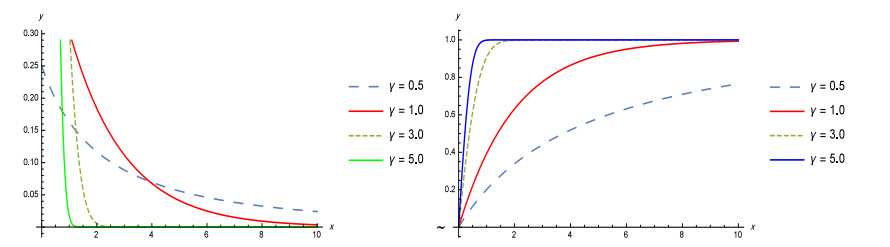
In recent years, recycling of plastics has opened several doors of advancements in the field of additive manufacturing (AM). The process of fused deposition modelling (FDM) has already been utilized for reprocessing waste plastics into filaments and finally printing them into useful products. Yet another concept of extrusion additive manufacturing (EAM) is gaining lots of attention. In this work, a screw assisted system based on EAM is designed and installed alongside a pre-existing FDM system. This system is referred to as the direct FDM (DFDM) system throughout this work. The DFDM system used operates with a 1.75 mm nozzle and has the ability of giving a high throughput. The focus of this work is to use this hybrid system (combination of FDM and DFDM systems) to print both virgin as well as recycled plastics. The scope of this work is to use one technology (either FDM or DFDM) at a time and to use both simultaneously for multi-material printing in future. After several trials of printing and setting up some printing parameters, the proposed system has been able to print with virgin as well as recycled PLA.
Citation: Tanay Kuclourya, Roberto Monroy, Miguel Castillo, David Baca, Rafiq Ahmad. Design of a hybrid high-throughput fused deposition modeling system for circular economy applications[J]. Clean Technologies and Recycling, 2022, 2(4): 170-198. doi: 10.3934/ctr.2022010
[1] | Mustafa M. Hasaballah, Oluwafemi Samson Balogun, M. E. Bakr . Frequentist and Bayesian approach for the generalized logistic lifetime model with applications to air-conditioning system failure times under joint progressive censoring data. AIMS Mathematics, 2024, 9(10): 29346-29369. doi: 10.3934/math.20241422 |
[2] | Bing Long, Zaifu Jiang . Estimation and prediction for two-parameter Pareto distribution based on progressively double Type-II hybrid censored data. AIMS Mathematics, 2023, 8(7): 15332-15351. doi: 10.3934/math.2023784 |
[3] | Samah M. Ahmed, Abdelfattah Mustafa . Estimation of the coefficients of variation for inverse power Lomax distribution. AIMS Mathematics, 2024, 9(12): 33423-33441. doi: 10.3934/math.20241595 |
[4] | Magdy Nagy, Khalaf S. Sultan, Mahmoud H. Abu-Moussa . Analysis of the generalized progressive hybrid censoring from Burr Type-Ⅻ lifetime model. AIMS Mathematics, 2021, 6(9): 9675-9704. doi: 10.3934/math.2021564 |
[5] | Hassan Okasha, Mazen Nassar, Saeed A. Dobbah . E-Bayesian estimation of Burr Type XII model based on adaptive Type-Ⅱ progressive hybrid censored data. AIMS Mathematics, 2021, 6(4): 4173-4196. doi: 10.3934/math.2021247 |
[6] | Mohamed S. Eliwa, Essam A. Ahmed . Reliability analysis of constant partially accelerated life tests under progressive first failure type-II censored data from Lomax model: EM and MCMC algorithms. AIMS Mathematics, 2023, 8(1): 29-60. doi: 10.3934/math.2023002 |
[7] | Hanan Haj Ahmad, Ehab M. Almetwally, Dina A. Ramadan . A comparative inference on reliability estimation for a multi-component stress-strength model under power Lomax distribution with applications. AIMS Mathematics, 2022, 7(10): 18050-18079. doi: 10.3934/math.2022994 |
[8] | Hatim Solayman Migdadi, Nesreen M. Al-Olaimat, Maryam Mohiuddin, Omar Meqdadi . Statistical inference for the Power Rayleigh distribution based on adaptive progressive Type-II censored data. AIMS Mathematics, 2023, 8(10): 22553-22576. doi: 10.3934/math.20231149 |
[9] | Ahmed R. El-Saeed, Ahmed T. Ramadan, Najwan Alsadat, Hanan Alohali, Ahlam H. Tolba . Analysis of progressive Type-Ⅱ censoring schemes for generalized power unit half-logistic geometric distribution. AIMS Mathematics, 2023, 8(12): 30846-30874. doi: 10.3934/math.20231577 |
[10] | Haiping Ren, Xue Hu . Estimation for inverse Weibull distribution under progressive type-Ⅱ censoring scheme. AIMS Mathematics, 2023, 8(10): 22808-22829. doi: 10.3934/math.20231162 |
In recent years, recycling of plastics has opened several doors of advancements in the field of additive manufacturing (AM). The process of fused deposition modelling (FDM) has already been utilized for reprocessing waste plastics into filaments and finally printing them into useful products. Yet another concept of extrusion additive manufacturing (EAM) is gaining lots of attention. In this work, a screw assisted system based on EAM is designed and installed alongside a pre-existing FDM system. This system is referred to as the direct FDM (DFDM) system throughout this work. The DFDM system used operates with a 1.75 mm nozzle and has the ability of giving a high throughput. The focus of this work is to use this hybrid system (combination of FDM and DFDM systems) to print both virgin as well as recycled plastics. The scope of this work is to use one technology (either FDM or DFDM) at a time and to use both simultaneously for multi-material printing in future. After several trials of printing and setting up some printing parameters, the proposed system has been able to print with virgin as well as recycled PLA.
Applying censoring techniques to a single population entails specific limitations. While progressive type-II censoring permits the exclusion of specific data, obtaining a sufficient number of observations remains a costly endeavor. Moreover, if our emphasis is on understanding the interactions and interdependencies among populations, experiments conducted solely on a single population may not provide conclusive evidence.
The joint progressive type-II censoring scheme (JPT-II-CS) provides notable benefits for comparing the lifespan distributions of products produced by different units within the same facility. JPT-II-CS has attracted considerable attention in the research community, with numerous authors exploring JPT-II-CS and related inference methods in the literature. For instance, Rasouli and Balakrishnan [32], Doostparast et al. [18], Balakrishnan et al. [15], Mondal and Kundu [26], Krishna and Goel [24], Goel and Krishna [21], and Goel and Krishna [22] have contributed to this body of work.
Recently, a multitude of researchers have investigated a range of strategies and different lifetime models in various applications. For more detailed information, please consult the publications by Pandey and Srivastava [29], Qiao and Gui [31], Abdel-Aty et al. [1], Ferreira and Silva [20], Celik and Guloksuz [16], Chiou and Chen [17], Panahi and Lone [28], Yan et al. [34], Asadi et al. [4,7,8,9,10,11,12].
Within the JPT-II-CS framework, two samples, one from Population-A (Pop-A) and the other from Population-B (Pop-B), each comprising m and n units, are amalgamated for a life-testing experiment. Let k be the total number of observed failures in this experiment. Moreover, let R1,R2,...,Rk signify the number of units removed, such that ∑ki=1(Ri+1)=m+n, where Ri=Si+Ti, and Ri is the sum of units removed from Pop-A (Si) and Pop-B (Ti) at the i-th stage. Considering the joint sample upon the first failure event denoted as W1, R1=S1+T1 units are randomly chosen from the remaining pool of m+n−1 surviving units. Here S1 and T1 represent the units removed from Population (A) and Population (B) respectively. In a similar fashion, at the second stage, R2=S2+T2 units are randomly selected from the remaining pool of m+n−2−R1 surviving units and so forth. Finally, at the k-th failure, all the remaining Rk=n+m−k−∑ki=1Ri surviving units are removed. It is crucial to highlight that when R1=R2=...=Rk=0, it implies that Si=Ti=0 for all i=1,2,...,k, simplifying the JPT-II-CS to the scenario of complete samples. Furthermore, if R1=R2=...=Rk−1=0, resulting in Rk=n+m−k, then the censoring scheme transforms back to a conventional Type-II censoring scheme for two distinct samples.
In the context of the JPT-II-CS framework, the observed data comprises (W,Z,S), where W is depicted as (W1,W2,...,Wk), where k is a specified integer such that 1⩽k<m+n. Z is denoted as (Z1,Z2,...,Zk), where
Zi={1if Wi drawn from the X-sample,0 if Wi drawn from the Y-sample . |
Moreover, S is depicted as (S1,S2,...,Sk). We employ k1 to indicate the number of failed units from Pop-A, and k2 to signify the count of failed units from Pop-B, where k1=∑ki=1Zi and k2=k−∑ki=1Zi, respectively.
The exponential distribution is renowned for its constant failure rate and memoryless property. However, when exploring phenomena in lifetime and reliability studies, opting for the exponential model may not be conducive, as it lacks the ability to exhibit both monotone (increasing and decreasing) and non-monotone (bathtub and upside-down bathtub) failure rate behaviors. To address this limitation and provide flexibility, alternative generalizations of the exponential distribution have been proposed, such as the Nadarajah–Haghighi distribution (NHD). Introduced by Nadarajah and Haghighi [27], this distribution serves as a widespread statistical extension of the conventional exponential distribution and has been recently named the NHD, abbreviating the authors' names. The cumulative distribution function (CDF) of the NHD is expressed as follows (see Figure 1):
F(x)=1−e[1−(1+αx)γ],x>0,α,γ>0. | (1.1) |
Additionally, its corresponding probability density function (PDF) is given by:
f(x)=αγ(1+αx)γ−1e[1−(1+αx)γ],x>0,α,γ>0. | (1.2) |
In this context, γ and α denote the shape and scale parameters, respectively. By setting γ=1 for the variable in Eq (1.1), the inverted exponential distribution is presented as a particular case. Nadarajah and Haghighi [27] demonstrated that the density of the NHD may exhibit a decreasing trend. Furthermore, its shapes, both unimodal and the hazard rate function, can resemble the increasing, decreasing, or constant patterns observed in gamma, Weibull, and generalized-exponential distributions.
Several researchers have delved into the estimation challenges associated with the NHD. For instance, Selim [33] investigated the estimation and prediction of the NHD based on record values. Inferences and ideal censoring techniques for the progressively first-failure censored NHD were covered by Ashour et al. [5]. Elshahhat et al. [19] used type-II adaptive progressive hybrid censoring with applications to investigate inferences for Nadarajah-Haghighighi parameters. The half logistic inverted NHD was studied by Alotaibi et al. [2] under ranked set Sampling with applications. With applications to COVID-19 mortality data and cancer data, Azimi and Esmailian [6] examined a novel generalisation of the NHD.
In this publication, we tackle the challenges associated with estimating the NHD. We present both Bayesian and maximum likelihood estimators (MLEs) for the NHD using the JPT-II-CS, accompanied by their corresponding confidence intervals. We derive Bayes estimators for the squared error (SE) and linear exponential (LINEX) loss functions, assuming independent gamma priors. Monte Carlo simulations are conducted to assess the performance of various estimators, evaluating them based on mean squared error (MSE) and average values. Additionally, we scrutinize the average confidence lengths of the 95% two-sided interval estimations to gauge their effectiveness. Finally, we illustrate the practical application of our approach using real-world datasets.
The following outlines the order of the remaining sections in the paper following this introduction: Section 2 examines the concept of sampling significance. Section 3 explains the process of obtaining maximum probability estimates for the unknown parameters. The construction of the Fisher information matrix (FIM) using MLEs is done in Section 4. In Section 5, Bayes estimation is performed with various loss functions using gamma and non-informative priors. Section 6 presents a Monte Carlo simulation analysis along with the results, serving as model validation. Two real data analyses to demonstrate each of the suggested methodologies are carried out in Section 7. Section 8 provides a concise summary of the main points.
In a particular context, we possess independent and identically distributed (iid) lifetimes, denoted as X1,X2,...,Xm, originating from Pop-A. These lifetimes adhere to a NHD characterized by the CDF F(x) and the PDF f(x). In the same vein, we possess Y1,Y2,...,Yn as iid lifetimes from Pop-B, also adhering to a NHD defined by the CDF G(y) and the PDF g(y). Given a JPT-II-CS (m,n);R1,R2,...,Rk, we can define B=(W,Z,S) as the JPT-II-CS of size k obtained from Pop-A and Pop-B. It comprises (w1,z1,s1),(w2,z2,s2),...,(wk,zk,sk). The likelihood function based on the observed JPT-II-CS can be expressed as follows:
L(α1,α2,γ1,γ2|data)=Cr∏i=1[[f(wi)]zi[g(wi)]1−zi][ˉF(wi)]si[ˉG(wi)]ti, | (2.1) |
where w1≤w2≤…≤wk, ˉF=1−F, ˉG=1−G, ∑ki=1si+∑ki=1ti=∑ki=1Ri \text{and} C=B1B2 with
B1=∏kj=1{(m−j−1∑i=1zi−j−1∑i=1si)zj+(n−j−1∑i=1(1−zi)−j−1∑i=1(Ri−si))(1−zj)}, |
B2=k∏j=1{(m−∑j−1i=1zi−∑j−1i=1sisi)(n−∑j−1i=1(1−zi)−∑j−1i=1(Ri−si)ti)(m+n−j−∑j−1i=1RiRi)}. |
MLE stands as a powerful statistical method widely employed in the field of data analysis and parameter estimation. In this manuscript, we employ MLE to estimate the parameters of the NHD. The main principle of MLE is to find the parameter values that maximize the likelihood function, which quantifies the probability of observing the given data under a specific set of parameter values. By maximizing the likelihood, we aim to identify the parameter values that make the observed data most plausible. The MLE estimates are obtained by solving an optimization problem, either analytically or numerically, to find the parameter values that maximize the likelihood function. These estimates are known to possess desirable properties, such as efficiency and consistency, which means that they converge to the true parameter values as the sample size increases. MLE is a versatile and widely applicable method that plays a crucial role in statistical inference and parameter estimation.
The following outcome is obtained by applying the CDF and PDF from Eqs (1.1) and (1.2) to the likelihood equation given in (2.1):
L(α1,α2,γ1,γ2|data)=αk11γk11αk22γk22e(γ1−1)∑ki=1ziln(1+α1wi)e∑ki=1zi[1−(1+α1wi)γ1]×e(γ2−1)∑ki=1(1−zi)ln(1+α2wi)e∑ki=1(1−zi)[1−(1+α2wi)γ2]×e∑ki=1si[1−(1+α1wi)γ1]e∑ki=1ti[1−(1+α2wi)γ2]. | (3.1) |
Given that the log-likelihood function exhibits the same monotonic behavior as the likelihood function, it is expressed as follows:
ℓ(α1,α2,γ1,γ2|data)=k1lnα1+k1lnγ1+k2lnα2+k2lnγ2+(γ1−1)k∑i=1ziln(1+α1wi)+k∑i=1zi[1−(1+α1wi)γ1]+(γ2−1)k∑i=1(1−zi)ln(1+α2wi)+k∑i=1(1−zi)[1−(1+α2wi)γ2]+k∑i=1si[1−(1+α1wi)γ1]+k∑i=1ti[1−(1+α2wi)γ2]. | (3.2) |
By partially differentiating Eq (3.2) with respect to α1,α2,γ1andγ2 and setting the derivatives equal to zero, the resulting equations are as follows.
k1α1+(γ1−1)k∑i=1ziwi(1+α1wi)−k∑i=1ziwiγ1(1+α1wi)γ1−1−k∑i=1siwiγ1(1+α1wi)γ1−1=0, | (3.3) |
k2α2+(γ2−1)k∑i=1(1−zi)wi(1+α2wi)−k∑i=1(1−zi)wiγ2(1+α2wi)γ2−1−k∑i=1tiwiγ2(1+α2wi)γ2−1=0, | (3.4) |
k1γ1+k∑i=1ziln(1+α1wi)−k∑i=1zi(1+α1wi)γ1ln(1+α1wi)−k∑i=1si(1+α1wi)γ1ln(1+α1wi)=0, | (3.5) |
k2γ2+k∑i=1(1−zi)ln(1+α2wi)−k∑i=1(1−zi)(1+α2wi)γ2ln(1+α2wi)−k∑i=1ti(1+α2wi)γ2ln(1+α2wi)=0. | (3.6) |
It's evident, as indicated in Eqs (3.3)–(3.6), that explicit expressions for the MLEs α1,α2,γ1, and γ2 are not available. Hence, we recommend using numerical iterative methods, like the Newton-Raphson procedure, to derive the values of α1,α2,γ1, and γ2.
We investigate approximate confidence intervals in this context for the unknown parameters (α1,α2,γ1,γ2), using large sample approximations of the MLEs, which are often known as asymptotic theory. We do this by using the observed FIM to calculate the MLEs' asymptotic variance for the unknown parameters. Next, the observed FIM is represented as:
I−1(α1,α2,γ1,γ2)=(−∂2ℓ∂α21−∂2ℓ∂α1∂α2−∂2ℓ∂α1∂γ1−∂2ℓ∂α1∂γ1−∂2ℓ∂α2∂α1−∂2ℓ∂α22−∂2ℓ∂α2∂γ1−∂2ℓ∂α2∂γ2−∂2ℓ∂γ1∂α1−∂2ℓ∂γ1∂α2−∂2ℓ∂γ21−∂2ℓ∂γ1∂γ2−∂2ℓ∂γ2∂α1−∂2ℓ∂γ2∂α2−∂2ℓ∂γ2∂γ1−∂2ℓ∂γ22)−1, | (4.1) |
I−1(α1,α2,γ1,γ2)=(^var(^α1)cov(^α1,^α2)cov(^α1,^γ1)cov(^α1,^γ2)cov(^α2,^α1)^var(^α2)cov(^α2,^γ1)cov(^α2,^γ2)cov(^γ1,^α1)cov(^γ1,^α2)^var(^γ1)cov(^γ1,^γ2)cov(^γ2,^α1)cov(^γ2,^α2)cov(^γ2,^γ1)^var(^γ2)). | (4.2) |
Derived from the log-likelihood function in (3.2), the following is evident:
−k1α21+(γ1−1)k∑i=1−2ziw2i(1+α1wi)(1+α1wi)2−k∑i=1ziw2iγ1(γ1−1)(1+α1wi)γ1−2−k∑i=1siw2iγ1(γ1−1)(1+α1wi)γ1−2=0, | (4.3) |
−k2α22+(γ2−1)k∑i=1−2(1−zi)w2i(1+α2wi)(1+α2wi)2−k∑i=1(1−zi)w2iγ2(γ2−1)(1+α2wi)γ2−2−k∑i=1tiw2iγ2(γ2−1)(1+α2wi)γ2−2=0, | (4.4) |
−k1γ21−k∑i=1zi(1+α1wi)α1[ln(1+α1wi)]2−k∑i=1si(1+α1wi)γ1[ln(1+α1wi)]2=0, | (4.5) |
−k2γ22−k∑i=1(1−zi)(1+α2wi)α2[ln(1+α2wi)]2−k∑i=1ti(1+α2wi)γ2[ln(1+α2wi)]2=0. | (4.6) |
Calculating the asymptotic confidence intervals (ACIs) for the parameters α1,α2,γ1, and γ2 is feasible by leveraging the asymptotic normality of the maximum likelihood estimators (MLEs). This enables us to estimate the (1−μ)100% ACIs for α1,α2,γ1, and γ2 in an approximate manner
(^α1±Zμ/2√^var(^α1)),(^α2±Zμ/2√^var(^α2)),(^γ1±Zμ/2√^var(^γ1))and(^γ2±Zμ/2√^var(^γ2)). | (4.7) |
Here, Zμ/2 represents the upper μ/2th percentile of the standard normal distribution.
In the field of reliability analysis, there are instances where classical estimation using the MLE approach may encounter challenges, particularly when the available data lacks sufficient sampling details. To address this issue, incorporating prior information in conjunction with Bayesian analysis proves beneficial. This section focuses on the Bayesian approach for estimating unknown parameters and deriving the corresponding credible intervals (CRIs).
In Bayesian statistical inference, the role of the prior distribution is pivotal as it represents our existing knowledge or beliefs regarding the parameters, facilitating a more accurate estimation of the posterior distribution. The selection of an appropriate prior distribution is crucial as it can influence the ultimate results of the inference. The gamma distribution, recognized for its flexibility and favorable properties, is a frequently chosen continuous probability distribution for Bayesian parameter priors. The parameters of the gamma distribution can be adjusted to accommodate diverse prior beliefs. Additionally, the gamma distribution exhibits conjugacy, signifying that when employed as a prior distribution, its product with the likelihood function remains a gamma distribution, simplifying posterior distribution calculations.
The choice of hyperparameter values, like any other parameter selection in Bayesian analysis, depends on various factors including prior knowledge, the characteristics of the data, and modeling considerations. Without specific details about the context of your simulation, I can provide some general considerations:
● Prior Knowledge: If you have prior information about the hyperparameters, such as from previous studies or domain expertise, you might set them based on that knowledge. The values could reflect your beliefs about the likely range or distribution of the true hyperparameters.
● Empirical Bayes: If you don't have strong prior knowledge, you might consider using an empirical Bayes approach. This involves estimating the hyperparameters from the data itself. In such cases, the hyperparameters are determined based on the observed data distribution.
● Conjugate Priors: If you choose hyperparameters that make your prior distribution conjugate to the likelihood, it can simplify computations. However, this choice might not always reflect your beliefs about the parameters.
● Non-Informative Priors: A common choice for hyperparameters, especially in the absence of strong prior information, is to set them to values that make the prior distribution non-informative or weakly informative. For instance, setting hyperparameters to achieve a flat or diffuse prior.
In this study, we assert that ci and di are both greater than 0, where i takes values 1, 2, 3, and 4. This results in the following relationships:
π1(α1)∝αc1−11e−d1α1,α1>0,c1,d1>0, |
π2(α2)∝αc2−12e−d2α2,α2>0,c2,d2>0, |
π3(γ1)∝γc3−11e−d3γ1,γ1>0,c3,d3>0. |
π4(γ2)∝γc4−12e−d4γ2,γ2>0,c4,d4>0. |
In this scenario, ci and di where i=1,2,3,4, are introduced to integrate prior information concerning the unidentified parameters. Consequently, the joint prior density function for α1, α2, γ1, and γ2 is structured as follows:
π(α1,α2,γ1,γ2)∝αc1−11αc2−12γc3−11γc4−12e−d1α1−d2α2−d3γ1−d4γ2. | (5.1) |
The posterior distribution is a fundamental concept in Bayesian statistics, playing a crucial role in the inference process. It represents the updated belief or knowledge about a parameter or set of parameters after taking into account both prior information and observed data. In Bayesian analysis, the posterior distribution is obtained by combining the prior distribution, which encapsulates our initial beliefs, with the likelihood function, which quantifies the probability of observing the data given the parameter values. The posterior distribution provides a comprehensive summary of uncertainty, allowing us to estimate parameters, make predictions, and conduct various analyses. It serves as a bridge between prior knowledge and observed evidence, enabling us to make informed decisions and update our understanding of the underlying phenomenon.
By merging Eqs (3.1) and (5.1), it becomes viable to represent the joint posterior density function for α1, α2, γ1 and γ2 as following
π∗(α1,α2,γ1,γ2|data)=π(α1,α2,γ1,γ2)L(α1,α2,γ1,γ2|data)∫∞0∫∞0∫∞0∫∞0π(α1,α2,γ1,γ2)L(α1,α2,γ1,γ2|data)dα1dα2dγ1dγ2. | (5.2) |
The joint posterior density function for α1, α2, γ1 and γ2 can be formulated as:
π∗(α1,α2,γ1,γ2|data)∝αk1+c1−11γk1+c2−11αk2+c3−12γk2+c4−12e(γ1−1)∑ki=1ziln(1+α1wi)×e∑ki=1zi[1−(1+α1wi)]γ1e(γ2−1)∑ki=1(1−zi)ln(1+α2wi)e∑ki=1(1−zi)[1−(1+α2wi)]γ2×e∑ki=1si[1−(1+α1wi)]γ1e∑ki=1ti[1−(1+α2wi)]γ2e−d1α1−d2α2−d3γ1−d4γ2. | (5.3) |
Evidently, owing to the nonlinear form of (5.3), there is no closed-form solution for the Bayes estimators of α1, α2, γ1, or γ2 when employing the SE and LINEX loss functions. Consequently, we recommend employing the Markov Chain Monte Carlo (MCMC) approach to acquire the Bayes estimates and establish the corresponding CRIs.
To generate samples using the MCMC approach, we must first determine the conditional posterior distributions of the unknown NHD parameters α1, α2, γ1 and γ2. The conditions are given by
π∗1(α1|α2,γ1,γ2)∝αk1+c1−11e−d1α1−∑ki=1ziln(1+α1wi)e∑ki=1zi[1−(1+α1wi)]γ1×e∑ki=1si[1−(1+α1wi)]γ1, | (5.4) |
π∗2(α2|α1,γ1,γ2)∝αk2+c2−12e−d2α2−∑ki=1(1−zi)ln(1+α2wi)e∑ki=1(1−zi)[1−(1+α2wi)]γ2×e∑ki=1ti[1−(1+α2wi)]γ2, | (5.5) |
π∗3(γ1|α1,α2,γ2)∝γk1+c3−11e−γ1[d3+∑ki=1ziln(1+α1wi)], | (5.6) |
π∗4(γ2|α1,α2,γ1)∝γk2+c4−12e−γ2[d4+∑ki=1(1−zi)ln(1+α2wi)]. | (5.7) |
From Eqs (5.6) and (5.7), it's apparent that the posterior densities of γ1 and γ2 follow the gamma distribution, given that
π∗3(γ1|α1,α2,γ2)∼Gamma(k1+c3,d3+k∑i=1ziln(1+α1wi)), | (5.8) |
and
π∗4(γ2|α1,α2,γ1)∼Gamma(k2+c4,d4+k∑i=1(1−zi)ln(1+α2wi)). | (5.9) |
It's evident that gamma densities can be used to generate random samples of γ1 and γ2. However, it's not feasible to algebraically simplify the density functions of π∗1(α1|α2,γ1,γ2) and π∗2(α2|α1,γ1,γ2) to well-known distributions. Therefore, obtaining samples directly through conventional procedures is not practical. Hence, we turn to the Markov Chain Monte Carlo (MCMC) approach and generate a sample using Gibbs sampling with the Metropolis-Hastings (M-H) algorithm (see Metropolis et al. [25] and Hastings [23]), employing a standard proposal, as described below.
● Step 1. Begin by employing the initial values (α(0)1,α(0)2,γ(0)1,γ(0)2).
● Step 2. Set j=1.
● Step 3. Generate γ(j)1 from Gamma (k1+c3,d3+∑ki=1ziln(1+α1wi)).
● Step 4. Generate γ(j)2 from Gamma (k2+c4,d4+∑ki=1(1−zi)ln(1+α2wi)).
● Step 5. Equations (5.4) and (5.5) can be employed to generate α(j)1 and α(j)2 using the Metropolis-Hastings (M-H) algorithm. The recommended normal distributions to use are N(α(j−1)1,var(α1)) and N(α(j−1)2,var(α2)). In this instance, the major diagonal of the inverted FIM can be utilized to calculate var(α1) and var(α2).
(I) Produce proposed values α∗1 and α∗2 from the corresponding normal distributions.\\ (II) Determine the acceptance probabilities using the following procedure:
r1=min[1,π∗1(α∗1|α(j−1)2,γj1,γj2,data)π∗1(α(j−1)1|α(j−1)2,γj1,γj2,data], |
r2=min[1,π∗2(α∗2|αj1,γj1,γj2,data)π∗2(α(j−1)2|αj1,γj1,γj2,data)]. |
(III) Produce a random value u from a uniform distribution within the range of (0,1).
(IV) If u≤r1, approve the proposal and assign α(j)1 as α∗1; otherwise, maintain α(j)1 as α(j−1)1.
(V) If u≤r2, approve the proposal and assign α(j)2 as α∗2; otherwise, maintain α(j)2 as α(j−1)2.
● Step 7. Set j = j + 1.
● Step 8. Repeat 2–7, for V times. Therefore, the estimated posterior means of (α1,α2,γ1,γ2) represented by λ under the squared error loss function, can be determined as:
ˆλBS=E[λ|x_]=1V−NV∑i=N+1λ(j). | (5.10) |
Lastly, calculate the Bayesian estimates of λ utilizing the LINEX loss function:
ˆλBL=−1aln[1V−NV∑i=N+1e−aλ(j)]. | (5.11) |
Here, N denotes the burn-in period.
This section's goal is to assess the potency of the various estimation techniques covered in earlier sections. We demonstrate this by examining an actual dataset and conducting a simulated experiment to evaluate the statistical performance of the estimators under the JPT-II-CS. The computations were carried out using Mathematica ver. 10 software.
In this section, we conduct simulation studies to assess the performance of the estimation methods developed in the preceding sections. We explore various sample sizes for the two populations, including (m,n) = (10,20),(20,30),(40,50), and different numbers of failures for each sample size, such as (15,20,30),(35,40,50),(60,70,90), respectively. The parameter values for the two populations are set as (α1,α2,γ1,γ2) = (1.5,1.3,0.4,0.3).
We computed MLEs along with 95% CIs for the parameters (α1,α2,γ1,γ2) across all specified scenarios. This process was repeated 1000 times, and we calculated the mean values of MLEs and their respective lengths. The results are presented in Tables 1–4. Additionally, we employed informative gamma priors for for α1, α2, γ1, and γ2 in the context of Bayesian estimation under SE and LINEX loss functions. The hyperparameters were set as ci=0.8 and di=2.5, where i=1,2,3,4, with a=3 denoting overestimation and a=−3 representing underestimation. We employed the(MCMC approach with 11,000 samples to derive Bayesian estimates for α1, α2, γ1, and γ2, along with 95% CRIs, through 1000 simulations. The initial 1000 values were excluded due to "burn-in". MSE was utilized to evaluate the performance of the generated estimators for α1, α2, γ1, and γ2. Following 1000 repetitions of this process, we computed the mean values of MLEs and their respective lengths. Tables 1–4 present the results.
(m,n) | r | Scheme | Non-Bayesian | Bayesian | |||||
MLE | Length | SE | LINEX | Length | |||||
a=−3 | a=3 | ||||||||
(10, 20) | 15 | (0(14),15) | 1.0089 | 7.7486 | 1.3852 | 1.3856 | 1.3849 | 0.0611 | |
(0.2412) | (0.0132) | (0.0131) | (0.0130) | ||||||
15 | (15,0(14)) | 1.9192 | 9.5216 | 2.5732 | 2.5736 | 2.5728 | 0.0604 | ||
(0.1757) | (1.1517) | (1.1526) | (1.1509) | ||||||
20 | (0(19),10) | 1.4528 | 8.1424 | 1.8099 | 1.8104 | 1.8095 | 0.0633 | ||
(0.0992) | (0.0961) | (0.0963) | (0.0958) | ||||||
20 | (10,0(19)) | 2.0877 | 9.2575 | 1.8322 | 1.8343 | 1.8302 | 0.1077 | ||
(0.3454) | (0.1104) | (0.1118) | (0.1090) | ||||||
25 | (0(7),2,0(4),1,0(3),2,0(8)) | 1.6978 | 6.5167 | 2.3954 | 2.3955 | 2.3952 | 0.0363 | ||
(0.0391) | (0.0175) | (0.0191) | (0.0144) | ||||||
30 | (0(30)) | 0.9524 | 4.0016 | 1.5637 | 1.5639 | 1.5635 | 0.0398 | ||
(0.2999) | (0.0041) | (0.0041) | (0.0040) | ||||||
(20, 30) | 35 | (0(34),15) | 1.4461 | 5.6789 | 1.7213 | 1.7214 | 1.7213 | 0.0272 | |
(0.0029) | (0.0490) | (0.0490) | (0.0490) | ||||||
35 | (15,0(34)) | 1.2765 | 3.9130 | 1.8908 | 1.8909 | 1.8908 | 0.0223 | ||
(0.1500) | (0.0527) | (0.0527) | (0.0527) | ||||||
40 | (0(39),10) | 1.9292 | 5.5891 | 1.2361 | 1.2366 | 1.2356 | 0.0540 | ||
(0.1843) | (0.0697) | (0.0685) | (0.0672) | ||||||
40 | (10,0(39)) | 1.9814 | 5.9534 | 1.8851 | 1.8855 | 1.8847 | 0.0601 | ||
(0.2318) | (0.1483) | (0.1486) | (0.1480) | ||||||
45 | (0(5),3,0(33),2,0(5)) | 1.3855 | 4.2144 | 0.7017 | 0.7025 | 0.7008 | 0.0709 | ||
(0.2131) | (0.0637) | (0.0534) | (0.0544) | ||||||
50 | (0(50)) | 1.7681 | 4.4709 | 1.8890 | 1.8890 | 1.8890 | 0.0261 | ||
(0.0719) | (0.0513) | (0.0514) | (0.0512) | ||||||
(40, 50) | 60 | (0(59),30) | 2.0131 | 6.0591 | 2.8808 | 2.8806 | 2.8802 | 0.0311 | |
(0.2633) | (0.0684) | (0.0688) | (0.0679) | ||||||
60 | (30,0(59)) | 0.8016 | 2.2477 | 0.5408 | 0.5407 | 0.5405 | 0.0130 | ||
(0.4878) | (0.0400) | (0.0321) | (0.201) | ||||||
70 | (0(69),20) | 0.8469 | 1.9784 | 0.6599 | 0.6599 | 0.6598 | 0.0164 | ||
(0.4265) | (0.1058) | (0.1057) | (0.1052) | ||||||
70 | (20,0(69)) | 1.0524 | 2.3534 | 1.2987 | 1.2987 | 1.2986 | 0.0140 | ||
(0.2003) | (0.0405) | (0.0402) | (0.0401) | ||||||
80 | (4,0(8),3,0(60),3,0(9)) | 2.4501 | 5.8484 | 4.4654 | 4.4656 | 4.4651 | 0.0423 | ||
(0.9027) | (0.7933) | (0.7922) | (0.7824) | ||||||
90 | (0(90)) | 1.8013 | 3.3309 | 1.6733 | 1.6734 | 1.6731 | 0.0265 | ||
(0.0908) | (0.0765) | (0.0768) | (0.0763) |
(m,n) | r | Scheme | Non-Bayesian | Bayesian | |||||
MLE | Length | SE | LINEX | Length | |||||
a=−3 | a=3 | ||||||||
(10, 20) | 15 | (0(14),15) | 0.9247 | 7.4446 | 1.3417 | 1.3422 | 1.3413 | 0.0636 | |
(0.1409) | (0.0017) | (0.0018) | (0.0017) | ||||||
15 | (15,0(14)) | 0.1539 | 0.9332 | 0.1811 | 0.1811 | 0.1811 | 0.0097 | ||
(0.3134) | (0.2519) | (0.2519) | (0.2519) | ||||||
20 | (0(19),10) | 0.6347 | 3.2751 | 0.5126 | 0.5127 | 0.5126 | 0.0150 | ||
(0.4426) | (0.6200) | (0.6210) | (0.6120) | ||||||
20 | (10,0(19)) | 2.6140 | 8.4100 | 2.8049 | 2.8055 | 2.8043 | 0.0712 | ||
(0.7267) | (0.2647) | (0.2664) | (0.2629) | ||||||
25 | (0(7),2,0(4),1,0(3),2,0(8)) | 0.6021 | 2.473 | 0.8028 | 0.8029 | 0.8028 | 0.0268 | ||
(0.4871) | (0.2472) | (0.2471) | (0.2470) | ||||||
30 | (0(30)) | 1.7301 | 5.7329 | 2.7105 | 2.7107 | 2.7103 | 0.0599 | ||
(0.1850) | (0.1795) | (0.1702) | (0.1601) | ||||||
(20, 30) | 35 | (0(34),15) | 0.3714 | 1.8375 | 1.3453 | 1.3453 | 1.3453 | 0.0082 | |
(0.1623) | (0.0021) | (0.0021) | (0.0021) | ||||||
35 | (15,0(34)) | 1.8284 | 5.0672 | 1.4205 | 1.4208 | 1.4202 | 0.0544 | ||
(0.2792) | (0.0145) | (0.0146) | (0.0144) | ||||||
40 | (0(39),10) | 2.0993 | 5.2002 | 0.9454 | 0.9455 | 0.9453 | 0.0287 | ||
(0.6390) | (0.1257) | (0.1250) | (0.1248) | ||||||
40 | (10,0(39)) | 2.5850 | 8.5699 | 2.4874 | 2.4876 | 2.4872 | 0.0414 | ||
(0.6512) | (0.4099) | (0.4095) | (0.4090) | ||||||
45 | (0(5),3,0(33),2,0(5)) | 2.649 | 7.6263 | 1.4086 | 1.4088 | 1.4083 | 0.0468 | ||
(0.8198) | (0.0118) | (0.0118) | (0.0117) | ||||||
50 | (0(50)) | 2.1930 | 5.6644 | 1.8755 | 1.8482 | 1.8655 | 0.0350 | ||
(0.7975) | (0.3469) | (0.3471) | (0.3468) | ||||||
(40, 50) | 60 | (0(59),30) | 1.0377 | 3.2544 | 1.5236 | 1.5236 | 1.5236 | 0.0207 | |
(0.0688) | (0.0500) | (0.0500) | (0.0500) | ||||||
60 | (30,0(59)) | 2.1438 | 4.4834 | 2.4051 | 2.4052 | 2.4050 | 0.0370 | ||
(0.7120) | (0.2213) | (0.2217) | (0.2210) | ||||||
70 | (0(69),20) | 0.969 | 2.4503 | 1.7391 | 1.7391 | 1.7391 | 0.0141 | ||
(0.1096) | (0.0928) | (0.0924) | (0.0920) | ||||||
70 | (20,0(69)) | 1.5667 | 3.193 | 1.7013 | 1.7012 | 1.7010 | 0.0154 | ||
(0.0712) | (0.0161) | (0.0162) | (0.0158) | ||||||
80 | (4,0(8),3,0(60),3,0(9)) | 1.3662 | 2.709 | 1.6230 | 1.6222 | 1.6220 | 0.0167 | ||
(0.1244) | (0.1043) | (0.1041) | (0.1032) | ||||||
90 | (0(90)) | 1.4794 | 2.6736 | 0.8824 | 0.8820 | 0.8811 | 0.0112 | ||
(0.0322) | (0.0174) | (0.0171) | (0.0160) |
(m,n) | r | Scheme | Non-Bayesian | Bayesian | |||||
MLE | Length | SE | LINEX | Length | |||||
a=−3 | a=3 | ||||||||
(10, 20) | 15 | (0(14),15) | 0.4500 | 2.0736 | 1.0201 | 1.2450 | 0.8765 | 1.3259 | |
(0.0025) | (0.0023) | (0.0020) | (0.0020) | ||||||
15 | (15,0(14)) | 0.3766 | 0.8338 | 0.5666 | 0.7000 | 0.4843 | 1.0085 | ||
(0.0201) | (0.0270) | (0.0278) | (0.0268) | ||||||
20 | (0(19),10) | 0.3205 | 0.8781 | 0.3128 | 0.2280 | 0.2623 | 0.8047 | ||
(0.1963) | (0.1108) | (0.1832) | (0.0688) | ||||||
20 | (10,0(19)) | 0.4035 | 0.7331 | 0.6691 | 0.7739 | 0.5954 | 0.9246 | ||
(0.0854) | (0.0724) | (0.0724) | (0.0382) | ||||||
25 | (0(7),2,0(4),1,0(3),2,0(8)) | 0.5471 | 1.0630 | 0.8072 | 0.9125 | 0.7279 | 0.9644 | ||
(0.0216) | (0.0165) | (0.0127) | (0.0107) | ||||||
30 | (0(30)) | 0.6514 | 1.3684 | 0.745 | 0.8343 | 0.6765 | 0.8887 | ||
(0.1632) | (0.1191) | (0.1286) | (0.0765) | ||||||
(20, 30) | 35 | (0(34),15) | 0.7796 | 0.7379 | 0.7796 | 0.8391 | 0.7307 | 0.7379 | |
(0.1291) | (0.1241) | (0.1028) | (0.1094) | ||||||
35 | (15,0(34)) | 0.4192 | 0.5150 | 0.5787 | 0.6148 | 0.5477 | 0.5777 | ||
(0.1010) | (0.0320) | (0.0461) | (0.0461) | ||||||
40 | (0(39),10) | 0.2902 | 0.3452 | 0.8947 | 0.9753 | 0.8295 | 0.8497 | ||
(0.2144) | (0.0125) | (0.0122) | (0.0121) | ||||||
40 | (10,0(39)) | 0.4189 | 0.4923 | 0.4864 | 0.4291 | 0.4499 | 0.2145 | ||
(0.0930) | (0.0820) | (0.0724) | (0.0620) | ||||||
45 | (0(5),3,0(33),2,0(5)) | 0.3938 | 0.5113 | 0.4844 | 0.4782 | 0.4653 | 0.3813 | ||
(0.434) | (0.0354) | (0.0352) | (0.0344) | ||||||
50 | (0(50)) | 0.3731 | 0.3433 | 0.4990 | 0.4265 | 0.4744 | 0.3129 | ||
(0.0898) | (0.0396) | (0.0394) | (0.0390) | ||||||
(40, 50) | 60 | (0(59),30) | 0.2999 | 0.4200 | 0.5524 | 0.4799 | 0.5277 | 0.3152 | |
(0.0954) | (0.0779) | (0.0462) | (0.0563) | ||||||
60 | (30,0(59)) | 0.6208 | 0.8487 | 0.5244 | 0.5301 | 0.5030 | 0.4250 | ||
(0.0488) | (0.0244) | (0.0223) | (0.0222) | ||||||
70 | (0(69),20) | 0.4048 | 0.4479 | 0.3692 | 0.3196 | 0.3245 | 0.1888 | ||
(0.4478) | (0.1177) | (0.3900) | (0.3548) | ||||||
70 | (20,0(69)) | 0.6135 | 0.6612 | 0.6751 | 0.6168 | 0.6376 | 0.4304 | ||
(0.0456) | (0.0258) | (0.0671) | (0.0915) | ||||||
80 | (4,0(8),3,0(60),3,0(9)) | 0.3866 | 0.3552 | 0.5170 | 0.5274 | 0.5070 | 0.3215 | ||
(0.0212) | (0.0137) | (0.0162) | (0.0115) | ||||||
90 | (0(90)) | 0.3746 | 0.2523 | 0.4336 | 0.4443 | 0.4234 | 0.2224 | ||
(0.1064) | (0.0178) | (0.0208) | (0.0152) |
(m,n) | r | Scheme | Non-Bayesian | Bayesian | |||||
MLE | Length | SE | LINEX | Length | |||||
a=−3 | a=3 | ||||||||
(10, 20) | 15 | (0(14),15) | 0.4110 | 2.0336 | 1.0052 | 1.2843 | 0.8484 | 1.4092 | |
(0.0123) | (0.0119) | (0.0112) | (0.0101) | ||||||
15 | (15,0(14)) | 0.8193 | 2.8032 | 1.0506 | 1.2216 | 0.9307 | 1.2030 | ||
(0.2696) | (0.3634) | (0.4493) | (0.3978) | ||||||
20 | (0(19),10) | 0.5335 | 1.5963 | 1.3381 | 1.6395 | 1.1490 | 1.5159 | ||
(0.0545) | (0.0777) | (0.7941) | (0.7208) | ||||||
20 | (10,0(19)) | 0.2255 | 0.2141 | 0.3820 | 0.3992 | 0.3666 | 0.4006 | ||
(0.0085) | (0.0067) | (0.0078) | (0.0044) | ||||||
25 | (0(7),2,0(4),1,0(3),2,0(8)) | 0.4095 | 0.6813 | 0.6038 | 0.6416 | 0.5715 | 0.5963 | ||
(0.0120) | (0.0092) | (0.0081) | (0.0073) | ||||||
30 | (0(30)) | 0.2772 | 0.2753 | 0.3940 | 0.4056 | 0.3833 | 0.3367 | ||
(0.0188) | (0.0088) | (0.0075) | (0.0069) | ||||||
(20, 30) | 35 | (0(34),15) | 0.7089 | 2.2453 | 0.8012 | 0.8529 | 0.7567 | 0.6981 | |
(0.1672) | (0.1512) | (0.1057) | (0.1086) | ||||||
35 | (15,0(34)) | 0.2850 | 0.2466 | 0.5229 | 0.5426 | 0.5049 | 0.4333 | ||
(0.1491) | (0.0497) | (0.0589) | (0.0420) | ||||||
40 | (0(39),10) | 0.3109 | 0.3064 | 0.3965 | 0.3623 | 0.3410 | 0.2909 | ||
(0.5851) | (0.4866) | (0.4851) | (0.4840) | ||||||
40 | (10,0(39)) | 0.3604 | 0.2478 | 0.3265 | 0.3382 | 0.3156 | 0.2302 | ||
(0.1458) | (0.0160) | (0.0191) | (0.0134) | ||||||
45 | (0(5),3,0(33),2,0(5)) | 0.6419 | 0.6039 | 0.5232 | 0.5379 | 0.5095 | 0.3788 | ||
(0.3430) | (0.0498) | (0.0566) | (0.0439) | ||||||
50 | (0(50)) | 0.2571 | 0.1927 | 0.2427 | 0.2527 | 0.2332 | 0.1163 | ||
(0.1144) | (0.0204) | (0.0233) | (0.0177) | ||||||
(40, 50) | 60 | (0(59),30) | 0.4642 | 0.7702 | 0.9308 | 0.9680 | 0.8969 | 0.6030 | |
(0.0270) | (0.0179) | (0.0171) | (0.0165) | ||||||
60 | (30,0(59)) | 0.2766 | 0.2781 | 0.2510 | 0.2591 | 0.2430 | 0.1898 | ||
(0.1222) | (0.0228) | (0.0254) | (0.0204) | ||||||
70 | (0(69),20) | 0.3746 | 0.4258 | 0.3499 | 0.3682 | 0.3329 | 0.2235 | ||
(0.4441) | (0.1225) | (0.1356) | (0.1108) | ||||||
70 | (20,0(69)) | 0.2747 | 0.1723 | 0.4435 | 0.4506 | 0.4368 | 0.2654 | ||
(0.1324) | (0.0206) | (0.0227) | (0.0187) | ||||||
80 | (4,0(8),3,0(60),3,0(9)) | 0.6604 | 0.5647 | 0.5858 | 0.5983 | 0.5741 | 0.3527 | ||
(0.1204) | (0.0817) | (0.0890) | (0.0751) | ||||||
90 | (0(90)) | 0.2699 | 0.1466 | 0.2436 | 0.2524 | 0.2351 | 0.1170 | ||
(0.1100) | (0.0593) | (0.0637) | (0.0553) |
Based on the aforementioned data, several conclusions can be drawn:
(1) Tables 1 to 4 reveal that, in most cases, Bayesian estimates outperform MLEs in terms of MSEs.
(2) Examining Tables 1 to 4, it becomes apparent that CRIs exhibit the shortest average length of intervals compared to the average length of CIs, indicating the superiority of Bayesian estimators over MLEs.
(3) Notably, Bayesian estimation using the LINEX loss function at a=3 surpasses Bayesian estimators using the LINEX loss function at a=−3 and the SE loss function in terms of MSEs.
(4) The results indicate that both MLEs and Bayesian estimators yield favorable outcomes for two sample lines, whether they have the same or different population numbers, suggesting the model's suitability for various sample situations.
The data represent the air-conditioning system failure times (in hours) for planes 7913 and 7914, originally sourced from Proschan [30]. The assumption is made that the two datasets are independent, and within each dataset, the failure times are also considered independent. The data is provided below.
Data 1. (Plane 7913): 1, 4, 11, 16, 18, 18, 18, 24, 31, 39, 46, 51, 54, 63, 68, 77, 80, 82, 97, 106, 111, 141, 142, 163, 191, 206, 216.
Data 2. (Plane 7914): 3, 5, 5, 13, 14, 15, 22, 22, 23, 30, 36, 39, 44, 46, 50, 72, 79, 88, 97, 102, 139, 188, 197, 210.
Table 5 displays the outcomes of the Kolmogorov-Smirnov (K-S) test, employed to assess the data's adherence to the NHD.
Data set | Size (n) | K-S (Calculated) | K-S (5% Significance) | P-value |
I | 27 | 0.1011 | 0.2544 | 0.9192 |
II | 24 | 0.0870 | 0.2693 | 0.9858 |
Taking into account the details in Table 5, it is apparent that the computed K-S values for the data are lower than the corresponding values expected at a significance level of 5%. Additionally, we have noted notably high P-values. Consequently, we can reasonably conclude that the NHD serves as a well-fitted model for the data. Moreover, we have generated empirical S(x) and fitted S(x) for each dataset, as depicted in Figures 2 and 3, respectively. These plots provide additional confirmation that the NHD model offers a superior fit to the data.
We generated a JPT-IISC sample from the aforementioned datasets using the following censoring scheme. For the first sample, set m=27, and for the second sample, set n=24, implementing JPT-IISC with r=20. The censoring vectors are defined as:
S=(0,0,0,0,0,0,0,0,0,0,0,0,0,0,0,0,0,0,0,16),R=(0,0,0,0,0,0,0,0,0,0,0,0,0,0,0,0,0,0,0,31),T=(0,0,0,0,0,0,0,0,0,0,0,0,0,0,0,0,0,0,0,15). |
Here are the generated datasets:
w=(1,3,4,5,5,11,13,14,15,16,18,18,18,22,22,23,24,30,31,36),z=(1,0,1,0,0,1,0,0,0,1,1,1,1,0,0,0,1,0,1,0). |
We obtained estimates for α1,α2,γ1, and γ2 using the MLE method, depending on the data type used in this study. The corresponding results are displayed in Table 6, whereas Tables 7 and 8 present the 95% ACIs for α1,α2,γ1, and γ2. We utilized the MCMC method for Bayesian estimation conducting 20000 iterations to ensure convergence we excluded the initial 5000 iterations as 'burn in' We have selected hyperparameters ci and di as 0.0001, approaching values near zero for the prior distributions. Bayesian estimates for α1,α2,γ1, and γ2 were derived using both the SE loss and LINEX loss functions, with the corresponding results detailed in Table 6. Additionally, the 95% CRIs for α1,α2,γ1, and γ2 are provided in Tables 7 and 8.
Parameters | MLE | MCMC | |||
SE | LINEX | ||||
a=−3.0 | a=10−4 | a=3.0 | |||
α1 | 0.0211 | 0.0612 | 0.0612 | 0.0612 | 0.0612 |
α2 | 0.0112 | 0.0406 | 0.0406 | 0.0406 | 0.0406 |
γ1 | 0.6548 | 1.5915 | 2.2824 | 1.5915 | 1.2740 |
γ2 | 1.2882 | 2.0091 | 2.8235 | 2.0091 | 1.6094 |
Method | α1 | α2 | |||||
Lower | Upper | Length | Lower | Upper | Length | ||
CI | -0.0801 | 0.1223 | 0.2024 | -0.0392 | 0.0615 | 0.1008 | |
CRI | 0.0606 | 0.0621 | 0.0014 | 0.0401 | 0.0411 | 0.0010 |
Method | γ1 | γ2 | |||||
Lower | Upper | Length | Lower | Upper | Length | ||
CI | -1.7852 | 3.0948 | 4.8801 | -3.6776 | 6.2541 | 9.9317 | |
CRI | 0.7213 | 2.8120 | 2.0906 | 1.0133 | 3.3537 | 2.3404 |
In this paper, we explored statistical inference for two populations characterized by NHD. These distributions feature distinct shape and scale parameters, and our analysis focused on a JPT-II-CS. Assuming life distribution for both populations, we derived maximum likelihood estimates for unknown parameters and performed Bayesian estimation under gamma and non-information priors. Two loss functions, namely the SE and LINEX loss functions, were employed. To assess the effectiveness of the proposed estimates, we conducted Monte Carlo simulation experiments, revealing that Bayes estimates, along with their associated CRIs, outperform other estimators. Finally, we presented a numerical example to illustrate the inferential results established in this study.
The authors declare they have not used Artificial Intelligence (AI) tools in the creation of this article.
This research project was supported by the Researchers Supporting Project Number (RSP2024R488), King Saud University, Riyadh, Saudi Arabia.
The authors declare no conflict of interest.
[1] |
van Giezen A, Wiegmans B (2020) Spoilt-Ocean Cleanup: Alternative logistics chains to accommodate plastic waste recycling: An economic evaluation. Transp Res Interdiscip Perspect 5: 100115. https://doi.org/10.1016/j.trip.2020.100115 doi: 10.1016/j.trip.2020.100115
![]() |
[2] |
Nikiema J, Asiedu Z (2022) A review of the cost and effectiveness of solutions to address plastic pollution. Environ Sci Pollut R 29: 24547–24573. https://doi.org/10.1007/s11356-021-18038-5 doi: 10.1007/s11356-021-18038-5
![]() |
[3] |
Stefaniak AB, Bowers LN, Cottrell G, et al. (2022) Towards sustainable additive manufacturing: The need for awareness of particle and vapor releases during polymer recycling, making filament, and fused filament fabrication 3-D printing. Resour Conserv Recycl 176: 105911. https://doi.org/10.1016/j.resconrec.2021.105911 doi: 10.1016/j.resconrec.2021.105911
![]() |
[4] | Zander NE (2019) Recycled polymer feedstocks for material extrusion additive manufacturing, In: Seppala EJ, Kotula AP, Snyder CR, Polymer-Based Additive Manufacturing: Recent Developments, Washington DC: ACS Publications, 37–51. https://doi.org/10.1021/bk-2019-1315.ch003 |
[5] | Rosli NA, Ahmad I (2021) Mechanical properties of recycled plastics, Recent Developments in Plastic Recycling, Singapore: Springer, 239–258. https://doi.org/10.1007/978-981-16-3627-1_11 |
[6] |
Kuclourya T, Monroy R, Cuan-Urquizo E, et al. (2022) Scientometric analysis and critical review of fused deposition modeling in the plastic recycling context. Clean Waste Syst 2: 100008. https://doi.org/10.1016/j.clwas.2022.100008 doi: 10.1016/j.clwas.2022.100008
![]() |
[7] |
Garcia JM, Robertson ML (2017) The future of plastics recycling. Science 358: 870–872. https://doi.org/10.1126/science.aaq0324 doi: 10.1126/science.aaq0324
![]() |
[8] |
Attaran M (2017) The rise of 3-D printing: The advantages of additive manufacturing over traditional manufacturing. Bus Horiz 60: 677–688. https://doi.org/10.1016/j.bushor.2017.05.011 doi: 10.1016/j.bushor.2017.05.011
![]() |
[9] |
Lemu HG (2012) Study of capabilities and limitations of 3D printing technology. AIP Conf Proc 1431: 857–865. https://doi.org/10.1063/1.4707644 doi: 10.1063/1.4707644
![]() |
[10] |
Fenollosa F, Uceda R, Tejo A, et al. (2021) Research on desktop 3D Printing Multi-Material New Concepts. IOP Conf Ser Mater Sci Eng 1193: 012043. https://doi.org/10.1088/1757-899X/1193/1/012043 doi: 10.1088/1757-899X/1193/1/012043
![]() |
[11] |
Netto JMJ, Idogava HT, Santos LEF, et al. (2021) Screw-assisted 3D printing with granulated materials: A systematic review. Int J Adv Manuf Tech 115: 2711–2727. https://doi.org/10.1007/s00170-021-07365-z doi: 10.1007/s00170-021-07365-z
![]() |
[12] |
Netto JMJ, Silveira ZC (2018) Design of an innovative three-dimensional print head based on twin-screw extrusion. J Mech Design 140: 125002. https://doi.org/10.1115/1.4041175 doi: 10.1115/1.4041175
![]() |
[13] |
Feuerbach T, Thommes M (2021) Design and characterization of a screw extrusion hot-end for fused deposition modeling. Molecules 26: 590. https://doi.org/10.3390/molecules26030590 doi: 10.3390/molecules26030590
![]() |
[14] |
Johann KS, Reißing A, Bonten C (2022) Comparative analysis of the solid conveying of regrind, virgin and powdery polyolefins in single-screw extrusion. J Manuf Mater Process 6: 56. https://doi.org/10.3390/jmmp6030056 doi: 10.3390/jmmp6030056
![]() |
[15] |
Khondoker MAH, Sameoto D (2019) Direct coupling of fixed screw extruders using flexible heated hoses for FDM printing of extremely soft thermoplastic elastomers. Prog Addit Manuf 4: 197–209. https://doi.org/10.1007/s40964-019-00088-4 doi: 10.1007/s40964-019-00088-4
![]() |
[16] |
Alexandre A, Sanchez FAC, Boudaoud H, et al. (2020) Mechanical properties of direct waste printing of polylactic acid with universal pellets extruder: comparison to fused filament fabrication on open-source desktop three-dimensional printers. 3D Print Addit Manuf 7: 237–247. https://doi.org/10.1089/3dp.2019.0195 doi: 10.1089/3dp.2019.0195
![]() |
[17] |
Kumar N, Jain PK, Tandon P, et al. (2018) Extrusion-based additive manufacturing process for producing flexible parts. J Braz Soc Mech Sci Eng 40: 1–12. https://doi.org/10.1007/s40430-018-1068-x doi: 10.1007/s40430-018-1068-x
![]() |
[18] |
Reddy BV, Reddy NV, Ghosh A (2007) Fused deposition modelling using direct extrusion. Virtual Phys Prototyp 2: 51–60. https://doi.org/10.1080/17452750701336486 doi: 10.1080/17452750701336486
![]() |
[19] |
Leng J, Wu J, Chen N, et al. (2019) The development of a conical screw-based extrusion deposition system and its application in fused deposition modeling with thermoplastic polyurethane. Rapid Prototyp J 26: 409–417. https://doi.org/10.1108/RPJ-05-2019-0139 doi: 10.1108/RPJ-05-2019-0139
![]() |
[20] |
Canessa E, Baruzzo M, Fonda C (2017) Study of Moineau-based pumps for the volumetric extrusion of pellets. Addit Manuf 17: 143–150. https://doi.org/10.1016/j.addma.2017.08.015 doi: 10.1016/j.addma.2017.08.015
![]() |
[21] |
Magnoni P, Rebaioli L, Fassi I, et al. (2017) Robotic AM system for plastic materials: tuning and on-line adjustment of process parameters. Procedia Manuf 11: 346–354. https://doi.org/10.1016/j.promfg.2017.07.117 doi: 10.1016/j.promfg.2017.07.117
![]() |
[22] |
Woern AL, Byard DJ, Oakley RB, et al. (2018) Fused particle fabrication 3-D printing: Recycled materials' optimization and mechanical properties. Materials 11: 1413. https://doi.org/10.3390/ma11081413 doi: 10.3390/ma11081413
![]() |
[23] |
Whyman S, Arif KM, Potgieter J (2018) Design and development of an extrusion system for 3D printing biopolymer pellets. Int J Adv Manuf Technol 96: 3417–3428. https://doi.org/10.1007/s00170-018-1843-y doi: 10.1007/s00170-018-1843-y
![]() |
[24] | Valkenaers H, Vogeler F, Ferraris E, et al. (2013) A novel approach to additive manufacturing: screw extrusion 3D-printing. Proceedings of the 10th International Conference on Multi-Material Micro Manufacture, Singapore, 235–238. https://doi.org/10.3850/978-981-07-7247-5-359 |
[25] |
Bellini A, Güçeri S, Bertoldi M (2004) Liquefier dynamics in fused deposition. J Manuf Sci Eng 126: 237–246. https://doi.org/10.1115/1.1688377 doi: 10.1115/1.1688377
![]() |
[26] |
Chiruvella RV, Jaluria Y, Sernas V, et al. (1996) Extrusion of non‐Newtonian fluids in a single‐screw extruder with pressure back flow. Polym Eng Sci 36: 358–367. https://doi.org/10.1002/pen.10422 doi: 10.1002/pen.10422
![]() |
[27] | Eslami H, Understanding screw design for film extrusion process. Macro Engineering and Technology, 2014. Available from: http://www.macroeng.com/understanding-screw-design-for-film-extrusion-process.php. |
[28] |
Pitayachaval P, Watcharamaisakul P (2019) A review of a machine design of chocolate extrusion based co-rotating twin screw extruder. IOP Conf Ser Mater Sci Eng 703: 012012. https://doi.org/10.1088/1757-899X/703/1/012012 doi: 10.1088/1757-899X/703/1/012012
![]() |
[29] | Osswald TA (2017) Understanding Polymer Processing, 2Eds., München: Hanser. https://doi.org/10.3139/9781569906484.fm |
[30] |
Netto JMJ, Silveira ZC (2018) Design of an innovative three-dimensional print head based on twin-screw extrusion. J Mech Design 140: 125002. https://doi.org/10.1115/1.4041175 doi: 10.1115/1.4041175
![]() |
[31] |
Sikora JW, Samujlo B, Stasiek A, et al. (2015) The mechanical properties of plasticized PVC processed in an extruder with a modified feed zone. Int Polym Proc 30: 359–365. https://doi.org/10.3139/217.3040 doi: 10.3139/217.3040
![]() |
[32] |
Bereaux Y, Charmeau JY, Moguedet M (2009) A simple model of throughput and pressure development for single screw. J Mater Process Tech 209: 611–618. https://doi.org/10.1016/j.jmatprotec.2008.02.070 doi: 10.1016/j.jmatprotec.2008.02.070
![]() |
[33] | Frankland J, Extrusion: How much L/D do you really need? Plastics Technology, 2011. Available from: https://www.ptonline.com/articles/how-much-ld-do-you-really-need. |
[34] |
Garcia D, Balart R, Sánchez L, et al. (2007) Compatibility of recycled PVC/ABS blends. Effect of previous degradation. Polym Eng Sci 47: 789–796. https://doi.org/10.1002/pen.20755 doi: 10.1002/pen.20755
![]() |
[35] |
Housz JFI, Meijer HEH (1981) The melting performance of single screw extruders. Polym Eng Sci 21: 352–359. https://doi.org/10.1002/pen.760210606 doi: 10.1002/pen.760210606
![]() |
[36] | Mount EM (2017) Extrusion processes, Applied Plastics Engineering Handbook, Norwich: William Andrew Publishing, 217–264. https://doi.org/10.1016/B978-0-323-39040-8.00012-2 |
[37] |
Shankar TJ, Sokhansanj S, Bandyopadhyay S, et al. (2010) A case study on optimization of biomass flow during single-screw extrusion cooking using genetic algorithm (GA) and response surface method (RSM). Food Bioproc Tech 3: 498–510. https://doi.org/10.1007/s11947-008-0172-9 doi: 10.1007/s11947-008-0172-9
![]() |
[38] |
Sokhey AS, Ali Y, Hanna MA (1997) Effects of die dimensions on extruder performance. J Food Eng 31: 251–261. https://doi.org/10.1016/S0260-8774(96)00025-8 doi: 10.1016/S0260-8774(96)00025-8
![]() |
[39] |
Sikora JW, Sasimowski E (2005) Influence of the length of the plasticating system on selected characteristics of an autothermal extrusion process. Adv Polym Tech 24: 21–28. https://doi.org/10.1002/adv.20021 doi: 10.1002/adv.20021
![]() |
[40] |
Chevanan N, Rosentrater KA, Muthukumarappan K (2010) Effects of processing conditions on single screw extrusion of feed ingredients containing DDGS. Food Bioproc Tech 3: 111–120. https://doi.org/10.1007/s11947-008-0065-y doi: 10.1007/s11947-008-0065-y
![]() |
[41] |
Vincelette AR, Guerrero CS, Carreau PJ, et al. (1989) A model for single-screw plasticating extruders. Int Polym Proc 4: 232–241. https://doi.org/10.3139/217.890232 doi: 10.3139/217.890232
![]() |
[42] |
Rauwendaal C (2004) Finite element studies of flow and temperature evolution in single screw extruders. Plast Rubber Compos 33: 390–396. https://doi.org/10.1179/174328904X24880 doi: 10.1179/174328904X24880
![]() |
[43] |
Chiruvella RV, Jaluria Y, Karwe MV (1996) Numerical simulation of the extrusion process for food materials in a single-screw extruder. J Food Eng 30: 449–467. https://doi.org/10.1016/S0260-8774(96)00022-2 doi: 10.1016/S0260-8774(96)00022-2
![]() |
[44] |
Roland W, Marschik C, Krieger M, et al. (2019) Symbolic regression models for predicting viscous dissipation of three-dimensional non-Newtonian flows in single-screw extruders. J Non-Newton Fluid 268: 12–29. https://doi.org/10.1016/j.jnnfm.2019.04.006 doi: 10.1016/j.jnnfm.2019.04.006
![]() |
[45] | Drotman D, Diagne M, Bitmead R, et al. (2016) Control-oriented energy-based modeling of a screw extruder used for 3D printing. Proceedings of the ASME 2016 Dynamic Systems and Control Conference, Minneapolis, USA, 12–14. https://doi.org/10.1115/DSCC2016-9651 |
[46] |
Smith D, Spalding MA, Gould RJ (2008) Selecting equipment to minimize production costs and maximize profitability. J Plast Film Sheet 24: 21–33. https://doi.org/10.1177/8756087907087171 doi: 10.1177/8756087907087171
![]() |
[47] | Frankland J, Get smarter on extruder sizes. Plastics Technology, 2012. Available from: https://www.ptonline.com/articles/get-smarter-on-extruder-sizes. |
[48] | Drobny JG (2014) Thermoplastic Polyurethane Elastomers, Handbook of Thermoplastic Elastomers, Amsterdam: Elsevier, 233–253. https://doi.org/10.1016/B978-0-323-22136-8.00009-0 |
[49] |
Abeykoon C, McMillan A, Nguyen BK (2021) Energy efficiency in extrusion-related polymer processing: A review of state of the art and potential efficiency improvements. Renewable Sustainable Energy Rev 147: 111219. https://doi.org/10.1016/j.rser.2021.111219 doi: 10.1016/j.rser.2021.111219
![]() |
[50] | Berthiaume D (1991) Justification for AC vs. DC drive systems. Conference Record of 1991 Annual Pulp and Paper Industry Technical Conference, Montreal, Canada, IEEE, 234–238. https://doi.org/10.1109/PAPCON.1991.239643 |
[51] |
Das A, Gilmer EL, Biria S, et al. (2021) Importance of polymer rheology on material extrusion additive manufacturing: Correlating process physics to print properties. ACS Appl Polym Mater 3: 1218–1249. https://doi.org/10.1021/acsapm.0c01228 doi: 10.1021/acsapm.0c01228
![]() |
[52] |
Whyman S, Arif KM, Potgieter J (2018) Design and development of an extrusion system for 3D printing biopolymer pellets. Int J Adv Manuf Technol 96: 3417–3428. https://doi.org/10.1007/s00170-018-1843-y doi: 10.1007/s00170-018-1843-y
![]() |
[53] |
Tian X, Liu T, Yang C, et al. (2016) Interface and performance of 3D printed continuous carbon fiber reinforced PLA composites. Compos Part A-Appl S 88: 198–205. https://doi.org/10.1016/j.compositesa.2016.05.032 doi: 10.1016/j.compositesa.2016.05.032
![]() |
[54] |
Papadakis SE, Bahu RE (1992) The sticky issues of drying. Dry Technol 10: 817–837. https://doi.org/10.1080/07373939208916484 doi: 10.1080/07373939208916484
![]() |
[55] | Maynard E, Mehos G, Maynard E, Handle bulk solids safely and effectively, fluids and solids handling. AIChE, 2009. Available from: https://www.aiche.org/resources/publications/cep/2009/september/handle-bulk-solids-safely-and-effectively. |
[56] |
Lipár S, Noga P, Hulkó G (2013) Modelling and control of extruder barrel temperature field. IFAC-PapersOnLine 46: 191–196. https://doi.org/10.3182/20130925-3-FR-4043.00078 doi: 10.3182/20130925-3-FR-4043.00078
![]() |
[57] |
La Gala A, Fiorio R, Erkoç M, et al. (2020) Theoretical evaluation of the melting efficiency for the single-screw micro-extrusion process: the case of 3D printing of ABS. Processes 8: 1522. https://doi.org/10.3390/pr8111522 doi: 10.3390/pr8111522
![]() |
[58] | Gedde UW, Hedenqvist MS, Hakkarainen M, et al. (2021) Processing of polymeric materials, Applied Polymer Science, Cham: Springer, 453–487. https://doi.org/10.1007/978-3-030-68472-3_8 |
[59] |
Grizzuti N, Bifulco O (1997) Effects of coalescence and breakup on the steady-state morphology of an immiscible polymer blend in shear flow. Rheol Acta 36: 406–415. https://doi.org/10.1007/BF00396327 doi: 10.1007/BF00396327
![]() |
[60] | Fenner RT (1979) Introduction to the main polymer processes, Principles of Polymer Processing, London: Palgrave. https://doi.org/10.1007/978-1-349-16234-5 |
[61] | Rosato DV, Rosato DV, Rosato MG (2020) Plasticizing, Injection Molding Handbook, Boston: Springer, 151–220. https://doi.org/10.1007/978-1-4615-4597-2_3 |
[62] | Frankland J, Understanding melting in single-screw extruders. Plastics Technology, 2021. Available from: https://www.ptonline.com/articles/understanding-melting-in-single-screw-extruders-. |
[63] |
Abeykoon C, Kelly AL, Brown EC, et al. (2014) Investigation of the process energy demand in polymer extrusion: A brief review and an experimental study. Appl Energ 136: 726–737. https://doi.org/10.1016/j.apenergy.2014.09.024 doi: 10.1016/j.apenergy.2014.09.024
![]() |
[64] |
Abeykoon C, Kelly AL, Brown EC, et al. (2016) The effect of materials, process settings and screw geometry on energy consumption and melt temperature in single screw extrusion. Appl Energ 180: 880–894. https://doi.org/10.1016/j.apenergy.2016.07.014 doi: 10.1016/j.apenergy.2016.07.014
![]() |
[65] |
DiNunzio JC, Brough C, Hughey JR, et al. (2010) Fusion production of solid dispersions containing a heat-sensitive active ingredient by hot melt extrusion and Kinetisol® dispersing. Eur J Pharm Biopharm 74: 340–351. https://doi.org/10.1016/j.ejpb.2009.09.007 doi: 10.1016/j.ejpb.2009.09.007
![]() |
[66] | Rtelligent, T60 Closed Loop Stepper Driver User Manual, Rtelligent, n.d. Available from: http://www.rtelligent.net/upload/wenjian/Stepper/T60%20User%20Manual.pdf. |
[67] |
Zheng Y, Zhang W, Lopez DMB, et al. (2021) Scientometric analysis and systematic review of multi-material additive manufacturing of polymers. Polymers 13: 1957. https://doi.org/10.3390/polym13121957 doi: 10.3390/polym13121957
![]() |
[68] | Trenchlesspedia, Hoop Stress. Trenchlesspedia, 2021. Available from: https://www.trenchlesspedia.com/definition/2799/hoop-stress. |
[69] | Capelle J, Dmytrakh I, Gilgert J (2006) A comparison of experimental results and computations for cracked tubes subjected to internal pressure. Mater Tehnol 40: 233. |
[70] | Birley AW, Haworth B, Batchelor J, Single-screw extrusion the extruder characteristic. Deike Library, 1992. Available from: https://zeus.plmsc.psu.edu/~manias/MatSE447/21_Extrusion2.pdf. |
[71] |
Yoo HM, Jeong SY, Choi SW (2021) Analysis of the rheological property and crystallization behavior of polylactic acid (IngeoTM Biopolymer 4032D) at different process temperatures. e-Polymers 21: 702–709. https://doi.org/10.1515/epoly-2021-0071 doi: 10.1515/epoly-2021-0071
![]() |
[72] | Engineering ToolBox, Factors of Safety. Engineering ToolBox, 2010. Available from: https://www.engineeringtoolbox.com/factors-safety-fos-d_1624.html. |
[73] |
Song R, Xiang J, Hou D (2011) Characteristics of mechanical properties and microstructure for 316L austenitic stainless steel. J Iron Steel Res Int 18: 53–59. https://doi.org/10.1016/S1006-706X(11)60117-9 doi: 10.1016/S1006-706X(11)60117-9
![]() |
[74] |
Mahfuz H, Adnan A, Rangari VK, et al. (2005) Manufacturing and characterization of carbon nanotube/polyethylene composites. Int J Nanosci 4: 55–72. https://doi.org/10.1142/S0219581X05002961 doi: 10.1142/S0219581X05002961
![]() |
[75] | Njobet NL, Energy analysis in the extrusion of plastics. Theseus, 2012. Available from: https://www.theseus.fi/handle/10024/53774. |
[76] |
Lay M, Thajudin NLN, Hamid ZAA, et al. (2019) Comparison of physical and mechanical properties of PLA, ABS and nylon 6 fabricated using fused deposition modeling and injection molding. Compos Part B-Eng 176: 107341. https://doi.org/10.1016/j.compositesb.2019.107341 doi: 10.1016/j.compositesb.2019.107341
![]() |
[77] | Frankland J, How much horsepower do you need? Plastics Technology, 2009. Available from: https://www.ptonline.com/articles/how-much-horsepower-do-you-need. |
[78] |
Zmeskal O, Marackova L, Lapcikova T, et al. (2020) Thermal properties of samples prepared from polylactic acid by 3D printing. AIP Conf Proc 2305: 020022. https://doi.org/10.1063/5.0033857 doi: 10.1063/5.0033857
![]() |
[79] |
Liang JZ (2008) Effects of extrusion conditions on die-swell behavior of polypropylene/diatomite composite melts. Polym Test 27: 936–940. https://doi.org/10.1016/j.polymertesting.2008.08.001 doi: 10.1016/j.polymertesting.2008.08.001
![]() |
[80] | Walter M, Holmström J, Yrjölä H (2004) Rapid manufacturing and its impact on supply chain management. Proceedings of the Logistics Research Network Annual Conference, Dublin, Ireland. |
[81] | Kohut A, Idea Reality: Rapid prototyping and the art of failing forward. Ultimaker, 2019. Available from: https://ultimaker.com/learn/idea-reality-rapid-prototyping-art-of-failing-forward. |
[82] | 3D.directory, 3D Printing Companies in Canada. 3D.directory, n.d. Available from: https://www.3d.directory/directory/3d-printing-services?country=Canada. |
[83] | All3DP Pro, 3D Printing From Plastic Waste: 10 Successful Projects. All3DP Pro, n.d. Available from: https://all3dp.com/1/3d-printing-from-plastic-waste/. |
[84] | TechSpot, Ford and HP are recycling 3D printer waste into truck parts. TechSpot, n.d. Available from: https://www.techspot.com/news/89074-ford-hp-recycling-3d-printer-waste-truck-parts.html. |
1. | Mustafa M. Hasaballah, Oluwafemi Samson Balogun, M. E. Bakr, Frequentist and Bayesian approach for the generalized logistic lifetime model with applications to air-conditioning system failure times under joint progressive censoring data, 2024, 9, 2473-6988, 29346, 10.3934/math.20241422 | |
2. | Dina A. Ramadan, Yusra A. Tashkandy, M. E. Bakr, Oluwafemi Samson Balogun, Mustafa M. Hasaballah, Analysis of Marshall–Olkin extended Gumbel type-II distribution under progressive type-II censoring with applications, 2024, 14, 2158-3226, 10.1063/5.0210905 | |
3. | Mustafa M Hasaballah, Oluwafemi Samson Balogun, M E Bakr, Point and interval estimation based on joint progressive censoring data from two Rayleigh-Weibull distribution with applications, 2024, 99, 0031-8949, 085239, 10.1088/1402-4896/ad6107 | |
4. | Mustafa M. Hasaballah, Yusra A. Tashkandy, Oluwafemi Samson Balogun, Mahmoud E. Bakr, Bayesian inference for two populations of Lomax distribution under joint progressive Type‐II censoring schemes with engineering applications, 2024, 40, 0748-8017, 4335, 10.1002/qre.3633 | |
5. | Maysaa Elmahi Abd Elwahab, Ahmed Elshahhat, Ohud A. Alqasem, Mazen Nassar, Reliability analysis of new jointly Type-II hybrid NH censored data and its modeling for three engineering cases, 2025, 113, 11100168, 347, 10.1016/j.aej.2024.11.032 | |
6. | Ohud A. Alqasem, Ahmed Elshahhat, Maysaa Elmahi Abd Elwahab, Mazen Nassar, Evaluation of two inverted Nadarajah–Haghighi production lines via joint progressive type-II censoring with two physical applications, 2025, 15, 2158-3226, 10.1063/5.0249890 |
(m,n) | r | Scheme | Non-Bayesian | Bayesian | |||||
MLE | Length | SE | LINEX | Length | |||||
a=−3 | a=3 | ||||||||
(10, 20) | 15 | (0(14),15) | 1.0089 | 7.7486 | 1.3852 | 1.3856 | 1.3849 | 0.0611 | |
(0.2412) | (0.0132) | (0.0131) | (0.0130) | ||||||
15 | (15,0(14)) | 1.9192 | 9.5216 | 2.5732 | 2.5736 | 2.5728 | 0.0604 | ||
(0.1757) | (1.1517) | (1.1526) | (1.1509) | ||||||
20 | (0(19),10) | 1.4528 | 8.1424 | 1.8099 | 1.8104 | 1.8095 | 0.0633 | ||
(0.0992) | (0.0961) | (0.0963) | (0.0958) | ||||||
20 | (10,0(19)) | 2.0877 | 9.2575 | 1.8322 | 1.8343 | 1.8302 | 0.1077 | ||
(0.3454) | (0.1104) | (0.1118) | (0.1090) | ||||||
25 | (0(7),2,0(4),1,0(3),2,0(8)) | 1.6978 | 6.5167 | 2.3954 | 2.3955 | 2.3952 | 0.0363 | ||
(0.0391) | (0.0175) | (0.0191) | (0.0144) | ||||||
30 | (0(30)) | 0.9524 | 4.0016 | 1.5637 | 1.5639 | 1.5635 | 0.0398 | ||
(0.2999) | (0.0041) | (0.0041) | (0.0040) | ||||||
(20, 30) | 35 | (0(34),15) | 1.4461 | 5.6789 | 1.7213 | 1.7214 | 1.7213 | 0.0272 | |
(0.0029) | (0.0490) | (0.0490) | (0.0490) | ||||||
35 | (15,0(34)) | 1.2765 | 3.9130 | 1.8908 | 1.8909 | 1.8908 | 0.0223 | ||
(0.1500) | (0.0527) | (0.0527) | (0.0527) | ||||||
40 | (0(39),10) | 1.9292 | 5.5891 | 1.2361 | 1.2366 | 1.2356 | 0.0540 | ||
(0.1843) | (0.0697) | (0.0685) | (0.0672) | ||||||
40 | (10,0(39)) | 1.9814 | 5.9534 | 1.8851 | 1.8855 | 1.8847 | 0.0601 | ||
(0.2318) | (0.1483) | (0.1486) | (0.1480) | ||||||
45 | (0(5),3,0(33),2,0(5)) | 1.3855 | 4.2144 | 0.7017 | 0.7025 | 0.7008 | 0.0709 | ||
(0.2131) | (0.0637) | (0.0534) | (0.0544) | ||||||
50 | (0(50)) | 1.7681 | 4.4709 | 1.8890 | 1.8890 | 1.8890 | 0.0261 | ||
(0.0719) | (0.0513) | (0.0514) | (0.0512) | ||||||
(40, 50) | 60 | (0(59),30) | 2.0131 | 6.0591 | 2.8808 | 2.8806 | 2.8802 | 0.0311 | |
(0.2633) | (0.0684) | (0.0688) | (0.0679) | ||||||
60 | (30,0(59)) | 0.8016 | 2.2477 | 0.5408 | 0.5407 | 0.5405 | 0.0130 | ||
(0.4878) | (0.0400) | (0.0321) | (0.201) | ||||||
70 | (0(69),20) | 0.8469 | 1.9784 | 0.6599 | 0.6599 | 0.6598 | 0.0164 | ||
(0.4265) | (0.1058) | (0.1057) | (0.1052) | ||||||
70 | (20,0(69)) | 1.0524 | 2.3534 | 1.2987 | 1.2987 | 1.2986 | 0.0140 | ||
(0.2003) | (0.0405) | (0.0402) | (0.0401) | ||||||
80 | (4,0(8),3,0(60),3,0(9)) | 2.4501 | 5.8484 | 4.4654 | 4.4656 | 4.4651 | 0.0423 | ||
(0.9027) | (0.7933) | (0.7922) | (0.7824) | ||||||
90 | (0(90)) | 1.8013 | 3.3309 | 1.6733 | 1.6734 | 1.6731 | 0.0265 | ||
(0.0908) | (0.0765) | (0.0768) | (0.0763) |
(m,n) | r | Scheme | Non-Bayesian | Bayesian | |||||
MLE | Length | SE | LINEX | Length | |||||
a=−3 | a=3 | ||||||||
(10, 20) | 15 | (0(14),15) | 0.9247 | 7.4446 | 1.3417 | 1.3422 | 1.3413 | 0.0636 | |
(0.1409) | (0.0017) | (0.0018) | (0.0017) | ||||||
15 | (15,0(14)) | 0.1539 | 0.9332 | 0.1811 | 0.1811 | 0.1811 | 0.0097 | ||
(0.3134) | (0.2519) | (0.2519) | (0.2519) | ||||||
20 | (0(19),10) | 0.6347 | 3.2751 | 0.5126 | 0.5127 | 0.5126 | 0.0150 | ||
(0.4426) | (0.6200) | (0.6210) | (0.6120) | ||||||
20 | (10,0(19)) | 2.6140 | 8.4100 | 2.8049 | 2.8055 | 2.8043 | 0.0712 | ||
(0.7267) | (0.2647) | (0.2664) | (0.2629) | ||||||
25 | (0(7),2,0(4),1,0(3),2,0(8)) | 0.6021 | 2.473 | 0.8028 | 0.8029 | 0.8028 | 0.0268 | ||
(0.4871) | (0.2472) | (0.2471) | (0.2470) | ||||||
30 | (0(30)) | 1.7301 | 5.7329 | 2.7105 | 2.7107 | 2.7103 | 0.0599 | ||
(0.1850) | (0.1795) | (0.1702) | (0.1601) | ||||||
(20, 30) | 35 | (0(34),15) | 0.3714 | 1.8375 | 1.3453 | 1.3453 | 1.3453 | 0.0082 | |
(0.1623) | (0.0021) | (0.0021) | (0.0021) | ||||||
35 | (15,0(34)) | 1.8284 | 5.0672 | 1.4205 | 1.4208 | 1.4202 | 0.0544 | ||
(0.2792) | (0.0145) | (0.0146) | (0.0144) | ||||||
40 | (0(39),10) | 2.0993 | 5.2002 | 0.9454 | 0.9455 | 0.9453 | 0.0287 | ||
(0.6390) | (0.1257) | (0.1250) | (0.1248) | ||||||
40 | (10,0(39)) | 2.5850 | 8.5699 | 2.4874 | 2.4876 | 2.4872 | 0.0414 | ||
(0.6512) | (0.4099) | (0.4095) | (0.4090) | ||||||
45 | (0(5),3,0(33),2,0(5)) | 2.649 | 7.6263 | 1.4086 | 1.4088 | 1.4083 | 0.0468 | ||
(0.8198) | (0.0118) | (0.0118) | (0.0117) | ||||||
50 | (0(50)) | 2.1930 | 5.6644 | 1.8755 | 1.8482 | 1.8655 | 0.0350 | ||
(0.7975) | (0.3469) | (0.3471) | (0.3468) | ||||||
(40, 50) | 60 | (0(59),30) | 1.0377 | 3.2544 | 1.5236 | 1.5236 | 1.5236 | 0.0207 | |
(0.0688) | (0.0500) | (0.0500) | (0.0500) | ||||||
60 | (30,0(59)) | 2.1438 | 4.4834 | 2.4051 | 2.4052 | 2.4050 | 0.0370 | ||
(0.7120) | (0.2213) | (0.2217) | (0.2210) | ||||||
70 | (0(69),20) | 0.969 | 2.4503 | 1.7391 | 1.7391 | 1.7391 | 0.0141 | ||
(0.1096) | (0.0928) | (0.0924) | (0.0920) | ||||||
70 | (20,0(69)) | 1.5667 | 3.193 | 1.7013 | 1.7012 | 1.7010 | 0.0154 | ||
(0.0712) | (0.0161) | (0.0162) | (0.0158) | ||||||
80 | (4,0(8),3,0(60),3,0(9)) | 1.3662 | 2.709 | 1.6230 | 1.6222 | 1.6220 | 0.0167 | ||
(0.1244) | (0.1043) | (0.1041) | (0.1032) | ||||||
90 | (0(90)) | 1.4794 | 2.6736 | 0.8824 | 0.8820 | 0.8811 | 0.0112 | ||
(0.0322) | (0.0174) | (0.0171) | (0.0160) |
(m,n) | r | Scheme | Non-Bayesian | Bayesian | |||||
MLE | Length | SE | LINEX | Length | |||||
a=−3 | a=3 | ||||||||
(10, 20) | 15 | (0(14),15) | 0.4500 | 2.0736 | 1.0201 | 1.2450 | 0.8765 | 1.3259 | |
(0.0025) | (0.0023) | (0.0020) | (0.0020) | ||||||
15 | (15,0(14)) | 0.3766 | 0.8338 | 0.5666 | 0.7000 | 0.4843 | 1.0085 | ||
(0.0201) | (0.0270) | (0.0278) | (0.0268) | ||||||
20 | (0(19),10) | 0.3205 | 0.8781 | 0.3128 | 0.2280 | 0.2623 | 0.8047 | ||
(0.1963) | (0.1108) | (0.1832) | (0.0688) | ||||||
20 | (10,0(19)) | 0.4035 | 0.7331 | 0.6691 | 0.7739 | 0.5954 | 0.9246 | ||
(0.0854) | (0.0724) | (0.0724) | (0.0382) | ||||||
25 | (0(7),2,0(4),1,0(3),2,0(8)) | 0.5471 | 1.0630 | 0.8072 | 0.9125 | 0.7279 | 0.9644 | ||
(0.0216) | (0.0165) | (0.0127) | (0.0107) | ||||||
30 | (0(30)) | 0.6514 | 1.3684 | 0.745 | 0.8343 | 0.6765 | 0.8887 | ||
(0.1632) | (0.1191) | (0.1286) | (0.0765) | ||||||
(20, 30) | 35 | (0(34),15) | 0.7796 | 0.7379 | 0.7796 | 0.8391 | 0.7307 | 0.7379 | |
(0.1291) | (0.1241) | (0.1028) | (0.1094) | ||||||
35 | (15,0(34)) | 0.4192 | 0.5150 | 0.5787 | 0.6148 | 0.5477 | 0.5777 | ||
(0.1010) | (0.0320) | (0.0461) | (0.0461) | ||||||
40 | (0(39),10) | 0.2902 | 0.3452 | 0.8947 | 0.9753 | 0.8295 | 0.8497 | ||
(0.2144) | (0.0125) | (0.0122) | (0.0121) | ||||||
40 | (10,0(39)) | 0.4189 | 0.4923 | 0.4864 | 0.4291 | 0.4499 | 0.2145 | ||
(0.0930) | (0.0820) | (0.0724) | (0.0620) | ||||||
45 | (0(5),3,0(33),2,0(5)) | 0.3938 | 0.5113 | 0.4844 | 0.4782 | 0.4653 | 0.3813 | ||
(0.434) | (0.0354) | (0.0352) | (0.0344) | ||||||
50 | (0(50)) | 0.3731 | 0.3433 | 0.4990 | 0.4265 | 0.4744 | 0.3129 | ||
(0.0898) | (0.0396) | (0.0394) | (0.0390) | ||||||
(40, 50) | 60 | (0(59),30) | 0.2999 | 0.4200 | 0.5524 | 0.4799 | 0.5277 | 0.3152 | |
(0.0954) | (0.0779) | (0.0462) | (0.0563) | ||||||
60 | (30,0(59)) | 0.6208 | 0.8487 | 0.5244 | 0.5301 | 0.5030 | 0.4250 | ||
(0.0488) | (0.0244) | (0.0223) | (0.0222) | ||||||
70 | (0(69),20) | 0.4048 | 0.4479 | 0.3692 | 0.3196 | 0.3245 | 0.1888 | ||
(0.4478) | (0.1177) | (0.3900) | (0.3548) | ||||||
70 | (20,0(69)) | 0.6135 | 0.6612 | 0.6751 | 0.6168 | 0.6376 | 0.4304 | ||
(0.0456) | (0.0258) | (0.0671) | (0.0915) | ||||||
80 | (4,0(8),3,0(60),3,0(9)) | 0.3866 | 0.3552 | 0.5170 | 0.5274 | 0.5070 | 0.3215 | ||
(0.0212) | (0.0137) | (0.0162) | (0.0115) | ||||||
90 | (0(90)) | 0.3746 | 0.2523 | 0.4336 | 0.4443 | 0.4234 | 0.2224 | ||
(0.1064) | (0.0178) | (0.0208) | (0.0152) |
(m,n) | r | Scheme | Non-Bayesian | Bayesian | |||||
MLE | Length | SE | LINEX | Length | |||||
a=−3 | a=3 | ||||||||
(10, 20) | 15 | (0(14),15) | 0.4110 | 2.0336 | 1.0052 | 1.2843 | 0.8484 | 1.4092 | |
(0.0123) | (0.0119) | (0.0112) | (0.0101) | ||||||
15 | (15,0(14)) | 0.8193 | 2.8032 | 1.0506 | 1.2216 | 0.9307 | 1.2030 | ||
(0.2696) | (0.3634) | (0.4493) | (0.3978) | ||||||
20 | (0(19),10) | 0.5335 | 1.5963 | 1.3381 | 1.6395 | 1.1490 | 1.5159 | ||
(0.0545) | (0.0777) | (0.7941) | (0.7208) | ||||||
20 | (10,0(19)) | 0.2255 | 0.2141 | 0.3820 | 0.3992 | 0.3666 | 0.4006 | ||
(0.0085) | (0.0067) | (0.0078) | (0.0044) | ||||||
25 | (0(7),2,0(4),1,0(3),2,0(8)) | 0.4095 | 0.6813 | 0.6038 | 0.6416 | 0.5715 | 0.5963 | ||
(0.0120) | (0.0092) | (0.0081) | (0.0073) | ||||||
30 | (0(30)) | 0.2772 | 0.2753 | 0.3940 | 0.4056 | 0.3833 | 0.3367 | ||
(0.0188) | (0.0088) | (0.0075) | (0.0069) | ||||||
(20, 30) | 35 | (0(34),15) | 0.7089 | 2.2453 | 0.8012 | 0.8529 | 0.7567 | 0.6981 | |
(0.1672) | (0.1512) | (0.1057) | (0.1086) | ||||||
35 | (15,0(34)) | 0.2850 | 0.2466 | 0.5229 | 0.5426 | 0.5049 | 0.4333 | ||
(0.1491) | (0.0497) | (0.0589) | (0.0420) | ||||||
40 | (0(39),10) | 0.3109 | 0.3064 | 0.3965 | 0.3623 | 0.3410 | 0.2909 | ||
(0.5851) | (0.4866) | (0.4851) | (0.4840) | ||||||
40 | (10,0(39)) | 0.3604 | 0.2478 | 0.3265 | 0.3382 | 0.3156 | 0.2302 | ||
(0.1458) | (0.0160) | (0.0191) | (0.0134) | ||||||
45 | (0(5),3,0(33),2,0(5)) | 0.6419 | 0.6039 | 0.5232 | 0.5379 | 0.5095 | 0.3788 | ||
(0.3430) | (0.0498) | (0.0566) | (0.0439) | ||||||
50 | (0(50)) | 0.2571 | 0.1927 | 0.2427 | 0.2527 | 0.2332 | 0.1163 | ||
(0.1144) | (0.0204) | (0.0233) | (0.0177) | ||||||
(40, 50) | 60 | (0(59),30) | 0.4642 | 0.7702 | 0.9308 | 0.9680 | 0.8969 | 0.6030 | |
(0.0270) | (0.0179) | (0.0171) | (0.0165) | ||||||
60 | (30,0(59)) | 0.2766 | 0.2781 | 0.2510 | 0.2591 | 0.2430 | 0.1898 | ||
(0.1222) | (0.0228) | (0.0254) | (0.0204) | ||||||
70 | (0(69),20) | 0.3746 | 0.4258 | 0.3499 | 0.3682 | 0.3329 | 0.2235 | ||
(0.4441) | (0.1225) | (0.1356) | (0.1108) | ||||||
70 | (20,0(69)) | 0.2747 | 0.1723 | 0.4435 | 0.4506 | 0.4368 | 0.2654 | ||
(0.1324) | (0.0206) | (0.0227) | (0.0187) | ||||||
80 | (4,0(8),3,0(60),3,0(9)) | 0.6604 | 0.5647 | 0.5858 | 0.5983 | 0.5741 | 0.3527 | ||
(0.1204) | (0.0817) | (0.0890) | (0.0751) | ||||||
90 | (0(90)) | 0.2699 | 0.1466 | 0.2436 | 0.2524 | 0.2351 | 0.1170 | ||
(0.1100) | (0.0593) | (0.0637) | (0.0553) |
Data set | Size (n) | K-S (Calculated) | K-S (5% Significance) | P-value |
I | 27 | 0.1011 | 0.2544 | 0.9192 |
II | 24 | 0.0870 | 0.2693 | 0.9858 |
Parameters | MLE | MCMC | |||
SE | LINEX | ||||
a=−3.0 | a=10−4 | a=3.0 | |||
α1 | 0.0211 | 0.0612 | 0.0612 | 0.0612 | 0.0612 |
α2 | 0.0112 | 0.0406 | 0.0406 | 0.0406 | 0.0406 |
γ1 | 0.6548 | 1.5915 | 2.2824 | 1.5915 | 1.2740 |
γ2 | 1.2882 | 2.0091 | 2.8235 | 2.0091 | 1.6094 |
Method | α1 | α2 | |||||
Lower | Upper | Length | Lower | Upper | Length | ||
CI | -0.0801 | 0.1223 | 0.2024 | -0.0392 | 0.0615 | 0.1008 | |
CRI | 0.0606 | 0.0621 | 0.0014 | 0.0401 | 0.0411 | 0.0010 |
Method | γ1 | γ2 | |||||
Lower | Upper | Length | Lower | Upper | Length | ||
CI | -1.7852 | 3.0948 | 4.8801 | -3.6776 | 6.2541 | 9.9317 | |
CRI | 0.7213 | 2.8120 | 2.0906 | 1.0133 | 3.3537 | 2.3404 |
(m,n) | r | Scheme | Non-Bayesian | Bayesian | |||||
MLE | Length | SE | LINEX | Length | |||||
a=−3 | a=3 | ||||||||
(10, 20) | 15 | (0(14),15) | 1.0089 | 7.7486 | 1.3852 | 1.3856 | 1.3849 | 0.0611 | |
(0.2412) | (0.0132) | (0.0131) | (0.0130) | ||||||
15 | (15,0(14)) | 1.9192 | 9.5216 | 2.5732 | 2.5736 | 2.5728 | 0.0604 | ||
(0.1757) | (1.1517) | (1.1526) | (1.1509) | ||||||
20 | (0(19),10) | 1.4528 | 8.1424 | 1.8099 | 1.8104 | 1.8095 | 0.0633 | ||
(0.0992) | (0.0961) | (0.0963) | (0.0958) | ||||||
20 | (10,0(19)) | 2.0877 | 9.2575 | 1.8322 | 1.8343 | 1.8302 | 0.1077 | ||
(0.3454) | (0.1104) | (0.1118) | (0.1090) | ||||||
25 | (0(7),2,0(4),1,0(3),2,0(8)) | 1.6978 | 6.5167 | 2.3954 | 2.3955 | 2.3952 | 0.0363 | ||
(0.0391) | (0.0175) | (0.0191) | (0.0144) | ||||||
30 | (0(30)) | 0.9524 | 4.0016 | 1.5637 | 1.5639 | 1.5635 | 0.0398 | ||
(0.2999) | (0.0041) | (0.0041) | (0.0040) | ||||||
(20, 30) | 35 | (0(34),15) | 1.4461 | 5.6789 | 1.7213 | 1.7214 | 1.7213 | 0.0272 | |
(0.0029) | (0.0490) | (0.0490) | (0.0490) | ||||||
35 | (15,0(34)) | 1.2765 | 3.9130 | 1.8908 | 1.8909 | 1.8908 | 0.0223 | ||
(0.1500) | (0.0527) | (0.0527) | (0.0527) | ||||||
40 | (0(39),10) | 1.9292 | 5.5891 | 1.2361 | 1.2366 | 1.2356 | 0.0540 | ||
(0.1843) | (0.0697) | (0.0685) | (0.0672) | ||||||
40 | (10,0(39)) | 1.9814 | 5.9534 | 1.8851 | 1.8855 | 1.8847 | 0.0601 | ||
(0.2318) | (0.1483) | (0.1486) | (0.1480) | ||||||
45 | (0(5),3,0(33),2,0(5)) | 1.3855 | 4.2144 | 0.7017 | 0.7025 | 0.7008 | 0.0709 | ||
(0.2131) | (0.0637) | (0.0534) | (0.0544) | ||||||
50 | (0(50)) | 1.7681 | 4.4709 | 1.8890 | 1.8890 | 1.8890 | 0.0261 | ||
(0.0719) | (0.0513) | (0.0514) | (0.0512) | ||||||
(40, 50) | 60 | (0(59),30) | 2.0131 | 6.0591 | 2.8808 | 2.8806 | 2.8802 | 0.0311 | |
(0.2633) | (0.0684) | (0.0688) | (0.0679) | ||||||
60 | (30,0(59)) | 0.8016 | 2.2477 | 0.5408 | 0.5407 | 0.5405 | 0.0130 | ||
(0.4878) | (0.0400) | (0.0321) | (0.201) | ||||||
70 | (0(69),20) | 0.8469 | 1.9784 | 0.6599 | 0.6599 | 0.6598 | 0.0164 | ||
(0.4265) | (0.1058) | (0.1057) | (0.1052) | ||||||
70 | (20,0(69)) | 1.0524 | 2.3534 | 1.2987 | 1.2987 | 1.2986 | 0.0140 | ||
(0.2003) | (0.0405) | (0.0402) | (0.0401) | ||||||
80 | (4,0(8),3,0(60),3,0(9)) | 2.4501 | 5.8484 | 4.4654 | 4.4656 | 4.4651 | 0.0423 | ||
(0.9027) | (0.7933) | (0.7922) | (0.7824) | ||||||
90 | (0(90)) | 1.8013 | 3.3309 | 1.6733 | 1.6734 | 1.6731 | 0.0265 | ||
(0.0908) | (0.0765) | (0.0768) | (0.0763) |
(m,n) | r | Scheme | Non-Bayesian | Bayesian | |||||
MLE | Length | SE | LINEX | Length | |||||
a=−3 | a=3 | ||||||||
(10, 20) | 15 | (0(14),15) | 0.9247 | 7.4446 | 1.3417 | 1.3422 | 1.3413 | 0.0636 | |
(0.1409) | (0.0017) | (0.0018) | (0.0017) | ||||||
15 | (15,0(14)) | 0.1539 | 0.9332 | 0.1811 | 0.1811 | 0.1811 | 0.0097 | ||
(0.3134) | (0.2519) | (0.2519) | (0.2519) | ||||||
20 | (0(19),10) | 0.6347 | 3.2751 | 0.5126 | 0.5127 | 0.5126 | 0.0150 | ||
(0.4426) | (0.6200) | (0.6210) | (0.6120) | ||||||
20 | (10,0(19)) | 2.6140 | 8.4100 | 2.8049 | 2.8055 | 2.8043 | 0.0712 | ||
(0.7267) | (0.2647) | (0.2664) | (0.2629) | ||||||
25 | (0(7),2,0(4),1,0(3),2,0(8)) | 0.6021 | 2.473 | 0.8028 | 0.8029 | 0.8028 | 0.0268 | ||
(0.4871) | (0.2472) | (0.2471) | (0.2470) | ||||||
30 | (0(30)) | 1.7301 | 5.7329 | 2.7105 | 2.7107 | 2.7103 | 0.0599 | ||
(0.1850) | (0.1795) | (0.1702) | (0.1601) | ||||||
(20, 30) | 35 | (0(34),15) | 0.3714 | 1.8375 | 1.3453 | 1.3453 | 1.3453 | 0.0082 | |
(0.1623) | (0.0021) | (0.0021) | (0.0021) | ||||||
35 | (15,0(34)) | 1.8284 | 5.0672 | 1.4205 | 1.4208 | 1.4202 | 0.0544 | ||
(0.2792) | (0.0145) | (0.0146) | (0.0144) | ||||||
40 | (0(39),10) | 2.0993 | 5.2002 | 0.9454 | 0.9455 | 0.9453 | 0.0287 | ||
(0.6390) | (0.1257) | (0.1250) | (0.1248) | ||||||
40 | (10,0(39)) | 2.5850 | 8.5699 | 2.4874 | 2.4876 | 2.4872 | 0.0414 | ||
(0.6512) | (0.4099) | (0.4095) | (0.4090) | ||||||
45 | (0(5),3,0(33),2,0(5)) | 2.649 | 7.6263 | 1.4086 | 1.4088 | 1.4083 | 0.0468 | ||
(0.8198) | (0.0118) | (0.0118) | (0.0117) | ||||||
50 | (0(50)) | 2.1930 | 5.6644 | 1.8755 | 1.8482 | 1.8655 | 0.0350 | ||
(0.7975) | (0.3469) | (0.3471) | (0.3468) | ||||||
(40, 50) | 60 | (0(59),30) | 1.0377 | 3.2544 | 1.5236 | 1.5236 | 1.5236 | 0.0207 | |
(0.0688) | (0.0500) | (0.0500) | (0.0500) | ||||||
60 | (30,0(59)) | 2.1438 | 4.4834 | 2.4051 | 2.4052 | 2.4050 | 0.0370 | ||
(0.7120) | (0.2213) | (0.2217) | (0.2210) | ||||||
70 | (0(69),20) | 0.969 | 2.4503 | 1.7391 | 1.7391 | 1.7391 | 0.0141 | ||
(0.1096) | (0.0928) | (0.0924) | (0.0920) | ||||||
70 | (20,0(69)) | 1.5667 | 3.193 | 1.7013 | 1.7012 | 1.7010 | 0.0154 | ||
(0.0712) | (0.0161) | (0.0162) | (0.0158) | ||||||
80 | (4,0(8),3,0(60),3,0(9)) | 1.3662 | 2.709 | 1.6230 | 1.6222 | 1.6220 | 0.0167 | ||
(0.1244) | (0.1043) | (0.1041) | (0.1032) | ||||||
90 | (0(90)) | 1.4794 | 2.6736 | 0.8824 | 0.8820 | 0.8811 | 0.0112 | ||
(0.0322) | (0.0174) | (0.0171) | (0.0160) |
(m,n) | r | Scheme | Non-Bayesian | Bayesian | |||||
MLE | Length | SE | LINEX | Length | |||||
a=−3 | a=3 | ||||||||
(10, 20) | 15 | (0(14),15) | 0.4500 | 2.0736 | 1.0201 | 1.2450 | 0.8765 | 1.3259 | |
(0.0025) | (0.0023) | (0.0020) | (0.0020) | ||||||
15 | (15,0(14)) | 0.3766 | 0.8338 | 0.5666 | 0.7000 | 0.4843 | 1.0085 | ||
(0.0201) | (0.0270) | (0.0278) | (0.0268) | ||||||
20 | (0(19),10) | 0.3205 | 0.8781 | 0.3128 | 0.2280 | 0.2623 | 0.8047 | ||
(0.1963) | (0.1108) | (0.1832) | (0.0688) | ||||||
20 | (10,0(19)) | 0.4035 | 0.7331 | 0.6691 | 0.7739 | 0.5954 | 0.9246 | ||
(0.0854) | (0.0724) | (0.0724) | (0.0382) | ||||||
25 | (0(7),2,0(4),1,0(3),2,0(8)) | 0.5471 | 1.0630 | 0.8072 | 0.9125 | 0.7279 | 0.9644 | ||
(0.0216) | (0.0165) | (0.0127) | (0.0107) | ||||||
30 | (0(30)) | 0.6514 | 1.3684 | 0.745 | 0.8343 | 0.6765 | 0.8887 | ||
(0.1632) | (0.1191) | (0.1286) | (0.0765) | ||||||
(20, 30) | 35 | (0(34),15) | 0.7796 | 0.7379 | 0.7796 | 0.8391 | 0.7307 | 0.7379 | |
(0.1291) | (0.1241) | (0.1028) | (0.1094) | ||||||
35 | (15,0(34)) | 0.4192 | 0.5150 | 0.5787 | 0.6148 | 0.5477 | 0.5777 | ||
(0.1010) | (0.0320) | (0.0461) | (0.0461) | ||||||
40 | (0(39),10) | 0.2902 | 0.3452 | 0.8947 | 0.9753 | 0.8295 | 0.8497 | ||
(0.2144) | (0.0125) | (0.0122) | (0.0121) | ||||||
40 | (10,0(39)) | 0.4189 | 0.4923 | 0.4864 | 0.4291 | 0.4499 | 0.2145 | ||
(0.0930) | (0.0820) | (0.0724) | (0.0620) | ||||||
45 | (0(5),3,0(33),2,0(5)) | 0.3938 | 0.5113 | 0.4844 | 0.4782 | 0.4653 | 0.3813 | ||
(0.434) | (0.0354) | (0.0352) | (0.0344) | ||||||
50 | (0(50)) | 0.3731 | 0.3433 | 0.4990 | 0.4265 | 0.4744 | 0.3129 | ||
(0.0898) | (0.0396) | (0.0394) | (0.0390) | ||||||
(40, 50) | 60 | (0(59),30) | 0.2999 | 0.4200 | 0.5524 | 0.4799 | 0.5277 | 0.3152 | |
(0.0954) | (0.0779) | (0.0462) | (0.0563) | ||||||
60 | (30,0(59)) | 0.6208 | 0.8487 | 0.5244 | 0.5301 | 0.5030 | 0.4250 | ||
(0.0488) | (0.0244) | (0.0223) | (0.0222) | ||||||
70 | (0(69),20) | 0.4048 | 0.4479 | 0.3692 | 0.3196 | 0.3245 | 0.1888 | ||
(0.4478) | (0.1177) | (0.3900) | (0.3548) | ||||||
70 | (20,0(69)) | 0.6135 | 0.6612 | 0.6751 | 0.6168 | 0.6376 | 0.4304 | ||
(0.0456) | (0.0258) | (0.0671) | (0.0915) | ||||||
80 | (4,0(8),3,0(60),3,0(9)) | 0.3866 | 0.3552 | 0.5170 | 0.5274 | 0.5070 | 0.3215 | ||
(0.0212) | (0.0137) | (0.0162) | (0.0115) | ||||||
90 | (0(90)) | 0.3746 | 0.2523 | 0.4336 | 0.4443 | 0.4234 | 0.2224 | ||
(0.1064) | (0.0178) | (0.0208) | (0.0152) |
(m,n) | r | Scheme | Non-Bayesian | Bayesian | |||||
MLE | Length | SE | LINEX | Length | |||||
a=−3 | a=3 | ||||||||
(10, 20) | 15 | (0(14),15) | 0.4110 | 2.0336 | 1.0052 | 1.2843 | 0.8484 | 1.4092 | |
(0.0123) | (0.0119) | (0.0112) | (0.0101) | ||||||
15 | (15,0(14)) | 0.8193 | 2.8032 | 1.0506 | 1.2216 | 0.9307 | 1.2030 | ||
(0.2696) | (0.3634) | (0.4493) | (0.3978) | ||||||
20 | (0(19),10) | 0.5335 | 1.5963 | 1.3381 | 1.6395 | 1.1490 | 1.5159 | ||
(0.0545) | (0.0777) | (0.7941) | (0.7208) | ||||||
20 | (10,0(19)) | 0.2255 | 0.2141 | 0.3820 | 0.3992 | 0.3666 | 0.4006 | ||
(0.0085) | (0.0067) | (0.0078) | (0.0044) | ||||||
25 | (0(7),2,0(4),1,0(3),2,0(8)) | 0.4095 | 0.6813 | 0.6038 | 0.6416 | 0.5715 | 0.5963 | ||
(0.0120) | (0.0092) | (0.0081) | (0.0073) | ||||||
30 | (0(30)) | 0.2772 | 0.2753 | 0.3940 | 0.4056 | 0.3833 | 0.3367 | ||
(0.0188) | (0.0088) | (0.0075) | (0.0069) | ||||||
(20, 30) | 35 | (0(34),15) | 0.7089 | 2.2453 | 0.8012 | 0.8529 | 0.7567 | 0.6981 | |
(0.1672) | (0.1512) | (0.1057) | (0.1086) | ||||||
35 | (15,0(34)) | 0.2850 | 0.2466 | 0.5229 | 0.5426 | 0.5049 | 0.4333 | ||
(0.1491) | (0.0497) | (0.0589) | (0.0420) | ||||||
40 | (0(39),10) | 0.3109 | 0.3064 | 0.3965 | 0.3623 | 0.3410 | 0.2909 | ||
(0.5851) | (0.4866) | (0.4851) | (0.4840) | ||||||
40 | (10,0(39)) | 0.3604 | 0.2478 | 0.3265 | 0.3382 | 0.3156 | 0.2302 | ||
(0.1458) | (0.0160) | (0.0191) | (0.0134) | ||||||
45 | (0(5),3,0(33),2,0(5)) | 0.6419 | 0.6039 | 0.5232 | 0.5379 | 0.5095 | 0.3788 | ||
(0.3430) | (0.0498) | (0.0566) | (0.0439) | ||||||
50 | (0(50)) | 0.2571 | 0.1927 | 0.2427 | 0.2527 | 0.2332 | 0.1163 | ||
(0.1144) | (0.0204) | (0.0233) | (0.0177) | ||||||
(40, 50) | 60 | (0(59),30) | 0.4642 | 0.7702 | 0.9308 | 0.9680 | 0.8969 | 0.6030 | |
(0.0270) | (0.0179) | (0.0171) | (0.0165) | ||||||
60 | (30,0(59)) | 0.2766 | 0.2781 | 0.2510 | 0.2591 | 0.2430 | 0.1898 | ||
(0.1222) | (0.0228) | (0.0254) | (0.0204) | ||||||
70 | (0(69),20) | 0.3746 | 0.4258 | 0.3499 | 0.3682 | 0.3329 | 0.2235 | ||
(0.4441) | (0.1225) | (0.1356) | (0.1108) | ||||||
70 | (20,0(69)) | 0.2747 | 0.1723 | 0.4435 | 0.4506 | 0.4368 | 0.2654 | ||
(0.1324) | (0.0206) | (0.0227) | (0.0187) | ||||||
80 | (4,0(8),3,0(60),3,0(9)) | 0.6604 | 0.5647 | 0.5858 | 0.5983 | 0.5741 | 0.3527 | ||
(0.1204) | (0.0817) | (0.0890) | (0.0751) | ||||||
90 | (0(90)) | 0.2699 | 0.1466 | 0.2436 | 0.2524 | 0.2351 | 0.1170 | ||
(0.1100) | (0.0593) | (0.0637) | (0.0553) |
Data set | Size (n) | K-S (Calculated) | K-S (5% Significance) | P-value |
I | 27 | 0.1011 | 0.2544 | 0.9192 |
II | 24 | 0.0870 | 0.2693 | 0.9858 |
Parameters | MLE | MCMC | |||
SE | LINEX | ||||
a=−3.0 | a=10−4 | a=3.0 | |||
α1 | 0.0211 | 0.0612 | 0.0612 | 0.0612 | 0.0612 |
α2 | 0.0112 | 0.0406 | 0.0406 | 0.0406 | 0.0406 |
γ1 | 0.6548 | 1.5915 | 2.2824 | 1.5915 | 1.2740 |
γ2 | 1.2882 | 2.0091 | 2.8235 | 2.0091 | 1.6094 |
Method | α1 | α2 | |||||
Lower | Upper | Length | Lower | Upper | Length | ||
CI | -0.0801 | 0.1223 | 0.2024 | -0.0392 | 0.0615 | 0.1008 | |
CRI | 0.0606 | 0.0621 | 0.0014 | 0.0401 | 0.0411 | 0.0010 |
Method | γ1 | γ2 | |||||
Lower | Upper | Length | Lower | Upper | Length | ||
CI | -1.7852 | 3.0948 | 4.8801 | -3.6776 | 6.2541 | 9.9317 | |
CRI | 0.7213 | 2.8120 | 2.0906 | 1.0133 | 3.3537 | 2.3404 |