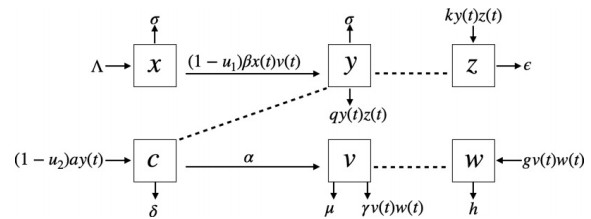
In this paper, a mathematical model describing the hepatitis B virus (HBV) infection of hepatocytes with the intracellular HBV-DNA containing capsids, cytotoxic T-lymphocyte (CTL), antibodies including drug therapy (blocking new infection and inhibiting viral production) with two-time delays is studied. It incorporates the delay in the productively infected hepatocytes and the delay in an antigenic stimulation generating CTL. We verify the positivity and boundedness of solutions and determine the basic reproduction number. The local and global stability of three equilibrium points (infection-free, immune-free, and immune-activated) are investigated. Finally, the numerical simulations are established to show the role of these therapies in reducing viral replication and HBV infection. Our results show that the treatment by blocking new infection gives more significant results than the treatment by inhibiting viral production for infected hepatocytes. Further, both delays affect the number of infections and duration i.e. the longer the delay, the more severe the HBV infection.
Citation: Pensiri Yosyingyong, Ratchada Viriyapong. Global dynamics of multiple delays within-host model for a hepatitis B virus infection of hepatocytes with immune response and drug therapy[J]. Mathematical Biosciences and Engineering, 2023, 20(4): 7349-7386. doi: 10.3934/mbe.2023319
[1] | Sarah Kadelka, Stanca M Ciupe . Mathematical investigation of HBeAg seroclearance. Mathematical Biosciences and Engineering, 2019, 16(6): 7616-7658. doi: 10.3934/mbe.2019382 |
[2] | Suqi Ma . Low viral persistence of an immunological model. Mathematical Biosciences and Engineering, 2012, 9(4): 809-817. doi: 10.3934/mbe.2012.9.809 |
[3] | Dong-Me Li, Bing Chai, Qi Wang . A model of hepatitis B virus with random interference infection rate. Mathematical Biosciences and Engineering, 2021, 18(6): 8257-8297. doi: 10.3934/mbe.2021410 |
[4] | Ting Guo, Zhipeng Qiu . The effects of CTL immune response on HIV infection model with potent therapy, latently infected cells and cell-to-cell viral transmission. Mathematical Biosciences and Engineering, 2019, 16(6): 6822-6841. doi: 10.3934/mbe.2019341 |
[5] | Maysaa Al Qurashi, Saima Rashid, Fahd Jarad . A computational study of a stochastic fractal-fractional hepatitis B virus infection incorporating delayed immune reactions via the exponential decay. Mathematical Biosciences and Engineering, 2022, 19(12): 12950-12980. doi: 10.3934/mbe.2022605 |
[6] | A. M. Elaiw, A. S. Shflot, A. D. Hobiny . Stability analysis of general delayed HTLV-I dynamics model with mitosis and CTL immunity. Mathematical Biosciences and Engineering, 2022, 19(12): 12693-12729. doi: 10.3934/mbe.2022593 |
[7] | Cameron Browne . Immune response in virus model structured by cell infection-age. Mathematical Biosciences and Engineering, 2016, 13(5): 887-909. doi: 10.3934/mbe.2016022 |
[8] | Yiping Tan, Yongli Cai, Zhihang Peng, Kaifa Wang, Ruoxia Yao, Weiming Wang . Dynamics of a stochastic HBV infection model with drug therapy and immune response. Mathematical Biosciences and Engineering, 2022, 19(8): 7570-7585. doi: 10.3934/mbe.2022356 |
[9] | Tinevimbo Shiri, Winston Garira, Senelani D. Musekwa . A two-strain HIV-1 mathematical model to assess the effects of chemotherapy on disease parameters. Mathematical Biosciences and Engineering, 2005, 2(4): 811-832. doi: 10.3934/mbe.2005.2.811 |
[10] | Xuejuan Lu, Lulu Hui, Shengqiang Liu, Jia Li . A mathematical model of HTLV-I infection with two time delays. Mathematical Biosciences and Engineering, 2015, 12(3): 431-449. doi: 10.3934/mbe.2015.12.431 |
In this paper, a mathematical model describing the hepatitis B virus (HBV) infection of hepatocytes with the intracellular HBV-DNA containing capsids, cytotoxic T-lymphocyte (CTL), antibodies including drug therapy (blocking new infection and inhibiting viral production) with two-time delays is studied. It incorporates the delay in the productively infected hepatocytes and the delay in an antigenic stimulation generating CTL. We verify the positivity and boundedness of solutions and determine the basic reproduction number. The local and global stability of three equilibrium points (infection-free, immune-free, and immune-activated) are investigated. Finally, the numerical simulations are established to show the role of these therapies in reducing viral replication and HBV infection. Our results show that the treatment by blocking new infection gives more significant results than the treatment by inhibiting viral production for infected hepatocytes. Further, both delays affect the number of infections and duration i.e. the longer the delay, the more severe the HBV infection.
Hepatitis B virus (HBV) infection is a significant worldwide health issue. It is a liver infection caused by the hepatitis B virus. Generally, the infection is classified as either acute or chronic and can lead to more serious long-term complications, such as liver inflammation, cirrhosis or liver cancer [1]. According to World Health Organisation (WHO) reports, HBV infection is mostly notified in Africa, Southern Europe, Asia and Latin America. 257 million people were living with chronic HBV infection in 2015 [2] and about 887,000 people died. In extremely endemic areas, hepatitis B is most commonly spread from mother to child at birth or transmitted through contact with the blood or other body fluids of an infected person. From the global epidemic situation, it is essential to have some effective prevention and treatment measures for hepatitis B infection.
HBV can replicate within hepatocytes without causing direct cell damage, this can be seen in those who are asymptomatic HBV carriers. Approximately 5-10% of HBV-infected adults may progress to a chronic state. The immune responses to HBV antigens are responsible for both disease pathogenesis and viral clearance. The adaptive immune response specifically the virus-specific cytotoxic T lymphocytes (CTL) is shown to play a key role in eliminating the infected cells and inhibiting viral replication [3,4,5,6,7,8,9,10,11]. Another adaptive immune response is the antibodies, which are produced by the B cells, that neutralize virus particles and prevent the reinfection of cells [10,12]. Further, the body's immune response would take time from being attacked by viruses to the cell becoming productively infected, therefore time delay regarding to this circumstance should not be ignored [13,14,15,16,17,18,19,20]. In addition, HBV infections have shown some time delay in virus amplification and spreading through the liver [21].
People with chronic hepatitis B infection are recommended to have some medication to reduce the risk of disease progression, prevent transmission to others and decrease the risk of complications of hepatitis B. There are two main types of drugs which are standard PEGylated interferon (PEG-IFN) and nucleoside analogues (NAs). IFN has a role in suppressing viral protein synthesis, preventing viral infection of cells and degradation of viral mRNA. The NAs play a role in elongating DNA in order to inhibit HBV replication [21,22,23]. In addition, in some cases, the treatment may include antiviral medications (e.g. lamivudine, adefovir, entecavir) and the interferon alfa-2b injection [24]. However, mentioned drugs can hardly clear the viral covalently closed circular DNA (cccDNA) which is responsible for the persistence of HBV [25,26]. The alternative therapies have been recently in clinical trials and proposed, they base on viral gene silencing by controlling the RNA interference (RNAi) pathway which suppresses HBV replication and may result in disabling cccDNA during chronic infection [25,26]. With the fact mentioned above, although the HBV vaccines are widely used, safe and effective and there are some drugs that could cure and greatly reduce the viral burden [27,28], there are limitations against chronic infection. Hence, HBV infection is still a major health problem around the world.
Mathematical models have been shown to greatly contribute to a better understanding of HBV infection. The work by Nowak et al. [29] is one of the earliest models about the HBV infection of hepatocytes consisting of three variables which are the concentration of uninfected cells, infected cells and free virus particles. There are a number of mathematical models that have been proposed after that (e.g. [30,31,32,33,34,35,36,37,38,39]). Some models involve treatments or drug efficacy (e.g. [38,40,41]). In some studies, the time delay has been considered. The models which involve the time delay from being infected to the release of free virus particles and free movement of virus particles in the liver are of the works by Gourley et al., 2008 [42]; Xie et al., 2010 [43]; Guo and Cai, 2011 [16]; Wang et al., 2008 [44]. Further, some studies involve the effect of humoral immunity or CTL-mediated cellular immunity e.g. the work by Yousfi et al., 2011 [34] and Fisicaro et al., 2009 [45]. Recently, Sun et al., 2017 [46] proposed a delay model with 6 variables including exposed state, CTL and alanine aminotransferases (ALT), where the delay was put on the CTL process. In 2015, Manna and Chakrabarty, 2015 [47] proposed a model which included the intracellular HBV DNA-containing capsids and a delay in the production of the infected hepatocytes. Later on, Guo et al., 2018 [48] extended the work of Manna and Chakrabarty, 2015 [47] by adding a delay during the time when the infected cells create new intracellular HBV DNA-containing capsids due to the penetration by the virus. Furthermore, Aniji et al., 2020 [49] proposed the model involves a delay as a time between antigenic stimulation and the production of CTL including a delay during the decay of CTL. With the important role of antibodies against HBV infection, Meskaf et al., 2017 [18], Sun et al., 2017 [46] and Allali et al., 2018 [50] had added antibodies as variables into their models. In addition, some researchers have developed HBV models which involve both diffusion and delay (e.g. [51,52]). Among the above studies, in some studies drug therapies have also been applied in the models e.g the work by Hattaf et al., 2009 [53], Manna and Chakrabarty, 2018 [54] and in particular Danane and Allali, 2018 [20] had included the drug therapies in their delay model. Further, some researchers have proposed models involving infection in the form of fractional differential equations (e.g. [55,56]).
In this paper, we have developed a model for HBV infection which incorporates the intracellular HBV DNA-containing capsids, CTL and antibodies with a time delay from being attacked by the virus to being infected hepatocytes and a time delay in the antigenic stimulation generating CTL. Further, two drug therapies, i.e., blocking new infection and inhibiting viral production have been applied in the model. The structure of the paper starts with a description of the proposed model in section 2, followed by the model properties, the basic reproduction number, three equilibrium states and their global stability. In section 3, the numerical simulations are presented and discussed. Finally, we end this paper with conclusions in section 4.
We have developed a delay model describing the hepatitis B virus (HBV) dynamics involving immune response and drug therapy by extending the work of Danane and Allali, 2018 [20] by adding the delay time that an antigenic stimulation generating CTL, which is τ2 in our model. This is because we take into account the fact that the antigenic stimulation generates CTL cells may require a time lag and in this model, we assume that CTL produced depends on infected cells. This model is described by a system of delay differential equations (2.1), it includes six variables: the concentration of uninfected hepatocytes x(t), infected hepatocytes y(t), intracellular HBV DNA-containing capsids c(t), free viruses v(t), antibodies w(t), and CTL z(t). The uninfected hepatocytes x(t) are produced at a constant rate Λ and die at a rate σ. The infection of hepatocytes in this model incorporates the uninfected become infected hepatocytes by the free virus with a rate β with involvement of the efficiency of drug therapy in blocking new infection u1. The e−mτ1 is the probability of surviving hepatocytes in the time period from t−τ1 to t, where m is a constant rate of the death average of infected hepatocytes which are still not virus-producing cells. Time τ1 is the delay in the productively infected hepatocytes. This infection term is represented by the nonlinear term (1−u1)e−mτ1βx(t)v(t). The infected hepatocytes y(t) are eliminated by the CTL, z(t), with a rate q and die at a rate σ, which has the same rate as the mortality rate of uninfected hepatocytes as we assume there is no increase in death rate of infected hepatocytes due to an infection. The production of intracellular HBV DNA-containing capsids c(t) incorporates the efficiency of drug therapy in inhibiting viral production u2 with a production rate a, described by the term (1−u2)ay(t). The intracellular HBV DNA-containing capsids are transmitted into the bloodstream to become free viruses at a rate α and are decomposed at a rate δ. The free viruses are reduced by the neutralization rate of antibodies γ and die at a rate μ. The antibodies are enhanced in response to the free viruses at a rate g and decay at a rate h. Further, the second time delay in this model cannot be ignored for the immune response, that is the activation of CTL producing antigens may require a period of time τ2. Therefore, we propose the form ky(t−τ2)z(t−τ2) and the CTL decay at the rate ϵ. The flow chart of the model is presented in Figure 1.
This model can be written into a form of system of delay differential equations as follows:
dxdt=Λ−σx(t)−(1−u1)βx(t)v(t)dydt=(1−u1)βe−mτ1x(t−τ1)v(t−τ1)−σy(t)−qy(t)z(t)dcdt=(1−u2)ay(t)−αc(t)−δc(t)dvdt=αc(t)−γv(t)w(t)−μv(t)dwdt=gv(t)w(t)−hw(t)dzdt=ky(t−τ2)z(t−τ2)−ϵz(t), | (2.1) |
with initial condition
x(0)≥0,y(0)≥0,c(0)≥0,v(0)≥0,w(0)≥0,z(0)≥0, | (2.2) |
for τ1>0 and τ2>0. Here, 0<u1<1 and 0<u2<1.
The Banach space of continuous functions mapping the interval [−τ,0] into R6+ is defined by C=C([−τ,0],R6+), where τ=max[τ1,τ2]. For any φ∈C([−τ,0],R6+) by the fundamental theory of functional differential equations (see [59]) there exists a unique solution P(t,φ)=((x(t,φ),y(t,φ),c(t,φ),v(t,φ),w(t,φ),z(t,φ)) of the system (2.1), which satisfies P0=φ. The initial conditions are given by x(θ)≥0,y(θ)≥0,c(θ)≥0,v(θ)≥0,w(θ)≥0,z(θ)≥0 with θ∈[−τ,0] and y(0),c(0),v(0),w(0),z(0)>0.
For system (2.1) to be epidemiologically meaningful, we prove that all state variables are non-negative. Since it is irrational to have a negative hepatocytes density and system (2.1) describes the dynamics of HBV infection of hepatocytes, we show that all state variables stay non-negative and the solutions of system (2.1) with non-negative initial conditions will remain non-negative for fall t>0. The following lemma is applied.
Lemma 1. Given that the initial solutions and parameters of system (2.1) are non-negative, the solutions x(t),y(t),c(t),v(t),w(t) and z(t) stay non-negative for all t>0.
Proof. Consider the first equation in system (2.1) we have,
dxdt=Λ−σx−(1−u1)βxvdxdt+(σ+(1−u1)βv)x=Λ. | (2.3) |
We multiply both sides of the differential equation by the integrating factor which is defined as
I=e∫t0(σ+(1−u1)βv(s))ds. | (2.4) |
Multiply equation (2.3) by I, we have
(e∫t0(σ+(1−u1)βv(s))ds)dxdt+(e∫t0(σ+(1−u1)βv(s))ds)(σ+(1−u1)βv)x=(e∫t0(σ+(1−u1)βv(s))ds)Λ. | (2.5) |
We integrate both side between 0 and t, then
∫t0(e∫t0(σ+(1−u1)βv(s)) ds)(dx(s)dt+(σ+(1−u1)βv(s))x(s))ds=∫t0(e∫t0(σ+(1−u1)βv(s)) ds)Λds. |
Thus, x(t)=(e−∫t0(σ+(1−u1)βv(s)) ds)(x(0)+∫t0(e∫t0(σ+(1−u1)βv(s)) ds)Λds), leads to x(t)≥0.
Similarly,
y(t)=e−∫t0(σ+qz(s))ds(y(0)+∫t0e∫t0(σ+qz(s))ds(1−u1)βe−mτ1x(s−τ1)v(s−τ1)ds)≥0c(t)=e−(α+δ)t(c(0)+∫t0(1−u2)ay(s)e(α+δ)ds)≥0v(t)=e−∫t0(γw(s)+μ)ds(v(0)+∫t0e∫t0(γw(s)+μ)dsαc(s)ds)≥0w(t)=w(0)e∫t0(gv(s)−h)ds≥0z(t)=e−ϵt(z(0)+∫t0ky(s−τ2)z(s−τ2)eϵtds)≥0. | (2.6) |
Therefore, x(t)≥0, y(t)≥0, c(t)≥0, v(t)≥0, w(t)≥0, z(t)≥0 for all t>0 given that x(0)≥0, y(0)≥0, c(0)≥0, v(0)≥0, w(0)≥0, z(0)≥0.
Theorem 1. Under the given initial conditions, all solutions of system (2.1) are non-negative and bounded for all t≥0.
Proof. First, we use the following function to help determining the boundness of the solutions of system (2.1):
N(t)=e−mτ1x(t−τ1)+y(t)+qkz(t+τ2)+σ2(1−u2)ac(t)+σ2(1−u2)av(t)+σγ2(1−u2)agw(t). | (2.7) |
By differentiating (2.7) with respect to t and with system (2.1), we have
dN(t)dt= e−mτ1dx(t−τ1)dt+dydt+qdkz(t+τ2)dt+σ2(1−u2)adc(t)dt+σ2(1−u2)adv(t)dt+σγ2(1−u2)gadw(t)dt= Λe−mτ1−σe−mτ1x(t−τ1)−(1−u1)βe−mτ1x(t−τ1)v(t−τ1)+(1−u1)βe−mτ1x(t−τ1)v(t−τ1)−(σ−σ2)y(t)−qy(t)z(t)+qy(t)z(t)−qϵkz(t+τ2)−σα2(1−u2)ac(t)−σδ2(1−u2)ac(t)+σα2(1−u2)ac(t)−σγ2(1−u2)av(t)w(t)−σμ2(1−u2)av(t)+σγ2(1−u2)av(t)w(t)−σγh2(1−u2)gaw(t)= Λe−mτ1−σe−mτ1x(t−τ1)−σ2y(t)−qϵkz(t+τ2)−σδ2(1−u2)ac(t)−σμ2(1−u2)av(t)−σγh2(1−u2)gaw(t)≤ Λe−mτ1−min(σ,σ2,ϵ,δ,μ,h)(e−mτ1x(t−τ1)+y(t)+qkz(t+τ2)+σ2(1−u1)ac(t)+σ2(1−u1)av(t)+σγ2(1−u2)gaw(t))= Λe−mτ1−min(σ,σ2,ϵ,δ,μ,h)N(t). | (2.8) |
Let Q=min(σ,σ2,ϵ,δ,μ,h).
Thus, we have
dN(t)dt≤Λe−mτ1−QN(t). | (2.9) |
By integrating both sides,
∫t0dN(t)Λe−mτ1−QN(t)≤∫t0dtNt≤Λe−mτ1−e−Qt(Λe−mτ1−QN0)Q. |
By taking t→∞, we have
Nt≤Λe−mτ1Q. | (2.10) |
Hence, we have that N(t) is bounded, which leads to the variables x(t),y(t),c(t),v(t),w(t) and z(t) are bounded.
In this section, we compute steady states of system (2.1). There are five steady states as follows.
1. The infection-free steady state E0 is (x0,y0,c0,v0,w0,z0)=(Λσ,0,0,0,0,0).
2. The immune-free steady state E1 is (x1,y1,c1,v1,0,0) where
x1=σμ(α+δ)(1−u1)(1−u2)βe−mτ1aα,y1=(α+δ)c1(1−u2)a,c1=σμ(1−u1)βα(R0−1),v1=αc1μ. E1 exists when R0>1.
3. The immune-activated infection steady state E2 is (x2,y2,c2,v2,w2,z2) where
x2=Λgσg+(1−u1)βh,y2=ϵk,c2=(1−u2)aϵk(α+δ),v2=hg,w2=(1−u2)aϵαg(α+δ)γhk−μγ,
z2=(1−u1)βΛhke−mτ1(σg+(1−u1)βh)qϵ−σq. E2 exists when (1−u2)aϵαg(α+δ)hk>μ and (1−u1)βΛhke−mτ1(σg+(1−u1)βh)ϵ)>σ.
4. The andibody-free steady state E3 is (x3,y3,c3,v3,0,z3) where
x3=Λσ−(1−u1)βv3,y3=ϵk,c3=(1−u2)ay3α+δ,v3=αc3μ,w3=0,
z3=(1−u1)βe−mτ1x3v3−σy3qy3. E3 exists when σ>(1−u1)βv3 and (1−u1)βe−mτ1x3v3>σy3.
5. The CTL-free steady state E4 is (x4,y4,c4,v4,w4,0) where
x4=Λσ−(1−u1)βv4,y4=(1−u1)βe−mτ1x4v4σ,c4=(1−u2)ay4(α+δ),v4=hg,w4=αc4−μv4γv4,z4=0.
E4 exists when σ>(1−u1)βv4 and αc4>μv4.
To calculate R0, we used the next-generation matrix method by van den Driessche et al., 2002 [60] and we obtain
F=[(1−u1)βe−mτ1xv00]and V=[σy+qzyαc+δc−(1−u2)ayγvw+μv−αc]. | (2.11) |
Then we have
F=[0 0 (1−u1)βe−mτ1x0 000 00]and V=[σ+qz 00−(1−u2)a α+δ00 −αγw+μ]. | (2.12) |
By substituting the infection-free equilibrium point (2.1.3) in the Jacobian matrices above, we get
F=[0 0 (1−u1)βe−mτ1Λσ0 000 00]and V=[σ 00−(1−u2)a α+δ00 −αμ]. | (2.13) |
Next,
V−1=1μσ(α+δ)[μ(α+δ)00μ(1−u2)a μσ0(1−u2)aα ασ σ(α+δ)]. | (2.14) |
The next generation matrix is
FV−1=[(1−u1)(1−u2)βe−mτ1Λaασ2μ(α+δ)(1−u1)βe−mτ1Λασ2μ(α+δ)(1−u1)βe−mτ1Λσ2μ000000]. | (2.15) |
The basic reproduction number is given by ρ(FV−1), thus
R0=(1−u1)(1−u2)βe−mτ1Λaασ2μ(α+δ). | (2.16) |
Theorem 2. (local stability at E0) If R0<1, then the infection-free equilibrium point (E0) is locally asymptotically stable. Otherwise, it is unstable.
Proof. The Jacobian matrix of system (2.1) at E0 is
J(E0)=[−σ00−(1−u1)βx0000−σ0(1−u1)βe−(m+λ)τ1x0000(1−u2)a−(α+δ)00000α−μ000000−h000000−ϵ]. | (2.17) |
From Jacobian matrix above, we have the characteristic equation as
(λ+ϵ)(λ+h)(λ+σ)((λ+σ)(λ+α+δ)(λ+μ)−(1−u1)(1−u2)aαβe−(m+λ)τ1x0)=0. | (2.18) |
Thus, λ1=−ϵ<0, λ2=−h<0, λ3=−σ<0.
Since, x0=Λσ and R0=(1−u1)(1−u2)βe−mτ1Λaασ2μ(α+δ), we write the rest of the term as
(λ+σ)(λ+α+δ)(λ+μ)−(1−u1)(1−u2)aαβe−(m+λ)τ1Λσ=0,(λ+σ)(λ+α+δ)(λ+μ)=(1−u1)(1−u2)aαβe−(m+λ)τ1Λσ,(λ+σ)(λ+α+δ)(λ+μ)=μσ(α+δ)R0e−λτ1. | (2.19) |
For R0<1, if λ has a non-negative real part then the modulus of the left-hand side of equation (2.19) satisfies
⏐(λ+σ)(λ+α+δ)(λ+μ)⏐≥σ(α+δ)μ. | (2.20) |
Consider the modulus of the right-hand side of equation (2.19),
⏐μσ(α+δ)R0e−λτ1⏐≤μσ(α+δ)R0<μσ(α+δ), | (2.21) |
which is contradiction. Hence, when R0<1, the real part of λ has no non-negative real part and the infection-free state E0 is locally asymptotically stable.
For R0>1, we let
h(λ)=(λ+σ)(λ+α+δ)(λ+μ)−μσ(α+δ)R0e−λτ1. | (2.22) |
Then,
h(0)=μσ(α+δ)−μσ(α+δ)R0<0, | (2.23) |
and limλ→∞h(λ)=+∞.
By the continuity of h(λ), there exists at least one positive root of h(λ)=0. Thus, the infection-free equilibrium point, E0 is unstable when R0>1. This completes the proof.
Theorem 3. If R0<1, the infection-free equilibrium point (E0) is globally asymptotically stable.
Proof. Let the Lyapunov functions be
L(t)= x0(xx0−ln(xx0)−1)+emτ1y(t)+(1−u1)βΛαc(t)μσ(α+δ)+(1−u1)βΛv(t)μσ+(1−u1)βΛγw(t)gμσ+(1−u1)β∫tt−τ1x(s)v(s)ds, | (2.24) |
where L is positive definite. The derivative of L along the solutions of the system (2.1) is
dLdt= (1−x0x)(Λ−σx−(1−u1)βxv)+emτ1((1−u1)βe−mτ1x(t−τ1)v(t−τ1)−σy−qyz)+(1−u1)βΛαμσ(α+δ)((1−u2)ay−(α+δ)c)+(1−u1)βΛμσ(αc−γvw−μv)+(1−u1)βΛγgμσ(gvw−hw)+(1−u1)β(xv−x(t−τ1)v(t−τ1)). | (2.25) |
Since,
dx0dt=Λ−σx0−(1−u1)βx0v0=0,we have Λ=σx0. | (2.26) |
Then, dLdt= (1−x0x)(σx0−σx−(1−u1)βxv)+(1−u1)βx(t−τ1)v(t−τ1)−σyemτ1−qyzemτ1+(1−u1)(1−u2)βΛαayμσ(α+δ)−(1−u1)βΛαcμσ+(1−u1)βΛαcμσ−(1−u1)βΛγvwμσ−(1−u1)βΛvσ+(1−u1)βΛγvwμσ−(1−u1)βΛγhwgμσ+(1−u1)βxv−(1−u1)βx(t−τ1)v(t−τ1)= −σ(x−x0)2x−qyzemτ1+σyemτ1((1−u1)(1−u2)βΛαae−mτ1σ2μ(α+δ)−1)−(1−u1)βΛγhwgμσ= −σ2xσ(x−x0)2−qyzemτ1+σyemτ1(R0−1)−(1−u1)βΛγhwgμσ. | (2.27) |
We obtain that dLdt<0 when R0<1 and dLdt=0 at E0. Therefore, E0 is globally asymptotically stable when R0<1.
Theorem 4. (local stability at E1) If 1<R0<1+inf{A1,A2},
where A1=(1−u1)(1−u2)ϵaβαkσμ(α+δ) and A2=(1−u1)hβgσ, then the immune-free equilibrium point (E1) is locally asymptotically stable. If R0>1+inf{A1,A2}, then E1 is unstable.
Proof. We first set det(J(E1)−λI)=0 to find eigenvalues, then we obtain det(J(E1)−λI)
=⏐−σ−(1−u1)βv1−λ00−(1−u1)βx100(1−u1)βv1e−(m+λ)τ1−σ−λ0(1−u1)βe−(m+λ)τ1x10−qy10(1−u2)a−(α+δ)−λ00000α−μ−λ−γv100000gv1−h−λ000000ky1e−λτ2−ϵ−λ⏐. | (2.28) |
=(ky1e−λτ2−ϵ−λ)(gv1−h−λ)⏐−σ−(1−u1)βv1−λ00−(1−u1)βx1(1−u1)βv1e−(m+λ)τ1−σ−λ0(1−u1)βx1e−(m+λ)τ10(1−u2)a−(α+δ)−λ000α−μ−λ⏐ | (2.29) |
= (ky1e−λτ2−ϵ−λ)(gv1−h−λ)(−σ−(1−u1)βv1−λ)|−σ−λ0(1−u1)βx1e−(m+λ)τ1(1−u2)a−(α+δ)−λ00α−μ−λ|−(ky1e−λτ2−ϵ−λ)(gv1−h−λ)(1−u1)βv1e−(m+λ)τ1|00−(1−u1)βx1(1−u2)a−(α+δ)−λ00α−μ−λ| | (2.30) |
= (ky1e−λτ2−ϵ−λ)(gv1−h−λ)(σ+(1−u1)βv1+λ)(σ+λ)|−(α+δ)−λ0α−μ−λ|+(ky1e−λτ2−ϵ−λ)(gv1−h−λ)(σ+(1−u1)βv1+λ)(1−u2)a|0(1−u1)βx1e−(m+λ)τ1α−μ−λ|+(ky1e−λτ2−ϵ−λ)(gv1−h−λ)(1−u1)(1−u2)βv1ae−(m+λ)τ1|0−(1−u1)βx1α−μ−λ| | (2.31) |
By calculating above expression, we have characteristic equation as
(ky1e−(m+λ)τ2−ϵ−λ)(gv1−h−λ)[λ4+a1λ3+a2λ2+a3λ+a4+(a5λ+a6)e−λτ1]=0 | (2.32) |
where
a1=α+δ+μ+2σ+(1−u1)βv1,a2=(2σ+(1−u1)βv1)(α+δ+μ)+(σ+(1−u1)βv1)σ+μ(α+δ),a3=(2σ+(1−u1)βv1)(α+δ)μ+(σ+(1−u1)βv1)σ(α+δ+μ),a4=(σ+(1−u1)βv1)σ(α+δ)μ,a5=−(1−u1)(1−u2)βaαx1e−mτ1,a6=−(1−u1)(1−u2)βaαx1(σ+(1−u1)βv1)e−mτ1+(1−u1)2(1−u2)β2x1v1αae−mτ1. | (2.33) |
Therefore, it gives λ1=ky1(t−τ2)e−λτ2−ϵ and λ2=gv1−h.
First, we consider
λ1=ky1e−λτ2−ϵ. | (2.34) |
For τ2=0, If 1<R0<1+(1−u1)(1−u2)ϵaβαkσμ(α+δ). Then, we have λ1=ky1−ϵ, since y1=σμ(α+δ)(R0−1)(1−u1)(1−u2)βαa.
Thus,
λ1=k(σμ(α+δ)(R0−1)(1−u1)(1−u2)βαa)−ϵ=kσμ(α+δ)(R0−1)−ϵ(1−u1)(1−u2)βαa(1−u1)(1−u2)βαa<kσμ(α+δ)(1+(1−u1)(1−u2)ϵaβαkσμ(α+δ)−1)−ϵ(1−u1)(1−u2)βαa(1−u1)(1−u2)βαa=0 |
Thus, λ1<0. This shows that λ1<0 for τ2=0. Next, we consider the case when τ2>0. By letting λ1=ωi (ω>0) be a purely imaginary root for some ω>0, we have
(iω)−ky1e−iωτ2+ϵ=0iω−ky1(cos(ωτ2)−isin(ωτ2))+ϵ=0(iω)+ϵ=ky1(cos(ωτ2)−isin(ωτ2)). |
Thus, this implies that ϵ=ky1cos(ωτ2) and ω=−ky1sin(ωτ2).
Then,
ω2+ϵ2=(ky1)2(cos2(ωτ2)+sin2(ωτ2))ω2=(ky1)2−ϵ2ω2=(kσμ(α+δ)(R0−1)(1−u1)(1−u2)βαa)2−ϵ2. |
Since 1<R0<1+(1−u1)(1−u2)ϵaβαkσμ(α+δ), then
ω2<(kσμ(α+δ)(1+(1−u1)(1−u2)ϵaβαkσμ(α+δ)−1)(1−u1)(1−u2)βαa)2−ϵ2=0 |
Thus, ω2<0 which is contradiction.
Next, suppose that λ1=b+ωi where b is positive real number and ω>0, we can write
λ1=h−ϵ, where h=ky1e−λτ2. | (2.35) |
Then, the magnitude of h is as follows when b is positive real number,
|h|=|ky1e−(b+ωi)τ2|=ky1e−bτ2|e−iωτ2|. |
Since
e−ωiτ2=cos(ωτ2)−isin(ωτ2),and|e−iωτ2|=1,then|h|=ky1e−bτ2≤ky1. | (2.36) |
Substituting y1 into (2.36), we have
|h|≤kσμ(α+δ)(R0−1)(1−u1)(1−u2)aβα<kσμ(α+δ)(1+(1−u1)(1−u2)ϵaβαkσμ(α+δ)−1)(1−u1)(1−u2)aβα=ϵ. | (2.37) |
Thus, |h|<ϵ implie that h∈B(0,ϵ). If h=D+Ci where D>0, then h is complex number in the right-half of complex plane. However, if h−ϵ=D+Ci−ϵ, then D−ϵ is negative real part. Therefore, we have h−ϵ is a complex number in the left-half of complex plane, then consider the left hand side of the equation (2.35) as
λ1=b+ωi. | (2.38) |
Since we suppose that b>0 and λ1=h−ϵ, then λ1 will be a complex number on the right-half of complex plane. We have
b+ωi=D−ϵ+Ci | (2.39) |
By assumption b>0, but D−ϵ<0. This is contradiction, because b can not be a positive real part.
Therefore, λ1 has a negative real part, when 1<R0<1+(1−u1)(1−u2)ϵaβαkσμ(α+δ).
Next, we consider λ2=gv1−h. If 1<R0<1+h(1−u1)βgσ, then
λ2=g(σ(R0−1)(1−u1)β)−h<gσ(1+h(1−u1)βgσ−1)(1−u1)β−h=0 |
Thus, λ2<0. Therefore, λ2 is negative when 1<R0<1+h(1−u1)βgσ.
Then, we consider the characteristic equation where τ1>0,
λ4+a1λ3+a2λ2+a3λ+a4+(a5λ+a6)e−λτ1=0 | (2.40) |
where a1−a6 are defined in (2.33).
Thus, we have
|λ4+a1λ3+a2λ2+a3λ+a4|2=|a5λ+a6|2|e−λτ1|2. | (2.41) |
Suppose (2.40) has a purely imaginary root λ=iω (ω>0), by substituting λ=iω into (2.41) and separating the real and imaginary parts, we have
|(iω)4+a1(iω)3+a2(iω)2+a3(iω)+a4|2=|a5(iω)+a6|2|e−iωτ1|2. |
Since |e−iωτ1|=|cos(−ωτ1)+isin(−ωτ1)|=√cos2(ωτ1)+sin2(ωτ1)=1, then we have
|ω4−a1ω3i−a2ω2+a3ωi+a4|2=|a5ωi+a6|.2 | (2.42) |
Thus, we have
|ω4−a1ω3i−a2ω2+a3ωi+a4|2=(ω4−a1ω3i−a2ω2+a3ωi+a4)(ω4+a1ω3i−a2ω2−a3ωi+a4)=ω8−a2ω6+a4ω4+a21ω6−a1a3ω4−a2ω6+a22ω4−a2a4ω2−a1a3ω4+a23ω2+a4ω4−a2a4ω2+a24, | (2.43) |
and
|a5ωi+a6|2=(a5ωi+a6)(−a5ωi+a6)=a25ω2+a26. | (2.44) |
Thus, equation (2.42) becomes
ω8+D1ω6+D2ω4+D3ω2+D4=0 | (2.45) |
where D1=−2a2+a21, D2=2a4−2a1a3+a22, D3=a23−2a2a4−a25, D4=a24−a26. | (2.46) |
We let X=ω2 and define a function G(X) as the left-hand side of (2.45), the above equation can be simplified to
G(X)=X4+D1X3+D2X2+D3X+D4. | (2.47) |
Therefore, if the characteristic equation (2.40) has a purely imaginary root (λ=iω), it is equivalent to the fact that G(X)=0 has a positive real root (X=ω2).
Theorem 5. If G(X)=0 has no positive real roots, then the positive equilibrium point E1 is locally asymptotically stable for any τ1>0.
Proof. If G(X)=0 has no positive real roots, we obtain that X can be zero or negative root. Since X=ω2, so ω can be either zero or bi for b>0. But from the hypothesis that ω>0, we then have ω=bi, implying that (2.40) have negative roots i.e. λ=ωi=(bi)i=−b. Therefore, the equilibrium E1 is locally asymptotically stable for any τ1>0 when G(X)=0 has no positive real roots.
Next, we consider E1 being locally asymptotically stable for [0,τ01) such that τ01=min{τj1n|1≤n≤˜n} where ˜n is the number of roots of G(X).
Substituting λ=iω into (2.40), we obtain the real part as
ω4−a2ω2+a4+a6cos(ωτ1)+a5ωsin(ωτ1)=0 | (2.48) |
and the imaginary part as
a1ω3−a3ω+a6sin(ωτ1)−a5ωcos(ωτ1)=0. | (2.49) |
Next, we solve for cos(ωτ1) and sin(ωτ1) from equation (2.48) and (2.49). Assuming that G(X)=0 has (1≤˜n≤4) positive real roots, denoted by Xn(1≤n≤˜n). As √Xn=ω, (2.49) then becomes
a1(√Xn)3−a3√Xn−a5cos(√Xnτ1)=−a6sin√Xnτ1. |
Thus,
sin(√Xnτ1)=a3√Xn+a5cos(√Xnτ1)−a1(√Xn)3a6. | (2.50) |
Substituting (2.50) into (2.48), we have
cos(√Xnτ1)=[(a1a5−a6)X2n+(a2a6−a3a5)Xn−a4a6]a26+a25√Xn. | (2.51) |
Then, substitute (2.51) into (2.50), gives
sin(√Xnτ1)=a2a5Xn+a3a6√Xn−a5a6X2n−a1a6(√Xn)3−a4a5a26+a25√Xn. | (2.52) |
Let
cos(√Xnτ1)=Qn=[(a1a5−a6)X2n+(a2a6−a3a5)Xn−a4a6]a26+a25√Xnsin(√Xnτ1)=Pn=a2a5Xn+a3a6√Xn−a5a6X2n−a1a6(√Xn)3−a4a5a26+a25√Xn. | (2.53) |
Therefore, for the imaginary root λ=iω of (2.40), we have two sequences as follows:
τj1n={1√Xn(arccos(Qn)+2jπ),if Pn≥01√Xn(2π−arccos(Qn)+2jπ), ifPn<0 |
where 1≤n≤˜n and j=0,1,2,3,...
Assuming τ(0)1n=min{τ(j)1n|1≤n≤˜n,j=0,1,2}, i.e., τ(0)1n is the minimum value associated with the imaginary solution iω0 of the characteristic equation (2.40). Therefore, the characteristic equation (2.40) has a pair of purely imaginary roots ±i√Xn.
For every integer j and 1≤n≤˜n, define λ(j)n(τ1)=α(j)n(τ1)+iω(j)n(τ1) as the root of (2.40) near τ(j)1n, satisfying α(j)1n(τ(j)1n)=0 and ω(j)n(τ(j)1n)=√Xn.
Theorem 6. If G(X)=0 has some positive real roots, then E1 is locally asymptotically stable for τ1∈[0,τ(0)1n), when τ(0)1n=min{τ(j)1n|1≤n≤˜n,j=0,1,2,...}.
Proof. For τ(0)1n=min{τ(j)1n≤n≤˜n,j=0,1,2,...}, G(X)=0 has no positive real roots when τ1∈[0,τ(0)1n), which means that all the roots of (2.40) have strictly negative real part when τ1∈[0,τ(0)1n). Therefore, E1 is locally asymptotically stable for τ1∈[0,τ(0)1n).
Theorem 7. If Xn0 is a simple root of G(X)=0, then there is a Hopf bifurcation for the system as τ1 increases past τ(0)1n0.
Proof. The characteristic equation (2.40) can be written into the following form:
f0(λ)+f1(λ)e−λτ1=0, | (2.54) |
where f0(λ)=λ4+a1λ3+a2λ2+a3λ+a4 and f1(λ)=a5λ+a6, and f0(λ) and f1(λ) are continuously differentiable to λ.
Next, we determine sign{dRe(λ)dτ1|τ1=τ(0)1n}, where sign is the sign function and Re(λ) is the real part of λ. We assume that λ(τ1)=v(τ1)+iω(τ1) is the solution of (2.40) with respect to τ1. Suppose that one of the roots of (2.54) is λ(τ1)=α(τ1)+iω(τ1), satisfying α(τ10)=0 and ω(τ10)=ω0 for a positive real number τ10.
Let
ϕ(ω)=|f0(iω)|2−|f1(iω)|2. | (2.55) |
Since
|f0(iω)|2= (¯f0(iω))(f0(iω))= ω8+a1ω7i−a2ω6−a3ω5i+a4ω4−a1ω7i+a21ω6+a1a2ω5i−a1a3ω4−a1a4ω3i−a2ω6−a1a2ω5i+a22ω4+a2a3ω3i−a2a4ω2+a3ω5i−a1a3ω4−a2a3ω3i+a23ω2+a3a4ωi+a4ω4+a1a4ω3i−a2a4ω2−a3a4ωi+a24. | (2.56) |
Then,
d(|f0(iω)|2)dω=8ω7+(6a21−12a2)ω5+(4a22+8a4−8a1a3)ω3+(2a23−4a2a4)ω. | (2.57) |
And since f1(iω)=a5(iω)+a6=a5iω+a6,
|f1(iω)|2=(f1(iω))(¯f1(iω))=(a5iω+a6)(−a5iω+a6)=a25ω2+a6. | (2.58) |
Then, d|f1(iω)|2dω=2a25ω.
Thus, we have
12ωdϕdω=12ωd(|f0(iω)|2−|f1(iω)|2)dω=12ω(d|f0(iω)|2dω−d|f1(iω)|2dω)=12ω(−2Im(¯f0(iω)˙f0(iω))+2Im(¯f1(iω)˙f1(iω)))=Im[˙f1(iω)¯f1(iω)f1(iω)ωf1(iω)−˙f0(iω)¯f0(iω)f0(iω)ωf0(iω)]=Im[|f1(iω)|2˙f1(iω)ωf1(iω)−|f0(iω)|2˙f0(iω)ωf0(iω)]. | (2.59) |
Because |f0(iω0)|2=|f1(iω0)|2, we have
(12ωdϕdω)|ω=ω0=|f0(iω)|2Im[˙f1(iω0)ω0f1(iω0)−˙f0(iω0)ω0f0(iω0)]. | (2.60) |
Next, differentiate both sides of (2.54) with respect to τ1, we have
˙f0(λ)dλdτ1+˙f1(λ)dλdτ1e−λτ1−(λ+τ1dλdτ1)f1(λ)e−λτ1=0. | (2.61) |
We can write (2.61) as
(dλdτ1)−1=˙f0(λ)+˙f1(λ)e−λτ1−f1(λ)τ1e−λτ1λf1(λ)e−λτ1=˙f0(λ)eλτ1+˙f1(λ)λf1(λ)−τ1λ. | (2.62) |
Since f0(iω0)+f1(iω0)e−iω0τ1=0, we obtain that
Re[(dλdτ1)−1|τ1=τ0]=Re[˙f0(iω0)eiω0τ1+˙f1(iω0)iω0f1(iω0)]=Re[˙f0(iω0)eiω0τ1iω0f1(iω0)+˙f1(iω0)iω0f1(iω0)]=Re[−˙f0(iω0)eiω0τ1iω0f0(iω0)eiω0τ1+˙f1(iω0)iω0f1(iω0)]=Re[˙f0(iω0)eiω0τ1ω0f0(iω0)eiω0τ1(i)−˙f1(iω0)ω0f1(iω0)(i)]=Im[˙f1(iω0)ω0f1(iω0)−˙f0(iω0)ω0f0(iω0)]. | (2.63) |
From (2.60) and (2.63), we have
sign[dRe(λ)dτ1|τ1=τ0]=sign Re[(dλdτ1|τ1=τ0)]=sign Re[dλdτ1|τ1=τ0]−1=sign Re[Im[˙f1(iω0)ω0f1(iω0)−˙f0(iω0)ω0f0(iω0)]]=sign Re[|f0(iω)|2Im[˙f1(iω0)ω0f1(iω0)−˙f0(iω0)ω0f0(iω0)]]=sign [(12ω×dϕdω)|ω=ω0]. | (2.64) |
When Re(λ)=α(j)n(τ1), we have
sign [dαjn(τ1)dτ1|τ1=τj1n]=sign [(dGdx)|X=Xn]. | (2.65) |
As Xn0 is a simple root of G(X)=0, we know ˙G(Xn0)≠0. From (2.65), we know (dα(0)n0dτ1|τ1=τ(0)1n≠0). If dα(0)n0dτ1|τ1=τ(0)1n<0, then we obtain that the root of (2.40) has positive real part when τ1∈[0,τ(0)1n0) which contrasts to Theorem 6. Hence, we can see that dα(0)n0dτ1|τ1=τ(0)1n>0. When τ1=τ(0)1n0, except for the pair of purely imaginary root, the remaining roots of (2.40) have strictly negative real parts, so the system has Hopf bifurcation. This completes the proof.
Theorem 8. The immune-free equilibrium point E1 is globally asymptotically stable when 1<R0<1+inf{A1,A2}, where A1=(1−u1)(1−u2)ϵaβαkσμ(α+δ) and A2=(1−u1)hβgσ.
Proof. We consider the function G(x)=x−1−lnx (x>0). Note that G(x)≥0,∀x and that G(x)=0 if and only if x=1. We define a Lyapunov function L1 as follows:
L1= x1(xx1−1−lnxx1)+emτ1y1(yy1−1−lnyy1)+(1−u1)βx1v1c1(α+δ)c1(cc1−1−lncc1)+(1−u1)βx1v1v1αc1(vv1−1−lnvv1)+(1−u1)βx1v1γwgαc1+qemτ1zk+(1−u1)βx1v1∫tt−τ1G(x(s)v(s)x1v1)ds+qemτ1∫tt−τ2y(s)z(s)ds. | (2.66) |
dL1dt= (1−x1x)(Λ−σx−(1−u1)βxv)+emτ1(1−y1y)((1−u1)βe−mτ1x(t−τ1)v(t−τ1)−σy−qyz)+(1−u1)βx1v1c1(α+δ)(1−c1c)((1−u2)ay−(α+δ)c)+(1−u1)βx1v1c1(α+δ)(1−v1v)(αc−γvw−μv)+(1−u1)βx1v1γgαc1(gvw−hw)+qemτ1k(ky(t−τ2)z(t−τ2)−ϵz)+(1−u1)βx1v1(xvx1v1−x(t−τ1)v(t−τ1)x1v1+lnx(t−τ1)v(t−τ1)xv)+qemτ1(yz−y(t−τ2)z(t−τ2)). | (2.67) |
Since dx1dt=0, then Λ=σx1+(1−u1)βx1v1. Therefore,
dL1dt= (1−x1x)(σx1+(1−u1)βx1v1−σ−(1−u1)βxv)+(1−u1)βx(t−τ1)v(t−τ1)−σemτ1y−qemτ1yz−y1y(1−u1)βx(t−τ1)v(t−τ1)+σemτ1y1+qemτ1y1z+(1−u1)(1−u2)βx1v1ay(α+δ)c1−(1−u1)βx1v1cc1−(1−u1)(1−u2)βx1v1ayc1(α+δ)c1c+(1−u1)βx1v1+(1−u1)βx1v1cc1−(1−u1)βx1v1γvwαc1−(1−u1)βx1v1μvαc1−(1−u1)βx1v21αcαc1v+(1−u1)βx1v21γvwαc1v+(1−u1)βx1v21μαc1+(1−u1)βx1v1vwαc1−(1−u1)βx1v1γhwgαc1+qemτ1ky(t−τ2)z(t−τ2)k−qemτ1ϵzk+(1−u1)βx1v1(xvx1v1−x(t−τ1)v(t−τ1)x1v1+lnx(t−τ1)v(t−τ1)xv)+qemτ1yz−qemτ1y(t−τ2)z(t−τ2). | (2.68) |
dL1dt= −σ(x−x1)2x+2(1−u1)βx1v1−(1−u1)βx1v1x1x+(1−u1)βx1v+(1−u1)βx(t−τ1)v(t−τ1)−σemτ1y−y1y(1−u1)βx(t−τ1)v(t−τ1)+σemτ1y1+qemτ1y1z+(1−u1)(1−u2)βx1v1ay(α+δ)c1−(1−u1)(1−u2)βx1v1ayc1(α+δ)c1c−(1−u1)βx1v1μvαc1−(1−u1)βx1v21cc1v+(1−u1)βx1v21γwαc1+(1−u1)βx1v21μαc1−(1−u1)βx1v1γhwgαc1−qemτ1ϵzk+(1−u1)βx1v1(−x(t−τ1)v(t−τ1)x1v1+lnx(t−τ1)v(t−τ1)xv). | (2.69) |
Since c1=(1−u2)ay1α+δ, we have (1−u1)(1−u2)βx1v1ayc1(α+δ)c1c=(1−u1)βx1v1yc1y1c and v1=αc1μ then (1−u1)βx1v1μv1αc1=(1−u1)βx1v1 and dy1dt=0, we have (1−u1)βx1v1=σy1emτ1.
Then,
dL1dt=−σ(x−x1)2x+(1−u1)βx1v1(4−x1x−y1x(t−τ1)v(t−τ1)yx1v1−yc1y1c−v1cvc1+lnx(t−τ1)v(t−τ1)xv)−σemτ1y+qemτ1y1z+(1−u1)(1−u2)βx1v1ay(α+δ)c1+(1−u1)βx1v1γwv1αc1−(1−u1)βx1v1γhwgαc1−qemτ1ϵzk. | (2.70) |
Substituting x1=σμ(α+δ)(1−u1)(1−u2)βe−mτ1aα,c1=(1−u2)ay1α+δ and v1=αc1μ into (1−u1)(1−u2)βx1v1ay(α+δ)c1=σemτ1y. We have v1=σ(R0−1)(1−u1)β from 1<R0<1+(1−u1)gβgσ then v1<hg and y1=(α+δ)σμ(R0−1)(1−u1)(1−u2)βaα from 1<R0<(1−u1)(1−u2)ϵaβαkσμ(α+δ), we have y1<ϵk. Then,
dL1dt=−σ(x−x1)2x+(1−u1)βx1v1(4−x1x−y1x(t−τ1)v(t−τ1)yx1v1−yc1y1c−v1cvc1+lnx(t−τ1)v(t−τ1)xv)+qemτ1z(y1−ϵk)+(1−u1)βx1v1γwαc1(v1−hg). | (2.71) |
We obtain that dLdt<0 when 1<R0<1+inf{A1,A2}, where A1=(1−u1)(1−u2)ϵaβαkσμ(α+δ) and A2=(1−u1)hβgσ and dLdt=0 at E1. Therefore, E1 is globally asymptotically stable when 1<R0<1+inf{A1,A2}, where A1=(1−u1)(1−u2)ϵaβαkσμ(α+δ) and A2=(1−u1)hβgσ.
Theorem 9. The immune-activated infection equilibrium point E2 is globally asymptotically stable when R0>1 and A>B (where A and B are defined in the proof).
Proof. We consider the function G(x)=x−1−lnx (x>0). Note that G(x)≥0,∀x and that G(x)=0 if and only if x=1. We define a Lyapunov function L2 as follows:
L2= x2(xx2−1−lnxx2)+emτ1y2(yy2−1−lnyy2)+((1−u1)βx2v2(α+δ)c2)c2(cc2−1−lncc2)+((1−u1)βx2v2αc2)v2(vv2−1−lnvv2)+((1−u1)βγx2v2αgc2)w2(ww2−1−lnww2)+(qemτ1k)z2(zz2−1−lnzz2)+(1−u1)βx2v2∫tt−τ1G(x(θ)v(θ)x2v2)dθ+qemτ1y2z2∫tt−τ2G(y(θ)z(θ)y2z2)dθ. | (2.72) |
Then,
dL2dt= (1−x2x)(Λ−σx(t)−(1−u1)βx(t)v(t))+emτ1(1−y2y)((1−u1)βe−mτ1x(t−τ1)v(t−τ1)−σy(t)−qy(t)z(t))+((1−u1)βx2v2(α+δ)c2)(1−c2c)((1−u2)ay(t)−αc(t)−δc(t))+((1−u1)βx2v2αc2)(1−v2v)(αc(t)−γv(t)w(t)−μv(t))+((1−u1)βγx2v2αgc2)(1−w2w)(gv(t)w(t)−hw(t))+(qemτ1k)(1−z2z)(ky(t−τ2)z(t−τ2)−ϵz(t))+(1−u1)βx2v2(x(t)v(t)x2v2−x(t−τ1)v(t−τ1)x2v2+lnx(t−τ1)v(t−τ1)x(t)v(t))+qemτ1y2z2(y(t)z(t)y2z2−y(t−τ2)z(t−τ2)y2z2+lny(t−τ2)z(t−τ2)y(t)z(t)). | (2.73) |
Since dx2dt=0 then Λ=σx2+(1−u1)βx2v2 and y2=ϵk, we have
dL2dt=−σ(x−x2)2x−x2x(1−u1)βx2v2+(1−u1)βx2v+(1−u1)βx2v2−σemτ1y−y2y(1−u1)βx(t−τ1)v(t−τ1)+σmτ1y2+(1−u1)(1−u2)βx2v2ay(α+δ)c2−(1−u1)βx2v2cc2−(1−u1)(1−u2)βx2v2ay(α+δ)c+(1−u1)βx2v2+((1−u1)βx2v2αc2)(1−v2v)(αc(t)−γv(t)w(t)−μv(t))+((1−u1)βγx2v2αgc2)(1−w2w)(gv(t)w(t)−hw(t))−z2zqemτ1y(t−τ2)z(t−τ2)+qemτ1y2z2+(1−u1)βx2v2lnx(t−τ1)v(t−τ1)xv+qemτ1y2z2lny(t−τ2)z(t−τ2)y(t)z(t). | (2.74) |
dL2dt=−σ(x−x2)2x+(1−u1)βx2v2(2−x2x−y2yx(t−τ1)v(t−τ1)x2v2−cc2+lnx(t−τ1)v(t−τ1)xv)+qemτ1y2z2(1−z2zy(t−τ2)z(t−τ2)y2z2+lny(t−τ2)z(t−τ2)yz)+(1−u1)βx2v−σemτ1y+σemτ1y2+(1−u1)(1−u2)βx2v2ay(α+δ)c2−(1−u1)(1−u2)βx2v2ay(α+δ)c+((1−u1)βx2v2αc2)(1−v2v)(αc(t)−γv(t)w(t)−μv(t))+((1−u1)βrx2v2αgc2)(1−w2w)(gv(t)w(t)−hw(t)). | (2.75) |
From
dy2dt=0 and dc2dt=0, |
we have
(1−u1)βx2v2−qy2z2emτ1=σemτ1y2and(1−u2)ay2=(α+δ)c2. | (2.76) |
Then,
dL2dt=−σ(x−x2)2x+(1−u1)βx2v2(3−x2x−y2yx(t−τ1)v(t−τ1)x2v2−cc2+lnx(t−τ1)v(t−τ1)xv−c2ycy2)+qemτ1y2z2(2−y2y−y(t−τ2)z(t−τ2)y2z+lny(t−τ2)z(t−τ2)yz)+qemτ1y22z2y+(1−u1)βx2v+qz2emτ1y−2qemτ1y2z2+(1−u1)βx2v2cc2−(1−u1)βx2v2αc2γvw−(1−u1)βx2v2cv2c2v+(1−u1)βx2v22γwαc2+(1−u1)βx2v22μαc2−(1−u1)βx2v2μvαc2+(1−u1)βx2v2γvwαc2−(1−u1)βx2v2γhwαgc2−(1−u1)βx2v2γw2vαc2+(1−u1)βx2v2γhw2αgc2. | (2.77) |
And since, dv2dt=0, γw2=αc2−μv2v2 and v2=hg, then
dL2dt=−σ(x−x2)2x+(1−u1)βx2v2(4−x2x−y2yx(t−τ1)v(t−τ1)x2v2−cv2c2v−c2ycy2+lnx(t−τ1)v(t−τ1)xv)+qemτ1y2z2(2−y2y−y(t−τ2)z(t−τ2)y2z+lny(t−τ2)z(t−τ2)yz)+qemτ1y22z2y+qemτ1yz2−2qemτ1y2z2. | (2.78) |
Let A=σ(x−x2)2x−(1−u1)βx2v2(4−x2x−y2yx(t−τ1)v(t−τ1)x2v2−cv2c2v−c2ycy2+lnx(t−τ1)v(t−τ1)xv)− qemτ1y2z2(2−y2y−y(t−τ2)z(t−τ2)y2z+lny(t−τ2)z(t−τ2)yz)+ 2qemτ1y2z2 and B=qemτ1yz2+qemτ1y22z2y. Thus, the global stability of immune-activated steady state equilibrium point is globally asymptotically stable when R0>1 and A>B.
Next, we perform numerical simulation for system (2.1) to confirm global stability of the three above equilibrium points.
Case I: infection-free equilibrium point
In this case, we used β=3×10−13, then the infection-free equilibrium point (E0=(368.6455,0,0,0,0,0)) is globally asymptotically stable when R0=2.9178×10−10<1 as shown in Figure 2.
Case II: immune-free equilibrium point
In this case, 1<R0=1.3616<inf{A1,A2}=2.1932 at β=0.0014 and k=0.001 the immune-free equilibrium point (270.7360,92.6698,56.8294,5.6829,0,0) is globally asymptotically stable as shown in Figure 3.
Case III: immune-activated infection equilibrium point
The last critical point is the immune-activated infection equilibrium is globally asymtotically stable when R0=13.6164>1 as shown in Figure 4. We use a=1.5, then E2=(168.0870,50,306.6211,18.7500,44.0279,30.7616).
In this section, the numerical simulations of the system (2.1) are performed with the use of parameters values from Table 1. We divide the results into 4 cases as follows to investigate the impact of drug therapy (u1 and u2) and to explore the dynamics of model in the different values of time delays.
Parameter | Description | Value | Unit | Ref |
x | the concentration of uninfected hepatocytes. | |||
y | the concentration of infected hepatocytes. | |||
c | the concentration of intracellular HBV | |||
DNA-containing capsids. | ||||
v | the concentration of free viruses. | |||
w | the concentration of antibodies expansion | |||
in response to free viruses. | ||||
z | the concentration of cytotoxic T lymphocyte | |||
(CTL) cells. | ||||
Λ | the production rate of the uninfected hepatocytes. | 4.0551 | day−1mm−3 | [46] |
σ | the death rate of hepatocytes. | 0.011 | day−1 | [42] |
u1 | the efficiency of drug therapy in blocking | 0.5 | - | assume |
new infection. | ||||
u2 | the efficiency of drug therapy in inhibiting | 0.5 | - | assume |
viral production. | ||||
β | the infection rate of uninfected hepatocytes | 0.0014 | mm3virion−1day−1 | [33] |
by the free virus. | ||||
e−mτ1 | the probaility of surviving of hepatocytes in | |||
the time period from t−τ1 to t | ||||
τ1 | the delay in the productively infected hepatocytes. | 5 | day | assume |
τ2 | the delay in an antigenic stimulation | 5 | day | assume |
generating CTL. | ||||
m | the constant rate of the death average of infected | 0.011 | day−1 | [18] |
hepatocytes which still not virus-producing cells. | ||||
q | the death rate of infected hepatocytes | 0.001 | mm3day−1 | [18] |
by the CTL response. | ||||
a | the production rate of intracellular HBV | 0.15 | day−1 | assume |
DNA-containing capsids. | ||||
α | the growth rate of virions in blood. | 0.0693 | day−1 | [29] |
δ | the clearance rate of intracellular HBV DNA-containing | 0.053 | day−1 | [19] |
capsids. | ||||
γ | the rate that viruses are neutralized by antibodies. | 0.01 | mm3day−1 | [18] |
μ | the death rate of free viruses. | 0.693 | day−1 | [42] |
g | the expansion rate of antibodies in | 0.008 | mm3virion−1day−1 | [57] |
response to free viruses. | ||||
h | the decay rate of antibodies. | 0.15 | day−1 | [18] |
k | the expansion rate of CTL in response to viral antigen | 0.001 | mm3day−1 | assume |
derived from infected hepatocytes. | ||||
ϵ | the decay rate of CTL in the absence of antigenic | 0.5 | day−1 | [58] |
stimulation. |
(i) when u1 varies and τ1=τ2=0
(ii) when u2 varies and τ1=τ2=0
(iii) when τ1 varies and τ2=5
(iv) when τ2 varies and τ1=5.
(i) when u1 varies and τ1=τ2=0.
Figure 5 (a)–(f) shows the dynamics of the concentration of the uninfected hepatocytes, infected hepatocytes, intracellular HBV DNA-containing capsid, free viruses, antibodies, and CTL, respectively where they are treated by u1 and u2 representing the efficiency of drug therapy in blocking new infection and the efficiency of drug therapy in inhibiting viral production, respectively. We choose u1 = 0.2, 0.4, 0.6 and u2 = 0.5. From Figure 5(a), we can see that a larger value of u1 can slow down the decline of the concentration of uninfected hepatocytes when compare with the smaller u1. At the end, they tend to reach the same equilibrium value. Figures 5(b) and 5(c) give a similar pattern, the concentration of infected hepatocytes and intracellular HBV DNA-containing capsids rises since the beginning for all values of u1. Figure 5(b) shows that the greater value of u1, the smaller the peak of the concentration of infected hepatocytes with a slightly slower time for the peak to occur. In the case when u1=0.2 and 0.4, it tends to give the second peak in the period of 80th to 150th day, whereas when u1=0.6 there is no second peak. Further, it reaches a lower equilibrium value when compared with a smaller u1. The difference between Figure 5(c) and Figure 5(b) is that the first peak of all three cases are at the same level. At the start in Figure 5(d), the concentration of free viruses decreases for a few days and goes up sharply to reach a peak. When u1 increases, the peak height is smaller, respectively with a slower time for the peak to occur and reaches the smaller equilibrium value. Further, for the case u1=0.2 and 0.4, the second peak is observed between 50th-150th day. Figure 5(e) shows interesting results i.e. there are two peaks of the concentration of antibodies when u1=0.2 and 0.4, where their second peak is larger than their first peak. Only one peak of the concentration of antibodies is obtained for u1=0.6. Time for the peak to occur is slightly slower when u1 increases. The dynamics tend to reach a lower equilibrium value with the larger value of u1. Interestingly, Figure 5(f) shows a significant reduction of the concentration of CTL and a slower time for the peak to occur when u1 increases. Further, in the case of u1=0.2, on the 100th day, the concentration of CTL rises again to reach a small peak ranging the period of 50 days then goes down to zero. Overall, from the results above u1 has been shown to play a main role in significantly reducing the concentration of infected hepatocytes, free viruses and CTL.
(ii) when u2 varies and τ1=τ2=0
In Figure 6, the value of u2 is varied by choosing u2=0.2,0.4,0.6 and u1=0.5. In Figure 6(a), our results show that with an increase of u2, the concentration of uninfected hepatocytes decreases slightly slower than the concentration of the smaller u2 and it tends towards the same equilibrium value at the end. Figure 6(b) demonstrates double peaks of the concentration of infected hepatocytes where the higher value of u2, the lower peak height for both peaks. It reaches a peak at 1000 cells/ml in the case u2=0.2, whereas it reaches a peak at less than 900 cells/ml for u2=0.6. After the first peak, they drop down to between 200-300 cells/ml and gradually rise up again as the second peak on approximately 100th day. Figure 6(c) gives a very interesting result i.e. with u2=0.2,0.4 and 0.6, the concentration of intracellular HBV DNA-containing capsids go up to reach the peak at 800 cells/ml, 600 cells/ml and 400cells/ml, respectively. Although when u2=0.2 and u2=0.4, it tends to give the second peak in the period of 100th to 150th day, with u2=0.6 there is no second peak. Further, with the larger value of u2, it tends to reach a lower equilibrium value. Figure 6(d) shows a significant decrease of the concentration of free viruses when u2 increases, and the time for the peak to occur is slightly slower. Figure 6(e) shows the concentration of antibodies increases from the beginning for all u2 values, there is a double peak for u2=0.2, it reaches the first peak at 400 cells/ml on the 45th day and slightly declines to 350 cells/ml then it rises up again to the higher second peak. At u2=0.4, the double peak is smaller than the case of u2 and than its first peak. With a higher value of u2, the concentration of antibodies decreases largely, respectively and tends to reach a lower equilibrium value. Figure 6(f) shows that when u2 increases, the concentration of CTL decreases significantly, and the time for the peak to occur is slightly slower, respectively. On the whole, from the results above u2 has been shown to play a main role in greatly reducing the concentration of intracellular HBV DNA-containing capsids, free viruses, antibodies and CTL.
(iii) when τ1 varies and τ2=5
In Figure 7, we vary the value of τ1 where τ2 is 5. From Figure 7 (a), we can see that the dynamics of concentration of uninfected hepatocytes hardly changed when τ1 varies. Figures 7(b) and 7(c) show a similar pattern, the concentration of infected hepatocyte and intracellular HBV DNA-containing capsids go up since the beginning for all values of τ1. They show that the higher the value of τ1, the smaller the peak and the longer it takes for the peak to appear. Further, it reaches a lower equilibrium value when compared with a smaller τ1. Figure 7(d) shows double peaks in the concentration of free viruses, the lower peak height for both peaks obtained with the larger value of τ1. They drop down after the first peak, then gradually rise to the second peak, which occurs between the 150th and 250th day. Finally, it tends to reach a lower equilibrium value when τ1 increases. Figures 7(e) and 7(f) show that in the case when τ1 increases, the concentration of antibodies and CTL decrease with a slower time for the peak to occur, respectively. In summary, the result above τ1 has shown to have an impact to a reduction in the concentration of infected hepatocytes, intracellular HBV DNA-containing capsids, free viruses, antibodies and CTL. Also, the epidemic peak occurs slower when τ1 increases. (iv) when τ2 varies and τ1=5.
When τ2 increases, the concentration of uninfected hepatocytes drops faster on the first 100th day, as shown in Figure 8(a). After that, however, the concentration of uninfected hepatocytes tends to decrease slower than in the case of smaller τ2. Figure 8(b) and 8(c) give a similar pattern when τ2 increases, the concentration of infected hepatocytes and intracellular HBV DNA-containing capsids largely increase, with a slower time for the peak to occur. Interestingly, with τ2=0.5, there are two peaks occurred, whereas only one peak observed in case τ2=5 and 15. Further, with τ2=15 it reaches a lower equilibrium value when compared to τ2=0.5, and 5. When τ2 increases, the concentration of free viruses increases to almost the same level of the peak as shown in Figure 8(d). However, it tends to give the second peak for case τ2=0.5 and 5, while in case τ2=15 there is only one peak. At the start in Figure 8(e), when τ2 increases, the concentration of antibodies significantly increases with a slower time for the peak to occur, with τ2=0.5, after the 70th day, it goes up again to the small second peak at a smaller level. On the other hand, Figure 8(f) shows a large reduction of the concentration of CTL with a slower time for the peak to occur, when τ2 increases. Further, in the case τ2=0.5, on the 80th day, it tends to rise to give the second peak ranging the period of 70 days then goes down to zero. On the whole, from the results above, τ2 has shown to give an impact in boosting up the concentration of infected hepatocytes, intracellular HBV DNA-containing capsids, free viruses, and antibodies with a longer period of an epidemic time. However, it shows to play a main role in greatly reducing the concentration of CTL. This means that the delay of antigenic stimulation generating CTL causes a longer duration with a large quantity of the hepatitis B virus infection.
In this paper, different from other existing models we propose multiple delays within-host model for HBV infection with 6 variables consisting of the uninfected hepatocytes, infected hepatocytes, intracellular HBV-DNA containing capsids, free viruses, antibodies, and cytotoxic T-lymphocyte (CTL). We incorporate the two delays which are the delay in the productively infected since viruses attack and an additional delay in an antigenic stimulation generating CTL. The model also involves two drug therapies. We have proved that all solutions are non-negative and bounded. Three equilibrium states are determined in this model i.e. infection-free, the immune-free and the immune-activated infection. The basic reproduction number is determined and becomes the threshold in determining the stability of the infection-free equilibrium point. Further, the global stability of immune-free and immune-activated infection equilibrium points are analyzed and presented in Theorem 8 and 9, respectively. Our numerical simulations have shown that both drug therapies play a key role in reducing an HBV infection overall. From Figure 7, we obtain that τ1 affects the time for the peak to occur i.e. it is slower when τ1 increases. Also, a smaller epidemic is observed in a larger value of τ1. In addition, the results of Figure 8 obtained, they show that the greater the delay in an antigenic stimulation generating CTL (τ2), the more severe HBV infection occurs. Our findings have confirmed the great role of both drug therapies in reducing HBV infection as shown in the work of Danane and Allali, 2018 [20]. However, the greater the delay in an antigenic stimulation generating CTL cells has been shown to make the HBV infection more severe, this can be found in the work of Sun and Liu, 2017 [46] that this time delay gives a big effect on the model dynamics. Overall, including both adaptive immune responses which are CTL and antibodies with time delays would make this model more realistic and this could bring better understanding of HBV infection. As a future work, it might be reasonable to include spatial components and diffusion for viruses into the model.
This work has been supported by the Department of Mathematics, Faculty of Science, Naresuan University, Thailand. Pensiri Yosyingyong has been funded by a DPST scholarship from the Thai government.
The authors declare there is no conflict of interest.
[1] |
R. M. Ribeiro, A. Lo, A. S. Perelson, Dynamics of hepatitis b virus infection, Microb. Infect., 4 (2002), 829–835, https://doi.org/10.1016/S1286-4579(02)01603-9 doi: 10.1016/S1286-4579(02)01603-9
![]() |
[2] | World Health Organization, Hepatitis B, 2020, Available from: http://www.who.int/mediacentre/factsheets/fs204/en |
[3] |
L. V. Tsui, L. G. Guidotti, T. Ishikawa, F. V. Chisari, Posttranscriptional clearance of hepatitis B virus RNA by cytotoxic t lymphocyte-activated hepatocytes, Proceed. Nat. Acad. Sci., 92 (1995), 12398–12402. https://doi.org/10.1073/pnas.92.26.12398 doi: 10.1073/pnas.92.26.12398
![]() |
[4] |
L. G. Guidotti, T. Ishikawa, M. V. Hobbs, B. Matzke, R. Schreiber, F. V. Chisari, Intracellular inactivation of the hepatitis B virus by cytotoxic t lymphocytes, Immunity, 4 (1996), 25–36. https://doi.org/10.1016/S1074-7613(00)80295-2 doi: 10.1016/S1074-7613(00)80295-2
![]() |
[5] |
L. G. Guidotti, F. V. Chisari, To kill or to cure: Options in host defense against viral infection, Current Opin. Immunol., 8 (1996), 478–483. https://doi.org/10.1016/S0952-7915(96)80034-3 doi: 10.1016/S0952-7915(96)80034-3
![]() |
[6] |
L. G. Guidotti, R. Rochford, J. Chung, M. Shapiro, R. Purcell, F. V. Chisari, Viral clearance without destruction of infected cells during acute hbv infection, Science, 284 (1999), 825–829. https://doi.org/10.1126/science.284.5415.825 doi: 10.1126/science.284.5415.825
![]() |
[7] |
M. Iannacone, G. Sitia, L. G. Guidotti, Pathogenetic and antiviral immune responses against hepatitis b virus, Future Virol., 1 (2006), 189–196. https://doi.org/10.2217/17460794.1.2.189 doi: 10.2217/17460794.1.2.189
![]() |
[8] | C. Long, H. Qi, S.-H. Huang, Mathematical modeling of cytotoxic lymphocyte-mediated immune response to hepatitis B virus infection, J. Biomed. Biotechnol., 2008. https: //doi.org/10.1155/2008/743690 |
[9] |
S. Phillips, S. Chokshi, A. Riva, A. Evans, R. Williams, N. V. Naoumov, Cd8+ T cell control of hepatitis B virus replication: Direct comparison between cytolytic and noncytolytic functions, J. Immunol., 184 (2010), 287–295. https://doi.org/10.4049/jimmunol.0902761 doi: 10.4049/jimmunol.0902761
![]() |
[10] |
F. V. Chisari, M. Isogawa, S. F. Wieland, Pathogenesis of hepatitis b virus infection, Pathol. Biol., 58 (2010), 258–266. https://doi.org/10.1016/j.patbio.2009.11.001 doi: 10.1016/j.patbio.2009.11.001
![]() |
[11] |
A. Busca, A. Kumar, Innate immune responses in hepatitis B virus (HBV) infection, Virol. J., 11 (2014), 1–8, https://doi.org/10.1186/1743-422X-11-22 doi: 10.1186/1743-422X-11-22
![]() |
[12] |
A. Elaiw, N. Alshamrani, Global analysis for a delay-distributed viral infection model with antibodies and general nonlinear incidence rate, J. Korean Soc. Indust. Appl. Math., 18 (2014), 317–335. https://doi.org/10.12941/jksiam.2014.18.317 doi: 10.12941/jksiam.2014.18.317
![]() |
[13] |
Y. Wang, Y. Zhou, J. Wu, J. Heffernan, Oscillatory viral dynamics in a delayed HIV pathogenesis model, Math. Biosci., 219 (2009), 104–112. https://doi.org/10.1016/j.mbs.2009.03.003 doi: 10.1016/j.mbs.2009.03.003
![]() |
[14] |
S. Wang, X. Song, Z. Ge, Dynamics analysis of a delayed viral infection model with immune impairment, Appl. Math. Model., 35 (2011), 4877–4885. https://doi.org/10.1016/j.apm.2011.03.043 doi: 10.1016/j.apm.2011.03.043
![]() |
[15] |
N. Bairagi, D. Adak, Global analysis of HIV-1 dynamics with hill type infection rate and intracellular delay, Appl. Math. Model., 38 (2014), 5047–5066. https://doi.org/10.1016/j.apm.2014.03.010 doi: 10.1016/j.apm.2014.03.010
![]() |
[16] |
B.-Z. Guo, L.-M. Cai, A note for the global stability of a delay differential equation of hepatitis B virus infection, Math. Biosci. Eng., 8 (2011), 689. https://doi.org/10.3934/mbe.2011.8.689 doi: 10.3934/mbe.2011.8.689
![]() |
[17] |
H. Song, W. Jiang, S. Liu, Virus dynamics model with intracellular delays and immune response, Math. Biosci. Eng., 12 (2015), 185. https://doi.org/10.3934/mbe.2015.12.185 doi: 10.3934/mbe.2015.12.185
![]() |
[18] |
A. Meskaf, K. Allali, Y. Tabit, Optimal control of a delayed hepatitis B viral infection model with cytotoxic T-lymphocyte and antibody responses, Int. J. Dynam. Control, 5 (2017), 893–902. https://doi.org/10.1007/s40435-016-0231-4 doi: 10.1007/s40435-016-0231-4
![]() |
[19] |
K. Manna, S. P. Chakrabarty, Global stability of one and two discrete delay models for chronic hepatitis B infection with HBV DNA-containing capsids, Comput. Appl. Math., 36 (2017), 525–536. https://doi.org/10.1007/s40314-015-0242-3 doi: 10.1007/s40314-015-0242-3
![]() |
[20] |
J. Danane, K. Allali, Mathematical analysis and treatment for a delayed hepatitis B viral infection model with the adaptive immune response and DNA-containing capsids, High-throughput, 7 (2018), 35. https://doi.org/10.3390/ht7040035 doi: 10.3390/ht7040035
![]() |
[21] |
A. Bertoletti, M. K. Maini, C. Ferrari, The host-pathogen interaction during HBV infection: Immunological controversies, Antiviral Therapy, 15 (2010), 15–24. https://doi.org/10.3851/IMP1620 doi: 10.3851/IMP1620
![]() |
[22] |
S. J. Hadziyannis, N. C. Tassopoulos, E. J. Heathcote, T.-T. Chang, G. Kitis, M. Rizzetto, et al., Adefovir dipivoxil for the treatment of hepatitis B e antigen–negative chronic hepatitis B, New England J. Med., 348 (2003), 800–807. https://doi.org/10.1056/NEJMoa021812 doi: 10.1056/NEJMoa021812
![]() |
[23] |
L. L. Stein, R. Loomba, Drug targets in hepatitis b virus infection, Infect. Disorders-Drug Targets (Formerly Current Drug Targets-Infectious Disorders), 9 (2009), 105–116. https://doi.org/10.2174/187152609787847677 doi: 10.2174/187152609787847677
![]() |
[24] |
S. Hagiwara, N. Nishida, M. Kudo, Antiviral therapy for chronic hepatitis B: Combination of nucleoside analogs and interferon, World J. Hepatol., 7 (2015), 2427. https://doi.org/10.4254/wjh.v7.i23.2427 doi: 10.4254/wjh.v7.i23.2427
![]() |
[25] |
F. van den Berg, S. W. Limani, N. Mnyandu, M. B. Maepa, A. Ely, P. Arbuthnot, Advances with rnai-based therapy for hepatitis b virus infection, Viruses, 12 (2020), 851. https://doi.org/10.3390/v12080851 doi: 10.3390/v12080851
![]() |
[26] | J. S. Nayagam, Z. C. Cargill, K. Agarwal, The role of rna interference in functional cure strategies for chronic hepatitis B, Current Hepatol. Rep., 1–8. https: //doi.org/10.1007/s11901-020-00548-4 |
[27] |
E. De Clercq, G. Férir, S. Kaptein, J. Neyts, Antiviral treatment of chronic hepatitis B virus (HBV) infections, Viruses, 2 (2010), 1279–1305. https://doi.org/10.3390/v2061279 doi: 10.3390/v2061279
![]() |
[28] |
G. K. Lau, T. Piratvisuth, K. X. Luo, P. Marcellin, S. Thongsawat, G. Cooksley, et al., Peginterferon alfa-2a, lamivudine, and the combination for hbeag-positive chronic hepatitis B, New England J. Med., 352 (2005), 2682–2695. https://doi.org/10.1056/NEJMoa043470 doi: 10.1056/NEJMoa043470
![]() |
[29] |
M. A. Nowak, S. Bonhoeffer, A. M. Hill, R. Boehme, H. C. Thomas, H. McDade, Viral dynamics in hepatitis b virus infection, Proceed. Nat. Acad. Sci., 93 (1996), 4398–4402. https://doi.org/10.1073/pnas.93.9.4398 doi: 10.1073/pnas.93.9.4398
![]() |
[30] |
A. Goyal, R. M. Ribeiro, A. S. Perelson, The role of infected cell proliferation in the clearance of acute HBV infection in humans, Viruses, 9 (2017), 350. https://doi.org/10.3390/v9110350 doi: 10.3390/v9110350
![]() |
[31] |
S. M. Ciupe, R. M. Ribeiro, P. W. Nelson, G. Dusheiko, A. S. Perelson, The role of cells refractory to productive infection in acute hepatitis b viral dynamics, Proceed. Nat. Acad. Sci., 104 (2007), 5050–5055. https://doi.org/10.1073/pnas.0603626104 doi: 10.1073/pnas.0603626104
![]() |
[32] |
S. M. Ciupe, R. M. Ribeiro, P. W. Nelson, A. S. Perelson, Modeling the mechanisms of acute hepatitis b virus infection, J. Theor. Biol., 247 (2007), 23–35. https://doi.org/10.1016/j.jtbi.2007.02.017 doi: 10.1016/j.jtbi.2007.02.017
![]() |
[33] |
S. Hews, S. Eikenberry, J. D. Nagy, Y. Kuang, Rich dynamics of a hepatitis b viral infection model with logistic hepatocyte growth, J. Math. Biol., 60 (2010), 573–590. https://doi.org/10.1007/s00285-009-0278-3 doi: 10.1007/s00285-009-0278-3
![]() |
[34] |
N. Yousfi, K. Hattaf, A. Tridane, Modeling the adaptive immune response in HBV infection, J. Math. Biol., 63 (2011), 933–957. https://doi.org/10.1007/s00285-010-0397-x doi: 10.1007/s00285-010-0397-x
![]() |
[35] |
A. M. Elaiw, N. Almuallem, Global properties of delayed-HIV dynamics models with differential drug efficacy in cocirculating target cells, Appl. Math. Comput., 265 (2015), 1067–1089. https://doi.org/10.1016/j.amc.2015.06.011 doi: 10.1016/j.amc.2015.06.011
![]() |
[36] |
K. Mboya, D. Makinde, E. Massawe, Cytotoxic cells and control strategies are effective in reducing the HBV infection through a mathematical modelling, Int. J. Prevent. Treatment, 4 (2015), 48–57. https://doi.org/10.5923/j.ijpt.20150403.02 doi: 10.5923/j.ijpt.20150403.02
![]() |
[37] | A. Tridane, K. Hattaf, R. Yafia, F. A. Rihan, Mathematical modeling of HBV with the antiviral therapy for the immunocompromised patients, Commun. Math. Biol. Neurosci., 2016 (2016). |
[38] |
P. Yosyingyong, R. Viriyapong, Global stability and optimal control for a hepatitis b virus infection model with immune response and drug therapy, J. Appl. Math. Comput., 60 (2019), 537–565. https://doi.org/10.1007/s12190-018-01226-x doi: 10.1007/s12190-018-01226-x
![]() |
[39] |
K. Manna, K. Hattaf, A generalized distributed delay model for hepatitis b virus infection with two modes of transmission and adaptive immunity: A mathematical study, Math. Methods Appl. Sci., 45 (2022), 11614–11634. https://doi.org/10.1002/mma.8470 doi: 10.1002/mma.8470
![]() |
[40] |
S. R. Lewin, R. M. Ribeiro, T. Walters, G. K. Lau, S. Bowden, S. Locarnini, et al., Analysis of hepatitis b viral load decline under potent therapy: Complex decay profiles observed, Hepatology, 34 (2001), 1012–1020. https://doi.org/10.1053/jhep.2001.28509 doi: 10.1053/jhep.2001.28509
![]() |
[41] |
S. Eikenberry, S. Hews, J. D. Nagy, Y. Kuang, The dynamics of a delay model of hepatitis b virus infection with logistic hepatocyte growth, Math. Biosci. Eng., 6 (2009), 283. https://doi.org/10.3934/mbe.2009.6.283 doi: 10.3934/mbe.2009.6.283
![]() |
[42] |
S. A. Gourley, Y. Kuang, J. D. Nagy, Dynamics of a delay differential equation model of hepatitis b virus infection, J. Biol. Dynam., 2 (2008), 140–153. https://doi.org/10.1080/17513750701769873 doi: 10.1080/17513750701769873
![]() |
[43] |
Q. Xie, D. Huang, S. Zhang, J. Cao, Analysis of a viral infection model with delayed immune response, Appl. Math. Model., 34 (2010), 2388–2395. https://doi.org/10.1016/j.apm.2009.11.005 doi: 10.1016/j.apm.2009.11.005
![]() |
[44] |
K. Wang, W. Wang, S. Song, Dynamics of an HBV model with diffusion and delay, J. Theor. Biol., 253 (2008), 36–44, https://doi.org/10.1016/j.jtbi.2007.11.007 doi: 10.1016/j.jtbi.2007.11.007
![]() |
[45] |
P. Fisicaro, C. Valdatta, C. Boni, M. Massari, C. Mori, A. Zerbini, et al., Early kinetics of innate and adaptive immune responses during hepatitis b virus infection, Gut, 58 (2009), 974–982. http://dx.doi.org/10.1136/gut.2008.163600 doi: 10.1136/gut.2008.163600
![]() |
[46] | D. Sun, F. Liu, Analysis of a new delayed HBV model with exposed state and immune response to infected cells and viruses, BioMed Res. Int., 2017. https: //doi.org/10.1155/2017/7805675 |
[47] |
K. Manna, S. P. Chakrabarty, Chronic hepatitis b infection and HBV DNA-containing capsids: Modeling and analysis, Commun. Nonlinear Sci. Numer. Simul., 22 (2015), 383–395. https://doi.org/10.1016/j.cnsns.2014.08.036 doi: 10.1016/j.cnsns.2014.08.036
![]() |
[48] |
T. Guo, H. Liu, C. Xu, F. Yan, Global stability of a diffusive and delayed HBV infection model with HBV DNA-containing capsids and general incidence rate, Discrete Continuous Dynam. Systems-B, 23 (2018), 4223. https://doi.org/10.3934/dcdsb.2018134 doi: 10.3934/dcdsb.2018134
![]() |
[49] |
M. Aniji, N. Kavitha, S. Balamuralitharan, Mathematical modeling of hepatitis b virus infection for antiviral therapy using lham, Adv. Differ. Equat., 2020 (2020), 1–19. https://doi.org/10.1186/s13662-020-02770-2 doi: 10.1186/s13662-020-02770-2
![]() |
[50] | K. Allali, A. Meskaf, A. Tridane, Mathematical modeling of the adaptive immune responses in the early stage of the HBV infection, Int. J. Differ. Equat., 2018. https: //doi.org/10.1155/2018/6710575 |
[51] | N. Chan Chí, E. AvilaVales, G. García Almeida, Analysis of a HBV model with diffusion and time delay, J. Appl. Math., 2012. https: //doi.org/10.1155/2012/578561 |
[52] |
K. Hattaf, N. Yousfi, A generalized HBV model with diffusion and two delays, Comput. Math. Appl., 69 (2015), 31–40. https://doi.org/10.1016/j.camwa.2014.11.010 doi: 10.1016/j.camwa.2014.11.010
![]() |
[53] | K. Hattaf, M. Rachik, S. Saadi, N. Yousfi, Optimal control of treatment in a basic virus infection model, Appl. Math. Sci., 3 (2009), 949–958. |
[54] |
K. Manna, S. P. Chakrabarty, Combination therapy of pegylated interferon and lamivudine and optimal controls for chronic hepatitis b infection, Int. J. Dynam. Control, 6 (2018), 354–368. https://doi.org/10.1007/s40435-017-0306-x doi: 10.1007/s40435-017-0306-x
![]() |
[55] |
K. Hattaf, A new generalized definition of fractional derivative with non-singular kernel, Computation, 8 (2020), 49. https://doi.org/10.3390/computation8020049 doi: 10.3390/computation8020049
![]() |
[56] |
K. Hattaf, On the stability and numerical scheme of fractional differential equations with application to biology, Computation, 10 (2022), 97. https://doi.org/10.3390/computation10060097 doi: 10.3390/computation10060097
![]() |
[57] |
S. M. Ciupe, R. M. Ribeiro, A. S. Perelson, Antibody responses during hepatitis b viral infection, PLoS Comput. Biol., 10 (2014), e1003730. https://doi.org/10.1371/journal.pcbi.1003730 doi: 10.1371/journal.pcbi.1003730
![]() |
[58] |
R. Ahmed, D. Gray, Immunological memory and protective immunity: Understanding their relation, Science, 272 (1996), 54–60. https://doi.org/10.1126/science.272.5258 doi: 10.1126/science.272.5258
![]() |
[59] | J. K. Hale, S. M. V. Lunel, Introduction to functional differential equations, vol. 99, Springer Science & Business Media, 2013. |
[60] |
P. Van den Driessche, J. Watmough, Reproduction numbers and sub-threshold endemic equilibria for compartmental models of disease transmission, Math. Biosci., 180 (2002), 29–48. https://doi.org/10.1016/S0025-5564(02)00108-6 doi: 10.1016/S0025-5564(02)00108-6
![]() |
1. | Severin Foko, Dynamical analysis of a general delayed HBV infection model with capsids and adaptive immune response in presence of exposed infected hepatocytes, 2024, 88, 0303-6812, 10.1007/s00285-024-02096-7 | |
2. | Bikash Modak, Muthu P, Stochastic approach to a delayed in-host model of DENV transmission, 2024, 99, 0031-8949, 075006, 10.1088/1402-4896/ad4ea6 | |
3. | Benito Chen-Charpentier, A Model of Hepatitis B Viral Dynamics with Delays, 2024, 4, 2673-9909, 182, 10.3390/appliedmath4010009 | |
4. | Chong Chen, Zhijian Ye, Yinggao Zhou, Zhoushun Zheng, Dynamics of a delayed cytokine-enhanced diffusive HIV model with a general incidence and CTL immune response, 2023, 138, 2190-5444, 10.1140/epjp/s13360-023-04734-3 | |
5. | B. Tamko Mbopda, S. Issa, R. Guiem, S. C. Oukouomi Noutchie, H. P. Ekobena, Travelling waves of a nonlinear reaction-diffusion model of the hepatitis B virus, 2023, 138, 2190-5444, 10.1140/epjp/s13360-023-04534-9 | |
6. | A.M. Elaiw, GH.S. Alsaadi, A.A. Raezah, A.D. Hobiny, Co-dynamics of hepatitis B and C viruses under the influence of CTL immunity, 2025, 119, 11100168, 285, 10.1016/j.aej.2025.01.029 | |
7. | Nabeela Anwar, Iftikhar Ahmad, Hijab Javaid, Adiqa Kausar Kiani, Muhammad Shoaib, Muhammad Asif Zahoor Raja, Dynamical analysis of hepatitis B virus through the stochastic and the deterministic model, 2025, 1025-5842, 1, 10.1080/10255842.2025.2470798 |
Parameter | Description | Value | Unit | Ref |
x | the concentration of uninfected hepatocytes. | |||
y | the concentration of infected hepatocytes. | |||
c | the concentration of intracellular HBV | |||
DNA-containing capsids. | ||||
v | the concentration of free viruses. | |||
w | the concentration of antibodies expansion | |||
in response to free viruses. | ||||
z | the concentration of cytotoxic T lymphocyte | |||
(CTL) cells. | ||||
Λ | the production rate of the uninfected hepatocytes. | 4.0551 | day−1mm−3 | [46] |
σ | the death rate of hepatocytes. | 0.011 | day−1 | [42] |
u1 | the efficiency of drug therapy in blocking | 0.5 | - | assume |
new infection. | ||||
u2 | the efficiency of drug therapy in inhibiting | 0.5 | - | assume |
viral production. | ||||
β | the infection rate of uninfected hepatocytes | 0.0014 | mm3virion−1day−1 | [33] |
by the free virus. | ||||
e−mτ1 | the probaility of surviving of hepatocytes in | |||
the time period from t−τ1 to t | ||||
τ1 | the delay in the productively infected hepatocytes. | 5 | day | assume |
τ2 | the delay in an antigenic stimulation | 5 | day | assume |
generating CTL. | ||||
m | the constant rate of the death average of infected | 0.011 | day−1 | [18] |
hepatocytes which still not virus-producing cells. | ||||
q | the death rate of infected hepatocytes | 0.001 | mm3day−1 | [18] |
by the CTL response. | ||||
a | the production rate of intracellular HBV | 0.15 | day−1 | assume |
DNA-containing capsids. | ||||
α | the growth rate of virions in blood. | 0.0693 | day−1 | [29] |
δ | the clearance rate of intracellular HBV DNA-containing | 0.053 | day−1 | [19] |
capsids. | ||||
γ | the rate that viruses are neutralized by antibodies. | 0.01 | mm3day−1 | [18] |
μ | the death rate of free viruses. | 0.693 | day−1 | [42] |
g | the expansion rate of antibodies in | 0.008 | mm3virion−1day−1 | [57] |
response to free viruses. | ||||
h | the decay rate of antibodies. | 0.15 | day−1 | [18] |
k | the expansion rate of CTL in response to viral antigen | 0.001 | mm3day−1 | assume |
derived from infected hepatocytes. | ||||
ϵ | the decay rate of CTL in the absence of antigenic | 0.5 | day−1 | [58] |
stimulation. |
Parameter | Description | Value | Unit | Ref |
x | the concentration of uninfected hepatocytes. | |||
y | the concentration of infected hepatocytes. | |||
c | the concentration of intracellular HBV | |||
DNA-containing capsids. | ||||
v | the concentration of free viruses. | |||
w | the concentration of antibodies expansion | |||
in response to free viruses. | ||||
z | the concentration of cytotoxic T lymphocyte | |||
(CTL) cells. | ||||
Λ | the production rate of the uninfected hepatocytes. | 4.0551 | day−1mm−3 | [46] |
σ | the death rate of hepatocytes. | 0.011 | day−1 | [42] |
u1 | the efficiency of drug therapy in blocking | 0.5 | - | assume |
new infection. | ||||
u2 | the efficiency of drug therapy in inhibiting | 0.5 | - | assume |
viral production. | ||||
β | the infection rate of uninfected hepatocytes | 0.0014 | mm3virion−1day−1 | [33] |
by the free virus. | ||||
e−mτ1 | the probaility of surviving of hepatocytes in | |||
the time period from t−τ1 to t | ||||
τ1 | the delay in the productively infected hepatocytes. | 5 | day | assume |
τ2 | the delay in an antigenic stimulation | 5 | day | assume |
generating CTL. | ||||
m | the constant rate of the death average of infected | 0.011 | day−1 | [18] |
hepatocytes which still not virus-producing cells. | ||||
q | the death rate of infected hepatocytes | 0.001 | mm3day−1 | [18] |
by the CTL response. | ||||
a | the production rate of intracellular HBV | 0.15 | day−1 | assume |
DNA-containing capsids. | ||||
α | the growth rate of virions in blood. | 0.0693 | day−1 | [29] |
δ | the clearance rate of intracellular HBV DNA-containing | 0.053 | day−1 | [19] |
capsids. | ||||
γ | the rate that viruses are neutralized by antibodies. | 0.01 | mm3day−1 | [18] |
μ | the death rate of free viruses. | 0.693 | day−1 | [42] |
g | the expansion rate of antibodies in | 0.008 | mm3virion−1day−1 | [57] |
response to free viruses. | ||||
h | the decay rate of antibodies. | 0.15 | day−1 | [18] |
k | the expansion rate of CTL in response to viral antigen | 0.001 | mm3day−1 | assume |
derived from infected hepatocytes. | ||||
ϵ | the decay rate of CTL in the absence of antigenic | 0.5 | day−1 | [58] |
stimulation. |