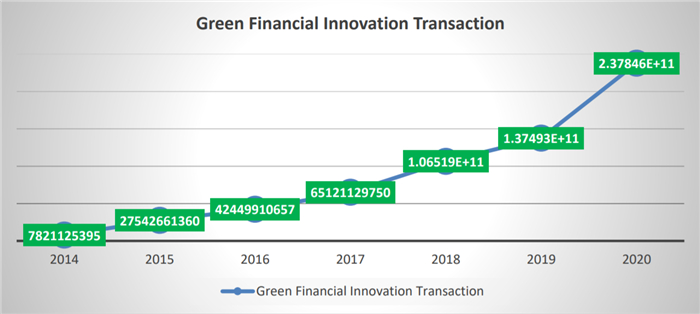
Green innovation is the creation of new and competitive products, services, processes, procedures and systems designed to use natural resources at a minimum level and to provide better quality of life on behalf of all that respects sustainability of the nature and of the future generations. The study objective was to examine the relationship between green innovation and financial performance. The study used an explanatory research design and a quantitative research approach to achieve the study's objective. Secondary time series data collected quarterly during the study period (2014–2020) was utilized to run the regression model. Autoregressive moving average (ARIMA) was used to forecast the growing level of green financial innovation transactions, and autoregressive distributed lag model (ARDL) was used to examine the effect of green financial innovation transactions on financial performance. According to forecasted results, on average green financial innovation transaction is expected to grow by 11 percent each quarter, and its impact on financial performance is found positive and significant in the short run. However, the long-run estimation of ARDL shows the positive and insignificant effect of green financial innovation on financial performance. Based on the study's findings, we recommend that the banking sector focuses on adopting green financial innovations to improve financial performance by taking into account both the short-run and long-run benefits of the products. At the same time, we suggest that the sector focus on those green financial innovations which have the lowest adoption and development costs compared to others since the long-run effect affects the overall financial performance of the sector. The main contribution of this study is to provide future indication on the relationship between the two variables in order to provide proper decision making in a bid to make green innovation investment.
Citation: Goshu Desalegn, Anita Tangl. Forecasting green financial innovation and its implications for financial performance in Ethiopian Financial Institutions: Evidence from ARIMA and ARDL model[J]. National Accounting Review, 2022, 4(2): 95-111. doi: 10.3934/NAR.2022006
[1] | Nuraddeen Umar Sambo, Ibrahim Sambo Farouq, Mukhtar Tijjani Isma'il . Asymmetric effect of exchange rate volatility on trade balance in Nigeria. National Accounting Review, 2021, 3(3): 342-359. doi: 10.3934/NAR.2021018 |
[2] | Jinhui Zhu, Zhenghui Li . Can digital financial inclusion effectively stimulate technological Innovation of agricultural enterprises?—A case study on China. National Accounting Review, 2021, 3(4): 398-421. doi: 10.3934/NAR.2021021 |
[3] | Luccas Assis Attílio . Impact of Chinese financial shocks: A GVAR approach. National Accounting Review, 2024, 6(1): 27-49. doi: 10.3934/NAR.2024002 |
[4] | Lloyd George Banda . Determinants of Commercial Banks' Performance in Malawi: An Autoregressive Distributed Lag (ARDL) Approach. National Accounting Review, 2021, 3(4): 422-438. doi: 10.3934/NAR.2021022 |
[5] | Olha Kravchenko, Nadiia Bohomolova, Oksana Karpenko, Maryna Savchenko, Nataliia Bondar . Scenario-based financial planning: the case of Ukrainian railways. National Accounting Review, 2020, 2(3): 217-248. doi: 10.3934/NAR.2020013 |
[6] | Ying Gao, Hui Yang . The measurement of financial support for real estate and house price bubbles and their dynamic relationship: An empirical study based on 31 major cities in China. National Accounting Review, 2024, 6(2): 195-219. doi: 10.3934/NAR.2024009 |
[7] | Basma Ben Néfissa, Faouzi Jilani . Impact of the mandatory adoption of IFRS on the quality of financial forecasts. National Accounting Review, 2020, 2(1): 95-109. doi: 10.3934/NAR.2020006 |
[8] | Gavin C Reid, Julia A Smith, Yu-Lin Hsu . Choices of financial reporting regimes and techniques and underlying decision-making processes: a case study analysis of a port authority. National Accounting Review, 2021, 3(2): 137-151. doi: 10.3934/NAR.2021007 |
[9] | Kgabo Johannes Dibete, Onoriode Collins Potokri . The functionality of finance committees in no-fee schools of Limpopo province, South Africa. National Accounting Review, 2021, 3(3): 256-271. doi: 10.3934/NAR.2021013 |
[10] | Mohammad Reza Abbaszadeh, Mehdi Jabbari Nooghabi, Mohammad Mahdi Rounaghi . Using Lyapunov's method for analysing of chaotic behaviour on financial time series data: a case study on Tehran stock exchange. National Accounting Review, 2020, 2(3): 297-308. doi: 10.3934/NAR.2020017 |
Green innovation is the creation of new and competitive products, services, processes, procedures and systems designed to use natural resources at a minimum level and to provide better quality of life on behalf of all that respects sustainability of the nature and of the future generations. The study objective was to examine the relationship between green innovation and financial performance. The study used an explanatory research design and a quantitative research approach to achieve the study's objective. Secondary time series data collected quarterly during the study period (2014–2020) was utilized to run the regression model. Autoregressive moving average (ARIMA) was used to forecast the growing level of green financial innovation transactions, and autoregressive distributed lag model (ARDL) was used to examine the effect of green financial innovation transactions on financial performance. According to forecasted results, on average green financial innovation transaction is expected to grow by 11 percent each quarter, and its impact on financial performance is found positive and significant in the short run. However, the long-run estimation of ARDL shows the positive and insignificant effect of green financial innovation on financial performance. Based on the study's findings, we recommend that the banking sector focuses on adopting green financial innovations to improve financial performance by taking into account both the short-run and long-run benefits of the products. At the same time, we suggest that the sector focus on those green financial innovations which have the lowest adoption and development costs compared to others since the long-run effect affects the overall financial performance of the sector. The main contribution of this study is to provide future indication on the relationship between the two variables in order to provide proper decision making in a bid to make green innovation investment.
Green innovation is known by the development of new and competitive products, services, processes, procedures, and systems that use natural resources as sparingly as possible while providing a higher quality of life for all (Asadi et al., 2020). Particularly at the product, material, and manufacturing process levels it contributes to sustainable development and natural regeneration. Green innovation has become a prominent issue from numerous scientific perspectives since the worldwide population is concerned about increasing global warming and environmental deterioration (Aron & Molina, 2020). Green innovation in business generally focuses on enhancements and renovations that reduce emissions, pollution, and costs (Asadi et al., 2020). From a business perspective, green innovation can be divided into two categories of green technology innovation and green management innovation (Li et al., 2018). Companies that are early adopters of green innovation techniques are thought to gain and maintain market competitiveness (Aguilera-Caracuel & Ortiz-de-Mandojana, 2013).
As a result, successful green innovation performance assists businesses in increasing efficiency while also creating and reinforcing core competencies. In the case of green financial technology, it is believed that the process by which a long tenure is used to label the generation of new and creative approaches to various financial circumstances (Calomiris, 2009).
Furthermore, it can be considered as a shift in the production function's shape. Hence, innovation, in general, can be explained as a process that allows an existing product or service to be offered at a lower cost, or a "new product or service" to be introduced to the market (Merton, 1992). The application of green innovations increases the competitiveness of a business entity and creates value for its owners (Merton, 1992). Additionally, green financial innovation is used as the strategic planning variable for outstripping the financial institution's objectives (Calomiris, 2009). Hence having successful green innovation planning generates an exclusive competitive position that grants the firm a competitive advantage and superior performance (Allen, 2012). In the case of the financial sector, green financial technologies aid in the benefits of optimizing taxes (Ahmad & Wu, 2022), increasing the liquidity of market-based products, (Li et al., 2018), lowering transaction costs, and lowering agency costs between executive management and shareholders of financial institutions (Aguilera-Caracuel & Ortiz-de-Mandojana, 2013). Apart from that, it creates beneficial opportunities for shareholders and creditors by reducing information asymmetry between corporate insiders and outsiders (Corrocher & Ozman, 2020), increasing risk-sharing opportunities, and making capital intermediation more efficient and cost-effective for clients (Adhikari & Momaya, 2021). In the financial sector, especially banking sectors the widely used products as means of green financial innovation are automated teller machines, debit cards, point-of-sale machines, internet banking, and mobile banking (Agarwal & Zhang, 2020). It is argued that there are many driving forces that initiate the financial sector for the adoption and development of green financial innovations.
According to (Benfratello et al., 2008) the driving forces are assumed to be the desire to improve performance, desire to improve customer relationships, rapidly changing customer needs and preferences, desire to improve organizational performance, desire to cover a wide geographical area, desire to build an organizational reputation, and desire to reduce costs. In general, the majority of empirical studies conducted on green innovation from different perspectives show that the overall importance of innovation is an aid in the advancement of financial performance (Aastvedt et al., 2021; Aguilera-Caracuel & Ortiz-de-Mandojana, 2013; Asadi et al., 2020; Asni & Agustia, 2021; Corrocher & Ozman, 2020).
In Ethiopia, the financial system is far behind the rest of the world in terms of adopting green financial innovations (Temam, 2018). However, when compared to branch expansion, the financial sector has shown massive growth and development in the adoption of financial innovations as well as growth in the capital base in recent years (National bank of Ethiopia, 2020). According to data from Ethiopia's national bank, the value of transactions made by green financial innovations are increasing from time to time. More specifically, only in the year 2020, the value of transactions made through green financial innovations was increased more than twice compared to the preceding year 2019. The following figure 1 of the study shows the trend over time of green financial innovations transaction value in Ethiopia.
The growing volume of these transactions via financial innovations may have a positive or negative impact on the banking sector's financial performance as well as future investment decisions in green financial innovations. As a result, forecasting the future trend of these variables and their impact on financial performance is important to make necessary investment preparations and identify the best alternative investment decision of green financial innovation. Furthermore, the result of this empirical study will benefit the banking sector as well as central banks in making green innovation policies that deliberately affects the financial performance of the banking sector. However, no studies have been conducted to investigate the issue. As a result, taking into account the research gap, this study will forecast the expected changes in the green financial innovation transaction value, as well as their impact on financial performance over time for the coming 5 years (2021–2025). In doing so, we used transactions made by automated teller machines, mobile banking, internet banking, debit card, and point of sales machines over 7 years (2014–2020). Hence the general objective of this study is to forecast the future values of green financial innovation transactions and to examine their effect on the financial performance of the Ethiopian banking sector. The main contribution of this study is to provide future indication on the relationship between the two variables in order to provide proper decision making in a bid to make green innovation investment. Furthermore, the study will contribute to empirical gaps observed in the study area, and provide implications on the relationship between variables used in the study, and finally the literature on the study provides an implication on the relationship between two variables under of different economies.
The following section scrutinizes the literature review conducted on the study. Section 3 describes the study's research methodology, while Section 4 discusses the findings of the study, and the conclusion and policy recommendations are presented in the final section.
Innovation is a broad phenomenon encompassing any new way of doing things in economic ways (Bakari et al., 2018). Innovation is any change, modification, improvement, or creation that has been implemented or applied in the market, regardless of its object (product, process, structure, method, etc.) (Corrocher & Ozman, 2020). Thus, innovation entails a multi-stage process in which new ideas must first be created, tested, put into production, and finally placed on the market to affect individuals, businesses, and society as a whole (Agarwal & Zhang, 2020). Currently, because of growing concern about the state of the environment, the business sector has paid close attention to green technology innovation, particularly those in heavily polluting manufacturing industries (Acquah et al., 2021).
Green process innovation and green product innovation are the two primary strategies for green technology innovation (Achi et al., 2022). The one which is aimed to change or modify product designs by using nontoxic compounds or biodegradable materials during the manufacturing process to reduce environmental impact and improve energy efficiency is known as a green product innovation (Cao et al., 2021).
In the financial sector, green financial innovation is defined as the process of developing and commercializing new financial products following various financial technologies, financial institutions, and financial markets (Akdere & Benli, 2018) same with the concept of green products innovation. Furthermore (Allen et al., 2009) defined green financial innovation as a process change that includes a change in various types of financial products. Furthermore the consequence is related to financial institutions' costs decreasing or increasing, because every change in their operations through financial technology leads to a change in their financial position (Baah et al., 2021). More specifically, the study conducted by (Akdere & Benli, 2018) shows that; these innovations are helpful in decreasing risks, lowering transaction costs, and improving products and services.
Apart from that; the study conducted by (Allen, 2012) implies that having these innovations can improve the quality of financial services as many of them are active in various technical breakthroughs (Bakari et al., 2018). To discuss the issue in specific sector, in the contemporary corporate environment, the most well-known financial firms, particularly the banking industry are most prominent in terms of products and services, organizational structures, and legal and procedural innovations (Amore & Bennedsen, 2016). The industry is most known from financial sectors in providing technology based variety services that increase customer satisfaction. However, there are variety of factors that affects the level of green innovation, and causes positive as well negative impact on the company's financial performance. According to (Yusr et al., 2020) there have been several factors that affect the level of green innovation to be used by companies which have a direct impact on the financial performance. Hence the relationship between green innovation and financial performance has gained more scholars attention in current academic writing.
The majority of previously conducted studies on the relationship between company's financial performance and green innovation implies that green innovation has a positive impact on firm performance (Aastvedt et al., 2021). As evidence, the study conducted by (Aastvedt et al., 2021) on the impact of green innovation's on oil and gas firms' financial performance implies that company's innovation has a positive impact on its financial performance. The study conducted by (Barra & Ruggiero, 2021) on firm innovation and local bank efficiency in Italy also founds a positive and significant effect of process innovation on banks efficiency. According to the findings of the study conducted by (Zhang et al., 2019), green innovation has a positive and significant effect on firms' performance.
Furthermore, the study conducted by (Lee & Min, 2015; Lin et al., 2019; Rezende et al., 2019) discovered that green innovation has a positive relationship with financial performance as evidenced from different industries. To this end, the study conducted by (Wang et al., 2021) on green process innovation, green product innovation, and its economic performance improvement paths in china implies that; green process innovation and green product innovation can effectively improve the economic performance of enterprises. Furthermore, the study discovered that green process innovation in an enterprise can positively promote green product innovation, and there is also room for technological innovation upgrading. The finding of this study can be supported by the study conducted (Xie et al., 2019), that shows green process innovation has a beneficial impact on green product innovation, and both green process and green product innovation can improve a company's financial performance. On other hand, the study conducted by Walley and Whitehead (1994), showed the negative impact of innovation on financial performance. Furthermore, the study conducted by (Wagner, 2005) lends argument to the study conducted by Walley and Whitehead (1994) suggesting that green innovation has no impact on boosting financial performance. Moreover, Bontis et al. (2018) found a negative influence of green innovation on a company's financial returns. It can be seen that the impact of green innovation on financial performance has a mixed result. This could be reasoned as the studies are conducted under different country, industry, economic position, geographical location, and socio-cultures. At the same time it is possible to observe that the majority of previous studies discovered a positive relationship between financial performance and green innovation.
The present study tries to look at the relationship between the two variables by taking in to account the inconsistency of previously established findings. In doing so, green financial innovation is represented by product innovation of the banking sector, and financial performance is measured by companies return on investment. Examining the relationship between two variables are found important to provide useful information that could deliberately influence the decision of many stake holders (Acar & Yilmaz, 2020). Financial performance is largely defined as the measurement of a firm's asset's ability to generate revenue (Achmad Kuncoro & Sari, 2015) which is determined through return on asset (ROA) and is primarily done by comparing the asset's overall revenue-generating capabilities (Bartolacci et al., 2020). Hence Firm performance is the sum of the firm's internal and external actions (Yang & Luo, 2020). Return on assets (ROA), average annual occupancy rate (AAR), net profit after tax (NPAT), and return on investment (ROI) are some of the most often utilized financial or accounting measures by businesses to measure the firm's financial performance (Temam, 2018). However, the return on assets is the most well-known option for gauging financial performance (Temam, 2018). As a result, return on asset was employed to assess the overall financial performance of Ethiopia's banking sector in this study. Apart from that, as the firm's primary objective is profit maximization, the adoption of green financial innovations needs to consider the metrics like profitability, productivity, growth, stakeholder satisfaction, market share, and competitive position (de Oliveira et al., 2018). As a result, the current study investigates the impact of green financial innovation on financial performance and forecasts future trends for both variables in the Ethiopian banking sector. This study is novel in that, it contributes new finding to the existing literature from product innovation side. Based on previously established theories and empirical studies, we hypothesized the following hypothesis for the study.
Hypothesis 1: Green financial innovation has a positive and significant effect on the financial performance of the Ethiopian banking sector.
To forecast future series and assess the effect of green financial innovation on the financial performance of the Ethiopian banking industry, this study used an explanatory research design and a quantitative research approach. Secondary data was gathered from various websites, publications, and annual reports of the banking sector. In Ethiopia as of 2021, there are 18 commercial banking companies, 16 of which are private and two of which are public. The target population of the study was only seventeen commercial banks, which includes sixteen private banks and one government bank.
Hence all seventeen commercial banks operating in Ethiopia were selected as the study sample by using the census method considering the operating period of 2014 up to 2020 G.C. Census method is used to include all the banking sector in the study sample as it is easily manageable. Census method can be used wherein each and every item in the universe is selected for the data collection. The sampling frame is based on time series quarterly data of the Dependent (Financial performance) and independent (green financial innovation). The data period was selected based on data availability of data for both variables, and the sample period includes 28 quarterly observations for both variables. The sample period was selected purposively to include all the backing sector in to the sample of the study. The rationale for selecting year 2014–2020 was in that; there are some banks which are young in the industry and have no data prior 2014.
The study's purpose was to predict the rate of growth in green financial innovation and its effect on financial performance. In doing so, the study applied two different models. The first model was used for forecasting future green financial innovation transaction value using the autoregressive moving average (ARIMA) model. The ARIMA model can be used to estimate the value of variables based on their trend or to allow them to explain themselves (Hằng & Dũng, 2022). As a result, the study used the mixed autoregressive-moving average model to forecast green financial innovation. The second model was used to examine the effect of green financial innovation on financial performance using the autoregressive distributed lag (ARDL) model.
Regarding the first model (ARIMA model). The process of autoregressive moving average begins with the specification of the model (Ahmar et al., 2022), as it is helpful in the determination of the degree of homogeneity in the time series. In this case, autocorrelation on functions for the time series and their differences are used to make the decision (Maan et al., 2022). Then, values for p and q must be chosen for the ARMA model that will be used to represent the differentiated series (Wanditra et al., 2021). The total and partial autocorrelation functions can provide some guidance on the choice of p and q (Aji et al., 2021).
It is necessary to estimate a model (or a mixture of models) after it has been specified (Tiwari et al., 2022). The model must then be placed through a diagnostic test after it has been estimated. This often entails examining the autocorrelation function of the residuals from the estimated model, and a basic chi-square test can be used to establish whether or not the residuals are uncorrelated in themselves (Aladağ, 2021a). A time series can be utilized for forecasting once it has been approximated and its original specification has been verified. The general equation for autoregressive moving average processes is defined as:
ɸ(β)ẋt=ɵ(β)et | (1) |
This equation is referred to as a mixed autoregressive moving average model of order p and q, ARMA (p, q) (Abbasi et al., 2021). However, when dealing with time-series data, the series can be non-stationary, and a model for such data requires differencing of a process that can reflect homogeneous non-stationary behavior. The equation form given for first differencing is derived from a general model that can represent homogeneous non-stationary behavior (Aladağ, 2021b).
ɸ(1−β)dẋt=ɵ(β)et | (2) |
Where d is the level of difference. The above equation is called an autoregressive integrated moving average (ARIMA) process of order (p, d, q) or ARIMA (p, d, q). Before the model identification, we tested the variables whether they are stationary or not. In doing so we used the Augmented Dickey-Fuller unit root-test method. The result of the augmented Dickey-Fuller unit root test implies that the variable green financial innovation has the t-statistics value of −5.205092 and p-value = 0.0002 which implies that the trend in green financial innovation overtime is stationary without differencing any period lag (Appendix Table A.1). However, the result of the collerogram test which implies autocorrelation of the variables change over time indicated that the variables have a nonstationary trend over time (Appendix Table A.1). The predicted autocorrelations show that the series fades slowly, indicating that it is non-stationary. The following (Figure 3) of the study shows the collerogram test result for the variable green financial innovation.
The second step was to make variables stationary by differentiating them by one-period lag. The same procedure was used and tested by the Augmented Dickey-Fuller unit root-test method and collerogram test method. The test results of both methods showed that the variable is stationary after first differencing (Refer Appendix Table B.1). The next step is to determine the order of the AR and MA terms after getting variable statistics and confirming that they are stationary (Maan et al., 2022). This is intended to be the moment where we choose our model. In this case, higher adjusted R square, the number of most significant variables, lowest Akaike information criterion, and lowest SIGMASQ (Volatility) are among the criteria used for decision (Bousqaoui et al., 2021). The result of output implies that there are two alternative available models suggested for forecasting the variable, and those are ARIMA (1, 1, 1), and ARIMA (2, 1, 1). The following (Table 1) of the study showed the details of alternative models output suggested by the collerogram test.
Model Choice | Significant variables | SIGMASQ (Volatility) | Adjusted R^2 | AIC |
AR111 | 1(AR) | 4.809720 | 0.699066 | −4.129336 |
AR211 | 2 (AR and MA) | 4.100120 | 0.700095 | −4.131372 |
Source: E-views. |
As recommended earlier the selection criteria may vary depending on the scholar's interests, but models with the greatest adjusted R square, the most significant variables, the lowest Akaike information criterion, and the lowest SIGMASQ (Volatility) are seen to be fit (Tiwari et al., 2022). Hence based on this assumption, the study proposed ARMA (211) for forecasting green financial innovation.
The next phase is diagnostic checking after the model has been estimated. That is, assuming the model is capable of forecasting. That means we'll have to look for white noise in the residual. This might be verified by looking at the residual series' stationery. We can utilize either the residuals or the plot of the residual autocorrelations (Appendix Table C.1). We can see that the autocorrelation factor (ACF) and partial autocorrelation (PACF) of the residuals are randomly distributed by looking at the ACF and PACF graphs. At any latency, none of the Q statistics for the ACF are statistically significant. All of these observations support the findings that the residuals are white noise. Furthermore, the following inverse roots show there is no issue of white noise since both AR and MA roots are inside the circle. Hence the model can be used for forecasting the trend of variables.
The second model (Autoregressive Distributed Lag, ARDL) was used to examine the effect of green financial innovation on financial performance in the short and long run. Autoregressive Distributed Lag (ARDL) Model can be used when the series is a combination of I (0), and I (1). The general equation form of the ARDL model is given as:
Yt=μoi+∑pi=1αjyt−1+∑qi=1βjXt−1+εit | (3) |
Yt is a vector, µoi is the intercept, and variables in Xt are allowed to be purely I (0) or I (1) or fractionally integrated; βandα are coefficients; j = 1, ….k is several independent variables. P is the lag length of the dependent variable and q is the optimal lag for independent variables. Finally, εit represents a vector of error terms.
After the general equation was specified, we tested the bound testing approach to investigate the existence of the long-run relationship between variables using the following equation.
ΔROAt=β0+β1ROAt−1+β2GFIt−1∑haλ2ΔGFIt−a+εt | (4) |
The bound test result confirms the existence of the long-run relationship between variables (Appendix Table D.1). Hence the existence of the long-run relationship between variables allow the study to estimate with an error correction model to know the short-run relationship among variables. The following equation is used to investigate the short-run relationship of the variables.
ROAt=β0+β1∑hai(ROAt−1)+β2∑hbi(GFIt−1)+⋯+μECM(−1)εt | (5) |
Once both models are specified, the study conducted several tests to see if the models are transparent and useful for policy recommendations. The tests imply that the model has passed on tests required by classical linear regression assumptions and is viable to use for policy recommendations. The tests included in the study were serial autocorrelation, heteroscedasticity, and Ramsey reset tests (Appendix Table E.1).
We forecasted the expected growth rate of green financial innovation for the coming five years after reviewing the results of the residual diagnostics of the variable. The forecasted results revealed that the variable responded to its historical values. The following figure 7 of the study showed the trend of forecasted and actual series of the variable.
The line for actual and forecast trend series of the variable is approximately fit, as shown in figure 7. In this scenario, it can be shown that the model used to forecast the value of the series is accurate. As a result, the model can be used to make policy recommendations. More specifically, according to forecasted figure results, on average green financial innovation transaction growth is predicted to be 11 percent each quarter, for a total of 44 percent growth per year when compared to the existing level transaction through green financial innovation. It is understood that the growing rate of transaction through green financial innovation is much greater in the coming five years. After forecasting the trend series of the variable we proceed to diagnostic testing. That is, to see if predicting series are good to use for the model. We can see from the autocorrelation plots and the Augmented Dickey-Fuller unit root test of the residuals (Appendix Table C.1) that all the Q statistics for the autocorrelation functions are statistically insignificant at any lag and that the null of the Augmented Dickey-Fuller unit root test is accepted.
After accepting the future implication of the variable. We were interested to examine the effect of green financial innovation on financial performance. As discussed in the methodology part, we applied the Autoregressive distributed lag model (ARDL) to see the short and long-run implications of green financial innovation on financial performance. We estimate the bound testing method to investigate the existence of the long-run relationship between variables and confirm the existence of the long-run relationship (Appendix Table D.1). The long-run regression output implies that; green financial innovation and financial performance have a positive and insignificant relationship in the long run (Refer Appendix Table D.1). Hence since the long estimation of our model shows an insignificant effect, we are interested to examine the effect of green financial innovation on financial performance in the short run. The following (Table 2) of the study shows the short-run estimation of the model.
ECM Regression | ||||||||
Variable | Coefficient | Std. Error | t-Statistic | Prob. | ||||
D (ROA (−1)) | 0.422915 | 0.104104 | 4.062436 | 0.0006 | ||||
D(INNOVATION) | 1.077896 | 0.266070 | 4.051172 | 0.0006 | ||||
CointEq (−1)* | −0.117043 | 0.027479 | −4.259308 | 0.0003 |
The short-run estimation of the model implies that; green financial innovation affects the financial performance of the banking sector positively and significantly at a 1 percent significant level. The coefficient on variable implies that; remaining other things constant 1 percent increase in green financial innovation causes financial performance to increase by 1 percent and statistically significant at 1 percent significant level. The result implies that the transactions made through green financial innovations have a high positive impact on the financial performance of the banking sector in the short run. Hence automated teller machines, mobile banking, internet banking, debit cards, and point of sales machines have a collective positive impact on the financial performance of the banking sector.
The positive link between the variables may be because point-of-sale terminals, online banking, mobile banking, automated teller machines, and debit cards offer a wide range of services to both customers and commercial banks. It gives a great opportunity for the banking industry to improve its financial performance in terms of generating fees from the services given and lowering operational costs. From the customer's standpoint, it is more secure than the traditional banking system since it allows them to ignore having a large cash amount on hand and shield themselves from the risk of having cash on hand.
Apart from that; the last period level of financial performance (one period lag) has a positive and significant effect on current financial performance. As it can be seen from (table 2) the ECM has a negative and significant value. In a dynamic model, the Error Correction Model (ECM) indicates the rate of adjustment to restore equilibrium (Hurley & Papanikolaou, 2021). If ECM is negative and statistically significant, it indicates that the variables are co-integrated over time. The ECM, which indicates the rate of adjustment, is 0.117. It is both correctly signed and statistically significant. This means that in the long run, the short-term disequilibrium, as well as inconsistencies, are adjusted and corrected at a rate of 11.7 percent. The negative sign confirms the existence of long-term equilibrium.
The study's main objective was to forecast the rate of growth of green financial innovation in Ethiopia's banking sector, as well as examine its impact on financial performance in the short and long run. The study used an explanatory research design and a quantitative research approach to achieve the study's objective. Secondary time series data collected quarterly during the study period (2014–2020) was utilized to run the regression model. An autoregressive moving average (ARIMA) was used to forecast the growing level of green financial innovation. According to forecasted results, on average green financial innovation transaction is expected to grow by 11 percent each quarter. To examine the effect of green financial innovation on the financial performance of the banking sector, an autoregressive distributed lag model (ARDL) was used. The finding of the study implies that green financial innovation has a positive and significant effect on financial performance in the short run. However, the long-run estimation shows a positive and insignificant effect of green financial innovation on financial performance. Based on the study's findings, we recommend that the banking sector focuses on adopting green financial innovations to improve financial performance by taking into account both the short-run and long-run benefits of the products. At the same time, we suggest that the sector focus on those green financial innovations which have the lowest application costs compared to others since the long-run effect affects the overall financial performance of the sector. At the same time, as the study's findings provide insight into insignificant long-run effect; our advice to the sector is to prioritize non-automated teller machine green financial innovation (mobile banking, internet banking, point of sales machines, and debit cards) over automated teller machine, as previously conducted studies argued that automated teller machines are not supposed to be beneficial to the financial sector because of the high cost of installation, restrictions on withdrawals, risk of robbery, run out of coins, energy consumption level, and unavailability of the product in the rural areas (Temam, 2018).
The study was limited to examining the effect of green financial innovation on financial performance based on aggregate data. However, we were interested to examine the response of each bank's financial performance to green financial innovation based on disaggregated data but could not find the data because of poor data management and the unwillingness of some banks. As a result, the researchers advise other scholars to find a way to collect disaggregated data and examine how green financial innovation responded to each bank's financial performance.
All authors declare no conflicts of interest in this paper.
[1] |
Aastvedt T, Behmiri N, Lu L (2021) Does green innovation damage financial performance of oil and gas companies? Resour Policy 73. https://doi.org/10.1016/j.resourpol.2021.102235 doi: 10.1016/j.resourpol.2021.102235
![]() |
[2] |
Abbasi A, Khalili K, Behmanesh J, Shirzad A (2021) Estimation of ARIMA model parameters for drought prediction using the genetic algorithm. Arab J Geosci 14. https://doi.org/10.1007/s12517-021-07140-0 doi: 10.1007/s12517-021-07140-0
![]() |
[3] |
Acar G, Yilmaz I (2020) The impact of discretionary accruals on corporate investment decisions: Evidence from GCC countries. Acad J Stud 9: 193-205. https://doi.org/10.36941/AJIS-2020-0124 doi: 10.36941/AJIS-2020-0124
![]() |
[4] |
Achi A, Adeola O, Achi FC (2022) CSR and green process innovation as antecedents of micro, small, and medium enterprise performance: Moderating role of perceived environmental volatility. J Bus Res 139: 771-781. https://doi.org/10.1016/j.jbusres.2021.10.016 doi: 10.1016/j.jbusres.2021.10.016
![]() |
[5] |
Achmad Kuncoro E, Sari SA (2015) Factors that affect competitive advantage in freight forwarding industry on Jakarta-Indonesia. Adv Sci Lett 21: 1008-1011. https://doi.org/10.1166/asl.2015.5968 doi: 10.1166/asl.2015.5968
![]() |
[6] |
Acquah ISK, Essel D, Baah C, et al., (2021) Investigating the efficacy of isomorphic pressures on the adoption of green manufacturing practices and its influence on organizational legitimacy and financial performance. J Manuf Technol Mana 32: 1399-1420. https://doi.org/10.1108/JMTM-10-2020-0404 doi: 10.1108/JMTM-10-2020-0404
![]() |
[7] |
Adhikari P, Momaya KS (2021) Innovation Capabilities, Environmentally Sustainable Practices and Export Competitiveness: An Exploratory Study of Firms From India. Int J Innov Technol Manage 18. https://doi.org/10.1142/S0219877021500358 doi: 10.1142/S0219877021500358
![]() |
[8] |
Agarwal S, Zhang J (2020) FinTech, Lending and Payment Innovation: A Review. Asia-Pacific J Financ Stud 49: 353-367. https://doi.org/10.1111/ajfs.12294 doi: 10.1111/ajfs.12294
![]() |
[9] |
Aguilera-Caracuel J, Ortiz-de-Mandojana N (2013) Green Innovation and Financial Performance: An Institutional Approach. Organ Environ 26: 365-385. https://doi.org/10.1177/1086026613507931 doi: 10.1177/1086026613507931
![]() |
[10] |
Ahmad M, Wu Y (2022) Combined role of green productivity growth, economic globalization, and eco-innovation in achieving ecological sustainability for OECD economies. J Environ Manage 302. https://doi.org/10.1016/j.jenvman.2021.113980 doi: 10.1016/j.jenvman.2021.113980
![]() |
[11] |
Ahmar AS, del Val EB, Safty MAE, et al., (2022) SutteARIMA: A novel method for forecasting the infant mortality rate in Indonesia. Comput Mater Con 70: 6007-6022. https://doi.org/10.32604/cmc.2022.021382 doi: 10.32604/cmc.2022.021382
![]() |
[12] |
Aji BS, Indwiarti, Rohmawati AA (2021) Forecasting Number of COVID-19 Cases in Indonesia with ARIMA and ARIMAX Models. 2021 9th International Conference on Information and Communication Technology (ICoICT) 71-75. https://doi.org/10.1109/ICoICT52021.2021.9527453 doi: 10.1109/ICoICT52021.2021.9527453
![]() |
[13] |
Akdere C, Benli P (2018) The Nature of Financial Innovation: A Post-Schumpeterian Analysis. J Econ Issues 52: 717-748. https://doi.org/10.1080/00213624.2018.1498717 doi: 10.1080/00213624.2018.1498717
![]() |
[14] |
Aladağ E (2021a) Forecasting of particulate matter with a hybrid ARIMA model based on wavelet transformation and seasonal adjustment. Urban Clim 39. https://doi.org/10.1016/j.uclim.2021.100930 doi: 10.1016/j.uclim.2021.100930
![]() |
[15] |
Aladağ E (2021b) Forecasting of particulate matter with a hybrid ARIMA model based on wavelet transformation and seasonal adjustment. Urban Clim 39. https://doi.org/10.1016/j.uclim.2021.100930 doi: 10.1016/j.uclim.2021.100930
![]() |
[16] |
Allen F (2012) Trends Financial Innovation and their Welfare Impact: An Overview. Eur Financ Manag 18: 493-514. https://doi.org/10.1111/j.1468-036X.2012.00658.x doi: 10.1111/j.1468-036X.2012.00658.x
![]() |
[17] | Allen F, Qian JQJ, Qian M, et al., (2009) A Review of China's Financial System and Initiatives for the Future, In: Barth, J.R., Tatom, J.A., Yago, G., China's Emerging Financial Markets, Springer, Boston, MA, 8: 3-72. https://doi.org/10.1007/978-0-387-93769-4_1 |
[18] |
Amore MD, Bennedsen M (2016) Corporate governance and green innovation. J Environ Econ Manag 75: 54-72. https://doi.org/10.1016/j.jeem.2015.11.003 doi: 10.1016/j.jeem.2015.11.003
![]() |
[19] |
Aron A, Molina O (2020) Green innovation in natural resource industries: The case of local suppliers in the Peruvian mining industry. Extract Ind Soc 7: 353-365. https://doi.org/10.1016/j.exis.2019.09.002 doi: 10.1016/j.exis.2019.09.002
![]() |
[20] |
Asadi S, OmSalameh Pourhashemi S, Nilashi M, et al., (2020) Investigating influence of green innovation on sustainability performance: A case on Malaysian hotel industry. J Clean Prod 258. https://doi.org/10.1016/j.jclepro.2020.120860 doi: 10.1016/j.jclepro.2020.120860
![]() |
[21] |
Asni N, Agustia D (2021) The mediating role of financial performance in the relationship between green innovation and firm value: Evidence from ASEAN countries. Eur J Innov Manag. https://doi.org/10.1108/EJIM-11-2020-0459 doi: 10.1108/EJIM-11-2020-0459
![]() |
[22] |
Baah C, Opoku-Agyeman D, Acquah ISK, et al., (2021) Examining the correlations between stakeholder pressures, green production practices, firm reputation, environmental and financial performance: Evidence from manufacturing SMEs. Sustain Prod Consump 27: 100-114. https://doi.org/10.1016/j.spc.2020.10.015 doi: 10.1016/j.spc.2020.10.015
![]() |
[23] |
Bakari IH, Idi A, Ibrahim Y (2018) Innovation Determinants of Financial Inclusion in Top Ten African Countries: a System GMM Approach. Market Manag Innov 4: 98-106. https://doi.org/10.21272/mmi.2018.4-09 doi: 10.21272/mmi.2018.4-09
![]() |
[24] |
Barra C, Ruggiero N (2021) Firm innovation and local bank efficiency in Italy: Does the type of bank matter? Ann Public Coop Econ, 1-46. https://doi.org/10.1111/apce.12345 doi: 10.1111/apce.12345
![]() |
[25] |
Bartolacci F, Caputo A, Soverchia M (2020) Sustainability and financial performance of small and medium sized enterprises: A bibliometric and systematic literature review. Bus Strateg Environ 29: 1297-1309. https://doi.org/10.1002/bse.2434 doi: 10.1002/bse.2434
![]() |
[26] |
Benfratello L, Schiantarelli F, Sembenelli A (2008) Banks and innovation: Microeconometric evidence on Italian firms. J Financ Econ 90: 197-217. ELSEVIER SCIENCE SA. https://doi.org/10.1016/j.jfineco.2008.01.001 doi: 10.1016/j.jfineco.2008.01.001
![]() |
[27] |
Bontis N, Ciambotti M, Palazzi F, et al. (2018) Intellectual capital and financial performance in social cooperative enterprises. J Intellect Cap 19: 294-320. https://doi.org/10.1108/JIC-03-2017-0049 doi: 10.1108/JIC-03-2017-0049
![]() |
[28] |
Bousqaoui H, Slimani I, Achchab S (2021) Comparative analysis of short-term demand predicting models using ARIMA and deep learning. Int J Elec Comput Eng 11: 3319-3328. Scopus. https://doi.org/10.11591/ijece.v11i4.pp3319-3328 doi: 10.11591/ijece.v11i4.pp3319-3328
![]() |
[29] |
Calomiris CW (2009) Financial innovation, regulation, and reform. Cato J 29: 65-91. https://doi.org/10.7916/D87M0JFV doi: 10.7916/D87M0JFV
![]() |
[30] |
Cao S, Nie L, Sun H, et al., (2021) Digital finance, green technological innovation and energy-environmental performance: Evidence from China's regional economies. J Clean Prod 327. https://doi.org/10.1016/j.jclepro.2021.129458 doi: 10.1016/j.jclepro.2021.129458
![]() |
[31] |
Corrocher N, Ozman M (2020) Green technological diversification of European ICT firms: A patent-based analysis. Econ Innov New Tech 29: 559-581. https://doi.org/10.1080/10438599.2019.1645989 doi: 10.1080/10438599.2019.1645989
![]() |
[32] |
de Oliveira JA, Cruz Basso LF, Kimura H, et al., (2018) Innovation and financial performance of companies doing business in Brazil. Int J Innov Stud 2: 153-164. https://doi.org/10.1016/j.ijis.2019.03.001 doi: 10.1016/j.ijis.2019.03.001
![]() |
[33] | Hằng LTT, Dũng NX (2022) ARIMA Model-Vietnam's GDP Forecasting, In: Ngoc Thach, N., Ha, D.T., Trung, N.D., Kreinovich, V., Prediction and Causality in Econometrics and Related Topics, Springer, Cham, 983: 145-151. https://doi.org/10.1007/978-3-030-77094-5_14 |
[34] |
Hurley DT, Papanikolaou N (2021) Autoregressive Distributed Lag (ARDL) analysis of U.S.-China commodity trade dynamics. Q Rev Econ Financ 81: 454-467. https://doi.org/10.1016/j.qref.2020.10.019 doi: 10.1016/j.qref.2020.10.019
![]() |
[35] |
Lee KH, Min B (2015) Green R & D for eco-innovation and its impact on carbon emissions and firm performance. J Clean Prod 108: 534-542. https://doi.org/10.1016/j.jclepro.2015.05.114 doi: 10.1016/j.jclepro.2015.05.114
![]() |
[36] |
Li Y, Ye F, Sheu C, et al., (2018) Linking green market orientation and performance: Antecedents and processes. J Clean Prod 192: 924-931. https://doi.org/10.1016/j.jclepro.2018.05.052 doi: 10.1016/j.jclepro.2018.05.052
![]() |
[37] |
Lin WL, Cheah JH, Azali M, et al., (2019) Does firm size matter? Evidence on the impact of the green innovation strategy on corporate financial performance in the automotive sector. J Clean Prod 229: 974-988. https://doi.org/10.1016/j.jclepro.2019.04.214 doi: 10.1016/j.jclepro.2019.04.214
![]() |
[38] | Maan S, Devi G, Rizvi SAM (2022) To Predicit the Layout of COVID-19 by Using ARIMA Model. In: Somani, A.K., Mundra, A., Doss, R., Bhattacharya, S., Smart Innovation, Systems and Technologies, Singapore: Springer, 235: 633-641. https://doi.org/10.1007/978-981-16-2877-1_58 |
[39] |
Merton RC (1992) Financial Innovation and Economic Performance. J Appl Corp Financ 4: 12-22. https://doi.org/10.1111/j.1745-6622.1992.tb00214.x doi: 10.1111/j.1745-6622.1992.tb00214.x
![]() |
[40] | National Bank of Ethiopia (2020) Annual report 2020-2021. Available from: https://nbe.gov.et/annual-report/. |
[41] |
Rezende LA, Bansi AC, Alves MFR, et al., (2019) Take your time: Examining when green innovation affects financial performance in multinationals. J Clean Prod 233: 993-1003. https://doi.org/10.1016/j.jclepro.2019.06.135 doi: 10.1016/j.jclepro.2019.06.135
![]() |
[42] | Temam R (2018) The effect of financial innovation on financial performance of commercial banks in Ethiopia. Available from: http://etd.aau.edu.et/handle/123456789/13307 |
[43] | Tiwari SK, Kumaraswamidhas LA, Garg N (2022) Comparison of SVM and ARIMA Model in Time-Series Forecasting of Ambient Noise Levels, In: Bansal, R.C., Agarwal, A., Jadoun, V.K., Lecture Notes in Electrical Engineering, Singapore: Springer, 766: 777-786. https://doi.org/10.1007/978-981-16-1476-7_69 |
[44] | Wagner ED (2005) Enabling mobile learning. EDUCAUSE review 40: 41-42. |
[45] | Walley N, Whitehead B (1994) It's not easy being green. Reader Bus Environ 36. |
[46] |
Wanditra LC, Alamsyah IM, Rachmaputri G, et al., (2021) Forecasting total suspended solid using wavelet ARIMA model. AIP Conference Proceedings 2423. https://doi.org/10.1063/5.0075561 doi: 10.1063/5.0075561
![]() |
[47] |
Wang M, Li Y, Li J, et al., (2021) Green process innovation, green product innovation and its economic performance improvement paths: A survey and structural model. J Environ Manage 297. https://doi.org/10.1016/j.jenvman.2021.113282 doi: 10.1016/j.jenvman.2021.113282
![]() |
[48] |
Xie X, Huo J, Zou H (2019) Green process innovation, green product innovation, and corporate financial performance: A content analysis method. J Bus Res 101: 697-706. https://doi.org/10.1016/j.jbusres.2019.01.010 doi: 10.1016/j.jbusres.2019.01.010
![]() |
[49] |
Yang J, Luo P (2020) Review on international comparison of carbon financial market. Green Financ 2: 55-74. https://doi.org/10.3934/GF.2020004 doi: 10.3934/GF.2020004
![]() |
[50] |
Yusr MM, Salimon MG, Mokhtar SSM, et al., (2020) Green innovation performance! How to be achieved? A study applied on Malaysian manufacturing sector. Sustain Futures 2. https://doi.org/10.1016/j.sftr.2020.100040 doi: 10.1016/j.sftr.2020.100040
![]() |
[51] |
Zhang D, Rong Z, Ji Q (2019) Green innovation and firm performance: Evidence from listed companies in China. Resour Conserv Recy 144: 48-55. https://doi.org/10.1016/j.resconrec.2019.01.023 doi: 10.1016/j.resconrec.2019.01.023
![]() |
![]() |
![]() |
1. | Liping Zheng, Jia Liao, Yuan Yu, Bin Mo, Yun Liu, The effect credit term structure of monetary policy on firms' "short-term debt for long-term investment" behavior: empirical evidence from China, 2023, 31, 2688-1594, 1498, 10.3934/era.2023076 | |
2. | Zexia Zhao, Peiqiong Wang, The impact of managerial competence on corporate carbon performance: An empirical study based on Chinese heavy polluters, 2023, 11, 2296-598X, 10.3389/fenrg.2023.1130339 | |
3. | Zhenghui Li, Bin Chen, Siting Lu, The impact of information and communication technology on financial inclusion-based on a global perspective, 2022, 7, 2473-6988, 20930, 10.3934/math.20221147 | |
4. | Yue Liu, Jinzhi Liu, Lichang Zhang, Enterprise financialization and R&D innovation: A case study of listed companies in China, 2023, 31, 2688-1594, 2447, 10.3934/era.2023124 | |
5. | Yu Zhang, Swarn Chatterjee, Financial Well-Being in the United States: The Roles of Financial Literacy and Financial Stress, 2023, 15, 2071-1050, 4505, 10.3390/su15054505 | |
6. | Xinmiao Zhou, Hongyuan Lu, Shengchao Ye, The information transmission and risk contagion effect between green bond market and government bond market in China, 2023, 11, 2296-665X, 10.3389/fenvs.2023.1091203 | |
7. | Kaimeng Zhang, Xihe Liu, Jingjing Wang, Exploring the relationship between corporate ESG information disclosure and audit fees: evidence from non-financial A-share listed companies in China, 2023, 11, 2296-665X, 10.3389/fenvs.2023.1196728 | |
8. | Jia-Hui Meng, Jian Wang, Yawen Liu, How is government embedded in innovation process for breakthroughs? A meta-synthesis of qualitative case studies, 2023, 194, 00401625, 122735, 10.1016/j.techfore.2023.122735 | |
9. | Yue Ma, Zhongfei Li, Robust portfolio choice with limited attention, 2023, 31, 2688-1594, 3666, 10.3934/era.2023186 | |
10. | Yixian Wen, Yanting Xu, Statistical monitoring of economic growth momentum transformation: empirical study of Chinese provinces, 2023, 8, 2473-6988, 24825, 10.3934/math.20231266 | |
11. | Xueqin Dong, Dongdong Dong, Qing Yu, Green finance innovation and carbon neutrality strategies: sustainable development pathways and policy frameworks, 2024, 12, 2296-665X, 10.3389/fenvs.2024.1485614 |
Model Choice | Significant variables | SIGMASQ (Volatility) | Adjusted R^2 | AIC |
AR111 | 1(AR) | 4.809720 | 0.699066 | −4.129336 |
AR211 | 2 (AR and MA) | 4.100120 | 0.700095 | −4.131372 |
Source: E-views. |
ECM Regression | ||||||||
Variable | Coefficient | Std. Error | t-Statistic | Prob. | ||||
D (ROA (−1)) | 0.422915 | 0.104104 | 4.062436 | 0.0006 | ||||
D(INNOVATION) | 1.077896 | 0.266070 | 4.051172 | 0.0006 | ||||
CointEq (−1)* | −0.117043 | 0.027479 | −4.259308 | 0.0003 |
Model Choice | Significant variables | SIGMASQ (Volatility) | Adjusted R^2 | AIC |
AR111 | 1(AR) | 4.809720 | 0.699066 | −4.129336 |
AR211 | 2 (AR and MA) | 4.100120 | 0.700095 | −4.131372 |
Source: E-views. |
ECM Regression | ||||||||
Variable | Coefficient | Std. Error | t-Statistic | Prob. | ||||
D (ROA (−1)) | 0.422915 | 0.104104 | 4.062436 | 0.0006 | ||||
D(INNOVATION) | 1.077896 | 0.266070 | 4.051172 | 0.0006 | ||||
CointEq (−1)* | −0.117043 | 0.027479 | −4.259308 | 0.0003 |