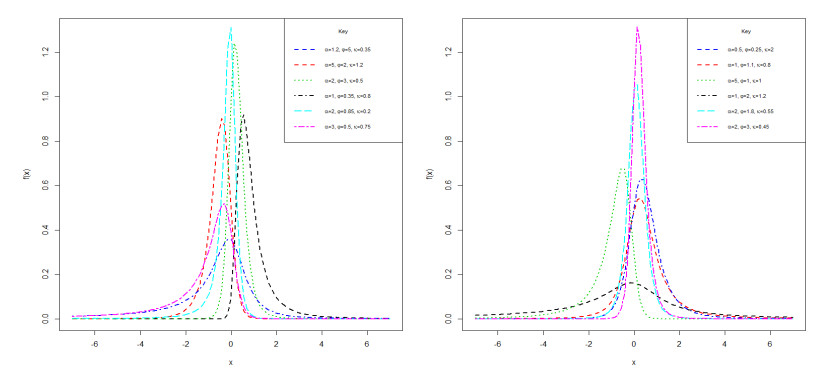
Most of the existing research on enterprise tax arrears prediction is based on the financial situation of enterprises. The influence of various relationships among enterprises on tax arrears is not considered. This paper integrates multivariate data to construct an enterprise knowledge graph. Then, the correlations between different enterprises and risk events are selected as the prediction variables from the knowledge graph. Finally, a tax arrears prediction machine learning model is constructed and implemented with better prediction power than earlier studies. The results show that the correlations between enterprises and tax arrears events through the same telephone number, the same E-mail address and the same legal person commonly exist. Based on these correlations, potential tax arrears can be effectively predicted by the machine learning model. A new method of tax arrears prediction is established, which provides new ideas and analysis frameworks for tax management practice.
Citation: Jie Zheng, Yijun Li. Machine learning model of tax arrears prediction based on knowledge graph[J]. Electronic Research Archive, 2023, 31(7): 4057-4076. doi: 10.3934/era.2023206
[1] | Nitesha Dwarika . The risk-return relationship in South Africa: tail optimization of the GARCH-M approach. Data Science in Finance and Economics, 2022, 2(4): 391-415. doi: 10.3934/DSFE.2022020 |
[2] | Moses Khumalo, Hopolang Mashele, Modisane Seitshiro . Quantification of the stock market value at risk by using FIAPARCH, HYGARCH and FIGARCH models. Data Science in Finance and Economics, 2023, 3(4): 380-400. doi: 10.3934/DSFE.2023022 |
[3] | Xiaoling Chen, Xingfa Zhang, Yuan Li, Qiang Xiong . Daily LGARCH model estimation using high frequency data. Data Science in Finance and Economics, 2021, 1(2): 165-179. doi: 10.3934/DSFE.2021009 |
[4] | Samuel Asante Gyamerah, Collins Abaitey . Modelling and forecasting the volatility of bitcoin futures: the role of distributional assumption in GARCH models. Data Science in Finance and Economics, 2022, 2(3): 321-334. doi: 10.3934/DSFE.2022016 |
[5] | Changlin Wang . Different GARCH model analysis on returns and volatility in Bitcoin. Data Science in Finance and Economics, 2021, 1(1): 37-59. doi: 10.3934/DSFE.2021003 |
[6] | Alexandra Piryatinska, Boris Darkhovsky . Retrospective technology of segmentation and classification for GARCH models based on the concept of the ϵ-complexity of continuous functions. Data Science in Finance and Economics, 2022, 2(3): 237-253. doi: 10.3934/DSFE.2022012 |
[7] | Kasra Pourkermani . VaR calculation by binary response models. Data Science in Finance and Economics, 2024, 4(3): 350-361. doi: 10.3934/DSFE.2024015 |
[8] | Changlin Wang . Correction: Different GARCH models analysis of returns and volatility in Bitcoin. Data Science in Finance and Economics, 2022, 2(3): 205-208. doi: 10.3934/DSFE.2022010 |
[9] | Deniz Sevinç . Volatility spillovers among MIST stock markets. Data Science in Finance and Economics, 2022, 2(2): 80-95. doi: 10.3934/DSFE.2022004 |
[10] | Katleho Makatjane, Ntebogang Moroke . Examining stylized facts and trends of FTSE/JSE TOP40: a parametric and Non-Parametric approach. Data Science in Finance and Economics, 2022, 2(3): 294-320. doi: 10.3934/DSFE.2022015 |
Most of the existing research on enterprise tax arrears prediction is based on the financial situation of enterprises. The influence of various relationships among enterprises on tax arrears is not considered. This paper integrates multivariate data to construct an enterprise knowledge graph. Then, the correlations between different enterprises and risk events are selected as the prediction variables from the knowledge graph. Finally, a tax arrears prediction machine learning model is constructed and implemented with better prediction power than earlier studies. The results show that the correlations between enterprises and tax arrears events through the same telephone number, the same E-mail address and the same legal person commonly exist. Based on these correlations, potential tax arrears can be effectively predicted by the machine learning model. A new method of tax arrears prediction is established, which provides new ideas and analysis frameworks for tax management practice.
Asymmetric datasets modeling utilizing generalized continuous distributions is quite an interesting area of research in recent times, and quite a number of generalized continuous distributions have been proposed, established and utilized in different fields of study. Besides with the new progressions in probability distribution theory, more vigorous forms of continuous distributions have been proposed utilizing the exponentiated method, transformer-transformer method, beta-generated method and gamma-generated method, among others. Shockingly, these recent distributions have been valuable in resolving issues in different fields of study other than in GARCH-type volatility models. Along these lines, it is important to develop new conditional innovation distributions capable of handling both significant skewness and excess kurtosis present in financial asset returns. Jones (2001) proposed a simplified extension of the symmetric Student's-t distribution known as the skew-t distribution to handle real-life asymmetric datasets. Jones and Faddy (2003) described the distribution as a simplified skew-t distribution and provided some important structural properties. Some other forms of the skew-t distribution that have been developed can be found in the literature (Johnson et al., 1995; Sahu et al., 2003; Azzalini and Capitanio, 2003). More so, the statistical literature consists several extended forms of the skew-t distribution. For example, the generalized hyperbolic skew-t distribution (Aas and Haff, 2006), beta skew-t distribution (Shittu et al., 2014), Balakrishnan skew-t distribution (Shafiei and Doostparast, 2014), Kumaraswamy skew-t distribution (Khamis et al., 2017), exponentiated skew-t distribution (Dikko and Agboola, 2017), generalized alpha skew-t distribution (Altun et al., 2018), odd exponentiated skew-t distribution (Adubisi et al., 2021a), and type I half-logistic skew-t distribution (Adubisi et al., 2021b).
For any baseline distribution with parameter vector ζ, Cordeiro et al. (2014) proposed the exponentiated half-logistic-G (EHL-G) family based on the T-X method (Alzaatreh et al., 2013). Given that k(t) and K(t) are the probability density function (pdf) and cumulative distribution function (cdf) of a random variable (r.v) T∈[c,d] for [c,d]∈(−∞,+∞), respectively. Let R[H(y;ζ)] be a function of the cdf of another r. v Y satisfying the following conditions: [i] R[H(y;ζ)]∈[c,d]; [ii] R[H(y;ζ)]→c as y→−∞ and R[H(y;ζ)]→d as y→∞; and [iii] R[H(y;ζ)] is differentiable and monotonically non-decreasing. The cdf of the T-X method is given by
F(y)=∫R[H(y;ζ)]ck(t)dt=K(R[H(y;ζ)]), | (1) |
Setting k(t)=2αφe−αt[1−e−αt]φ−1(1+e−αt)φ+1,t>0, where α,φ>0 are the shape parameters, and
21R[H(y;ζ)]=− log [1−H(y;ζ)], the cdf of the EHL-G family is given by
F(y;α,φ,ζ)={1−[1−H(y;ζ)]α1+[1−H(y;ζ)]α}φ, | (2) |
and the corresponding pdf to Equation 2 is
f(y;α,φ,ζ)=2αφh(y;ζ)[1−H(y;ζ)]α−1{1−[1−H(y;ζ)]α}φ−1{1+[1−H(y;ζ)]α}φ+1, | (3) |
where α,φ>0 are two additional shape parameters. H(y;ζ) and h(y;ζ) are the baseline cdf and pdf.
In this research work, a hybrid form of the skew-t distribution developed by Jones and Faddy (2003) using the exponentiated half logistic-G family of distributions is proposed. The new three-parameter model called the exponentiated half logistic skew t (EHLST) distribution appropriate for modeling right or left skewed and heavy-tailed datasets is developed. By inserting the skew-t cdf H(y;κ)=12(1+y√κ+y2) into Equation 2 leads to
F(y)={1−[1−(12(1+y√κ+y2))]α1+[1−(12(1+y√κ+y2))]α}φ, | (4) |
The corresponding pdf to Equation 4 is
f(y)=2αφκ[1−(12(1+y√κ+y2))]α−1{1−[1−(12(1+y√κ+y2))]α}φ−12(κ+y2)32{1+[1−(12(1+y√κ+y2))]α}φ+1, | (5) |
where α>0 and φ>0 are two shape parameters, and κ denote the skew parameter. From now onward, Y∼EHLST(α,φ,κ) denotes a random variable with pdf Equation 5. The hazard rate function (hrf) of Y is
η(y)=αφ(κ(κ+y2)32)[1−(12(1+y√κ+y2))]α−1{1−[1−(12(1+y√κ+y2))]α}φ−1({1+[1−(12(1+y√κ+y2))]α}φ−{1−[1−(12(1+y√κ+y2))]α}φ{1+[1−(12(1+y√κ+y2))]α}). | (6) |
Detailed properties of the EHLST model can be found in Adubisi et al. (2021c). Lately, there has been a key interest in the comparison of different estimation procedures for the parameter estimation of several distributions. For example, the extended exponential geometric (Louzada et al., 2016), Binomial exponential 2 (Bakouch et al., 2017), Poisson exponential (Rodrigues et al., 2018), type-I half-logistic Top-Leone (ZeinEldin et al., 2019), polynomial exponential (Chesneau et al., 2020), Fréchet (Ramos et al., 2020), odd exponential half-logistic exponential (Aldahlan and Afify, 2020), among others. The motivation in developing the new model is to create a more flexible heavy-tailed distribution with right-skewed, left-skewed, symmetric and unimodal features capable of handling the stylized features in financial datasets. The aim of this work is to estimate the parameters of the EHLST distribution using different frequentist estimation criterions such as the maximum likelihood, Anderson-Darling, maximum product of spacing, ordinary least squares, Cramer-von mises and weighted least squares. These estimation criterions using extensive Monte Carlo simulation are compared to discourse their performance. Also, Altun (2019) noted that the assumption of the conditional innovation density of the GARCH volatility model directly impacts on the accuracy of volatility predictions. Hence, the standardized form of the EHLST model is also derived to serve as an alternative conditional innovation density in modeling and forecasting the volatility of the inflation rates in sub-Sahara Africa, specifically Nigeria inflation rate using the GARCH-type volatility models. The rest of this research work is designed as follows. The quantile function of the EHLST is derived in Section 2. Six estimation criterions for estimating the parameters of the EHLST model are presented in Section 3. Simulation study to compare these criterions and real-life application are presented in Section 4. The application of the EHLST density in volatility modeling is illustrated via GARCH-type models in Section 5. The empirical results of the GARCH process are presented in Section 6. Conclusion of the work in Section 7.
Figures 1 and 2 provides plots of the pdf and hazard rate function of the EHLST distribution for selected values of the parameters. The plot in figure 2, shows that the hazard rate function can be J-shape, increasing and decreasing.
The quantile function, Q(u)=F−1(u) of Y can be derived by inverting Equation 4. The quantile function of the EHLST distribution takes the form:
Q(u)=κ12[1−2(1−u1φ1+u1φ)1α][1−(1−2(1−u1φ1+u1φ)1α)2]12,0≤u≤1. | (7) |
The quantile function in Equation 7 can define various quantile measures such as Bowley's skewness and Moor's kurtosis of the EHLST distribution. Figure 3 depicts the three-dimensional plots of these skewness and kurtosis measures. It is clear that the skewness was increasing in φ and decreasing in α while the kurtosis is increasing in α and decreasing in φ, and were independent of κ.
This section discusses six estimation criterions, these are the maximum likelihood (ML), maximum product of spacing (MPS), Anderson-Darling (ANDA), ordinary least squares (OLS), weighted least squares (WLS) and Cramer-von Mises (CVM) to estimate the new model parameters.
The ML estimation is considered the best for estimating distribution parameters given its excellent characteristics. Let y1,…,yT be the observed values of sizes (T) from the EHLST distribution. The log-likelihood function derived from Equation 6 is given by
l(α,φ,κ)=T ln α+T ln φ+T ln κ−32T∑k=1 ln (κ+y2k)+(α−1)T∑k=1 ln (zk)+(φ−1)T∑k=1 ln (1−(zk)α)−(φ+1)∑Tk=1 ln (1+(zk)α) | (8) |
where zk=(1−12(1+yk√κ+y2k)) | (9) |
Let ˆαMLE,ˆφMLE and ˆκMLE be the ML estimates of α,φ and κ. These can be obtained numerically by maximizing l(α,φ,κ) or solving the following differential equations:
Uα=Tα+∑Tk=1 ln (zk)−(φ−1)∑Tk=1(zk)α ln (zk){1−(zk)α}−(φ+1)∑Tk=1(zk)α ln (zk){1+(zk)α}=0, | (10) |
Uφ=Tφ+∑Tk=1 ln (1−(zk)α)−∑Tk=1 ln {1+(zk)α}=0, | (11) |
and
Uη=Tκ−32T∑k=11(κ+y2k)+(α−1)T∑k=1yk4(κ+y2k)32(zk)−α(φ−1)T∑k=1yk(zk)α4(κ+y2k)32(zk){1+(zk)α}−α(φ+1)∑Tk=1yk(zk)α4(κ+y2k)32(zk){1+(zk)α}=0 | (12) |
Let y(1:T),y(2:T),…,y(T:T) be the ordered sample of size (T) from the EHLST distribution with cdf in Equation 5. The OLS estimates ˆαOLS,ˆφOLS and ˆκOLS of α,φ and κ can be obtained numerically by minimizing
OL(α,φ,κ)=∑Tk=1[{1−[1−(12(1+y(k:T)√κ+y2(k:T)))]α1+[1−(12(1+y(k:T)√κ+y2(k:T)))]α}φ−℘(k,T)]2, | (13) |
where ℘(k,T)=(T+1−k)(T+1), with respect to α,φ and κ.Also, the model estimates can be obtained by solving the following differential equations:
∂OL(α,φ,κ)∂α=∑Tk=1[{1−[z(k:T)]α1+[z(k:T)]α}φ−℘(k,T)]Φ1(y(k:T)|α,φ,κ)=0, | (14) |
∂OL(α,φ,κ)∂φ=∑Tk=1[{1−[z(k:T)]α1+[z(k:T)]α}φ−℘(k,T)]Φ2(y(k:T)|α,φ,κ)=0, | (15) |
and
∂OL(α,φ,κ)∂κ=∑Tk=1[{1−[z(k:T)]α1+[z(k:T)]α}φ−℘(k,T)]Φ3(y(k:T)|α,φ,κ)=0, | (16) |
where z(k:T) is the order transformed value of zk given by Equation 9, and
Φ1(y(k:T ) |α,φ,κ)={(1−(z(k:T ) )α1+(z(k:T ) )α)φφ(−(z(k:T ) )α log (z(k:T ) )1+(z(k:T ) )α−[1−(z(k:T ) )α ](z(k:T ) )α log (z(k:T ) )[1+(z(k:T ) )α ] 2)[1+(z(k:T ) )α ]}1−(z(k:T ) )α | (17) |
Φ2(y(k:T ) |α,φ,κ)=(1−(z(k:T ) )α1+(z(k:T ) )α)φ log (1−(z(k:T ) )α1+(z(k:T ) )α) | (18) |
and
Φ3(y(k:T ) |α,φ,κ)={(1−(z(k:T ) )α1+(z(k:T ) )α)φφ(−αy(z(k:T ) )α4(κ+y2(k:T ) )32(z(k:T ) )[1+(z(k:T ) )α ]−αy[1−(z(k:T ) )α ](z(k:T ) )α4[1+(z(k:T ) )α ]2(κ+y2(k:T ) )32(z(k:T ) ))[1+(z(k:T ) )α ]}1−(z(k:T ) )α | (19) |
Applying the same symbolization as in the preceding subsection, the WLS estimates ˆαWLS,ˆφWLS and ˆκWLS of α,φ and κ can be obtained numerically by minimizing
WL(α,φ,κ)=∑Tk=1Ψ(k,T)[{1−[1−(12(1+y(k:T ) √κ+y2(k:T ) )) ]α1+[1−(12(1+y(k:T ) √κ+y2(k:T ) )) ]α}φ−℘(k,T) ]2, | (20) |
where Ψ(k,T)=(T+1)2(T+2)k(T−k+1), relative to α,φ and κ. Also, the model estimates can be obtained by solving the following differential equations:
∂WL(α,φ,κ)∂α=∑Tk=1Ψ(k,T)[{1−[z(k:T)]α1+[z(k:T)]α}φ−℘(k,T)]Φ1(y(k:T)|α,φ,κ)=0, | (21) |
∂WL(α,φ,κ)∂φ=∑Tk=1Ψ(k,T)[{1−[z(k:T)]α1+[z(k:T)]α}φ−℘(k,T)]Φ2(y(k:T)|α,φ,κ)=0, | (22) |
and
∂OL(α,φ,κ)∂κ=∑Tk=1Ψ(k,T)[{1−[z(k:T)]α1+[z(k:T)]α}φ−℘(k,T)]Φ3(y(k:T)|α,φ,κ)=0, | (23) |
where z(k:T) and Φi(y(k:T)|α,φ,κ), i=1,2,3 are given by Eqs 9, 17, 18 and 19, respectively.
Cheng and Amin(1979, 1983) proposed the MPS estimator for the estimation of unknown parameters with ordered sample y(1:T),y(2:T),…,y(T:T) from the EHLST distribution and uniform spacing for this random sample is given by:
Dk(α,φ,κ)=F(y(k:T)|α,φ,κ)−F(y(k−1:T)|α,φ,κ), for k=1,2,…,T, | (24) |
where F(y(0:T)|α,φ,κ)=0 and F(y(T+1:T)|α,φ,κ)=1. The MPS estimates ˆαMPS, ˆφMPS and ˆκMPS of α,φ, and κ can be obtained by maximizing
MP(α,φ,κ)=1T+1∑T+1k=1 log ⟨Dk(α,φ,κ)⟩ | (25) |
relative to α,φ, and κ. Also, the estimates can be obtained by solving the following differential equations:
∂MP(α,φ,κ)∂α=∑T+1k=11Dk(α,φ,κ)⟨Φ1(y(k:T)|α,φ,κ)−Φ1(y(k−1:T)|α,φ,κ)⟩=0, | (26) |
∂MP(α,φ,κ)∂φ=∑T+1k=11Dk(α,φ,κ)⟨Φ2(y(k:T)|α,φ,κ)−Φ2(y(k−1:T)|α,φ,κ)⟩=0, | (27) |
and
∂MP(α,φ,κ)∂κ=∑T+1k=11Dk(α,φ,κ)⟨Φ3(y(k:T)|α,φ,κ)−Φ3(y(k−1:T)|α,φ,κ)⟩=0 | (28) |
where Φi(.|α,φ,κ), i=1,2,3 are specified in Equation s 17, 18 and 19, respectively.
The ANDA estimates ˆαANDA, ˆφANDA and ˆκANDA of the EHLST parameters can be obtained by minimizing relative to α,φ, and κ, the function
AD(α,φ,κ)=−T−1T∑Tk=1(2k−1)⟨ log [F(y(k:T)|α,φ,κ)]+ log [ˉF(y(T+1−k:n)|α,φ,κ)]⟩, | (29) |
Also, estimates obtained by solving the following nonlinear equations:
∂AD(α,φ,κ)∂α=∑Tk=1(2k−1)[Φ1(y(k:T ) |α,φ,κ)F(y(k:T ) |α,φ,κ)−Φ1(y(T+1−k:T ) |α,φ,κ)ˉF(y(T+1−k:T ) |α,φ,κ)]=0, | (30) |
∂AD(α,φ,κ)∂φ=∑Tk=1(2k−1)[Φ2(y(k:T ) |α,φ,κ)F(y(k:T ) |α,φ,κ)−Φ2(y(T+1−k:T ) |α,φ,κ)ˉF(y(T+1−k:T ) |α,φ,κ)]=0, | (31) |
and
∂AD(α,φ,κ)∂κ=∑Tk=1(2k−1)[Φ3(y(k:T ) |α,φ,κ)F(y(k:T ) |α,φ,κ)−Φ3(y(T+1−k:T ) |α,φ,κ)ˉF(y(T+1−k:T ) |α,φ,κ)]=0, | (32) |
where Φi(.|α,φ,κ), i=1,2,3 are specified in Eqs 17, 18 and 19, respectively.
The CVM estimates ˆαCVM, ˆφCVM and ˆκCVM of the EHLST parameters can be obtained by minimizing relative to α,φ, and κ, the function
CV(α,φ,κ)=112T+∑Tk=1[{1−[1−(12(1+y(k:T ) √κ+y2(k:T ) ))]α1+[1−(12(1+y(k:T ) √κ+y2(k:T ) ))]α}φ−{2(k−1)+12T}]2, | (33) |
Solving the following nonlinear equations, the CVM estimates can also be obtained as follows:
∂OL(α,φ,κ)∂α=∑Tk=1[{1−[z(k:T)]α1+[z(k:T)]α}φ−{2(k−1)+12T}]Φ1(y(k:T)|α,φ,κ)=0, | (34) |
∂OL(α,φ,κ)∂φ=∑Tk=1[{1−[z(k:T)]α1+[z(k:T)]α}φ−{2(k−1)+12T}]Φ2(y(k:T)|α,φ,κ)=0, | (35) |
and
∂OL(α,φ,κ)∂κ=∑Tk=1[{1−[z(k:T)]α1+[z(k:T)]α}φ−{2(k−1)+12T}]Φ3(y(k:T)|α,φ,κ)=0, | (36) |
where z(k:T) and Φi(y(k:T)|α,φ,κ), i=1,2,3 are given by Eqs 9, 17, 18 and 19, respectively.
The performance of the six estimators defined in Section 5 are compared using Monte Carlo simulations. The parameter combinations (Comb), i.e., Comb 1 (α=1.0,φ=1.3,κ=0.5), Comb 2 (α=1.3,φ=1.7,κ=1.0), Comb 3 (α=2.0,φ=2.5,κ=1.5), and Comb 4 (α=2.5,φ=3.0,κ=2.0). The datasets are produced from the EHLST distribution under these combinations by selecting n = 10, 50,100 and 200. For each process, 1000 random samples from the EHLST distribution are produced using the quantile function in Eq 6. The parameter estimates performance are evaluated through the average values (AVEs), average absolute biases (AVABs), mean square errors (MSEs) and root mean square errors (RMSEs) for the different sample sizes (n). For the estimates, the AVAB, MSE and RMSE are computed using
AVAB(ˆϑi)=110001000∑j=1|ˆϑi,j−ϑi|,MSE(ˆϑi)=110001000∑j=1(ˆϑi,j−ϑi)2, |
RMSE(ˆϑi)=√110001000∑j=1(ˆϑi,j−ϑi)2 |
where, ˆϑ=(ˆα,ˆφ,ˆκ) and ϑ=(α,φ,κ). The partial and overall ranks of the six estimators for the combinations to determine the best method for estimating the EHLST parameters are provided in Table 1. Based on the results, it is realistic to use the ML and MPS methods for estimating the unknown parameters of the EHLST distribution.
Parameter (pa) | n | ML | MPS | ANDA | CVM | OLS | WLS |
α=1.0,φ=1.3,κ=0.5 | 10 | 2 | 5 | 6 | 4 | 1 | 3 |
50 | 2 | 1 | 6 | 5 | 4 | 3 | |
100 | 2 | 1 | 4 | 6 | 5 | 3 | |
200 | 3 | 1 | 2 | 6 | 5 | 4 | |
α=1.3,φ=1.7,κ=1.0 | 10 | 2 | 5 | 6 | 4 | 3 | 1 |
50 | 2 | 1 | 4.5 | 6 | 4.5 | 3 | |
100 | 3 | 1 | 2 | 6 | 5 | 4 | |
200 | 3 | 1 | 2 | 6 | 5 | 4 | |
α=2.0,φ=2.5,κ=1.5 | 10 | 1 | 5 | 6 | 4 | 2 | 3 |
50 | 2 | 1 | 3 | 6 | 5 | 4 | |
100 | 2 | 1 | 3 | 6 | 4 | 5 | |
200 | 2 | 1 | 3 | 6 | 5 | 4 | |
α=2.5,φ=3.0,κ=2.0 | 10 | 1 | 5 | 6 | 4 | 2 | 3 |
50 | 1.5 | 1.5 | 6 | 6 | 3 | 4 | |
100 | 1.5 | 1.5 | 5 | 6 | 3 | 4 | |
200 | 2 | 1 | 3 | 6 | 6 | 4 | |
∑ranks | 32 | 33 | 67.5 | 87 | 62.5 | 56 | |
Overall rank | 1 | 2 | 5 | 6 | 4 | 3 |
Tables 2–5 provides the AVEs, AVABs, MSEs and RMSEs of the six estimators. More so, these tables show the rank of each estimator among all the estimators in each row, the superscripts are the rank indicators and ∑ranks is the partial sum of the ranks for each column and sample size (n).
n | Measures | Pa. | ML | MPS | ANDA | CVM | OLS | WLS |
10 | AVE | α | 1.4661(5) | 1.4066(4) | 1.6544(6) | 1.3840(3) | 1.1710(2) | 1.1590(1) |
φ | 2.4233(3) | 4.8935(5) | 8.1802(6) | 2.6132(4) | 1.9540(1) | 2.0060(2) | ||
κ | 1.1683(1) | 2.3008(5) | 2.4332(6) | 1.3781(4) | 1.2180(2) | 1.2700(3) | ||
AVAB | α | 0.4661(5) | 0.4066(4) | 0.6544(6) | 0.3840(3) | 0.1713(2) | 0.1594(1) | |
φ | 1.1233(3) | 3.5935(5) | 6.8802(6) | 1.3132(4) | 0.6536(1) | 0.7063(2) | ||
κ | 0.6683(1) | 1.8008(5) | 1.9332(6) | 0.8781(4) | 0.7178(2) | 0.7701(3) | ||
MSE | α | 0.8648(3) | 2.3453(5) | 3.3241(6) | 1.0758(4) | 0.6714(1) | 0.7021(2) | |
φ | 4.1873(1) | 172.4338(5) | 472.9212(6) | 19.7630(4) | 15.0648(2) | 15.7997(3) | ||
κ | 2.4706(1) | 35.4217(5) | 36.2999(6) | 6.9764(3) | 5.3805(2) | 7.9437(4) | ||
RMSE | α | 0.9300(3) | 1.5314(5) | 1.8232(6) | 1.0372(4) | 0.8194(1) | 0.8379(2) | |
φ | 2.0463(1) | 13.1314(5) | 21.7468(6) | 4.4456(4) | 3.8813(2) | 3.9749(3) | ||
κ | 1.5718(1) | 5.9516(6) | 6.0249(7) | 2.6413(3) | 2.3196(2) | 2.8185(4) | ||
∑ranks | 28(2) | 49(5) | 73(6) | 44(4) | 20(1) | 29(3) | ||
50 | AVE | α | 1.1687(5) | 0.9853(1) | 1.1595(3) | 1.2278(6) | 1.1672(4) | 1.1501(2) |
φ | 1.5906(2) | 1.3920(1) | 2.1469(6) | 1.8565(5) | 1.7203(4) | 1.6610(3) | ||
κ | 0.7185(2) | 0.6255(1) | 0.8535(5) | 0.8632(6) | 0.8289(4) | 0.7911(3) | ||
AVAB | α | 0.1687(5) | 0.0147(1) | 0.1595(3) | 0.2278(6) | 0.1672(4) | 0.1501(2) | |
φ | 0.2906(2) | 0.0920(1) | 0.8469(6) | 0.5565(5) | 0.4203(4) | 0.3610(3) | ||
κ | 0.2185(2) | 0.1255(1) | 0.3535(4) | 0.3632(5) | 1.3812(6) | 0.2911(3) | ||
MSE | α | 0.2171(1) | 0.2513(2) | 0.5655(6) | 0.4314(5) | 0.3657(4) | 0.3091(3) | |
φ | 0.5725(1) | 2.5172(2) | 38.3736(6) | 3.8641(3) | 3.9756(4) | 4.4224(5) | ||
κ | 0.3991(1) | 0.9812(2) | 3.3622(6) | 1.5146(4) | 1.3812(3) | 1.8538(5) | ||
RMSE | α | 0.4659(1) | 0.5013(2) | 0.7520(6) | 0.6568(5) | 0.6047(4) | 0.5559(3) | |
φ | 0.7567(1) | 1.5866(2) | 6.1946(6) | 1.9657(3) | 1.9939(4) | 2.1029(5) | ||
κ | 0.6317(1) | 0.9905(2) | 1.8336(6) | 1.2307(4) | 1.1752(3) | 1.3616(5) | ||
∑ranks | 24(2) | 18(1) | 63(6) | 57(5) | 48(4) | 42(3) | ||
100 | AVE | α | 1.0768(4) | 0.9461(1) | 1.0561(2) | 1.1320(6) | 1.0928(5) | 1.0652(3) |
φ | 1.4224(2) | 1.2463(1) | 1.5157(4) | 1.6517(6) | 1.5443(5) | 1.4523(3) | ||
κ | 0.6022(2) | 0.4996(1) | 0.6226(4) | 0.7279(6) | 0.6878(5) | 0.6203(3) | ||
AVAB | α | 0.0768(4) | 0.0539(1) | 0.0561(2) | 0.1320(6) | 0.0928(5) | 0.0652(3) | |
φ | 0.1224(2) | 0.0537(1) | 0.2157(4) | 0.3517(6) | 0.2443(5) | 0.1523(3) | ||
κ | 0.1022(2) | 0.0004(1) | 0.1226(5) | 0.2279(6) | 0.1878(3) | 0.1203(4) | ||
MSE | α | 0.0831(2) | 0.0682(1) | 0.1692(4) | 0.2889(6) | 0.2350(5) | 0.1393(3) | |
φ | 0.1652(2) | 0.1829(3) | 6.2211(6) | 3.6319(5) | 2.1739(4) | 1.4473(1) | ||
κ | 0.1402(1) | 0.1508(2) | 1.0192(5) | 1.1513(6) | 0.8198(4) | 0.3606(3) | ||
RMSE | α | 0.2882(2) | 0.2612(1) | 0.4113(4) | 0.5375(6) | 0.4848(5) | 0.3732(3) | |
φ | 0.4065(1) | 0.4277(2) | 2.4942(6) | 1.9058(5) | 1.4744(4) | 1.2030(3) | ||
κ | 0.3744(1) | 0.3883(2) | 1.0096(5) | 1.0730(6) | 0.9054(4) | 0.6005(3) | ||
∑ranks | 25(2) | 17(1) | 51(4) | 70(6) | 54(5) | 35(3) | ||
200 | AVE | α | 1.0360(4) | 0.9541(1) | 1.0240(2) | 1.0588(6) | 1.0415(5) | 1.0285(3) |
φ | 1.3576(3) | 1.2478(1) | 1.3482(2) | 1.4147(6) | 1.3846(5) | 1.3593(4) | ||
κ | 0.5436(3) | 0.4775(1) | 0.5384(2) | 0.5821(6) | 0.5722(5) | 0.5493(4) | ||
AVAB | α | 0.0360(3) | 0.0459(5) | 0.0240(1) | 0.0588(6) | 0.0415(4) | 0.0285(2) | |
φ | 0.0576(3) | 0.0522(2) | 0.0482(1) | 0.1147(6) | 0.0846(5) | 0.0593(4) | ||
κ | 0.0436(3) | 0.0225(1) | 0.0384(2) | 0.0821(6) | 0.0722(5) | 0.0493(4) | ||
MSE | α | 0.0329(2) | 0.0270(1) | 0.0441(3) | 0.0917(6) | 0.0824(5) | 0.0567(4) | |
φ | 0.0624(2) | 0.0455(1) | 0.0849(3) | 0.2907(6) | 0.2382(5) | 0.1839(4) | ||
κ | 0.0417(2) | 0.0291(1) | 0.0552(3) | 0.1570(6) | 0.1399(5) | 0.0947(4) | ||
RMSE | α | 0.1813(2) | 0.1645(1) | 0.2101(3) | 0.3028(6) | 0.2871(5) | 0.2380(4) | |
φ | 0.2498(2) | 0.2133(1) | 0.2914(3) | 0.5392(6) | 0.4881(5) | 0.4289(4) | ||
κ | 0.2041(2) | 0.1706(1) | 0.2350(3) | 0.3963(6) | 0.3741(5) | 0.3077(4) | ||
∑ranks | 31(3) | 17(1) | 28(2) | 82(6) | 59(5) | 45(4) |
n | Measures | Pa. | ML | MPS | ANDA | CVM | OLS | WLS |
10 | AVE | α | 1.1024(3) | 1.1175(5) | 1.4136(6) | 1.0918(4) | 0.9769(2) | 0.9432(1) |
φ | 2.7852(4) | 5.2337(5) | 9.8213(6) | 2.7427(3) | 2.0152(2) | 1.8648(1) | ||
κ | 1.9428(1) | 4.5719(5) | 6.0877(6) | 2.7247(3) | 2.9305(4) | 2.4634(2) | ||
AVAB | α | 0.3024(4) | 0.3175(5) | 0.6136(6) | 0.2918(3) | 0.1769(2) | 0.1432(1) | |
φ | 1.2852(4) | 3.7337(5) | 8.3213(6) | 1.2427(3) | 0.5152(2) | 0.3648(1) | ||
κ | 0.9428(1) | 3.5719(5) | 5.0877(6) | 1.7247(3) | 1.9305(4) | 1.4634(2) | ||
MSE | α | 0.5045(3) | 1.4348(5) | 2.5202(6) | 0.5695(4) | 0.4175(2) | 0.3182(1) | |
φ | 7.3621(3) | 151.9961(5) | 634.1503(6) | 16.9030(4) | 6.2433(2) | 3.1990(1) | ||
κ | 7.4544(1) | 153.2414(5) | 298.9136(6) | 33.2122(3) | 40.8208(4) | 13.8077(2) | ||
RMSE | α | 0.7103(3) | 1.1978(5) | 1.5875(6) | 0.7546(4) | 0.6461(2) | 0.5641(1) | |
φ | 2.7133(3) | 12.3287(5) | 25.1823(6) | 4.1113(4) | 2.4987(2) | 1.7886(1) | ||
κ | 2.7303(1) | 12.3791(5) | 17.2891(6) | 5.7630(3) | 6.3891(4) | 3.7159(2) | ||
∑ranks | 31(2) | 60(5) | 72(6) | 41(4) | 32(3) | 16(1) | ||
50 | AVE | α | 0.8833(4) | 0.7896(1) | 0.8815(3) | 0.9182(6) | 0.8904(5) | 0.8811(2) |
φ | 1.6996(3) | 1.4800(1) | 1.8893(6) | 1.7904(5) | 1.7388(4) | 1.6990(2) | ||
κ | 1.2704(2) | 1.1696(1) | 1.4091(3) | 1.4440(5) | 1.4889(6) | 1.4167(4) | ||
AVAB | α | 0.0833(4) | 0.0104(1) | 0.0815(3) | 0.1182(6) | 0.0904(5) | 0.0811(2) | |
φ | 0.1996(3) | 0.0200(1) | 0.3893(6) | 0.2904(5) | 0.2388(4) | 0.1990(2) | ||
κ | 0.2704(2) | 0.1696(1) | 0.4091(3) | 0.4440(5) | 0.4889(6) | 0.4167(4) | ||
MSE | α | 0.0794(2) | 0.0681(1) | 0.1771(6) | 0.1309(4) | 0.1464(5) | 0.1199(3) | |
φ | 0.3262(2) | 0.3085(1) | 20.5402(6) | 0.7886(3) | 1.7903(5) | 1.0740(4) | ||
κ | 0.9907(1) | 1.1406(2) | 7.5480(6) | 1.9706(3) | 4.2142(5) | 3.7125(4) | ||
RMSE | α | 0.2819(2) | 0.2610(1) | 0.4209(6) | 0.3618(4) | 0.3826(5) | 0.3462(3) | |
φ | 0.5711(2) | 0.5554(1) | 4.5321(6) | 0.8880(3) | 1.3380(5) | 1.0363(4) | ||
κ | 0.9907(1) | 1.0680(2) | 2.7474(6) | 1.4038(3) | 2.0528(5) | 1.9268(4) | ||
∑ranks | 28(2) | 14(1) | 60(4.5) | 62(6) | 60(4.5) | 38(3) | ||
100 | AVE | α | 0.8324(5) | 0.7737(1) | 0.8214(2) | 0.8476(6) | 0.8292(4) | 0.8239(3) |
φ | 1.5765(5) | 1.4469(1) | 1.5593(3) | 1.6181(6) | 1.5676(4) | 1.5566(2) | ||
κ | 1.1040(3) | 1.0233(1) | 1.0901(2) | 1.1638(6) | 1.1483(5) | 1.1139(4) | ||
AVAB | α | 0.0324(5) | 0.0263(3) | 0.0214(1) | 0.0476(6) | 0.0292(4) | 0.0239(2) | |
φ | 0.0765(5) | 0.0531(1) | 0.0593(3) | 0.1181(6) | 0.0676(4) | 0.0566(2) | ||
κ | 0.1040(3) | 0.0233(1) | 0.0901(2) | 0.1638(6) | 0.1483(5) | 0.1139(4) | ||
MSE | α | 0.0258(2) | 0.0222(1) | 0.0298(3) | 0.0507(6) | 0.0445(5) | 0.0323(4) | |
φ | 0.0948(2) | 0.0745(1) | 0.1090(3) | 0.2038(6) | 0.1641(5) | 0.1151(4) | ||
κ | 0.2745(2) | 0.0233(1) | 0.2965(3) | 0.5554(6) | 0.4886(5) | 0.3328(4) | ||
RMSE | α | 0.1607(2) | 0.1491(1) | 0.1727(3) | 0.2251(6) | 0.2109(5) | 0.1797(4) | |
φ | 0.3079(2) | 0.2729(1) | 0.3301(3) | 0.4514(6) | 0.4051(5) | 0.3392(4) | ||
κ | 0.5240(2) | 0.4679(1) | 0.5445(3) | 0.7453(6) | 0.6990(5) | 0.5769(4) | ||
∑ranks | 38(3) | 14(1) | 31(2) | 72(6) | 56(5) | 41(4) | ||
200 | AVE | α | 0.8155(5) | 0.7798(1) | 0.8117(3) | 0.8206(6) | 0.8122(4) | 0.8102(2) |
φ | 1.5382(5) | 1.4621(1) | 1.5325(3) | 1.5549(6) | 1.5328(4) | 1.5277(2) | ||
κ | 1.0486(3) | 0.9959(1) | 1.0469(2) | 1.0692(6) | 1.0643(5) | 1.0491(4) | ||
AVAB | α | 0.0155(4) | 0.0202(5) | 0.0117(2) | 0.0206(6) | 0.0122(3) | 0.0102(1) | |
φ | 0.0382(5) | 0.0359(4) | 0.0325(2) | 0.0549(6) | 0.0328(3) | 0.0277(1) | ||
κ | 0.0486(3) | 0.0041(1) | 0.0469(2) | 0.0692(6) | 0.0643(5) | 0.0491(4) | ||
MSE | α | 0.0110(2) | 0.0109(1) | 0.0141(3) | 0.0204(6) | 0.0193(5) | 0.0144(4) | |
φ | 0.0380(2) | 0.0359(1) | 0.0460(3) | 0.0648(6) | 0.0597(5) | 0.0466(4) | ||
κ | 0.1041(2) | 0.0949(1) | 0.1258(3) | 0.1858(6) | 0.1794(5) | 0.1280(4) | ||
RMSE | α | 0.1049(1.5) | 0.1049(1.5) | 0.1186(3) | 0.1427(6) | 0.1390(5) | 0.1198(4) | |
φ | 0.1950(2) | 0.1894(1) | 0.2144(3) | 0.2546(6) | 0.2443(5) | 0.2158(4) | ||
κ | 0.3227(2) | 0.3080(1) | 0.3547(3) | 0.4310(6) | 0.4236(5) | 0.3578(4) | ||
∑ranks | 36.5(3) | 19.5(1) | 32(2) | 72(6) | 64(5) | 38(4) |
n | Measures | Pa. | ML | MPS | ANDA | CVM | OLS | WLS |
10 | AVE | α | 2.8336(3) | 2.5944(1) | 3.1558(5) | 3.3131(6) | 2.8120(2) | 2.9230(4) |
φ | 2.0036(1) | 8.0269(5) | 19.3064(6) | 5.4068(4) | 4.1680(2) | 4.4550(3) | ||
κ | 1.4579(1) | 3.2311(5) | 3.6263(6) | 3.0043(4) | 2.8950(2) | 2.9920(3) | ||
AVAB | α | 0.8336(3) | 0.5944(1) | 1.1558(5) | 1.3131(6) | 0.8124(2) | 0.9231(4) | |
φ | 1.0036(1) | 7.0269(5) | 18.3064(6) | 4.4068(4) | 3.1675(2) | 3.4554(3) | ||
κ | 0.7579(1) | 2.5311(5) | 2.9263(6) | 2.3043(4) | 2.1952(2) | 2.2918(3) | ||
MSE | α | 2.1274(1) | 6.7714(5) | 9.2849(6) | 5.0302(4) | 3.5630(2) | 3.9500(3) | |
φ | 3.3320(1) | 648.1073(5) | 3383.9749(6) | 204.2319(4) | 177.4580(3) | 152.0100(2) | ||
κ | 2.8070(1) | 49.8495(5) | 60.4505(6) | 28.6379(3) | 27.8840(2) | 33.0500(4) | ||
RMSE | α | 1.4586(1) | 2.6022(5) | 3.0471(6) | 2.2428(4) | 1.8870(2) | 1.9870(3) | |
φ | 1.8254(1) | 25.4580(5) | 58.1719(6) | 14.2910(4) | 13.3210(3) | 12.3290(2) | ||
κ | 1.6754(1) | 7.0404(5) | 7.7750(6) | 5.3514(3) | 5.2800(2) | 5.7490(4) | ||
∑ranks | 16(1) | 52(5) | 70(6) | 50(4) | 26(2) | 38(3) | ||
50 | AVE | α | 2.2547(3) | 1.8578(1) | 2.1777(2) | 2.5788(6) | 2.4490(5) | 2.3520(4) |
φ | 1.2645(2) | 1.0723(1) | 1.5965(3) | 2.3717(6) | 2.1870(5) | 2.0330(4) | ||
κ | 0.9587(2) | 0.7912(1) | 1.0157(3) | 1.4816(6) | 1.4490(5) | 1.2750(4) | ||
AVAB | α | 0.2547(3) | 0.1422(1) | 0.1777(2) | 0.5788(6) | 0.4486(5) | 0.3518(4) | |
φ | 0.2645(2) | 0.0723(1) | 0.5965(3) | 1.3717(6) | 1.1873(5) | 1.0332(4) | ||
κ | 0.2587(2) | 0.0912(1) | 0.3157(3) | 0.7816(6) | 0.7489(5) | 0.5751(4) | ||
MSE | α | 0.4982(1) | 0.6380(2) | 0.8915(3) | 1.6694(6) | 1.5060(5) | 1.1900(4) | |
φ | 0.4962(1) | 1.4375(2) | 32.6387(5) | 30.5760(4) | 28.1190(3) | 49.6000(6) | ||
κ | 0.5600(1) | 0.7722(2) | 1.7465(3) | 3.9829(5) | 4.1170(6) | 3.2910(4) | ||
RMSE | α | 0.7058(1) | 0.7988(2) | 0.9442(3) | 1.2921(6) | 1.2270(5) | 1.0910(4) | |
φ | 0.7044(1) | 1.1990(2) | 5.7130(5) | 5.5296(4) | 5.3030(3) | 7.0430(6) | ||
κ | 0.7483(1) | 0.8788(2) | 1.3215(3) | 1.9957(5) | 2.0290(6) | 1.8140(4) | ||
∑ranks | 20(2) | 18(1) | 28(3) | 64(6) | 58(5) | 52(4) | ||
100 | AVE | α | 2.1444(3) | 1.8589(1) | 2.0854(2) | 2.3221(6) | 2.2590(5) | 2.1566(4) |
φ | 1.1384(3) | 0.9579(1) | 1.1305(2) | 1.6180(5) | 1.5510(4) | 1.6937(6) | ||
κ | 0.1510(1) | 0.6947(2) | 0.8403(3) | 1.1163(6) | 1.0970(5) | 0.9608(4) | ||
AVAB | α | 0.1444(3) | 0.1411(2) | 0.0854(1) | 0.3221(6) | 0.2591(5) | 0.1566(4) | |
φ | 0.1384(3) | 0.0421(1) | 0.1305(2) | 0.6180(5) | 0.5507(4) | 0.6937(6) | ||
κ | 0.1510(3) | 0.0053(1) | 0.1403(2) | 0.4163(6) | 0.3975(5) | 0.2608(4) | ||
MSE | α | 0.2375(1) | 0.2656(2) | 0.3276(3) | 0.8324(6) | 0.7795(5) | 0.5226(4) | |
φ | 0.1731(2) | 0.1389(1) | 0.3441(3) | 9.3730(5) | 9.3187(4) | 168.1577(6) | ||
κ | 0.2335(2) | 0.2183(1) | 0.3689(3) | 1.5424(5) | 1.5302(4) | 1.6901(6) | ||
RMSE | α | 0.4873(1) | 0.5154(2) | 0.5724(3) | 0.9123(6) | 0.8829(5) | 0.7229(4) | |
φ | 0.4161(2) | 0.3728(1) | 0.5866(3) | 3.0615(5) | 3.0526(4) | 12.9676(6) | ||
κ | 0.4832(2) | 0.4672(1) | 0.6074(3) | 1.2420(5) | 1.2370(4) | 1.3001(6) | ||
∑ranks | 26(2) | 16(1) | 30(3) | 66(6) | 54(4) | 60(5) | ||
200 | AVE | α | 2.0655(4) | 1.8856(1) | 2.0368(2) | 2.1254(6) | 2.0920(5) | 2.0566(3) |
φ | 1.0615(3) | 0.9484(1) | 1.0528(2) | 1.1499(6) | 1.1250(5) | 1.0714(4) | ||
κ | 0.7647(3) | 0.6606(1) | 0.7589(2) | 0.8454(6) | 0.8340(5) | 0.7822(4) | ||
AVAB | α | 0.0655(3) | 0.1144(5) | 0.0368(1) | 0.1254(6) | 0.0922(4) | 0.0566(2) | |
φ | 0.0615(3) | 0.0516(1) | 0.0528(2) | 0.1499(6) | 0.1246(5) | 0.0714(4) | ||
κ | 0.0647(3) | 0.0394(1) | 0.0589(2) | 0.1454(6) | 0.1340(5) | 0.0822(4) | ||
MSE | α | 0.1055(1) | 0.1232(2) | 0.1489(3) | 0.2735(6) | 0.2627(5) | 0.1648(4) | |
φ | 0.0582(2) | 0.0498(1) | 0.0816(3) | 0.2593(6) | 0.2387(5) | 0.1083(4) | ||
κ | 0.0765(2) | 0.0688(1) | 0.1080(3) | 0.2400(6) | 0.2333(5) | 0.1343(4) | ||
RMSE | α | 0.3248(1) | 0.3509(2) | 0.3858(3) | 0.5229(6) | 0.5126(5) | 0.4059(4) | |
φ | 0.2412(2) | 0.2231(1) | 0.2857(3) | 0.5092(6) | 0.4886(5) | 0.3290(4) | ||
κ | 0.2766(2) | 0.2622(1) | 0.3286(3) | 0.4899(6) | 0.4830(5) | 0.3665(4) | ||
∑ranks | 25(2) | 18(1) | 29(3) | 72(6) | 59(5) | 45(4) |
n | Measures | Pa. | ML | MPS | ANDA | CVM | OLS | WLS |
10 | AVE | α | 1.9113(4) | 1.8080(3) | 2.1893(6) | 2.0102(5) | 1.6790(1) | 1.7030(2) |
φ | 3.4196(3) | 6.6547(5) | 13.4896(6) | 4.6929(4) | 3.1980(1.5) | 3.1980(1.5) | ||
κ | 1.6351(1) | 2.9747(5) | 3.4465(6) | 2.4926(4) | 2.2030(3) | 2.1720(2) | ||
AVAB | α | 0.4113(4) | 0.3080(3) | 0.6893(6) | 0.5102(5) | 0.1787(1) | 0.2032(2) | |
φ | 1.4196(3) | 4.6547(5) | 11.4896(6) | 2.6929(4) | 1.1978(1) | 1.1980(2) | ||
κ | 0.6351(1) | 1.9747(5) | 2.4465(6) | 1.4926(4) | 1.2034(3) | 1.1716(2) | ||
MSE | α | 0.8424(1) | 2.6556(5) | 4.2739(6) | 1.6544(4) | 1.0310(2) | 1.0990(3) | |
φ | 6.2459(1) | 242.8573(5) | 1099.2808(6) | 63.1670(4) | 34.3340(2) | 36.4950(3) | ||
κ | 2.8238(1) | 37.3047(5) | 47.8131(6) | 17.0243(4) | 12.9340(3) | 10.7970(2) | ||
RMSE | α | 0.9178(1) | 1.6296(5) | 2.0673(6) | 1.2862(4) | 1.0150(3) | 1.0480(2) | |
φ | 2.4992(1) | 15.5839(5) | 33.1554(6) | 7.9478(4) | 5.8600(2) | 6.0410(3) | ||
κ | 1.6804(1) | 6.1078(5) | 6.9147(6) | 4.1261(4) | 3.5960(3) | 3.2860(2) | ||
∑ranks | 22(1) | 56(5) | 72(6) | 50(4) | 25.5(2) | 26.5(3) | ||
50 | AVE | α | 1.6952(2) | 1.4342(1) | 1.8042(5) | 1.8992(6) | 1.7690(3) | 1.8000(4) |
φ | 2.4984(2) | 2.1884(1) | 4.9115(6) | 3.7973(4) | 3.1380(3) | 3.3830(5) | ||
κ | 1.3226(2) | 1.1667(1) | 1.9301(6) | 1.8651(5) | 1.7060(3) | 1.7400(4) | ||
AVAB | α | 0.1952(2) | 0.0658(1) | 0.3042(5) | 0.3992(6) | 0.2692(3) | 0.3001(4) | |
φ | 0.4984(2) | 0.1884(1) | 2.9115(6) | 1.7973(5) | 1.1377(3) | 1.3826(4) | ||
κ | 0.3226(2) | 0.1667(1) | 0.9301(6) | 0.8651(5) | 0.7060(3) | 0.7402(4) | ||
MSE | α | 0.3337(1) | 0.5070(2) | 1.5355(6) | 1.0502(5) | 0.7593(3) | 0.8669(4) | |
φ | 1.5498(1) | 4.0449(2) | 155.5950(6) | 60.8746(5) | 17.0518(3) | 29.0772(4) | ||
κ | 0.9269(1) | 1.7944(2) | 11.4674(6) | 6.4988(5) | 4.3962(3) | 5.1792(4) | ||
RMSE | α | 0.5776(1) | 0.7120(2) | 1.2391(6) | 1.0248(5) | 0.8714(3) | 0.9311(4) | |
φ | 1.2449(1) | 2.0112(2) | 12.4738(6) | 7.8022(5) | 4.1294(4) | 5.3923(3) | ||
κ | 0.9627(1) | 1.3396(2) | 3.3864(6) | 2.5493(5) | 2.0967(3) | 2.2758(4) | ||
∑ranks | 18(1.5) | 18(1.5) | 70(6) | 61(5) | 37(3) | 48(4) | ||
100 | AVE | α | 1.6264(2) | 1.3950(1) | 1.6954(3) | 1.7879(6) | 1.7060(4) | 1.7390(5) |
φ | 2.3116(2) | 1.9354(1) | 3.4363(6) | 3.2511(5) | 2.8230(3) | 3.0510(4) | ||
κ | 1.2179(2) | 0.9918(1) | 1.5569(4) | 1.6311(6) | 1.5010(3) | 1.5570(5) | ||
AVAB | α | 0.1264(2) | 0.1050(1) | 0.1954(3) | 0.2879(6) | 0.2062(4) | 0.2393(5) | |
φ | 0.3116(2) | 0.0646(1) | 1.4363(6) | 1.2511(5) | 0.8234(3) | 1.0510(4) | ||
κ | 0.2179(2) | 0.0082(1) | 0.5569(5) | 0.6311(6) | 0.5009(3) | 0.5567(4) | ||
MSE | α | 0.2014(1) | 0.4738(2) | 0.8545(6) | 0.7787(5) | 0.5939(3) | 0.6521(4) | |
φ | 0.8984(1) | 0.9734(2) | 56.0050(6) | 25.6484(5) | 9.4794(3) | 21.8101(4) | ||
κ | 0.5434(1) | 0.6022(2) | 5.2431(6) | 3.9995(5) | 2.7885(3) | 3.5394(4) | ||
RMSE | α | 0.4488(1) | 0.4738(2) | 0.9244(6) | 0.8824(5) | 0.7706(3) | 0.8075(4) | |
φ | 0.9478(1) | 0.9734(2) | 7.4836(6) | 5.0644(5) | 3.0789(3) | 4.6701(4) | ||
κ | 0.7372(1) | 0.7760(2) | 2.2898(6) | 1.9999(5) | 1.6699(3) | 1.8813(4) | ||
∑ranks | 18(1.5) | 18(1.5) | 63(5) | 64(6) | 38(3) | 51(4) | ||
200 | AVE | α | 1.5676(2) | 1.4003(1) | 1.5936(3) | 1.6640(6) | 1.6350(5) | 1.6210(4) |
φ | 2.1555(2) | 1.8840(1) | 2.4471(3) | 2.5978(6) | 2.5470(5) | 2.4900(4) | ||
κ | 1.1155(2) | 0.9290(1) | 1.2360(3) | 1.3342(6) | 1.2990(5) | 1.2790(4) | ||
AVAB | α | 0.0676(1) | 0.0997(3) | 0.0936(2) | 0.1640(6) | 0.1254(5) | 0.1210(4) | |
φ | 0.1555(2) | 0.1160(1) | 0.4471(3) | 0.5978(6) | 0.5470(5) | 0.4895(4) | ||
κ | 0.1155(2) | 0.0710(1) | 0.2360(3) | 0.3342(6) | 0.2988(5) | 0.2790(4) | ||
MSE | α | 0.0927(1) | 0.0972(2) | 0.3192(3) | 0.4075(6) | 0.3785(5) | 0.3419(4) | |
φ | 0.3186(2) | 0.2844(1) | 8.2814(5) | 5.1207(3) | 10.0634(6) | 5.8513(4) | ||
κ | 0.2216(2) | 0.1781(1) | 1.5037(5) | 1.4824(4) | 1.5757(6) | 1.4326(3) | ||
RMSE | α | 0.3045(2) | 0.3117(1) | 0.5650(3) | 0.6384(6) | 0.6152(5) | 0.5847(4) | |
φ | 0.5645(2) | 0.5333(1) | 2.8777(5) | 2.2629(3) | 3.1723(6) | 2.4190(4) | ||
κ | 0.4708(2) | 0.4221(1) | 1.2263(5) | 1.2175(4) | 1.2553(6) | 1.1969(3) | ||
∑ranks | 22(2) | 15(1) | 43(3) | 62(5) | 64(6) | 46(4) |
The results in Tables 2–5 show that the mean square errors (MSEs) decrease for all parameter combinations as the sample size (n) increases, that is, all the estimators are quite consistent since their RMSE tend to zero for large sample size (n). Hence, the estimates of the EHLST parameters provide credible MSEs and low AVABs for all the parameter combinations using the six estimation methods for large sample size (n). Additionally, the average absolute biases (AVABs) approach zero as the sample size (n) increase, proving that all the estimators are quite asymptotically unbiased.
The model evaluation in relation to some existing distributions from the same family of distributions using the inflation rates (INF) monthly dataset is performed. The few existing distributions from the same family are the exponentiated half logistic Lomax (EHLL), exponentiated half logistic Fréchet (EHLF) and exponentiated half logistic log-logistic (EHLLL). The model parameters are estimated using the maximum likelihood and maximum product of spacing estimation procedures. Using the R-software package "AdequacyModel", the following performance measures: negative log-likelihood, Akaike Information Criterion (AIC), Kolmogorov-Smirnov (K-S) statistic and its p-value including the ML estimates (MLEs) and standard errors (SEs) of the parameters are provided in Table 6 while the MPS estimates (MPSEs), log-likelihood and other measures are provided in Table 7. The results in Tables 6 and 7 show that the new model is quite competitive with the existing four-parameter distributions in fitting the inflation rates dataset.
Model | Par | MLE (SE) | -LL | AIC | K-S | p-value |
EHLST | ˆα | 3.2407(0.5773) | 588.256 | 1182.513 | 0.070 | 0.263 |
ˆφ | 67.8208(27.2116) | |||||
ˆκ | 217.1097(81.7760) | |||||
EHLL | ˆα | 4.2120(2.2915) | 590.130 | 1188.260 | 0.077 | 0.165 |
ˆβ | 9.9874(1.6320) | |||||
ˆa | 4.2120(2.2951) | |||||
ˆb | 0.0186(0.0047) | |||||
EHLF | ˆα | 1.2744(0.4305) | 585.933 | 1179.867 | 0.069 | 0.267 |
ˆλ | 26.4325(15.3670) | |||||
ˆa | 0.7669(0.1661) | |||||
ˆb | 48.0005(23.9203) | |||||
EHLLL | ˆα | 0.5109(0.4213) | 583.900 | 1175.800 | 0.052 | 0.627 |
ˆλ | 0.7105(0.6145) | |||||
ˆa | 11.3008(0.9864) | |||||
ˆb | 7.5646(5.2527) |
Model | Par | MPS (SE) | LL | AIC | K-S | p-value |
EHLST | ˆα | 3.1763(0.0286) | −590.015 | 1186.000 | 0.069 | 0.269 |
ˆφ | 64.0223(1.7981) | |||||
ˆκ | 218.3550(4.2330) | |||||
EHLL | ˆα | 3.3253(0.0749) | −592.43 | 1192.900 | 0.089 | 0.072 |
ˆβ | 9.9379(0.1238) | |||||
ˆa | 3.3075(0.0745) | |||||
ˆb | 0.0318(0.0007) | |||||
EHLF | ˆα | 1.4956(0.3689) | −587.234 | 1182.500 | 0.068 | 0.300 |
ˆλ | 30.5011(13.4873) | |||||
ˆa | 0.7660(0.0970) | |||||
ˆb | 50.1275(26.6395) | |||||
EHLLL | ˆα | 0.5220(0.2593) | −585.169 | 1178.300 | 0.059 | 0.460 |
ˆλ | 0.8105(0.58140) | |||||
ˆa | 11.5673(0.7104) | |||||
ˆb | 6.9976 (7.3261) |
The standardized form of the EHLST density function is derived using the transformed random variable z=⟨y−μ⟩√h2, where E(z)=0 and var(z)=1. Thus, the standardized EHLST density function with z=ε√h2 and ∂z∂ε=1√h2 is given by
f(ε;α,φ,κ,h2t)=(1{h2t}12)2αφκ[1−(12(1+εt√h2t√κ+(εt√h2t)2))]α−1{1−[1−(12(1+εt√h2t√κ+(εt√h2t)2))]α}φ−12(κ+(εt√h2t)2)32{1+[1−(12(1+εt√h2t√κ+(εt√h2t)2))]α}φ+1 | (37) |
The log-likelihood function (LL) is given by
LL(ξ)=n log α+n log φ+n log κ−32n∑t=1 log (κ+(εt√h2t)2)+(α−1)n∑t=1 log {1−(12(1+εt√h2t√κ+(εt√h2t)2))} |
+(φ−1)∑nt=1 log {1−[1−(12(1+εt√h2t√κ+(εt√h2t)2))]ϑ} | (38) |
−(φ+1)∑nt=1 log {1+[1−(12(1+εt√h2t√κ+(εt√h2t)2))]ϑ}−12∑nt=1 log (h2t) |
where ξ=(α,φ,κ,h2t) and h2t denote the GARCH-type volatility models with unknown vector parameters.
The applicability of the standardized EHLST density function as the conditional innovation density in GARCH-type volatility models are demonstrated using the monthly all-items Nigeria inflation rates (year on change) dataset from January 2003 to August 2021. The dataset can be sourced from the Central Bank of Nigeria website at www.cbn.gov.ng.
In financial time series, volatility modeling is a dynamic direction for both advisors and financial expert. Engle (1982) and Bollerslev (1986) introduced the autoregressive conditional heteroscedasticity (ARCH) and the generalized ARCH (GARCH) models for volatility modeling. The log-return is denoted as rt, and the GARCH (1, 1) model is expressed as follows:
rt=μ+εt,εt=zt√h2t,zt∼i.i.d. |
h2t=ω+η1ε2t−1+η2h2t−1, | (39) |
where ω>0, η1>0, η2>0, zt is the conditional innovation distribution with E(zt)=0 and var(zt)=1. The conditional variance and mean are denoted as h2t and μ, respectively. In this research work, the GARCH model (Bollerslev, 1986) and GJRGARCH model (Glosten et al., 1993) are considered. The GARCH model is used as the benchmark in this research. The GJRGARCH (1, 1) model conditional variance functional form is expressed as:
h2t=ω+η1ε2t−1+η3It−1ε2t−1+η2h2t−1 | (40) |
where η3 is the leverage effect, and It−1 is an indicator function expressed as:
It−1={1,ifεt−1<0,0,ifεt−1≥0, |
The GJRGARCH model checks for leverage effects and when the parameter η3 is positive it implies that negative shocks have a larger effect on volatility than positive shocks. The common conditional innovations are provided in Appendix A.
The accuracy of both models is appraised using the modified information criteria of Brooks and Burke (2003). The modified Akaike Information Criterion (AIC) and Bayesian Information Criterion (BIC), criteria are given by
AIC=2cT−2LLTBIC=c log e(T)T−2LLT | (41) |
where T is the number of observations, c is number of estimated parameters, and LL denotes the log-likelihood value. The model with the least AIC and BIC values is regarded as the finest model in terms of the specified conditional innovation density.
The predictive ability of the GARCH-type models is evaluated using the mean square error (MSE), root mean square root (RMSE), and mean absolute error (MAE). The MSE, RMSE, and MAE for the volatility forecasts are given by
MSE=1TT∑t=1(ˆht−ht)2,RMSE=√1TT∑t=1(ˆht−ht)2,MAE=1TT∑t=1|ˆht−ht| |
where ht and ˆht represent the realized volatility and predicted volatility, and T is the sample size. The model with the least MSE, RMSE, and MAE values is regarded as the most suitable for predicting the volatility of the financial dataset.
To assess the ability of the GARCH-type volatility models using the EHLST density in relation to the commonly existing densities in modeling and predicting volatility, the all-items Nigeria inflation rates is utilized. The utilized data consist of 224 inflation rates from January 2003 to August 2021. Table 8 presents the summary statistics of 223 monthly Inflation rate (INF) log-returns. Negative skewness and excess kurtosis are quite evident, leading to large Jarque-Bera (JB) test statistic value (p < 0.001) indicating that the monthly log-returns are non-normally distributed. More so, the Lagrange-multiplier (LM) test specifies the prevalence of ARCH effects in the conditional variance. The graphical plots of the log-returns, squared log-returns and absolute log-returns including their autocorrelation functions are depicted in Figure 4.
INF | |
Number of observations | 223 |
Mean | 0.2121 |
Median | 0.4906 |
Minimum | −104.1454 |
Maximum | 53.2217 |
Standard Deviation | 14.3681 |
Skewness | −1.3377 |
Kurtosis | 13.1953 |
Jarque-Bera | 1721.3 (p < 0.001) |
ARCH (2) | 15.406 (p < 0.001) |
The plots in Figure 4(b, c) show evidence of volatility clustering; that is low volatility values followed by low volatility values and high volatility values followed by high volatility values. More so, the monthly log-returns show no clear evidence of serial correlation, but the squared and absolute log-returns are positively autocorrelated as depicted in Figures 4(e, f).
The GARCH-type volatility models are estimated under the normal (NORM), Student-t (STD), generalized error (GED) and their skewed versions, generalized hyperbolic (GHYP), Johnson (SU) reparametrized (JSU), generalized hyperbolic skew-Student (GHST), Normal inverse Gaussian (NIG) and EHLST innovation densities. To acquire the parameter estimates of both models under NORM, STD, GED, SNORM, SSTD, SGED, GHYP, JSU, GHST and NIG densities, the rugarch package in R-software is used. The Optim function in R-software is utilized for the estimation of the model's parameter with the EHLST innovation density. Table 9 shows the GARCH (1, 1) and GJRGARCH (1, 1) models parameter estimates under eleven innovation densities.
GARCH (1, 1) | |||||||||||
Pa. | NORM | STD | GED | SNORM | SSTD | SGED | GHYP | JSU | GHST | NIG | EHLST |
μ | 0.6403 | 0.5656 | 0.6504'***' | 0.5158 | 0.3304 | 0.3277'***' | 0.4667 | 0.3513 | 0.1605 | 0.3589 | 0.1824 |
ω | 0.0900 | 0.7026 | 1.0661 | 0.1468'*' | 0.9007 | 1.4755'***' | 0.8444 | 1.0241'.' | 0.9637'.' | 1.2821'.' | 0.1891 |
η1 | 0.0602'*' | 0.2777'*' | 0.2536'.' | 0.0586'***' | 0.3115'*' | 0.2957'***' | 0.2904'*' | 0.3100'*' | 0.2876'*' | 0.3088'*' | 0.1112 |
η2 | 0.9292'***' | 0.7213'***' | 0.7454'***' | 0.9289'***' | 0.6875'***' | 0.7033'***' | 0.7086'***' | 0.6889'***' | 0.7114'***' | 0.6902'***' | 0.6069'***' |
η3 | - | - | - | - | - | - | - | - | - | - | - |
α | - | - | - | 0.9423'***' | - | - | 0.2500 | - | 8.1967'***' | 0.6823'.' | 1.7778'***' |
ξ | - | - | - | - | 0.8956'***' | - | - | - | - | - | - |
ν | - | 4.1458'***' | 0.9782'***' | - | 4.1743'***' | 0.8941'***' | - | - | - | - | - |
η | - | - | - | - | - | 0.9696'***' | - | - | - | - | - |
β | - | - | - | - | - | - | -0.3260 | - | -1.1452'' | -0.1358 | - |
λ | - | - | - | - | - | - | -1.9370'*' | - | - | - | - |
υ | - | - | - | - | - | - | - | -0.1940 | - | - | - |
ϑ | - | - | - | - | - | - | - | 1.3328'***' | - | - | - |
φ | - | - | - | - | - | - | - | - | - | - | 1.2070'***' |
κ | - | - | - | - | - | - | - | - | - | - | 6.6593 |
GJRGARCH (1, 1) | |||||||||||
Pa. | NORM | STD | GED | SNORM | SSTD | SGED | GHYP | JSU | GHST | NIG | EHLST |
μ | 0.8364'.' | 0.5669'.' | 0.6504'***' | 0.7550'' | 0.3233 | 0.3215'***' | 0.4650 | 0.3452 | -0.0555 | 0.3574 | 0.2001 |
ω | 0.0000 | 0.6843 | 1.1503 | 0.0000 | 0.8795 | 1.4925'***' | 0.8253 | 1.0071 | 1.2293'.' | 1.2783'' | 0.2750 |
η1 | 0.0864'*' | 0.2833'*' | 0.2414'.' | 0.0804'*' | 0.3254'*' | 0.2893'***' | 0.2978'*' | 0.3194'*' | 0.2800'*' | 0.3105'.' | 0.1692 |
η2 | 0.9397'***' | 0.7255'***' | 0.7322'***' | 0.9402'***' | 0.6912'***' | 0.7004'***' | 0.7125'***' | 0.6918'***' | 0.7322'***' | 0.6908'***' | 0.6113'***' |
η3 | −0.0643 | −0.0195 | 0.0508 | −0.0562 | −0.0371 | 0.0200 | −0.0232 | −0.0258 | −0.0292 | −0.0049 | −0.0004 |
α | - | - | - | 0.9651'***' | - | - | 0.2500 | - | 8.1943'***' | 0.6824'*' | 1.7571'***' |
ξ | - | - | - | - | 0.8923'***' | - | - | - | - | - | - |
ν | - | 4.1432'***' | 0.9821'***' | - | 4.1776'***' | 0.8923'***' | - | - | - | - | - |
η | - | - | - | - | - | 0.9694'***' | - | - | - | - | - |
β | - | - | - | - | - | - | −0.3324 | - | -1.8837'.' | −0.1365 | - |
λ | - | - | - | - | - | - | −1.9378'**' | - | - | - | - |
υ | - | - | - | - | - | - | - | -0.1988 | - | - | - |
ϑ | - | - | - | - | - | - | - | 1.3332'***' | - | - | - |
φ | - | - | - | - | - | - | - | - | - | - | 1.1858'***' |
κ | - | - | - | - | - | - | - | - | - | - | 4.2176 |
Significance level 0'***', 0.01'*', 0.05'*', 0.1'', 1 |
The parameter estimates of the conditional variance for the GARCH-EHLST are statistically significant at various standard levels including the leverage effect parameter η3. Hence, the impact of the shocks is asymmetric in nature which implies that the impact of positive shocks is higher than negative shocks of the same magnitude on volatility. Tables 10 provides vital statistics for model selection, which shows that the GARCH-EHLST has the highest log-likelihood, and least AIC and BIC values relative to other models. Hence, the GARCH-EHLST model is selected as the best model for modeling the volatility of the financial data series.
Model | Log-Likelihood | AIC | BIC |
GARCH-NORM | −808.135 | 7.2837 | 7.3448 |
GARCH-STD | −772.170 | 6.9701 | 7.0665 |
GARCH-GED | −776.818 | 7.0118 | 7.0882 |
GARCH-SNORM | −807.730 | 7.2891 | 7.3655 |
GARCH-SSTD | −771.494 | 6.9730 | 7.0647 |
GARCH-SGED | −775.870 | 7.0123 | 7.1040 |
GARCH-GHYP | −772.006 | 6.9866 | 7.0936 |
GARCH-JSU | −772.083 | 6.9783 | 7.0700 |
GARCH-GHST | −778.603 | 7.0368 | 7.1285 |
GARCH- NIG | −773.341 | 6.9896 | 7.0813 |
GARCH- EHLST | −768.492 | 6.9551 | 7.0620 |
GJRGARCH-NORM | −807.280 | 7.2850 | 7.3614 |
GJRGARCH-STD | −772.159 | 6.9790 | 7.0900 |
GJRGARCH-GED | −776.764 | 7.0203 | 7.1120 |
GJRGARCH-SNORM | −807.129 | 7.2926 | 7.3843 |
GJRGARCH-SSTD | −771.455 | 6.9817 | 7.0923 |
GJRGARCH-SGED | −775.843 | 7.0210 | 7.1280 |
GJRGARCH-GHYP | −771.991 | 6.9954 | 7.1177 |
GJRGARCH-JSU | −772.066 | 6.9871 | 7.0941 |
GJRGARCH-GHST | −781.977 | 7.0760 | 7.1830 |
GJRGARCH- NIG | −773.340 | 6.9986 | 7.1055 |
GJRGARCH- EHLST | −768.846 | 6.9672 | 7.0895 |
Table 11 provides the diagnostic analysis tests for the GARCH-type volatility models which indicates that the GARCH-EHLST model did well in describing the conditional variance dynamics as well as other models. Basically, the Ljung-Box statistic (p-value) indicates no sign of serial-correlation in the squared standardized residuals, and the ARCH-LM statistic indicates no extra ARCH processes in the standardized residuals which implies that the conditional variance equations are well-defined.
Model | Ljung-Box Statistic |
p-value | ARCH-LM Statistic | p-value |
GARCH-NORM | 3.331 | 0.9725 | 2.932 | 0.9830 |
GARCH-STD | 1.542 | 0.9988 | 1.715 | 0.9981 |
GARCH-GED | 1.797 | 0.9977 | 1.991 | 0.9964 |
GARCH-SNORM | 3.226 | 0.9756 | 2.848 | 0.9848 |
GARCH-SSTD | 1.735 | 0.9980 | 1.982 | 0.9965 |
GARCH-SGED | 1.891 | 0.9971 | 2.141 | 0.9951 |
GARCH-GHYP | 1.679 | 0.9983 | 1.893 | 0.9971 |
GARCH-JSU | 1.810 | 0.9976 | 2.073 | 0.9958 |
GARCH-GHST | 1.800 | 0.9977 | 2.033 | 0.9961 |
GARCH- NIG | 1.898 | 0.9965 | 2.176 | 0.9948 |
GARCH- EHLST | 1.511 | 0.9989 | 1.774 | 0.9978 |
GJRGARCH-NORM | 4.328 | 0.9313 | 3.617 | 0.9630 |
GJRGARCH-STD | 1.545 | 0.9988 | 1.714 | 0.9981 |
GJRGARCH-GED | 1.832 | 0.9975 | 2.054 | 0.9959 |
GJRGARCH-SNORM | 4.292 | 0.9332 | 3.610 | 0.9632 |
GJRGARCH-SSTD | 3.429 | 0.9991 | 1.997 | 0.9964 |
GJRGARCH-SGED | 1.903 | 0.9970 | 2.165 | 0.9949 |
GJRGARCH-GHYP | 1.684 | 0.9982 | 1.895 | 0.9971 |
GJRGARCH-JSU | 1.815 | 0.9976 | 2.075 | 0.9957 |
GJRGARCH-GHST | 1.849 | 0.9974 | 2.038 | 0.9960 |
GJRGARCH- NIG | 1.897 | 0.9971 | 2.172 | 0.9948 |
GJRGARCH- EHLST | 1.472 | 0.9990 | 1.711 | 0.9981 |
The in-sample volatility predictive measures for the models are provided in Table 12, and Table 13 provides the partial and total ranking of the innovation densities based on the three predictive performance measures. The results show that the GARCH (1, 1) and GJRGARCH (1, 1) models has the least MSE, RMSE and MAE values under the EHLST innovation density. Overall, the results indicate that the GARCH-EHLST model is statistically effective in forecasting the volatility of the inflation log-returns relative to other models based on the MSE and RMSE results while the GJRGARCH-EHLST model is statistically effective based on the MAE result. Furthermore, the EHLST innovation density has the least total rank value based on the performance measures among the eleven innovation densities considered in this study.
Model | MSE | RMSE | MAE |
GARCH-NORM | 112.720 | 10.617 | 7.011 |
GARCH-STD | 122.686 | 11.076 | 6.671 |
GARCH-GED | 122.269 | 11.057 | 6.776 |
GARCH-SNORM | 111.427 | 10.556 | 6.952 |
GARCH-SSTD | 123.930 | 11.132 | 6.650 |
GARCH-SGED | 123.902 | 11.131 | 6.764 |
GARCH-GHYP | 123.204 | 11.100 | 6.673 |
GARCH-JSU | 123.939 | 11.133 | 6.670 |
GARCH-GHST | 123.355 | 11.106 | 6.693 |
GARCH- NIG | 124.100 | 11.140 | 6.712 |
GARCH- EHLST | 109.109 | 10.445 | 5.396 |
GJRGARCH-NORM | 156.588 | 12.513 | 9.119 |
GJRGARCH-STD | 124.678 | 11.166 | 6.757 |
GJRGARCH-GED | 117.606 | 10.845 | 6.555 |
GJRGARCH-SNORM | 149.356 | 12.221 | 8.828 |
GJRGARCH-SSTD | 127.418 | 11.288 | 6.791 |
GJRGARCH-SGED | 122.197 | 11.054 | 6.686 |
GJRGARCH-GHYP | 125.462 | 11.201 | 6.768 |
GJRGARCH-JSU | 126.321 | 11.239 | 6.768 |
GJRGARCH-GHST | 126.353 | 11.241 | 6.911 |
GJRGARCH- NIG | 124.527 | 11.159 | 6.730 |
GJRGARCH- EHLST | 104.874 | 10.241 | 5.446 |
GARCH (1, 1) | |||||||||||
NORM | STD | GED | SNORM | SSTD | SGED | GHYP | JSU | GHST | NIG | EHLST | |
MSE | 3 | 5 | 4 | 2 | 9 | 8 | 6 | 10 | 7 | 11 | 1 |
RMSE | 3 | 5 | 4 | 2 | 9 | 8 | 6 | 10 | 7 | 11 | 1 |
MAE | 11 | 4 | 9 | 10 | 2 | 8 | 5 | 3 | 6 | 7 | 1 |
TOTAL | 17 | 14 | 17 | 14 | 20 | 24 | 17 | 23 | 20 | 29 | 3 |
GJRGARCH (1, 1) | |||||||||||
NORM | STD | GED | SNORM | SSTD | SGED | GHYP | JSU | GHST | NIG | EHLST | |
MSE | 11 | 5 | 2 | 10 | 9 | 3 | 6 | 7 | 8 | 4 | 1 |
RMSE | 11 | 5 | 2 | 10 | 9 | 3 | 6 | 7 | 8 | 4 | 1 |
MAE | 11 | 5 | 2 | 10 | 8 | 3 | 6.5 | 6.5 | 9 | 4 | 1 |
TOTAL | 33 | 15 | 6 | 30 | 26 | 9 | 18.5 | 20.5 | 25 | 12 | 3 |
Overall, the comparison between the volatility models in this study basically supports the use of the symmetric GARCH-EHLST model for estimating and forecasting the volatility of the inflation rates. More so, the proposed EHLST density seems generally the best innovation density for both the GARCH (1, 1) and GJRGARCH (1, 1) models.
In this research, the estimation of the exponentiated half logistic skew-t (EHLST) distribution parameters using six classical estimation procedures, namely the maximum likelihood, maximum product of spacing, least squares, Anderson-Darling, weighted least squares and Cramer-von mises is considered. Given that the theoretical comparison between these classical estimation procedures is not quite practicable, an extensive Monte Carlo simulation study is performed to compare the estimators in terms of average absolute biases, mean square error of each estimate and average parameter estimates. The results show that the criterions performance ordering from finest to poorest, using the overall ranks is MLE, MPS, WLS, OLS, ANDA, and CVM for all the parameter combinations. The MLE outperform all the other criterions with an overall rank of 32. Consequently, the performance of the EHLST model in fitting the inflation rates, showed that the EHLST model is quite competitive against the existing four-parameter models from the same family of distributions. Furthermore, volatility modeling of the inflation log-returns using the GARCH (1, 1) and GJRGARCH (1, 1) models with EHLST innovation density relative to the normal, student-t, generalized error and their skewed versions, generalized hyperbolic, Johnson (SU) reparametrized, generalized hyperbolic skew-Student, Normal inverse Gaussian innovation densities in terms of predictive performance using three forecast performance measures was carried-out. The research findings confirm that GARCH (1, 1) and GJRGARCH (1, 1) models with EHLST innovation density is optimally the best model based on model selection criteria and forecasts performance measures among other models. Overall, the results validate the superiority of the GARCH (1, 1) and GJRGARCH (1, 1) models with EHLST innovation density in both in-and-out samples performance over other models for inflation rates volatility modeling.
This research did not receive any specific grant from funding agencies in the public, commercial, or not-for-profit sector.
All authors declare no conflicts of interest in this paper.
[1] |
H. Krut, X. Peng, Does corporate social performance lead to better financial performance? Evidence from Turkey, Green Finance, 3 (2021), 464–482. https://doi.org/10.3934/gf.2021021 doi: 10.3934/gf.2021021
![]() |
[2] | D. Marghescu, M. Kallio, B. Back, Using financial ratios to select companies for tax auditing: a preliminary study, in Communications in Computer and Information Science. Springer, Berlin, 2010. https://doi.org/10.1007/978-3-642-16324-1_45 |
[3] | A. Su, Z. He, J. Su, Y. Zhou, Y. Fan, Y. Kong, Detection of tax arrears based on ensemble learning model, in Proceedings of the 2018 International Conference on Wavelet Analysis and Pattern Recognition, Piscataway, NJ, (2018), 270–274. https://doi.org/10.1109/icwapr.2018.8521362 |
[4] | A. Ippolito, A. C. G. Lozano, Sammon mapping-based gradient boosted trees for tax crime prediction in the city of São Paulo, in Enterprise Information Systems, ICEIS 2020, (2020), 293–316. https://doi.org/10.1007/978-3-030-75418-1_14 |
[5] |
J. Vanhoeyveld, D. Martens, B. Peeters, Value-added tax fraud detection with scalable anomaly detection techniques, Appl. Soft. Comput., 86 (2020), 1–38. https://doi.org/10.1016/j.asoc.2019.105895 doi: 10.1016/j.asoc.2019.105895
![]() |
[6] |
M. Z. Abedin, G. Chi, M. M. Uddin, M. S. Satu, M. I. Khan, P. Hajek, Tax default prediction using feature transformation-based machine learning, IEEE Access, 9 (2021), 19864–19881. https://doi.org/10.1109/access.2020.3048018 doi: 10.1109/access.2020.3048018
![]() |
[7] |
E. I. Altman, M. Balzano, A. Giannozzi, S. Srhoj, Revisiting SME default predictors: The Omega Score, J. Small Bus. Manage., 2022 (2022), 1–35. https://doi.org/10.1080/00472778.2022.2135718 doi: 10.1080/00472778.2022.2135718
![]() |
[8] |
O. Lukason, A. Andresson, Tax arrears versus financial ratios in bankruptcy prediction, J. Risk Financ. Manag., 12 (2019), 187–200. https://doi.org/10.3390/jrfm12040187 doi: 10.3390/jrfm12040187
![]() |
[9] |
S. Chen, J. Zhong, P. Failler, Does China transmit financial cycle spillover effects to the G7 countries, Econ. Res. -Ekon. Istraz., 35 (2022), 5184-5201. https://doi.org/10.1080/1331677X.2021.2025123 doi: 10.1080/1331677X.2021.2025123
![]() |
[10] |
F. Misra, R. Kurniawan, The role of audit information dissemination in curbing the contagion of tax noncompliance, J. Innov. Bus. Econ., 4 (2020). 1–11. https://doi.org/10.22219/jibe.v4i01.10223 doi: 10.22219/jibe.v4i01.10223
![]() |
[11] |
Z. Li, J. Zhu, J. He, The effects of digital financial inclusion on innovation and entrepreneurship: A network perspective, Electron. Res. Arch., 30 (2022), 4697–4715. https://doi.org/10.3934/era.2022238 doi: 10.3934/era.2022238
![]() |
[12] |
G. Kou, Y. Xu, Y. Peng, F. Shen, Y. Chen, K. Chang, et al., Bankruptcy prediction for SMEs using transactional data and two-stage multiobjective feature selection, Decis. Support Syst., 140 (2021), 113429. https://doi.org/10.1016/j.dss.2020.113429 doi: 10.1016/j.dss.2020.113429
![]() |
[13] |
P. Giudici, B. H. Misheva, A. Spelta, Network based credit risk models, Qual. Eng., 32 (2020), 199–211. https://doi.org/10.1080/08982112.2019.1655159 doi: 10.1080/08982112.2019.1655159
![]() |
[14] |
K. Peng, G. Yan, A survey on deep learning for financial risk prediction, Quant. Finance. Econ., 5 (2021), 716–737. https://doi.org/10.3934/qfe.2021032 doi: 10.3934/qfe.2021032
![]() |
[15] |
Õ. R. Siimon, O. Lukason, A decision support system for corporate tax arrears prediction, Sustainability, 13 (2021), 8363. https://doi.org/10.3390/su13158363 doi: 10.3390/su13158363
![]() |
[16] |
V. Chaudhri, C. Baru, N. Chittar, X. Dong, M. Genesereth, J. Hendler, Knowledge graphs: introduction, history and, perspectives, AI Mag., 43 (2022), 17–29. https://doi.org/10.1609/aimag.v43i1.19119 doi: 10.1609/aimag.v43i1.19119
![]() |
[17] |
R. Angles, C. Gutierrez, Survey of graph database models, ACM Comput. Surv., 40 (2008), 1–39. https://doi.org/10.1145/1322432.1322433 doi: 10.1145/1322432.1322433
![]() |
[18] | N. Ahbali, X. Liu, A. Nanda, J. Stark, A. Talukder, R. P. Khandpur, Identifying corporate credit risk sentiments from financial news, in Proceedings of the 2022 Conference of the North American Chapter of the Association for Computational Linguistics: Human Language Technologies: Industry Track, (2022), 362–370. http://dx.doi.org/10.18653/v1/2022.naacl-industry.40 |
[19] |
Z. Li, L. Chen, H. Dong, What are bitcoin market reactions to its-related events, Int. Rev. Econ. Finance, 73 (2021), 1–10. https://doi.org/10.1016/j.iref.2020.12.020 doi: 10.1016/j.iref.2020.12.020
![]() |
[20] | T. Ruan, L. Xue, H. Wang, F. Hu, L. Zhao, J. Ding, Building and exploring an enterprise knowledge graph for investment analysis, in International Semantic Web Conference 2016, (2016), 418–436. https://doi.org/10.1007/978-3-319-46547-0_35 |
[21] |
X. Chang, The impact of corporate tax outcomes on forced CEO turnover, Natl. Account. Rev., 4 (2022), 218–236. https://doi.org/10.3934/nar.2022013 doi: 10.3934/nar.2022013
![]() |
[22] |
A. Sousa, A. Braga, J. Cunha, Impact of macroeconomic indicators on bankruptcy prediction models: Case of the Portuguese construction sector, Quant. Finance. Econ., 6 (2022), 405–432. https://doi.org/10.3934/qfe.2022018 doi: 10.3934/qfe.2022018
![]() |
[23] |
Z. Li, Z. Huang, Y. Su, New media environment, environmental regulation and corporate green technology innovation: Evidence from China, Energy Econ., 119 (2023), 106545. https://doi.org/10.1016/j.eneco.2023.106545 doi: 10.1016/j.eneco.2023.106545
![]() |
[24] |
Y. Liu, Z. Li, M. Xu, The influential factors of financial cycle spillover: evidence from China, Emerg. Mark. Finance Trade, 56 (2020), 1336–1350. https://doi.org/10.1080/1540496x.2019.1658076 doi: 10.1080/1540496x.2019.1658076
![]() |
[25] |
G. Aytkhozhina, A. Miller, State tax control strategies: Theoretical aspects, Contaduría y Administración, 63 (2018), 25. https://doi.org/10.22201/fca.24488410e.2018.1672 doi: 10.22201/fca.24488410e.2018.1672
![]() |
[26] |
Z. Li, B. Mo, H. Nie, Time and frequency dynamic connectedness between cryptocurrencies and financial assets in China, Int. Rev. Econ. Finance, 86 (2023), 46–57. https://doi.org/10.1016/j.iref.2023.01.015 doi: 10.1016/j.iref.2023.01.015
![]() |
[27] |
Z. Li, H. Dong, C. Floros, A. Charemis, P. Failler, Re-examining bitcoin volatility: a CAViaR-based approach, Emerg. Mark. Finance Trade, 58 (2022), 1320–1338. https://doi.org/10.1080/1540496x.2021.1873127 doi: 10.1080/1540496x.2021.1873127
![]() |
[28] |
A. Chang, L. Yang, R. Tsaih, S. Lin, Machine learning and artificial neural networks to construct P2P lending credit-scoring model: A case using Lending Club data, Quant. Finance Econ., 6 (2022), 303–325. https://doi.org/10.3934/qfe.2022013 doi: 10.3934/qfe.2022013
![]() |
[29] |
D. Wang, L. Li, D. Zhao, Corporate finance risk prediction based on LightGBM, Inf. Sci., 602 (2022), 259–268. https://doi.org/10.1016/j.ins.2022.04.058 doi: 10.1016/j.ins.2022.04.058
![]() |
[30] |
B. Gao, V. Balyan, Construction of a financial default risk prediction model based on the LightGBM algorithm, J. Intell. Syst., 31 (2022), 767–779. https://doi.org/10.1515/jisys-2022-0036 doi: 10.1515/jisys-2022-0036
![]() |
[31] |
L. Zhang, Q. Song, Multimodel integrated enterprise credit evaluation method based on attention mechanism, Comput. Intell. Neurosci., 2022 (2022), 1–12. https://doi.org/10.1155/2022/8612759 doi: 10.1155/2022/8612759
![]() |
[32] | J. G. Ponsam, S.V. J. B. Gracia, G. Geetha, S. Karpaselvi, K. Nimala, Credit risk analysis using LightGBM and a comparative study of popular algorithms, in International Conference on Computing and Communications Technologies (ICCCT), 2021. https://doi.org/10.1109/iccct53315.2021.9711896 |
[33] |
D. G. Kirikos, An evaluation of quantitative easing effectiveness based on out-of-sample forecasts, Natl. Account. Rev., 4 (2022), 378–389. https://doi.org/10.3934/nar.2022021 doi: 10.3934/nar.2022021
![]() |
[34] |
F. Corradin, M. Billio, R. Casarin, Forecasting economic indicators with robust factor models, Natl. Account. Rev., 4 (2022), 167–190. https://doi.org/10.3934/nar.2022010 doi: 10.3934/nar.2022010
![]() |
[35] | P. Harrington, Machine Learning in Action, Manning Publications, (2012), 143–149. |
[36] | J. Davis, M. Goadrich, The relationship between Precision-Recall and ROC curves, in William C. ICML '06: Proceedings of the 23rd international conference on Machine learning, (2006), 233–240. https://doi.org/10.1145/1143844.1143874 |
[37] |
T. Fawcett, An introduction to ROC analysis, Pattern Recognit. Lett., 27 (2006), 861–874. https://doi.org/10.1016/j.patrec.2005.10.010 doi: 10.1016/j.patrec.2005.10.010
![]() |
[38] | W. H. J. David, S. Lemeshow, R. X. Sturdivant, Applied Logistic Regression, 3 edition, John Wiley & Sons, (2013), 177–178. https://doi.org/10.1002/9781118548387 |
[39] |
Z. Li, C. Yang, Z. Huang, How does the fintech sector react to signals from central bank digital currencies, Finance Res. Lett., 50 (2022), 103308. https://doi.org/10.1016/j.frl.2022.103308 doi: 10.1016/j.frl.2022.103308
![]() |
[40] |
D. L. Wilsin, Asymptotic properties of nearest neighbor rules using edited data, IEEE Trans. Syst. Man Cybern., 3 (1972), 408–421. https://doi.org/10.1109/tsmc.1972.4309137 doi: 10.1109/tsmc.1972.4309137
![]() |
[41] | I. Tomek, Two modifications of CNN, IEEE Trans. Syst. Man Cybern., 6 (1976), 769–772. https://doi.org/10.1109/tsmc.1976.4309452 |
[42] |
N. V. Chawla, K. W. Bowyer, L. O. Hall, W. P. Kegelmeyer, Smote: synthetic minority over-sampling technique, J. Artif. Intell. Res., 16 (2002), 321–357. https://doi.org/10.1613/jair.953 doi: 10.1613/jair.953
![]() |
[43] | H. Han, W. Y. Wang, B. H. Mao, Borderline-smote: a new over-sampling method in imbalanced data sets learning, in International Conference on Intelligent Computing, (2005), 878–887. https://doi.org/10.1007/11538059_91 |
[44] | B. Y. Li, Y. Liu, X. G. Wang, Gradient harmonized single-stage detector, in The 33rd AAAI Conference on Artificial Intelligence, (2019), 8577–8584. https://doi.org/10.1609/aaai.v33i01.33018577 |
[45] | T. Lin, P. Goyal, R. Girshick, K. He, P. Dollar, Focal loss for dense object detection, in 2017 IEEE International Conference on Computer Vision (ICCV), 2017. https://doi.org/10.1109/iccv.2017.324 |
[46] |
T. Li, J. Wen, D. Zeng, K. Liu, Has enterprise digital transformation improved the efficiency of enterprise technological innovation? A case study on Chinese listed companies, Math. Biosci. Eng., 19 (2022), 12632–12654. https://doi.org/10.3934/mbe.2022590 doi: 10.3934/mbe.2022590
![]() |
1. | O.D. Adubisi, A. Abdulkadir, D.J. Adashu, Improved parameter estimators for the flexible extended skew-t model with extensive simulations, applications and volatility modeling, 2023, 19, 24682276, e01443, 10.1016/j.sciaf.2022.e01443 |
Parameter (pa) | n | ML | MPS | ANDA | CVM | OLS | WLS |
α=1.0,φ=1.3,κ=0.5 | 10 | 2 | 5 | 6 | 4 | 1 | 3 |
50 | 2 | 1 | 6 | 5 | 4 | 3 | |
100 | 2 | 1 | 4 | 6 | 5 | 3 | |
200 | 3 | 1 | 2 | 6 | 5 | 4 | |
α=1.3,φ=1.7,κ=1.0 | 10 | 2 | 5 | 6 | 4 | 3 | 1 |
50 | 2 | 1 | 4.5 | 6 | 4.5 | 3 | |
100 | 3 | 1 | 2 | 6 | 5 | 4 | |
200 | 3 | 1 | 2 | 6 | 5 | 4 | |
α=2.0,φ=2.5,κ=1.5 | 10 | 1 | 5 | 6 | 4 | 2 | 3 |
50 | 2 | 1 | 3 | 6 | 5 | 4 | |
100 | 2 | 1 | 3 | 6 | 4 | 5 | |
200 | 2 | 1 | 3 | 6 | 5 | 4 | |
α=2.5,φ=3.0,κ=2.0 | 10 | 1 | 5 | 6 | 4 | 2 | 3 |
50 | 1.5 | 1.5 | 6 | 6 | 3 | 4 | |
100 | 1.5 | 1.5 | 5 | 6 | 3 | 4 | |
200 | 2 | 1 | 3 | 6 | 6 | 4 | |
∑ranks | 32 | 33 | 67.5 | 87 | 62.5 | 56 | |
Overall rank | 1 | 2 | 5 | 6 | 4 | 3 |
n | Measures | Pa. | ML | MPS | ANDA | CVM | OLS | WLS |
10 | AVE | α | 1.4661(5) | 1.4066(4) | 1.6544(6) | 1.3840(3) | 1.1710(2) | 1.1590(1) |
φ | 2.4233(3) | 4.8935(5) | 8.1802(6) | 2.6132(4) | 1.9540(1) | 2.0060(2) | ||
κ | 1.1683(1) | 2.3008(5) | 2.4332(6) | 1.3781(4) | 1.2180(2) | 1.2700(3) | ||
AVAB | α | 0.4661(5) | 0.4066(4) | 0.6544(6) | 0.3840(3) | 0.1713(2) | 0.1594(1) | |
φ | 1.1233(3) | 3.5935(5) | 6.8802(6) | 1.3132(4) | 0.6536(1) | 0.7063(2) | ||
κ | 0.6683(1) | 1.8008(5) | 1.9332(6) | 0.8781(4) | 0.7178(2) | 0.7701(3) | ||
MSE | α | 0.8648(3) | 2.3453(5) | 3.3241(6) | 1.0758(4) | 0.6714(1) | 0.7021(2) | |
φ | 4.1873(1) | 172.4338(5) | 472.9212(6) | 19.7630(4) | 15.0648(2) | 15.7997(3) | ||
κ | 2.4706(1) | 35.4217(5) | 36.2999(6) | 6.9764(3) | 5.3805(2) | 7.9437(4) | ||
RMSE | α | 0.9300(3) | 1.5314(5) | 1.8232(6) | 1.0372(4) | 0.8194(1) | 0.8379(2) | |
φ | 2.0463(1) | 13.1314(5) | 21.7468(6) | 4.4456(4) | 3.8813(2) | 3.9749(3) | ||
κ | 1.5718(1) | 5.9516(6) | 6.0249(7) | 2.6413(3) | 2.3196(2) | 2.8185(4) | ||
∑ranks | 28(2) | 49(5) | 73(6) | 44(4) | 20(1) | 29(3) | ||
50 | AVE | α | 1.1687(5) | 0.9853(1) | 1.1595(3) | 1.2278(6) | 1.1672(4) | 1.1501(2) |
φ | 1.5906(2) | 1.3920(1) | 2.1469(6) | 1.8565(5) | 1.7203(4) | 1.6610(3) | ||
κ | 0.7185(2) | 0.6255(1) | 0.8535(5) | 0.8632(6) | 0.8289(4) | 0.7911(3) | ||
AVAB | α | 0.1687(5) | 0.0147(1) | 0.1595(3) | 0.2278(6) | 0.1672(4) | 0.1501(2) | |
φ | 0.2906(2) | 0.0920(1) | 0.8469(6) | 0.5565(5) | 0.4203(4) | 0.3610(3) | ||
κ | 0.2185(2) | 0.1255(1) | 0.3535(4) | 0.3632(5) | 1.3812(6) | 0.2911(3) | ||
MSE | α | 0.2171(1) | 0.2513(2) | 0.5655(6) | 0.4314(5) | 0.3657(4) | 0.3091(3) | |
φ | 0.5725(1) | 2.5172(2) | 38.3736(6) | 3.8641(3) | 3.9756(4) | 4.4224(5) | ||
κ | 0.3991(1) | 0.9812(2) | 3.3622(6) | 1.5146(4) | 1.3812(3) | 1.8538(5) | ||
RMSE | α | 0.4659(1) | 0.5013(2) | 0.7520(6) | 0.6568(5) | 0.6047(4) | 0.5559(3) | |
φ | 0.7567(1) | 1.5866(2) | 6.1946(6) | 1.9657(3) | 1.9939(4) | 2.1029(5) | ||
κ | 0.6317(1) | 0.9905(2) | 1.8336(6) | 1.2307(4) | 1.1752(3) | 1.3616(5) | ||
∑ranks | 24(2) | 18(1) | 63(6) | 57(5) | 48(4) | 42(3) | ||
100 | AVE | α | 1.0768(4) | 0.9461(1) | 1.0561(2) | 1.1320(6) | 1.0928(5) | 1.0652(3) |
φ | 1.4224(2) | 1.2463(1) | 1.5157(4) | 1.6517(6) | 1.5443(5) | 1.4523(3) | ||
κ | 0.6022(2) | 0.4996(1) | 0.6226(4) | 0.7279(6) | 0.6878(5) | 0.6203(3) | ||
AVAB | α | 0.0768(4) | 0.0539(1) | 0.0561(2) | 0.1320(6) | 0.0928(5) | 0.0652(3) | |
φ | 0.1224(2) | 0.0537(1) | 0.2157(4) | 0.3517(6) | 0.2443(5) | 0.1523(3) | ||
κ | 0.1022(2) | 0.0004(1) | 0.1226(5) | 0.2279(6) | 0.1878(3) | 0.1203(4) | ||
MSE | α | 0.0831(2) | 0.0682(1) | 0.1692(4) | 0.2889(6) | 0.2350(5) | 0.1393(3) | |
φ | 0.1652(2) | 0.1829(3) | 6.2211(6) | 3.6319(5) | 2.1739(4) | 1.4473(1) | ||
κ | 0.1402(1) | 0.1508(2) | 1.0192(5) | 1.1513(6) | 0.8198(4) | 0.3606(3) | ||
RMSE | α | 0.2882(2) | 0.2612(1) | 0.4113(4) | 0.5375(6) | 0.4848(5) | 0.3732(3) | |
φ | 0.4065(1) | 0.4277(2) | 2.4942(6) | 1.9058(5) | 1.4744(4) | 1.2030(3) | ||
κ | 0.3744(1) | 0.3883(2) | 1.0096(5) | 1.0730(6) | 0.9054(4) | 0.6005(3) | ||
∑ranks | 25(2) | 17(1) | 51(4) | 70(6) | 54(5) | 35(3) | ||
200 | AVE | α | 1.0360(4) | 0.9541(1) | 1.0240(2) | 1.0588(6) | 1.0415(5) | 1.0285(3) |
φ | 1.3576(3) | 1.2478(1) | 1.3482(2) | 1.4147(6) | 1.3846(5) | 1.3593(4) | ||
κ | 0.5436(3) | 0.4775(1) | 0.5384(2) | 0.5821(6) | 0.5722(5) | 0.5493(4) | ||
AVAB | α | 0.0360(3) | 0.0459(5) | 0.0240(1) | 0.0588(6) | 0.0415(4) | 0.0285(2) | |
φ | 0.0576(3) | 0.0522(2) | 0.0482(1) | 0.1147(6) | 0.0846(5) | 0.0593(4) | ||
κ | 0.0436(3) | 0.0225(1) | 0.0384(2) | 0.0821(6) | 0.0722(5) | 0.0493(4) | ||
MSE | α | 0.0329(2) | 0.0270(1) | 0.0441(3) | 0.0917(6) | 0.0824(5) | 0.0567(4) | |
φ | 0.0624(2) | 0.0455(1) | 0.0849(3) | 0.2907(6) | 0.2382(5) | 0.1839(4) | ||
κ | 0.0417(2) | 0.0291(1) | 0.0552(3) | 0.1570(6) | 0.1399(5) | 0.0947(4) | ||
RMSE | α | 0.1813(2) | 0.1645(1) | 0.2101(3) | 0.3028(6) | 0.2871(5) | 0.2380(4) | |
φ | 0.2498(2) | 0.2133(1) | 0.2914(3) | 0.5392(6) | 0.4881(5) | 0.4289(4) | ||
κ | 0.2041(2) | 0.1706(1) | 0.2350(3) | 0.3963(6) | 0.3741(5) | 0.3077(4) | ||
∑ranks | 31(3) | 17(1) | 28(2) | 82(6) | 59(5) | 45(4) |
n | Measures | Pa. | ML | MPS | ANDA | CVM | OLS | WLS |
10 | AVE | α | 1.1024(3) | 1.1175(5) | 1.4136(6) | 1.0918(4) | 0.9769(2) | 0.9432(1) |
φ | 2.7852(4) | 5.2337(5) | 9.8213(6) | 2.7427(3) | 2.0152(2) | 1.8648(1) | ||
κ | 1.9428(1) | 4.5719(5) | 6.0877(6) | 2.7247(3) | 2.9305(4) | 2.4634(2) | ||
AVAB | α | 0.3024(4) | 0.3175(5) | 0.6136(6) | 0.2918(3) | 0.1769(2) | 0.1432(1) | |
φ | 1.2852(4) | 3.7337(5) | 8.3213(6) | 1.2427(3) | 0.5152(2) | 0.3648(1) | ||
κ | 0.9428(1) | 3.5719(5) | 5.0877(6) | 1.7247(3) | 1.9305(4) | 1.4634(2) | ||
MSE | α | 0.5045(3) | 1.4348(5) | 2.5202(6) | 0.5695(4) | 0.4175(2) | 0.3182(1) | |
φ | 7.3621(3) | 151.9961(5) | 634.1503(6) | 16.9030(4) | 6.2433(2) | 3.1990(1) | ||
κ | 7.4544(1) | 153.2414(5) | 298.9136(6) | 33.2122(3) | 40.8208(4) | 13.8077(2) | ||
RMSE | α | 0.7103(3) | 1.1978(5) | 1.5875(6) | 0.7546(4) | 0.6461(2) | 0.5641(1) | |
φ | 2.7133(3) | 12.3287(5) | 25.1823(6) | 4.1113(4) | 2.4987(2) | 1.7886(1) | ||
κ | 2.7303(1) | 12.3791(5) | 17.2891(6) | 5.7630(3) | 6.3891(4) | 3.7159(2) | ||
∑ranks | 31(2) | 60(5) | 72(6) | 41(4) | 32(3) | 16(1) | ||
50 | AVE | α | 0.8833(4) | 0.7896(1) | 0.8815(3) | 0.9182(6) | 0.8904(5) | 0.8811(2) |
φ | 1.6996(3) | 1.4800(1) | 1.8893(6) | 1.7904(5) | 1.7388(4) | 1.6990(2) | ||
κ | 1.2704(2) | 1.1696(1) | 1.4091(3) | 1.4440(5) | 1.4889(6) | 1.4167(4) | ||
AVAB | α | 0.0833(4) | 0.0104(1) | 0.0815(3) | 0.1182(6) | 0.0904(5) | 0.0811(2) | |
φ | 0.1996(3) | 0.0200(1) | 0.3893(6) | 0.2904(5) | 0.2388(4) | 0.1990(2) | ||
κ | 0.2704(2) | 0.1696(1) | 0.4091(3) | 0.4440(5) | 0.4889(6) | 0.4167(4) | ||
MSE | α | 0.0794(2) | 0.0681(1) | 0.1771(6) | 0.1309(4) | 0.1464(5) | 0.1199(3) | |
φ | 0.3262(2) | 0.3085(1) | 20.5402(6) | 0.7886(3) | 1.7903(5) | 1.0740(4) | ||
κ | 0.9907(1) | 1.1406(2) | 7.5480(6) | 1.9706(3) | 4.2142(5) | 3.7125(4) | ||
RMSE | α | 0.2819(2) | 0.2610(1) | 0.4209(6) | 0.3618(4) | 0.3826(5) | 0.3462(3) | |
φ | 0.5711(2) | 0.5554(1) | 4.5321(6) | 0.8880(3) | 1.3380(5) | 1.0363(4) | ||
κ | 0.9907(1) | 1.0680(2) | 2.7474(6) | 1.4038(3) | 2.0528(5) | 1.9268(4) | ||
∑ranks | 28(2) | 14(1) | 60(4.5) | 62(6) | 60(4.5) | 38(3) | ||
100 | AVE | α | 0.8324(5) | 0.7737(1) | 0.8214(2) | 0.8476(6) | 0.8292(4) | 0.8239(3) |
φ | 1.5765(5) | 1.4469(1) | 1.5593(3) | 1.6181(6) | 1.5676(4) | 1.5566(2) | ||
κ | 1.1040(3) | 1.0233(1) | 1.0901(2) | 1.1638(6) | 1.1483(5) | 1.1139(4) | ||
AVAB | α | 0.0324(5) | 0.0263(3) | 0.0214(1) | 0.0476(6) | 0.0292(4) | 0.0239(2) | |
φ | 0.0765(5) | 0.0531(1) | 0.0593(3) | 0.1181(6) | 0.0676(4) | 0.0566(2) | ||
κ | 0.1040(3) | 0.0233(1) | 0.0901(2) | 0.1638(6) | 0.1483(5) | 0.1139(4) | ||
MSE | α | 0.0258(2) | 0.0222(1) | 0.0298(3) | 0.0507(6) | 0.0445(5) | 0.0323(4) | |
φ | 0.0948(2) | 0.0745(1) | 0.1090(3) | 0.2038(6) | 0.1641(5) | 0.1151(4) | ||
κ | 0.2745(2) | 0.0233(1) | 0.2965(3) | 0.5554(6) | 0.4886(5) | 0.3328(4) | ||
RMSE | α | 0.1607(2) | 0.1491(1) | 0.1727(3) | 0.2251(6) | 0.2109(5) | 0.1797(4) | |
φ | 0.3079(2) | 0.2729(1) | 0.3301(3) | 0.4514(6) | 0.4051(5) | 0.3392(4) | ||
κ | 0.5240(2) | 0.4679(1) | 0.5445(3) | 0.7453(6) | 0.6990(5) | 0.5769(4) | ||
∑ranks | 38(3) | 14(1) | 31(2) | 72(6) | 56(5) | 41(4) | ||
200 | AVE | α | 0.8155(5) | 0.7798(1) | 0.8117(3) | 0.8206(6) | 0.8122(4) | 0.8102(2) |
φ | 1.5382(5) | 1.4621(1) | 1.5325(3) | 1.5549(6) | 1.5328(4) | 1.5277(2) | ||
κ | 1.0486(3) | 0.9959(1) | 1.0469(2) | 1.0692(6) | 1.0643(5) | 1.0491(4) | ||
AVAB | α | 0.0155(4) | 0.0202(5) | 0.0117(2) | 0.0206(6) | 0.0122(3) | 0.0102(1) | |
φ | 0.0382(5) | 0.0359(4) | 0.0325(2) | 0.0549(6) | 0.0328(3) | 0.0277(1) | ||
κ | 0.0486(3) | 0.0041(1) | 0.0469(2) | 0.0692(6) | 0.0643(5) | 0.0491(4) | ||
MSE | α | 0.0110(2) | 0.0109(1) | 0.0141(3) | 0.0204(6) | 0.0193(5) | 0.0144(4) | |
φ | 0.0380(2) | 0.0359(1) | 0.0460(3) | 0.0648(6) | 0.0597(5) | 0.0466(4) | ||
κ | 0.1041(2) | 0.0949(1) | 0.1258(3) | 0.1858(6) | 0.1794(5) | 0.1280(4) | ||
RMSE | α | 0.1049(1.5) | 0.1049(1.5) | 0.1186(3) | 0.1427(6) | 0.1390(5) | 0.1198(4) | |
φ | 0.1950(2) | 0.1894(1) | 0.2144(3) | 0.2546(6) | 0.2443(5) | 0.2158(4) | ||
κ | 0.3227(2) | 0.3080(1) | 0.3547(3) | 0.4310(6) | 0.4236(5) | 0.3578(4) | ||
∑ranks | 36.5(3) | 19.5(1) | 32(2) | 72(6) | 64(5) | 38(4) |
n | Measures | Pa. | ML | MPS | ANDA | CVM | OLS | WLS |
10 | AVE | α | 2.8336(3) | 2.5944(1) | 3.1558(5) | 3.3131(6) | 2.8120(2) | 2.9230(4) |
φ | 2.0036(1) | 8.0269(5) | 19.3064(6) | 5.4068(4) | 4.1680(2) | 4.4550(3) | ||
κ | 1.4579(1) | 3.2311(5) | 3.6263(6) | 3.0043(4) | 2.8950(2) | 2.9920(3) | ||
AVAB | α | 0.8336(3) | 0.5944(1) | 1.1558(5) | 1.3131(6) | 0.8124(2) | 0.9231(4) | |
φ | 1.0036(1) | 7.0269(5) | 18.3064(6) | 4.4068(4) | 3.1675(2) | 3.4554(3) | ||
κ | 0.7579(1) | 2.5311(5) | 2.9263(6) | 2.3043(4) | 2.1952(2) | 2.2918(3) | ||
MSE | α | 2.1274(1) | 6.7714(5) | 9.2849(6) | 5.0302(4) | 3.5630(2) | 3.9500(3) | |
φ | 3.3320(1) | 648.1073(5) | 3383.9749(6) | 204.2319(4) | 177.4580(3) | 152.0100(2) | ||
κ | 2.8070(1) | 49.8495(5) | 60.4505(6) | 28.6379(3) | 27.8840(2) | 33.0500(4) | ||
RMSE | α | 1.4586(1) | 2.6022(5) | 3.0471(6) | 2.2428(4) | 1.8870(2) | 1.9870(3) | |
φ | 1.8254(1) | 25.4580(5) | 58.1719(6) | 14.2910(4) | 13.3210(3) | 12.3290(2) | ||
κ | 1.6754(1) | 7.0404(5) | 7.7750(6) | 5.3514(3) | 5.2800(2) | 5.7490(4) | ||
∑ranks | 16(1) | 52(5) | 70(6) | 50(4) | 26(2) | 38(3) | ||
50 | AVE | α | 2.2547(3) | 1.8578(1) | 2.1777(2) | 2.5788(6) | 2.4490(5) | 2.3520(4) |
φ | 1.2645(2) | 1.0723(1) | 1.5965(3) | 2.3717(6) | 2.1870(5) | 2.0330(4) | ||
κ | 0.9587(2) | 0.7912(1) | 1.0157(3) | 1.4816(6) | 1.4490(5) | 1.2750(4) | ||
AVAB | α | 0.2547(3) | 0.1422(1) | 0.1777(2) | 0.5788(6) | 0.4486(5) | 0.3518(4) | |
φ | 0.2645(2) | 0.0723(1) | 0.5965(3) | 1.3717(6) | 1.1873(5) | 1.0332(4) | ||
κ | 0.2587(2) | 0.0912(1) | 0.3157(3) | 0.7816(6) | 0.7489(5) | 0.5751(4) | ||
MSE | α | 0.4982(1) | 0.6380(2) | 0.8915(3) | 1.6694(6) | 1.5060(5) | 1.1900(4) | |
φ | 0.4962(1) | 1.4375(2) | 32.6387(5) | 30.5760(4) | 28.1190(3) | 49.6000(6) | ||
κ | 0.5600(1) | 0.7722(2) | 1.7465(3) | 3.9829(5) | 4.1170(6) | 3.2910(4) | ||
RMSE | α | 0.7058(1) | 0.7988(2) | 0.9442(3) | 1.2921(6) | 1.2270(5) | 1.0910(4) | |
φ | 0.7044(1) | 1.1990(2) | 5.7130(5) | 5.5296(4) | 5.3030(3) | 7.0430(6) | ||
κ | 0.7483(1) | 0.8788(2) | 1.3215(3) | 1.9957(5) | 2.0290(6) | 1.8140(4) | ||
∑ranks | 20(2) | 18(1) | 28(3) | 64(6) | 58(5) | 52(4) | ||
100 | AVE | α | 2.1444(3) | 1.8589(1) | 2.0854(2) | 2.3221(6) | 2.2590(5) | 2.1566(4) |
φ | 1.1384(3) | 0.9579(1) | 1.1305(2) | 1.6180(5) | 1.5510(4) | 1.6937(6) | ||
κ | 0.1510(1) | 0.6947(2) | 0.8403(3) | 1.1163(6) | 1.0970(5) | 0.9608(4) | ||
AVAB | α | 0.1444(3) | 0.1411(2) | 0.0854(1) | 0.3221(6) | 0.2591(5) | 0.1566(4) | |
φ | 0.1384(3) | 0.0421(1) | 0.1305(2) | 0.6180(5) | 0.5507(4) | 0.6937(6) | ||
κ | 0.1510(3) | 0.0053(1) | 0.1403(2) | 0.4163(6) | 0.3975(5) | 0.2608(4) | ||
MSE | α | 0.2375(1) | 0.2656(2) | 0.3276(3) | 0.8324(6) | 0.7795(5) | 0.5226(4) | |
φ | 0.1731(2) | 0.1389(1) | 0.3441(3) | 9.3730(5) | 9.3187(4) | 168.1577(6) | ||
κ | 0.2335(2) | 0.2183(1) | 0.3689(3) | 1.5424(5) | 1.5302(4) | 1.6901(6) | ||
RMSE | α | 0.4873(1) | 0.5154(2) | 0.5724(3) | 0.9123(6) | 0.8829(5) | 0.7229(4) | |
φ | 0.4161(2) | 0.3728(1) | 0.5866(3) | 3.0615(5) | 3.0526(4) | 12.9676(6) | ||
κ | 0.4832(2) | 0.4672(1) | 0.6074(3) | 1.2420(5) | 1.2370(4) | 1.3001(6) | ||
∑ranks | 26(2) | 16(1) | 30(3) | 66(6) | 54(4) | 60(5) | ||
200 | AVE | α | 2.0655(4) | 1.8856(1) | 2.0368(2) | 2.1254(6) | 2.0920(5) | 2.0566(3) |
φ | 1.0615(3) | 0.9484(1) | 1.0528(2) | 1.1499(6) | 1.1250(5) | 1.0714(4) | ||
κ | 0.7647(3) | 0.6606(1) | 0.7589(2) | 0.8454(6) | 0.8340(5) | 0.7822(4) | ||
AVAB | α | 0.0655(3) | 0.1144(5) | 0.0368(1) | 0.1254(6) | 0.0922(4) | 0.0566(2) | |
φ | 0.0615(3) | 0.0516(1) | 0.0528(2) | 0.1499(6) | 0.1246(5) | 0.0714(4) | ||
κ | 0.0647(3) | 0.0394(1) | 0.0589(2) | 0.1454(6) | 0.1340(5) | 0.0822(4) | ||
MSE | α | 0.1055(1) | 0.1232(2) | 0.1489(3) | 0.2735(6) | 0.2627(5) | 0.1648(4) | |
φ | 0.0582(2) | 0.0498(1) | 0.0816(3) | 0.2593(6) | 0.2387(5) | 0.1083(4) | ||
κ | 0.0765(2) | 0.0688(1) | 0.1080(3) | 0.2400(6) | 0.2333(5) | 0.1343(4) | ||
RMSE | α | 0.3248(1) | 0.3509(2) | 0.3858(3) | 0.5229(6) | 0.5126(5) | 0.4059(4) | |
φ | 0.2412(2) | 0.2231(1) | 0.2857(3) | 0.5092(6) | 0.4886(5) | 0.3290(4) | ||
κ | 0.2766(2) | 0.2622(1) | 0.3286(3) | 0.4899(6) | 0.4830(5) | 0.3665(4) | ||
∑ranks | 25(2) | 18(1) | 29(3) | 72(6) | 59(5) | 45(4) |
n | Measures | Pa. | ML | MPS | ANDA | CVM | OLS | WLS |
10 | AVE | α | 1.9113(4) | 1.8080(3) | 2.1893(6) | 2.0102(5) | 1.6790(1) | 1.7030(2) |
φ | 3.4196(3) | 6.6547(5) | 13.4896(6) | 4.6929(4) | 3.1980(1.5) | 3.1980(1.5) | ||
κ | 1.6351(1) | 2.9747(5) | 3.4465(6) | 2.4926(4) | 2.2030(3) | 2.1720(2) | ||
AVAB | α | 0.4113(4) | 0.3080(3) | 0.6893(6) | 0.5102(5) | 0.1787(1) | 0.2032(2) | |
φ | 1.4196(3) | 4.6547(5) | 11.4896(6) | 2.6929(4) | 1.1978(1) | 1.1980(2) | ||
κ | 0.6351(1) | 1.9747(5) | 2.4465(6) | 1.4926(4) | 1.2034(3) | 1.1716(2) | ||
MSE | α | 0.8424(1) | 2.6556(5) | 4.2739(6) | 1.6544(4) | 1.0310(2) | 1.0990(3) | |
φ | 6.2459(1) | 242.8573(5) | 1099.2808(6) | 63.1670(4) | 34.3340(2) | 36.4950(3) | ||
κ | 2.8238(1) | 37.3047(5) | 47.8131(6) | 17.0243(4) | 12.9340(3) | 10.7970(2) | ||
RMSE | α | 0.9178(1) | 1.6296(5) | 2.0673(6) | 1.2862(4) | 1.0150(3) | 1.0480(2) | |
φ | 2.4992(1) | 15.5839(5) | 33.1554(6) | 7.9478(4) | 5.8600(2) | 6.0410(3) | ||
κ | 1.6804(1) | 6.1078(5) | 6.9147(6) | 4.1261(4) | 3.5960(3) | 3.2860(2) | ||
∑ranks | 22(1) | 56(5) | 72(6) | 50(4) | 25.5(2) | 26.5(3) | ||
50 | AVE | α | 1.6952(2) | 1.4342(1) | 1.8042(5) | 1.8992(6) | 1.7690(3) | 1.8000(4) |
φ | 2.4984(2) | 2.1884(1) | 4.9115(6) | 3.7973(4) | 3.1380(3) | 3.3830(5) | ||
κ | 1.3226(2) | 1.1667(1) | 1.9301(6) | 1.8651(5) | 1.7060(3) | 1.7400(4) | ||
AVAB | α | 0.1952(2) | 0.0658(1) | 0.3042(5) | 0.3992(6) | 0.2692(3) | 0.3001(4) | |
φ | 0.4984(2) | 0.1884(1) | 2.9115(6) | 1.7973(5) | 1.1377(3) | 1.3826(4) | ||
κ | 0.3226(2) | 0.1667(1) | 0.9301(6) | 0.8651(5) | 0.7060(3) | 0.7402(4) | ||
MSE | α | 0.3337(1) | 0.5070(2) | 1.5355(6) | 1.0502(5) | 0.7593(3) | 0.8669(4) | |
φ | 1.5498(1) | 4.0449(2) | 155.5950(6) | 60.8746(5) | 17.0518(3) | 29.0772(4) | ||
κ | 0.9269(1) | 1.7944(2) | 11.4674(6) | 6.4988(5) | 4.3962(3) | 5.1792(4) | ||
RMSE | α | 0.5776(1) | 0.7120(2) | 1.2391(6) | 1.0248(5) | 0.8714(3) | 0.9311(4) | |
φ | 1.2449(1) | 2.0112(2) | 12.4738(6) | 7.8022(5) | 4.1294(4) | 5.3923(3) | ||
κ | 0.9627(1) | 1.3396(2) | 3.3864(6) | 2.5493(5) | 2.0967(3) | 2.2758(4) | ||
∑ranks | 18(1.5) | 18(1.5) | 70(6) | 61(5) | 37(3) | 48(4) | ||
100 | AVE | α | 1.6264(2) | 1.3950(1) | 1.6954(3) | 1.7879(6) | 1.7060(4) | 1.7390(5) |
φ | 2.3116(2) | 1.9354(1) | 3.4363(6) | 3.2511(5) | 2.8230(3) | 3.0510(4) | ||
κ | 1.2179(2) | 0.9918(1) | 1.5569(4) | 1.6311(6) | 1.5010(3) | 1.5570(5) | ||
AVAB | α | 0.1264(2) | 0.1050(1) | 0.1954(3) | 0.2879(6) | 0.2062(4) | 0.2393(5) | |
φ | 0.3116(2) | 0.0646(1) | 1.4363(6) | 1.2511(5) | 0.8234(3) | 1.0510(4) | ||
κ | 0.2179(2) | 0.0082(1) | 0.5569(5) | 0.6311(6) | 0.5009(3) | 0.5567(4) | ||
MSE | α | 0.2014(1) | 0.4738(2) | 0.8545(6) | 0.7787(5) | 0.5939(3) | 0.6521(4) | |
φ | 0.8984(1) | 0.9734(2) | 56.0050(6) | 25.6484(5) | 9.4794(3) | 21.8101(4) | ||
κ | 0.5434(1) | 0.6022(2) | 5.2431(6) | 3.9995(5) | 2.7885(3) | 3.5394(4) | ||
RMSE | α | 0.4488(1) | 0.4738(2) | 0.9244(6) | 0.8824(5) | 0.7706(3) | 0.8075(4) | |
φ | 0.9478(1) | 0.9734(2) | 7.4836(6) | 5.0644(5) | 3.0789(3) | 4.6701(4) | ||
κ | 0.7372(1) | 0.7760(2) | 2.2898(6) | 1.9999(5) | 1.6699(3) | 1.8813(4) | ||
∑ranks | 18(1.5) | 18(1.5) | 63(5) | 64(6) | 38(3) | 51(4) | ||
200 | AVE | α | 1.5676(2) | 1.4003(1) | 1.5936(3) | 1.6640(6) | 1.6350(5) | 1.6210(4) |
φ | 2.1555(2) | 1.8840(1) | 2.4471(3) | 2.5978(6) | 2.5470(5) | 2.4900(4) | ||
κ | 1.1155(2) | 0.9290(1) | 1.2360(3) | 1.3342(6) | 1.2990(5) | 1.2790(4) | ||
AVAB | α | 0.0676(1) | 0.0997(3) | 0.0936(2) | 0.1640(6) | 0.1254(5) | 0.1210(4) | |
φ | 0.1555(2) | 0.1160(1) | 0.4471(3) | 0.5978(6) | 0.5470(5) | 0.4895(4) | ||
κ | 0.1155(2) | 0.0710(1) | 0.2360(3) | 0.3342(6) | 0.2988(5) | 0.2790(4) | ||
MSE | α | 0.0927(1) | 0.0972(2) | 0.3192(3) | 0.4075(6) | 0.3785(5) | 0.3419(4) | |
φ | 0.3186(2) | 0.2844(1) | 8.2814(5) | 5.1207(3) | 10.0634(6) | 5.8513(4) | ||
κ | 0.2216(2) | 0.1781(1) | 1.5037(5) | 1.4824(4) | 1.5757(6) | 1.4326(3) | ||
RMSE | α | 0.3045(2) | 0.3117(1) | 0.5650(3) | 0.6384(6) | 0.6152(5) | 0.5847(4) | |
φ | 0.5645(2) | 0.5333(1) | 2.8777(5) | 2.2629(3) | 3.1723(6) | 2.4190(4) | ||
κ | 0.4708(2) | 0.4221(1) | 1.2263(5) | 1.2175(4) | 1.2553(6) | 1.1969(3) | ||
∑ranks | 22(2) | 15(1) | 43(3) | 62(5) | 64(6) | 46(4) |
Model | Par | MLE (SE) | -LL | AIC | K-S | p-value |
EHLST | ˆα | 3.2407(0.5773) | 588.256 | 1182.513 | 0.070 | 0.263 |
ˆφ | 67.8208(27.2116) | |||||
ˆκ | 217.1097(81.7760) | |||||
EHLL | ˆα | 4.2120(2.2915) | 590.130 | 1188.260 | 0.077 | 0.165 |
ˆβ | 9.9874(1.6320) | |||||
ˆa | 4.2120(2.2951) | |||||
ˆb | 0.0186(0.0047) | |||||
EHLF | ˆα | 1.2744(0.4305) | 585.933 | 1179.867 | 0.069 | 0.267 |
ˆλ | 26.4325(15.3670) | |||||
ˆa | 0.7669(0.1661) | |||||
ˆb | 48.0005(23.9203) | |||||
EHLLL | ˆα | 0.5109(0.4213) | 583.900 | 1175.800 | 0.052 | 0.627 |
ˆλ | 0.7105(0.6145) | |||||
ˆa | 11.3008(0.9864) | |||||
ˆb | 7.5646(5.2527) |
Model | Par | MPS (SE) | LL | AIC | K-S | p-value |
EHLST | ˆα | 3.1763(0.0286) | −590.015 | 1186.000 | 0.069 | 0.269 |
ˆφ | 64.0223(1.7981) | |||||
ˆκ | 218.3550(4.2330) | |||||
EHLL | ˆα | 3.3253(0.0749) | −592.43 | 1192.900 | 0.089 | 0.072 |
ˆβ | 9.9379(0.1238) | |||||
ˆa | 3.3075(0.0745) | |||||
ˆb | 0.0318(0.0007) | |||||
EHLF | ˆα | 1.4956(0.3689) | −587.234 | 1182.500 | 0.068 | 0.300 |
ˆλ | 30.5011(13.4873) | |||||
ˆa | 0.7660(0.0970) | |||||
ˆb | 50.1275(26.6395) | |||||
EHLLL | ˆα | 0.5220(0.2593) | −585.169 | 1178.300 | 0.059 | 0.460 |
ˆλ | 0.8105(0.58140) | |||||
ˆa | 11.5673(0.7104) | |||||
ˆb | 6.9976 (7.3261) |
INF | |
Number of observations | 223 |
Mean | 0.2121 |
Median | 0.4906 |
Minimum | −104.1454 |
Maximum | 53.2217 |
Standard Deviation | 14.3681 |
Skewness | −1.3377 |
Kurtosis | 13.1953 |
Jarque-Bera | 1721.3 (p < 0.001) |
ARCH (2) | 15.406 (p < 0.001) |
GARCH (1, 1) | |||||||||||
Pa. | NORM | STD | GED | SNORM | SSTD | SGED | GHYP | JSU | GHST | NIG | EHLST |
μ | 0.6403 | 0.5656 | 0.6504'***' | 0.5158 | 0.3304 | 0.3277'***' | 0.4667 | 0.3513 | 0.1605 | 0.3589 | 0.1824 |
ω | 0.0900 | 0.7026 | 1.0661 | 0.1468'*' | 0.9007 | 1.4755'***' | 0.8444 | 1.0241'.' | 0.9637'.' | 1.2821'.' | 0.1891 |
η1 | 0.0602'*' | 0.2777'*' | 0.2536'.' | 0.0586'***' | 0.3115'*' | 0.2957'***' | 0.2904'*' | 0.3100'*' | 0.2876'*' | 0.3088'*' | 0.1112 |
η2 | 0.9292'***' | 0.7213'***' | 0.7454'***' | 0.9289'***' | 0.6875'***' | 0.7033'***' | 0.7086'***' | 0.6889'***' | 0.7114'***' | 0.6902'***' | 0.6069'***' |
η3 | - | - | - | - | - | - | - | - | - | - | - |
α | - | - | - | 0.9423'***' | - | - | 0.2500 | - | 8.1967'***' | 0.6823'.' | 1.7778'***' |
ξ | - | - | - | - | 0.8956'***' | - | - | - | - | - | - |
ν | - | 4.1458'***' | 0.9782'***' | - | 4.1743'***' | 0.8941'***' | - | - | - | - | - |
η | - | - | - | - | - | 0.9696'***' | - | - | - | - | - |
β | - | - | - | - | - | - | -0.3260 | - | -1.1452'' | -0.1358 | - |
λ | - | - | - | - | - | - | -1.9370'*' | - | - | - | - |
υ | - | - | - | - | - | - | - | -0.1940 | - | - | - |
ϑ | - | - | - | - | - | - | - | 1.3328'***' | - | - | - |
φ | - | - | - | - | - | - | - | - | - | - | 1.2070'***' |
κ | - | - | - | - | - | - | - | - | - | - | 6.6593 |
GJRGARCH (1, 1) | |||||||||||
Pa. | NORM | STD | GED | SNORM | SSTD | SGED | GHYP | JSU | GHST | NIG | EHLST |
μ | 0.8364'.' | 0.5669'.' | 0.6504'***' | 0.7550'' | 0.3233 | 0.3215'***' | 0.4650 | 0.3452 | -0.0555 | 0.3574 | 0.2001 |
ω | 0.0000 | 0.6843 | 1.1503 | 0.0000 | 0.8795 | 1.4925'***' | 0.8253 | 1.0071 | 1.2293'.' | 1.2783'' | 0.2750 |
η1 | 0.0864'*' | 0.2833'*' | 0.2414'.' | 0.0804'*' | 0.3254'*' | 0.2893'***' | 0.2978'*' | 0.3194'*' | 0.2800'*' | 0.3105'.' | 0.1692 |
η2 | 0.9397'***' | 0.7255'***' | 0.7322'***' | 0.9402'***' | 0.6912'***' | 0.7004'***' | 0.7125'***' | 0.6918'***' | 0.7322'***' | 0.6908'***' | 0.6113'***' |
η3 | −0.0643 | −0.0195 | 0.0508 | −0.0562 | −0.0371 | 0.0200 | −0.0232 | −0.0258 | −0.0292 | −0.0049 | −0.0004 |
α | - | - | - | 0.9651'***' | - | - | 0.2500 | - | 8.1943'***' | 0.6824'*' | 1.7571'***' |
ξ | - | - | - | - | 0.8923'***' | - | - | - | - | - | - |
ν | - | 4.1432'***' | 0.9821'***' | - | 4.1776'***' | 0.8923'***' | - | - | - | - | - |
η | - | - | - | - | - | 0.9694'***' | - | - | - | - | - |
β | - | - | - | - | - | - | −0.3324 | - | -1.8837'.' | −0.1365 | - |
λ | - | - | - | - | - | - | −1.9378'**' | - | - | - | - |
υ | - | - | - | - | - | - | - | -0.1988 | - | - | - |
ϑ | - | - | - | - | - | - | - | 1.3332'***' | - | - | - |
φ | - | - | - | - | - | - | - | - | - | - | 1.1858'***' |
κ | - | - | - | - | - | - | - | - | - | - | 4.2176 |
Significance level 0'***', 0.01'*', 0.05'*', 0.1'', 1 |
Model | Log-Likelihood | AIC | BIC |
GARCH-NORM | −808.135 | 7.2837 | 7.3448 |
GARCH-STD | −772.170 | 6.9701 | 7.0665 |
GARCH-GED | −776.818 | 7.0118 | 7.0882 |
GARCH-SNORM | −807.730 | 7.2891 | 7.3655 |
GARCH-SSTD | −771.494 | 6.9730 | 7.0647 |
GARCH-SGED | −775.870 | 7.0123 | 7.1040 |
GARCH-GHYP | −772.006 | 6.9866 | 7.0936 |
GARCH-JSU | −772.083 | 6.9783 | 7.0700 |
GARCH-GHST | −778.603 | 7.0368 | 7.1285 |
GARCH- NIG | −773.341 | 6.9896 | 7.0813 |
GARCH- EHLST | −768.492 | 6.9551 | 7.0620 |
GJRGARCH-NORM | −807.280 | 7.2850 | 7.3614 |
GJRGARCH-STD | −772.159 | 6.9790 | 7.0900 |
GJRGARCH-GED | −776.764 | 7.0203 | 7.1120 |
GJRGARCH-SNORM | −807.129 | 7.2926 | 7.3843 |
GJRGARCH-SSTD | −771.455 | 6.9817 | 7.0923 |
GJRGARCH-SGED | −775.843 | 7.0210 | 7.1280 |
GJRGARCH-GHYP | −771.991 | 6.9954 | 7.1177 |
GJRGARCH-JSU | −772.066 | 6.9871 | 7.0941 |
GJRGARCH-GHST | −781.977 | 7.0760 | 7.1830 |
GJRGARCH- NIG | −773.340 | 6.9986 | 7.1055 |
GJRGARCH- EHLST | −768.846 | 6.9672 | 7.0895 |
Model | Ljung-Box Statistic |
p-value | ARCH-LM Statistic | p-value |
GARCH-NORM | 3.331 | 0.9725 | 2.932 | 0.9830 |
GARCH-STD | 1.542 | 0.9988 | 1.715 | 0.9981 |
GARCH-GED | 1.797 | 0.9977 | 1.991 | 0.9964 |
GARCH-SNORM | 3.226 | 0.9756 | 2.848 | 0.9848 |
GARCH-SSTD | 1.735 | 0.9980 | 1.982 | 0.9965 |
GARCH-SGED | 1.891 | 0.9971 | 2.141 | 0.9951 |
GARCH-GHYP | 1.679 | 0.9983 | 1.893 | 0.9971 |
GARCH-JSU | 1.810 | 0.9976 | 2.073 | 0.9958 |
GARCH-GHST | 1.800 | 0.9977 | 2.033 | 0.9961 |
GARCH- NIG | 1.898 | 0.9965 | 2.176 | 0.9948 |
GARCH- EHLST | 1.511 | 0.9989 | 1.774 | 0.9978 |
GJRGARCH-NORM | 4.328 | 0.9313 | 3.617 | 0.9630 |
GJRGARCH-STD | 1.545 | 0.9988 | 1.714 | 0.9981 |
GJRGARCH-GED | 1.832 | 0.9975 | 2.054 | 0.9959 |
GJRGARCH-SNORM | 4.292 | 0.9332 | 3.610 | 0.9632 |
GJRGARCH-SSTD | 3.429 | 0.9991 | 1.997 | 0.9964 |
GJRGARCH-SGED | 1.903 | 0.9970 | 2.165 | 0.9949 |
GJRGARCH-GHYP | 1.684 | 0.9982 | 1.895 | 0.9971 |
GJRGARCH-JSU | 1.815 | 0.9976 | 2.075 | 0.9957 |
GJRGARCH-GHST | 1.849 | 0.9974 | 2.038 | 0.9960 |
GJRGARCH- NIG | 1.897 | 0.9971 | 2.172 | 0.9948 |
GJRGARCH- EHLST | 1.472 | 0.9990 | 1.711 | 0.9981 |
Model | MSE | RMSE | MAE |
GARCH-NORM | 112.720 | 10.617 | 7.011 |
GARCH-STD | 122.686 | 11.076 | 6.671 |
GARCH-GED | 122.269 | 11.057 | 6.776 |
GARCH-SNORM | 111.427 | 10.556 | 6.952 |
GARCH-SSTD | 123.930 | 11.132 | 6.650 |
GARCH-SGED | 123.902 | 11.131 | 6.764 |
GARCH-GHYP | 123.204 | 11.100 | 6.673 |
GARCH-JSU | 123.939 | 11.133 | 6.670 |
GARCH-GHST | 123.355 | 11.106 | 6.693 |
GARCH- NIG | 124.100 | 11.140 | 6.712 |
GARCH- EHLST | 109.109 | 10.445 | 5.396 |
GJRGARCH-NORM | 156.588 | 12.513 | 9.119 |
GJRGARCH-STD | 124.678 | 11.166 | 6.757 |
GJRGARCH-GED | 117.606 | 10.845 | 6.555 |
GJRGARCH-SNORM | 149.356 | 12.221 | 8.828 |
GJRGARCH-SSTD | 127.418 | 11.288 | 6.791 |
GJRGARCH-SGED | 122.197 | 11.054 | 6.686 |
GJRGARCH-GHYP | 125.462 | 11.201 | 6.768 |
GJRGARCH-JSU | 126.321 | 11.239 | 6.768 |
GJRGARCH-GHST | 126.353 | 11.241 | 6.911 |
GJRGARCH- NIG | 124.527 | 11.159 | 6.730 |
GJRGARCH- EHLST | 104.874 | 10.241 | 5.446 |
GARCH (1, 1) | |||||||||||
NORM | STD | GED | SNORM | SSTD | SGED | GHYP | JSU | GHST | NIG | EHLST | |
MSE | 3 | 5 | 4 | 2 | 9 | 8 | 6 | 10 | 7 | 11 | 1 |
RMSE | 3 | 5 | 4 | 2 | 9 | 8 | 6 | 10 | 7 | 11 | 1 |
MAE | 11 | 4 | 9 | 10 | 2 | 8 | 5 | 3 | 6 | 7 | 1 |
TOTAL | 17 | 14 | 17 | 14 | 20 | 24 | 17 | 23 | 20 | 29 | 3 |
GJRGARCH (1, 1) | |||||||||||
NORM | STD | GED | SNORM | SSTD | SGED | GHYP | JSU | GHST | NIG | EHLST | |
MSE | 11 | 5 | 2 | 10 | 9 | 3 | 6 | 7 | 8 | 4 | 1 |
RMSE | 11 | 5 | 2 | 10 | 9 | 3 | 6 | 7 | 8 | 4 | 1 |
MAE | 11 | 5 | 2 | 10 | 8 | 3 | 6.5 | 6.5 | 9 | 4 | 1 |
TOTAL | 33 | 15 | 6 | 30 | 26 | 9 | 18.5 | 20.5 | 25 | 12 | 3 |
Parameter (pa) | n | ML | MPS | ANDA | CVM | OLS | WLS |
α=1.0,φ=1.3,κ=0.5 | 10 | 2 | 5 | 6 | 4 | 1 | 3 |
50 | 2 | 1 | 6 | 5 | 4 | 3 | |
100 | 2 | 1 | 4 | 6 | 5 | 3 | |
200 | 3 | 1 | 2 | 6 | 5 | 4 | |
α=1.3,φ=1.7,κ=1.0 | 10 | 2 | 5 | 6 | 4 | 3 | 1 |
50 | 2 | 1 | 4.5 | 6 | 4.5 | 3 | |
100 | 3 | 1 | 2 | 6 | 5 | 4 | |
200 | 3 | 1 | 2 | 6 | 5 | 4 | |
α=2.0,φ=2.5,κ=1.5 | 10 | 1 | 5 | 6 | 4 | 2 | 3 |
50 | 2 | 1 | 3 | 6 | 5 | 4 | |
100 | 2 | 1 | 3 | 6 | 4 | 5 | |
200 | 2 | 1 | 3 | 6 | 5 | 4 | |
α=2.5,φ=3.0,κ=2.0 | 10 | 1 | 5 | 6 | 4 | 2 | 3 |
50 | 1.5 | 1.5 | 6 | 6 | 3 | 4 | |
100 | 1.5 | 1.5 | 5 | 6 | 3 | 4 | |
200 | 2 | 1 | 3 | 6 | 6 | 4 | |
∑ranks | 32 | 33 | 67.5 | 87 | 62.5 | 56 | |
Overall rank | 1 | 2 | 5 | 6 | 4 | 3 |
n | Measures | Pa. | ML | MPS | ANDA | CVM | OLS | WLS |
10 | AVE | α | 1.4661(5) | 1.4066(4) | 1.6544(6) | 1.3840(3) | 1.1710(2) | 1.1590(1) |
φ | 2.4233(3) | 4.8935(5) | 8.1802(6) | 2.6132(4) | 1.9540(1) | 2.0060(2) | ||
κ | 1.1683(1) | 2.3008(5) | 2.4332(6) | 1.3781(4) | 1.2180(2) | 1.2700(3) | ||
AVAB | α | 0.4661(5) | 0.4066(4) | 0.6544(6) | 0.3840(3) | 0.1713(2) | 0.1594(1) | |
φ | 1.1233(3) | 3.5935(5) | 6.8802(6) | 1.3132(4) | 0.6536(1) | 0.7063(2) | ||
κ | 0.6683(1) | 1.8008(5) | 1.9332(6) | 0.8781(4) | 0.7178(2) | 0.7701(3) | ||
MSE | α | 0.8648(3) | 2.3453(5) | 3.3241(6) | 1.0758(4) | 0.6714(1) | 0.7021(2) | |
φ | 4.1873(1) | 172.4338(5) | 472.9212(6) | 19.7630(4) | 15.0648(2) | 15.7997(3) | ||
κ | 2.4706(1) | 35.4217(5) | 36.2999(6) | 6.9764(3) | 5.3805(2) | 7.9437(4) | ||
RMSE | α | 0.9300(3) | 1.5314(5) | 1.8232(6) | 1.0372(4) | 0.8194(1) | 0.8379(2) | |
φ | 2.0463(1) | 13.1314(5) | 21.7468(6) | 4.4456(4) | 3.8813(2) | 3.9749(3) | ||
κ | 1.5718(1) | 5.9516(6) | 6.0249(7) | 2.6413(3) | 2.3196(2) | 2.8185(4) | ||
∑ranks | 28(2) | 49(5) | 73(6) | 44(4) | 20(1) | 29(3) | ||
50 | AVE | α | 1.1687(5) | 0.9853(1) | 1.1595(3) | 1.2278(6) | 1.1672(4) | 1.1501(2) |
φ | 1.5906(2) | 1.3920(1) | 2.1469(6) | 1.8565(5) | 1.7203(4) | 1.6610(3) | ||
κ | 0.7185(2) | 0.6255(1) | 0.8535(5) | 0.8632(6) | 0.8289(4) | 0.7911(3) | ||
AVAB | α | 0.1687(5) | 0.0147(1) | 0.1595(3) | 0.2278(6) | 0.1672(4) | 0.1501(2) | |
φ | 0.2906(2) | 0.0920(1) | 0.8469(6) | 0.5565(5) | 0.4203(4) | 0.3610(3) | ||
κ | 0.2185(2) | 0.1255(1) | 0.3535(4) | 0.3632(5) | 1.3812(6) | 0.2911(3) | ||
MSE | α | 0.2171(1) | 0.2513(2) | 0.5655(6) | 0.4314(5) | 0.3657(4) | 0.3091(3) | |
φ | 0.5725(1) | 2.5172(2) | 38.3736(6) | 3.8641(3) | 3.9756(4) | 4.4224(5) | ||
κ | 0.3991(1) | 0.9812(2) | 3.3622(6) | 1.5146(4) | 1.3812(3) | 1.8538(5) | ||
RMSE | α | 0.4659(1) | 0.5013(2) | 0.7520(6) | 0.6568(5) | 0.6047(4) | 0.5559(3) | |
φ | 0.7567(1) | 1.5866(2) | 6.1946(6) | 1.9657(3) | 1.9939(4) | 2.1029(5) | ||
κ | 0.6317(1) | 0.9905(2) | 1.8336(6) | 1.2307(4) | 1.1752(3) | 1.3616(5) | ||
∑ranks | 24(2) | 18(1) | 63(6) | 57(5) | 48(4) | 42(3) | ||
100 | AVE | α | 1.0768(4) | 0.9461(1) | 1.0561(2) | 1.1320(6) | 1.0928(5) | 1.0652(3) |
φ | 1.4224(2) | 1.2463(1) | 1.5157(4) | 1.6517(6) | 1.5443(5) | 1.4523(3) | ||
κ | 0.6022(2) | 0.4996(1) | 0.6226(4) | 0.7279(6) | 0.6878(5) | 0.6203(3) | ||
AVAB | α | 0.0768(4) | 0.0539(1) | 0.0561(2) | 0.1320(6) | 0.0928(5) | 0.0652(3) | |
φ | 0.1224(2) | 0.0537(1) | 0.2157(4) | 0.3517(6) | 0.2443(5) | 0.1523(3) | ||
κ | 0.1022(2) | 0.0004(1) | 0.1226(5) | 0.2279(6) | 0.1878(3) | 0.1203(4) | ||
MSE | α | 0.0831(2) | 0.0682(1) | 0.1692(4) | 0.2889(6) | 0.2350(5) | 0.1393(3) | |
φ | 0.1652(2) | 0.1829(3) | 6.2211(6) | 3.6319(5) | 2.1739(4) | 1.4473(1) | ||
κ | 0.1402(1) | 0.1508(2) | 1.0192(5) | 1.1513(6) | 0.8198(4) | 0.3606(3) | ||
RMSE | α | 0.2882(2) | 0.2612(1) | 0.4113(4) | 0.5375(6) | 0.4848(5) | 0.3732(3) | |
φ | 0.4065(1) | 0.4277(2) | 2.4942(6) | 1.9058(5) | 1.4744(4) | 1.2030(3) | ||
κ | 0.3744(1) | 0.3883(2) | 1.0096(5) | 1.0730(6) | 0.9054(4) | 0.6005(3) | ||
∑ranks | 25(2) | 17(1) | 51(4) | 70(6) | 54(5) | 35(3) | ||
200 | AVE | α | 1.0360(4) | 0.9541(1) | 1.0240(2) | 1.0588(6) | 1.0415(5) | 1.0285(3) |
φ | 1.3576(3) | 1.2478(1) | 1.3482(2) | 1.4147(6) | 1.3846(5) | 1.3593(4) | ||
κ | 0.5436(3) | 0.4775(1) | 0.5384(2) | 0.5821(6) | 0.5722(5) | 0.5493(4) | ||
AVAB | α | 0.0360(3) | 0.0459(5) | 0.0240(1) | 0.0588(6) | 0.0415(4) | 0.0285(2) | |
φ | 0.0576(3) | 0.0522(2) | 0.0482(1) | 0.1147(6) | 0.0846(5) | 0.0593(4) | ||
κ | 0.0436(3) | 0.0225(1) | 0.0384(2) | 0.0821(6) | 0.0722(5) | 0.0493(4) | ||
MSE | α | 0.0329(2) | 0.0270(1) | 0.0441(3) | 0.0917(6) | 0.0824(5) | 0.0567(4) | |
φ | 0.0624(2) | 0.0455(1) | 0.0849(3) | 0.2907(6) | 0.2382(5) | 0.1839(4) | ||
κ | 0.0417(2) | 0.0291(1) | 0.0552(3) | 0.1570(6) | 0.1399(5) | 0.0947(4) | ||
RMSE | α | 0.1813(2) | 0.1645(1) | 0.2101(3) | 0.3028(6) | 0.2871(5) | 0.2380(4) | |
φ | 0.2498(2) | 0.2133(1) | 0.2914(3) | 0.5392(6) | 0.4881(5) | 0.4289(4) | ||
κ | 0.2041(2) | 0.1706(1) | 0.2350(3) | 0.3963(6) | 0.3741(5) | 0.3077(4) | ||
∑ranks | 31(3) | 17(1) | 28(2) | 82(6) | 59(5) | 45(4) |
n | Measures | Pa. | ML | MPS | ANDA | CVM | OLS | WLS |
10 | AVE | α | 1.1024(3) | 1.1175(5) | 1.4136(6) | 1.0918(4) | 0.9769(2) | 0.9432(1) |
φ | 2.7852(4) | 5.2337(5) | 9.8213(6) | 2.7427(3) | 2.0152(2) | 1.8648(1) | ||
κ | 1.9428(1) | 4.5719(5) | 6.0877(6) | 2.7247(3) | 2.9305(4) | 2.4634(2) | ||
AVAB | α | 0.3024(4) | 0.3175(5) | 0.6136(6) | 0.2918(3) | 0.1769(2) | 0.1432(1) | |
φ | 1.2852(4) | 3.7337(5) | 8.3213(6) | 1.2427(3) | 0.5152(2) | 0.3648(1) | ||
κ | 0.9428(1) | 3.5719(5) | 5.0877(6) | 1.7247(3) | 1.9305(4) | 1.4634(2) | ||
MSE | α | 0.5045(3) | 1.4348(5) | 2.5202(6) | 0.5695(4) | 0.4175(2) | 0.3182(1) | |
φ | 7.3621(3) | 151.9961(5) | 634.1503(6) | 16.9030(4) | 6.2433(2) | 3.1990(1) | ||
κ | 7.4544(1) | 153.2414(5) | 298.9136(6) | 33.2122(3) | 40.8208(4) | 13.8077(2) | ||
RMSE | α | 0.7103(3) | 1.1978(5) | 1.5875(6) | 0.7546(4) | 0.6461(2) | 0.5641(1) | |
φ | 2.7133(3) | 12.3287(5) | 25.1823(6) | 4.1113(4) | 2.4987(2) | 1.7886(1) | ||
κ | 2.7303(1) | 12.3791(5) | 17.2891(6) | 5.7630(3) | 6.3891(4) | 3.7159(2) | ||
∑ranks | 31(2) | 60(5) | 72(6) | 41(4) | 32(3) | 16(1) | ||
50 | AVE | α | 0.8833(4) | 0.7896(1) | 0.8815(3) | 0.9182(6) | 0.8904(5) | 0.8811(2) |
φ | 1.6996(3) | 1.4800(1) | 1.8893(6) | 1.7904(5) | 1.7388(4) | 1.6990(2) | ||
κ | 1.2704(2) | 1.1696(1) | 1.4091(3) | 1.4440(5) | 1.4889(6) | 1.4167(4) | ||
AVAB | α | 0.0833(4) | 0.0104(1) | 0.0815(3) | 0.1182(6) | 0.0904(5) | 0.0811(2) | |
φ | 0.1996(3) | 0.0200(1) | 0.3893(6) | 0.2904(5) | 0.2388(4) | 0.1990(2) | ||
κ | 0.2704(2) | 0.1696(1) | 0.4091(3) | 0.4440(5) | 0.4889(6) | 0.4167(4) | ||
MSE | α | 0.0794(2) | 0.0681(1) | 0.1771(6) | 0.1309(4) | 0.1464(5) | 0.1199(3) | |
φ | 0.3262(2) | 0.3085(1) | 20.5402(6) | 0.7886(3) | 1.7903(5) | 1.0740(4) | ||
κ | 0.9907(1) | 1.1406(2) | 7.5480(6) | 1.9706(3) | 4.2142(5) | 3.7125(4) | ||
RMSE | α | 0.2819(2) | 0.2610(1) | 0.4209(6) | 0.3618(4) | 0.3826(5) | 0.3462(3) | |
φ | 0.5711(2) | 0.5554(1) | 4.5321(6) | 0.8880(3) | 1.3380(5) | 1.0363(4) | ||
κ | 0.9907(1) | 1.0680(2) | 2.7474(6) | 1.4038(3) | 2.0528(5) | 1.9268(4) | ||
∑ranks | 28(2) | 14(1) | 60(4.5) | 62(6) | 60(4.5) | 38(3) | ||
100 | AVE | α | 0.8324(5) | 0.7737(1) | 0.8214(2) | 0.8476(6) | 0.8292(4) | 0.8239(3) |
φ | 1.5765(5) | 1.4469(1) | 1.5593(3) | 1.6181(6) | 1.5676(4) | 1.5566(2) | ||
κ | 1.1040(3) | 1.0233(1) | 1.0901(2) | 1.1638(6) | 1.1483(5) | 1.1139(4) | ||
AVAB | α | 0.0324(5) | 0.0263(3) | 0.0214(1) | 0.0476(6) | 0.0292(4) | 0.0239(2) | |
φ | 0.0765(5) | 0.0531(1) | 0.0593(3) | 0.1181(6) | 0.0676(4) | 0.0566(2) | ||
κ | 0.1040(3) | 0.0233(1) | 0.0901(2) | 0.1638(6) | 0.1483(5) | 0.1139(4) | ||
MSE | α | 0.0258(2) | 0.0222(1) | 0.0298(3) | 0.0507(6) | 0.0445(5) | 0.0323(4) | |
φ | 0.0948(2) | 0.0745(1) | 0.1090(3) | 0.2038(6) | 0.1641(5) | 0.1151(4) | ||
κ | 0.2745(2) | 0.0233(1) | 0.2965(3) | 0.5554(6) | 0.4886(5) | 0.3328(4) | ||
RMSE | α | 0.1607(2) | 0.1491(1) | 0.1727(3) | 0.2251(6) | 0.2109(5) | 0.1797(4) | |
φ | 0.3079(2) | 0.2729(1) | 0.3301(3) | 0.4514(6) | 0.4051(5) | 0.3392(4) | ||
κ | 0.5240(2) | 0.4679(1) | 0.5445(3) | 0.7453(6) | 0.6990(5) | 0.5769(4) | ||
∑ranks | 38(3) | 14(1) | 31(2) | 72(6) | 56(5) | 41(4) | ||
200 | AVE | α | 0.8155(5) | 0.7798(1) | 0.8117(3) | 0.8206(6) | 0.8122(4) | 0.8102(2) |
φ | 1.5382(5) | 1.4621(1) | 1.5325(3) | 1.5549(6) | 1.5328(4) | 1.5277(2) | ||
κ | 1.0486(3) | 0.9959(1) | 1.0469(2) | 1.0692(6) | 1.0643(5) | 1.0491(4) | ||
AVAB | α | 0.0155(4) | 0.0202(5) | 0.0117(2) | 0.0206(6) | 0.0122(3) | 0.0102(1) | |
φ | 0.0382(5) | 0.0359(4) | 0.0325(2) | 0.0549(6) | 0.0328(3) | 0.0277(1) | ||
κ | 0.0486(3) | 0.0041(1) | 0.0469(2) | 0.0692(6) | 0.0643(5) | 0.0491(4) | ||
MSE | α | 0.0110(2) | 0.0109(1) | 0.0141(3) | 0.0204(6) | 0.0193(5) | 0.0144(4) | |
φ | 0.0380(2) | 0.0359(1) | 0.0460(3) | 0.0648(6) | 0.0597(5) | 0.0466(4) | ||
κ | 0.1041(2) | 0.0949(1) | 0.1258(3) | 0.1858(6) | 0.1794(5) | 0.1280(4) | ||
RMSE | α | 0.1049(1.5) | 0.1049(1.5) | 0.1186(3) | 0.1427(6) | 0.1390(5) | 0.1198(4) | |
φ | 0.1950(2) | 0.1894(1) | 0.2144(3) | 0.2546(6) | 0.2443(5) | 0.2158(4) | ||
κ | 0.3227(2) | 0.3080(1) | 0.3547(3) | 0.4310(6) | 0.4236(5) | 0.3578(4) | ||
∑ranks | 36.5(3) | 19.5(1) | 32(2) | 72(6) | 64(5) | 38(4) |
n | Measures | Pa. | ML | MPS | ANDA | CVM | OLS | WLS |
10 | AVE | α | 2.8336(3) | 2.5944(1) | 3.1558(5) | 3.3131(6) | 2.8120(2) | 2.9230(4) |
φ | 2.0036(1) | 8.0269(5) | 19.3064(6) | 5.4068(4) | 4.1680(2) | 4.4550(3) | ||
κ | 1.4579(1) | 3.2311(5) | 3.6263(6) | 3.0043(4) | 2.8950(2) | 2.9920(3) | ||
AVAB | α | 0.8336(3) | 0.5944(1) | 1.1558(5) | 1.3131(6) | 0.8124(2) | 0.9231(4) | |
φ | 1.0036(1) | 7.0269(5) | 18.3064(6) | 4.4068(4) | 3.1675(2) | 3.4554(3) | ||
κ | 0.7579(1) | 2.5311(5) | 2.9263(6) | 2.3043(4) | 2.1952(2) | 2.2918(3) | ||
MSE | α | 2.1274(1) | 6.7714(5) | 9.2849(6) | 5.0302(4) | 3.5630(2) | 3.9500(3) | |
φ | 3.3320(1) | 648.1073(5) | 3383.9749(6) | 204.2319(4) | 177.4580(3) | 152.0100(2) | ||
κ | 2.8070(1) | 49.8495(5) | 60.4505(6) | 28.6379(3) | 27.8840(2) | 33.0500(4) | ||
RMSE | α | 1.4586(1) | 2.6022(5) | 3.0471(6) | 2.2428(4) | 1.8870(2) | 1.9870(3) | |
φ | 1.8254(1) | 25.4580(5) | 58.1719(6) | 14.2910(4) | 13.3210(3) | 12.3290(2) | ||
κ | 1.6754(1) | 7.0404(5) | 7.7750(6) | 5.3514(3) | 5.2800(2) | 5.7490(4) | ||
∑ranks | 16(1) | 52(5) | 70(6) | 50(4) | 26(2) | 38(3) | ||
50 | AVE | α | 2.2547(3) | 1.8578(1) | 2.1777(2) | 2.5788(6) | 2.4490(5) | 2.3520(4) |
φ | 1.2645(2) | 1.0723(1) | 1.5965(3) | 2.3717(6) | 2.1870(5) | 2.0330(4) | ||
κ | 0.9587(2) | 0.7912(1) | 1.0157(3) | 1.4816(6) | 1.4490(5) | 1.2750(4) | ||
AVAB | α | 0.2547(3) | 0.1422(1) | 0.1777(2) | 0.5788(6) | 0.4486(5) | 0.3518(4) | |
φ | 0.2645(2) | 0.0723(1) | 0.5965(3) | 1.3717(6) | 1.1873(5) | 1.0332(4) | ||
κ | 0.2587(2) | 0.0912(1) | 0.3157(3) | 0.7816(6) | 0.7489(5) | 0.5751(4) | ||
MSE | α | 0.4982(1) | 0.6380(2) | 0.8915(3) | 1.6694(6) | 1.5060(5) | 1.1900(4) | |
φ | 0.4962(1) | 1.4375(2) | 32.6387(5) | 30.5760(4) | 28.1190(3) | 49.6000(6) | ||
κ | 0.5600(1) | 0.7722(2) | 1.7465(3) | 3.9829(5) | 4.1170(6) | 3.2910(4) | ||
RMSE | α | 0.7058(1) | 0.7988(2) | 0.9442(3) | 1.2921(6) | 1.2270(5) | 1.0910(4) | |
φ | 0.7044(1) | 1.1990(2) | 5.7130(5) | 5.5296(4) | 5.3030(3) | 7.0430(6) | ||
κ | 0.7483(1) | 0.8788(2) | 1.3215(3) | 1.9957(5) | 2.0290(6) | 1.8140(4) | ||
∑ranks | 20(2) | 18(1) | 28(3) | 64(6) | 58(5) | 52(4) | ||
100 | AVE | α | 2.1444(3) | 1.8589(1) | 2.0854(2) | 2.3221(6) | 2.2590(5) | 2.1566(4) |
φ | 1.1384(3) | 0.9579(1) | 1.1305(2) | 1.6180(5) | 1.5510(4) | 1.6937(6) | ||
κ | 0.1510(1) | 0.6947(2) | 0.8403(3) | 1.1163(6) | 1.0970(5) | 0.9608(4) | ||
AVAB | α | 0.1444(3) | 0.1411(2) | 0.0854(1) | 0.3221(6) | 0.2591(5) | 0.1566(4) | |
φ | 0.1384(3) | 0.0421(1) | 0.1305(2) | 0.6180(5) | 0.5507(4) | 0.6937(6) | ||
κ | 0.1510(3) | 0.0053(1) | 0.1403(2) | 0.4163(6) | 0.3975(5) | 0.2608(4) | ||
MSE | α | 0.2375(1) | 0.2656(2) | 0.3276(3) | 0.8324(6) | 0.7795(5) | 0.5226(4) | |
φ | 0.1731(2) | 0.1389(1) | 0.3441(3) | 9.3730(5) | 9.3187(4) | 168.1577(6) | ||
κ | 0.2335(2) | 0.2183(1) | 0.3689(3) | 1.5424(5) | 1.5302(4) | 1.6901(6) | ||
RMSE | α | 0.4873(1) | 0.5154(2) | 0.5724(3) | 0.9123(6) | 0.8829(5) | 0.7229(4) | |
φ | 0.4161(2) | 0.3728(1) | 0.5866(3) | 3.0615(5) | 3.0526(4) | 12.9676(6) | ||
κ | 0.4832(2) | 0.4672(1) | 0.6074(3) | 1.2420(5) | 1.2370(4) | 1.3001(6) | ||
∑ranks | 26(2) | 16(1) | 30(3) | 66(6) | 54(4) | 60(5) | ||
200 | AVE | α | 2.0655(4) | 1.8856(1) | 2.0368(2) | 2.1254(6) | 2.0920(5) | 2.0566(3) |
φ | 1.0615(3) | 0.9484(1) | 1.0528(2) | 1.1499(6) | 1.1250(5) | 1.0714(4) | ||
κ | 0.7647(3) | 0.6606(1) | 0.7589(2) | 0.8454(6) | 0.8340(5) | 0.7822(4) | ||
AVAB | α | 0.0655(3) | 0.1144(5) | 0.0368(1) | 0.1254(6) | 0.0922(4) | 0.0566(2) | |
φ | 0.0615(3) | 0.0516(1) | 0.0528(2) | 0.1499(6) | 0.1246(5) | 0.0714(4) | ||
κ | 0.0647(3) | 0.0394(1) | 0.0589(2) | 0.1454(6) | 0.1340(5) | 0.0822(4) | ||
MSE | α | 0.1055(1) | 0.1232(2) | 0.1489(3) | 0.2735(6) | 0.2627(5) | 0.1648(4) | |
φ | 0.0582(2) | 0.0498(1) | 0.0816(3) | 0.2593(6) | 0.2387(5) | 0.1083(4) | ||
κ | 0.0765(2) | 0.0688(1) | 0.1080(3) | 0.2400(6) | 0.2333(5) | 0.1343(4) | ||
RMSE | α | 0.3248(1) | 0.3509(2) | 0.3858(3) | 0.5229(6) | 0.5126(5) | 0.4059(4) | |
φ | 0.2412(2) | 0.2231(1) | 0.2857(3) | 0.5092(6) | 0.4886(5) | 0.3290(4) | ||
κ | 0.2766(2) | 0.2622(1) | 0.3286(3) | 0.4899(6) | 0.4830(5) | 0.3665(4) | ||
∑ranks | 25(2) | 18(1) | 29(3) | 72(6) | 59(5) | 45(4) |
n | Measures | Pa. | ML | MPS | ANDA | CVM | OLS | WLS |
10 | AVE | α | 1.9113(4) | 1.8080(3) | 2.1893(6) | 2.0102(5) | 1.6790(1) | 1.7030(2) |
φ | 3.4196(3) | 6.6547(5) | 13.4896(6) | 4.6929(4) | 3.1980(1.5) | 3.1980(1.5) | ||
κ | 1.6351(1) | 2.9747(5) | 3.4465(6) | 2.4926(4) | 2.2030(3) | 2.1720(2) | ||
AVAB | α | 0.4113(4) | 0.3080(3) | 0.6893(6) | 0.5102(5) | 0.1787(1) | 0.2032(2) | |
φ | 1.4196(3) | 4.6547(5) | 11.4896(6) | 2.6929(4) | 1.1978(1) | 1.1980(2) | ||
κ | 0.6351(1) | 1.9747(5) | 2.4465(6) | 1.4926(4) | 1.2034(3) | 1.1716(2) | ||
MSE | α | 0.8424(1) | 2.6556(5) | 4.2739(6) | 1.6544(4) | 1.0310(2) | 1.0990(3) | |
φ | 6.2459(1) | 242.8573(5) | 1099.2808(6) | 63.1670(4) | 34.3340(2) | 36.4950(3) | ||
κ | 2.8238(1) | 37.3047(5) | 47.8131(6) | 17.0243(4) | 12.9340(3) | 10.7970(2) | ||
RMSE | α | 0.9178(1) | 1.6296(5) | 2.0673(6) | 1.2862(4) | 1.0150(3) | 1.0480(2) | |
φ | 2.4992(1) | 15.5839(5) | 33.1554(6) | 7.9478(4) | 5.8600(2) | 6.0410(3) | ||
κ | 1.6804(1) | 6.1078(5) | 6.9147(6) | 4.1261(4) | 3.5960(3) | 3.2860(2) | ||
∑ranks | 22(1) | 56(5) | 72(6) | 50(4) | 25.5(2) | 26.5(3) | ||
50 | AVE | α | 1.6952(2) | 1.4342(1) | 1.8042(5) | 1.8992(6) | 1.7690(3) | 1.8000(4) |
φ | 2.4984(2) | 2.1884(1) | 4.9115(6) | 3.7973(4) | 3.1380(3) | 3.3830(5) | ||
κ | 1.3226(2) | 1.1667(1) | 1.9301(6) | 1.8651(5) | 1.7060(3) | 1.7400(4) | ||
AVAB | α | 0.1952(2) | 0.0658(1) | 0.3042(5) | 0.3992(6) | 0.2692(3) | 0.3001(4) | |
φ | 0.4984(2) | 0.1884(1) | 2.9115(6) | 1.7973(5) | 1.1377(3) | 1.3826(4) | ||
κ | 0.3226(2) | 0.1667(1) | 0.9301(6) | 0.8651(5) | 0.7060(3) | 0.7402(4) | ||
MSE | α | 0.3337(1) | 0.5070(2) | 1.5355(6) | 1.0502(5) | 0.7593(3) | 0.8669(4) | |
φ | 1.5498(1) | 4.0449(2) | 155.5950(6) | 60.8746(5) | 17.0518(3) | 29.0772(4) | ||
κ | 0.9269(1) | 1.7944(2) | 11.4674(6) | 6.4988(5) | 4.3962(3) | 5.1792(4) | ||
RMSE | α | 0.5776(1) | 0.7120(2) | 1.2391(6) | 1.0248(5) | 0.8714(3) | 0.9311(4) | |
φ | 1.2449(1) | 2.0112(2) | 12.4738(6) | 7.8022(5) | 4.1294(4) | 5.3923(3) | ||
κ | 0.9627(1) | 1.3396(2) | 3.3864(6) | 2.5493(5) | 2.0967(3) | 2.2758(4) | ||
∑ranks | 18(1.5) | 18(1.5) | 70(6) | 61(5) | 37(3) | 48(4) | ||
100 | AVE | α | 1.6264(2) | 1.3950(1) | 1.6954(3) | 1.7879(6) | 1.7060(4) | 1.7390(5) |
φ | 2.3116(2) | 1.9354(1) | 3.4363(6) | 3.2511(5) | 2.8230(3) | 3.0510(4) | ||
κ | 1.2179(2) | 0.9918(1) | 1.5569(4) | 1.6311(6) | 1.5010(3) | 1.5570(5) | ||
AVAB | α | 0.1264(2) | 0.1050(1) | 0.1954(3) | 0.2879(6) | 0.2062(4) | 0.2393(5) | |
φ | 0.3116(2) | 0.0646(1) | 1.4363(6) | 1.2511(5) | 0.8234(3) | 1.0510(4) | ||
κ | 0.2179(2) | 0.0082(1) | 0.5569(5) | 0.6311(6) | 0.5009(3) | 0.5567(4) | ||
MSE | α | 0.2014(1) | 0.4738(2) | 0.8545(6) | 0.7787(5) | 0.5939(3) | 0.6521(4) | |
φ | 0.8984(1) | 0.9734(2) | 56.0050(6) | 25.6484(5) | 9.4794(3) | 21.8101(4) | ||
κ | 0.5434(1) | 0.6022(2) | 5.2431(6) | 3.9995(5) | 2.7885(3) | 3.5394(4) | ||
RMSE | α | 0.4488(1) | 0.4738(2) | 0.9244(6) | 0.8824(5) | 0.7706(3) | 0.8075(4) | |
φ | 0.9478(1) | 0.9734(2) | 7.4836(6) | 5.0644(5) | 3.0789(3) | 4.6701(4) | ||
κ | 0.7372(1) | 0.7760(2) | 2.2898(6) | 1.9999(5) | 1.6699(3) | 1.8813(4) | ||
∑ranks | 18(1.5) | 18(1.5) | 63(5) | 64(6) | 38(3) | 51(4) | ||
200 | AVE | α | 1.5676(2) | 1.4003(1) | 1.5936(3) | 1.6640(6) | 1.6350(5) | 1.6210(4) |
φ | 2.1555(2) | 1.8840(1) | 2.4471(3) | 2.5978(6) | 2.5470(5) | 2.4900(4) | ||
κ | 1.1155(2) | 0.9290(1) | 1.2360(3) | 1.3342(6) | 1.2990(5) | 1.2790(4) | ||
AVAB | α | 0.0676(1) | 0.0997(3) | 0.0936(2) | 0.1640(6) | 0.1254(5) | 0.1210(4) | |
φ | 0.1555(2) | 0.1160(1) | 0.4471(3) | 0.5978(6) | 0.5470(5) | 0.4895(4) | ||
κ | 0.1155(2) | 0.0710(1) | 0.2360(3) | 0.3342(6) | 0.2988(5) | 0.2790(4) | ||
MSE | α | 0.0927(1) | 0.0972(2) | 0.3192(3) | 0.4075(6) | 0.3785(5) | 0.3419(4) | |
φ | 0.3186(2) | 0.2844(1) | 8.2814(5) | 5.1207(3) | 10.0634(6) | 5.8513(4) | ||
κ | 0.2216(2) | 0.1781(1) | 1.5037(5) | 1.4824(4) | 1.5757(6) | 1.4326(3) | ||
RMSE | α | 0.3045(2) | 0.3117(1) | 0.5650(3) | 0.6384(6) | 0.6152(5) | 0.5847(4) | |
φ | 0.5645(2) | 0.5333(1) | 2.8777(5) | 2.2629(3) | 3.1723(6) | 2.4190(4) | ||
κ | 0.4708(2) | 0.4221(1) | 1.2263(5) | 1.2175(4) | 1.2553(6) | 1.1969(3) | ||
∑ranks | 22(2) | 15(1) | 43(3) | 62(5) | 64(6) | 46(4) |
Model | Par | MLE (SE) | -LL | AIC | K-S | p-value |
EHLST | ˆα | 3.2407(0.5773) | 588.256 | 1182.513 | 0.070 | 0.263 |
ˆφ | 67.8208(27.2116) | |||||
ˆκ | 217.1097(81.7760) | |||||
EHLL | ˆα | 4.2120(2.2915) | 590.130 | 1188.260 | 0.077 | 0.165 |
ˆβ | 9.9874(1.6320) | |||||
ˆa | 4.2120(2.2951) | |||||
ˆb | 0.0186(0.0047) | |||||
EHLF | ˆα | 1.2744(0.4305) | 585.933 | 1179.867 | 0.069 | 0.267 |
ˆλ | 26.4325(15.3670) | |||||
ˆa | 0.7669(0.1661) | |||||
ˆb | 48.0005(23.9203) | |||||
EHLLL | ˆα | 0.5109(0.4213) | 583.900 | 1175.800 | 0.052 | 0.627 |
ˆλ | 0.7105(0.6145) | |||||
ˆa | 11.3008(0.9864) | |||||
ˆb | 7.5646(5.2527) |
Model | Par | MPS (SE) | LL | AIC | K-S | p-value |
EHLST | ˆα | 3.1763(0.0286) | −590.015 | 1186.000 | 0.069 | 0.269 |
ˆφ | 64.0223(1.7981) | |||||
ˆκ | 218.3550(4.2330) | |||||
EHLL | ˆα | 3.3253(0.0749) | −592.43 | 1192.900 | 0.089 | 0.072 |
ˆβ | 9.9379(0.1238) | |||||
ˆa | 3.3075(0.0745) | |||||
ˆb | 0.0318(0.0007) | |||||
EHLF | ˆα | 1.4956(0.3689) | −587.234 | 1182.500 | 0.068 | 0.300 |
ˆλ | 30.5011(13.4873) | |||||
ˆa | 0.7660(0.0970) | |||||
ˆb | 50.1275(26.6395) | |||||
EHLLL | ˆα | 0.5220(0.2593) | −585.169 | 1178.300 | 0.059 | 0.460 |
ˆλ | 0.8105(0.58140) | |||||
ˆa | 11.5673(0.7104) | |||||
ˆb | 6.9976 (7.3261) |
INF | |
Number of observations | 223 |
Mean | 0.2121 |
Median | 0.4906 |
Minimum | −104.1454 |
Maximum | 53.2217 |
Standard Deviation | 14.3681 |
Skewness | −1.3377 |
Kurtosis | 13.1953 |
Jarque-Bera | 1721.3 (p < 0.001) |
ARCH (2) | 15.406 (p < 0.001) |
GARCH (1, 1) | |||||||||||
Pa. | NORM | STD | GED | SNORM | SSTD | SGED | GHYP | JSU | GHST | NIG | EHLST |
μ | 0.6403 | 0.5656 | 0.6504'***' | 0.5158 | 0.3304 | 0.3277'***' | 0.4667 | 0.3513 | 0.1605 | 0.3589 | 0.1824 |
ω | 0.0900 | 0.7026 | 1.0661 | 0.1468'*' | 0.9007 | 1.4755'***' | 0.8444 | 1.0241'.' | 0.9637'.' | 1.2821'.' | 0.1891 |
η1 | 0.0602'*' | 0.2777'*' | 0.2536'.' | 0.0586'***' | 0.3115'*' | 0.2957'***' | 0.2904'*' | 0.3100'*' | 0.2876'*' | 0.3088'*' | 0.1112 |
η2 | 0.9292'***' | 0.7213'***' | 0.7454'***' | 0.9289'***' | 0.6875'***' | 0.7033'***' | 0.7086'***' | 0.6889'***' | 0.7114'***' | 0.6902'***' | 0.6069'***' |
η3 | - | - | - | - | - | - | - | - | - | - | - |
α | - | - | - | 0.9423'***' | - | - | 0.2500 | - | 8.1967'***' | 0.6823'.' | 1.7778'***' |
ξ | - | - | - | - | 0.8956'***' | - | - | - | - | - | - |
ν | - | 4.1458'***' | 0.9782'***' | - | 4.1743'***' | 0.8941'***' | - | - | - | - | - |
η | - | - | - | - | - | 0.9696'***' | - | - | - | - | - |
β | - | - | - | - | - | - | -0.3260 | - | -1.1452'' | -0.1358 | - |
λ | - | - | - | - | - | - | -1.9370'*' | - | - | - | - |
υ | - | - | - | - | - | - | - | -0.1940 | - | - | - |
ϑ | - | - | - | - | - | - | - | 1.3328'***' | - | - | - |
φ | - | - | - | - | - | - | - | - | - | - | 1.2070'***' |
κ | - | - | - | - | - | - | - | - | - | - | 6.6593 |
GJRGARCH (1, 1) | |||||||||||
Pa. | NORM | STD | GED | SNORM | SSTD | SGED | GHYP | JSU | GHST | NIG | EHLST |
μ | 0.8364'.' | 0.5669'.' | 0.6504'***' | 0.7550'' | 0.3233 | 0.3215'***' | 0.4650 | 0.3452 | -0.0555 | 0.3574 | 0.2001 |
ω | 0.0000 | 0.6843 | 1.1503 | 0.0000 | 0.8795 | 1.4925'***' | 0.8253 | 1.0071 | 1.2293'.' | 1.2783'' | 0.2750 |
η1 | 0.0864'*' | 0.2833'*' | 0.2414'.' | 0.0804'*' | 0.3254'*' | 0.2893'***' | 0.2978'*' | 0.3194'*' | 0.2800'*' | 0.3105'.' | 0.1692 |
η2 | 0.9397'***' | 0.7255'***' | 0.7322'***' | 0.9402'***' | 0.6912'***' | 0.7004'***' | 0.7125'***' | 0.6918'***' | 0.7322'***' | 0.6908'***' | 0.6113'***' |
η3 | −0.0643 | −0.0195 | 0.0508 | −0.0562 | −0.0371 | 0.0200 | −0.0232 | −0.0258 | −0.0292 | −0.0049 | −0.0004 |
α | - | - | - | 0.9651'***' | - | - | 0.2500 | - | 8.1943'***' | 0.6824'*' | 1.7571'***' |
ξ | - | - | - | - | 0.8923'***' | - | - | - | - | - | - |
ν | - | 4.1432'***' | 0.9821'***' | - | 4.1776'***' | 0.8923'***' | - | - | - | - | - |
η | - | - | - | - | - | 0.9694'***' | - | - | - | - | - |
β | - | - | - | - | - | - | −0.3324 | - | -1.8837'.' | −0.1365 | - |
λ | - | - | - | - | - | - | −1.9378'**' | - | - | - | - |
υ | - | - | - | - | - | - | - | -0.1988 | - | - | - |
ϑ | - | - | - | - | - | - | - | 1.3332'***' | - | - | - |
φ | - | - | - | - | - | - | - | - | - | - | 1.1858'***' |
κ | - | - | - | - | - | - | - | - | - | - | 4.2176 |
Significance level 0'***', 0.01'*', 0.05'*', 0.1'', 1 |
Model | Log-Likelihood | AIC | BIC |
GARCH-NORM | −808.135 | 7.2837 | 7.3448 |
GARCH-STD | −772.170 | 6.9701 | 7.0665 |
GARCH-GED | −776.818 | 7.0118 | 7.0882 |
GARCH-SNORM | −807.730 | 7.2891 | 7.3655 |
GARCH-SSTD | −771.494 | 6.9730 | 7.0647 |
GARCH-SGED | −775.870 | 7.0123 | 7.1040 |
GARCH-GHYP | −772.006 | 6.9866 | 7.0936 |
GARCH-JSU | −772.083 | 6.9783 | 7.0700 |
GARCH-GHST | −778.603 | 7.0368 | 7.1285 |
GARCH- NIG | −773.341 | 6.9896 | 7.0813 |
GARCH- EHLST | −768.492 | 6.9551 | 7.0620 |
GJRGARCH-NORM | −807.280 | 7.2850 | 7.3614 |
GJRGARCH-STD | −772.159 | 6.9790 | 7.0900 |
GJRGARCH-GED | −776.764 | 7.0203 | 7.1120 |
GJRGARCH-SNORM | −807.129 | 7.2926 | 7.3843 |
GJRGARCH-SSTD | −771.455 | 6.9817 | 7.0923 |
GJRGARCH-SGED | −775.843 | 7.0210 | 7.1280 |
GJRGARCH-GHYP | −771.991 | 6.9954 | 7.1177 |
GJRGARCH-JSU | −772.066 | 6.9871 | 7.0941 |
GJRGARCH-GHST | −781.977 | 7.0760 | 7.1830 |
GJRGARCH- NIG | −773.340 | 6.9986 | 7.1055 |
GJRGARCH- EHLST | −768.846 | 6.9672 | 7.0895 |
Model | Ljung-Box Statistic |
p-value | ARCH-LM Statistic | p-value |
GARCH-NORM | 3.331 | 0.9725 | 2.932 | 0.9830 |
GARCH-STD | 1.542 | 0.9988 | 1.715 | 0.9981 |
GARCH-GED | 1.797 | 0.9977 | 1.991 | 0.9964 |
GARCH-SNORM | 3.226 | 0.9756 | 2.848 | 0.9848 |
GARCH-SSTD | 1.735 | 0.9980 | 1.982 | 0.9965 |
GARCH-SGED | 1.891 | 0.9971 | 2.141 | 0.9951 |
GARCH-GHYP | 1.679 | 0.9983 | 1.893 | 0.9971 |
GARCH-JSU | 1.810 | 0.9976 | 2.073 | 0.9958 |
GARCH-GHST | 1.800 | 0.9977 | 2.033 | 0.9961 |
GARCH- NIG | 1.898 | 0.9965 | 2.176 | 0.9948 |
GARCH- EHLST | 1.511 | 0.9989 | 1.774 | 0.9978 |
GJRGARCH-NORM | 4.328 | 0.9313 | 3.617 | 0.9630 |
GJRGARCH-STD | 1.545 | 0.9988 | 1.714 | 0.9981 |
GJRGARCH-GED | 1.832 | 0.9975 | 2.054 | 0.9959 |
GJRGARCH-SNORM | 4.292 | 0.9332 | 3.610 | 0.9632 |
GJRGARCH-SSTD | 3.429 | 0.9991 | 1.997 | 0.9964 |
GJRGARCH-SGED | 1.903 | 0.9970 | 2.165 | 0.9949 |
GJRGARCH-GHYP | 1.684 | 0.9982 | 1.895 | 0.9971 |
GJRGARCH-JSU | 1.815 | 0.9976 | 2.075 | 0.9957 |
GJRGARCH-GHST | 1.849 | 0.9974 | 2.038 | 0.9960 |
GJRGARCH- NIG | 1.897 | 0.9971 | 2.172 | 0.9948 |
GJRGARCH- EHLST | 1.472 | 0.9990 | 1.711 | 0.9981 |
Model | MSE | RMSE | MAE |
GARCH-NORM | 112.720 | 10.617 | 7.011 |
GARCH-STD | 122.686 | 11.076 | 6.671 |
GARCH-GED | 122.269 | 11.057 | 6.776 |
GARCH-SNORM | 111.427 | 10.556 | 6.952 |
GARCH-SSTD | 123.930 | 11.132 | 6.650 |
GARCH-SGED | 123.902 | 11.131 | 6.764 |
GARCH-GHYP | 123.204 | 11.100 | 6.673 |
GARCH-JSU | 123.939 | 11.133 | 6.670 |
GARCH-GHST | 123.355 | 11.106 | 6.693 |
GARCH- NIG | 124.100 | 11.140 | 6.712 |
GARCH- EHLST | 109.109 | 10.445 | 5.396 |
GJRGARCH-NORM | 156.588 | 12.513 | 9.119 |
GJRGARCH-STD | 124.678 | 11.166 | 6.757 |
GJRGARCH-GED | 117.606 | 10.845 | 6.555 |
GJRGARCH-SNORM | 149.356 | 12.221 | 8.828 |
GJRGARCH-SSTD | 127.418 | 11.288 | 6.791 |
GJRGARCH-SGED | 122.197 | 11.054 | 6.686 |
GJRGARCH-GHYP | 125.462 | 11.201 | 6.768 |
GJRGARCH-JSU | 126.321 | 11.239 | 6.768 |
GJRGARCH-GHST | 126.353 | 11.241 | 6.911 |
GJRGARCH- NIG | 124.527 | 11.159 | 6.730 |
GJRGARCH- EHLST | 104.874 | 10.241 | 5.446 |
GARCH (1, 1) | |||||||||||
NORM | STD | GED | SNORM | SSTD | SGED | GHYP | JSU | GHST | NIG | EHLST | |
MSE | 3 | 5 | 4 | 2 | 9 | 8 | 6 | 10 | 7 | 11 | 1 |
RMSE | 3 | 5 | 4 | 2 | 9 | 8 | 6 | 10 | 7 | 11 | 1 |
MAE | 11 | 4 | 9 | 10 | 2 | 8 | 5 | 3 | 6 | 7 | 1 |
TOTAL | 17 | 14 | 17 | 14 | 20 | 24 | 17 | 23 | 20 | 29 | 3 |
GJRGARCH (1, 1) | |||||||||||
NORM | STD | GED | SNORM | SSTD | SGED | GHYP | JSU | GHST | NIG | EHLST | |
MSE | 11 | 5 | 2 | 10 | 9 | 3 | 6 | 7 | 8 | 4 | 1 |
RMSE | 11 | 5 | 2 | 10 | 9 | 3 | 6 | 7 | 8 | 4 | 1 |
MAE | 11 | 5 | 2 | 10 | 8 | 3 | 6.5 | 6.5 | 9 | 4 | 1 |
TOTAL | 33 | 15 | 6 | 30 | 26 | 9 | 18.5 | 20.5 | 25 | 12 | 3 |