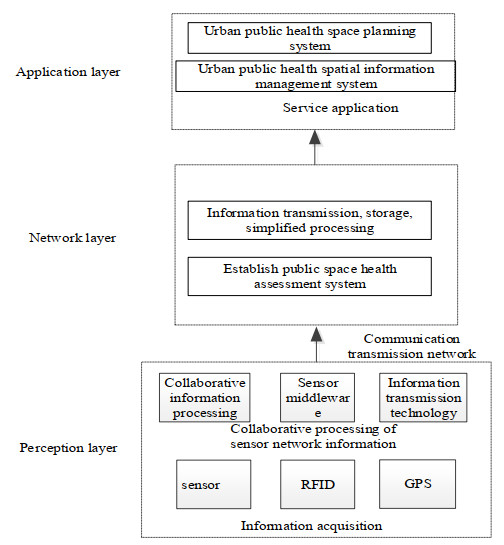
The planning of urban public health spatial can not only help people's physical and mental health but also help to optimize and protect the urban environment. It is of great significance to study the planning methods of urban public health spatial. The application effect of traditional urban public health spatial planning is poor, in this paper, urban public health spatial planning using big data technology and visual communication in the Internet of Things (IoT) is proposed. First, the urban public health spatial planning architecture is established in IoT, which is divided into the perception layer, the network layer and the application layer; Second, information collection is performed at the perception layer, and big data technology is used at the network layer to simplify spatial model information, automatically sort out spatial data, and establish a public health space evaluation system according to the type and characteristics of spatial data; Finally, the urban public health space is planned based on the health assessment results and the visual communication design concept through the application layer. The results show that when the number of regions reaches 60,000, the maximum time of region merging is 7.86s. The percentage of spatial fitting error is 0.17. The height error of spatial model is 0.31m. The average deviation error of the spatial coordinates is 0.23, which can realize the health planning of different public spaces.
Citation: Meiting Qu, Shaohui Liu, Lei Li. Urban public health spatial planning using big data technology and visual communication in IoT[J]. Mathematical Biosciences and Engineering, 2023, 20(5): 8583-8600. doi: 10.3934/mbe.2023377
[1] | Zhen Yu, Sheng-Huang Lin, Chia-Ching Cho, Changping Chen . Performance management algorithm of financial shared service center based on Internet of Things public cloud privacy protection. Mathematical Biosciences and Engineering, 2023, 20(7): 12510-12528. doi: 10.3934/mbe.2023557 |
[2] | Radi P. Romansky, Irina S. Noninska . Challenges of the digital age for privacy and personal data protection. Mathematical Biosciences and Engineering, 2020, 17(5): 5288-5303. doi: 10.3934/mbe.2020286 |
[3] | Kenneth Li-minn Ang, Kah Phooi Seng . Biometrics-based Internet of Things and Big data design framework. Mathematical Biosciences and Engineering, 2021, 18(4): 4461-4476. doi: 10.3934/mbe.2021226 |
[4] | Xiaodong Yang, Haoqi Wen, Lei Liu, Ningning Ren, Caifen Wang . Blockchain-enhanced certificateless signature scheme in the standard model. Mathematical Biosciences and Engineering, 2023, 20(7): 12718-12730. doi: 10.3934/mbe.2023567 |
[5] | Faten S. Alamri, Khalid Haseeb, Tanzila Saba, Jaime Lloret, Jose M. Jimenez . Multimedia IoT-surveillance optimization model using mobile-edge authentic computing. Mathematical Biosciences and Engineering, 2023, 20(11): 19174-19190. doi: 10.3934/mbe.2023847 |
[6] | Jiayu Fu, Haiyan Wang, Risu Na, A JISAIHAN, Zhixiong Wang, Yuko OHNO . Recent advancements in digital health management using multi-modal signal monitoring. Mathematical Biosciences and Engineering, 2023, 20(3): 5194-5222. doi: 10.3934/mbe.2023241 |
[7] | Qinwang Niu, Haoyue Li, Yu Liu, Zhibo Qin, Li-bo Zhang, Junxin Chen, Zhihan Lyu . Toward the Internet of Medical Things: Architecture, trends and challenges. Mathematical Biosciences and Engineering, 2024, 21(1): 650-678. doi: 10.3934/mbe.2024028 |
[8] | Kittur Philemon Kibiwott , Yanan Zhao , Julius Kogo, Fengli Zhang . Verifiable fully outsourced attribute-based signcryption system for IoT eHealth big data in cloud computing. Mathematical Biosciences and Engineering, 2019, 16(5): 3561-3594. doi: 10.3934/mbe.2019178 |
[9] | Naila Naz, Muazzam A Khan, Suliman A. Alsuhibany, Muhammad Diyan, Zhiyuan Tan, Muhammad Almas Khan, Jawad Ahmad . Ensemble learning-based IDS for sensors telemetry data in IoT networks. Mathematical Biosciences and Engineering, 2022, 19(10): 10550-10580. doi: 10.3934/mbe.2022493 |
[10] | Shitharth Selvarajan, Hariprasath Manoharan, Celestine Iwendi, Taher Al-Shehari, Muna Al-Razgan, Taha Alfakih . SCBC: Smart city monitoring with blockchain using Internet of Things for and neuro fuzzy procedures. Mathematical Biosciences and Engineering, 2023, 20(12): 20828-20851. doi: 10.3934/mbe.2023922 |
The planning of urban public health spatial can not only help people's physical and mental health but also help to optimize and protect the urban environment. It is of great significance to study the planning methods of urban public health spatial. The application effect of traditional urban public health spatial planning is poor, in this paper, urban public health spatial planning using big data technology and visual communication in the Internet of Things (IoT) is proposed. First, the urban public health spatial planning architecture is established in IoT, which is divided into the perception layer, the network layer and the application layer; Second, information collection is performed at the perception layer, and big data technology is used at the network layer to simplify spatial model information, automatically sort out spatial data, and establish a public health space evaluation system according to the type and characteristics of spatial data; Finally, the urban public health space is planned based on the health assessment results and the visual communication design concept through the application layer. The results show that when the number of regions reaches 60,000, the maximum time of region merging is 7.86s. The percentage of spatial fitting error is 0.17. The height error of spatial model is 0.31m. The average deviation error of the spatial coordinates is 0.23, which can realize the health planning of different public spaces.
Urban economic development has also brought about unhealthy impacts. The "urban disease" brought about by urban expansion and information construction is gradually affecting people's physical and mental health [1,2]. In particular, the reduction of public space has greatly restricted the free activities of residents and made the city lack the initial vitality and attraction [3,4,5]. Facing this situation, how to create a healthy living environment is the focus of urban designers [6], and urban public health spatial planning has become one of the important means to address this problem. For the design and change of a healthy city, the planning and design of public space are very important. Reasonable space layout and green ecological environment are the core of the establishment of public health space [7,8]. IoT is an emerging development technology, which is unique and advanced in information collection, transmission, processing and service methods. Therefore, the study of urban public health spatial planning in IoT is a real demand for urban public spatial planning and development, which will greatly improve the effectiveness of the planning system [9,10].
To effectively address the problems of the above methods, this paper proposes an urban public health spatial planning method based on big data technology and visual communication in IoT. According to the type and features of spatial data, the proposed method establishes a public health spatial evaluation system to provide support for urban spatial planning. The contributions of this paper are as follows: 1) An urban public health spatial planning method based on big data technology and visual communication in IoT is proposed. The advantages of IoT technology are used to ensure the efficiency of spatial information collection, transmission, processing and application. 2) Combine big data technology with visual communication to ensure the quality and efficiency of urban public health spatial planning while giving attention to health and beauty. 3) The results using different data sets show that the spatial information processing efficiency of the proposed urban public health spatial planning method is higher and the data is more accurate, which can realize the planning of different public health spaces.
This section provides the structure of this paper. Section 1 is the introduction of the proposed work. Section 2 describes the related works of this paper. Section 3 gives the methodology of this paper. Section 4 described the results and discussion of the proposed work. Finally, the conclusion and future work of this paper are explained in this section 5.
At present, there are no unified norms and standards for the research of urban public health space, and there is still unreasonable spatial planning in many areas [11,12,13]. In response to this problem, foreign research experts have joined forces with many government departments in planning public health space, such as the Ministry of health and mental health, the Ministry of transport, the Ministry of design and construction, and extensively consulted experts in various fields [14,15]. In domestic research, the overall development direction of the research on public health space is similar. However, there are some differences. Based on the current research situation, the current urban public spatial planning only pursues one-sided formal beauty. Pursuing urban development and accepting urban multiculturalism, it does not meet people's health needs [16,17]. Therefore, to address the problem of urban public health spatial planning, different planning methods have been gradually developed. For example, Zheng et al. [18] proposed an urban public health spatial planning method based on taxi trajectory data. Using taxi track data, this paper studies the spatial layout and management resource allocation of urban public health from the perspective of spatial interaction. Through rasterizing and visualizing the percentage of boarding and alighting points in the buffer zone of urban public health space, the urban public health space is re-planned in combination with the percentage. However, this method is difficult to obtain and has the problem of a long time for regional consolidation. Liu et al. [19] proposed a map-based urban public health spatial planning method. Collect satellite images and Google Earth images, use object-based image classification and visual interpretation to quantify the urban public health space, use multiple indicators to evaluate the existing urban public health space, and re-plan the urban public health space according to the evaluation results. However, it is found in the practical application that the spatial fitting error percentage is relatively high after the application of this method, and the practical application effect is not good. Wang et al. [20] analyzed the management of barrier-free facilities in urban public space based on IoT, used CMOS sensors and related equipment to collect public space image information, transmits and processes public space information through sensor node communication, to judge the standardization of facilities and transform them to complete barrier-free facility management. However, this method has the problem of high space height error, and the actual application effect is not good. Yuan et al. [21] put forward a method of urban public sports spatial planning based on influencing factors. The urban public transport, urban management system, urban public functions, reasonable service radius of public sports facilities and other factors are analyzed and construct urban public sports spatial planning in combination with the excessive service radius and accessibility. The output of the model is the result of urban public sports spatial planning. However, the average deviation error of spatial coordinates of this method is high, and it is difficult to achieve the ideal application effect. Zeng et al. [22] proposed a method of urban park spatial planning based on ArcGIS. The spatial layout of urban parks in the study area is analyzed by using the road traffic network accessibility and facility service area analysis tools of ArcGIS. Combined with the existing layout of urban parks and the needs of residents, new urban parks are added to realize the planning of urban parks. However, the health index of this method is not high and the practical application effect is poor.
In conclusion, various methods of urban public health spatial planning have been discussed in the related works. However, there is no unified norm and standard for the study of urban public health spatial planning for these methods. In this study, we propose an urban public health spatial planning method based on big data technology and visual communication in IoT, and establish a public health spatial planning assessment system based on spatial data types and features.
IoT is a network that connects any item to the Internet for information exchange and communication through the use of information collection devices such as radio frequency identification, sensors, infrared sensors, global positioning systems, laser scanners, and so on, according to an agreed protocol, to achieve intelligent identification, positioning, tracking, monitoring and management. The analysis of urban public health space networks using IoT technology is very different from the traditional urban public space analysis methods, which can be reflected in information perception, network layer and application layer.
The traditional urban public health space information collection adopts manual collection, passive collection and other methods. The information collection in IoT technology has a very strong initiative and mobility. The object information collection is completed through tags, sensors and other means, which is more intelligent. On the network layer, the traditional urban public health space information transmission is mostly wired and unidirectional, while the information transmission in IoT technology is mainly mobile and wireless, which is more digital and intelligent, and can effectively overcome the limitations of time and space. On the application level, the application level of traditional urban public health space information is limited, and the information application in IoT technology is more high-speed and intelligent. Therefore, this paper establishes the urban public health spatial planning architecture in IoT, and the architecture is established according to the three-tier architecture model of IoT.
In Figure1. The sensing layer collects information through sensors, Radio Frequency Identification (RFID), Global Positioning System (GPS) and so on, including the distribution of urban public space buildings, the placement of equipment and facilities and so on. The sensor network is used to process the information cooperatively. The network layer is responsible for using the computer cluster to analyze the collected information, complete information transmission, storage and other processing, this paper simplifies the urban public space information in the network layer and establishes a public space health assessment system. The application layer forms the urban public health space information management information system and planning system based on the network layer information processing results and completes the public health spatial planning.
To efficiently complete urban public health spatial planning in IoT, big data is used at the network layer to simplify urban public space information, and then a public space health assessment system is established. On the application level, urban public health spatial planning is completed based on visual communication technology.
Using conventional technical means to obtain the three-dimensional (3D) model of urban public space. To save processing time and calculation costs, using big data technology to simplify spatial information [23]. Since the spatial 3D model is composed of multiple pieces, the main means to simplify the spatial information is to reduce the number of pieces on the surface of the model as much as possible. Therefore, the model is folded and the minimum folding cost is calculated by using big data technology. The calculation formula is as follows:
Δr=∑p∈P(r)d2p(r) | (1) |
where r represents the vertex of the spatial model. P(r) is all associated sets of planes around r.p is the plane Equation. The calculation Equation is as follows:
p=(a,b,c,d)T | (2) |
Then the following Equation holds
ax+by+cz+d=0 | (3) |
a2+b2+c2=1 | (4) |
dp(r)=(pTr) | (5) |
where dp(r) represents all plane sets. pTr represents the folded plane set, and its calculation Equation is as follows:
(pTr)2=rTGpr | (6) |
where Gp represents the quadratic error, as shown in Eq (7).
Gp=[a2abacadabbbcbdacbcc2cdadbdcdd2] | (7) |
Assuming that r1 and r2 represent two random vertices of the folding process, and K represents the sum of the errors of the two vertices, its calculation Equation is as follows:
K(r)=Δr(r1+r2) | (8) |
where Δr represents the error, which is the sum of squares of distances d from any vertex to all associated triangles. As shown in Eq (9).
K(r) = ∑p∈P(r)Gp | (9) |
ΔH=rT0Kr0 | (10) |
where ΔH represents the folding point with the lowest cost.
After determining the best point of the fold, the normal vector angle between two triangular surfaces of the spatial model is calculated and an arithmetically weighted average is performed [24,25]. Calculate the vertex sharpness with the following Equation
α(r)=n∑i=0(Si+Si+1)2Sr×ω(Ni,Ni+1)+(Sn+S0)2Sr×ω(Nn,N0)n | (11) |
where α(r) indicates the sharpness of the vertex. Si and Si+1 represent the areas of the i and i+1 faces in the n patches adjacent to the vertex r, respectively. Sr represents area. Ni and Ni+1 represent the normal vectors of the i and i+1 planes, respectively.ω represents the included angle between normal vectors. The sharpness factor is introduced into matrix K to obtain the optimized cost function:
ΔH′=rT0Bα(r)r0 | (12) |
where B represents a real number, which plays a role in adjusting the impact of cost. After the simplification of all spatial information is completed, the public health space is planned by using visual communication technology based on the results of the public space health evaluation.
This paper uses big data technology to simplify spatial model information and automatically sort out spatial data. According to the types and features of spatial data, a public health spatial evaluation system is established. The urban public health space is mainly extended and expanded based on the healthy city and health theory. Its design is from the perspective of people and aims to meet people's health needs. Therefore, urban public health space needs to have sufficient sunshine, convenient transportation and multi-functional space types. Internally, it has the spiritual pursuit of diversification and high grade.
According to the above, a public health space evaluation index system is established to measure the health level of urban public space. The evaluation indicators of public health space are divided into two categories, one is objective evaluation and the other is subjective evaluation. Both of them aim to promote the physical and mental health of urban residents, and establish an urban public health space evaluation system, as shown in Table1.
Category | Primary evaluation index | Secondary evaluation index |
Objective evaluation | Cultural connotation |
style |
environment | ||
Historical and cultural heritage | ||
Visual experience |
Spatial continuity | |
Building coordination | ||
Proportional rationality | ||
Visual patency | ||
Color harmony | ||
Security | Traffic accident rate | |
crime rate | ||
Emergency shelter | ||
Comfortable | Building density | |
Facility density | ||
Height of surrounding buildings | ||
Scale and quantity of public open space | ||
Various | Cultural Services | |
Business services | ||
Communication services | ||
Environmental health services | ||
Vision | Entry pointing sign | |
Public space internal pointing sign | ||
Contour line, skyline | ||
Equipment and facilities | Functional lighting | |
Landscape lighting | ||
Systematization of facility setting | ||
Facility materials and colors | ||
Barrier-free design | ||
Subjective evaluation | Environmental quality | Air cleanliness |
Water cleanliness | ||
noise pollution | ||
Naturalness |
Greening rate | |
Green space rate | ||
Plant species | ||
Maintenance management | Greening maintenance level | |
Environmental sanitation maintenance | ||
Facility operation maintenance |
According to Table1.The urban public health space evaluation system consists of subjective and objective indicators, of which the objective evaluation consists of multiple first-class indicators such as cultural connotation, visual experience, safety, comfort, diversity, vision, equipment and facilities, and consists of style, environment, historical and cultural heritage, spatial continuity, building coordination, proportional rationality, visual smoothness, color coordination, traffic accident rate, crime rate, emergency shelters, building density facility density, the height of surrounding buildings, scale and quantity of public open space, cultural services, commercial services, communication services, environmental health services, entrance directional signs, internal directional signs of public space, contour lines, skylines, functional lighting, landscape lighting, systematization of facility facilities, facility materials, colors, barrier-free design and other secondary evaluation indicators.
The subjective evaluation indicators are composed of multiple first-class indicators such as environmental quality, naturalness, maintenance and management, and multiple second-class evaluation indicators such as air cleanliness, water cleanliness, noise pollution, greening rate, greening rate, plant species, greening maintenance level, environmental sanitation maintenance, facility operation maintenance. According to the established urban public health space evaluation system, check the urban public spatial planning to make the planning results meet the health requirements.
From the evaluation system of urban public health space, it can be seen that the planning of public space is related to the degree of naturalness. To achieve a better visual experience, it is necessary to consider the exposure of sunlight in spatial planning. Therefore, before starting public spatial planning, calculate some contents related to the sun. The solar angle is calculated as follows:
β=2π×(N−N0)/2π×(N−N0)365.2422365.2422 | (13) |
where N represents the total number of days between the calculation day and New Year's Day. N0 is the serial number with the date within the year. Assuming that H represents the solar altitude angle, the Equation is as follows:
sinH=(sinγ×sinκ+cosγ×cosκ×cosθ) | (14) |
where γ is the solar declination angle. κ represents geographical dimension. θ is the solar hour angle. Then the following Equation holds
cosθ=−tanκ⋅tanγ | (15) |
Assuming that ψ represents the solar irradiation angle, the following Equation holds
cosψ=(sinHsinκ−sinγ)/(sinHsinκ−sinγ)cosHcoscosHcosκ | (16) |
In terms of urban public health spatial planning, the whole space area is divided into leisure area, landscape area and activity area according to the features and functional needs of the planning location. Each area has a clear division of labor. However, they connect in space, forming a continuous, green open urban public space.
Visual communication design mainly transmits information through symbols and visual elements. Based on this feature, the text and color are used to plan the urban public space. Considering the change of natural light source, a large number of flowers and plants are arranged in the leisure area to enable residents to have close contact with plants. According to the geographical features of the public space location, plants of different fluorescence and colors are used to construct a unique landscape, creating a dizzying visual experience and atmosphere.
At the same time, according to the element features of mountain culture and water culture, based on the existing layout, some strong lines are used to plant flowers and plants, so that the spatial planning is both rigid and flexible. In the activity area, the residents' fitness and exercise needs are the main factors. Different equipment and facilities are designed according to the different needs of children, adolescents and the elderly. At the same time, to highlight the coordination of the space atmosphere, some buildings are designed according to different needs such as viewing, sun shading, rain shelter and rest. For the sake of the safety of different people, the regional space of their activities is divided, and some plants with targeted functions are planted according to the features of people in different areas. When planting plants, to optimize the structural level of public space, create artistic effects, and attach green plants to various buildings, such planning and design will also help to give play to the functions of plant noise reduction and dust collection.
In addition to the above three areas, according to the needs of residents, the entire empty public space is divided into spaces for people to rest, eat and shop. The element design of these spaces can be composed of color blocks with rich colors and high color saturation. In addition, the connection of various regional spaces is composed of elevated trestles, sidewalks and driveways, and some trunk roads can be combined with sports runways to provide more humanized and diversified leisure routes. The above is the horizontal design of public space. In the vertical design of the space, the local geographical features shall be followed, and the vertical space shall be designed to ensure the minimum amount of excavation and filling under the premise of meeting the healthy design specifications. For common height difference problems, the platform type can be designed by using the terrain, so that the vertical space forms a progressive platform space, creating a three-dimensional sense of space.
In addition, when planting plants, the height features of different plants are considered, and the plants are arranged in the space to meet the vertical features, showing a sense of three-dimensional hierarchy and integrating with the public space. For some public facilities such as toilets and garbage cans, the specific planning is based on the spatial distribution features. It is better to distribute them with a service radius of 500 meters, which can be more convenient for people to use. So far, the design of urban public health spatial planning methods based on big data technology and visual communication has been completed. The urban public health spatial planning process based on big data technology and visual communication in IoT is shown in Figure 2.
In this paper, the Cityscapes data set is an urban landscape data set. The Cityscapes data set contains a variety of stereo video sequences recorded in street scenes from 50 different cities. In addition to 20000 weak annotation frames, it also contains 5000 high-quality pixel-level annotations. The city in a regional data set is used as the study area (including the city boundary), and public urban image data, mainly including project information such as buildings, roads, squares and vegetation, are obtained through remote sensing, monitoring and other means. The data set information collection is completed by deploying several wireless sensor nodes in the public space of a built area of a city. When the sensor nodes collect relevant information, they will immediately send signals to the upper network. Two groups of experimental data are drawn from the obtained image data for experimental research. The Cityscapes data set is defined as Data set1. The city in a regional area data set is defined as a Data set2. It is shown in Table 2.
Project | Data set1 | Data set2 |
Architecture | 19.23% | 23.63% |
road | 6.29% | 8.91% |
Water body | 12.22% | 7.63% |
playground | 1.57% | 1.84% |
Square | 1.81% | 2.06% |
Unused land | 20.09% | 17.22% |
vegetation | 18.25% | 20.45% |
Building clearance | 20.54% | 18.26% |
The proportion of urban public space is calculated according to the data in Table 1. The calculation Equation is as follows:
Q=SR+SOST | (17) |
where Q represents the proportion of urban public space, SR is the road area, SO represents the area of open public space, ST refers to the total area of the built-up area.
In this experiment, when collecting information based on IoT, the wireless sensor network nodes are deployed on buildings, roads, squares, vegetation and other space objects. The layout area of each location is set to 90 square meters, and four AP nodes are distributed. The signal received by each sensor is four-dimensional information. The frequency of IoT equipment is 200GHz, and it is connected to the computer through a wireless sensor network. Select 5 groups of data from the two data sets to construct the experimental data set. Randomly select 20% of the data for testing, and the remaining 80% of the group data for training. Set the number of algorithm runs to 50. Before algorithm training, data preprocessing is performed to filter invalid data and enhance useful data. All experimental data are normalized and the data type is unified, which is convenient for subsequent data operation and calculation.
After determining the proportion of urban public space, the proposed spatial planning method is used to ensure the standard health level of public space as the goal for planning. At the same time, several existing spatial planning methods are introduced to perform spatial planning under the same experimental conditions, including Zheng et al. [18] method (method 1), Liu et al. [19] method (method 2), Wang et al. [20] method (method 3), Yuan et al. [21] method (method 4) and Zeng et al. [22] method (method 5), Taking the experimental analysis of regional consolidation efficiency and spatial fitting effect as experimental indicators, the urban public health spatial planning method is analyzed and verified through the validation of these experiments.
The calculation formula for regional consolidation efficiency is as follows:
Z=N∑i=1zi | (18) |
where zi represents the time taken for the i-th region to merge projects
Taking the initial vectorization figure of the experimental area as an example, considering the noise interference and the limitation of spatial resolution, some vector boundaries will lack the proper geometric features. In this regard, the experiment of spatial fitting effect is designed. Different spatial planning methods are used to get the fitting results of spatial vector graphics. Based on the original data, the error percentage between the original data and the fitting results is calculated. The calculation Equation is as follows:
σ = |σs|S | (19) |
where σ is the percentage of spatial fitting error. |σs| is the absolute value of area error. S represents the area of the original polygon.
It is proposed to establish a public space building, take the planned area of public space as an experimental variable, implement different planning methods, and obtain the height error of the spatial model in the planned space by computer. The calculation Equation is as follows:
h=|h1−h2| | (20) |
where h1 represents the ideal height of the spatial model. h2 represents the height value of the spatial model constructed by different methods.
In the process of planning public space, it is necessary to master the accurate position of each model in the public space, to achieve the optimal spatial planning and ensure the health of urban public space. Taking the three-dimensional coordinates of the spatial model as the experimental objective, different planning methods are used to plan, and the offset error between the real coordinate value and the estimated coordinate value is calculated. The calculation Equation is as follows
e=|e1−e2| | (21) |
where e1 represents the real coordinate value. e2 are coordinate values estimated by different methods.
According to the standard of urban public health space, the health of the planned space is evaluated from the aspects of place, function, sharing, environmental quality and naturalness. The health index is between 0 and 1. The larger the index, the higher the health level. The standard threshold is 0.6. The health index is calculated as follows:
H=100−∑L⋅k1−∑O⋅k1−∑P⋅k3 | (22) |
where L,O,P represent health alarm level, object level and performance parameters, respectively. k1,k2,k3 represent the weights corresponding to L,O,P, respectively.
In the regional merging efficiency, taking the experimental data in Table 2 as the target, a comparative experiment was conducted with several existing spatial planning methods and the proposed spatial planning methods, and the results are shown in Figure 3.
It can be seen from the results under different conditions in Figure 3 that, compared with data set1, the spatial planning method in the case of data set2 is more efficient in area consolidation. By vertically comparing the experimental results of various planning methods, for data set1, when the number of areas reaches 60,000, the area region merging time of different methods reaches the maximum. The region merging time of the proposed method is 7.86s, which is 5.77s, 8.94s, 10.24s, 14.31s and 15.29s lower than that of method 1, method 2, method 3, method 4 and method 5, respectively. For dataset 2, when the number of regions reaches 60,000, the region merging time of different methods reaches the maximum. The region merging time of the proposed method is 5.88s, which is 1.13, 1.98, 10.13, 11.47 and 16.76s lower than that of method 1, method 2, method 3, method 4 and method 5, respectively. The above data shows that the spatial planning method based on big data and visual communication proposed in this paper, facing large volume regions, takes the shortest time and has the highest efficiency in region merging. Among other methods results, the planning method based on the spatial disorder perspective has the lowest efficiency and takes a long time in region merging, which is difficult to meet the actual needs.
After calculation and statistics, the results of the spatial fitting effect are shown in Table 3.
Method | Data sets | Original polygon area(m2) | Error percentage (%) |
Proposed | Data set1 | 665.7 | 0.17 |
Data set2 | 600.4 | 0.22 | |
Method 1 | Data set1 | 500.9 | 2.41 |
Data set2 | 625.8 | 3.48 | |
Method 2 | Data set1 | 529.4 | 2.79 |
Data set2 | 569.4 | 4.94 | |
Method 3 | Data set1 | 565.6 | 4.99 |
Data set2 | 567.1 | 4.71 | |
Method 4 | Data set1 | 505.2 | 2.01 |
Data set2 | 686.7 | 3.85 | |
Method 5 | Data set1 | 537.5 | 2.99 |
Data set2 | 618.7 | 3.75 |
It can be seen from the experimental results in Table 3 that the original polygon area produced by each planning method is different. This is because the randomly selected spatial models are different. The experimental process is random. However, it does not affect the experimental analysis.
After calculating the error between the real area and the estimated area of each group, for data set 1, the spatial fitting error percentage of the proposed method is 0.17, which is 0.24, 2.09, 4.82, 1.84 and 2.82% lower than that of method 1, method 2, method 3, method 4 and method 5, respectively. For dataset 2, the spatial fitting error percentage of the proposed method is 0.22, which is 3.26, 4.72, 4.49, 3.62 and 3.52% lower than that of method 1, method 2, method 3, method 4 and method 5, respectively. In contrast, the error of the proposed public spatial planning method is very small, which can be ignored, and will not have a great impact on the actual planning. Therefore, the proposed planning method has a better spatial fitting effect.
The results of the height error of the spatial model are shown in Figure 4.
In the experimental results in Figure 4, different experimental parameters produce different experimental results. For data set 1, the height error of the spatial model of the proposed method is 0.31m, which is 1.95m, 0.54m, 1.98m, 0.32m and 1.47m lower than that of method 1, method 2, method 3, method 4 and method 5, respectively. For dataset 2, the spatial model height error of the proposed method is 0.42m, which is 0.34m, 2.16m, 1.77m, 0.94m and 0.36m lower than that of method 1, method 2, method 3, method 4 and method 5, respectively. Method 3 has the most obvious error and has a higher error under different parameter conditions. In contrast, the height error of other methods is low, but not ideal. Only the proposed spatial planning method has the smallest height error of the spatial model, which has little impact on public spatial planning.
The results of spatial coordinate offset error are shown in Table 4.
Method | Data sets | X-Y-Z average deviation error |
Proposed | Data set1 | 0.23 |
Data set 2 | 0.77 | |
Method1 | Data set 1 | 1.23 |
Data set 2 | 1.92 | |
Method2 | Data set 1 | 4.45 |
Data set 2 | 4.71 | |
Method3 | Data set 1 | 1.27 |
Data set 2 | 1.28 | |
Method4 | Data set 1 | 2.89 |
Data set 2 | 3.07 | |
Method5 | Data set 1 | 4.77 |
Data set 2 | 2.22 |
By observing the experimental results shown in Table 4, it can be seen from the results in Table4 that the error of the proposed spatial planning method is small in the experimental results of spatial coordinate offset error, while other methods have high spatial coordinate offset error, particularly under the condition of data set2, the offset is the most obvious. For dataset 1, the average migration error of the proposed method is 0.23, which is 1, 4.22, 1.04, 2.66 and 4.54 lower than that of method 1, method 2, method 3, method 4 and method 5, respectively. For dataset 2, the average migration error of the proposed method is 0.77, which is 1.15, 3.94, 0.51, 2.3 and 1.45 lower than that of method 1, method 2, method 3, method 4 and method 5, respectively. It can be seen from table 4 that in the process of spatial planning, other methods are prone to model overlap and abnormal spacing, which affect the visual effect of spatial planning. The planning method proposed in this paper can address this problem well.
The experimental results of the target spatial planning after the completion of each planning method are shown in Table 5.
Method | Data sets | Health index |
Proposed | Data set1 | 8.5 |
Data set2 | 9.3 | |
Method1 | Data set1 | 4.6 |
Data set2 | 5.5 | |
Method2 | Data set1 | 4.2 |
Data set2 | 5.4 | |
Method3 | Data set1 | 3.6 |
Data set2 | 5.6 | |
Method4 | Data set1 | 6.3 |
Data set2 | 4.7 | |
Method5 | Data set1 | 6.5 |
Data set2 | 5.8 |
According to the experimental results in Table 5, it can be seen the public space health index of each planning method. For data set 1, the health index of the proposed method was 8.5, which was 3.9, 4.3, 4.9, 2.2 and 2 higher than that of method 1, method 2, method 3, method 4 and method 5, respectively; For dataset 2, the health index of the proposed method is 9.3, which is 3.8, 3.9, 3.7, 4.6 and 3.5 higher than that of method 1, method 2, method 3, method 4 and method 5, respectively. Particularly for data set 2, only the proposed spatial planning method is above 0.6, which is far higher than the standard value. The public space planned by the proposed method is healthier and meets the standard requirements.
Based on all the above experimental results, it can be seen that the proposed urban public health spatial planning method based on big data and visual communication can ensure the efficiency and accuracy of spatial information processing under different experimental conditions, taking into account the spatial health level. The proposed method is superior to other existing planning methods and has certain application value.
Urban public space is an important place for people to perform social activities. Healthy public spatial planning plays a very significant role in promoting people's physical and mental health. Urban public spatial planning has the characteristics of a wide area, many points, and strong mobility. There is a problem with the low utilization rate. The emergence of IoT technology has brought opportunities to improve the effect of urban public spatial planning. This paper takes the urban public health spatial planning in IoT as the main research. With the support of many documents and materials, it summarizes the historical experience and proposes a spatial planning method based on big data technology and visual communication in IoT and combines theory with practice to achieve the optimal planning of urban public health space. At the same time, through the research on some existing spatial planning methods, a large number of experimental schemes are designed according to their advantages and disadvantages. After a large number of comparative analyses, the various capabilities of the proposed spatial planning methods are verified one by one. The results show that the planning method proposed in this paper is efficient and feasible in practical work and can provide a reference for urban public health spatial planning. In future work, the data will be classified by a deep learning model to continuously correct and improve the spatial planning content.
This work was supported by the effective path and practice of reasonable "load increasing" for college students majoring in Art and Design in comprehensive colleges No. SJGY20200082.
The authors declare that they have no conflicts of interest.
[1] |
N. P. Skinner, T. T. LaPlumm, J. D. Bullough, Warning light flash frequency as a method for visual communication to drivers, J. Transp. Res. Board, 2675 (2021), 88–93. https://doi.org/10.1177/0361198120983325 doi: 10.1177/0361198120983325
![]() |
[2] |
M. Liu, J. Wang, J. Zhou, Simulation study on extraction method of graphic elements of ocean plane based on visual communication, J. Coastal Res., 108 (2020), 113–117. https://doi.org/10.2112/JCR-SI108-023.1 doi: 10.2112/JCR-SI108-023.1
![]() |
[3] |
M. V. Shatskaya, A. A. Abramov, N. A. Fedorov, V. I. Kostenko, S. F. Likhachev, S. I. Seliverstov, et al., Data processing center of RadioAstron space VLBI project, Adv. Space Res., 65 (2020), 813–820. https://doi.org/10.1016/j.asr.2019.05.043 doi: 10.1016/j.asr.2019.05.043
![]() |
[4] |
J. Chang, S. N. Kadry, S. Krishnamoorthy, Review and synthesis of Big Data analytics and computing for smart sustainable cities, IET Intell. Transp. Syst., 14 (2020), 1363–1370. https://doi.org/10.1049/iet-its.2020.0006 doi: 10.1049/iet-its.2020.0006
![]() |
[5] |
J. Zhang, Z. Yu, B. Zhao, R. Sun, H. Vejre, Links between green space and public health: a bibliometric review of global research trends and future prospects from 1901 to 2019, Environ. Res. Lett., 15 (2020), 063001. https://doi.org/10.1088/1748-9326/ab7f64 doi: 10.1088/1748-9326/ab7f64
![]() |
[6] |
T. Bianchi-Aguiar, A. Hübner, M. A. Carravilla, J. F. Oliveira, Retail shelf space planning problems: A comprehensive review and classification framework, Eur. J. Oper. Res., 289 (2020), 1–16. https://doi.org/10.1016/j.ejor.2020.06.018 doi: 10.1016/j.ejor.2020.06.018
![]() |
[7] |
Q. Huang, K. Kieffer, An intelligent internet of things (IoT) sensor system for building environmental monitoring, J. Mobile multimedia, 15 (2019), 29–50. https://doi.org/10.13052/jmm1550-4646.15122 doi: 10.13052/jmm1550-4646.15122
![]() |
[8] |
D. Ma, R. Guo, Jing Ying, Y. Jing, Y. Zheng, Z. Zhao, et al., Intra-urban scaling properties examined by automatically extracted city hotspots from street data and nighttime light imagery, Remote Sens., 13 (2021), 1322–1322. https://doi.org/10.3390/rs13071322 doi: 10.3390/rs13071322
![]() |
[9] |
S. P. Gopalan, C. L. Chowdhary, C. Iwendi, M. A. Farid, L. K. Ramasamy, An efficient and privacy-preserving scheme for disease prediction in modern healthcare systems, Sensors, 22 (2022), 5574. https://doi.org/10.3390/s22155574 doi: 10.3390/s22155574
![]() |
[10] |
S. Thandapani, M. I. Mahaboob, C. Iwendi, D. Selvaraj, A. Dumka, M. Rashid, et al., IoMT with deep CNN: AI-based intelligent support system for pandemic diseases, Electronics, 12 (2023), 424. https://doi.org/10.3390/electronics12020424 doi: 10.3390/electronics12020424
![]() |
[11] |
W. Xu, C. Yuan, K. Peng, H. Du, Big data driven urban railway planning: Shenzhen metro case study, J. Comb. Optim., 42 (2019), 1–23. https://doi.org/10.1007/s10878-019-00422-0 doi: 10.1007/s10878-019-00422-0
![]() |
[12] | H. Guo, K. L. Man, Q. Ren, Q. Huang, V. Hahanov, E. Litvinova, et al., FPGA Implementation of VLC Communication Technology, in 2017 31st International Conference on Advanced Information Networking and Applications Workshops (WAINA), (2017), 586–590. https://doi.org/10.1109/WAINA.2017.54 |
[13] | S. N. Ismail, M. H. Salih, A review of visible light communication (VLC) technology, in AIP Conference Proceedings, 2213 (2020). https://doi.org/10.1063/5.0000109 |
[14] |
Q. Liu, L. Hou, S. Shaukat, U. Tariq, R. Riaz, S. S. Rizvi, Perceptions of spatial patterns of visitors in urban green spaces for the sustainability of smart city, Int. J. Distrib. Sens. Networks, 17 (2021), 15501477211. https://doi.org/10.1177/15501477211034069 doi: 10.1177/15501477211034069
![]() |
[15] |
X. Ma, H. Xue, Intelligent smart city parking facility layout optimization based on intelligent IoT analysis, Comput. Commun., 153 (2020), 145–151. https://doi.org/10.1016/j.comcom.2020.01.055 doi: 10.1016/j.comcom.2020.01.055
![]() |
[16] |
H. Shin, K. Lee, H. Kwon, A comparative experimental study of distributed storage engines for big spatial data processing using GeoSpark, J. Supercomput., 78 (2021), 2556–2579. https://doi.org/10.1007/s11227-021-03946-7 doi: 10.1007/s11227-021-03946-7
![]() |
[17] |
M. Wlodarczyk-Sielicka, W. Blaszczak-Bak, Processing of bathymetric data: The fusion of new reduction methods for spatial big data, Sensors, 20 (2020), 6207. https://doi.org/10.3390/s20216207 doi: 10.3390/s20216207
![]() |
[18] |
Q. Zheng, X. Zhao, M. Jin, Research on urban public green space planning based on taxi data: A case study on three districts of Shenzhen, China, Sustainability, 11 (2019), 1–20. https://doi.org/10.3390/su11041132 doi: 10.3390/su11041132
![]() |
[19] |
M. Liu, X. Li, D. Song, H. Zhai, Evaluation and monitoring of urban public greenspace planning using landscape metrics in Kunming, Sustainability, 13 (2021), 3704–3715. https://doi.org/10.3390/su13073704 doi: 10.3390/su13073704
![]() |
[20] |
C. Wang, L. Shi, S. Liu, C. Lu, Design of loT-based barrier-free facilities management system for urban public space, Mod. Electron. Tech., 44 (24), (2021), 49–53. https://doi.org/10.16652/j.issn.1004-373x.2021.24.011 doi: 10.16652/j.issn.1004-373x.2021.24.011
![]() |
[21] |
Y. Yuan, Z. Li, H. Liang, Selection and spatial layout of urban sports facilities guided by residents' demand, Open House Int., 44 (2019), 9–12. https://doi.org/10.1108/OHI-03-2019-B0003 doi: 10.1108/OHI-03-2019-B0003
![]() |
[22] |
J. Zeng, W. Zhang, J. Chen, X. Cheng, Evaluation of the rationality of urban park spatial layout based on disaster prevention objective, Open Access Library J., 9 (2022), 1–12. https://doi.org/10.4236/oalib.1108496 doi: 10.4236/oalib.1108496
![]() |
[23] |
Kristina Perić, Zdenko Šimić, Željko Jurić, Characterization of uncertainties in smart city planning: a case study of the smart metering deployment, Energies, 15 (2022), 2040. https://doi.org/10.3390/en15062040 doi: 10.3390/en15062040
![]() |
[24] | J. Chen, Z. Zhang, Y. Long, Strategies for improving the quality of urban street space oriented to promoting public health: perspective from spatial quality, City Plann. Rev., 44 (2020), 35–47. |
[25] |
M. Esmaeilbeigi, O. Chatrabgoun, A. Hosseinian-Far, R. Montasari, A. Daneshkhah, A low cost and highly accurate technique for big data spatial-temporal interpolation, Appl. Numer. Math., 153 (2020), 492–502. https://doi.org/10.1016/j.apnum.2020.03.009 doi: 10.1016/j.apnum.2020.03.009
![]() |
1. | Weishan Zhang, Tao Leng, Hongyan Sun, 2024, Chapter 72, 978-3-031-78275-6, 737, 10.1007/978-3-031-78276-3_72 |
Category | Primary evaluation index | Secondary evaluation index |
Objective evaluation | Cultural connotation |
style |
environment | ||
Historical and cultural heritage | ||
Visual experience |
Spatial continuity | |
Building coordination | ||
Proportional rationality | ||
Visual patency | ||
Color harmony | ||
Security | Traffic accident rate | |
crime rate | ||
Emergency shelter | ||
Comfortable | Building density | |
Facility density | ||
Height of surrounding buildings | ||
Scale and quantity of public open space | ||
Various | Cultural Services | |
Business services | ||
Communication services | ||
Environmental health services | ||
Vision | Entry pointing sign | |
Public space internal pointing sign | ||
Contour line, skyline | ||
Equipment and facilities | Functional lighting | |
Landscape lighting | ||
Systematization of facility setting | ||
Facility materials and colors | ||
Barrier-free design | ||
Subjective evaluation | Environmental quality | Air cleanliness |
Water cleanliness | ||
noise pollution | ||
Naturalness |
Greening rate | |
Green space rate | ||
Plant species | ||
Maintenance management | Greening maintenance level | |
Environmental sanitation maintenance | ||
Facility operation maintenance |
Project | Data set1 | Data set2 |
Architecture | 19.23% | 23.63% |
road | 6.29% | 8.91% |
Water body | 12.22% | 7.63% |
playground | 1.57% | 1.84% |
Square | 1.81% | 2.06% |
Unused land | 20.09% | 17.22% |
vegetation | 18.25% | 20.45% |
Building clearance | 20.54% | 18.26% |
Method | Data sets | Original polygon area(m2) | Error percentage (%) |
Proposed | Data set1 | 665.7 | 0.17 |
Data set2 | 600.4 | 0.22 | |
Method 1 | Data set1 | 500.9 | 2.41 |
Data set2 | 625.8 | 3.48 | |
Method 2 | Data set1 | 529.4 | 2.79 |
Data set2 | 569.4 | 4.94 | |
Method 3 | Data set1 | 565.6 | 4.99 |
Data set2 | 567.1 | 4.71 | |
Method 4 | Data set1 | 505.2 | 2.01 |
Data set2 | 686.7 | 3.85 | |
Method 5 | Data set1 | 537.5 | 2.99 |
Data set2 | 618.7 | 3.75 |
Method | Data sets | X-Y-Z average deviation error |
Proposed | Data set1 | 0.23 |
Data set 2 | 0.77 | |
Method1 | Data set 1 | 1.23 |
Data set 2 | 1.92 | |
Method2 | Data set 1 | 4.45 |
Data set 2 | 4.71 | |
Method3 | Data set 1 | 1.27 |
Data set 2 | 1.28 | |
Method4 | Data set 1 | 2.89 |
Data set 2 | 3.07 | |
Method5 | Data set 1 | 4.77 |
Data set 2 | 2.22 |
Method | Data sets | Health index |
Proposed | Data set1 | 8.5 |
Data set2 | 9.3 | |
Method1 | Data set1 | 4.6 |
Data set2 | 5.5 | |
Method2 | Data set1 | 4.2 |
Data set2 | 5.4 | |
Method3 | Data set1 | 3.6 |
Data set2 | 5.6 | |
Method4 | Data set1 | 6.3 |
Data set2 | 4.7 | |
Method5 | Data set1 | 6.5 |
Data set2 | 5.8 |
Category | Primary evaluation index | Secondary evaluation index |
Objective evaluation | Cultural connotation |
style |
environment | ||
Historical and cultural heritage | ||
Visual experience |
Spatial continuity | |
Building coordination | ||
Proportional rationality | ||
Visual patency | ||
Color harmony | ||
Security | Traffic accident rate | |
crime rate | ||
Emergency shelter | ||
Comfortable | Building density | |
Facility density | ||
Height of surrounding buildings | ||
Scale and quantity of public open space | ||
Various | Cultural Services | |
Business services | ||
Communication services | ||
Environmental health services | ||
Vision | Entry pointing sign | |
Public space internal pointing sign | ||
Contour line, skyline | ||
Equipment and facilities | Functional lighting | |
Landscape lighting | ||
Systematization of facility setting | ||
Facility materials and colors | ||
Barrier-free design | ||
Subjective evaluation | Environmental quality | Air cleanliness |
Water cleanliness | ||
noise pollution | ||
Naturalness |
Greening rate | |
Green space rate | ||
Plant species | ||
Maintenance management | Greening maintenance level | |
Environmental sanitation maintenance | ||
Facility operation maintenance |
Project | Data set1 | Data set2 |
Architecture | 19.23% | 23.63% |
road | 6.29% | 8.91% |
Water body | 12.22% | 7.63% |
playground | 1.57% | 1.84% |
Square | 1.81% | 2.06% |
Unused land | 20.09% | 17.22% |
vegetation | 18.25% | 20.45% |
Building clearance | 20.54% | 18.26% |
Method | Data sets | Original polygon area(m2) | Error percentage (%) |
Proposed | Data set1 | 665.7 | 0.17 |
Data set2 | 600.4 | 0.22 | |
Method 1 | Data set1 | 500.9 | 2.41 |
Data set2 | 625.8 | 3.48 | |
Method 2 | Data set1 | 529.4 | 2.79 |
Data set2 | 569.4 | 4.94 | |
Method 3 | Data set1 | 565.6 | 4.99 |
Data set2 | 567.1 | 4.71 | |
Method 4 | Data set1 | 505.2 | 2.01 |
Data set2 | 686.7 | 3.85 | |
Method 5 | Data set1 | 537.5 | 2.99 |
Data set2 | 618.7 | 3.75 |
Method | Data sets | X-Y-Z average deviation error |
Proposed | Data set1 | 0.23 |
Data set 2 | 0.77 | |
Method1 | Data set 1 | 1.23 |
Data set 2 | 1.92 | |
Method2 | Data set 1 | 4.45 |
Data set 2 | 4.71 | |
Method3 | Data set 1 | 1.27 |
Data set 2 | 1.28 | |
Method4 | Data set 1 | 2.89 |
Data set 2 | 3.07 | |
Method5 | Data set 1 | 4.77 |
Data set 2 | 2.22 |
Method | Data sets | Health index |
Proposed | Data set1 | 8.5 |
Data set2 | 9.3 | |
Method1 | Data set1 | 4.6 |
Data set2 | 5.5 | |
Method2 | Data set1 | 4.2 |
Data set2 | 5.4 | |
Method3 | Data set1 | 3.6 |
Data set2 | 5.6 | |
Method4 | Data set1 | 6.3 |
Data set2 | 4.7 | |
Method5 | Data set1 | 6.5 |
Data set2 | 5.8 |