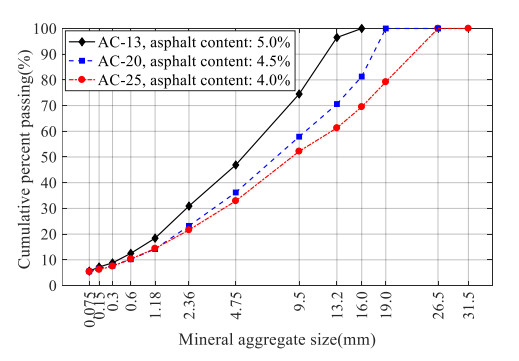
Asphalt mixture is composed of asphalt binder with aggregates of different sizes and compacted under static or dynamic forces. In practical engineering, compaction is a critical step in asphalt pavement construction to determine the quality and service life of pavement. Since the dynamic response characteristics of asphalt pavement can reflect the compaction state of asphalt mixture in the process of compaction, the establishment of the relationship between dynamic response characteristics and compaction degree is definitely significant. In this paper, a series of vibration sensors were adopted to capture the dynamic response signal of the vibration drum and asphalt mixture in the process of vibrating compaction for different surface courses of pavement. Then, the change regulations of vibration acceleration of vibrating drum and asphalt mixture were analyzed, and the quantitative linear relationship was established between accelerations of vibrating drum and asphalt pavement compactness. Further, the concept of evaluation unit (i.e., within 2 meters along the driving direction of the roller) and prediction method of compaction degree were proposed as well. The results showed that under the same vibration compaction condition, the compaction degree values of the top, middle and bottom layers have obvious differences, which should be taken seriously into consideration in the compaction process. Meanwhile, there is little difference which respectively are 2.8, 1.3 and 0.82% for the top, middle and bottom layers between the compaction degrees obtained by the proposed method and measured test. Therefore, the average value of the acceleration peak value of vibration drum within the evaluation unit can be adopted as the characterization index of the compaction degree of asphalt pavement. The investigation of this study can provide the technical reference for compaction control of asphalt pavement to a large extent.
Citation: Hongyu Shan, Han-Cheng Dan, Shiping Wang, Zhi Zhang, Renkun Zhang. Investigation on dynamic response and compaction degree characterization of multi-layer asphalt pavement under vibration rolling[J]. Electronic Research Archive, 2023, 31(4): 2230-2251. doi: 10.3934/era.2023114
[1] | Zhao Dong, Zhiyi Sai, Jinglin Zhang, Guangji Xu . Fatigue damage calculation of cold recycled asphalt pavement considering measured temperature field and traffic volume distribution. Electronic Research Archive, 2023, 31(7): 3722-3740. doi: 10.3934/era.2023189 |
[2] | Han-Cheng Dan, Yongcheng Long, Hui Yao, Songlin Li, Yanhao Liu, Quanfeng Zhou . Investigation on the fractal characteristic of asphalt pavement texture roughness incorporating 3D reconstruction technology. Electronic Research Archive, 2023, 31(4): 2337-2357. doi: 10.3934/era.2023119 |
[3] | Jiuda Huang, Chao Han, Wuju Wei, Chengjun Zhao . Analysis of long-term maintenance decision for asphalt pavement based on analytic hierarchy process and network level optimization decision. Electronic Research Archive, 2023, 31(9): 5894-5916. doi: 10.3934/era.2023299 |
[4] | Hui Sun, Xiupeng Yue, Haining Wang, Liang Wang, Yuexiang Li . Investigation of the dynamic response of subgrade vibration compaction based on the finite element method. Electronic Research Archive, 2023, 31(5): 2758-2774. doi: 10.3934/era.2023139 |
[5] | Xin Gao, Hao Liu, Zhou Fang, Yang Zhang . Microscopic mechanism of subgrade vibration compaction based on discrete element method. Electronic Research Archive, 2023, 31(11): 7061-7077. doi: 10.3934/era.2023358 |
[6] | Hui Yao, Ke Han, Yanhao Liu, Dawei Wang, Zhanping You . Research and comparison of pavement performance prediction based on neural networks and fusion transformer architecture. Electronic Research Archive, 2024, 32(2): 1239-1267. doi: 10.3934/era.2024059 |
[7] | Yuan Ma, Yingcheng Luan, Shuangquan Jiang, Jianming Zhang, Chuanle Wang . Numerical simulation analysis for the effect of water content on the intelligent compaction quality of roadbed. Electronic Research Archive, 2023, 31(8): 4968-4984. doi: 10.3934/era.2023254 |
[8] | Yutong Xie, Yingli Gao, Meijie Liao, Weiwei Tian . Study on the storage stability performance enhancement mechanism of graphene on rubber-modified asphalt based on size effect. Electronic Research Archive, 2023, 31(4): 2048-2070. doi: 10.3934/era.2023105 |
[9] | Liting Yu, Lenan Wang, Jianzhong Pei, Rui Li, Jiupeng Zhang, Shihui Cheng . Structural optimization study based on crushing of semi-rigid base. Electronic Research Archive, 2023, 31(4): 1769-1788. doi: 10.3934/era.2023091 |
[10] | Chengyong Chen, Fagang Chang, Li Li, Wenqiang Dou, Changjing Xu . Optimization of intelligent compaction based on finite element simulation and nonlinear multiple regression. Electronic Research Archive, 2023, 31(5): 2775-2792. doi: 10.3934/era.2023140 |
Asphalt mixture is composed of asphalt binder with aggregates of different sizes and compacted under static or dynamic forces. In practical engineering, compaction is a critical step in asphalt pavement construction to determine the quality and service life of pavement. Since the dynamic response characteristics of asphalt pavement can reflect the compaction state of asphalt mixture in the process of compaction, the establishment of the relationship between dynamic response characteristics and compaction degree is definitely significant. In this paper, a series of vibration sensors were adopted to capture the dynamic response signal of the vibration drum and asphalt mixture in the process of vibrating compaction for different surface courses of pavement. Then, the change regulations of vibration acceleration of vibrating drum and asphalt mixture were analyzed, and the quantitative linear relationship was established between accelerations of vibrating drum and asphalt pavement compactness. Further, the concept of evaluation unit (i.e., within 2 meters along the driving direction of the roller) and prediction method of compaction degree were proposed as well. The results showed that under the same vibration compaction condition, the compaction degree values of the top, middle and bottom layers have obvious differences, which should be taken seriously into consideration in the compaction process. Meanwhile, there is little difference which respectively are 2.8, 1.3 and 0.82% for the top, middle and bottom layers between the compaction degrees obtained by the proposed method and measured test. Therefore, the average value of the acceleration peak value of vibration drum within the evaluation unit can be adopted as the characterization index of the compaction degree of asphalt pavement. The investigation of this study can provide the technical reference for compaction control of asphalt pavement to a large extent.
Asphalt pavement construction is closely related to pavement performance and durability [1,2]. Specifically, the compaction quality has a direct impact on the density, uniformity, pavement surface roughness, thickness and aggregate skeleton structure of pavement. Therefore, the asphalt pavement compactness is one of the main criteria of the durability of asphalt pavement, which is generally expressed by the ratio of the bulk density in the field to that in the laboratory for the compacted material [3,4,5]. In engineering practice, the "under compaction" and "over compaction" are prone to reduce the performance of asphalt mixture pavement [6,7]. Generally speaking, the physical and mechanical properties of "under-compacted" asphalt mixture do not reach the target compaction degree, that is, the compacted quality does not meet the design requirements [8]. Likewise, over compaction is easy to lead to aggregate breakage which makes pavement performance decline [9]. Therefore, the detection of compaction degree can largely judge whether the compaction quality meets the requirements of the specification. At present, the compaction quality of asphalt mixture is mainly detected by traditional method of drilling core sampling, numerical simulation and intelligent compaction technology [10,11,12]. Although the traditional core sampling method can accurately measure the density or compaction degree of the core samples, the core sampling has great randomness and essentially cannot represents a large area of pavement compaction quality. Moreover, this approach destroys the integrity of the pavement structure, and the location of the drill core becomes a weak area [13]. Therefore, the limited detection points and destructive detection method cannot ensure the uniformity and quality requirement of pavement compaction in the conventional spot tests [14].
In order to improve the compaction quality of asphalt pavement, some advanced methods have emerged which can be divided into numerical simulation, theoretical analysis, and field test. As a matter of fact, the dynamic response analysis of asphalt pavement structure is a complicated problem in the process of vibrating compaction. Liu et al. [15] simulated the aggregates movement and rotation in different asphalt mixtures (i.e., AC-11 and PAC-11) during the process of pre-compaction through the discrete element method incorporating finite element method. The results showed that the AC-11 asphalt mixture with dense gradation is easier to be compacted than the porous asphalt mixture (i.e., PAC-11). Masad et al. [16,17] carried out a simulation of the compaction process of asphalt pavement under different rolling modes and pavement structure conditions through 3D DEM incorporating the constitutive model of asphalt mixture. Meanwhile, the influence of vibration frequency and amplitude of roller on the compaction quality was investigated as well. Chen et al. [11] developed a DEM model to simulate the vibration compaction process of asphalt mixture, and mainly focused on the investigation of void variation characteristics of asphalt mixture. Liu et al. [18] established a three-dimensional model of asphalt pavement compaction based on PFC3D software (i.e., DEM simulation) by considering the morphological characteristics of aggregate and the influence of temperature during compaction. It was pointed out that displacement, rotation, contact force between aggregates and energy dissipation can reflect the microscopic characteristics of asphalt mixture in the compaction process. Nevertheless, most of the current numerical simulation studies on asphalt pavement compaction are limited to the laboratory scenario [19], mainly because the field compaction process of asphalt pavement is greatly complex, resulting in a high degree of complexity of the numerical models, especially, the discrete element model, its computational efficiency and accuracy are extremely poor and even deviate from the actual situation. In addition, the mechanical properties and temperature of asphalt mixture change significantly during the compaction process, which makes it difficult for the numerical simulation results to present the real compaction process.
In terms of theoretical analysis, researches presented a series of theoretical models to simulate the dynamic response process under vibratory roller [2,20,21,22,23,24,25]. The typical and representative studies are taken for instance herein. Beainy et al. [2,20,21,22,23] and Imran et al. [24] focused the theoretical modelling on the asphalt-roller interaction during compaction. The proposed theoretical model could take into account the viscoelastic-plasticity of asphalt mixture, the thickness of pavement structure, the variation of temperature and the vibration frequency of roller. Therefore, the proposed model can better characterize the dynamic characteristics of asphalt pavement in the compaction process, and make a great contribution to a deeper understanding of the interaction mechanism between pavement and roller.
With the popularity of vibratory roller and the development of sensors, the intelligent compaction (IC) technology has been proposed in recent decades, which can monitor the pavement compaction degree in real time according to the IC measurement value (ICMV) reflected by the roller response [26,27,28,29]. Chen et al. [30] developed an intelligent system to control the asphalt pavement compaction quality in terms of the rolling uniformity index and rolling standard index. Jiao et al. [31] detected the vibrating compaction value (VCV) was by the intelligent compaction system continuously during the rolling process. The relationship between VCV value and compaction degree of pavement layers was fitted and show that the compaction uniformity of asphalt pavement is good through intelligent compaction. However, due to the influence of mixture type, pavement structure and roller, ICMV has poor correlation with field measured data and cannot accurately characterize the compaction degree of asphalt mixture [32]. Some researchers pointed out more attention needs to be paid to the aggregate movement and the dynamic response information of vibration drum in the process of vibration rolling for different layers of asphalt pavement [33,34,35]. Moreover, the investigation on the state of asphalt mixture from loose to dense can help adjust the construction technology to ensure the target compaction degree and uniformity of paving material [22,36]. As mentioned, the material type and pavement structure affect the compaction effect of pavement, which inevitably leads to the difference of compaction degree under the same vibratory rolling process conditions. Up to now, some researchers investigated the single layer of asphalt mixture in the field test. DAN et al. [34,35] conducted a field and laboratory test to investigate the response regulation of bottom layer of asphalt pavement under vibrating roller. PENG et al. [37] carried out a field investigation and numerical simulation related to the dynamic response of asphalt mixture paved on the concrete bridge deck under vibratory compaction. By considering the potential relationship between the movement characteristics of aggregate and the compactability of asphalt mixture, Yu and Shen [38] adopted SmartRock (i.e., a wireless sensor) to capture the movement signal data of aggregates in the process of vibration compaction. On basis of the collected data, a machine learning method was proposed to predict the asphalt pavement compactness, of which the accuracy is more than 98%.
Although most construction personnel have extensive experience in controlling the compaction quality, numerous poor compaction problems such as under-compaction, over-compaction, and uneven pavement surfaces still occur. This kind of poor quality control of compaction can be attributed to a lack of understanding of the compaction mechanism between the roller and asphalt pavement. Therefore, in order to distinguish the influence of pavement structure and material on compaction effect, sensors such as SmartRock and HCF accelerometers will be employed to monitor the dynamic response of aggregates in the asphalt mixture of different pavement layers and the response regulation of vibration drum in the vibrating rolling process. Based on the relationship between compaction degree and dynamic response law of different pavement layers, this paper will try to put forward the characterization method of compaction degree of each surface layer of asphalt pavement. The proposed method will also be used to predict the compaction degree of pavement, which provides the technical reference for compaction control of asphalt pavement to a large extent. The study content of this paper is organized as follows.
Section 2 introduces the test equipment and method of vibration compaction of asphalt pavement in the field. Section 3 illustrates the temperature variation of asphalt layers, acceleration response characteristics of asphalt mixture and vibration drum during in the process of vibration rolling for top, middle, and bottom layers. In the Section 4, it is expected to establish the relationship between compaction degree and acceleration of vibrating drum, based on which the pavement compaction degree during the process of vibration rolling can be predicted by the acceleration of vibrating drum. Finally, the Section of Conclusion clarify the main results, limitation and outlook of this paper.
The site of field test is located in the LM-3 and LM-4 sections of Zheng-Xi highway. The bottom, middle and top layers of pavement are respectively paved with AC-25, AC-20 and AC-13 asphalt mixture. The layer thicknesses from bottom to top are 8 cm, 6 cm and 4 cm, respectively. The gradation curves and asphalt binder content of asphalt mixtures are shown in the Figure 1. The double-steel-drum vibratory roller (Dynapac CC624HF) is adopted for compacting the asphalt mixture, and the work parameters of vibratory roller are shown in the Table 1.
Total weight / kg | Drum weight /kg | Drum size (diameter/width) /mm | Vibration frequency/ Hz | Excitation force / kN | Rolling speed km/h |
13,600 | 6000 | 1300 × 2130 | 51 | 166 | 4.5 |
The sensor (SmartRock) was embedded in loose asphalt mixture to capture the response signal in real time to investigate the particle movement and internal response of asphalt mixture in the rolling process. The size of the SmartRock is 27 mm × 27 mm × 27 mm, as shown in Figure 2(a). The SmartRock is a kind of ultra-small high temperature resistant sensor of which the size is similar to the coarse aggregate of bottom layer (AC-25) [35,39] and is larger than the aggregates that in top layer (AC-13) and middle layer (AC-20). The outer shell of SmartRock has good compatibility and cohesiveness with asphalt and can transmits the dynamic response data of the mixture particle by 100 Hz sampling frequency to the computer through wireless connection. After paving the asphalt mixture, the SmartRock was embedded in the middle of mixture layer as shown in Figure 2b, and then the excavated mixture was backfilled to restore the smoothness of pavement surface. In order to facilitate data processing, the z-axis, y-axis and x-axis of SmartRock were corresponding to the vertical, transverse and driving direction, respectively.
VOGELE Super-2100 paver was used to pave asphalt mixture in pavement construction. After the paving is completed, the SmartRock were embedded at the preset test points which was marked for compaction positioning and surface temperature measurement. The SmartRock collected the internal response of mixture during the rolling process and received the field data through the laptop connected to the WDQ1 signal receiver. Meanwhile, The HCF sensor was used to collect the acceleration signal of vibration drum and transmitted to laptop computer through USB gateway. It should be noted that the data will be collected and recorded when the roller is about 10 m from the test point, until the repeated rolling is completed. After each rolling of the vibratory roller, the non-nuclear densimeter, the infrared thermal imager recorder (FLIR) and TP700 multi-channel data recorder were used to measure the compaction degree, pavement surface temperature and internal temperature, respectively. According to the vibratory rolling construction, the bottom layer, the middle layer and the top layer were respectively compacted in order, and the field test picture is shown in Figure 3.
Generally speaking, the temperature has a great influence on the compaction performance of asphalt mixture. When the rolling temperature is too high, the asphalt mixture is easy to produce longitudinal rutting, resulting in poor pavement smoothness; On the contrary, when the rolling temperature is too low, the asphalt mixture is difficult to be compacted, resulting in the porosity of the asphalt mixture is greater than the target porosity. Therefore, the field rolling temperature of asphalt mixture will directly affect the compaction quality of asphalt mixture. In this paper, the surface temperature and internal temperature were measured synchronously during the rolling construction of each surface layer, and the temperature test data are shown in Figure 4. It can be seen from the Figure 4a that the surface temperature of asphalt mixture decreases continuously during the rolling process, and the lowest temperature is about 90℃ when the rolling is completed. The pavement surface temperature drops of the top, middle and bottom layers are 34.82, 39.31 and 34.65% respectively. The temperature changes of different surface layers are similar, and gradually decreases with the number of rolling times. According to the Figure 4b, the internal temperature of asphalt mixture with different surface layers increases first and then decreases in the process of rolling. Among them, the internal temperature of the top layer starts to show the turning point of temperature decrease at the earliest, while the internal temperature of the bottom layer decreases at the latest. This indicates that the larger the aggregate size of asphalt mixture and the layer thickness, the less likely the internal heat of the mixture is to lose during the rolling process. Therefore, in order to ensure the compaction effect of asphalt pavement, the thin layer with faster heat dissipation needs to improve its compaction efficiency.
In order to eliminate the noise of signal, according to the band-pass filtering method [35], the field measured data of internal response were processed, and the acceleration data of each layer after each rolling is obtained as shown in Figures 5(a), 6(a) and 7(a).
According to the acceleration time-history curve of asphalt pavement in the bottom, middle and top layers during the vibration rolling process, it can be found that there are obvious acceleration vibration responses in the z-, x- and y- directions. When the drum of the vibratory roller passes above the SmartRock, the acceleration in the three directions has obvious fluctuation, and the peak value in the z-direction (vertical direction) is the largest, while the peak value in the other two horizontal directions is relatively small. Moreover, it can be seen that the acceleration response of asphalt mixture is mainly concentrated in the vertical direction in the process of vibration rolling.
The peak values of x-, y- and z- accelerations of each rolling are plotted as scatter diagram, and the relationship between the number of rolling times and peak values of acceleration are obtained by fitting for the bottom, middle and top layers, which are shown in Figures 5(b), 6(b) and 7(b), respectively. As can be seen from these figures, the peak acceleration of each pavement layer shows a decreasing trend with the progress of vibration and rolling. For the acceleration of the mixture in the bottom layer, the peak accelerations in the z-direction, x-direction and y-direction are 0.9108, 0.6157 and 0.1151 g in the first rolling, while the accelerations in the three directions are 0.1646, 0.1154 and 0.01656 g in the seventh rolling. It can be interpreted that the asphalt mixture is in a relatively loose state before rolling, and the aggregate particles move violently with large displacement for the first and second times of rolling. Then the dense skeleton of asphalt mixture is basically formed, and the acceleration changes tend to be basically stable. It also can be found that the acceleration drops sharply after three times of vibration rolling, which indicates the first three times of rolling can make the asphalt mixture in a relatively dense state, but it still does not meet the specification requirements.
As mentioned above, the peak acceleration of aggregate particles mainly concentrated in the vertical direction (z-direction) and that in rolling direction (x-direction) is relatively lower, and the relationship between the rolling times and peak acceleration in these two directions are very high, while the acceleration in lateral direction (y) has extremely low correlation with rolling times. It can be concluded that the compaction work (energy) is mainly contributed to vertical compaction deformation and forward rubbing, while the lateral extrusion effect is not obvious.
Based on the fitting curve in the Figures 5–7, the relationship between peak acceleration and rolling times can be also established in logarithmic coordinate system, and the linear relationships are obtained as shown in Figures 8 and 9. It can be found that the line slope reflects the compaction ability of asphalt mixture, that is to say, the greater line slope (namely |k|) of the asphalt mixture has better compatibility, and can very likely quicker to form stable solid structure [33].
It can be seen from Figures 8 and 9 that the slope of the fitting curve of the lower layer |k|BL (line slope for bottom layer) is the largest and the |k|TL (line slope for top layer) is the smallest. That is to say, in the process of vibration compaction, the acceleration of asphalt mixture in the bottom layer changes faster with the rolling times than that in the top layer. It fully indicates that the properties of the underlying layer will affect the compaction quality of the compacted material under the same compaction conditions. Meanwhile, it shows that the asphalt mixture of the bottom layer can form a stable skeleton structure quickly by vibration rolling on site. However, it should be pointed out herein that faster skeleton structure formation does not mean higher final compacting degree because it is related to the gradation of the compacted material as well [33]. In general, the skeleton structure of asphalt mixture is mainly composed of coarse aggregate embedded squeeze each other, and the bottom layer mixture of the particle size is larger to form a stable skeleton structure faster. Obviously, it leads to internal mixture of coarse aggregate skeleton structure is difficult to move even though absorb more energy from the vibratory roller compaction. Therefore, the mixture with larger particle or coarse grading material may be difficult to be completely compacted and requires more rolling times.
According to the internal acceleration data of the mixture collected by the SmartRock in the field test, it can be found that the acceleration response is concentrated in the time range of about 1.6 s, while the acceleration amplitude outside the time range is not obvious and decreases quickly with roller drum passing, as shown in Figure 10. As mentioned in Table 1, the moving speed of the roller is about 4.5 km /h, and it can be evaluated that the distance range of the vibration drum passing on the measuring point is about 2 m. Therefore, the testing distance range of vibration acceleration for investigation was set as 2 m in this paper which is 1 m before and after the location of embedded Smartrock, as shown in the Figure 11.
During the field compaction test, the HCF wireless acceleration sensor was used to collect the acceleration signal of the vibrating drum, and the acceleration curves of the vibrating drum in the test area during the rolling of the bottom, middle and top layers are plotted and shown in Figures 12–14.
According to vibration drum acceleration curve, the vibration drum of roller is not a single harmonic motion, but a complex motion superimposed by many different amplitudes in the field rolling process. It shows that the dynamic response of the roller drum in the system composed of the roller and asphalt mixture changes constantly and is affected by the compacted material. Based on the characteristics of vibration acceleration curve, the peak value within a single vibration cycle of the vibrating drum is defined as the representative value of vibration acceleration (as shown in Figure 15(a)), and the representative value curve of acceleration in the test area can be obtained as shown in Figure 15(b).
The average value of peak vibration acceleration in the test area during each rolling is taken as the representative value, and the fitting curve relationship between the representative vibration acceleration value of each surface layer and the compaction times can be established in the logarithmic coordinate system, as shown in Figure 16. It can be seen that the vibration acceleration increases in the rolling process, and the slope of the fitting curve of vibration acceleration of the top layer is the largest, while that of the bottom layer is the smallest. It indicates that the mixture of the bottom layer is easier to form the skeleton structure and the particles interlock with each other thereby resulting in slow growth of vibration acceleration.
Through the internal acceleration of the mixture collected by the SmartRocks, it can be seen that the internal acceleration and vibration acceleration of the mixture have a high linear correlation with the number of rolling times. As a matter of fact, the internal acceleration of the mixture can reflect the mechanical properties of the compacted material. In order to further investigate the relationship between compaction degree of asphalt pavement and the response information of vibrating drum, the relationship between the accelerations of asphalt mixture and vibrating drum is established at first and shown in Figure 17 and the Eqs (1) to (3).
(1) |
(2) |
(3) |
As mentioned in the Section 2.3, the compaction degree is obtained by a non-nuclear densimeter on site after each vibration rolling. Meanwhile, the dynamic response of the aggregates in asphalt mixture can be also captured by the acceleration. Taken the z-direction acceleration as example, the relationship between acceleration and compaction degree can be established respectively for top, middle and bottom layers (See the Figure 18), which have a good linear correlation and are expressed in Eqs (4)–(6). As shown in the Figure 17, it is a very obvious correlation between the accelerations of vibrating drum and asphalt pavement. Further, by combining Eqs (1)–(3) and (4)–(6), the calculation expression of compaction degree of each surface layer can be obtained based on the acceleration of vibrating drum, as shown in Eqs (7)–(9).
(4) |
(5) |
(6) |
(7) |
(8) |
(9) |
Based on the calculation of compaction degree mentioned in the Section 4.1, the predicted compaction degree of asphalt pavement in the rolling process is obtained according to the vibration acceleration data measured in the field and illustrated for different rolling times in the Figures 19–21. The comparison between predicted and tested results are shown in the Table 2.
Surface course | Average CD (%) Calculation | Variance | Average CD (%) Test | Difference (%) |
Top layer (AC-13) | 95.5 | 1.1 | 98.2 | 2.8 |
Middle layer (AC-20) | 96.9 | 1.5 | 97.9 | 1.3 |
Bottom layer (AC-25) | 96.5 | 1.7 | 97.3 | 0.82 |
Taken the Figure 19 as an example, the left half figure indicates the different compaction degree for each rolling time along the driving direction of vibration rolling. The color bar shows the range of compaction degree in which the red color represent the high value. Therefore, it can be seen that the low compaction degree for the first rolling time with yellow color. What's more, the color gradually changes from yellow to red and the color gradually deepens form the top to down. It demonstrates the compaction degree gradually grows up with the number of rolling time. In the Figures 19–21, each square represents a test area for each time (2.13 m × 2.0 m), and the value of compaction degree is plotted in a line chart which indicates the value fluctuation along the driving direction. The average vale of compaction degree for each rolling is calculated and listed in the Table 2. After the field surface construction is completed, random drilling core sampling is carried out in the test area, and the actual compaction degree of asphalt surface is calculated based on the standard density of asphalt mixture in each surface layer.
It can be seen from Table 2 that the calculated compaction degree is lower than the measured compaction degree, because the average value of the vibration drum acceleration peak within the range of the test area is used in the prediction of the compaction degree, and the maximum acceleration peak value of the vibration drum is adopted in the expression of the relationship between the compaction degree and the vibration drum acceleration. Therefore, the predicted compaction degree is lower on the whole, that is, the actual compaction degree is underestimated. It can be inferred that the use of peak vibration acceleration may cause the predicted value to be greater than the measured value of compaction degree, which will lead to premature stop of rolling and the actual compaction degree is not up to the requirements of relevant specification to compactness control. Therefore, the predicted value would rather be small than large, which is a conservative choice from the point of view of practical engineering. At the same time, from the comparison between the predicted value of compaction degree and the measured value of the top, middle and bottom layers, the difference of the top layer is larger, which is very likely due to the difference between the size of SmartRock and the aggregate size of the top layer, which leads to the test of vibration acceleration deviating from the real acceleration value of aggregate. As a whole, it can be considered that it is reasonable and accurate to use vibration acceleration to predict the compaction degree of asphalt pavement.
In this paper, the dynamic response characteristics of asphalt mixture under the condition of vibration rolling were revealed through field test from the particle scale, and the variation regulations of compaction degrees of different asphalt layers (i.e., top, middle and bottom) under the same compaction process conditions were illustrated. Furthermore, the quantitative relationship between the peak value of vibrating drum acceleration and the pavement compaction degree was established, and the compactness in the process of compaction were predicted, which provides technical reference for the compaction control of asphalt pavement. The following summary and conclusions can be drawn in brief.
1) The peak acceleration of aggregate particles mainly concentrated in the vertical direction (z-direction) and that in rolling direction (x-direction) is relatively lower. It indicates that the compaction work (energy) is mainly contributed to vertical compaction deformation and forward rubbing, while the lateral extrusion effect is not obvious.
2) According to the characteristics that the acceleration response is concentrated in the time range of about 1.6 seconds, and the compacting degree evaluation unit is determined to be within 2 meters along the driving direction of the roller.
3) The asphalt mixture in the bottom layer can forms a skeleton structure more quickly, resulting in more difficult movement of particles and absorption of more compaction energy from the roller, which is difficult to compact and requires more compaction times.
4) The different slopes of linear relation between peak value of aggregate acceleration and rolling times show the degree of difficulty of compaction that the top layer is relatively hard to be compacted due to the influence by the middle and the bottom layers under the same compaction conditions.
5) It is reasonable and accurate to use acceleration of vibrating drum in the proposed evaluation unit to predict the compaction degree of asphalt pavement which provides technical reference for the compaction control of asphalt pavement.
Although the compaction dynamic response of different asphalt surface layers were tested in this paper, there are some problems that need further consideration. First, the size of SmartRock is larger than that of the top layer (AC-13) and the middle layer (AC-20), the vibration acceleration of the tested aggregates may deviate from the real value. Second, the increase of compaction degree of asphalt mixture is accompanied by the decrease of temperature, which leads to the decrease of vibration acceleration of asphalt pavement in the process of vibration rolling. Therefore, field test cannot independently distinguish the quantitative influence of temperature on vibration response. Most importantly, the linear relation between the compaction degree and the acceleration of the vibrating drum established in this paper is only accurately applicable to this working condition. The type of pavement material, pavement structure and construction environment (temperature and wind speed) will have certain influence on the linear relation. Therefore, if the linear relation is applied to predict the compaction degree of pavement in the process of compaction, it is necessary to calibrate the linear model in advance to determine more accurate slope and intercept.
This work was funded by the National Natural Science Foundation of China (Grant No. 52278468; U22A20235), the Natural Science Foundation of Hunan Province (CN) (Grant No. 2020JJ4702), and the Jiangxi Transportation Science and Technology Foundation (CN) (Grant No. 2020H0028). Special acknowledgement to the Zhonghai Construction Co., Ltd for providing the test assistance and data collection.
The authors declare there is no conflict of interest.
[1] |
S. C. Zhu, X. D. Li, H. Y. Wang, D. X. Yu, Development of an automated remote asphalt paving quality control system, Transp. Res. Record, 2672 (2018), 28–39. https://doi.org/10.1177/0361198118758690 doi: 10.1177/0361198118758690
![]() |
[2] |
F. Beainy, S. Commuri, M. Zaman, Dynamical response of vibratory rollers during the compaction of asphalt pavements, J. Eng. Mech., 140 (2014), 04014039. https://doi.org/10.1061/(ASCE)EM.1943-7889.0000730 doi: 10.1061/(ASCE)EM.1943-7889.0000730
![]() |
[3] |
R. Micaelo, C. Azevedo, J. Ribeiro, Hot-mix asphalt compaction evaluation with field test, Balt. J. Road Bridge Eng., 9 (2014), 306–316. https://doi.org/10.3846/BJRBE.2014.37 doi: 10.3846/BJRBE.2014.37
![]() |
[4] |
T. Jia, T. He, Z. D. Qian, J. Lv, K. X. Cao, An improved low-cost continuous compaction detection method for the construction of asphalt pavement, Adv. Civil Eng., 2019 (2019), 4528230. https://doi.org/10.1155/2019/4528230 doi: 10.1155/2019/4528230
![]() |
[5] | Ministry of Transport of the People's Republic of China, Standard Test Methods for Bitumen and Bituminous Mixtures for Highway Engineering, JTG E20, China Communications Press, Beijing, China, 2019. |
[6] |
H. C. Dan, Z. Zhang, J. Q. Chen, H. Wang, Numerical simulation of an indirect tensile test for asphalt mixtures using discrete element method software, J. Mater. Civ. Eng., 30 (2018), 04018067. https://doi.org/10.1061/(ASCE)MT.1943-5533.0002252 doi: 10.1061/(ASCE)MT.1943-5533.0002252
![]() |
[7] |
H. C. Dan, J. W. Tan, J. Q. Chen, Temperature distribution of asphalt bridge deck pavement with groundwater circulation temperature control system under high- and low temperature conditions, Road Mater. Pavement Des., 20 (2019), 528–553. https://doi.org/10.1080/14680629.2017.1397048 doi: 10.1080/14680629.2017.1397048
![]() |
[8] |
H. C. Dan, J. W. Tan, Y. F. Du, J. M. Cai, Simulation and optimization of road deicing salt usage based on Water-Ice-Salt Model, Cold Reg. Sci. Technol., 169 (2020), 102917. https://doi.org/10.1016/j.coldregions.2019.102917 doi: 10.1016/j.coldregions.2019.102917
![]() |
[9] |
Y. Q. Tan, H. P. Wang, S. J. Mao, H. N. Xu, Quality control of asphalt pavement compaction using fibre Bragg grating sensing technology, Constr. Build. Mater., 54 (2014), 53–59. https://doi.org/10.1016/j.conbuildmat.2013.12.032 doi: 10.1016/j.conbuildmat.2013.12.032
![]() |
[10] |
Q. Xu, G. K. Chang, Experimental and numerical study of asphalt material geospatial heterogeneity with intelligent compaction technology on roads, Constr. Build. Mater., 72 (2014), 189–198. https://doi.org/10.1016/j.conbuildmat.2014.09.00 doi: 10.1016/j.conbuildmat.2014.09.00
![]() |
[11] |
J. S. Chen, B. S. Huang, X. Shu, C. C. Hu, DEM simulation of laboratory compaction of asphalt mixtures using an open source code, J. Mater. Civ. Eng., 27 (2015), 04014130. https://doi.org/10.1061/(ASCE)MT.1943-5533.0001069 doi: 10.1061/(ASCE)MT.1943-5533.0001069
![]() |
[12] |
G. K. Chang, K. Mohanraj, W. A. Stone, D. J. Oesch, V. Gallivan, Leveraging intelligent compaction and thermal profiling technologies to improve asphalt pavement construction quality: A case study, Transp. Res. Record, 2672 (2018), 48–56. https://doi.org/10.1177/0361198118758285 doi: 10.1177/0361198118758285
![]() |
[13] |
P. Shangguan, I. Al-Qadi, A. Coenen, Algorithm development for the application of ground-penetrating radar on asphalt pavement compaction monitoring, Int. J. Pavement Eng., 17 (2016), 189–200. https://doi.org/10.1080/10298436.2014.973027 doi: 10.1080/10298436.2014.973027
![]() |
[14] |
S. Sivagnanasuntharam, A. Sounthararajah, J. Ghorbani, D. Bodin, J. Kodikara, A state-of-the-art review of compaction control test methods and intelligent compaction technology for asphalt pavements, Road Mater. Pavement Des., 2021. https://doi.org/10.1080/14680629.2021.2015423 doi: 10.1080/14680629.2021.2015423
![]() |
[15] |
P. F. Liu, C.H. Wang, W. Lu, M. Moharekpour, M. Oeser, D. W. Wang, Development of an FEM-DEM model to investigate preliminary compaction of asphalt pavements, Buildings, 12 (2022), 932. https://doi.org/10.3390/buildings12070932 doi: 10.3390/buildings12070932
![]() |
[16] |
E. Masad, A. Scarpas, A. Alipour, K. R. Rajagopal, C. Kasbergen, Finite element modelling of field compaction of hot mix asphalt. Part Ⅰ: Theory, Int. J. Pavement Eng., 17 (2015), 13–23. https://doi.org/10.1080/10298436.2013.863309 doi: 10.1080/10298436.2013.863309
![]() |
[17] |
E. Masad, A. Scarpas, A. Alipour, K. R. Rajagopal, E. Kassem, S. Koneru, et al., Finite element modelling of field compaction of hot mix asphalt. Part Ⅱ: Applications, Int. J. Pavement Eng., 17 (2015), 24–38. https://doi.org/10.1080/10298436.2013.863310 doi: 10.1080/10298436.2013.863310
![]() |
[18] |
W. Liu, X. Gong, Y. Gao, L. Li, Microscopic characteristics of field compaction of asphalt mixture using discrete element method, J. Test. Eval., 47 (2019), 20180633. https://doi.org/10.1520/JTE20180633 doi: 10.1520/JTE20180633
![]() |
[19] |
F. Gong, Y. Liu, X. Zhou, Lab assessment and discrete element modeling of asphalt mixture during compaction with elongated and flat coarse aggregates, Constr. Build. Mater., 182 (2018), 573–579. https://doi.org/10.1016/j.conbuildmat.2018.06.059 doi: 10.1016/j.conbuildmat.2018.06.059
![]() |
[20] |
B. Fares, C. Sesh, Z. Musharraf, Dynamical response of vibratory rollers during the compaction of asphalt pavements, J. Eng. Mech., 140 (2014), 04014039. https://doi.org/10.1061/(ASCE)EM.1943-7889.0000730 doi: 10.1061/(ASCE)EM.1943-7889.0000730
![]() |
[21] |
G. P. Qian, K. K. Hu, J. Li, X. Bai, N. Li, Compaction process tracking for asphalt mixture using discrete element method, Constr. Build. Mater., 235 (2020), 117478. https://doi.org/10.1016/j.conbuildmat.2019.117478 doi: 10.1016/j.conbuildmat.2019.117478
![]() |
[22] |
F. Beainy, S. Commuri, M. Zaman, Quality assurance of hot mix asphalt pavements using the intelligent asphalt compaction analyzer, J. Constr. Eng. Manage., 138 (2012), 178–187. https://doi.org/10.1061/(ASCE)CO.1943-7862.0000420 doi: 10.1061/(ASCE)CO.1943-7862.0000420
![]() |
[23] |
F. Beainy, S. Commuri, M. Zaman, I. Syed, Viscoelastic-plastic model of asphalt-roller interaction, Int. J. Geomech., 13 (2013), 581–594. https://doi.org/10.1061/(ASCE)GM.1943-5622.0000240 doi: 10.1061/(ASCE)GM.1943-5622.0000240
![]() |
[24] |
S. A. Imran, S. Commuri, M. Barman, M. Zaman, F. Beainy, Modeling the dynamics of asphalt-roller interaction during compaction, J. Constr. Eng. Manage., 143 (2017), 04017015. https://doi.org/10.1061/(ASCE)CO.1943-7862.0001293 doi: 10.1061/(ASCE)CO.1943-7862.0001293
![]() |
[25] |
Y. Shi, H. Liu, G Wang, Modeling of asphalt mixture-screed interaction: a nonlinear dynamic vibration model for improving paving density, Constr. Build. Mater., 311 (2021), 125296. https://doi.org/10.1016/j.conbuildmat.2021.125296 doi: 10.1016/j.conbuildmat.2021.125296
![]() |
[26] |
X. Zhu, S. Bai, G. Xue, Assessment of compaction quality of multi-layer pavement structure based on intelligent compaction technology, Constr. Build. Mater., 161 (2018), 316–329. https://doi.org/10.1016/j.conbuildmat.2017.11.139 doi: 10.1016/j.conbuildmat.2017.11.139
![]() |
[27] |
X. Y. Zhu, S. J. Bai, G. P. Xue, J. Yang, Y. S. Cai, W. Hu, et al., Assessment of compaction quality of multi-layer pavement structure based on intelligent compaction technology, Constr. Build. Mater., 161 (2018), 316–329. https://doi.org/10.1016/j.conbuildmat.2017.11.139 doi: 10.1016/j.conbuildmat.2017.11.139
![]() |
[28] |
W. Hu, B. S. Huang, X. Shu, M. Woods, Utilising intelligent compaction meter values to evaluate construction quality of asphalt pavement layers, Road Mater. Pavement Des., 18 (2016), 1–12. https://doi.org/10.1080/14680629.2016.1194882 doi: 10.1080/14680629.2016.1194882
![]() |
[29] |
W. Hu, X. Y. Jia, X. Y. Zhu, H. R. Gong, G. P. Xue, B. S. Huang, Investigating key factors of intelligent compaction for asphalt paving: A comparative case study, Constr. Build. Mater., 229 (2019), 116876. https://doi.org/10.1016/j.conbuildmat.2019.116876 doi: 10.1016/j.conbuildmat.2019.116876
![]() |
[30] |
B. Chen, X. Yu, F. Dong, C. Zheng, G. Ding, W. Wu, Compaction quality evaluation of asphalt pavement based on intelligent compaction technology, J. Constr. Eng. Manage., 147 (2021), 04021099. https://doi.org/10.1061/(ASCE)CO.1943-7862.0002115 doi: 10.1061/(ASCE)CO.1943-7862.0002115
![]() |
[31] | X. L. Jiao, Z. G. Feng, S. J. Wang, M. W. Biboussi, X. J. Li, Correlation between intelligent compaction index and compaction degree of asphalt pavement, in International Conference on Smart Transportation and City Engineering 2021, 12050 (2021), 120503M. https://doi.org/10.1117/12.2613891 |
[32] |
R. V. Rinehart, M. A. Mooney, Instrumentation of a roller compactor to monitor vibration behavior during earthwork compaction, Autom. Constr., 17 (2008), 144–150. https://doi.org/10.1016/j.autcon.2006.12.006 doi: 10.1016/j.autcon.2006.12.006
![]() |
[33] |
H. C. Dan, D. Yang, L. H. Zhao, S. P. Wang, Z. Zhang, Meso-scale study on compaction characteristics of asphalt mixtures in Superpave gyratory compaction using SmartRock sensors, Constr. Build. Mater., 262 (2020), 120874. https://doi.org/10.1016/j.conbuildmat.2020.120874 doi: 10.1016/j.conbuildmat.2020.120874
![]() |
[34] |
S. P. Wang, H. C. Dan, L. Li, X. Liu, Z. Zhang, Dynamic response of asphalt pavement under vibration rolling load: Theory and calibration, Soil Dyn. Earthquake Eng., 143 (2021), 106633. https://doi.org/10.1016/j.soildyn.2021.106633 doi: 10.1016/j.soildyn.2021.106633
![]() |
[35] |
H. C. Dan, D. Yang, X. Liu, A. P. Peng, Z. Zhang, Experimental investigation on dynamic response of asphalt pavement using SmartRock sensor under vibrating compaction loading, Constr. Build. Mater., 247 (2020), 118592. https://doi.org/10.1016/j.conbuildmat.2020.118592 doi: 10.1016/j.conbuildmat.2020.118592
![]() |
[36] |
Q. W. Xu, G. K. Chang, V. L. Gallivan, R. D. Horan, Influences of intelligent compaction uniformity on pavement performances of hot mix asphalt, Constr. Build. Mater., 30 (2012), 746–752. https://doi.org/10.1016/j.conbuildmat.2011.12.082 doi: 10.1016/j.conbuildmat.2011.12.082
![]() |
[37] |
A. P. Peng, H. C. Dan, D. Yang, Experiment and numerical simulation of the dynamic response of bridges under vibratory compaction of bridge deck asphalt pavement, Math. Prob. Eng., 2019 (2019), 7020298. https://doi.org/10.1155/2019/2962154 doi: 10.1155/2019/2962154
![]() |
[38] |
S. Yu, S. H. Shen, Compaction prediction for asphalt mixtures using wireless sensor and machine learning algorithms, IEEE Trans. Intell. Transp. Syst., 2022. https://doi.org/10.1109/TITS.2022.3218692 doi: 10.1109/TITS.2022.3218692
![]() |
[39] |
X. Wang, S. H. Shen, H. Huang, L. C. Almeida, Characterization of particle movement in Superpave gyratory compactor at meso-scale using SmartRock sensors, Constr. Build. Mater., 175 (2018), 206–214. https://doi.org/10.1016/j.conbuildmat.2018.04.146 doi: 10.1016/j.conbuildmat.2018.04.146
![]() |
1. | Han-Cheng Dan, Peng Xie, Hongyu Shan, Zhi Zhang, Wei Cao, Investigation on the roller-pavement interaction and asphalt pavement compactness based on marginal spectrum of vibration signal energy, 2024, 411, 09500618, 134381, 10.1016/j.conbuildmat.2023.134381 |
Total weight / kg | Drum weight /kg | Drum size (diameter/width) /mm | Vibration frequency/ Hz | Excitation force / kN | Rolling speed km/h |
13,600 | 6000 | 1300 × 2130 | 51 | 166 | 4.5 |
Surface course | Average CD (%) Calculation | Variance | Average CD (%) Test | Difference (%) |
Top layer (AC-13) | 95.5 | 1.1 | 98.2 | 2.8 |
Middle layer (AC-20) | 96.9 | 1.5 | 97.9 | 1.3 |
Bottom layer (AC-25) | 96.5 | 1.7 | 97.3 | 0.82 |
Total weight / kg | Drum weight /kg | Drum size (diameter/width) /mm | Vibration frequency/ Hz | Excitation force / kN | Rolling speed km/h |
13,600 | 6000 | 1300 × 2130 | 51 | 166 | 4.5 |
Surface course | Average CD (%) Calculation | Variance | Average CD (%) Test | Difference (%) |
Top layer (AC-13) | 95.5 | 1.1 | 98.2 | 2.8 |
Middle layer (AC-20) | 96.9 | 1.5 | 97.9 | 1.3 |
Bottom layer (AC-25) | 96.5 | 1.7 | 97.3 | 0.82 |