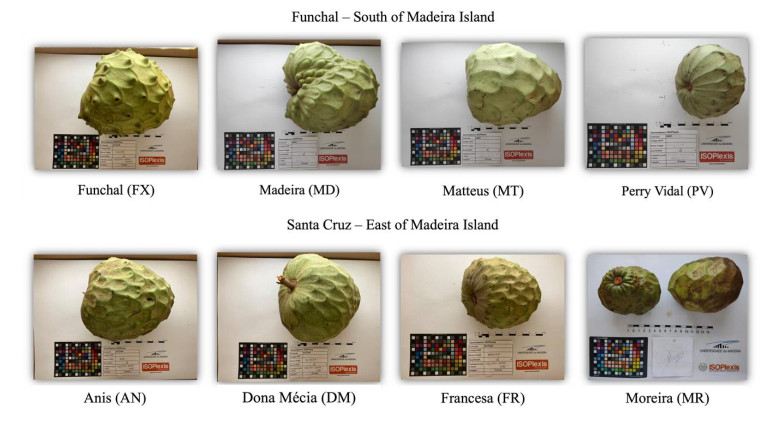
Blood cell image segmentation is an important part of the field of computer-aided diagnosis. However, due to the low contrast, large differences in cell morphology and the scarcity of labeled images, the segmentation performance of cells cannot meet the requirements of an actual diagnosis. To address the above limitations, we present a deep learning-based approach to study cell segmentation on pathological images. Specifically, the algorithm selects UNet++ as the backbone network to extract multi-scale features. Then, the skip connection is redesigned to improve the degradation problem and reduce the computational complexity. In addition, the atrous spatial pyramid pooling (ASSP) is introduced to obtain cell image information features from each layer through different receptive domains. Finally, the multi-sided output fusion (MSOF) strategy is utilized to fuse the features of different semantic levels, so as to improve the accuracy of target segmentation. Experimental results on blood cell images for segmentation and classification (BCISC) dataset show that the proposed method has significant improvement in Matthew's correlation coefficient (Mcc), Dice and Jaccard values, which are better than the classical semantic segmentation network.
Citation: Kun Lan, Jianzhen Cheng, Jinyun Jiang, Xiaoliang Jiang, Qile Zhang. Modified UNet++ with atrous spatial pyramid pooling for blood cell image segmentation[J]. Mathematical Biosciences and Engineering, 2023, 20(1): 1420-1433. doi: 10.3934/mbe.2023064
[1] | Nelson Mpumi, Revocatus L. Machunda, Kelvin M. Mtei, Patrick A. Ndakidemi . Insecticidal Efficacy of Syzygium aromaticum, Tephrosia vogelii and Croton dichogamus Extracts against Plutella xylostella and Trichoplusia ni on Brassica oleracea crop in Northern Tanzania. AIMS Agriculture and Food, 2021, 6(1): 185-202. doi: 10.3934/agrfood.2021012 |
[2] | María Cámara-Ruiz, José María García Beltrán, Francisco Antonio Guardiola, María Ángeles Esteban . In vitro and in vivo effects of purslane (Portulaca oleracea L.) on gilthead seabream (Sparus aurata L.). AIMS Agriculture and Food, 2020, 5(4): 799-824. doi: 10.3934/agrfood.2020.4.799 |
[3] | Tarik Ainane, Fatouma Mohamed Abdoul-Latif, Asmae Baghouz, Zineb El Montassir, Wissal Attahar, Ayoub Ainane, Angelo Maria Giuffrè . Correction: Essential oils rich in pulegone for insecticide purpose against legume bruchus species: Case of Ziziphora hispanica L. and Mentha pulegium L.. AIMS Agriculture and Food, 2023, 8(1): 236-238. doi: 10.3934/agrfood.2023013 |
[4] | Lenin O. Nevárez-Prado, Nubia Amaya-Olivas, Alejandro Sustaita-Rodriguez, Jesús Rodríguez-Zapién, Erick Zúñiga-Rodríguez, María Cordova-Lozoya, Antonio García-Triana, Fabiola Sandoval-Salas, León Hernández-Ochoa . Chemical composition and toxicity of extracts of Fouquieria splendens against Artemia salina. AIMS Agriculture and Food, 2022, 7(2): 357-369. doi: 10.3934/agrfood.2022023 |
[5] | The Han Nguyen, Patrick Achiever Blamo Jr, Xiaoyong Liu, Thi Van Anh Tran, Sang Moo Kim . β-Glucuronidase inhibitory activity of bromophenol isolated from red alga Grateloupia lancifolia. AIMS Agriculture and Food, 2021, 6(2): 551-559. doi: 10.3934/agrfood.2021032 |
[6] | Oksana B. Polivanova, Mikhail Yu. Cherednichenko, Elena A. Kalashnikova, Rima N. Kirakosyan . In vitro antibacterial effect of silver nanoparticles synthetized using Agastache foeniculum plant and callus extracts. AIMS Agriculture and Food, 2021, 6(2): 631-643. doi: 10.3934/agrfood.2021037 |
[7] | Gullo Gregorio, Dattola Antonio, Zappia Rocco . Comparative study of some fruit quality characteristics of two of Annona cherimola Mill. grown in southern Italy. AIMS Agriculture and Food, 2019, 4(3): 658-671. doi: 10.3934/agrfood.2019.3.658 |
[8] | Nicolas Nagahama, Bruno Gastaldi, Michael N. Clifford, María M. Manifesto, Renée H. Fortunato . The influence of environmental variations on the phenolic compound profiles and antioxidant activity of two medicinal Patagonian valerians (Valeriana carnosa Sm. and V. clarionifolia Phil.). AIMS Agriculture and Food, 2021, 6(1): 106-124. doi: 10.3934/agrfood.2021007 |
[9] | Patrick A. Blamo Jr, Hong Ngoc Thuy Pham, The Han Nguyen . Maximising phenolic compounds and antioxidant capacity from Laurencia intermedia using ultrasound-assisted extraction. AIMS Agriculture and Food, 2021, 6(1): 32-48. doi: 10.3934/agrfood.2021003 |
[10] | Saber Ibrahim, Amany Badr El-Deen Abd El-Aziz, Hanan Hassan Abdel-Khalek . Effect of γ irradiation on the antibacterial activity of poly lactic acid films encapsulated with essential oils against some common food borne pathogens. AIMS Agriculture and Food, 2020, 5(4): 715-733. doi: 10.3934/agrfood.2020.4.715 |
Blood cell image segmentation is an important part of the field of computer-aided diagnosis. However, due to the low contrast, large differences in cell morphology and the scarcity of labeled images, the segmentation performance of cells cannot meet the requirements of an actual diagnosis. To address the above limitations, we present a deep learning-based approach to study cell segmentation on pathological images. Specifically, the algorithm selects UNet++ as the backbone network to extract multi-scale features. Then, the skip connection is redesigned to improve the degradation problem and reduce the computational complexity. In addition, the atrous spatial pyramid pooling (ASSP) is introduced to obtain cell image information features from each layer through different receptive domains. Finally, the multi-sided output fusion (MSOF) strategy is utilized to fuse the features of different semantic levels, so as to improve the accuracy of target segmentation. Experimental results on blood cell images for segmentation and classification (BCISC) dataset show that the proposed method has significant improvement in Matthew's correlation coefficient (Mcc), Dice and Jaccard values, which are better than the classical semantic segmentation network.
The Annonaceae family is divided in 7 genera, and the genus Annona includes 2,400 species [1]. Custard apple is cultivated on tropical and subtropical areas around the globe for its exotic fruit with high nutritional value [2]. The semi-deciduous tree, slightly shrubby, can reach 9 m in height with alternate simple leaves shifting shape from elliptical to oval of variable size [1]. The hermaphrodite flowers are solitary in groups of 3 or 2 [3]. The fruit is typically conical or heart shaped with white succulent pulp, brown or black seeds, and a smooth peel [4]. Native to South America, custard apple was introduced to Europe in the mid-18th century [5] and has been cultivated in Madeira Island (Portugal) since the 19th century. Custard apple worldwide proliferation allowed the creation of cultivars with distinctive agronomical characteristics. A study showed that Madeira Island varieties form a homogenous group with less correlation to varieties found in other tropical areas, which indicates the existence of unique genetic pool with unique features [6]. The excellence, the differentiation and unique cultivation conditions of this fruit led to "Anona da Madeira" Protected Designation of Origin (PDO) registration by the European Union since 2000 (in agree with Regulation (EU) N.º 1187/2000) [7].
The food industry is a key sector and a leading producer of waste which negatively impacts global economy, environment and climate change [8]. Food waste cannot be prevented; however, new research and techniques can open a wide range of potential applications for biowaste and food by-products [9]. Annona fruits are consumed fresh for their pulp with sweet taste and health benefits, such as being rich in fiber, vitamins (B and C), antioxidants, iron, calcium, phosphorus, and potassium [10]), and there is a growing interest of establishing this species in Europe, but fruit processing and transportation represent a key issue to producer countries, due to its sensitivity and high moisture, leading to 75.8% post-harvest losses. During fruit consumption, it is estimated that 3–8.5% of waste is generated from seeds and 20% from peel [11]. Given its benefits and fruit underappreciation [12], studies including Annona cherimola are of high interest, generating new features and applications not only for its pulp but also its fruit waste. Different studies have been conducted with custard apple species which demonstrate its antifungal activity [13] due to its phytochemical composition and biological activity [14]. Synthetic chemicals are frequently used to prevent fungal infection. However, these antifungal factors carry undesired effects, such as environmental contamination, acquired pathogen resistance, and overall residual toxicity. Hence the need for bioproducts capable of controlling fungal threats with minimal consequences to the surroundings [13].
Fusarium oxysporum is a cosmopolitan soil pathogen found worldwide with specificity to more than 100 different hosts, including many plants of high agricultural interest [15]. The presence of this pathogen originates from an illness known as Fusarium wilt [16]. The host plant initiates the infection process by segregating biomolecules which act as signalers for F. oxysporum spore germination and consequent germ tube development. The germ tubes establish contact with the host tissues allowing the mycelial colonization leading to xylem invasion and restricting water flow. Ultimately, F. oxysporum excretes enzymes and toxins to aid infection alongside virulence factors that dictate pathogenesis [17]. The result is a scope of symptoms that limit plant growth, vascular discoloration, and seed decay [18]. Some Fusarium species produce secondary metabolites that contaminate plants and agricultural fields, decreasing crop yield and thereby impacting human health. Thus, F. oxysporum represents an ongoing threat to worldwide agriculture and crop yield [19]. Due to its pathogenicity, studies have been conducted to minimize plant impairment with the use of environmentally friendly biological agents which can constraint fungal growth.
Aspergillus niger is a common widespread fungus with high adaptability and tolerance to environmental changes [20]. Although is labelled with the GRAS status (Generally Recognized As Safe) from Food and Drug Administration of US government for certain industrial processes, A. niger is one of the most common fungi causing food spoilage [21]. This species can produce extracellular organic acids that enable them to cause decay of several cereals, nuts, beans, fruits, and vegetables [20,22]. Moreover, A. niger is associated to the production of two mycotoxins that are toxic for humans and animals, fumonisin B2 and ochratoxin A [23,24]. Contaminated post-harvest crops can show nutritional, sensorial and qualitative changes, like pigmentation, discoloration, deterioration, and development of off-odors and flavors that reduces its commercial value, thereby causing significant economic losses [20,21]. The produced mycotoxins can survive food production and processing leading to an infected by-product [25].
This study seeks to unfold the benefits of Annona cherimola variants in Madeira Island by showing their seed and peel bio-waste oil extracts antifungal action and further influence on agricultural research. These variants are not well known due to lack of research and this experiment intends to test their antifungal action against two pathogens, Fusarium oxysporum and Aspergillus niger. For data analysis, two measuring methods were used, calculated area (CA) and diameter average (DA), in order to analyze which one would provide more accurate growth ratios and consequently precise inhibition ratios (IR).
Eight A. cherimola varieties were used in this study, of which 4 varieties from Centro Experimental do Bom Sucesso (Funchal, South of Madeira Island), Funchal (FX), Madeira (MD), Matteus (MT), Perry Vidal (PV), and another 4 varieties from a local producer (Santa Cruz, East of Madeira Island), Anis (AN), Dona Mécia (DM), Francesa (FR), and Moreira (MR) (Figure 1).
The unripe fruits were collected between December 2020 and January 2021. The ripening process was done naturally on a bench. Once finished, the ripe fruit mass was registered, and the pulp, peel, and seeds were separated. Seed and peel samples were then dehydrated in a ventilated oven (Heratherm, Thermo-Scientific, Waltham, MA, USA) at 65 ℃ for 48 h and transformed into flour (IKA, Werke M20, Staufen, Germany). The resulting flour was stored in vacuum at −20 ℃ (Liebherr Profiline, Ochsenhausen, Germany) for oil extraction.
Ten grams of seed and peel flour of each variety were used for the oil extracted by Soxhlet method. Each extraction cycle lasted 8 h and was performed in triplicate, using 96% ethanol as solvent. The resulting extract was stored at 4 ℃ (Liebherr Profiline, Ochsenhausen, Germany) overnight. The remaining solvent was separated from the oil by roto-evaporation (Heidolph, Schwabach, Germany) at 40 ℃ and 60 rpm. The oil was poured in petri dishes and placed in a ventilated oven (Heratherm, Thermo-Scientific, Waltham, MA, USA) at 100 ℃ for 48 h to remove any excess solvent. Lastly, the final product was moved to a desiccator, then scrapped off and transferred to 10 mL flasks. The oils were stored at 4 ℃ for further application.
The strains selected for this antifungal assessment were Fusarium oxysporum (MT10) and Aspergillus niger (MT23) obtained from the fungal collection of ISOPlexis, Centre for Sustainable Agriculture and Food Technology. The mycelia preserved at −20 ℃ was transferred to 4 ℃ to acclimate, then cultivated on potato dextrose agar medium (PDA) (Liofilchem, Italy), and incubated in the dark at 25 ℃. Growth was observed throughout a week under natural conditions to guarantee colony viability. Confirmation of the affiliation of each fungal strain to the species was made through molecular techniques. Fungal DNA was isolated according to Yeates et al. [26], with adaptations. The PCR was performed using the primers ITS1 (5'-TCCGTAGGTGAACCTGCGG-3') and ITS4 (5-TCCTCCGCTTATTATTGATATGC-3') and the fragments were sequenced by the laboratories of STAB VIDA (Lisbon, Portugal). The sequences were analyzed using the GenBank database and the BLAST tool (See Appendix A).
The antifungal assessment involved closely monitoring duplicate replicas over the course of a week. Length (L) and width (W) measurements of Fusarium oxysporum (MT10) and Aspergillus niger (MT23) fungal mycelia were rigorously recorded on the third and sixth days, and the average diameter (DA) was accurately calculated using a ruler (Figure 2A). Furthermore, the precise calculated area (CA) was obtained using the program ImageJ (Figure 2B).
The colony development and morphology were meticulously captured for further in-depth examination. To conduct a rigorous preliminary examination, the treatments for the two fungi implied inoculating seven concentrations of oil extract originating from the skin and seed of the cherimoya varieties in study. The highest concentration, C7, was 100 mg of oil extract per milliliter, followed by C6 (80 mg/mL), C5 (60 mg/mL), C4 (40 mg/mL), C3 (20 mg/mL), C2 (15 mg/mL), and C1 (10 mg/mL). These concentrations were meticulously prepared by diluting C7 in 25% ethanol (EtOH) to a total volume of 1 mL. Three controls were included to this assay, as well as in duplicate replicas: an absolute control (PDA + fungus), a negative control (PDA + fungus + EtOH), and a positive control (PDA + fungus + Benomyl). Benomyl, a commercial systemic benzimidazole fungicide sold as Benlate (Be), was deliberately used for the positive control. The petri dishes were divided into 4 quadrants, each representing one individual concentration. Each treatment involved applying the selected oil extract concentration to a specific quadrant on PDA medium. The same rigorous technique was employed for the positive and negative controls using EtOH and Benomyl, respectively.
The measurements collected on the third and sixth days of the fungal mycelia development were analyzed following the procedure described by Hendricks et al. [27] and Guerrero-Álvarez and Giraldo-Rivera [31]. The duplicated L and W values using a ruler were used to calculate DA and transformed into the area of a circle [27] in cm2, using Equation 1:
DA=πr2=π(AverageDiameter2)² | (1) |
The fungal mycelia development CA, in cm2, were determined using ImageJ, an open-source image processing program for scientific image analysis, from pictures previously obtained on both days.
The values obtained from DA and CA were both converted separately to IR, with Equation 2:
IR=(AverageControlArea−AverageSampleArea)AverageControlArea | (2) |
The IR values for both methods were evaluated using principal component analysis (PCA) on MVSP and Spearman test to determine correlations on SPSS. Data variance was analyzed on SPSS using Kruskal-Wallis H test disclosed with Mann-Whitney U test, non-parametric statistical tests used to compare the statistical differences (p ≤ 0.05) among and within variables in study. The custard apple samples were sorted in two groups according to their local of harvest: South of Island for MD, FX, PV, and MT, and East of Island for MR, AN, DM, and FR, to test their oil concentration (C4–C7) against the F. oxysporum (MT10) and A. niger (MT23) fungal growth measured according to age (3- and 6-day old colonies).
All the IR values obtained were calculated always comparing treatment values with absolute control. The inoculation with higher oil concentrations of 100 mg/mL (C7), 80 mg/mL (C6), 60 mg/mL (C5), and 40 mg/mL (C4) had a positive effect in the IR mycelia growth. In contrast, the lower oil concentrations of 20 mg/mL (C3), 15 mg/mL (C2), and 10 mg/mL (C1) did not demonstrate any inhibitory effect on fungal mycelia growth. Therefore, we included in the results discussion the oil concentrations that have a minimum positive effect in the IR mycelia growth (C7–C4), excluding the lower concentrations (C3–C1).
There were no differences between absolute control (fungus) and negative control (fungus + EtOH). On the other hand, positive control (fungus + Benlate) greatly constrained F. oxysporum (MT10) growth reaching 100% inhibition, especially on younger colonies, when for A. niger (MT23) positive control IRs ranges from 83% to 56%.
Overall, the peel oil had a greater inhibition effect on F. oxysporum (MT10) than on A. niger (MT23), with higher IRs occurring always on the third day of the experience. Inhibition followed the same pattern, being higher on the third day for all concentrations and lower on the sixth day (Figure 3).
For F. oxysporum (MT10) colonies exposed to C7, the variants which had a bigger influence on growth were FX, PV, MT, and DM, reaching all values of 60% inhibition on the third day. DM, FX, FR, and MT extracts exhibited continuous action on MT10, until the third day. PV extract initially showed an inhibition action on fungal colony development, but after 6 days showed little control over the fungus. The PCA (Figure S1A) stated that after 3 days. The PCA eigenvalues for MT10 were 0.017 on Axis 1 and 0.002 on Axis 2, with a cumulative percentage of 81.016% and 92.796% respectively and the highest variable loading of 0.571 on Axis 1 for C7. The spatial distribution of cases shows that FX and MT differed from other varieties extracts with higher inhibition values on MT10, followed by PV, DM, and FR. The vectorial tendencies of C4 differed from C5, C6 and C7. Spearman's test displayed strong correlations, |r|≥0.5, between all concentrations. The correlation between C7 and C4 was lower, |r|=0.549(p≤0.01) and conversely, the similarities between C7/C6 |r|=0.838(p≤0.01) were higher. The inhibitory action was predominantly due to C7 and C6 on both days, while C5 of FR, MD, and PV extracts had a bigger impact on fungal mycelia development. A continuous inhibition was observed with MT, FX, DM, and FR extracts on the sixth day. Over time, higher concentrations (C7 and C6) controlled fungal growth better than lower concentrations (C5 and C4) apart for MT extract, which had stronger action on C4 and C5 even after 6 days. The peel samples of AN showed little effect on MT10 throughout the days.
The inhibitory action of oil extracts on A. niger (MT23) was lower than F. oxysporum (MT10), except for AN which reached 89% inhibition, following the same inhibition pattern on other concentrations and on 6 days old colonies (Figure 3). The FX and MD varieties displayed IRs of 50% on the third day, with lower inhibition on the sixth day (31%–14%). In the PCA (Figure S1B) shows the eigenvalues of 0.075 for Axis 1 and 0.005 for Axis 2, explaining a cumulative variation of 90.012% and 95.662% accordingly, and the highest variable loading of 0.566 on Axis 1 for C7. Most cases (cherimoya variant oil) were separated following the C7 vector. However, FX, MD and PV showed tendency close to the C4 vector separation. Once again, it was observed significant correlations between all concentrations, |r|≥0.6(p≤0.01). Contrary to MT10, there is no evident pattern between the different concentrations, and the highest correlation value was attained between C7 and C5 |r|=0.867(p≤0.01). AN extract overperformed when compared to other custard apple extracts, presenting high IRs, during the whole test and the best inhibition results on the third day. The PCA biplot supports the obtained graphic for A. niger (MT23) (Figure 3), showing FX and MD with the highest IRs after AN, and PV showing greater IR on the sixth day. In contrast, MT peel oil failed to inhibit MT23 growth on both days.
The Kruskal-Wallis test revealed significant differences between the peel oil from East and South groups (p≤0.05) for all concentrations and both fungi. On F. oxysporum (MT10), samples were all grouped according to age, being this the distinctive factor. For A. niger (MT23), samples were grouped only by age on C4. On C5, 6-day old South harvested custard apple samples showed significant changes when compared to other groups. On C6 and C7 there were no significant differences between all groups.
Unlike the peel, the seed oil performed similar against F. oxysporum (MT10) and A. niger (MT23), with a slight weaker inhibition on MT23. Again, the third day showed higher growth delay than the sixth day on every concentration and on both fungi (Figure 4).
For F. oxysporum (MT10) on the third day, FX dominated exhibiting IR values higher than 55% on every concentration, followed by PV, FR, and MR. On the sixth day, FX and MR extracts suppressed MT10 proliferation better than other varieties extracts. Both FX and MR showed similar IRs for all concentrations, while DM only showed high IR on C7, reaching 66% of inhibition. On the third day PV and FR surpassed MR, but inhibition decayed over time. The PCA biplot separated MT10 colonies in two clusters (Figure S2A) according to their age, with the third day demonstrating the best results once more. The PCA eigenvalues were 0.027 on Axis 1 and 0.003 on Axis 2, with cumulative percentages of 80.338% and 89.731% respectively, and with the highest variable loading being C7 with 0.537 on Axis 1. The tested concentrations showed different vectorial tendencies, where C7 had greater impact on DM during the third day, while other 3-day old colonies scattered according to C5 and C4. All concentrations showed significant correlations except for C5/C4 (p≥0.01). C6/C7 obtained the highest correlation coefficient |r|=0.744(p≤0.01). The case scores showed FX accomplished the best inhibition results on both days, followed by MR which had the second-best result for the sixth day. The remaining samples from 6-day old colonies agglomerated similarly, with a higher load on the variables C6 and C5.
Regarding A. niger (MT23) colonies, on 3-day old, AN obtained IR values of 26%, 50%, 72%, and 41% on C7, C6, C5, and C4, respectively, while MD established the highest IR value for C7, reaching 59% inhibition and over 40% inhibition on other concentrations. The MT23 PCA biplot (Figure S2B) pointed that C5 had a heavy influence on AN scattering on both days and C7 on MR on the 3rd day. The PCA eigenvalues for MT23 were 0.025 on Axis 1 and 0.007 on Axis 2 with cumulative percentages of 74.496% and 94.662%, respectively. The highest variable loading value was C5 with 0.651 on Axis 1. All correlations between concentrations were significant, with C4/C5, C4/C6, C5/C6 and C7/C6 presenting strong correlations at (p≤0.01), and C7/C4, C7/C5 displaying significant correlation values at (p≤0.05). The lowest correlation value was observed between C7 and C4 |r|=0.394(p≤0.05) and the highest between C4 and C6 |r|=0.702(p≤0.01). Apart from MR, AN and DM, all samples followed the C4/C6 vector tendencies on the third day. On the sixth day distribution was mainly affected by C6 and C4 vectors and with lower inhibition than third day IRs except for AN. MD also exceeded inhibition on the 3rd and 6th days. Contrary to MT10, MR seed oil performed poorly on MT23, specially on the sixth day.
The Kruskal-Wallis test affirmed the existence of significant differences between the seed oil from East and South groups (p≤0.05) for all concentrations on F. oxysporum (MT10) and A. niger (MT23). For MT10, substantial differences were observed between 3-day old and 6-day old groups, with a noticeable split in C7. On MT23, the grouping pattern was repeated except for C5, which was not able to disclaim any differences between the presented groups. Furthermore, C4 exhibited greater differences between East variants from the sixth day and South variants from the third day.
The peel IR from CA values for F. oxysporum (MT10) and A. niger (MT23) do not discriminate a higher inhibition tendency on any strain (Figure 5). Instead, similar to the peel DA inhibition ratios, the third day has highest IRs than the 6th day on both fungi, and C7 attained higher IRs than C4.
For F. oxysporum (MT10), MR and PV performed the best on every concentration on the 3rd day, reaching values of 60% and 57% inhibition on C7, respectively. Afterwards, MR and DM action were maintained while PV had reduced influence on growth, reaching low IRs on the sixth day. The PCA biplot for MT10 (Figure S3A) sorted samples according to their age, except for FX and MD. Each variable formed a distinct vector, with most samples scattering according to C7 vector. The PCA eigenvalues were 0.050 on Axis 1 and 0.009 on Axis 2 with cumulative percentages of 76.866% and 90.641%, respectively. The highest variable loading value was 0.526 on C6. The case scores showed MR high influence on MT10 colony growth on the third day and sixth day, performing the best when compared to other variants, followed by PV and DM. The PCA biplot supports data previously observed which showed PV scarce influence on 6th day growth while DM prevailed on both days. Both FX and MD exerted poor inhibitory action on MT10 throughout a week. All concentrations are strongly correlated, with C6/C5 presenting significant correlation at p≤0.05 and the remaining at p≤0.01. The strongest correlation was observed between C7 and C4 |r|=0.842(p≤0.01).
On A. niger (MT23), AN peel oil had the highest IRs on every concentration and age, reaching 100% inhibition on C6 after 3 days of development. Both PV and MR limited fungal expansion continuously, contrariwise after 6 days of growth MT and FX showed low impact on MT23 maturation. The PCA obtained for MT23 samples (Figure S3B), failed to form clear clusters, contrasting previous results, and suggesting a lower inhibitory action overall. The eigenvalues were 0.108 on Axis 1 and 0.007 on Axis 2 with cumulative percentages of 89.685% and 95.510%, respectively, the variable with the highest loading was C6 with 0.591 on Axis 1. Most samples dispersed according to C7 and C6, except for AN and FR which followed C4 and C5, respectively. The case scores scatter, confirmed AN high inhibitory activity on both days and MT poor action against colonies. The four variables are all strongly correlated once more, with C5/C4, C5/C6, C5/C7 at p≤0.05 and the remaining at p≤0.01. C5 exhibited lower correlation values with other concentrations |r|≤0.6, while C7, C6 and C4 obtained high correlation coefficients |r|≥0.85. The highest correlation value was observed between C6 and C4 |r|=0.913(p≤0.01).
The Kruskal-Wallis test of the peel inhibition ratios obtained from CA outcome diverged from previous results obtained for peel oil DA inhibition ratios. For F. oxysporum (MT10), only C7 displayed significant statistical differences (p≤0.05), with other variables not showing prominent differences between East and South cherimoya variants. C7 East variants on the third day attained significant differences from South variants and East variants on the sixth day. For A. niger (MT23), the same pattern was repeated, with only C5 attaining significant differences (p≤0.05).
The CA suggests that the seed oil performed similar on both fungi and IR was greater on the third day (Figure 6). MR and PV attenuated F. oxysporum (MT10) growth on all concentrations, with MR reaching 70% IR on C7 and C6 and over 60% inhibition on both C5 and C4. MR effect was prolonged throughout the 6 days of testing on MT10, along with DM and FR. Meanwhile, MD, FX and MT acted poorly on MT10 colonies, allowing the colonies to grow bigger. The MT10 PCA case scores (Figure S4A) distributed similarly, following C7, C5, and C4 mostly, except for DM and FX on the sixth day of growth, on which C6 had a higher impact. The eigenvalues obtained by MT10 samples were 0.096 for Axis 1 and 0.006 for Axis 2 with cumulative percentages of 88.560% and 94.129%, respectively. MR, PV, and AN, attenuated growth from the beginning, with MR prolonging its action throughout the week. DM maintained the inhibit action, initially with C7 restraining growth the most and after the 6 days C6 exhibited better action. On the contrary, MD, FX, and MT had low impact on fungal growth. C7 was the variable with the heaviest loading of 0.540 on Axis 1. All variables strongly correlated with each other, |r|≥0.8(p≤0.01). The strongest correlation was between C7 and C4 |r|=0.929(p≤0.01).
Again, AN performed the best against A. niger (MT23) growth achieving 100% inhibition on C5, with further 58% growth constrain on the sixth day. AN, FX and MR also limited colony expansion on every concentration, but FX lost effectiveness on the sixth day. The PCA biplot for MT23 (Figure S4B), resulted on centered case scores, with no evidence of clear clusters. The eigenvalues were 0.030 on Axis 1 and 0.010 on Axis 2, with cumulative percentages of 67.723% and 90.929%, respectively. The variable with the highest loading was C5 with 0.777 and FR placed along the C5 vector while other variants aggregated with the remaining concentrations. On the sixth day, FX clearly displayed the worst IRs, positioning it far apart from other samples. The variables C7, C6, and C4 were all correlated, with C4/C6, C4/C7 |r|≥0.7(p≤0.01), and C5/C4, C6/C7 |r|≥0.5(p≤0.05). C5 was only correlated with C4 |r|=0.510(p≤0.05) and the strongest correlation was C6/C4 |r|=0.714(p≤0.01).
Kruskal-Wallis test showed that there were no significant statistical differences between East and South variants on both fungi, except for A. niger (MT23) at seed oil C4 (p≤0.05).
The morphologies observed for some treatments and negative control can be observed in Figure 7.
Most F. oxysporum colonies (MT10) exposed to the oils developed phenotypical differences when compared to absolute control colonies. The absolute control colonies exhibited a pinkish white color and a cottony-like aerial mycelium. The center of the colonies had pink coloration with white hyphae colonizing a whole quadrant on the sixth day of growth. Meanwhile, MT10 colonies subjected to Annona oils presented an overall dark pink coloration and slower growth when compared to control. Both FX and MD caused MT10 colonies to develop an intense pink color, but the colonies grew as much as the control, taking over the whole plate. In most variants, the peel oil induced the development of stronger pigmentation on exposed colonies, except for AN. The colonies which interact with MR and PV oils, grew less when compared to the control and other variants but exhibited lighter coloration. However, on C7 it was possible to note a darker pigmentation than other concentrations, especially on MR seed oil.
A. niger colonies (MT23) presents yellow coloration on controls and treatments. The colonies exposed to oils grew less when compared to the negative control but overall, there were no significant morphological changes induced by the oils.
The Plant kingdom is a reliable source of diverse phytochemicals and bioactive elements with strong action against various pathogens. These bioactive substances have numerous botanical origins and chemical classifications, the most abundant of which are polyphenols [29]. It has been stated that Annona sp. incorporates various phytochemicals which are normally classified as flavonoids, terpenoids, alkaloids, and polyphenols [4,30]. Various studies have accomplished data that demonstrates its rich phytochemical composition and added antimicrobial action [2]. The following experiment reinforces this idea, showing that Annona cherimola Mill. variants found exclusively in Madeira Island stimulated a defense response on Fusarium oxysporum and Aspergillus niger. Interestingly, different variants originate different levels of inhibition on the tested fungi, suggesting phytochemical differences among them. Gentile et al. [30] conducted nutritional analysis on 7 different A. cherimola variants: Campas, Fino de Jete, Chaffey, White, Daniela, Torre I, and Torre II. All variants presented differences in nutritional, mineral, and vitamin contents, as well as total phenolic content (TPC) demonstrated changes between variants, proposing also heterogenous biological activity. Likewise, Puccio et al. [29] carried out phytochemical analysis on two variants, Fino de Jete and Torre I, concluding there were bioactive differences. AN peel demonstrated effective action against A. niger ranging from 56% to 100% inhibition on both used fungal growth measuring methods, CA and DA, and performing better than the commercial fungicide which inhibited growth up to 86%. Comparing to other variants, this suggests that AN composition might differ resulting in inhibition against this specific fungus [32]. Data related to fungal radial growth was analyzed with two different methods previously described [28,33]. On both methods, sensitivity was observed on the two fungi, with greater results on F. oxysporum (MT10). The attained IRs revealed that the oils had a heavier impact on 3-day old colonies than 6-day old colonies, an effect that was previously observed in another study [33]. This outcome can be related to the fast adaptative response characteristic of these fungal strains and efficient stress-induced defense mechanisms [34,35]. Overall, both seed and peel oils caused similar growth limitations with different levels of inhibition depending on concentration and variant. Although there were no significant differences between all tested concentrations, since all of them were correlated to each other, it was possible to observe an inhibition pattern, where the higher the concentration, the greater the inhibition. This phenomenon was equally observed by Méndez-Chávez et al. [13], opening room for further research, testing higher concentrations in order to reach total inhibition.
When comparing both growth measuring methods, some variants presented incongruent results: the estimated DA areas showed a great inhibitory action while CA method contradicted this fact or vice-versa (Figures 4, 6). CA however has a higher sensitivity to small changes in colony morphology and growth than DA areas. Hendricks et al. [27] tested these two methods on Phyllosticta critricarpa and also observed significant differences between DA and CA values, especially at lower fungicide concentrations: CA was more sensitive to growth changes than DA. Radial growth estimations should be performed in fungal colonies which have an equal superficial rate growth in all directions, describing a circular shape. This condition can be applied to F. oxysporum and A. niger. Nevertheless, the results attained in this study confirm that both methods successfully showed growth differences between control colonies and colonies exposed to the different oils, but CA has a greater perceptiveness to slight changes which are imperceptible to an estimated area.
The study exposed the bioaction diversity present in these variants when fighting different fungi. MR peel and seed oil performed greatly against F. oxysporum (MT10), along with DM and PV and curiously, some variants presented prolongated control over colony growth (MR and DM), while others constrained early growth only (PV) (Figures 5, 6). For A. niger (MT23), most variants revealed little control over colony expansion apart from AN, which reached 100% inhibition. A. cherimola Mill. antifungal action has been also described by Méndez-Chávez et al. [13], using leaf extracts against F. oxysporum. The extract limited colony growth on all tested concentrations, ranging from 49% to 100% inhibition. The statistical results on this study were supported by morphological differences observed on the colonies (Figure 7). According to previous research, F. oxysporum negative control colonies produced a phenotype expected under normal conditions [36]. The F. oxysporum (MT10) colonies cultured in the presence of A. cherimola oils developed a different morphology: most of the colonies presented a much darker coloration especially when in direct contact with the oil. This occurrence can be described as phenotypic plasticity, a short-time response to environmental changes [37] and it has been shown that the Fusarium genus produce different pigments according to growth conditions [38]. The different variants promoted morphological change, for instance, although MD and FX colonies grew as much as absolute and negative controls, they produced stronger pigmentation than other oils. These pigmentation changes could be related to an alteration of spores development [39] and it has been stated that darker color in F. oxysporum equals reduced spore production resulting in reduced virulence. This discover could be a promising solution for food contamination and storage or soil health. The controlled virulence equals less spore production and reduced reproductive action. Although colony development was not fully inhibited as observed with Benlate, MR and PV led to lighter pink colorations on F. oxysporum (MT10) colonies and restricted growth originating smaller colonies when compared to negative control, which could suggest a lack of defensive response from the fungi due to oil toxicity (Figure 7). Seemingly this indicates that the oils did in fact trigger distinctive responses in F. oxysporum (MT10) which could be justified with the possible phytochemical heterogenicity present in Madeira variants. On the other hand, there were no significant morphological differences between the absolute control of A. niger (MT23) and the tested colonies apart from size.
Furthermore, Annona sp. properties have been investigated by several authors which demonstrated both its antifungal and antibacterial action. Abdul-Hammed et al. [39] tested the inhibitory influence of 131 phytochemicals isolated from Annona muricata against Candida albicans. The results showed that some Annona flavonoids and alkaloids achieved better inhibition against the tested pathogens than fluconazole and standard voriconazole, medicine used to treat fungal infections. Rabêlo et al. [14] achieved antimicrobial activity using leaf and stem extracts of Annona atemoya. The study concluded that Gram-positive bacteria exhibited a stronger reaction to the oil, suggesting a possible interaction with the peptidoglycans present in the cell membrane. Additionally, three different solvents were tested for oil extraction (methanol, ethanol, and hexanol), resulting in different levels of inhibition with only ethanol and methanol-based extracts inducing microbial sensitivity. The outcome was justified with the high concentration of flavonoids present in the ethanol and methanol substrates due to their molecular polarity, while the hexanol solvent extracted mostly terpenoids. However, Abdel-Rahman et al. [40] demonstrated that terpenoids extracted from Annona seeds have an inhibitory effect against diverse bacterial strains; the resulting extracts constrained P. aeruginosa proliferation greatly and controlled Escherichia coli and Staphylococcus aureus growth. Guerrero-Álvarez & Giraldo-Rivera [31] reported how different Annona species behave against selected bacteria. The results showed that A. cherimola exhibited action against E. faecalis and A. montana, while A. glabra and A. reticulata originated a response on S. aureus. The study concluded that this antimicrobial property could be associated to saponins which interact with the cell membrane present in Gram-positive bacteria. Once more, it was showed that the extracts obtained from different species had phytochemical differences which expressed distinctive bioaction.
The Anonanaceae family possesses exclusive substances of high interest that have been described previously by multiple studies [41,42]. Annonaceae acetogenins (ACGs) derive from long-chain fatty acids and are formed by hydroxyl groups, one to three tetrahydrofuran (THF) rings and 35 to 37 carbons attached to a terminal γ-lactone. ACGs can be found on different parts of Annona plants, including peel and seed which are not consumed and represent a considerable percentage of the fruit. Therefore, the biowaste has significant interest due to ACGs strong antimicrobial and antitumoral properties and studies have been carried with the intent of optimizing its extraction and further applications [43]. Research has unveiled the main mechanisms of action involved in ACGs activity. These metabolites compromise aerobic respiration by inhibiting ubiquinone oxidoreductase NADH, thus constraining the mitochondrial respiratory complex I. ACGs also interact with plasma enzymes responsible for the production of ATP under anoxic conditions, and histones interfering in gene regulation. Lastly, ACGs can induce early apoptosis by mitochondrial interaction or caspases 9 and 8 activation [44]. These characteristics make Annona ACGs great candidates for the creation of bio-antimicrobial products and further research to discover which mechanisms provoke sensitivity and growth inhibition in certain fungal and bacterial species.
Like other Annona species, A. cherimola Mill. composition is rich in phytochemicals, with distinctive properties and biological activity [42]. Research conducted with A. cherimola extracts, compared its antioxidant activity to Annona muricata L. extracts, showing higher yields on A. cherimola, associated to the presence of ACGs. Aguilar-Villalva et al. [44] confirmed once more the inhibitory impact of A. cherimola against Gram-positive bacteria. Thereby, A. cherimola is an effective source of ACGs, comprising an array of 41 ACGs, isolated from seeds, leaves, stems, and roots. These compounds present chemical diversity which originates distinct substances: the number of hydroxyl groups and positioning of the THF ring plays a crucial role, defining bioactivity [2]. Some identified ACGs remain unstudied and their effects unknown. There are no current studies describing the ACG profile of A. cherimola variants found in Madeira Island, and these clearly trigger defensive mechanisms in F. oxysporum and A. niger, suggesting the presence of antifungal compounds. The nutritional value and phytochemical analysis of Madeira variants are of high importance in order to understand the agents responsible for antifungal activity and to unfold potential new properties.
Plants are a rich source of bioactive elements with various beneficial effects, and diverse studies have shown that Annona cherimola Mill. has beneficial properties that act against fungal threats. All cherimoya variants tested in the present study triggered a reaction on both Fusarium oxysporum and Aspergillus niger, showing promising results for the use of peel and seed residues as ingredients for a potential bio-fungicide. The fungi presented different responses, from growth constriction to morphological changes when exposed to peel and seed oils and compared to absolute and negative controls. There were no significant differences between the tested tissues, but data suggests that 100 mg/mL peel oils from FX, PV, MT, and DM controlled better F. oxysporum growth, reducing up to 60% on the third day of post-inoculation and AN limited A. niger growth, reaching 100% inhibition. Apart from growth limitations, the oils originated morpho-changes on Fusarium oxysporum, which could be related to attenuated virulence. The different concentrations applied to the colonies exhibited similar action, with no considerable change between treatments. However, the measuring method using CA values to calculate IR obtained more accurate results when compared to the pictures, and is therefore a better representation of IR. The data acquired in this study confirmed the value of A. cherimola variants found in Madeira Island and provided useful information about their antifungal activity. Further research should be carried out to better understand the phytochemical background responsible for these results and ideally establish a nutritional profile on all variants, as well as the extraction and isolation of present ACGs.
The authors declare they have not used Artificial Intelligence (AI) tools in the creation of this article.
The authors acknowledge the funding from the project FRUTTMAC (INTERREGMAC 2014-2020, Ref. MAC/1.1.b/310). For the authors integrated in the CITAB research Center, this work was co-supported by National Funds by FCT – Portuguese Foundation for Science and Technology, under the projects UID/04033/2020: Centro de Investigação e de Tecnologias Agro-Ambientais e Biológicas and LA/P/0126/2020 (https://doi.org/10.54499/LA/P/0126/2020). We thank the Regional Secretary of Agriculture and Rural Development of the Autonomous Region of Madeira and Mr. Henrique Alves for providing the cherimoya varieties in study.
The authors declare that they have no known competing financial interests or personal relationships that could have appeared to influence the work reported in this paper.
Conceptualization, C.T. and C.G.; methodology, C.T.; software, C.T. and C.G.; validation, M.O. and M.C.; formal analysis, C.T., C.G. and C.O.; investigation, C.T. and C.G; resources, H.N. and J.F.; data curation, C.T. and C.G.; writing – original draft preparation, C.T. and C.G.; writing – review and editing, C.T., C.G., M.O, H.N., J.F. and M.C.; visualization, C.T. and C.G.; supervision, C.G. and M.C.; project administration, C.G. and M.C.; funding acquisition, M.C. All authors have read and agreed to the published version of the manuscript.
MT10–ITS1
CCAACCCCTGTGAACATACCACTTGTTGCCTCGGCGGATCAGCCCGCTCCCGGTAAAACGGGACGGCCCGCCAGAGGACCCCTAAACTCTGTTTCTATATGTAACTTCTGAGTAAAACCATAAATAAATCAAAACTTTCAACAACGGATCTCTTGGTTCTGGCATCGATGAAGAACGCAGCAAAATGCGATAAGTAATGTGAATTGCAGAATTCAGTGAATCATCGAATCTTTGAACGCACATTGCGCCCGCCAGTATTCTGGCGGGCATGCCTGTTCGAGCGTCATTTCAACCCTCAAGCACAGCTTGGTGTTGGGACTCGCGTTAATTCGCGTTCCCCAAATTGATTGGCGGTCACGTCGAGCTTCCATAGCGTAGTAGTAAAACCCTCGTTACTGGTAATCGTCGCGGCCACGCCGTTAAACCCCAACTTCTGAATGTTGACCTCGGATCAGGTAGGAATACCCGCTGAACTTAAGCATATCAATAAGCCGGAGGAA
Reference strain: Fusarium oxysporum Fo47; Sequence ID (Genebank): NC_072843.1; Percent identity: 99, 60%; Query cover: 99%
MT23–ITS1
CTTTGGGCCCACCTCCCATCCGTGTCTATTGTACCCTGTTGCTTCGGCGGGCCCGCCGCTTGTCGGCCGCCGGGGGGGCGCCTCTGCCCCCCGGGCCCGTGCCCGCCGGAGACCCCAACACGAACACTGTCTGAAAGCGTGCAGTCTGAGTTGATTGAATGCAATCAGTTAAAACTTTCAACAATGGATCTCTTGGTTCCGGCATCGATGAAGAACGCAGCGAAATGCGATAACTAATGTGAATTGCAGAATTCAGTGAATCATCGAGTCTTTGAACGCACATTGCGCCCCCTGGTATTCCGGGGGGCATGCCTGTCCGAGCGTCATTGCTGCCCTCAAGCCCGGCTTGTGTGTTGGGTCGCCGTCCCCCTCTCCGGGGGGACGGGCCCGAAAGGCAGCGGCGGCACCGCGTCCGATCCTCGAGCGTATGGGGCTTTGTCACATGCTCTGTAGGATTGGCCGGCGCCTGCCGACGTTTTCCAACCATTCTTTCCAGGTTGACCTCGGATCAGGTAGGGATACCCGCTGAACTTAAGCATATCAATAAGCCGGAGGAA
Reference strain: Aspergillus niger supercontig An03; Sequence ID (Genebank): NT_166520.1; Percent identity: 99, 64%; Query cover: 100%
[1] |
B. Dourthe, N. Shaikh, S. A. Pai, S. Fels, S. H. M. Brown, D. R. Wilson, et al., Automated segmentation of spinal muscles from upright open MRI using a multiscale pyramid 2D convolutional neural network, Sping, 47 (2022), 1179–1186. https://doi.org/10.1097/BRS.0000000000004308 doi: 10.1097/BRS.0000000000004308
![]() |
[2] |
K. Mariam, O. M. Afzal, W. Hussain, M. U. Javed, A. Kiyani, N. Rajpoot, et al., On smart gaze based annotation of histopathology images for training of deep convolutional neural networks, IEEE J. Biomed. Health Inf., 26 (2022), 3025–3036. https://doi.org/10.1109/JBHI.2022.3148944 doi: 10.1109/JBHI.2022.3148944
![]() |
[3] |
X. Y. Wei, Y. Y. Wang, L. Ge, B. Peng, Q. He, R. Wang, et al., Unsupervised convolutional neural network for motion estimation in ultrasound elastography, IEEE Trans. Ultrason. Ferroelectr. Freq. Control, 69 (2022), 2236–2247. https://doi.org/10.1109/TUFFC.2022.3171676 doi: 10.1109/TUFFC.2022.3171676
![]() |
[4] |
W. Ba, H. Wu, W. W. Chen, S. H. Wang, Z. Y. Zhang, X. J. Wei, et al., Convolutional neural network assistance significantly improves dermatologists' diagnosis of cutaneous tumours using clinical images, Eur. J. Cancer, 169 (2022), 156–165. https://doi.org/10.1016/j.ejca.2022.04.015 doi: 10.1016/j.ejca.2022.04.015
![]() |
[5] |
A. Iqbal, M. Sharif, M. A. Khan, W. Nisar, M. Alhaisoni, FF-UNet: a u-shaped deep convolutional neural network for multimodal biomedical image segmentation, Cognit. Comput., 14 (2022), 1287–1302. https://doi.org/10.1007/s12559-022-10038-y doi: 10.1007/s12559-022-10038-y
![]() |
[6] | O. Ronneberger, P. Fischer, T. Brox, U-net: convolutional networks for biomedical image segmentation, in International Conference on Medical Image Computing and Computer-assisted Intervention, (2015), 234–241. https://doi.org/10.1007/978-3-319-24574-4_28 |
[7] |
Z. Li, H. Zhang, Z. Li, Z. Ren, Residual-attention UNet++: a nested residual-attention U-Net for medical image segmentation, Appl. Sci., 12 (2022), 7149. https://doi.org/10.3390/app12147149 doi: 10.3390/app12147149
![]() |
[8] |
L. F. Yu, Z. Qin, Y. Ding, Z. G. Qin, MIA-UNet: multi-scale iterative aggregation U-Network for retinal vessel segmentation, Comput. Model. Eng. Sci., 129 (2021), 805–828. https://doi.org/10.32604/cmes.2021.017332 doi: 10.32604/cmes.2021.017332
![]() |
[9] |
Y. J. He, J. S. Li, S. Shen, K. Liu, K. K. Wong, T. C. He, et al., Image-to-image translation of label-free molecular vibrational images for a histopathological review using the UNet plus/seg-cGAN model, BioMed. Opt. Express, 13 (2020), 1924–1938. https://doi.org/10.1364/BOE.445319 doi: 10.1364/BOE.445319
![]() |
[10] |
Y. Zhang, X. Liu, S. Wa, Y. Liu, J. Kang, C. Lv, GenU-Net++: an automatic intracranial brain tumors segmentation algorithm on 3D image series with high performance, Symmetry, 13 (2021), 2395. https://doi.org/10.3390/sym13122395 doi: 10.3390/sym13122395
![]() |
[11] |
C. Wang, Z. Y. Zhao, Y. Yu, Fine retinal vessel segmentation by combining Nest U-net and patch-learning, Soft Comput., 25 (2021), 5519–5532. https://doi.org/10.1007/s00500-020-05552-w doi: 10.1007/s00500-020-05552-w
![]() |
[12] |
M. Lei, J. Li, M. Li, L. Zou, H. Yu, An improved UNet++ model for congestive heart failure diagnosis using short-term RR intervals, Diagnostics, 11 (2021), 534. https://doi.org/10.3390/diagnostics11030534 doi: 10.3390/diagnostics11030534
![]() |
[13] |
Y. Lu, X. J. Qin, H. Y. Fan, T. T. Lai, Z. Y. Li, WBC-Net: a white blood cell segmentation network based on UNet++ and ResNet, Appl. Soft Comput., 101 (2021), 107006. https://doi.org/10.1016/j.asoc.2020.107006 doi: 10.1016/j.asoc.2020.107006
![]() |
[14] | Z. W. Zhou, M. M. R. Siddiquee, N. Tajbakhsh. J. M. Liang, UNet++: a nested U-Net architecture for medical image segmentation, in Deep Learning in Medical Image Analysis and Multimodal Learning for Clinical Decision Support, (2018), 3–11. https://doi.org/10.1007/978-3-030-00889-5_1 |
[15] | K. He, X. Zhang, S. Ren, J. Sun, Deep residual learning for image recognition, in Proceedings of the IEEE Conference on Computer Vision and Pattern Recognition, (2016), 770–778. https://doi.org/10.1109/CVPR.2016.90 |
[16] |
S. X. Chan, C. Huang, C. Bai, W. L. Ding, S. Y. Chen, Res2-UNeXt: a novel deep learning framework for few-shot cell image segmentation, Multimedia Tools Appl., 81 (2021), 13275–13288. https://doi.org/10.1007/s11042-021-10536-5 doi: 10.1007/s11042-021-10536-5
![]() |
[17] |
V. Jumutc, D. Bliznuks, A. Lihachev, Multi-Path U-Net architecture for cell and colony-forming unit image segmentation, Sensors, 22 (2022), 990. https://doi.org/10.3390/s22030990 doi: 10.3390/s22030990
![]() |
[18] |
P. Thi Le, T. Pham, Y. C. Hsu, J. C. Wang, Convolutional blur attention network for cell nuclei segmentation, Sensors, 22 (2022), 1586. https://doi.org/10.3390/s22041586 doi: 10.3390/s22041586
![]() |
[19] |
M. Makem, A. Tiedeu, G. Kom, Y. Nkandeu, A robust algorithm for white blood cell nuclei segmentation, Multimedia Tools Appl., 81 (2022), 17849–17874. https://doi.org/10.1007/s11042-022-12285-5 doi: 10.1007/s11042-022-12285-5
![]() |
[20] |
B. Yang, M. X. Wu, W. Teizer, Modified UNet++ with attention gate for graphene identification by optical microscopy, Carbon, 195 (2022), 246–252. https://doi.org/10.1016/j.carbon.2022.03.035 doi: 10.1016/j.carbon.2022.03.035
![]() |
[21] |
S. Bhagat, M. Kokare, V. Haswani, P. Hambarde, R. Kamble, Eff-UNet++: a novel architecture for plant leaf segmentation and counting, Ecol. Inf., 68 (2022), 101583. https://doi.org/10.1016/j.ecoinf.2022.101583 doi: 10.1016/j.ecoinf.2022.101583
![]() |
[22] |
F. Hoorali, H. Khosravi, B. Moradib, Automatic Bacillus anthracis bacteria detection and segmentation in microscopic images using UNet++, J. Microbiol. Methods, 177 (2020), 106056. https://doi.org/10.1016/j.mimet.2020.106056 doi: 10.1016/j.mimet.2020.106056
![]() |
[23] |
W. Zhao, Y. Zhao, L. Feng, J. Tang, Attention enhanced serial Unet++ network for removing unevenly distributed haze, Electronics, 10 (2021), 2868. https://doi.org/10.3390/electronics10222868 doi: 10.3390/electronics10222868
![]() |
[24] |
H. Zhao, H. Zhang, X. Zheng, A multiscale attention-guided UNet++ with edge constraint for building extraction from high spatial resolution imagery, Appl. Sci., 12 (2022), 5960. https://doi.org/10.3390/app12125960 doi: 10.3390/app12125960
![]() |
[25] |
S. Safarov, T. K. Whangbo, A-DenseUNet: adaptive densely connected UNet for polyp segmentation in colonoscopy images with atrous convolution, Sensors, 21 (2021), 1441. https://doi.org/10.3390/s21041441 doi: 10.3390/s21041441
![]() |
[26] |
J. J. Li, Y. Han, M. Zhang, G. Li, B. H. Zhang, Multi-scale residual network model combined with global average pooling for action recognition, Multimedia Tools Appl., 81 (2022), 1375–1393. https://doi.org/10.1007/s11042-021-11435-5 doi: 10.1007/s11042-021-11435-5
![]() |
[27] |
R. L. Kumar, J. Kakarla, B. V. Isunuri, M. Singh, Multi-class brain tumor classification using residual network and global average pooling, Multimedia Tools Appl., 80 (2021), 13429–13438. https://doi.org/10.1007/s11042-020-10335-4 doi: 10.1007/s11042-020-10335-4
![]() |
[28] |
R. Arora, I. Saini, N. Sood, Multi-label segmentation and detection of COVID-19 abnormalities from chest radiographs using deep learning, Optik, 246 (2021), 167780. https://doi.org/10.1016/j.ijleo.2021.167780 doi: 10.1016/j.ijleo.2021.167780
![]() |
[29] |
X. M. Liu, S. C. Wang, Y. Zhang, D. Liu, W. Hu, Automatic fluid segmentation in retinal optical coherence tomography images using attention based deep learning, Neurocomputing, 452 (2021), 576–591. https://doi.org/10.1016/j.neucom.2020.07.143 doi: 10.1016/j.neucom.2020.07.143
![]() |
[30] |
T. D. T. Phan, S. H. Kim, H. J. Yang, G. S. Lee, Skin lesion segmentation by U-Net with adaptive skip connection and structural awareness, Appl. Sci., 11 (2021), 4528. https://doi.org/10.3390/app11104528 doi: 10.3390/app11104528
![]() |
[31] | fpklipic, Dataset, 2019. Available from: https://github.com/fpklipic/BCISC. |
[32] |
M. Jiang, F. Zhai, J. Kong, A novel deep learning model DDU-net using edge features to enhance brain tumor segmentation on MR images, Artif. Intell. Med., 121 (2021), 102180. https://doi.org/10.1016/j.artmed.2021.102180 doi: 10.1016/j.artmed.2021.102180
![]() |
[33] |
A. Oulefki, S. Agaian, T. Trongtirakul, A. K. Laouar, Automatic COVID-19 lung infected region segmentation and measurement using CT-scans images, Pattern Recognit., 114 (2021), 107747. https://doi.org/10.1016/j.patcog.2020.107747 doi: 10.1016/j.patcog.2020.107747
![]() |
[34] |
Y. Y. Yang, C. Feng, R. F. Wang, Automatic segmentation model combining U-Net and level set method for medical images, Expert Syst. Appl., 153 (2020), 113419. https://doi.org/10.1016/j.eswa.2020.113419 doi: 10.1016/j.eswa.2020.113419
![]() |
[35] |
J. You, P. L. Yu, A. C. Tsang, E. L. Tsui, P. P. Woo, C. S. Lui, et al., 3D dissimilar-siamese-U-Net for hyperdense middle cerebral artery sign segmentation, Comput. Med. Imaging Graphics, 90 (2021), 101898. https://doi.org/10.1016/j.compmedimag.2021.101898 doi: 10.1016/j.compmedimag.2021.101898
![]() |
[36] |
S. Mohajerani, P. Saeedi, Cloud and cloud shadow segmentation for remote sensing imagery via filtered Jaccard loss function and parametric augmentation, IEEE J. Sel. Top. Appl. Earth Obs. Remote Sens., 14 (2021), 4254–4266. https://doi.org/10.1109/JSTARS.2021.3070786 doi: 10.1109/JSTARS.2021.3070786
![]() |
[37] |
V. S. Bochkov, L. Y. Kataeva, wUUNet: Advanced fully convolutional neural network for multiclass fire segmentation, Symmetry, 13 (2021), 98. https://doi.org/10.3390/sym13010098 doi: 10.3390/sym13010098
![]() |
[38] | G. Huang, Z. Liu, V. Laurens, K. Q. Weinberger, Densely connected convolutional networks, in: IEEE Conference on Computer Vision and Pattern Recognition, (2017), 2261–2269. https://doi.org/10.1109/CVPR.2017.243 |
[39] | J. Long, E. Shelhamer, T Darrell, Fully convolutional networks for semantic segmentation, in Proceedings of the IEEE Conference on Computer Vision and Pattern Recognition, (2015), 3431–3440. https://doi.org/10.1109/CVPR.2015.7298965 |
[40] |
V. Badrinarayanan, A. Kendall, R. Cipolla, SegNet: a deep convolutional encoder-decoder architecture for image segmentation, IEEE Trans. Pattern Anal. Mach. Intell., 39 (2017), 2481–2495. https://doi.org/10.1109/TPAMI.2016.2644615 doi: 10.1109/TPAMI.2016.2644615
![]() |
[41] | H. Huang, L. Lin, R. Tong, H. Hu, Q. Zhang, Y. Iwamoto, et al., UNet 3+: a full-scale connected UNet for medical image segmentation, in IEEE International Conference on Acoustics, Speech and Signal Processing, (2020), 1055–1059. https://doi.org/10.1109/ICASSP40776.2020.9053405 |