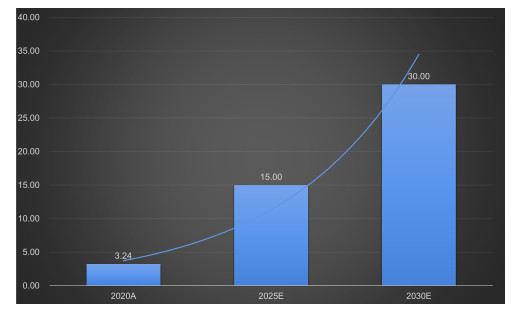
Over the past decade, the alternative fuel vehicle industry in the world has sprung up with huge speed. For example, the annual output has increased from less than 2000 vehicles to now 3,500,000 vehicles in China. It enjoys more than 50% of the market share worldwide in the global market. A spurt of progress in the alternative fuel vehicle industry has built a foundation for carbon peaking and carbon neutrality goals. Financial leasing has unique advantages which not only can provide guarantees for this industry in many aspects concerning related equipment, systems and infrastructures but also offer financial support for green projects. Nevertheless, financial leasing firms are encountering a string of problems to solve, such as selecting optimal green projects and cooperative businesses, designing transaction structures, and controlling project risks. This study contains several main sections: connecting the incremental alternative fuel vehicle investment and purchase project of a leading regional enterprise; building the structure of the financial leasing project; and analyzing the project leasing property using a fuzzy logic model, the financial structure and the repayment capacity of the project main company so as to comprehensively evaluate the feasibility of the project. This paper aims to provide a reference for future financing of alternative fuel vehicle operation enterprises with a real case study. The case study results show that our introduced fuzzy logic method can obtain the satisfying performance and traffic allocation.
Citation: Junlin Zhu, Hua Wang, Lin Miao, Zitong Yu. Case study of financial leasing model driven by fuzzy logic control for alternative fuel vehicles operation[J]. Mathematical Biosciences and Engineering, 2023, 20(1): 894-912. doi: 10.3934/mbe.2023041
[1] | Yongqiang Yao, Nan Ma, Cheng Wang, Zhixuan Wu, Cheng Xu, Jin Zhang . Research and implementation of variable-domain fuzzy PID intelligent control method based on Q-Learning for self-driving in complex scenarios. Mathematical Biosciences and Engineering, 2023, 20(3): 6016-6029. doi: 10.3934/mbe.2023260 |
[2] | Yong Xiong, Lin Pan, Min Xiao, Han Xiao . Motion control and path optimization of intelligent AUV using fuzzy adaptive PID and improved genetic algorithm. Mathematical Biosciences and Engineering, 2023, 20(5): 9208-9245. doi: 10.3934/mbe.2023404 |
[3] | Chaoyue Wang, Zhiyao Ma, Shaocheng Tong . Adaptive fuzzy output-feedback event-triggered control for fractional-order nonlinear system. Mathematical Biosciences and Engineering, 2022, 19(12): 12334-12352. doi: 10.3934/mbe.2022575 |
[4] | Jiliang Lv, Chenxi Qu, Shaofeng Du, Xinyu Zhao, Peng Yin, Ning Zhao, Shengguan Qu . Research on obstacle avoidance algorithm for unmanned ground vehicle based on multi-sensor information fusion. Mathematical Biosciences and Engineering, 2021, 18(2): 1022-1039. doi: 10.3934/mbe.2021055 |
[5] | Kangsen Huang, Zimin Wang . Research on robust fuzzy logic sliding mode control of Two-DOF intelligent underwater manipulators. Mathematical Biosciences and Engineering, 2023, 20(9): 16279-16303. doi: 10.3934/mbe.2023727 |
[6] | Fulin Dang, Chunxue Wu, Yan Wu, Rui Li, Sheng Zhang, Huang Jiaying, Zhigang Liu . Cost-based multi-parameter logistics routing path optimization algorithm. Mathematical Biosciences and Engineering, 2019, 16(6): 6975-6989. doi: 10.3934/mbe.2019350 |
[7] | Muhammad Akram, Maheen Sultan, José Carlos R. Alcantud, Mohammed M. Ali Al-Shamiri . Extended fuzzy $ N $-Soft PROMETHEE method and its application in robot butler selection. Mathematical Biosciences and Engineering, 2023, 20(2): 1774-1800. doi: 10.3934/mbe.2023081 |
[8] | Chao Wang, Cheng Zhang, Dan He, Jianliang Xiao, Liyan Liu . Observer-based finite-time adaptive fuzzy back-stepping control for MIMO coupled nonlinear systems. Mathematical Biosciences and Engineering, 2022, 19(10): 10637-10655. doi: 10.3934/mbe.2022497 |
[9] | Jinxin Du, Lei Liu . Adaptive fuzzy fixed time formation control of state constrained nonlinear multi-agent systems against FDI attacks. Mathematical Biosciences and Engineering, 2024, 21(3): 4724-4741. doi: 10.3934/mbe.2024207 |
[10] | Pasqual Martí, Jaume Jordán, Pablo Chamoso, Vicente Julian . Taxi services and the carsharing alternative: a case study of valencia city. Mathematical Biosciences and Engineering, 2022, 19(7): 6680-6698. doi: 10.3934/mbe.2022314 |
Over the past decade, the alternative fuel vehicle industry in the world has sprung up with huge speed. For example, the annual output has increased from less than 2000 vehicles to now 3,500,000 vehicles in China. It enjoys more than 50% of the market share worldwide in the global market. A spurt of progress in the alternative fuel vehicle industry has built a foundation for carbon peaking and carbon neutrality goals. Financial leasing has unique advantages which not only can provide guarantees for this industry in many aspects concerning related equipment, systems and infrastructures but also offer financial support for green projects. Nevertheless, financial leasing firms are encountering a string of problems to solve, such as selecting optimal green projects and cooperative businesses, designing transaction structures, and controlling project risks. This study contains several main sections: connecting the incremental alternative fuel vehicle investment and purchase project of a leading regional enterprise; building the structure of the financial leasing project; and analyzing the project leasing property using a fuzzy logic model, the financial structure and the repayment capacity of the project main company so as to comprehensively evaluate the feasibility of the project. This paper aims to provide a reference for future financing of alternative fuel vehicle operation enterprises with a real case study. The case study results show that our introduced fuzzy logic method can obtain the satisfying performance and traffic allocation.
With the proposal of achieving a carbon peak and carbon neutrality, practicing green finance and promoting green and low-carbon transformation have become significant directions for the development of the financial industry. As financial leasing has the dual properties of "financing + leasing assets", leasing companies can not only directly give financial support for green projects but also provide necessary guarantees for related equipment and infrastructure, which has unique advantages in guiding capital to flow to green industries. Moreover, financial leasing (FL) has become a common solution to financing problems encountered by firms [1]. At present, energy conservation and environmental protection, new energy, and other national strategic emerging industries have become the main focus of financing leasing companies. For that matter, alternative fuel vehicles operation has also become a major industry field.
To overcome the burden on fossil fuels, more attention has been drawn to fossil fuel substitution [2]. Due to a lack of resources and environmental constraints, there is a global consensus that alternative fuel vehicles will eventually replace traditional fuel vehicles. It is predicted that Chinese electric vehicles will reach 48% of the market share worldwide in the passenger vehicles market. Based on the prediction, by 2025, the total units sold will hit 15 million; this number will continue to grow to 30 million by 2030 (Figure 1). It is safe to predict that, shortly, from 2021 to 2025, the compound annual growth rate (CAGR) of the industry will be 36.37%; from 2026 to 2030, CAGR will be at a lower level, at 14.87% [3].
It is further predicted that the share of oil as a primary transportation power source in global travel fleets will fall from 94% today [4] to approximately 85% by 2040 under the Evolving Transition Scenario [5] or approximately 40% by 2050 under the Sustainable Development Scenario [6]. In terms of the quantity of AFVs in global travel fleets, it is estimated that the present global automobile count will approach 2–2.5 billion by 2050, with AFVs accounting for less than half of the total amount in 2050, and oil will continue to be utilized in the near future [7]. With the use of zero-emission AFVs in the transport industry [8], it will be unnecessary to spend such expenditures on the installation and upkeep of emission catalytic converters [9], and they can also mitigate the drawbacks of emission catalytic converters [10]. By 2025, it is anticipated that the market for fast and slow charging kinds of EV charging infrastructure for residential and commercial applications in the main EV-using nations will be less than USD 20 billion, with a compound annual growth rate of around 32% [11].
Never before has the alternative fuel vehicle industry been so booming; however, many new brands and players flooded into the market, causing product qualities to differ drastically. The market is transitioning from a policy-driven-type market to a market-driven-type one [12].
In the past decade, the financial leasing industry achieved continuous growth, and China has become the second largest financial leasing market in the world. The industry growth slowed down due to COVID-19; nevertheless, the total scale of the industry keeps rising. By 2030, the global market size of the financial leasing market will reach approximately 2.54 trillion USD. Based on public statistics, 181 entities conducted financial leasing business in 2021, and these entities include listed companies and subsidiaries. Fifty-three new market participants entered the industry in 2021. A total of 340 business transactions occurred throughout the year, a year-on-year increase of 41%; the total contract value was 96.8 billion CNY, a year-on-year increase of 76%.
By the end of 2021, there were 2 financial leasing firms and 6 commercial leasing firms honored with green certification. Statistics from Deutsche Bank Research illustrate that the market scale of the green finance industry will likely reach 100 trillion CNY by 2060. Assuming that the market saturation rate is 10%, the green financial leasing market scale would exceed 10 trillion CNY in 2060.
Clients who rent alternative fuel vehicles for official use mainly are enterprises and governments. Firms provide personalized programs of automobiles to clients, which covers integrated services of car rental, including vehicle configuration planning, routing algorithms, periodic maintenance, regular maintenance, reporting guidance and so on.
The rental income of official vehicles is charged according to the vehicle models and rental time, and it is directly recorded into the revenue in accounting. The leasing of official vehicles can be divided into temporary and long-term leasing. Contracts are signed to confirm the income, and the rental shall be paid annually or quarterly as agreed in contracts. Temporary rental charges a daily rate after each usage on the basis of vehicle models [13,14].
Costs primarily consist of vehicle purchasing fees, vehicle insurance, and maintenance fees. Outlay of temporary leasing also includes the cost of recharging.
Car-hailing licenses need to be acquired before new energy vehicle service providers can apply for car-hailing operation permissions governed by the local Management of Transportation Authority. The Company employs innovative Internet thinking to construct a full-scene online car-hailing platform, combining the platform + taxi with platform + driving services, and getting new resource automobiles to market operation.
Models of online car-hailing businesses can be divided into two groups, namely, pure rental mode and rental + commission mode. Monthly fees for individual drivers and quarterly fees for B-side rental companies, as follows in Figure 2; in the leasing + commission mode, vehicles affiliated with the enterprise's APP will charge a higher commission according to the fare paid by passengers, while vehicles attached to other cooperation platforms will charge a lower commission. Income of ride-hailing shall be directly recognized in the operating income in terms of accounting [15].
The rental cost of new energy online car-hailing mainly covers vehicle purchasing fees, as well as insurance, and the driver bears the cost of vehicle maintenance and charging. In terms of the composition of online car-hailing revenue, the commission of the business platform is relatively small, and the main source of income remains vehicle rental [16,17].
As an effective supplement to the business, new resource car operation enterprises usually layout the construction of charging piles. Charging pile products include floor type and wall-mounted type, to adapt to different application scenarios, and DC and AC models, for different specifications of equipment, with features of convenience, rapidity, security, environmental protection, etc. They are widely applied in residential areas, underground garages, expressway service areas, high-speed railway stations and other places [18]. Users recharge and pay through the APP, depending on the time and degree of using the charging pile.
The construction cost of the charging service basically consists of civil engineering, electric power installation and equipment procurement costs invested in the early stage of station construction. Operating costs primarily include electricity charges, site rent of the charging station, and charge pile maintenance costs and so on.
This paper proposes a case study for alternative fuel vehicle investment and purchasing method and extending its application. We propose a fuzzy logic control method to manage the economic model. This study contains several main sections: connecting the incremental alternative fuel vehicle investment and purchase project of a leading regional enterprise; building the structure of the financial leasing project; and analyzing the project leasing property using a fuzzy logic model, the financial structure and repayment capacity of the project main company so as to comprehensively evaluate the feasibility of the project. This paper aims to provide a reference for future financing of alternative fuel vehicle operation enterprises with a real case study. The case study results show that our introduced fuzzy logic method can obtain a satisfying performance.
The structure of this paper is as follows: First, the case study of a sale-leaseback project by a leading regional alternative fuel operating enterprise using fuzzy logic is presented in Section 2. The detailed implementation for the 3D simulation and bioinspired incremental teleoperation are described in Section 3. The application demonstration is illustrated in Section 4. In Section 5, discussion and conclusions are given.
Company Z is subordinate to Y Province Energy Investment Group Co., Ltd. It closely follows the green energy policies of Y Province and builds an integrated alternative fuel vehicles industry chain with alternative fuel vehicles as its core, involving official car leasing and alternative fuel vehicle operation, charging infrastructure construction and a network platform (Figure 3).
Based on its budget, Company Z will invest in 200 petrol-driven vehicles, 1300 alternative fuel vehicles and the construction of supporting electric charging facilities in 2022, with an annual investment budget of CNY 25.2702 million. Due to incremental vehicle investment procurement and electric charging infrastructure construction in 2022, Company Z plans to employ the financing lease sale-leaseback mode to finance, taking the subordinate charging stations and piles, and supporting power equipment as leasing items [19].
Based on the characteristics of the lessee, the lessor and the finance leasing industry of the project, the project team carried out systematic due diligence on the status and compliance of the leasehold and the operating and financial conditions of the lessee. Due diligence includes reviewing a large number of original documents, data and materials provided by the company; visiting the project site; interviewing related personnel; and discussing with the project team.
Company Z is a state-owned mixed-ownership enterprise with the characteristics of cross-regional, information, and intelligence, which integrates the business operations of "vehicles, piles, and nets" for alternative fuel vehicles. According to the list of the top 200 securities holders of Z Company (stock code: 872164) issued by China Securities Depository and Clearing Co, Ltd. The charter of the lessee's equity structure is as follows (Figure 4):
According to the requirements of relevant laws and regulations, the leased property must be a non-public welfare asset with full value, clear ownership and specific classification [20]. The leased items of this case study are the charging stations and piles and supporting power equipment used by the lessee. The charging station is invested in and constructed by Company Z. This case study mainly includes civil construction, electrical installation and design installation. Some charging piles are developed and produced by the lessee's subsidiary and sold to the lessee for installation at the charging station.
It can be proved that the charging stations, charging piles, and supporting power equipment are used by the lessee according to the construction contracts, engineering invoices, procurement contracts of charging piles and invoices during the project construction [21]. Through the credit reports, financing schedules, and official information of the Credit Reference Center, it can be demonstrated that the leased objects tenure is clear.
As of the end of December 2021, Company Z owns operating vehicle assets of 5807 in total, including 1000 motorcycles, 4021 alternative fuel vehicles, 766 fuel vehicles, and 20 electric bicycles. In 2018, 2019, 2020 and January-September 2021, Company Z realized operating incomes of CNY 65.7942 million, CNY 156.7412 million, CNY 105.0139 million, and CNY 107.0172 million, respectively. The composition of the operating income is as follows (Figure 5):
Car rental business: From 2018 to 2020 and January to September 2021, the lessee's car rental business achieved operating incomes of CNY 57.4211 million, CNY 71.3525 million, CNY 73.3309 million and CNY 83.3989 million, respectively, which were the main sources of the lessee's operating income. The income scale enlarged year by year, accounting for 87.27, 45.52, 69.83, and 77.93% of the main business income, respectively. In 2018, 2019, 2020 and January – September 2021, the operating costs of the lessee's car rental business were CNY 43.9003 million, CNY 47.1087 million, CNY 63.6392 million and CNY 67.4944 million, respectively; and the gross profit margins were 23.55, 33.98, 13.22 and 19.07% respectively.
The lessee's car rental business is divided into official car rental and online car rental. At present, there are 2857 official vehicles in operation, with an annual income of official vehicle leasing of about CNY 106.0053 million, under the current scale. Company Z operates a total of 1830 alternative fuel car-hailing vehicles, with an annual revenue of alternative fuel car-hailing rental of about CNY 73.017 million, under the current scale.
Transportation business: The Company's alternative fuel taxi operating income mostly derives from the transportation business. In 2018, 2019, 2020 and January – September 2021, the transportation business achieved operating incomes of CNY 0, CNY 1.1278 million, CNY 12.0518 million, and CNY 11.8219 million, respectively. The income scale increased annually, accounting for 0, 0.72, 11.48 and 11.05% of the main business income. In 2018, 2019, 2020 and January – September 2021, the operating costs of the lessee's transportation business were CNY 0, CNY 5.3401 million, CNY 2.5408 million, and CNY 7.5557 million, respectively; and gross profit margins were -373.48, 78.92 and 36.09%, respectively [22].
At present, company Z operates a total of 1100 alternative fuel taxis. The rental income of taxis varies according to the service life of the vehicles. Under the current scale, the annual income of alternative fuel taxis is approximately CNY 56.43 million.
Charging service business: As of the end of October 2021, the company has constructed 93 charging stations across the province, with a total of 1611 charging piles. In 2018, 2019, 2020 and January – September 2021, the lessee's charging service business achieved operating incomes of CNY 0.756 million, CNY 0.6328 million, CNY 7.2583 million, and CNY 7.3718 million, respectively, accounting for 1.15, 0.40, 6.91 and 6.89% of the main business income. From 2018 to 2020 and from January to September 2021, the operating costs of the lessee's charging service business were CNY 0.7172 million, CNY 6.6744 million, CNY 14.6376 million and CNY 14.3556 million, respectively; and the gross profit margins were 5.13, -954.68, -101.67 and -94.74%.
Asset structure analysis: In 2018, 2019, 2020 and January – September 2021, Company Z's total assets were CNY 216.7948 million, CNY 924.7179 million, CNY 865.7715 million and CNY 933.7956 million, respectively. The total current assets were CNY 41.7592 million, CNY 439.2318 million, CNY 352.4948 million and CNY 386.0767 million, respectively, accounting for 19.26, 47.50, 40.71 and 41.34% of the total assets, respectively. The non-current assets were CNY 175.0356 million, CNY 485.4861 million, CNY 513.2767 million, and CNY 547.7189 million, respectively, accounting for 80.74, 52.50, 59.29 and 58.66% of the total assets respectively.
Liability structure analysis: In 2018, 2019, 2020 and January – September 2021, the total liabilities of Company Z were CNY 181.6052 million, CNY 888.5371 million, CNY 888.5371 million, and CNY 899.1026 million, respectively (Figure 6). The total current liabilities were CNY 152.4379 million, CNY 759.5845 million, CNY 387.4508 million and CNY 490.533 million CNY, accounting for 83.94, 85.49, 46.80, and 54.56% of the total liabilities, respectively. The non-current liabilities were CNY 29.1673 million, CNY 128.9526 million, CNY 440.3585 million and CNY 408.5696 million, respectively, accounting for 16.06, 14.51, 53.20, and 45.44% of the total liabilities, respectively [23].
The lessee has a clear asset-liability structure but a relatively high asset-liability ratio. The reasons are as follows: First, the lessee's equity is relatively low, and the registered capital is only CNY 55 million. In March 2022, after multiple rounds of negotiations between state-owned shareholders and private shareholders, it was determined that the plan for the first phase of capital increase was directional issuance with the price per share being not less than CNY 1.01. The number of issued shares shall not exceed 55 million, and the raised amount shall not exceed CNY 55.55 million, which will be in place in the first half of 2022. After the capital increase, the registered capital of the lessee will increase to CNY 110.55 million, with the asset-liability ratio decreasing. Second, Company Z conducts its business and financing based on vehicle assets. The lessee's assets are mainly composed of monetary funds, fixed assets and construction in progress. The core assets concerning the vehicles and the charging stations under construction can bring stable income and cash flow to the firm [24]. The liability side consists of notes payable, long-term liabilities due within one year and long-term payable, mainly arising from notes to purchase vehicles and existing vehicles for financing.
Analysis of income and profit: From the perspective of operating gross profit, the company's operating gross profits were CNY 12.7321 million, CNY 41.2917 million, CNY 12.8770 million and CNY 13.2767 million, respectively in 2018, 2019, 2020 and January – September 2021. The comprehensive gross profit margins were 19.35, 26.34, 12.26, and 12.41%, respectively. In 2020, the gross profit margin of Z Company's car rental business was 13.22%, with the gross profit margin of the transportation business at 78.92% and the gross profit margin of automobile and accessories sales at 3.94% [25].
In 2018, 2019, 2020 and January – September 2021, Z Company's operating profits were CNY -14.7108 million, CNY 1.6668 million, CNY 0.9007 million and CNY -3.1373, respectively; and the net profits were CNY -15.1411 million, CNY 1.6148 million, CNY 1.7422 million and CNY -3.5278 million (Figure 7).
Analysis of cash flow: In 2018, 2019, 2020 and January – September 2021, the lessee's net cash flows from operating activities were CNY 38.0633 million, CNY 63.1995 million, CNY 95.2224 million and CNY 88.0061 million, respectively. The lessee's net cash flows from operating activities are all positive, and there are still many remaining operating cash inflows after meeting operating expenses, which can be used to repay debts. In 2018, 2019, 2020 and January – September 2021, the net cash flows from the lessee's investment activities were CNY -47.9309 million, CNY -356.4511 million, CNY-138.789 million and CNY -118.9426 million, respectively. The lessee's cash outflow from investing activities is mainly due to the purchase of vehicles and investment in the construction of charging stations. In 2018, 2019, 2020 and January – September 2021, the net cash flows from the lessee's financing activities were CNY -25.092 million, CNY 322.8549 million, CNY 155.7473 million and CNY -32.6331 million, respectively. This year, the lessee's projects under construction, investment projects, and business development require a large investment, and financing methods such as borrowing can effectively support the lessee's development [26] in (Figures 8 and 9).
Key financial indicators: In terms of operational status, in 2018, 2019, 2020 and January – September 2021, the company's accounts receivable collection efficiency was relatively high within one year of the account period. The overall inventory turnover ratio was at a healthy level. The company has a relatively mature and stable operating project team with assets in good use, funds running normally, and strong operational capabilities. In terms of solvency, in 2018, 2019, 2020 and January – September 2021, the company's asset-liability ratio was relatively high, but its liquidity was a little low. Since the company is currently in the stage of rapid investment and construction, the proportion of capital investment is also high.
The funds invested in this case study mainly flow into the official vehicles and alternative fuel online car-hailing sectors. According to the analysis mentioned above of the incremental fuel vehicle rental projects and alternative fuel vehicle promotion projects in 2022, and the additional 200 fuel rental vehicles will bring CNY 13.608 million. The newly added 500 alternative fuel official vehicles will generate CNY 24.696 million in operating income [27]. Due to the relatively stable gross profit per unit from official vehicle leasing, the project can bring fairly stable income and profit growth. In terms of online car-hailing, according to the company's alternative fuel vehicle promotion project in 2022, the newly launched 800 alternative fuel online-hailing cars will bring an operating income of CNY 31.92 million. The charging service business will also bring in an operating income of CNY 15 million per year. After the project is put into operation, it will play a crucial role in improving the operation of the lessee and also become an essential source of repayment for the project. We predicted the cash flow of the lessee based on operating activities, investment activities, and financing activities in (Figure 10).
The cash flow forecast shows that as long as the company retains the current operating capacity and maintains a low level of working capital financing, it can firmly support the operating activities of the company in the future. At the same time, as the main operating member of alternative fuel vehicles and charging piles, Company Z is strongly supported by relevant policies with a promising market prospect. In 2022, the cash flow will be greatly improved with the continuous investment in the main business.
Financial risks: Company Z's asset-liability ratio continues to rise, along with the average profitability and the company's uncertain future development.
Policy risk: As an industry encouraged by national policies, the rapid development and future potentials of the car rental industry have indispensable support from national policies. If there are any major adverse changes in the above policies, it will bring uncertainty to the sustainable and stable development of the company.
Market risk: Along with continuous growth of the national economy and income per capita, the improvement of urbanization and road infrastructure, the car rental industry is ushering in unprecedented development opportunities, with huge market space. The industry has attracted many domestic and foreign car rental companies and the involvement of venture capital funds. With the support of venture capital and the innovation of the Internet-based business model [28], competition has intensified in the car rental industry, with the risk of increasingly fierce market competition [29].
The fuzzification idea of dummy variables has been acquired from the non-linear part of econometric regime-switching models. In these models, the concept of transfer functions is like the notion of fuzzy membership functions, but no principle or linguistic sentence has been used for inputs [30,31,32]. Since 1965, the FLC algorithm proposed Sattar et al. [33,34,35,36] has been widely used in various fields of economic management. Especially in the field of the economy, the flow chart of FLC execution is shown in Figure 11. It can be divided into four parts: fuzzification, rule base, fuzzy inference and defuzzification.
Fuzzification is to convert several input parameter values into fuzzy logic [37], and these parameter values will correspond to the membership function to derive the corresponding output membership degree. The rule library is to store all the possible rules of the input terminal, and it is established based on the experience of experts or training from samples. Fuzzy inference is when the input parameter value may have one or more applicable rules to make an appropriate output. Defuzzification is to restore the result of fuzzy inference to the value needed for the actual problem.
Generally speaking, the method of the defuzzification calculation is divided into the maximum average method and the center of gravity method. For example, suppose there is an input terminal that is fuzzified to obtain an output membership degree, and then the output membership degree is determined from the rule library and fuzzy inference to find the output selection that meets the problem. Finally, the actual output value required by the problem is obtained through defuzzification; the flow chart of fuzzy logic control is shown in in Figure 12.
In the paper [35], a generalized fuzzy logic controller (FLC) was used to optimize fuel economy and reduce the emissions of hybrid vehicles with parallel configurations. Using the driver input, the energy storage's state of charge (SOC) was been developed in a fuzzy controller to effectively determine the power split between the electric machine and the internal combustion engine (ICE). In addition, a power train of the fuel cell vehicles includes the battery as a secondary power source to improve the vehicle's performance, and two EMSs (Energy Management Systems) are introduced to control the energy in the fuel cell hybrid vehicle (FCHV) in the paper [38].
This section describes how the FLC model can be used to adjust the output transmission rate correlation of the aggregation node. However, the weight parameter is fixed in the algorithm. If the change in the transmitted data is too large, the error will be very large, and the performance of the entire network would not be optimized. Therefore, this method algorithm uses FLC to adjust the weight parameter of the WPRC algorithm according to the change in the transmitted data, which enables the sink node to obtain the optimal system output. In addition, this method uses the priority of each child node to adjust the transmission rate. Its membership funcition can be found in Figure 13.
Company Z has a relatively high asset-liability ratio with certain repayment risks. If its state-owned shareholders raise CNY 55.55 million through directional issuance of shares, the asset-liability ratio will decline. We adopted the FLC model to adjust the output transmission rate correlation of the aggregation node. The funds invested in this project are used for the purchase of incremental alternative fuel vehicles and the construction of charging facilities, which will play a vital role in improving the lessee's operation. At the same time, the lessee's charging pile business has promising prospects with sufficient project repayment sources. The charging piles of the leasing object are a standard separable property lease, in line with the concepts of new energy, environmental protection and carbon neutrality. Meanwhile, it is beneficial to the company's refinancing through ABS, ABN and bank credit [39].
As this project belongs to the green energy industry, the lessee's main business is supported by policies, with strong sustainability and relatively manageable project risks.
With the accompanying policies of national and local government, China's alternative fuel vehicle operation industry has flourished with a great leap in industrialization and scale [40]. As is so often the case, the model of the alternative fuel vehicle operation enterprise can be classified into three categories: official vehicle rental, online car-hailing rental and charging piles and supporting facilities construction, with different incomes and costs. This case study analyzed the sale-leaseback project of Z Company, a leading regional enterprise affiliated with Y Province Energy Investment Group Co., Ltd. Leased assets include charging stations and piles, supporting power equipment, etc. As a new energy operation enterprise, Company Z integrates "vehicles, piles and nets" for alternative fuel vehicles in business operations, demonstrating strong trade representativeness. Ownership of leased assets can be proven through contracts and invoices. Car leasing is the main source of income for alternative fuel automobile operating companies, and its gross margin is around 20%. From the perspective of financial analysis, the assets of new energy operating enterprises are chiefly composed of currency funds, fixed assets, and projects under construction. Its overall profit is not considerable, but the asset-liability ratio is high in general, and cash flow from operating activities remains healthy [41]. In the aspect of reimbursement capacity, the gross profit of the official vehicle rental is relatively constant, which can bring stable growth of income and profit. Normally, the rapid development and future potential of alternative fuel vehicle operating enterprises cannot live without the support of national policies. Although these companies are supported by venture capital and the internet-based model, they will be inevitably confronted with policy and market risks. This study contains several main sections: connecting the incremental alternative fuel vehicle investment and purchase project of a leading regional enterprise; building the structure of the financial leasing project; and analyzing the project leasing property using a fuzzy logic model, the financial structure and repayment capacity of the project main company so as to comprehensively evaluate the feasibility of the project. This paper aims to provide a reference for future financing of alternative fuel vehicle operation enterprises with a real case study. The case study results show that our introduced fuzzy logic method can obtain satisfying performance and traffic allocation. The multi-dimensional evaluation in this paper will provide a reference for the financing of alternative fuel vehicle operation enterprises or green energy leasing projects in the future.
This study was supported by the Yunnan Provincial Education Department Scientific Research Fund Project (2021J0133).
The authors declare there is no conflict of interest.
[1] |
W. Wang, L. Feng, Y. Li, F. Xu, Q. Deng, Role of financial leasing in a capital-constrained service supply chain, Transp. Res. Part E: Logist. Transp. Rev., 143 (2020), 102097. https://doi.org/10.1016/j.tre.2020.102097 doi: 10.1016/j.tre.2020.102097
![]() |
[2] |
H. Yaqoob, Y. H. Teoh, F. Sher, M. A. Jamil, D. Murtaza, M. A. Qubeissi, et al., Current status and potential of tire pyrolysis oil production as an alternative fuel in developing countries, Sustainability, 13 (2021), 3214. https://doi.org/10.3390/su13063214 doi: 10.3390/su13063214
![]() |
[3] |
S. Beggs, S. Cardell, J. Hausman, Assessing the potential demand for electric cars, J. Econom., 17 (1981), 1–19. https://doi.org/10.1016/0304-4076(81)90056-7 doi: 10.1016/0304-4076(81)90056-7
![]() |
[4] | L. Capuano, Us energy information administration's international energy outlook 2020 (ieo2020), US Department of Energy: Washington DC, 2020. |
[5] | BP Energy Outlook, 2019 edition, London, United Kingdom, 2019. Available from: https://www.bp.com/content/dam/bp/business-sites/en/global/corporate/pdfs/energy-economics/energy-outlook/bp-energy-outlook-2019.pdf. |
[6] | U. Jahn, D. Mayer, M. Heidenreich, R. Dahl, S. Castello, L. Clavadetscher, et al., International energy agency pvps task 2: analysis of the operational performance of the iea database pv systems, in Sixteenth European Photovoltaic Solar Energy Conference, Routledge, (2020), 2673–2677. Available from: https://www.researchgate.net/publication/324727795. |
[7] | M. A. Ghadikolaei, P. K. Wong, C. S. Cheung, J. Zhao, Z. Ning, K. Yung, et al., Why is the world not yet ready to use alternative fuel vehicles? Heliyon, 7 (2021), e07527. https://doi.org/10.1016/j.heliyon.2021.e07527 |
[8] |
S. Dey, G. C. Dhal, Controlling carbon monoxide emissions from automobile vehicle exhaust using copper oxide catalysts in a catalytic converter, Mater. Today Chem., 17 (2020), 100282. https://doi.org/10.1016/j.mtchem.2020.100282 doi: 10.1016/j.mtchem.2020.100282
![]() |
[9] |
S. Ramalingam, S. Rajendran, P. Ganesan, Performance improvement and exhaust emissions reduction in biodiesel operated diesel engine through the use of operating parameters and catalytic converter: a review, Renewable Sustainable Energy Rev., 81 (2018), 3215–3222. https://doi.org/10.1016/j.rser.2017.08.069 doi: 10.1016/j.rser.2017.08.069
![]() |
[10] | M. de Almeida D'Agosto, Air pollutant and greenhouse gas emissions (ghg), in Transportation, Energy Use and Environmental Impacts, Elsevier, (2019), 227–257. https://doi.org/10.1016/C2016-0-04814-3 |
[11] |
T. Chen, X. Zhang, J. Wang, J. Li, C. Wu, M. Hu, et al., A review on electric vehicle charging infrastructure development in the UK, J. Mod. Power Syst. Clean Energy, 8 (2020), 193–205. https://doi.org/10.35833/MPCE.2018.000374 doi: 10.35833/MPCE.2018.000374
![]() |
[12] |
A. Ziegler, Individual characteristics and stated preferences for alternative energy sources and propulsion technologies in vehicles: a discrete choice analysis for germany, Transp. Res. Part A: Policy Pract., 46 (2012), 1372–1385. https://doi.org/10.1016/j.tra.2012.05.016 doi: 10.1016/j.tra.2012.05.016
![]() |
[13] |
H. Su, Y. Hu, H. R. Karimi, A. Knoll, G. Ferrigno, E. De Momi, Improved recurrent neural network-based manipulator control with remote center of motion constraints: experimental results, Neural Networks, 131 (2020), 291–299. https://doi.org/10.1016/j.neunet.2020.07.033 doi: 10.1016/j.neunet.2020.07.033
![]() |
[14] |
W. Qi, H. Su, A. Aliverti, A smartphone-based adaptive recognition and real-time monitoring system for human activities, IEEE Trans. Hum.-Mach. Syst., 50 (2020), 414–423. https://doi.org/10.1109/THMS.2020.2984181 doi: 10.1109/THMS.2020.2984181
![]() |
[15] |
M. Beise, K. Rennings, Lead markets and regulation: a framework for analyzing the international diffusion of environmental innovations, Ecol. Econ., 52 (2005), 5–17. https://doi.org/10.1016/j.ecolecon.2004.06.007 doi: 10.1016/j.ecolecon.2004.06.007
![]() |
[16] |
H. Su, A. Mariani, S. E. Ovur, A. Menciassi, G. Ferrigno, E. De Momi, Toward teaching by demonstration for robot-assisted minimally invasive surgery, IEEE Trans. Autom. Sci. Eng., 18 (2021), 484–494. https://doi.org/10.1109/TASE.2020.3045655 doi: 10.1109/TASE.2020.3045655
![]() |
[17] |
W. Qi, H. Su, A cybertwin based multimodal network for ecg patterns monitoring using deep learning, IEEE Trans. Ind. Inf., 18 (2022), 6663–6670. https://doi.org/10.1109/TII.2022.3159583 doi: 10.1109/TII.2022.3159583
![]() |
[18] |
D. Bolduc, N. Boucher, R. Alvarez-Daziano, Hybrid choice modeling of new technologies for car choice in Canada, Transp. Res. Rec., 2082 (2008), 63–71. https://doi.org/10.3141/2082-08 doi: 10.3141/2082-08
![]() |
[19] | H. Su, W. Qi, Y. Schmirander, S. E. Ovur, S. Cai, X. Xiong, A human activity-aware shared control solution for medical human–robot interaction, Assembly Autom., 2022 (2022). https://doi.org/10.1108/aa-12-2021-0174 |
[20] |
R. A. Daziano, D. Bolduc, Incorporating pro-environmental preferences towards green automobile technologies through a bayesian hybrid choice model, Transp. A: Transport Sci., 9 (2013), 74–106. https://doi.org/10.1080/18128602.2010.524173 doi: 10.1080/18128602.2010.524173
![]() |
[21] |
D. S. Bunch, M. Bradley, T. F. Golob, R. Kitamura, G. P. Occhiuzzo, Demand for clean-fuel vehicles in california: a discrete-choice stated preference pilot project, Transp. Res. Part A: Policy Pract., 27 (1993), 237–253. https://doi.org/10.1016/0965-8564(93)90062-P doi: 10.1016/0965-8564(93)90062-P
![]() |
[22] |
G. Ewing, E. Sarigöllü, Assessing consumer preferences for clean-fuel vehicles: a discrete choice experiment, J. Public Policy Mark., 19 (2000), 106–118. https://doi.org/10.1509/jppm.19.1.106.16946 doi: 10.1509/jppm.19.1.106.16946
![]() |
[23] |
G. O. Ewing, E. Sarigöllü, Car fuel-type choice under travel demand management and economic incentives, Transp. Res. Part D: Transp. Environ., 3 (1998), 429–444. https://doi.org/10.1016/S1361-9209(98)00019-4 doi: 10.1016/S1361-9209(98)00019-4
![]() |
[24] |
D. A. Hensher, M. J. Beck, J. M. Rose, Accounting for preference and scale heterogeneity in establishing whether it matters who is interviewed to reveal household automobile purchase preferences, Environ. Resour. Econ., 49 (2011), 1–22. https://doi.org/10.1007/s10640-010-9420-3 doi: 10.1007/s10640-010-9420-3
![]() |
[25] | J. Farrell, G. Saloner, Installed base and compatibility: innovation, product preannouncements, and predation, Am. Econ. Rev., 76 (1986), 940–955. Available from: https://www.researchgate.net/publication/243776630. |
[26] | R. D. Luce, Preference, utility and subjective probability, in Handbook of Mathematical Psychology, 1965. Available from: https://www.imbs.uci.edu/files/personnel/luce/pre1990/1965/LuceSuppes_Book%20Chapter_1965.pdf. |
[27] |
M. Achtnicht, German car buyers' willingness to pay to reduce co2 emissions, Clim. Change, 113 (2012), 679–697. https://doi.org/10.1007/s10584-011-0362-8 doi: 10.1007/s10584-011-0362-8
![]() |
[28] |
B. Oztaysi, S. C. Onar, C. Kahraman, M. Yavuz, Multi-criteria alternative-fuel technology selection using interval-valued intuitionistic fuzzy sets, Transp. Res. Part D: Transp. Environ., 53 (2017), 128–148. https://doi.org/10.1016/j.trd.2017.04.003 doi: 10.1016/j.trd.2017.04.003
![]() |
[29] |
W. L. Moore, M. B. Holbrook, Conjoint analysis on objects with environmentally correlated attributes: The questionable importance of representative design, J. Consum. Res., 16 (1990), 490–497. https://doi.org/10.1086/209234 doi: 10.1086/209234
![]() |
[30] |
A. Matviychuk, Bankruptcy prediction in transformational economy: discriminant and fuzzy logic approaches, Fuzzy Econ. Rev., 15 (2010), 21–38. https://doi.org/10.25102/fer.2010.01.02 doi: 10.25102/fer.2010.01.02
![]() |
[31] |
M. Bublyk, A. Kowalska-Styczeń, V. Lytvyn, V. Vysotska, The ukrainian economy transformation into the circular based on fuzzy-logic cluster analysis, Energies, 14 (2021), 5951. https://doi.org/10.3390/en14185951 doi: 10.3390/en14185951
![]() |
[32] |
R. Draeseke, D. E. A. Giles, A fuzzy logic approach to modelling the new zealand underground economy, Math. Comput. Simul., 59 (2002), 115–123. https://doi.org/10.1016/S0378-4754(01)00399-8 doi: 10.1016/S0378-4754(01)00399-8
![]() |
[33] | H. Sattar, I. S. Bajwa, R. ul Amin, J. Muhammad, M. F. Mushtaq, R. Kazmi, et al., Smart wound hydration monitoring using biosensors and fuzzy inference system, Wireless Commun. Mobile Comput., 2019 (2019). https://doi.org/10.1155/2019/8059629 |
[34] | K. S. Mayer, M. S. De Oliveira, C. Müller, F. C. C. De Castro, M. C. F. De Castro, Blind fuzzy adaptation step control for a concurrent neural network equalizer, Wireless Commun. Mobile Comput., 2019 (2019). https://doi.org/10.1155/2019/9082362 |
[35] |
N. A. Kheir, M. A. Salman, N. J. Schouten, Emissions and fuel economy trade-off for hybrid vehicles using fuzzy logic, Math. Comput. Simul., 66 (2004), 155–172. https://doi.org/10.1016/j.matcom.2003.11.007 doi: 10.1016/j.matcom.2003.11.007
![]() |
[36] |
K. V. Singh, H. O. Bansal, D. Singh, Feed-forward modeling and real-time implementation of an intelligent fuzzy logic-based energy management strategy in a series–parallel hybrid electric vehicle to improve fuel economy, Electr. Eng., 102 (2020), 967–987. https://doi.org/10.1007/s00202-019-00914-6 doi: 10.1007/s00202-019-00914-6
![]() |
[37] |
S. Safari, A.R. Erfani, A new method for fuzzification of nested dummy variables by fuzzy clustering membership functions and its application in financial economy, Iran. J. Fuzzy Syst., 17 (2020), 13–27. https://doi.org/10.22111/IJFS.2020.5108 doi: 10.22111/IJFS.2020.5108
![]() |
[38] |
S. Ahmadi, S. Bathaee, Multi-objective genetic optimization of the fuel cell hybrid vehicle supervisory system: fuzzy logic and operating mode control strategies, Int. J. Hydrogen Energy, 40 (2015), 12512–12521. https://doi.org/10.1016/j.ijhydene.2015.06.160 doi: 10.1016/j.ijhydene.2015.06.160
![]() |
[39] |
R. Batley, J. Toner, M. Knight, A mixed logit model of uk household demand for alternative-fuel vehicles, Int. J. Transport Econ., 31 (2004), 1000–1023. https://doi.org/10.1400/16901 doi: 10.1400/16901
![]() |
[40] | A. Körner, C. Tam, S. Bennett, J. Gagné, Technology roadmap-hydrogen and fuel cells, International Energy Agency (IEA), Paris, France, 2015. Available from: https://www.aeh2.org/images/stories/PDF/DOCS_SECTOR/technologyroadmaphydrogenandfuelcells.pdf. |
[41] |
J. I. Yellott Jr, The relationship between luce's choice axiom, thurstone's theory of comparative judgment, and the double exponential distribution, J. Math. Psychol., 15 (1977), 109–144. https://doi.org/10.1016/0022-2496(77)90026-8 doi: 10.1016/0022-2496(77)90026-8
![]() |
1. | Cengiz Kahraman, Elif Haktanır, 2024, Chapter 8, 978-3-031-54659-4, 117, 10.1007/978-3-031-54660-0_8 |