,663 | 551,990 | 513,698 | 479,352 | 447,964 | 418,866 | 391,609 | |
ω = 0.95 | 595,626 | 551,830 | 513,347 | 478,765 | 447,116 | 417,747 | 390,221 | |
Year | 2029 | 2030 | 2031 | 2032 | 2033 | 2034 | 2035 | |
Cases | ω = 0.8 | 369,259 | 345,381 | 322,660 | 300,998 | 280,332 | 260,619 | 241,832 |
ω = 0.9 | 365,888 | 341,499 | 318,303 | 296,209 | 275,157 | 255,106 | 236,028 | |
ω = 0.95 | 364,242 | 339,610 | 316,192 | 293,899 | 272,671 | 252,468 | 233,262 |
Age grouping | Mean number of incidences | 95%CI | Mean incidence rate | 95%CI |
0–5 | 2692 | 1645–3738 | 3.4073 | 2.0316–4.7830 |
5–10 | 3005 | 1619–4391 | 3.9197 | 2.1201–5.7192 |
10–15 | 6666 | 5284–8047 | 7.8952 | 7.1978–8.5925 |
15–20 | 58,335 | 51,904–64,766 | 56.2213 | 54.8779–57.5647 |
20–25 | 97,491 | 86,176–108,805 | 83.3704 | 69.7977–96.9431 |
25–30 | 79,469 | 74,453–84,486 | 80.3324 | 71.4972–89.1677 |
30–35 | 67,689 | 56,899–78,479 | 68.0293 | 61.3837–74.6749 |
35–40 | 69,529 | 57,746–81,312 | 59.7398 | 51.3281–68.1515 |
40–45 | 76,188 | 67,365–85,011 | 66.8514 | 57.3751–76.3276 |
45–50 | 74,451 | 71,112–77,790 | 73.0433 | 64.3025–81.7842 |
50–55 | 80,333 | 73,185–87,480 | 95.4184 | 87.3205–103.5163 |
55–60 | 78,587 | 72,486–84,689 | 101.3584 | 88.3025–114.4143 |
60–65 | 81,710 | 78,840–84,580 | 146.2743 | 131.4336–161.1151 |
65–70 | 71,538 | 67,286–75,789 | 170.6339 | 154.6441–186.6238 |
70–75 | 65,196 | 58,412–71,981 | 201.5498 | 172.0765–231.0232 |
75–80 | 44,653 | 41,831–47,474 | 195.5469 | 168.0045–223.0892 |
80–85 | 21,985 | 20,706–23,264 | 175.2472 | 151.4695–199.0249 |
85+ | 8164 | 7339–8988 | 163.7941 | 126.7235–200.8647 |
Case Number | Age Group | Cluster | Distance | Case Number | Age Group | Cluster | Distance |
1 | 0–5 | 1 | 6.846 | 10 | 45–50 | 2 | 30.071 |
2 | 5–10 | 1 | 5.865 | 11 | 50–55 | 2 | 93.997 |
3 | 10–15 | 1 | 11.941 | 12 | 55–60 | 2 | 106.104 |
4 | 15–20 | 2 | 90.778 | 13 | 60–65 | 3 | 133.339 |
5 | 20–25 | 2 | 51.670 | 14 | 65–70 | 3 | 58.259 |
6 | 25–30 | 2 | 32.120 | 15 | 70–75 | 3 | 106.789 |
7 | 30–35 | 2 | 33.865 | 16 | 75–80 | 3 | 80.648 |
8 | 35–40 | 2 | 63.602 | 17 | 80–85 | 3 | 32.058 |
9 | 40–45 | 2 | 40.032 |
Parameter | Value | Source | Parameter | Value | Source |
A | 1.644×107 | [18] | θ2 | 0.0067 | [19] |
ρ | 1 | [20] | a1 | 12 × 365 | [23] |
ω | 0.728 | Fitting | a2 | 10 × 365 | [23] |
μ1 | 0.0017 | [19] | a3 | 8 × 365 | [23] |
μ2 | 0.0023 | [19] | β1 | 1.325 × 10-4 | Fitting |
μ3 | 0.0367 | [19] | β2 | 7.402 × 10-5 | Fitting |
di | 0.0025 | [4] | β3 | 4.690 × 10-4 | Fitting |
γi | 0.496 | [22] | ε1 | 0.4 | Assumption |
σi | 6 | [21] | ε2 | 0.3 | Assumption |
θ1 | 0.079 | [19] | ε3 | 0.3 | Assumption |
Year | 2004 | 2005 | 2006 | 2007 | 2008 | 2009 | 2010 | 2011 | 2012 |
0–15 | 24,247 | 26,048 | 20,735 | 17,972 | 16,011 | 12,320 | 9751 | 8275 | 8058 |
15–60 | 688,080 | 881,944 | 795,790 | 811,150 | 814,952 | 759,035 | 705,680 | 679,621 | 662,458 |
60+ | 257,952 | 351,316 | 311,046 | 334,837 | 338,577 | 305,583 | 275,919 | 265,379 | 280,992 |
Sum | 970,279 | 1,259,308 | 1,127,571 | 1,163,959 | 116, 9540 | 1,076,938 | 991,350 | 953,275 | 951,508 |
Year | 2013 | 2014 | 2015 | 2016 | 2017 | 2018 | 2019 | 2020 | 2021 |
0–15 | 7070 | 6695 | 6861 | 6769 | 7037 | 7591 | 8116 | ||
15–60 | 626,042 | 606,179 | 575,854 | 553,732 | 543,950 | 526,626 | 499,140 | ||
60+ | 271,322 | 276,507 | 281,300 | 275,735 | 284,206 | 289,125 | 268,508 | ||
Sum | 904,434 | 889,381 | 864,015 | 836,236 | 835,193 | 823,342 | 775,764 | 670,538 | 639,548 |
Year | 2022 | 2023 | 2024 | 2025 | 2026 | 2027 | 2028 | |
Cases | ω = 0.8 | 595,736 | 552,311 | 514,403 | 480,536 | 449,676 | 421,138 | 394,438 |
ω = 0.9 | 595,663 | 551,990 | 513,698 | 479,352 | 447,964 | 418,866 | 391,609 | |
ω = 0.95 | 595,626 | 551,830 | 513,347 | 478,765 | 447,116 | 417,747 | 390,221 | |
Year | 2029 | 2030 | 2031 | 2032 | 2033 | 2034 | 2035 | |
Cases | ω = 0.8 | 369,259 | 345,381 | 322,660 | 300,998 | 280,332 | 260,619 | 241,832 |
ω = 0.9 | 365,888 | 341,499 | 318,303 | 296,209 | 275,157 | 255,106 | 236,028 | |
ω = 0.95 | 364,242 | 339,610 | 316,192 | 293,899 | 272,671 | 252,468 | 233,262 |