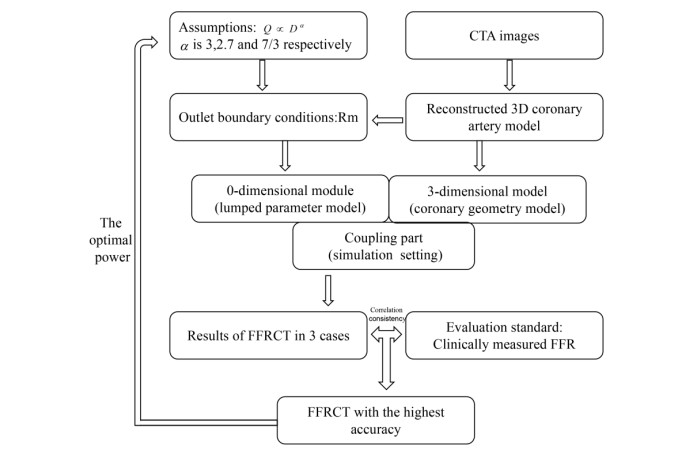
The unmanned aerial vehicle (UAV), as a remote sensing platform, has attracted many researchers in precision agriculture because of its operational flexibility and capability of producing high spatial and temporal resolution images of agricultural fields. This study proposed machine learning (ML) models and their ensembles for peanut yield prediction using UAV multispectral data. We utilized five bands (red, green, blue, near-infra-red (NIR) and red-edge) multispectral images acquired at various growth stages of peanuts using UAV. The correlation between spectral bands and yield was analyzed for each growth stage, which showed that the maturity stages had a significant correlation between peanut yield and spectral bands: red, green, NIR and red edge (REDE). Using these four bands spectral data, we assessed the potential for peanut yield prediction using multiple linear regression and seven non-linear ML models whose hyperparameters were optimized using simulated annealing (SA). The best three ML models, random forest (RF), support vector machine (SVM) and XGBoost, were then selected to construct a cooperative yield prediction framework with both the best ML model and the ensemble scheme from the best three as comparable recommendations to the farmers.
Citation: Tej Bahadur Shahi, Cheng-Yuan Xu, Arjun Neupane, Dayle B. Fleischfresser, Daniel J. O'Connor, Graeme C. Wright, William Guo. Peanut yield prediction with UAV multispectral imagery using a cooperative machine learning approach[J]. Electronic Research Archive, 2023, 31(6): 3343-3361. doi: 10.3934/era.2023169
[1] | Honghui Zhang, Jun Xia, Yinlong Yang, Qingqing Yang, Hongfang Song, Jinjie Xie, Yue Ma, Yang Hou, Aike Qiao . Branch flow distribution approach and its application in the calculation of fractional flow reserve in stenotic coronary artery. Mathematical Biosciences and Engineering, 2021, 18(5): 5978-5994. doi: 10.3934/mbe.2021299 |
[2] | Li Cai, Qian Zhong, Juan Xu, Yuan Huang, Hao Gao . A lumped parameter model for evaluating coronary artery blood supply capacity. Mathematical Biosciences and Engineering, 2024, 21(4): 5838-5862. doi: 10.3934/mbe.2024258 |
[3] | Benchawan Wiwatanapataphee, Yong Hong Wu, Thanongchai Siriapisith, Buraskorn Nuntadilok . Effect of branchings on blood flow in the system of human coronary arteries. Mathematical Biosciences and Engineering, 2012, 9(1): 199-214. doi: 10.3934/mbe.2012.9.199 |
[4] | Alberto M. Gambaruto, João Janela, Alexandra Moura, Adélia Sequeira . Sensitivity of hemodynamics in a patient specific cerebral aneurysm to vascular geometry and blood rheology. Mathematical Biosciences and Engineering, 2011, 8(2): 409-423. doi: 10.3934/mbe.2011.8.409 |
[5] | Mette S. Olufsen, Ali Nadim . On deriving lumped models for blood flow and pressure in the systemic arteries. Mathematical Biosciences and Engineering, 2004, 1(1): 61-80. doi: 10.3934/mbe.2004.1.61 |
[6] | Nattawan Chuchalerm, Wannika Sawangtong, Benchawan Wiwatanapataphee, Thanongchai Siriapisith . Study of Non-Newtonian blood flow - heat transfer characteristics in the human coronary system with an external magnetic field. Mathematical Biosciences and Engineering, 2022, 19(9): 9550-9570. doi: 10.3934/mbe.2022444 |
[7] | Xu Bie, Yuanyuan Tang, Ming Zhao, Yingxi Liu, Shen Yu, Dong Sun, Jing Liu, Ying Wang, Jianing Zhang, Xiuzhen Sun . Pilot study of pressure-flow properties in a numerical model of the middle ear. Mathematical Biosciences and Engineering, 2020, 17(3): 2418-2431. doi: 10.3934/mbe.2020131 |
[8] | A. Newton Licciardi Jr., L.H.A. Monteiro . A network model of social contacts with small-world and scale-free features, tunable connectivity, and geographic restrictions. Mathematical Biosciences and Engineering, 2024, 21(4): 4801-4813. doi: 10.3934/mbe.2024211 |
[9] | Derek H. Justice, H. Joel Trussell, Mette S. Olufsen . Analysis of Blood Flow Velocity and Pressure Signals using the Multipulse Method. Mathematical Biosciences and Engineering, 2006, 3(2): 419-440. doi: 10.3934/mbe.2006.3.419 |
[10] | Juan Palomares-Ruiz, Efrén Ruelas, Flavio Muñoz, José Castro, Angel Rodríguez . A fractional approach to 3D artery simulation under a regular pulse load. Mathematical Biosciences and Engineering, 2020, 17(3): 2516-2529. doi: 10.3934/mbe.2020138 |
The unmanned aerial vehicle (UAV), as a remote sensing platform, has attracted many researchers in precision agriculture because of its operational flexibility and capability of producing high spatial and temporal resolution images of agricultural fields. This study proposed machine learning (ML) models and their ensembles for peanut yield prediction using UAV multispectral data. We utilized five bands (red, green, blue, near-infra-red (NIR) and red-edge) multispectral images acquired at various growth stages of peanuts using UAV. The correlation between spectral bands and yield was analyzed for each growth stage, which showed that the maturity stages had a significant correlation between peanut yield and spectral bands: red, green, NIR and red edge (REDE). Using these four bands spectral data, we assessed the potential for peanut yield prediction using multiple linear regression and seven non-linear ML models whose hyperparameters were optimized using simulated annealing (SA). The best three ML models, random forest (RF), support vector machine (SVM) and XGBoost, were then selected to construct a cooperative yield prediction framework with both the best ML model and the ensemble scheme from the best three as comparable recommendations to the farmers.
The coronary fraction flow reserve (FFR) represents the gold standard for the evaluation of myocardial ischemia [1,2,3,4]. However, it is measured through an invasive operation with adenosine injection; this represents a limitation in some patients who are intolerant to adenosine [3,5]. The rapid advancements in computational fluid dynamics and medical images made it possible to perform a personalized hemodynamic simulation based on medical images. By combining the advantages of computed tomography imaging (CTA) and FFR, a new non-invasive technique (FFRCT) was proposed to assess the coronary artery stenosis from both anatomical and functional aspects [6,7,8,9]. The consistency of FFRCT and FFR was evaluated in the multi-center, early prospective study of DISCOVER-FLOW (Diagnosis of Ischemia-Causing Stenoses Obtained Via Noninvasive Fractional Flow Reserve) [7,10,11]. The results showed a good consistency.
Taylor et al. laid the foundation for the numerical calculation of FFRCT in HeartFlow NXT [4,9,10,11,12,13]. Calculating FFR based on the coronary CTA image includes five basic processes: 1) Based on the CTA images, the patient's accurate and personalized anatomical model of the epicardial coronary artery is constructed; 2) Based on the assumption that there is no vascular stenosis, the total coronary flow and the flow of each branch in the resting state are accurately evaluated; 3) Coronary microcirculation resistance is calculated at rest; 4) The change in the coronary microcirculation resistance is quantified under maximum hyperemia; 5) The control equation (N-S equation) of the coronary fluid is numerically calculated to obtain the flow rate and pressure in the coronary artery in the resting and hyperemic states, and FFR is calculated. Combining anatomy, physiology and computational fluid dynamics makes it possible to calculate the blood flow and pressure in the coronary arteries in a hyperemic state. However, in the second step, the coronary artery branch flow cannot be measured in the clinic, so the subsequent calculations cannot be performed. To solve this problem, the most commonly used method is based on the assumption of the blood flow diameter scaling law to evaluate the flow in bifurcated vessels.
The power-law scaling relationship has been assumed to be ubiquitous in biology and it is relevant to the domains of medicine, nutrition and ecology [14,15,16]. Eighty years ago, Murray proposed a compromise between the frictional and metabolic cost, expressed as a cost function. Murray's law predicts a universal exponent that is invariant (3.0) for all vascular trees with internal flows obeying laminar conditions [15,17,18]. Unlike Murray's law, Zhou et al. showed that the exponent of the blood-diameter scaling law is not necessarily equal to 3.0, but it depends on the ratio of the metabolic to viscous power dissipation of the tree of interest [19,20]. Subsequently, Lucian et al. proposed several power coefficient values (ranging from 2.1 to 3) based on the assumption that blood flow requires minimal energy, and they verified their suggestions with a bifurcated asymmetric vascular tree [21]. Eventually, 2.7 was extrapolated as the best exponent of the blood-diameter scaling law. Besides, Yunlong Huo et al. did not reference empirical parameters when constructing the coronary artery tree. They derived that the blood flow of the bifurcated vessel is proportional to the 7/3 power of the diameter based on the relationship between the predicted volume and diameter of the intraspecies scaling theory index 3.0 [17,22,23]. The above-mentioned widely used blood flow-diameter scaling laws are obtained by performing least-squares fitting of various organs through a variety of animal experiments. However, for many blood flow diameter scaling laws, which one is suitable for the blood flow distribution of human coronary bifurcation vessels and how to perform the numerical calculation of FFRCT [24,25,26].
This study retrospectively calculated the microcirculation resistance under different blood flow-diameter scaling laws of 3, 2.7 and 7/3 and used it as the exit boundary condition of FFRCT in the simulation calculation. The accuracy of FFRCT was evaluated with invasive FFR as the standard, which derived the optimal blood flow-diameter scaling law that is suitable for the FFRCT simulation calculation. This provides a theoretical basis for the blood flow distribution of coronary bifurcation vessels and is significant for modifying the 0D/3D coupling model to improve the accuracy of FFRCT.
In this study, an open-loop 0D/3D geometric multi-scale model was used to numerically simulate FFRCT under different blood flow diameter scaling laws. The accuracy of the simulation results of the three methods was evaluated with the clinically measured FFR as the evaluation standard. The whole research process included four main parts: the collection of physiological parameters and structural parameters, Rm calculation, the 0D/3D multi-scale model setting and comparative analysis of the simulation results, as shown in Figure 1.
This study was designed as a retrospective study and approved by the ethics committee of Peking University People's Hospital. The coronary artery disease (CAD) patients enrolled in the group signed an informed consent form.
The entire enrollment and exclusion process is shown in Figure 2. In detail, the inclusion and exclusion criteria of 50 stable and unstable CAD patients should meet the diagnostic criteria of local CAD patients. 9 patients were excluded due to clinical instability, 1 patient had arrhythmia, 3 patients had poor CT image quality resulting in incomplete reconstruction of the coronary artery tree, 7 patients were excluded due to stenosis before the first bifurcation node (the stenosis of 5 patients was located in the left main stem, while the stenosis of 2 patients was located in the proximal right coronary segment), 2 patients were excluded due to incomplete ultrasound results, 2 patients were excluded due to diabetes with suspected microcirculation dysfunction. Finally, 26 patients were enrolled in the group. Among which 19 patients had non-myocardial ischemia and 7 patients had myocardial ischemia.
Before invasive FFR measurement, multiple clinical examinations were required, such as brachial artery blood pressure measurement, CTA examination, ultrasound examination and biochemical examination. The FFR value was obtained in standard operating procedures. The patients were all in the supine position, and under the digital subtraction angiography machine (DSA, PHILIPS, Netherlands), adenosine triphosphate was injected at a uniform rate of 140~180 μg/kg/min to make the coronary blood vessels reach the maximum hyperemia state. The interval between various inspections should not exceed 30 days.
The necessary information for the calculation of Rm was the physiological parameters of the patient and the structural parameters of the coronary arteries. The patient's physiological parameters mainly included the heart rate (HR), systolic blood pressure (SP), diastolic blood pressure (DP), left ventricular end-systolic volume (ESV) and left ventricular end-diastolic volume (EDV), which could be retrieved from the Hospital Information System (HIS). As for the structural information of the coronary artery, it included the length of the blood vessel (Lv) and its cross-sectional area of the blood vessel (Av), which were measured from the coronary artery reconstructed based on the patient's CTA images.
The reconstruction of the three-dimensional (3D) model was based on the patient's computed tomography image (CT). In this study, all coronary CTA examinations were performed on CT scanners with ≥ 64 detector rows (Revolution, GE, USA) and double cylinder high-pressure syringe (STELLANT, MEDRAD Inc., USA). The CT images in DICOM format were imported into Mimics Version 19.0 (Materialise, Inc., Belgium) software. Two professional technicians manually reconstructed the coronary artery tree and performed cross-checking. Coronary angiography was used as a control, and coronary vessels with a diameter of more than 1 mm were preserved. If the reconstructed model was different, then a third professional technician would perform the reconstruction. Finally, physicians with more than 10 years of clinical experience were invited to check all reconstructed 3D models to ensure the accuracy of the models.
Each bifurcation of a blood vessel was represented as a node, and the distance between every two nodes was defined as the length of the vessel. The centerline measurement method was more accurate, which could avoid the error caused by the blood vessel surface measurement. The size of the cross-sectional area was the size of the area where the diameter of the blood vessel did not drastically change after each node. It was necessary to ensure that the cross-section was perpendicular to the centerline of the blood vessel as much as possible [27,28], as shown in Figure 3.
The numerical calculation of Rm was carried out in an ideal state, i.e., the blood vessel was not narrowed. Based on the pressure drop and the mean blood vessel flow, the equivalent resistance for each coronary artery tree could be calculated, as shown in Eq (11). However, the pressure drop and flow could not be directly obtained in the real coronary artery model, and the calculation needed to be started from the coronary artery root.
Taken the model in Figure 4A as an example, the stenosis was located in the proximal part of LAD, and the resistance of the arterioles and capillaries after the bifurcation of the blood vessel was R6m. The entire left coronary artery could be simplified as shown in Figure 4B. According to the principle of blood vessel modeling, the bifurcated blood vessel was equivalent to a parallel circuit [29], as shown in Figure 4C.
In this process, the pressure of the right atrium was assumed to be 0 mmHg. The cardiac output Qco could be obtained through ultrasonic measurement of EDV and ESV, assuming that the coronary flow Qcor was 4% of the cardiac output, and the left coronary flow Ql represented 60% of coronary flow [2,9,10,30]. The pressure P of the coronary artery was the mean arterial pressure, as shown in the Eqs (1)-(4).
Qco=(EDV−ESV)∗HR | (1) |
Qcor=Qco∗4% | (2) |
Ql=60%Qcor | (3) |
P=(SP+2DP)/3 | (4) |
The resistance of normal blood vessels was mainly caused by the blood viscosity. Coronary vessels were divided according to the bifurcation nodes, and the resistance of the vessels between each two nodes, such as R5, R6 and R11, could be calculated according to Eq (5).
Rv=8πηLVA2V | (5) |
where Lv denoted the length of the blood vessels of two branch nodes, Av was the cross-sectional area at the beginning of the vessel, and ηrepresented a blood viscosity 0.0035 Pa·s.
The blood flow was proportional to the power of the diameter, and the flow of bifurcated blood vessels was obtained by Eq (6), in which i represented the number of forked nodes, i.e., the number of forked layers (i = 1, 2, 3...), and j represented the number of bifurcated vessels on a bifurcation node (j = 1, 2, 3...). In this study, the Rm values when α was 3, 2.7 and 7/3 were calculated and recorded as 3Rm, 2.7Rm and 7/3Rm, respectively.
Qij=DαijDαi1+Dαi2⋯+DαijQl | (6) |
By subtracting the pressure drop of the blood vessel from that of the coronary artery root, the pressure of the coronary microcirculation could be obtained in LAD. Rm was calculated by Eq (7).
Rm=p−Δp1−Δp2⋯−Δpi0.024Qco∗(Dα11+Dα12⋯+Dα1jDα1j⋅Dα21+Dα22⋯+Dα2jDα2j⋯Dαi1+Dαi2⋯+DαijDαij) | (7) |
A widely recognized open-loop 0-3D geometric multi-scale model was used for FFRCT simulation calculation, as shown in Figure 5.
In this study, all models were meshed using the tetrahedral meshing method in the ANSYS workbench 14.5 software. The simulation parameters were set in the ANSYS CFX 14.5 software. The material properties of blood were set as adiabatic, isotropic, incompressible Newtonian fluid with a density of 1050 kg/m3 and a viscosity of 0.0035 Pa·s. The blood vessel wall was defined as a non-slip rigid wall.
In the geometric multi-scale model, the zero-dimensional (0D) part (lumped parameter model) was mainly composed of three modules: the heart module (inlet boundary conditions), systemic circulation module (systemic circulation exit boundary conditions) and coronary artery module (coronary exit boundary conditions). In each of these modules, the resistance (R) was used to simulate the flow resistance, the capacitance (C) was used to simulate the vascular compliance, and the inductance (L) was used to simulate the blood inertia. In the heart module, the heart valve was simulated by the diode, and the left ventricle was simulated by the variable capacitance C(t). At the same time, a left ventricular module (LV) was added at the distal end of the coronary module to simulate the effect of myocardial contraction on the coronary blood flow.
The pressure-volume relationship was used in the ventricular module to describe the change in the variable capacitance in a cardiac cycle [27,31], as shown in Eq (8).
E(t)=P(t)V(t)−V0 | (8) |
where E(t) denoted the time-varying elasticity (mmHg/ml), which was the reciprocal of the variable capacitance C, V(t) and P(t) represented the time-varying ventricular volume (ml) and pressure (mmHg), respectively, and V0 (ml) denoted the reference solvent. Mathematically, Eq (9) was used to describe E(t):
E(t)=(Emax−Emin)⋅En(tn)+Emin | (9) |
En(tn) represented the normalized time-varying elasticity, expressed in Eq (10):
En(tn)=1.55[(tn0.7)1.91+(tn0.7)1.9][11+(tn1.17)21.9] | (10) |
where tn=tTmax, Tmax=0.1+0.15tc, and tc denoted the cardiac cycle (s).
In the coronary artery module, the coronary exit boundary conditions were Rm, mainly consisting of the coronary arterial resistance Ra, coronary arterial microcirculation resistance Ra-m and coronary venous microcirculation resistance Ra-v. According to literature, their proportions were 0.32, 0.52 and 0.16, respectively. When the patient transitioned from a resting state to a hyperemic state, the Rm became 0.24 times that of the resting state [10,27].
The upstream and downstream blood vessels of the 3D model were represented by a lumped parameter model and solved by the displayed Euler method. The two models were connected by interface conditions (pressure and flow were continuous). To realize the 0D/3D coupling, the software development was performed. The secondary development system was a user-defined subroutine based on the FORTRAN language.
In order to verify the influence of the power law scaling of the blood flow diameter on the simulation results, we repeated the FFRCT simulation with 3Rm, 2.7Rm and 7/3Rm as the exit boundary conditions without changing any other settings, the simulation program or FFRCT measurement position. The results were recorded as 3FFRCT, 2.7FFRCT and 7/3FFRCT, respectively.
Statistical analysis was performed using the SPSS Version 23.0 (IBM, New York, USA) and MedCalc Version 15 (MedCalc Software, Ostend, Belgium) software. In the demographic analysis, continuous data were represented by the mean ± standard deviation and the correlation between continuous variables and FFR was expressed by ANOVA. Qualitative data were represented by the statistical frequency and percentage, and the chi-square test was used to express the correlation between qualitative variables and FFR. In the correlation analysis, a p-value of less than 0.05 indicated statistical significance.
The simulated FFRCT and the clinically measured FFR were continuous values, and the Bland-Altman diagram was chosen to analyze the consistency of the two methods. If the difference between the two measurement results was clinically acceptable, within the 95% limits of agreement (95% LoA), then a good agreement could be considered between the measurement results of the two methods. The smaller the 95% LoA, the better the consistency. The area under the curve (AUC) value of the receiver operating characteristic (ROC) was used as the criterion to evaluate the diagnostic performance. The closer the AUC to 1, the better the diagnostic performance.
As shown in Table 1, there was a clear correlation between the Rm calculated under the three powers and the clinically measured FFR value. The average values of 3Rm, 2.7Rm and 7/3Rm were 146.02 ± 80.73, 149.13 ± 80.94 and 154.17 ± 83.59 mmHg/ml/s, and their p-values were 0.004, 0.005 and 0.010, respectively. Subsequently, the p-values of the correlation between gender, age, HR, SP, DP, EDV, ESV and invasive FFR were all greater than 0.05, indicating that the correlation was statistically insignificant.
Variables | Value | P value |
Gender (male/female, percentage) | 20/6, 76.9% | 0.455 |
Age (−x±S, year) | 60.73 ± 8.79 | 0.573 |
Heart rate (−x±S, beat/min) | 66.86 ± 13.43 | 0.285 |
Systolic blood pressure (−x±S, mmHg) | 127.57 ± 25.64 | 0.275 |
Diastolic blood pressure (−x±S, mmHg) | 73.67 ± 16.24 | 0.293 |
Left ventricular end-systolic volume (ESV) (−x±S, ml/min) | 81.79 ± 50.96 | 0.449 |
Left ventricular end-diastolic volume (EDV) (−x±S, ml/min) | 60.45 ± 43.72 | 0.895 |
Calculated Rm under Q∝D3 (3Rm) (−x±S, mmHg/ml/s) | 146.02 ± 80.73 | 0.004 |
Calculated Rm under Q∝D2.7 (2.7Rm) (−x±S, mmHg/ml/s) | 149.13 ± 80.94 | 0.005 |
Calculated Rm under Q∝D7/3 (7/3Rm) (−x±S, mmHg/ml/s) | 154.17 ± 83.60 | 0.010 |
The average values of 3FFRCT, 2.7FFRCT and 7/3FFRCT were 0.827 ± 0.172, 0.825 ± 0.169 and 0.824 ± 0.167, respectively, which were slightly higher than the clinically measured FFR (0.814 ± 0.151). Figure 6 shows the scatter plot of FFRCT and FFR under the three different power-laws. The Pearson correlation coefficients of 3FFRCT, 2.7FFRCT and 7/3FFRCT with FFR were 0.95, 0.95 and 0.96, respectively, p-value < 0.001, indicating a good correlation between the results of the simulation calculation and those of the clinical measurement. In particular, the simulated 7/3FFRCT was slightly better than the other two.
Figure 7 shows the Bland-Altman diagram. The average difference between 3FFRCT, 2.7FFRCT, 7/3FFRCT and FFR was 0.01, and the 95% LoA were -0.11~0.12, -0.09~0.11 and -0.08~0.11 (n = 26), respectively. Obviously, FFRCT was slightly overestimated compared with the clinically measured FFR, but the results of 7/3FFRCT-FFRCT were most concentrated in the simulation results of the three different blood flow-diameter power laws.
The diagnostic performance of FFRCT was evaluated using the ROC curve analysis. Figure 8 shows the ROC curve of FFRCT using a cut-off clinically measured FFR of ≤ 0.80. In the case of 3FFRCT, 2.7FFRCT and 7/3FFRCT, the AUC values were 0.944 [95% (CI): 0.777-0.996], 0.962 [95% (CI): 0.805-0.999], and 0.962 [95% (CI): 0.805-0.999], respectively.
Correspondingly, the standard errors were 0.0536, 0.0400 and 0.0400, respectively, as shown in Table 2. The metrics of sensitivity, specificity, positive predictive value (NPV), negative predictive value (NPV) and accuracy were also important indicators for evaluating the diagnostic performance, as shown in Table 2.
Diagnostic metrics | 3FFRCT | 2.7FFRCT | 7/3FFRCT |
AUC (95% CI) | 0.944 (0.777-0.996) | 0.962 (0.805-0.999) | 0.962 (0.805-0.999) |
SE | 0.0536 | 0.0400 | 0.0400 |
Sensitivity | 94.7% | 100% | 100% |
Specificity | 85.7% | 85.7% | 85.7% |
PPV | 85.7% | 85.7% | 85.7% |
NPV | 94.7% | 100% | 100% |
Accuracy | 92.3% | 96.15% | 96.15% |
Threshold | 0.816 | 0.787 | 0.791 |
2.7FFRCT and 7/3FFRCT had the same sensitivity, specificity, PPV, NPV and accuracy in judging myocardial ischemia, which were higher than the metrics of 3FFRCT. During the whole calculation process, the corresponding thresholds for judging the myocardial ischemia were 0.816, 0.787 and 0.791, respectively. The diagnostic threshold of 7/3FFRCT was the closest to 0.8. Based on the above-presented data, it was found that FFRCT had the best diagnostic performance when the blood flow-diameter power-law scaling was 7/3.
The blood flow diameter power-law scaling combined the structure and function and represented the supply-demand relationship between blood and the myocardium. The variable power-law size affected the distribution of blood flow in the blood vessel. In addition, it further affected the setting of the CFD exit boundary condition Rm, which had special significance for the accuracy of the simulation results.
A significant correlation between Rm and the clinically measured FFR can be observed in Table 1, which was closely related to the calculation method and process of Rm. The calculation method was explained by the theoretical simplified model, as shown in Figure 9.
Blood flow from the left ventricle into the blood vessel, and finally circulates to the right atrium through the capillaries. Since the pressure (Pv) of the right atrium is smaller than the pressure (Pa) of the left ventricle, this work assumed Pv to be 0 mmHg. Vascular resistance (Rv) is mainly caused by the blood viscosity, and the blood pressure drop (Δp) can be obtained. Then, the calculation method of Rm can be obtained by Ohm's law, as shown in Eq (11).
Rm=Pa−ΔpQ | (11) |
Rm represented the resistance produced by all blood vessels at the back of the stenosis. In Equation 11, Δp denoted the pressure drop of a blood vessel, which is related to the length and cross-sectional area of the corresponding blood vessel. Similarly, Q represented the mean flow, which is affected by the diameter of the blood vessel. Throughout the entire calculation process, Rm did not only involve the patient's personalized physiological parameters, but also involved the patient's coronary artery structure. Therefore, the calculated Rm represented the predictive value of the comprehensive calculation of the patient's structure and function evaluation.
The prediction threshold of 3FFRCT (0.816) was greater than 0.8, but the prediction threshold of 2.7FFRCT (0.787) and 7/3FFRCT (0.791) was less than 0.8. This was related to the data distribution. ROC curve analysis could get the Yoden index, as shown in the Eq (12).
YoudenIndex=Sensitivity+Specificity−1 | (12) |
The value corresponding to the largest Youden index was the prediction threshold. In other words, the threshold was the value with the best sensitivity and specificity. The predicted threshold value distinguishes the result of ischemia and ischemia to the greatest extent consistent with the result of FFR judgment. According to the distribution of the simulation results, the ROC curve analysis automatically calculated the points with the highest sensitivity and specificity to obtain the predicted threshold. The distribution of FFRCT data was not the same, and the prediction threshold was also different. However, the closer the threshold was to 0.8, the closer the distribution of FFRCT was to that of FFR.
Figure 7 shows that the average difference between 2.7FFRCT, 7/3FFRCT and clinical FFR was 0.01, which showed that the simulation results overestimated FFR. Correspondingly, Table 2 shows that the prediction threshold of 2.7FFRCT and 7/3FFRCT was lower than 0.8. This phenomenon was related to the data processing method. FFR was a continuous variable when performing Bland-Altman diagram analysis. In contrast, the thresholds of the three methods were predicted by ROC curve analysis, with FFRCT as the test variable and FFR (0.8) as the state variable. FFR was a categorical variable. FFR ≥ 0.8 was defined as non-ischemic, and FFR < 0.8 was defined as ischemic. So, for non-ischemic data, no matter whether FFRCT was 0.8 or 1, there was almost no difference for the ROC curve.
The allometric scaling law represents the basis to understand the morphological and hemodynamic characteristics of biological vascular trees and is of great significance to the construction of vascular models. In this work, we found that having blood flow proportional to the power 7/3 of the diameter is more suitable for the simulation calculation of FFRCT, and it has a higher accuracy than the FFRCT calculated by the powers 3 and 2.7. This phenomenon may be related to the geometry of the coronary artery.
The influence of the coronary artery structure on the blood flow diameter scaling law was mainly reflected in the branch diameters of blood vessels of the same bifurcated vessel. Assuming that the stenosis was on vessel j of branch i, and there were two vessels at the bifurcation, the blood flow of the vessel could be calculated as in Eq (6). Dij/Di(j+1) could represent the relative size of the blood vessel in which the stenosis was located, represented by the letter A. Then the formula can be transformed into Eq (13).
Qij=(1−1Aα+1)Ql | (13) |
This is a piecewise function. When A was greater than 1, as the power increased, the flow gradually increased, and A when was less than 1, as the power increased, the flow gradually decreased. Correspondingly, only when A was 1, no matter how the power changed, it would not affect the flow. This phenomenon leads to a greater dispersion of FFRCT obtained by simulation when the power is 3. In the past 80 years, many papers on Murray's law and exponential verification have been published. These studies show that the 3.0 application has significant dispersibility in all coronary trees with an internal flow that obeys laminar flow conditions [14-20]. This may be the reason why 7/3FFRCT is better than the other two methods.
The simulation results of the three power scaling laws did not show much change, but this phenomenon was acceptable. The scatter plots of the difference between 3FFRCT and 2.7FFRCT, 3FFRCT and 7/3FFRCT, and 2.7FFRCT and 7/3FFRCT were shown in Figure 10A. It could be found that the maximum difference of the simulation results was 0.027, and its corresponding 3FFRCT and 7/3FFRCT values were 0.947 and 0.920, respectively, as shown in Figure 10B and 10C. Correspondingly, the difference between 3Rm and 7/3Rm was 84 mmHg/ml/s. Previous works that the Rm in the hyperemic state to be 0.24 times Rm in the resting state [29], which narrows the gap in the exit boundary conditions simulated by FFRCT. At the same time, FFR is a dimensionless value between 0-1 [8,29,31], which further narrows the gap between the simulation results of different power scaling laws.
The simulation of FFRCT makes non-invasive FFR calculation possible, which has passed the FDA certification [1,6]. However, it has disadvantages, including the long time of the entire process, large amount of calculation and complicated operation. This makes non-professionals unable to operate it, so the simulation results cannot be presented in real time and the clinical development of this technology is limited.
The rapid development of machine learning (ML) has made the application of FFRCT possible. The process of using ML generally consists of four parts: 1) the ML framework is built; 2) the training set is used for supervised learning; 3) the verification set is used to determine the parameters of the network; 4) the test set is used to verify the optimal performance of the model. Previous research focused on the ML framework, such as random forest (RF), self-organizing map (SOM), support vector machine (SVM), fully connected neural network (FC), long and short-term memory unit (LSTM), TreeVes -D, TreeVes-U and TreeVes-Net [32,33,34,35,36].
However, the training set includes a set of samples of known categories to adjust the parameters of the classifier to achieve the required performance. Hence, the accuracy of the training set is very important. The method of this research improves the accuracy of FFRCT, which can be applied to improve the accuracy of the training set and validation set. This lays the foundation for the use of machine learning in the flow field in the coronary arteries.
smartFFR was a new method of true onsite and real-time, geometrically derived coronary artery stenosis function assessment. Its inlet boundary condition was an average static pressure of 100 mmHg, and its outlet boundary condition was an increased transient flow profile. In order to do this, after evaluating the diameter and cross-sectional area of the branch, Murray's law was applied, which stated that the flow of the branch was proportional to 3 exponent of the corresponding diameter of the branch. This study pointed out that the accuracy of FFRCT simulation with an application of the 7/3 exponent was higher than that of the 3 exponent. In future work, it could be applied to smartFFR to check whether 7/3 could improve the accuracy of smartFFR [37].
This work had some limitations. Firstly, the number of enrolled groups was not large enough, the selection of data was biased, and there were fewer ischemic vessels than non-ischemic vessels. Secondly, the research performed based on the assumption that the ratio of flow distribution between the left and right coronary arteries was 6:4, which was not personalized. Finally, a constant blood viscosity value of 0.0035 Pa.s was applied in the simulation, which was not a personalized value.
The blood flow-diameter power-law scaling affects the calculation of blood flow distribution and microcirculation resistance; hence, it affects the simulation results of FFRCT. This paper simulated and calculated FFRCT in the case of 3, 2.7 and 7/3 powers. Through retrospective analysis between these results and invasive FFR, the accuracy of FFRCT was shown to be the highest when the blood flow diameter scale law was 7/3. This has positive significance for the improvement of the FFRCT model and the accuracy of the model.
This study was supported by National Natural Science Foundation of China (11832003, 11772016, 11702008), National Key R & D Program of China (2020YFC2004400), and Key Project of Science and Technology of Beijing Municipal Education Commission (KZ201810005007).
This study passed the inspection by the medical ethics committee of Peking University People's Hospital. All participants have signed an informed consent.
The authors declare that there is no conflict of interests of this article.
[1] | R. Nigam, R. Tripathy, S. Dutta, N. Bhagia, R. Nagori, K. Chandrasekar, et al., Crop type discrimination and health assessment using hyperspectral imaging, Curr. Sci., 116 (2019), 1108–1123. https://www.jstor.org/stable/27138003 |
[2] |
J. ten Harkel, H. Bartholomeus, L. Kooistra, Biomass and crop height estimation of different crops using UAV-based LiDAR, Remote Sens., 12 (2020), 17. https://doi.org/10.3390/rs12010017 doi: 10.3390/rs12010017
![]() |
[3] |
U. S. Panday, N. Shrestha, S. Maharjan, A. K. Pratihast, Shahnawaz, K. L. Shrestha, et al., Correlating the plant height of wheat with above-ground biomass and crop yield using drone imagery and crop surface model, a case study from nepal, Drones, 4 (2020), 28. https://doi.org/10.3390/drones4030028 doi: 10.3390/drones4030028
![]() |
[4] |
A. Michez, P. Lejeune, S. Bauwens, A. A. L. Herinaina, Y. Blaise, E. C. Muñoz, et al., Mapping and monitoring of biomass and grazing in pasture with an unmanned aerial system, Remote Sens., 11 (2019), 473. https://doi.org/10.3390/rs11050473 doi: 10.3390/rs11050473
![]() |
[5] |
A. I. de Castro, R. Ehsani, R. C. Ploetz, J. H. Crane, S. Buchanon, Detection of laurel wilt disease in avocado using low altitude aerial imaging, PloS ONE, 10 (2015), 1–13. https://doi.org/10.1371/journal.pone.0124642 doi: 10.1371/journal.pone.0124642
![]() |
[6] |
A. Mahlein, Plant disease detection by imaging sensors–parallels and specific demands for precision agriculture and plant phenotyping, Plant Dis., 100 (2016), 241–251. https://doi.org/10.1094/PDIS-03-15-0340-FE doi: 10.1094/PDIS-03-15-0340-FE
![]() |
[7] | P. Moghadam, D. Ward, E. Goan, S. Jayawardena, P. Sikka, E. Hernandez, Plant disease detection using hyperspectral imaging, in 2017 International Conference on Digital Image Computing: Techniques and Applications (DICTA), (2017), 1–8. https://doi.org/10.1109/DICTA.2017.8227476 |
[8] |
D. Gómez-Candón, J. Torres-Sanchez, S. Labbé, A. Jolivot, S. Martinez, J. L. Regnard, Water stress assessment at tree scale: high-resolution thermal UAV imagery acquisition and processing, Acta Hortic., 1150 (2017), 159–166. https://doi.org/10.17660/ActaHortic.2017.1150.23 doi: 10.17660/ActaHortic.2017.1150.23
![]() |
[9] |
C. A. Reynolds, M. Yitayew, D. C. Slack, C. F. Hutchinson, A. Huete, M. S. Petersen, Estimating crop yields and production by integrating the FAO Crop Specific Water Balance model with real-time satellite data and ground-based ancillary data, Int. J. Remote Sens., 21 (2000), 3487–3508. https://doi.org/10.1080/014311600750037516 doi: 10.1080/014311600750037516
![]() |
[10] |
S. S. Panda, D. P. Ames, S. Panigrahi, Application of vegetation indices for agricultural crop yield prediction using neural network techniques, Remote Sens., 2 (2010), 673–696. https://doi.org/10.3390/rs2030673 doi: 10.3390/rs2030673
![]() |
[11] |
Z. Fu, J. Jiang, Y. Gao, B. Krienke, M. Wang, K. Zhong, et al., Wheat growth monitoring and yield estimation based on multi-rotor unmanned aerial vehicle, Remote Sens., 12 (2020), 508. https://doi.org/10.3390/rs12030508 doi: 10.3390/rs12030508
![]() |
[12] |
S. Guan, K. Fukami, H. Matsunaka, M. Okami, R. Tanaka, H. Nakano, et al., Assessing correlation of high-resolution NDVI with fertilizer application level and yield of rice and wheat crops using small UAVs, Remote Sens., 11 (2019), 112. https://doi.org/10.3390/rs11020112 doi: 10.3390/rs11020112
![]() |
[13] |
M. Maimaitijiang, V. Sagan, P. Sidike, S. Hartling, F. Esposito, F. B. Fritschi, Soybean yield prediction from UAV using multimodal data fusion and deep learning, Remote Sens. Environ., 237 (2020), 111599. https://doi.org/10.1016/j.rse.2019.111599 doi: 10.1016/j.rse.2019.111599
![]() |
[14] |
Y. Guo, S. Chen, X. Li, M. Cunha, S. Jayavelu, D. Cammarano, et al., Machine learning-based approaches for predicting SPAD values of maize using multi-spectral images, Remote Sens., 14 (2022), 1337. https://doi.org/10.3390/rs14061337 doi: 10.3390/rs14061337
![]() |
[15] |
Z. Sun, X. Wang, Z. Wang, L. Yang, Y. Xie, Y. Huang, UAVs as remote sensing platforms in plant ecology: review of applications and challenges, J. Plant Ecol., 14 (2021), 1003–1023. https://doi.org/10.1093/jpe/rtab089 doi: 10.1093/jpe/rtab089
![]() |
[16] |
J. Xue, B. Su, Significant remote sensing vegetation indices: A review of developments and applications, J. Sens., 2017 (2017), 1–17. https://doi.org/10.1155/2017/1353691 doi: 10.1155/2017/1353691
![]() |
[17] |
L. Wan, H. Cen, J. Zhu, J. Zhang, Y. Zhu, D. Sun, et al., Grain yield prediction of rice using multi-temporal UAV-based RGB and multispectral images and model transfer-a case study of small farmlands in the South of China, Agric. For. Meteorol., 291 (2020), 108096. https://doi.org/10.1016/j.agrformet.2020.108096 doi: 10.1016/j.agrformet.2020.108096
![]() |
[18] |
J. Zhou, J. Zhou, H. Ye, M. L. Ali, P. Chen, H. T. Nguyen, Yield estimation of soybean breeding lines under drought stress using unmanned aerial vehicle-based imagery and convolutional neural network, Biosyst. Eng., 204 (2021), 90–103. https://doi.org/10.1016/j.biosystemseng.2021.01.017 doi: 10.1016/j.biosystemseng.2021.01.017
![]() |
[19] |
Y. Guo, H. Wang, Z. Wu, S. Wang, H. Sun, J. Senthilnath, et al., Modified red blue vegetation index for chlorophyll estimation and yield prediction of maize from visible images captured by UAV, Sensors, 20 (2020), 5055. https://doi.org/10.3390/s20185055 doi: 10.3390/s20185055
![]() |
[20] |
Y. Guo, Y. Fu, F. Hao, X. Zhang, W. Wu, X. Jin, et al., Integrated phenology and climate in rice yields prediction using machine learning methods, Ecol. Indic., 120 (2021), 106935. https://doi.org/10.1016/j.ecolind.2020.106935 doi: 10.1016/j.ecolind.2020.106935
![]() |
[21] | Peanut company of Australia, How peanuts are grown, 2023. Available from: https://pca.com.au/pca-profile/how-peanuts-are-grown/ |
[22] |
Z. Ji, Y. Pan, X. Zhu, D. Zhang, J. Wang, A generalized model to predict large-scale crop yields integrating satellite-based vegetation index time series and phenology metrics, Ecol. Indic., 137 (2022), 108759. https://doi.org/10.1016/j.ecolind.2022.108759 doi: 10.1016/j.ecolind.2022.108759
![]() |
[23] |
H. García-Martínez, H. Flores-Magdaleno, R. Ascencio-Hernández, A. Khalil-Gardezi, L. Tijerina-Chávez, O. R. Mancilla-Villa, et al., Corn grain yield estimation from vegetation indices, canopy cover, plant density, and a neural network using multispectral and RGB images acquired with unmanned aerial vehicles, Agriculture, 10 (2020), 277. https://doi.org/10.3390/agriculture10070277 doi: 10.3390/agriculture10070277
![]() |
[24] |
X. Zhou, H. B. Zheng, X. Q. Xu, J. Y. He, X. K. Ge, X. Yao, et al., Predicting grain yield in rice using multi-temporal vegetation indices from UAV-based multispectral and digital imagery, ISPRS J. Photogramm. Remote Sens., 130 (2017), 246–255. https://doi.org/10.1016/j.isprsjprs.2017.05.003 doi: 10.1016/j.isprsjprs.2017.05.003
![]() |
[25] |
D. C. Tsouros, S. Bibi, P. G. Sarigiannidis, A review on UAV-based applications for precision agriculture, Information, 10 (2019), 349. https://doi.org/10.3390/info10110349 doi: 10.3390/info10110349
![]() |
[26] |
J. Kim, S. Kim, C. Ju, H. Il Son, Unmanned aerial vehicles in agriculture: A review of perspective of platform, control, and applications, IEEE Access, 7 (2019), 105100–105115. https://doi.org/10.1109/ACCESS.2019.2932119 doi: 10.1109/ACCESS.2019.2932119
![]() |
[27] |
T. B. Shahi, C. Xu, A. Neupane, W. Guo, Machine learning methods for precision agriculture with UAV imagery: A review, Electron. Res. Arch., 30 (2022), 4277–4317. https://doi.org/10.3934/era.2022218 doi: 10.3934/era.2022218
![]() |
[28] |
A. P. M. Ramos, L. P. Osco, D. E. G. Furuya, W. N. Gonçalves, D. C. Santana, L. P. R. Teodoro, et al., A random forest ranking approach to predict yield in maize with uav-based vegetation spectral indices, Comput. Electron. Agric., 178 (2020), 105791. https://doi.org/10.1016/j.compag.2020.105791 doi: 10.1016/j.compag.2020.105791
![]() |
[29] |
J. Geipel, J. Link, W. Claupein, Combined spectral and spatial modeling of corn yield based on aerial images and crop surface models acquired with an unmanned aircraft system, Remote sens., 6 (2014), 10335–10355. https://doi.org/10.3390/rs61110335 doi: 10.3390/rs61110335
![]() |
[30] | A. Ashapure, S. Oh, T. G. Marconi, A. Chang, J. Jung, J. Landivar, et al., Unmanned aerial system based tomato yield estimation using machine learning, in Autonomous Air and Ground Sensing Systems for Agricultural Optimization and Phenotyping IV, (2019). https://doi.org/10.1117/12.2519129 |
[31] |
A. Matese, S. F. Di Gennaro, Beyond the traditional NDVI index as a key factor to mainstream the use of UAV in precision viticulture, Sci. Rep., 11 (2021), 1–13. https://doi.org/10.1038/s41598-021-81652-3 doi: 10.1038/s41598-021-81652-3
![]() |
[32] |
C. Bian, H. Shi, S. Wu, K. Zhang, M. Wei, Y. Zhao, et al., Prediction of field-scale wheat yield using machine learning method and multi-spectral UAV Ddata, Remote Sens., 14 (2022), 1474. https://doi.org/10.3390/rs14061474 doi: 10.3390/rs14061474
![]() |
[33] |
S. Fei, M. A. Hassan, Y. Xiao, X. Su, Z. Chen, Q. Cheng, et al., UAV-based multi-sensor data fusion and machine learning algorithm for yield prediction in wheat, Precision Agric., 24 (2022), 1–26. https://doi.org/10.1007/s11119-022-09938-8 doi: 10.1007/s11119-022-09938-8
![]() |
[34] |
A. Patrick, S. Pelham, A. Culbreath, C. C. Holbrook, I. J. De Godoy, C. Li, High throughput phenotyping of tomato spot wilt disease in peanuts using unmanned aerial systems and multispectral imaging, IEEE Instrum. Meas. Mag., 20 (2017), 4–12. https://doi.org/10.1109/MIM.2017.7951684 doi: 10.1109/MIM.2017.7951684
![]() |
[35] | QGIS development team, QGIS Geographic Information System, 2023. Available from: https://www.qgis.org |
[36] |
F. I. Matias, M. V. Caraza-Harter, J. B. Endelman, FIELDimageR: An R package to analyze orthomosaic images from agricultural field trials, Plant Phenom. J., 3 (2020), 20005. https://doi.org/10.1002/ppj2.20005 doi: 10.1002/ppj2.20005
![]() |
[37] |
A. J. Smola, B. Schö lkopf, A tutorial on support vector regression, Satistics Comput., 14 (2004), 199–222. https://doi.org/10.1023/B:STCO.0000035301.49549.88 doi: 10.1023/B:STCO.0000035301.49549.88
![]() |
[38] |
X. Zeng, S. Yuan, Y. Li, Q. Zou, Decision tree classification model for popularity forecast of Chinese colleges, J. Appl. Math., (2014), 1–7. https://doi.org/10.1155/2014/675806 doi: 10.1155/2014/675806
![]() |
[39] |
L. Breiman, Random forests, Mach. Learn., 45 (2001), 5–32. https://doi.org/10.1023/A:1010933404324 doi: 10.1023/A:1010933404324
![]() |
[40] | F. Pedregosa, G. Varoquaux, A. Gramfort, V. Michel, B. Thirion, O. Grisel, et al., Scikit-learn: Machine learning in Python, J. Mach. Learn. Res., 12 (2011), 2825–2830. |
[41] |
T. Hastie, S. Rosset, J. Zhu, H. Zou, Multi-class adaboost, Stat. Interface, 2 (2009), 349–360. https://doi.org/10.4310/SⅡ.2009.v2.n3.a8 doi: 10.4310/SⅡ.2009.v2.n3.a8
![]() |
[42] | T. Chen, C. Guestrin, Xgboost: A scalable tree boosting system, in Proceedings of the 22nd ACM SIGKDD International Conference on Knowledge Discovery and Data Mining, (2016), 785–794. https://doi.org/10.1145/2939672.2939785 |
[43] |
M. M. Li, W. Guo, B. Verma, K. Tickle, J. O'Connor, Intelligent methods for solving inverse problems of backscattering spectra with noise: a comparison between neural networks and simulated annealing, Neural Comput. Appl., 18 (2009), 423–430. https://doi.org/10.1007/s00521-008-0219-x doi: 10.1007/s00521-008-0219-x
![]() |
[44] |
C. Tsai, C. Hsia, S. Yang, S. Liu, Z. Fang, Optimizing hyperparameters of deep learning in predicting bus passengers based on simulated annealing, Appl. Soft Comput., 88 (2020), 106068. https://doi.org/10.1016/j.asoc.2020.106068 doi: 10.1016/j.asoc.2020.106068
![]() |
[45] |
Q. Yan, J. Chen, L. De Strycker, An outlier detection method based on Mahalanobis distance for source localization, Sensors, 18 (2018), 2186. https://doi.org/10.3390/s18072186 doi: 10.3390/s18072186
![]() |
[46] |
B. Mishra, T. B. Shahi, Deep learning-based framework for spatiotemporal data fusion: an instance of landsat 8 and sentinel 2 NDVI, J. Appl. Remote Sens., 15 (2021), 034520. https://doi.org/10.1117/1.JRS.15.034520 doi: 10.1117/1.JRS.15.034520
![]() |
1. | Honghui Zhang, Rile Wu, Ning Yang, Jinjie Xie, Yang Hou, Retracted: Research on individualized distribution approach of coronary resting blood flow for noninvasive calculation of fractional flow reserve, 2023, 240, 01692607, 107704, 10.1016/j.cmpb.2023.107704 | |
2. | Daniel J. Taylor, Harry Saxton, Ian Halliday, Tom Newman, D. R. Hose, Ghassan S. Kassab, Julian P. Gunn, Paul D. Morris, Systematic review and meta-analysis of Murray’s law in the coronary arterial circulation, 2024, 327, 0363-6135, H182, 10.1152/ajpheart.00142.2024 |
Variables | Value | P value |
Gender (male/female, percentage) | 20/6, 76.9% | 0.455 |
Age (−x±S, year) | 60.73 ± 8.79 | 0.573 |
Heart rate (−x±S, beat/min) | 66.86 ± 13.43 | 0.285 |
Systolic blood pressure (−x±S, mmHg) | 127.57 ± 25.64 | 0.275 |
Diastolic blood pressure (−x±S, mmHg) | 73.67 ± 16.24 | 0.293 |
Left ventricular end-systolic volume (ESV) (−x±S, ml/min) | 81.79 ± 50.96 | 0.449 |
Left ventricular end-diastolic volume (EDV) (−x±S, ml/min) | 60.45 ± 43.72 | 0.895 |
Calculated Rm under Q∝D3 (3Rm) (−x±S, mmHg/ml/s) | 146.02 ± 80.73 | 0.004 |
Calculated Rm under Q∝D2.7 (2.7Rm) (−x±S, mmHg/ml/s) | 149.13 ± 80.94 | 0.005 |
Calculated Rm under Q∝D7/3 (7/3Rm) (−x±S, mmHg/ml/s) | 154.17 ± 83.60 | 0.010 |
Diagnostic metrics | 3FFRCT | 2.7FFRCT | 7/3FFRCT |
AUC (95% CI) | 0.944 (0.777-0.996) | 0.962 (0.805-0.999) | 0.962 (0.805-0.999) |
SE | 0.0536 | 0.0400 | 0.0400 |
Sensitivity | 94.7% | 100% | 100% |
Specificity | 85.7% | 85.7% | 85.7% |
PPV | 85.7% | 85.7% | 85.7% |
NPV | 94.7% | 100% | 100% |
Accuracy | 92.3% | 96.15% | 96.15% |
Threshold | 0.816 | 0.787 | 0.791 |
Variables | Value | P value |
Gender (male/female, percentage) | 20/6, 76.9% | 0.455 |
Age (−x±S, year) | 60.73 ± 8.79 | 0.573 |
Heart rate (−x±S, beat/min) | 66.86 ± 13.43 | 0.285 |
Systolic blood pressure (−x±S, mmHg) | 127.57 ± 25.64 | 0.275 |
Diastolic blood pressure (−x±S, mmHg) | 73.67 ± 16.24 | 0.293 |
Left ventricular end-systolic volume (ESV) (−x±S, ml/min) | 81.79 ± 50.96 | 0.449 |
Left ventricular end-diastolic volume (EDV) (−x±S, ml/min) | 60.45 ± 43.72 | 0.895 |
Calculated Rm under Q∝D3 (3Rm) (−x±S, mmHg/ml/s) | 146.02 ± 80.73 | 0.004 |
Calculated Rm under Q∝D2.7 (2.7Rm) (−x±S, mmHg/ml/s) | 149.13 ± 80.94 | 0.005 |
Calculated Rm under Q∝D7/3 (7/3Rm) (−x±S, mmHg/ml/s) | 154.17 ± 83.60 | 0.010 |
Diagnostic metrics | 3FFRCT | 2.7FFRCT | 7/3FFRCT |
AUC (95% CI) | 0.944 (0.777-0.996) | 0.962 (0.805-0.999) | 0.962 (0.805-0.999) |
SE | 0.0536 | 0.0400 | 0.0400 |
Sensitivity | 94.7% | 100% | 100% |
Specificity | 85.7% | 85.7% | 85.7% |
PPV | 85.7% | 85.7% | 85.7% |
NPV | 94.7% | 100% | 100% |
Accuracy | 92.3% | 96.15% | 96.15% |
Threshold | 0.816 | 0.787 | 0.791 |