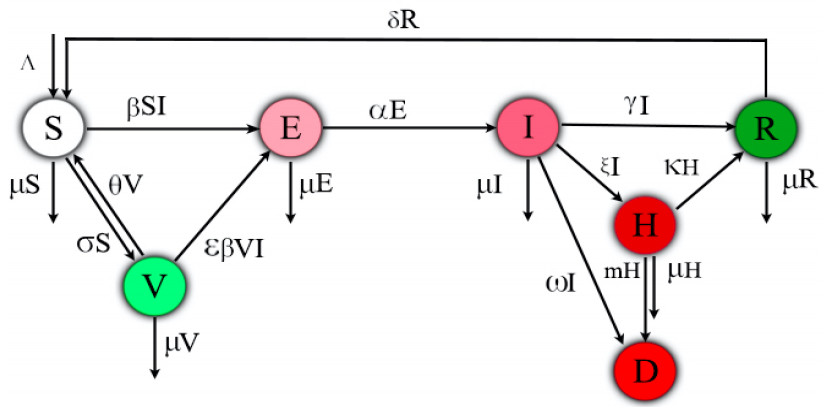
This paper is devoted to investigating the impact of vaccination on mitigating COVID-19 outbreaks. In this work, we propose a compartmental epidemic ordinary differential equation model, which extends the previous so-called SEIRD model [
Citation: Allison Fisher, Hainan Xu, Daihai He, Xueying Wang. Effects of vaccination on mitigating COVID-19 outbreaks: a conceptual modeling approach[J]. Mathematical Biosciences and Engineering, 2023, 20(3): 4816-4837. doi: 10.3934/mbe.2023223
[1] | Weike Zhou, Aili Wang, Fan Xia, Yanni Xiao, Sanyi Tang . Effects of media reporting on mitigating spread of COVID-19 in the early phase of the outbreak. Mathematical Biosciences and Engineering, 2020, 17(3): 2693-2707. doi: 10.3934/mbe.2020147 |
[2] | Pannathon Kreabkhontho, Watchara Teparos, Thitiya Theparod . Potential for eliminating COVID-19 in Thailand through third-dose vaccination: A modeling approach. Mathematical Biosciences and Engineering, 2024, 21(8): 6807-6828. doi: 10.3934/mbe.2024298 |
[3] | Fang Wang, Lianying Cao, Xiaoji Song . Mathematical modeling of mutated COVID-19 transmission with quarantine, isolation and vaccination. Mathematical Biosciences and Engineering, 2022, 19(8): 8035-8056. doi: 10.3934/mbe.2022376 |
[4] | Aili Wang, Xueying Zhang, Rong Yan, Duo Bai, Jingmin He . Evaluating the impact of multiple factors on the control of COVID-19 epidemic: A modelling analysis using India as a case study. Mathematical Biosciences and Engineering, 2023, 20(4): 6237-6272. doi: 10.3934/mbe.2023269 |
[5] | Xiaojing Wang, Yu Liang, Jiahui Li, Maoxing Liu . Modeling COVID-19 transmission dynamics incorporating media coverage and vaccination. Mathematical Biosciences and Engineering, 2023, 20(6): 10392-10403. doi: 10.3934/mbe.2023456 |
[6] | Biplab Dhar, Praveen Kumar Gupta, Mohammad Sajid . Solution of a dynamical memory effect COVID-19 infection system with leaky vaccination efficacy by non-singular kernel fractional derivatives. Mathematical Biosciences and Engineering, 2022, 19(5): 4341-4367. doi: 10.3934/mbe.2022201 |
[7] | Ahmed Alshehri, Saif Ullah . A numerical study of COVID-19 epidemic model with vaccination and diffusion. Mathematical Biosciences and Engineering, 2023, 20(3): 4643-4672. doi: 10.3934/mbe.2023215 |
[8] | Shina D. Oloniiju, Olumuyiwa Otegbeye, Absalom E. Ezugwu . Investigating the impact of vaccination and non-pharmaceutical measures in curbing COVID-19 spread: A South Africa perspective. Mathematical Biosciences and Engineering, 2022, 19(1): 1058-1077. doi: 10.3934/mbe.2022049 |
[9] | Yuto Omae, Yohei Kakimoto, Makoto Sasaki, Jun Toyotani, Kazuyuki Hara, Yasuhiro Gon, Hirotaka Takahashi . SIRVVD model-based verification of the effect of first and second doses of COVID-19/SARS-CoV-2 vaccination in Japan. Mathematical Biosciences and Engineering, 2022, 19(1): 1026-1040. doi: 10.3934/mbe.2022047 |
[10] | Yujie Sheng, Jing-An Cui, Songbai Guo . The modeling and analysis of the COVID-19 pandemic with vaccination and isolation: a case study of Italy. Mathematical Biosciences and Engineering, 2023, 20(3): 5966-5992. doi: 10.3934/mbe.2023258 |
This paper is devoted to investigating the impact of vaccination on mitigating COVID-19 outbreaks. In this work, we propose a compartmental epidemic ordinary differential equation model, which extends the previous so-called SEIRD model [
The pandemic of Coronavirus Disease 2019 (COVID-19) has given rise to an unprecedented public health crisis globally since the onset of the epidemic. As of June 9, 2022, over 533.7 million people in the world have been diagnosed with COVID-19 and more than 6.3 million have died. Thanks to the rapid progress in the development of the COVID vaccine. The vaccines are highly effective at preventing serious infection, hospitalization, and death from the disease. In many epidemic regions/countries, vaccination coverage rising has gradually reduced the economic and social disruptions caused by the pandemic. However, the progressively emerging SARS-CoV-2 variants, breakthrough infections and vaccine hesitancy have complicated the transmission and spread of COVID-19 infection, and posed new challenges for disease control. As a consequence, the developed COVID vaccines have raised important questions that remain unanswered or only partially answered regarding mitigating COVID-19 pandemic. For example, how effective vaccination coverage will be in the prevention and control of COVID-19 outbreaks and how vaccination coverage should be implemented in a region (e.g., a city, a state or a country) in short and long runs to protect the population there?
Many mathematical models have been published to study vaccination effects against the COVID-19 pandemic (see e.g., [5,6,7,8,9,10,11,12] and the references therein). For instance, Roy et al. [13] employed an SEIRD (susceptible-exposed-infected-recovered-dead) model to study the strategy of vaccination coverage in New York State. Saad-Roy et al. [6] applied a compartmental model of the SIR type to investigate a variety of scenarios of transmission and vaccine immunity. Amaral et al. [14] used a data-driven methodology (built upon an SIR-type model) to study the impact of vaccination rate against the COVID-19 in Brazil. The impulsive vaccination strategy was discussed by Etxeberria-Etxaniz et al. [15], whereas the mass vaccination was studied by Rachaniotis et al. [9] in Greece, Moghadas et al. [16] and Lin et al. [17] in the US and Shim [10] in South Korea. Goh et al. [18] proposed a country-specific model to compute the COVID-19 vaccination coverage needed to achieve herd immunity. Although the aforementioned works have provided valuable insights in disease prevention, most of these studies have been focused on the single or double doses of COVID-19 vaccine. In addition, none of these works have conducted a thorough mathematical analysis to study the transmission and spread of the disease with the inclusion of vaccination. This motivates our work on quantifying the impact of vaccination against COVID-19 outbreaks in a conceptual modeling study aiming to help us better understand the vaccination strategies in mitigating the disease.
The goal of this work is to predict the effectiveness of vaccination against the COVID-19 pandemic in short and long runs. To that end, we propose a compartmental model which extends a so-called SEIRD model with the inclusion of the vaccinated group, then we analyze the dynamics of this model under periodic vaccination programs and homogeneous disease transmission. Finally, to study the impact of vaccination, we fit our model to multiple COVID-19 waves in four locations including Hong Kong (SAR) China, Singapore, Japan, and South Korea as vaccine findings in reducing infection and mortality have shown to be spatially heterogeneous, and then forecast the prevalence of COVID-19 by the end of 2022 and in the future.
To study the impact of vaccination programs on the ongoing COVID-19 pandemic, we extend the previous susceptible-exposed-infectious-hospitalized-recovered-dead (SEIRD) model [1,2,3,4]. Our model is built upon the classical susceptible-exposed-infected-recovered (SEIR) compartmental framework by incorporating the birth and death of the population, disease-induced mortality and waning immunity induced by vaccine and infection, and adding a vaccinated compartment (V) to capture the vaccination. In the model, we divide the human population into seven compartments: susceptible (S), vaccinated (V), exposed (E), infected (I), hospitalized (H), disease-induced dead/removed (D) and recovered (R). Susceptible individuals can become exposed after contacting with infected people. Exposed individuals can progress to the infectious compartment after the latency period. Infectious individuals can move to the hospitalized compartment, which comes from either mild or severe cases. Infectious individuals can recover or be removed due to treatment or disease-induced death and then enter compartment D. Here V is the number of vaccinated individuals. We assumed that (i) susceptible individuals are vaccinated at time t at a rate σ(t), (ii) 100×ϵ% of vaccinated individuals can become infected; (iii) the vaccinated people can regain susceptibility and come back to the S compartment at the rate of θ due to the waning of vaccine-induced immunity. Besides, clinical and epidemiological evidence indicates that there are some individuals who never develop symptoms (see, e.g., [19,20] and references therein). However, there is no asymptomatic case data currently available. So, we synthesize asymptomatic and symptomatic transmission into an overall transmission route in our model. Our model, illustrated by a flow chart in Figure 1, can be written as:
dSdt=Λ−β(t)SI−(σ(t)+μ)S+θV+δRdVdt=σ(t)S−ϵβ(t)VI−(θ+μ)VdEdt=β(t)(S+ϵV)I−(α+μ)EdIdt=αE−(γ+ξ+ω+μ)IdHdt=ξI−(κ+m+μ)HdRdt=γI+κH−(μ+δ)RdDdt=ωI+mH. | (2.1) |
The definition of the model parameters is provided in Table 1. The parameter Λ represents the population influx, μ is the natural death rate of human individuals, α−1 is the latent period between exposure and infection, ω is the disease-induced death rate, γ (resp. κ) is the recovery rate from infection (resp. hospitalization), ξ is the rate of hospitalization, θ (resp. δ) is the rate of waning immunity induced by the vaccine (resp. infection), and δ is the removal rate of the virus from the environment. The function β(t) is the time-dependent transmission rate. The function σ(t) measures the rate at which susceptible individuals are vaccinated at time t. The vaccination is imperfect and it only has a degree of protection. Let ϵ=1−d∈(0,1), where d is the vaccine efficacy. Moreover, we assume that
Parameter | Description | Base value | Refs. |
Λ | recruitment rate of S | μN | Assumed |
θ | waning rate of vaccine-induced immunity | 365 d−1 | [21] |
δ | waning rate of infection-induced immunity | 182.5 d−1 | [22] |
ϵ | failure probability of vaccination | 0.1 | [23] |
μ | natural death rate | 0.00003424 d−1 | [24] |
1/α | latent period | 2 days | [25] |
γ | recovery rate of I | 0.3d−1 | [25] |
ξ | rate of hospitalization | 0.03267d−1 | [26] |
κ | recovery rate of H | 0.07917d−1 | [26] |
ω | disease induced death rate of I | 0.0006658 d−1 | [24] |
m | disease induced death rate of H | 0.004167 | [24] |
d | vaccine efficacy | 0.90 | [23] |
(H1) β(t) is a positive continuous function.
(H2) σ(t) is a nonnegative continuous function.
(H3) All parameters are positive.
To gain more analytical insight into our model, we conduct a mathematical analysis for a special case of this model (2.1) based on the following assumptions.
1) Transmission rate β(t) is assumed to be constant β.
2) Suppose that the vaccination program is provided on a regular basis, and we assume that σ(t) is T-periodic, where T is the vaccination period.
Then our original model (2.1) is reduced to a system of periodic ordinary differential equations.
First, we derive the basic reproduction number R0, a key indicator of the severity of the infection.
To study the disease-free steady state (DFS), we set E=I=H=R=D=0 and it leads to the following linear periodic system
dSdt=Λ−(σ(t)+μ)S+θVdVdt=σ(t)S−(θ+μ)V | (3.1) |
is cooperative and strongly subhomogeneous (see, e.g., [27, Section 2.3]). It follows from [27, Theorem 2.3.2] that there exists a unique T-periodic positive solution
(S∗(t),V∗(t)):=(Λ(θ+μ)μ(σ(t)+θ+μ),σ(t)Λμ(σ(t)+θ+μ)), |
which is globally attractive in R2.
By the theory of asymptotically periodic semiflows (see, e.g., [27, section 3.2] or [28]), it is easy to verify that when E(t)=I(t)=0,
limt→∞S(t)=S∗(t),limt→∞V(t)=V∗(t). | (3.2) |
Hence, the DFS of model (2.1) is given by E0:=(S∗(t),V∗(t),0,0,0,0,0,0). Linearizing model (2.1) at its DFS E0, we obtain the following periodic linear equations in terms of disease variables E and I:
dEdt=βN∗ϵ(t)I−(α+μ)EdIdt=αE−χI | (3.3) |
where
N∗ϵ(t)=S∗(t)+ϵV∗(t)=Λ(ϵσ(t)+θ+μ)μ(σ(t)+θ+μ),χ=γ+ξ+ω+μ. |
Define
F(t)=(0βN∗ϵ(t)00),V=(α+μ0−αχ) | (3.4) |
Since V is a constant matrix, the evolution operator, Y(t,s), t≥s, of the linear T-periodic model
dydt=−Vy | (3.5) |
is its fundamental matrix; i.e., for each s∈R, Y(t,s) satisfies
dY(t,s)dt=−VY(t,s),Y(s,s)=I |
where I is the 2×2 identity matrix. More specifically, if χ≠α+μ,
Y(t,s)=e−V(t−s)=(e−(α+μ)(t−s)0αχ−(α+μ)(e−(α+μ)(t−s)−e−χ(t−s))e−χ(t−s)); |
and if χ=α+μ (i.e., γ+ξ+ω+μ=α+μ which is equivalent to γ+ξ+ω=α),
Y(t,s)=e−V(t−s)=(e−χ(t−s)0α(t−s)e−χ(t−s)e−χ(t−s)). |
Let CT be the ordered Banach space of all T-periodic functions from R to R2, which is equipped with the maximum norm ‖⋅‖ and the positive cone C+T:={ψ∈CT:ψ(t)≥0, ∀ t∈R}.
Suppose that ψ(s)∈CT is the initial distribution of infected individuals. Then F(s)ψ(s) is the distribution of the new infections produced by the infected individuals who were introduced at time s. Given t≥s, then Y(t,s)F(s)ψ(s) gives the distribution of those infected individuals who were newly infected at time s and remain in the infected compartments at time t. It follows that
∫t−∞Y(t,s)F(s)ψ(s)ds=∫∞0Y(t,t−a)F(t−a)ψ(t−a)da |
gives the distribution of accumulative new infections at time t produced by all those infected individuals ψ(s) introduced at time previous to t. We define a linear operator L:CT→CT by
(Lϕ)(t)=∫∞0Y(t,t−a)F(t−a)ψ(t−a)da, ∀ t∈R, ϕ∈Cτ. | (3.6) |
which is referred to as the next generation operator. Motivated by the concept of next generation operators in [29,30,31,32,33], the basic reproduction number is defined as the spectral radius of L; that is, R0:=r(L).
In the special case where model (2.1) is autonomous, i.e., β(t)=β, σ(t)≡σ, N∗ϵ(t)≡Λ(ϵσ+θ+μ)μ(σ+θ+μ), the basic reproduction number is reduced to
R0=αα+μ⋅βγ+ξ+ω+μ⋅Λ(ϵσ+θ+μ)μ(σ+θ+μ). | (3.7) |
It is clear that R0 is a strictly decreasing function of the constant rate of vaccination σ as ϵ<1. This indicates that increasing vaccination rate could reduce the risk of infection.
In this subsection, we establish threshold dynamics of the disease in terms of the basic reproduction number R0.
Before proceeding, we need to review some basic results related to a monodromy matrix. Let M(t) be a continuous, cooperative, irreducible, and T-periodic m×m matrix function. Suppose ΦM(⋅)(t) is the monodromy matrix of the linear ordinary differential system
dx(t)dt=M(t)x, | (3.8) |
and r(ΦM(⋅)(T)) is the spectral radius of ΦM(⋅)(T). By [34, Lemma 2] or [35, Theorem 1.1]), it follows that ΦM(⋅)(t) is a matrix with all entries positive for each t>0. In view of the Perron-Frobenius theorem, r(ΦM(⋅)(T)) is the principal eigenvalue of ΦM(⋅)(T) in the sense that it is simple and admits a positive eigenvector.
By [32, Theorem 2.1], we have the following result.
Lemma 3.1.
1) If r(ΦF(⋅)μ−V(T))=1 has a positive solution μ=μ∗, then μ∗ is an eigenvalue of operator L, and R0>0.
2) If R0>0, then μ=R0 is the unique solution of r(ΦF(⋅)μ−V(T))=1.
3) R0=0 if and only if r(ΦF(⋅)μ−V(T))<1 for all μ>0.
It is usually difficult to compute R0 using the model (3.6). The part 2) of the above result, in particular, provides an alternative approach to numerically evaluating R0.
Moreover, by [32, Theorem 2.2], the following statement holds.
Lemma 3.2. ([32, Theorem 2.2])
R0<1(=1,>1) if and only if r(ΦF(⋅)−V(T))<1(=1,>1) |
Thus, the DFS E0(t) is locally asymptotically stable if R0<1, and unstable if R0>1.
The following result is an immediate consequence of [36, Lemma 2.1].
Lemma 3.3. ([36, Lemma 2.1]) Let λ=ln[r(ΦM(⋅)(T))]T. Then there exists a positive T-periodic function v(t) such that eλtv(t) is a solution of model (3.8).
Define
Fη(t)=(0β(N∗ϵ(t)+η)00). | (3.9) |
Recall that F and V are defined in model (3.4). Let
λη=1Tln[r(ΦFη(⋅)−V(T))]. | (3.10) |
If η=0, it is clear that F0=F and λ0=1Tln[r(ΦF(⋅)−V(T))].
Let X=R7+. Note that X is positively invariant for model (2.1). By [37, Theorem 5.2.1], for every x0=(S0,V0,E0,I0,H0,R0,D0)∈X, model (2.1) has a unique local nonnegative solution w(t,x0)=(S(t,x0),V(t,x0),E(t,x0),I(t,x0),H(t,x0),R(t,x0),D(t,x0))∈X for t≥0 with initial w(0,x0)=x0.
The following theorem establishes the global stability of the DFS E0 when R0<1.
Theorem 3.4. If R0<1, the DFS, E0, of model (2.1) is globally attractive; i.e.,
limt→∞(w(t,x0)−w∗(t))=0,∀ x0∈X, |
where w∗(t)=(S∗(t),V∗(t),0,0,0,0,0).
Proof. By R0<1, it follows from Lemma 3.2 that r(ΦF(⋅)−V(T))<1 and hence λ0<0. By continuity, there exists η0>0 such that
λη0<0. | (3.11) |
In view of the global attractivity of (S∗(t),V∗(t)) in model (3.1), there exists t0>0 such that
S(t)≤S∗(t)+η0/(1+ϵη0),V(t)≤V∗(t)+η0/(1+ϵη0),∀t≥t0. | (3.12) |
It follows from Lemma 3.3 that there exists a positive T-periodic solution vη0(t) such that ˉv(t)=ceλη0(t−t0)vη0(t) is a solution of model (3.8) with M(t)=Fη0(t)−V. Here constant c is chosen such that (E(t0),I(t0))≤ˉv(t0)=cvη0(t0).
In view of model (3.12),
dE(t)dt=β(S(t)+ϵV(t))I(t)−(α+μ)E(t)≤β(S∗(t)+ϵV∗(t)+η0)I(t)−(α+μ)E(t),=β(N∗ϵ(t)+η0)I(t)−(α+μ)E(t) | (3.13) |
for t≥t0. Thus
ddt(E(t)I(t))≤(Fη0(t)−V)(E(t)I(t)),∀ t≥t0. | (3.14) |
The comparison principle implies that
(E(t)I(t))≤ˉv(t)=keλη0tv(t),∀ t≥t0. | (3.15) |
Since λη0<0, it follows that
limt→∞(E(t),I(t))=(0,0). | (3.16) |
This implies that S(t) and V(t) in model (2.1) is asymptotic to model (3.1). By the theory of asymptotically periodic semiflows (see, e.g., [28] or [27, section 3.2]), it follows that limt→∞(S(t)−S∗(t))=limt→∞(V(t)−V∗(t))=0. Then H(t) is asymptotic to
dHdt=−(κ+m+μ)H |
and hence it follows from the theory of asymptotically periodic semiflows [28] that limt→∞H(t)=0.
Similarly one can verify that limt→∞R(t)=limt→∞H(t)=0. This completes the proof.
Let N(t)=S(t)+V(t)+E(t)+I(t)+H(t)+R(t) denote the total population size at time t. By model (2.1),
dNdt≤Λ−μN. | (3.17) |
It follows from the comparison principle that N(t) is ultimately bounded. By the positivity of the solution, we see that w(t) is ultimately bounded for w=S,V,E,I,H,R. Hence the solutions of model (2.1) admits a connected global attractor in X that attracts all positive orbits in X.
When R0>1, we assume that waning of infection-induced immunity is negligible, i.e., δ=0, for simplicity. In this case, the first four equations of model (2.1) are decoupled from the rest, and hence it suffices to consider the following system
dSdt=Λ−βSI−(σ(t)+μ)S+θVdVdt=σ(t)S−ϵβVI−(θ+μ)VdEdt=β(S+ϵV)I−(α+μ)EdIdt=αE−(γ+ξ+ω+μ)I | (3.18) |
Let Y=R4+. Let Q(t):Y→Y denote the solution map of model (3.18); i.e., for any z0∈Y,
Q(t)(z0)=u(t,z0) |
where u(t,z0) is the unique solution of model (3.18) with initial u(0,z0)=z0. Then ˆQ=Q(T) is the
Poincaré map associated with model (2.1) and
ˆQn(z0)=u(nT,z0),∀ z0∈Y,∀n∈N∪{0}. |
Moreover, {ˆQn:Y→Y}n≥0 defines a discrete-time dynamical system that admits a global attractor in Y. Let
Y0:={(S,V,E,I)∈Y:E≠0andI≠0}, |
and
∂Y0:=Y∖Y0={(S,V,E,I)∈Y:E≡0orI≡0}. |
If the waning of infection-induced immunity is neglected (i.e., δ=0), we have the following persistent result when R0>1.
Theorem 3.5. Suppose that δ=0 (i.e., the waning of infection-induced immunity is neglected). If R0>1, the solutions of model (3.18) are uniformly persistent; that is, there exists some constant c>0 such that any solution u(t,z0)=(S(t,z0),V(t,z0),E(t,z0),I(t,z0)) of model (3.18) with z0∈Y0 satisfies lim inft→∞E(t)≥c and lim inft→∞I(t)≥c, and model (3.18) admits at least one positive T-periodic solution.
Proof. Recall that the Poincaré map ˆQ:Y→Y associated with model (3.18) has a global attractor in Y. Now we proceed to prove that ˆQ is uniformly persistent with respect to (Y0,∂Y0). It is easy to verify that Y0 and ∂Y0 are positively invariant under the solution flow of model (3.18) satisfying Y0∪∂Y0=Y, Y0∩∂Y0=∅, and ∂Y0 is relatively closed in Y.
Since R0>1, it follows from Lemma 3.2 that r(ΦF(⋅)−V(T))>1. Thus,
λ0=1Tln[(ΦF(⋅)−V(T)]>0 |
and limη→0λη=λ0>0, where λη is defined in model (3.10). Hence, we can choose η1>0 sufficiently small such that λη1>0. By Lemma 3.3, model (3.18) has a positive T-periodic solution vη1(t) such that v_(t)=eλη1tvη1(t) is a solution of model (3.8) with M(t)=Fη1(t)−V.
In view of limϕ→E0‖Q(t)ϕ−Q(t)E0‖=0 uniformly for t∈[0,T], there exists ρ=ρ(η1)∈(0,η1) such that for any ϕ∈Y0 with ‖ϕ−E0‖<ρ, we have ‖Q(t)ϕ−Q(t)E0‖<η_:=η1/(1+ϵη1) for all t∈[0,T]. We further claim the following result.
Claim.
lim supn→∞‖ˆQnϕ−E0‖≥ρ,∀ϕ∈Y0. |
Suppose, by contradiction, that lim supn→∞‖ˆQnψ−E0‖<ρ for some ψ∈Y0. Then there exists N∈N such that ‖ˆQnψ−E0‖<ρ for all n≥N. For any t≥NT, there exist m≥N and t′∈[0,T) such that t=mT+t′, and hence ‖Q(t)ψ−Q(t)E0‖=‖Q(t′)(ˆQm(ψ))−Q(t′)E0‖<η_. It then follows that S(t)>S∗(t)−η_, V(t)>V∗(t)−η_, E(t)<η_ and I(t)<η_ for t≥NT, which implies that S(t)+ϵV(t)≥S∗(t)−η_+ϵ(V∗(t)−η_)</p><p>=N∗ϵ(t)−η1, E(t)<η1, and I(t)<η1 for t≥NT. Then we have
dE(t)dt=β(S(t)+ϵV(t))I(t)−(α+μ)E(t)≥β(N∗ϵ(t)−η1)I(t)−(α+μ)E(t),dI(t)dt=αE−dI, | (3.19) |
for t≥NT. Since ψ∈Y0, E(t,ψ)>0 and I(t,ψ)>0 for t≥0. Thus we can choose k>0 sufficiently small such that (E(NT,ψ),I(NT,ψ))T≥kv_(NT). It follows from the comparison principle that (E(t,ψ),I(t,ψ))T≥kv_(t)=keλη1tvη1(t) for t≥NT. Since λη1>0, limt→∞E(t,ψ)=limt→∞I(t,ψ)=∞, which contradicts the boundedness of the solutions of model (3.18). This proves the claim.
The result of this claim implies that E0 is an isolated invariant set for the Poincaré map ˆQ in Y0 and Ws(E0)∩Y0=∅, where Ws(E0) of the stable set of E0 with respect to ˆQ. Define
M∂={ϕ∈∂Y0:ˆQn(ϕ)∈∂Y0,∀n≥0}. |
For any given ψ∈M∂, ˆQn(ψ)∈∂Y0 for n≥0. Thus, for each n∈N, either E(nT,ψ)=0 or I(nT,ψ)=0. Using the last two equations in system model (3.18), we see that E(t,ψ)=0 or I(t,ψ)=0 for all t≥0. Case 1. Suppose I(t,ψ)≡0 for t≥0. By the third equation of model (3.18), dE/dt=−(α+μ)E for t≥0 and hence limt→∞E(t)=0. It follows from the theory of asymptotically periodic semiflows (see, e.g., [27, Section 3.2]) that limt→∞(S(t)−S∗(t))=limt→∞(V(t)−V∗(t))=0.
This implies that
ˆQn(ψ)→E0,asn→∞. | (3.20) |
Case 2. Suppose I(t,ψ)≢ for t\ge 0 . In this case, E(t, \psi)\equiv 0 for all t\ge 0 . Moreover, there exists t_0\ge 0 such that I(t_0, \psi) > 0 . By the fourth equation of model (3.18), I(t, \psi) > 0 for t\ge t_0 . Using the fourth equation of model (3.18), we see that \lim_{t\to\infty}I(t) = 0 . By the theory of asymptotically periodic semiflows (see, e.g., [27, Section 3.2]), it follows that \lim_{t\to\infty}(S(t)-S^*(t)) = \lim_{t\to\infty}(V(t)-V^*(t)) = 0 , which shows that model (3.20) holds. Therefore, every orbit in M_{\partial} approaches \mathcal{E}^0 as t\to\infty , and \mathcal{E}^0 is acyclic for \widehat{Q} in \partial \mathbb{Y}_0 . By the acyclicity theorem on uniform persistence for maps (see [27, Theorem 3.1.1]), it follows that \widehat{Q}: \mathbb{Y}\to\mathbb{Y} is uniformly persistent with respect to (\mathbb{Y}_0, \partial \mathbb{Y}_0) ; namely, there exists some constant c > 0 such that any solution u(t, \phi) = (S(t), V(t), E(t), I(t)) of model (3.18) with \phi\in \mathbb{Y}_0 satisfies \liminf_{t\to\infty}E(t)\ge c and \liminf_{t\to\infty}I(t)\ge c . Furthermore, by [27, Theorem 1.3.6], it follows that \widehat{Q} has a fixed point \hat{u}(0) = (\hat{S}(0), \hat{V}(0), \hat{E}(0), \hat{I}(0))\in \mathbb{Y}_0 . By the first equation of model (3.18), \hat{S}(t) = e^{-\int_0^tb(s_1)ds_1}\left[\hat{S}(0)+ \int_0^te^{\int_0^{s_2}b(s_1)ds_1}a(s_2)ds_2\right], where a(t) = \Lambda+\theta V(t)\ge \Lambda > 0 and b(t) = \beta I(t)+(\sigma(t)+\mu) , and hence \hat{S}(t) > 0 for all t > 0 . By the second equation of model (3.18), \hat{V}(t) = e^{-\int_0^td(s_1)ds_1}\left[\hat{V}(0)+ \int_0^te^{\int_0^{s_2}d(s_1)ds_1}g(s_2)ds_2\right], where g(t) = \sigma(t)S(t) > 0 and d(t) = {\epsilon} \beta I+\theta+\mu . Thus, \hat{V}(t) > 0 for all t > 0 . Additionally, \hat{E}(0) > 0, \ \hat{I}_I(0) > 0 . By [37, Theorem 4.1.1] as generalized to nonautonomous systems, the irreducibility of the cooperative matrix
\begin{eqnarray} \left(\begin{array}{ccc} -(\alpha+\mu) & \beta (S(t)+{\epsilon} V(t)) \\ \alpha & -d \end{array}\right) \end{eqnarray} | (3.21) |
implies that (\hat{E}(t), \hat{I}(t))\gg 0, \ \forall \ t > 0. We show that (\hat{S}(t), \hat{V}(t), \hat{E}(t), \hat{I}(t))\gg 0, \ \forall \ t > 0 which gives us is a positive T -periodic solution of the system. It completes the proof.
To illustrate the usage of the proposed model (2.1) in the evaluation of the long-term impact of vaccination, we perform a case study for COVID-19 epidemics, which is to show that (1) the model is appropriate that can be well fitted to multiple waves of COVID data and (2) this conceptional modeling approach can be useful for investigating the effects of vaccinations on this severe and long-lasting pandemic.
We now apply our model to study the COVID-19 epidemics in four locations, including Hong Kong (SAR) China, Singapore, Japan, and South Korea. We use the COVID-19 datasets published by the World Health Organization (WHO) and other sources [38,39] for the daily confirmed cases and daily vaccination data (fully vaccinated and third-dose vaccination) in each location. We convert daily cases to weekly data (since daily data includes weekday effects which we do not model). We assume a constant reporting ratio p , i.e., only p proportion infections are confirmed as cases (later we estimate p and the estimates fall in [0.3, 0.5] which is reasonable). Vaccination data are typically in the form per capita, namely the proportion of the population vaccinated, which cannot be directly incorporated into the model. We need the proportion of susceptible that are vaccinated daily. Susceptible individuals are among the group of unvaccinated. Given the daily vaccinated, we can estimate the proportion of the population that are unvaccinated. We convert vaccination (per capita) data to vaccination per unvaccinated [3,17,40]. Similarly, we convert vaccination daily (per capita) data to vaccination proportion per fully vaccinated, and move the proportion from V class to R class.
Following the previous works [3,4], we set the base parameter values as \alpha = 0.5 per day, \gamma = 0.3 per day, \xi = 0.03267 per day, \omega = 0.0006658 per day, \kappa = 0.07917 per day, m = 0.004167 per day, \mu = 0.00003424 per day, \sigma(t) = 0.3\% per day, \theta = 365 per day, \epsilon = 0.1 , \delta = 182.5 per day, and \Lambda = \mu N where N is the total population size of a location, where the references of these base parameter values are provided in Table 1. In data fitting, we use actual daily reported proportion of population received the second dose to fit \sigma(t) .
Additionally, due to the immune evasion of the Omicron variant, we assume \zeta proportion of recovered and \zeta proportion of vaccinated losing immunity protection and joining a susceptible pool, i.e., V\rightarrow (1-\zeta)V , R\rightarrow (1-\zeta)R and S\rightarrow S+\zeta(V+R) , when the Omicron variant evaded and dominated in a location. We compare four timings of this transition for each location: December 31, 2021, January 12, 2022, January 24, 2022, and February 5, 2022, for Hong Kong and South Korea, and November 24, 2021, December 6, 2021, December 18, 2021, and December 31, 2021, for Singapore and Japan to reflect the observation that the Omicron wave took off early in Singapore and Japan. We estimate \zeta and \beta . We assume that \beta is time-varying and modeled as an exponential cubic spline function with n_{\beta} nodes uniformly distributed over the entire period of the study. We assume \zeta in the range [0.3, 0.6] . Since the study period (January 23, 2020 – May 9, 2022) is relatively short, the population size N at each location is roughly constant and hence in the data fitting, we set \Lambda = \mu = 0 .
By employing the method of iterated filtering, we use our model to fit the multiple waves in each location in terms of weekly cases starting on January 23 2021. Our simulation is implemented with an R software package named partially observed Markov process (POMP) using maximum-likelihood-based iterated filtering technique [41]. A nice feature of our proposed model (2.1) is that it allows time-varying transmission rate, \beta(t) , which was set as an exponential cubic spline [42,43,44,45,46,47] to account for the simultaneous impact of all of the all-possible interventions. For more detailed information regarding the model fitting procedure, we refer the readers to Appendix A for an outline of the model fitting procedure, Appendix B for a pseudo code, and [43,48] for the theoretical basis and technique details of the general methodology and numerical implementation via examples.
Our model fitting is reasonably well, and the result is displayed in Figure 2. In top half of each panel, the green and blue curves show the daily data of fully vaccinated (second dose) coverage and booster (third dose) coverage. The brown curve shows the proportion of population that are temporally immunized via infection or vaccination. The drop in the brown curve shows the immune evasion, i.e., a proportion of immunized lose protection (moving to S ) due to the immune evasion ability of Omicron variant. In the bottom half, the red circles show the observed weekly cases, and the black curve (and gray region) shows the median (and 95% range) of 1,000 model simulation. The blue curve with cross shows the estimated transmission rate. We used n_{\beta} = 19 notes in the cubic spline and we fitted 120 weeks of data. After May 9, 2022, we forecast the trend of COVID-19 till the end of the year. We assume the \beta(t) will stable at the maximum value before May 9, 2022, after a transition, till the end of the year, while the second-dose coverage will reach 92% and the third-dose vaccination coverage will increase by 14% at the current level by the end of the year (2022). Our model framework sets a baseline model for the investigation of the long term trend.
In what follows, our investigation will be focused on the effects of vaccination against COVID-19 pandemic. We assume that the transmission rate \beta(t) is kept at the constant level after May 9, 2022, and its value is taken to be the estimated value of \beta(t) on that date (which is obtained from the data fitting). Before presenting the vaccination rate \sigma(t) , we need to introduce an auxiliary function. Let f(t) = \dfrac{\sigma_{max}}{1+e^{2(t-0.4T)}} for 0\le t\le T where \sigma_{max} is the maximal value of vaccination rate and T is the vaccination period. It is apparent that f(t) is a monotonically increasing function.
Let 0 < dt\ll T be given. We set \sigma(t) as follows.
\sigma(t) = \begin{cases} f(t),& 0\le t\le t_1,\\ \text{a line connecting}\; (t_1, f(t_1)) \;\text{ and}\; (T, f(0)) , & t_1 < t \le T, \end{cases} |
where t_1 = T-dt . The midnight blue curve in Figure 3(a) illustrates function \sigma(t) when vaccination coverage reaches C_{max} = 92\% by the end of T = 6 months, with dt = 10^{-4}T . In this case, \sigma_{max} is required to be 0.72\% per day to achieve 92\% coverage by the end of the vaccination period. One can see from these figures that vaccination rate \sigma(t) slowly increases in the beginning, then follows an exponential growth to approach its maximal value \sigma_{max} until t_1 , and finally linearly decay back to f(0) by the end of the period T . The red curve in Figure 3(a) plots the respective vaccination coverage as a function of time, which is a T -periodic increasing function and reaches its maximal value C_{max} at T . Figure 3(b) displays how \sigma_{max} is shaped by the period T with varied levels of vaccination coverage C_{max} . It shows that (a) when C_{max} is fixed, \sigma_{max} is a decreasing function of period T ; (b) when the value of T is fixed, \sigma_{max} is an increasing function of C_{max} . This indicates that (i) to achieve a certain level of vaccination coverage by the end of the period, the longer the vaccination period, the lower the maximal vaccination rate; (ii) on the other hand, if the vaccination period is kept constant, the higher the vaccination coverage, the more demanding requirement for the vaccination rate per day. For instance, if the final vaccination coverage is 60\% (i.e., C_{max} = 60\% ), \sigma_{max} = 0.73\% , 0.47\% , 0.24\% per day when T = 4, 6, and 12 months, respectively. whereas if vaccination coverage is 92\% (i.e., C_{max} = 92\% ), \sigma_{max} = 1.10\% , 0.73\% , 0.37\% per day when T = 4, 6, and 12 months, respectively.
Figure 4 illustrated the dynamics of model (2.1). Particularly, it shows that when \mathcal{R}_0 < 1 , the disease dies out; when \mathcal{R}_0 > 1 , the disease persists. This verifies our analytical results (Theorems 3.2 and 3.2) by numerical simulation.
To study the impact of vaccinations on the prevention and control of the disease, we compute the basic reproduction number \mathcal{R}_0 for these four locations as the vaccination period T increases. The value of \mathcal{R}_0 is numerically evaluated by using part 2 of Lemma 3.1. The result is plotted in Figure 5. For the displayed curves in each panel, the vaccination coverage is 60\%, 80\% and 92\% by the end of the vaccination period, respectively, from top to bottom. It shows that \mathcal{R}_0 is an increasing function of T . This indicates that the longer the vaccination period, the higher risk of infection we may have to expect. Furthermore, there appears to be a critical value of vaccination period T , denoted as T^c , such that \mathcal{R}_0 < 1, \mathcal{R}_0 = 1 , and \mathcal{R}_0 > 1 are equivalent to T < T^c, T = T^c , and T < T^c , respectively. In particular, our result predicts that T^c = 4.1 (resp. T^c = 5.1 , T^c = 5.8 ) months for Hong Kong when maximal vaccination coverage C_{max} can achieve 60\% (resp. 80\% , 92\% ). This indicates that if vaccination coverage is 60\% , the optimal vaccination period for Hong Kong is expected to be about 4.1 months, which indicates that if vaccination coverage will reach 60\% by the end of the vaccination period, the time between subsequent vaccinations should not be longer than 4.2 months for disease control in Hong Kong. Whereas if vaccination coverage is higher, then the optimal vaccination period may be longer. For instance, if C_{max} is 80\% or 90\% , the corresponding vaccination period T^c would expect to be 5.1 or 5.8 months. For Japan, T^c = 5.3 (resp. T^c = 6.7 , T^c = 7.5 ) months when C_{max} can achieve 60\% (resp. 80\% , 92\% ). In contrast, the optimal vaccination period T^c may have to be shortened for Singapore and South Korea. Especially, T^c = 3.1, 4.0 and 4.4 months for Singapore and T^c = 2.6, 3.1 and 3.5 months for South Korea, when C_{max} is 60\% , 80\% and 92\% , respectively. This indicates that the fourth-dose vaccination is very likely to be needed, particularly for the high-risk groups, this September (2022) in South Korea, October in Singapore and November in Hong Kong; whereas for Japan, the fourth dose may wait until early of next year (2023) if vaccination coverage can reach 92\% by the end of vaccination period.
The transmission and spread of COVID-19 involve complex biological and socioeconomic processes. The underlying mechanisms for COVID-19 outbreaks vary from place to place. In this work, we have attempted to use mathematical modeling and data fitting to investigate the multiple COVID-19 waves in four different locations (Hong Kong, Singapore, Japan and South Korea) in the East Asia and Southeast Asia, with an aim to better understand the impact of vaccinations on this severe and long-lasting epidemic of COVID-19.
If we assume that the basic reproduction number \mathcal{R}_0 will stable at the maximum value before May 9, 2022 after a transition, till the end of the year, while the second-dose coverage will reach 92% and the third-dose vaccination coverage will increase by 14% at the current level by the end of the year (2022), we can forecast the trend of the COVID-19 in these four locations. The trends are different across the four locations. Hong Kong will reach a low level of daily cases in summer and expect a bounce back at the end of the year. Japan will reach a low level at the end of the year, Singapore and South Korea will not reach a low level and see a bounce back at the end of the year. This indicates that a fourth dose among the high-risk group is probably needed by the end of the year. On the other hand, we numerically compute the basic reproduction number \mathcal{R}_0 , and our result shows that the longer of the vaccination period or the lower of the vaccination coverage, the higher risk of infection. More specifically, our findings indicate that the fourth-dose vaccination is very likely to be needed for the high-risk groups this September (of 2022) in South Korea, October in Singapore and November in Hong Kong; for Japan, the fourth dose may wait until early of next year (2023) provided that vaccination coverage can reach 92\% by the end of the vaccination period.
There are several limitations in our work. First, as it has been suggested by the clinical and epidemiological evidence from COVID-19 literature, the asymptomatic transmission may play an important role in the SARS-CoV-2 transmission. Since we modeled aggregated cases and the data for asymptomatic cases are currently not available, we synthesize asymptomatic and symptomatic transmission into an overall transmission route. For our present study, this simplification allows us to make the model analytically more trackable. We plan to investigate asymptomatic transmission and the related problems in our future study. Secondly, our mathematical epidemic model is a non-autonomous system, for which there is no general theory to define or compute the basic reproduction number. Thus, our analysis is focused on a special case where the model is a periodic system. Thirdly, more compartments could be added to the model for a representation of the different population sets and environmental components. For example, it would be interesting to introduce an age structure into the model to represent the fact that different age groups exhibit different disease risks, with the elderly being the most vulnerable. Fourthly, it may be more realistic to consider a multi-strain model to account for the new variants of SARS-CoV-2, particularly the Omicron variant that is contributing to a surge in cases throughout the world at present. Additionally, we assume that the transmission rate \beta is kept constant to forecast the trend of COVID-19 in the long term, which however is not quite realistic since disease transmission and spread are spatiotemporally heterogeneous in reality. Such complications haven't been explored in the current work, which will provide interesting topics for future research.
In conclusion, we proposed a simple SEIR type of epidemic model to study the effects of vaccination on mitigating COVID-19 outbreaks. We fit this model to reported COVID-19 cases in four locations (i.e., Hong Kong, Singapore, Japan and South Korea) and reconstructed the basic reproduction number \mathcal{R}_0 . We gained a qualitative idea of the long-term impact of vaccination. Our findings indicate that a fourth dose among high-risk group is likely needed by the end of the year. At the next step, we plan to associate the reconstructed \mathcal{R}_0 with government control measures and possible voluntary social distancing in each location.
DH acknowledges the support from the Research Grants Council of the Hong Kong Special Administrative Region, China (HKU C7123-20G).
The data used to support the findings of this study are available from the corresponding author upon request.
The authors declare that they have no known competing financial interests or personal relationships that could have appeared to influence the work reported in this paper.
To evaluate the long-term impact of vaccination, we fit our model (2.1) to the real COVID epidemic data in four locations: Hong Kong (SAR) China, Singapore, Japan, and South Korea. The time series of daily confirmed cases and daily deaths in each of these locations is modeled as a partially observed Markov process (POMP, also referred to as a hidden Markov process). The algorithm of iterated filtering [41,49] is utilized for the data fitting, implemented numerically in an R software package known as POMP via maximizing the log likelihood function.
In particular, model (2.1) has time-varying transmission rate \beta(t) . Following the previous studies (e.g., see [3,4,47] and the references therein), we define \beta(t) as an exponential cubic splines: \beta(t) = \exp(\text{cubic splines}), which has n_{\beta} nodes evenly spaced over the entire epidemic period T_s of the study. We simulate deaths weekly and fit it to the weekly reported mortality data. Let \Delta t be the reporting period, and \Delta t would be one week in this case. Based on the daily data, we simulate weekly deaths D_{t+\Delta t} as
D_{t+\Delta t} = \int_t^{t+\Delta t}m H(s) ds, |
where m is the disease induced death rate of hospitalized individuals (see Table 1), and H(s) is obtained by numerically solving model (2.1) for a given value of unknown parameters. This gives us a time-series death data \{D_1, D_2, \cdots, D_{T_s}\} . For each week t \; (1\le t\le T_s) , we denote Z_t to be the weekly reported deaths of that week. We assume that Z_t follows a negative binomial distribution, more specifically,
Z_{t}\sim {\text{NB}}(\text{mean} = D_{t} , \text{variance} = D_{t} (1+\tau D_{t}) . |
Here NB represents negative binomial distribution. \tau is an over-dispersion parameter which will be estimated. Basically parameter \tau is a measurement of noise due to surveillance and heterogeneity among individuals.
When \tau = 0 , the above negative binomial distribution reduces to a Poisson distribution. Let \Theta denote the vector of unknown parameters. Let D_{1:T_s} denote time-series data for deaths. Hence the likelihood of the week, \ell_t(\Theta): = \ell(\Theta|D_{1:T_s}, \tau), will be the probability of observing Z_t given D_{1:T_s} and \tau , which is a function of \Theta and can be directly calculated. The overall likelihood function is
{ L(\Theta) = \prod\limits_{t = 1}^{T_s}l_t(\Theta)}. |
Then the method of iterated filtering is used to estimate model parameter \Theta by maximizing the log likelihood function, \ln\Big(L(\Theta)\Big) .
For self completeness, a pseudo-code for modeling fitting procedure from [3] is included:
(1) Prepare the data.
(2) Set up the model and provide an initial guess for the values of model parameters to be fitted.
(3) Employ pfilter of the POMP package to calculate log likelihood of model given data.
(4) Apply mif function of the POMP package to obtain the maximum likelihood estimate of parameters.
(5) Repeat (3) and (4) until maximum log likelihood estimation converges.
(6) Use simulate function of the POMP package to perform the model simulation.
For a step-by-step instruction and hands-on examples on the methodology, we refer the readers to https://kingaa.github.io/sbied/.
[1] | H. Song, G. Fan, Y. Liu, X. Wang, D. He, The second wave of COVID-19 in South and Southeast Asia and the effects of vaccination, Front. Med., 8 (2021). https://doi.org/10.3389/fmed.2021.773110 |
[2] |
H. Song, G. Fan, S. Zhao, H. Li, Q. Huang, D. He, Forecast of the COVID-19 trend in India: a simple modelling approach, Math. Biosci. Eng., 8 (2021), 9775–9786. https://doi.org/10.3934/mbe.2021479 doi: 10.3934/mbe.2021479
![]() |
[3] | S. S. Musa, A. Tariq, L. Yuan, W. Haozhen, D. He, Infection fatality rate and infection attack rate of COVID-19 in South American countries, Infect. Dis. Poverty, 11 (2022). https://doi.org/10.1186/s40249-022-00961-5 |
[4] | S. S. Musa, X. Wang, S. Zhao, S. Li, N. Hussaini, W. Wang, et al., The heterogeneous severity of COVID-19 in African countries: a modeling approach, Bull. Math. Biol., 84 (2022), 1–16. Available from: https://link.springer.com/article/10.1007/s11538-022-00992-x. |
[5] |
G. B. Libotte, F. S. Lobato, G. M. Platt, A. J. S. Neto, Determination of an optimal control strategy for vaccine administration in COVID-19 pandemic treatment, Comput. Methods Programs Biomed., 196 (2020), 105664. https://doi.org/10.1016/j.cmpb.2020.105664 doi: 10.1016/j.cmpb.2020.105664
![]() |
[6] |
C. M. Saad-Roy, S. E. Morris, C. J. E. Metcalf, M. J. Mina, R. E. Baker, J. Farrar, et al., Epidemiological and evolutionary considerations of SARS-CoV-2 vaccine dosing regimes, Science, 372 (2021), 363–370. https://doi.org/10.1126/science.abg8663 doi: 10.1126/science.abg8663
![]() |
[7] |
M. Makhoul, H. H. Ayoub, H. Chemaitelly, S. Seedat, G. R. Mumtaz, S. Al-Omari, et al., Epidemiological impact of SARS-CoV-2 vaccination: mathematical modeling analyses, Vaccines, 8 (2020), 668. https://doi.org/10.3390/vaccines8040668 doi: 10.3390/vaccines8040668
![]() |
[8] | S. Berkane, I. Harizi, A. Tayebi, Modeling the effect of population-wide vaccination on the evolution of COVID-19 epidemic in Canada, medRxiv preprint, 2021. https://doi.org/10.1101/2021.02.05.21250572 |
[9] |
N. P. Rachaniotis, T. K. Dasaklis, F. Fotopoulos, P. Tinios, A two-phase stochastic dynamic model for COVID-19 mid-term policy recommendations in Greece: a pathway towards mass vaccination, Int. J. Environ. Res. Public Health, 18 (2021), 2497. https://doi.org/10.3390/ijerph18052497 doi: 10.3390/ijerph18052497
![]() |
[10] |
E. Shim, Optimal allocation of the limited COVID-19 vaccine supply in South Korea, J. Clin. Med., 10 (2021), 591. https://doi.org/10.3390/jcm10040591 doi: 10.3390/jcm10040591
![]() |
[11] |
E. Shim, Projecting the impact of SARS-CoV-2 variants and the vaccination program on the fourth wave of the COVID-19 pandemic in South Korea, Int. J. Environ. Res. Public Health, 18 (2021), 7578. https://doi.org/10.3390/ijerph18147578 doi: 10.3390/ijerph18147578
![]() |
[12] |
G. Webb, A COVID-19 epidemic model predicting the effectiveness of vaccination in the US, Infect. Dis. Rep., 13 (2021), 654–667. https://doi.org/10.3390/idr13030062 doi: 10.3390/idr13030062
![]() |
[13] |
S. Roy, R. Dutta, P. Ghosh, Optimal time-varying vaccine allocation amid pandemics with uncertain immunity ratios, IEEE Access, 9 (2021), 15110–15121. https://doi.org/10.1109/ACCESS.2021.3053268 doi: 10.1109/ACCESS.2021.3053268
![]() |
[14] |
F. Amaral, W. Casaca, C. M. Oishi, J. A. Cuminato, Simulating immunization campaigns and vaccine protection against COVID-19 pandemic in Brazil, IEEE Access, 9 (2021), 126011–126022. https://doi.org/10.1109/ACCESS.2021.3112036 doi: 10.1109/ACCESS.2021.3112036
![]() |
[15] |
M. Etxeberria-Etxaniz, S. Alonso-Quesada, M. De la Sen, On an SEIR epidemic model with vaccination of newborns and periodic impulsive vaccination with eventual on-line adapted vaccination strategies to the varying levels of the susceptible subpopulation, Appl. Sci., 10 (2020), 8296. https://doi.org/10.3390/app10228296 doi: 10.3390/app10228296
![]() |
[16] |
S. M. Moghadas, T. N. Vilches, K. Zhang, C. R. Wells, A. Shoukat, B. H. Singer, et al., The impact of vaccination on coronavirus disease 2019 (COVID-19) outbreaks in the United States, Clin. Infect. Dis., 73 (2021), 2257–2264. https://doi.org/10.1093/cid/ciab079 doi: 10.1093/cid/ciab079
![]() |
[17] |
L. Lin, Y. Zhao, B. Chen, D. He, Multiple COVID-19 waves and vaccination effectiveness in the United States, Int. J. Environ. Res. Public Health, 19 (2022), 2282. https://doi.org/10.3390/ijerph19042282 doi: 10.3390/ijerph19042282
![]() |
[18] |
F. T. Goh, Y. Z. Chew, C. C. Tam, C. F. Yung, H. Clapham, A country-specific model of COVID-19 vaccination coverage needed for herd immunity in adult only or population wide vaccination programme, Epidemics, 39 (2022), 100581. https://doi.org/10.1016/j.epidem.2022.100581 doi: 10.1016/j.epidem.2022.100581
![]() |
[19] | D. McEvoy, C. McAloon, A. Collins, K. Hunt, F. Butler, A. Byrne, et al., Relative infectiousness of asymptomatic SARS-CoV-2 infected persons compared with symptomatic individuals: a rapid scoping review, BMJ Open, 11 (2021), e042354. |
[20] |
B. K. Singh, J. Walker, P. Paul, S. Reddy, B. K. Gowler, J. Jernigan, et al., De-escalation of asymptomatic testing and potential of future COVID-19 outbreaks in US nursing homes amidst rising community vaccination coverage: a modeling study, Vaccine, 40 (2022), 3165–3173. https://doi.org/10.1016/j.vaccine.2022.04.040 doi: 10.1016/j.vaccine.2022.04.040
![]() |
[21] |
Y. Goldberg, M. Mandel, Y. M. Bar-On, O. Bodenheimer, L. Freedman, E. J. Haas, et al., Waning immunity after the BNT162b2 vaccine in Israel, N. Engl. J. Med., 385 (2021), e85. https://doi.org/10.1056/NEJMoa2114228 doi: 10.1056/NEJMoa2114228
![]() |
[22] |
W. Yang, J. Shaman, Development of a model-inference system for estimating epidemiological characteristics of SARS-CoV-2 variants of concern, Nat. Commun., 12 (2021), 1–9. https://doi.org/10.1038/s41467-021-25913-9 doi: 10.1038/s41467-021-25913-9
![]() |
[23] |
G. Fan, H. Song, S. Yip, T. Zhang, D. He, Impact of low vaccine coverage on the resurgence of COVID-19 in Central and Eastern Europe, One Health, 14 (2022), 100402. https://doi.org/10.1016/j.onehlt.2022.100402 doi: 10.1016/j.onehlt.2022.100402
![]() |
[24] |
J. S. Lavine, O. N. Bjornstad, R. Antia, Immunological characteristics govern the transition of COVID-19 to endemicity, Science, 371 (2021), 741–745. https://doi.org/10.1126/science.abe6522 doi: 10.1126/science.abe6522
![]() |
[25] |
X. Tang, S. S. Musa, S. Zhao, S. Mei, D. He, Using proper mean generation intervals in modeling of COVID-19, Front. Public Health, 9 (2021), 691262. https://doi.org/10.3389/fpubh.2021.691262 doi: 10.3389/fpubh.2021.691262
![]() |
[26] |
Y. Liu, K. Wang, L. Yang, D. He, Regional heterogeneity of in-hospital mortality of COVID-19 in Brazil, Infect. Dis. Modell., 7 (2022), 364–373. https://doi.org/10.1016/j.idm.2022.06.005 doi: 10.1016/j.idm.2022.06.005
![]() |
[27] | X. Q. Zhao, Dynamical Systems in Population Biology, 2^{nd} edition, Springer, Cham, 2017. |
[28] | X. Q. Zhao, Asymptotic behavior for asymptotically periodic semiflows with applications, 1996. |
[29] | N. Bacaër, S. Guernaoui, The epidemic threshold of vector-borne diseases with seasonality, J. Math. Biol., 53 (2006), 421–436. |
[30] |
J. M. Heffernan, R. J. Smith, L. M. Wahl, Perspectives on the basic reproductive ratio, J. R. Soc. Interface, 2 (2005), 281–293. https://doi.org/10.1098/rsif.2005.0042 doi: 10.1098/rsif.2005.0042
![]() |
[31] |
H. R. Thieme, Spectral bound and reproduction number for infinite-dimensional population structure and time heterogeneity, SIAM J. Appl. Math., 70 (2009), 188–211. https://doi.org/10.1137/080732870 doi: 10.1137/080732870
![]() |
[32] |
W. Wang, X. Q. Zhao, Threshold dynamics for compartmental epidemic models in periodic environments, J. Dyn. Differ. Equations, 20 (2008), 699–717. https://doi.org/10.1007/s10884-008-9111-8 doi: 10.1007/s10884-008-9111-8
![]() |
[33] |
X. Q. Zhao, Basic reproduction ratios for periodic compartmental models with time delay, J. Dyn. Differ. Equations, 29 (2017), 67–82. https://doi.org/10.1007/s10884-015-9425-2 doi: 10.1007/s10884-015-9425-2
![]() |
[34] |
G. Aronsson, R. B. Kellogg, On a differential equation arising from compartmental analysis, Math. Biosci., 38 (1978), 113–122. https://doi.org/10.1016/0025-5564(78)90021-4 doi: 10.1016/0025-5564(78)90021-4
![]() |
[35] |
M. W. Hirsch, Systems of differential equations that are competitive or cooperative II: convergence almost everywhere, SIAM J. Math. Anal., 16 (1985), 423–439. https://doi.org/10.1137/0516030 doi: 10.1137/0516030
![]() |
[36] |
F. Zhang, X. Q. Zhao, A periodic epidemic model in a patchy environment, J. Math. Anal. Appl., 325 (2007), 496–516. https://doi.org/10.1016/j.jmaa.2006.01.085 doi: 10.1016/j.jmaa.2006.01.085
![]() |
[37] | H. L. Smith, Monotone Dynamical Systems: an Introduction to the Theory of Competitive and Cooperative Systems, American Mathematical Society, 2008. |
[38] | E. Mathieu, H. Ritchie, E. Ortiz-Ospina, M. Roser, J. Hasell, C. Appel, et al., A global database of COVID-19 vaccinations, Nat. Hum. Behav., 5 (2021), 947–953. |
[39] | Owid, Dataset, 2022. Available from: https://covid.ourworldindata.org. |
[40] |
L. Lin, B. Chen, Y. Zhao, W. Wang, D. He, Two waves of COVID-19 in Brazilian cities and vaccination impact, Math. Biosci. Eng., 19 (2021), 4657–4671. http://dx.doi.org/10.2139/ssrn.3977464 doi: 10.2139/ssrn.3977464
![]() |
[41] |
E. L. Ionides, C. Breto, A. A. King, Inference for nonlinear dynamical systems, Proc. Natl. Acad. Sci. U.S.A., 103 (2006), 18438–18443. https://doi.org/10.1073/pnas.0603181103 doi: 10.1073/pnas.0603181103
![]() |
[42] |
S. S. Musa, S. Zhao, D. Gao, Q. Lin, G. Chowell, D. He, Mechanistic modelling of the large-scale Lassa fever epidemics in Nigeria from 2016 to 2019, J. Theor. Biol., 493 (2020), 110209. https://doi.org/10.1016/j.jtbi.2020.110209 doi: 10.1016/j.jtbi.2020.110209
![]() |
[43] | C. Breto, D. He, E. L. Ionides, A. A. King, Time series analysis via mechanistic models, Ann. Appl. Stat., 3 (2009), 319–348. Available from: https://www.jstor.org/stable/30244243. |
[44] | D. He, S. T. Ali, G. Fan, D. Gao, H. Song, Y. Lou, et al., Evaluation of effectiveness of global COVID-19 vaccination campaign, Emerging Infect. Dis., 28 (2022), 1873–1876. |
[45] |
D. He, S. Zhao, Q. Lin, S. S. Musa, L. Stone, New estimates of the Zika virus epidemic attack rate in Northeastern Brazil from 2015 to 2016: a modelling analysis based on Guillain-Barré Syndrome (GBS) surveillance data, PLoS Negl. Trop. Dis., 14 (2020), e0007502. https://doi.org/10.1371/journal.pntd.0007502 doi: 10.1371/journal.pntd.0007502
![]() |
[46] | L. Stone, D. He, S. Lehnstaedt, Y. Artzy-Randrup, Extraordinary curtailment of massive typhus epidemic in the Warsaw Ghetto, Sci. Adv., 6 (2020). https://doi.org/10.1126/sciadv.abc0927 |
[47] |
S. Zhao, L. Stone, D. Gao, D. He, Modelling the large-scale yellow fever outbreak in Luanda, Angola, and the impact of vaccination, PLoS Negl. Trop. Dis., 12 (2021), e0006158. https://doi.org/10.1371/journal.pntd.0006158 doi: 10.1371/journal.pntd.0006158
![]() |
[48] | Simulation-based inference for epidemiological dynamics. Available from: https://kingaa.github.io/sbied/ |
[49] | A. A. King, E. L. Ionides, M. Pascual, M. J. Bouma, Inapparent infections and cholera dynamics, Nature, 454 (2008), 877–880. |
1. | Farhad Waseel, George Streftaris, Bhuvendhraa Rudrusamy, Sarat C. Dass, Assessing the dynamics and impact of COVID-19 vaccination on disease spread: A data-driven approach, 2024, 9, 24680427, 527, 10.1016/j.idm.2024.02.010 |
Parameter | Description | Base value | Refs. |
\Lambda | recruitment rate of S | \mu N | Assumed |
\theta | waning rate of vaccine-induced immunity | 365 {\rm{d}}^{-1} | [21] |
\delta | waning rate of infection-induced immunity | 182.5 {\rm{d}}^{-1} | [22] |
\epsilon | failure probability of vaccination | 0.1 | [23] |
\mu | natural death rate | 0.00003424 {\rm{d}}^{-1} | [24] |
1/\alpha | latent period | 2 days | [25] |
\gamma | recovery rate of I | 0.3 \; {\rm{d}}^{-1} | [25] |
\xi | rate of hospitalization | 0.03267 \; {\rm{d}}^{-1} | [26] |
\kappa | recovery rate of H | 0.07917 \; {\rm{d}}^{-1} | [26] |
\omega | disease induced death rate of I | 0.0006658 {\rm{d}}^{-1} | [24] |
m | disease induced death rate of H | 0.004167 | [24] |
d | vaccine efficacy | 0.90 | [23] |
Parameter | Description | Base value | Refs. |
\Lambda | recruitment rate of S | \mu N | Assumed |
\theta | waning rate of vaccine-induced immunity | 365 {\rm{d}}^{-1} | [21] |
\delta | waning rate of infection-induced immunity | 182.5 {\rm{d}}^{-1} | [22] |
\epsilon | failure probability of vaccination | 0.1 | [23] |
\mu | natural death rate | 0.00003424 {\rm{d}}^{-1} | [24] |
1/\alpha | latent period | 2 days | [25] |
\gamma | recovery rate of I | 0.3 \; {\rm{d}}^{-1} | [25] |
\xi | rate of hospitalization | 0.03267 \; {\rm{d}}^{-1} | [26] |
\kappa | recovery rate of H | 0.07917 \; {\rm{d}}^{-1} | [26] |
\omega | disease induced death rate of I | 0.0006658 {\rm{d}}^{-1} | [24] |
m | disease induced death rate of H | 0.004167 | [24] |
d | vaccine efficacy | 0.90 | [23] |