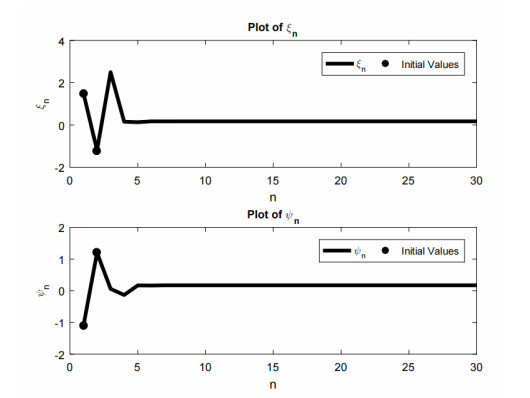
Starting from December 2019, the COVID-19 pandemic has globally strained medical resources and caused significant mortality. It is commonly recognized that the severity of SARS-CoV-2 disease depends on both the comorbidity and the state of the patient's immune system, which is reflected in several biomarkers. The development of early diagnosis and disease severity prediction methods can reduce the burden on the health care system and increase the effectiveness of treatment and rehabilitation of patients with severe cases. This study aims to develop and validate an ensemble machine-learning model based on clinical and immunological features for severity risk assessment and post-COVID rehabilitation duration for SARS-CoV-2 patients. The dataset consisting of 35 features and 122 instances was collected from Lviv regional rehabilitation center. The dataset contains age, gender, weight, height, BMI, CAT, 6-minute walking test, pulse, external respiration function, oxygen saturation, and 15 immunological markers used to predict the relationship between disease duration and biomarkers using the machine learning approach. The predictions are assessed through an area under the receiver-operating curve, classification accuracy, precision, recall, and F1 score performance metrics. A new hybrid ensemble feature selection model for a post-COVID prediction system is proposed as an automatic feature cut-off rank identifier. A three-layer high accuracy stacking ensemble classification model for intelligent analysis of short medical datasets is presented. Together with weak predictors, the associative rules allowed improving the classification quality. The proposed ensemble allows using a random forest model as an aggregator for weak repressors' results generalization. The performance of the three-layer stacking ensemble classification model (AUC 0.978; CA 0.920; F1 score 0.921; precision 0.924; recall 0.920) was higher than five machine learning models, viz. tree algorithm with forward pruning; Naïve Bayes classifier; support vector machine with RBF kernel; logistic regression, and a calibrated learner with sigmoid function and decision threshold optimization. Aging-related biomarkers, viz. CD3+, CD4+, CD8+, CD22+ were examined to predict post-COVID rehabilitation duration. The best accuracy was reached in the case of the support vector machine with the linear kernel (MAPE = 0.0787) and random forest classifier (RMSE = 1.822). The proposed three-layer stacking ensemble classification model predicted SARS-CoV-2 disease severity based on the cytokines and physiological biomarkers. The results point out that changes in studied biomarkers associated with the severity of the disease can be used to monitor the severity and forecast the rehabilitation duration.
Citation: Natalya Shakhovska, Vitaliy Yakovyna, Valentyna Chopyak. A new hybrid ensemble machine-learning model for severity risk assessment and post-COVID prediction system[J]. Mathematical Biosciences and Engineering, 2022, 19(6): 6102-6123. doi: 10.3934/mbe.2022285
[1] | Amira Khelifa, Yacine Halim . Global behavior of P-dimensional difference equations system. Electronic Research Archive, 2021, 29(5): 3121-3139. doi: 10.3934/era.2021029 |
[2] | Tran Hong Thai, Nguyen Anh Dai, Pham Tuan Anh . Global dynamics of some system of second-order difference equations. Electronic Research Archive, 2021, 29(6): 4159-4175. doi: 10.3934/era.2021077 |
[3] | Merve Kara . Investigation of the global dynamics of two exponential-form difference equations systems. Electronic Research Archive, 2023, 31(11): 6697-6724. doi: 10.3934/era.2023338 |
[4] | Haiyu Liu, Rongmin Zhu, Yuxian Geng . Gorenstein global dimensions relative to balanced pairs. Electronic Research Archive, 2020, 28(4): 1563-1571. doi: 10.3934/era.2020082 |
[5] | Bin Wang . Random periodic sequence of globally mean-square exponentially stable discrete-time stochastic genetic regulatory networks with discrete spatial diffusions. Electronic Research Archive, 2023, 31(6): 3097-3122. doi: 10.3934/era.2023157 |
[6] | Ling Xue, Min Zhang, Kun Zhao, Xiaoming Zheng . Controlled dynamics of a chemotaxis model with logarithmic sensitivity by physical boundary conditions. Electronic Research Archive, 2022, 30(12): 4530-4552. doi: 10.3934/era.2022230 |
[7] | Nouressadat Touafek, Durhasan Turgut Tollu, Youssouf Akrour . On a general homogeneous three-dimensional system of difference equations. Electronic Research Archive, 2021, 29(5): 2841-2876. doi: 10.3934/era.2021017 |
[8] | Miao Peng, Rui Lin, Zhengdi Zhang, Lei Huang . The dynamics of a delayed predator-prey model with square root functional response and stage structure. Electronic Research Archive, 2024, 32(5): 3275-3298. doi: 10.3934/era.2024150 |
[9] | Xiaoli Wang, Peter Kloeden, Meihua Yang . Asymptotic behaviour of a neural field lattice model with delays. Electronic Research Archive, 2020, 28(2): 1037-1048. doi: 10.3934/era.2020056 |
[10] | Jiali Shi, Zhongyi Xiang . Mathematical analysis of nonlinear combination drug delivery. Electronic Research Archive, 2025, 33(3): 1812-1835. doi: 10.3934/era.2025082 |
Starting from December 2019, the COVID-19 pandemic has globally strained medical resources and caused significant mortality. It is commonly recognized that the severity of SARS-CoV-2 disease depends on both the comorbidity and the state of the patient's immune system, which is reflected in several biomarkers. The development of early diagnosis and disease severity prediction methods can reduce the burden on the health care system and increase the effectiveness of treatment and rehabilitation of patients with severe cases. This study aims to develop and validate an ensemble machine-learning model based on clinical and immunological features for severity risk assessment and post-COVID rehabilitation duration for SARS-CoV-2 patients. The dataset consisting of 35 features and 122 instances was collected from Lviv regional rehabilitation center. The dataset contains age, gender, weight, height, BMI, CAT, 6-minute walking test, pulse, external respiration function, oxygen saturation, and 15 immunological markers used to predict the relationship between disease duration and biomarkers using the machine learning approach. The predictions are assessed through an area under the receiver-operating curve, classification accuracy, precision, recall, and F1 score performance metrics. A new hybrid ensemble feature selection model for a post-COVID prediction system is proposed as an automatic feature cut-off rank identifier. A three-layer high accuracy stacking ensemble classification model for intelligent analysis of short medical datasets is presented. Together with weak predictors, the associative rules allowed improving the classification quality. The proposed ensemble allows using a random forest model as an aggregator for weak repressors' results generalization. The performance of the three-layer stacking ensemble classification model (AUC 0.978; CA 0.920; F1 score 0.921; precision 0.924; recall 0.920) was higher than five machine learning models, viz. tree algorithm with forward pruning; Naïve Bayes classifier; support vector machine with RBF kernel; logistic regression, and a calibrated learner with sigmoid function and decision threshold optimization. Aging-related biomarkers, viz. CD3+, CD4+, CD8+, CD22+ were examined to predict post-COVID rehabilitation duration. The best accuracy was reached in the case of the support vector machine with the linear kernel (MAPE = 0.0787) and random forest classifier (RMSE = 1.822). The proposed three-layer stacking ensemble classification model predicted SARS-CoV-2 disease severity based on the cytokines and physiological biomarkers. The results point out that changes in studied biomarkers associated with the severity of the disease can be used to monitor the severity and forecast the rehabilitation duration.
The exploration of dynamic systems through mathematical models, particularly within the framework of Caputo-Fabrizio fractional-order models, has garnered significant attention in recent years due to its relevance to attractivity, stability, and periodic chaos in discrete dynamical systems. Through a series of research endeavors, including investigations into Caputo-Fabrizio fractional-order fuzzy competitive neural networks (CF-FCNNs, [1]), almost automorphic fuzzy neural networks [2], multi-delay fractional-order differential equations [3], and stochastic inertial neural networks with spatial diffusions [4], researchers have made strides in understanding the dynamics and convergence properties of these systems. These works have contributed new theories, methods, and computational techniques, shedding light on the existence of unique bounded asymptotically almost periodic solutions, global exponential stability, synchronization phenomena, and random periodicity. Collectively, these findings offer valuable insights into the behavior of difference equation systems and their applications across various scientific and engineering domains. Moreover, within the broader realm of mathematical frameworks, rational difference equations and systems emerge as powerful tools for capturing the evolution of discrete dynamical systems. Recently, there has been a noteworthy surge in mathematicians' interest in the intricate dynamics exhibited by rational difference equations and systems of difference equations. This research area has proven exceptionally fruitful, significantly contributing to the foundational theory surrounding the qualitative behavior of nonlinear rational difference equations and systems. Studying rational difference equations and systems is essential for several reasons. First, these equations serve as powerful tools for modeling dynamic systems across various fields, including electrical networks, biology genetics, sociology, probability theory, statistical analyses, stochastic time series, finance, economics, and more. Notably, references such as Ghezal et al. [5,6,7,8] offer insights into asymmetric time series models, while Ghezal et al. [9,10,11] provide valuable contributions to stochastic volatility time series models. Additionally, Ghezal et al. [12,13,14,15,16], as well as works by Elaydi [17], Grove and Ladas [18], Kocic and Ladas [19], and Kulenovic and Ladas [20], serve as exemplary references showcasing the diverse applications of these equations across various fields. By understanding the behavior of rational difference equations and systems, researchers can gain valuable insights into the underlying mechanisms governing complex phenomena observed in real-world systems. Second, rational difference equations and systems offer a versatile framework that can capture nonlinear interactions, delays, and other intricate dynamics present in many practical scenarios. This flexibility allows for the development of mathematical models that accurately represent the complexities of dynamic systems. Moreover, the study of rational difference equations and systems presents both theoretical challenges and practical applications. Analyzing the asymptotic stability and long-term behavior of solutions to rational difference equations and systems can be particularly challenging but is also rewarding in terms of understanding system behavior and making predictions. Additionally, advancements in the theory of rational difference equations and systems contribute to the advancement of mathematical knowledge and its applications. By developing new methods and techniques for analyzing rational difference equations and systems, researchers can expand the boundaries of mathematical theory and enhance our ability to model and predict the behavior of complex systems.
This paper rigorously explores and analyzes the qualitative behaviors of solutions, placing particular emphasis on both local and global stability within a specific form of a system of rational difference equations, outlined as follows:
∀n≥0,ξn+1=17−ψn−s(7−ξn−2s−1),ψn+1=17−ξn−s(7−ψn−2s−1), | (1.1) |
where s is a fixed positive integer. The system is initialized with conditions denoted as ξ−v, and ψ−v, v∈{0,1,…,2s+1}, and it is crucial that these initial conditions are real and nonzero.
Understanding the stability of dynamic systems is not only about unraveling intricate behaviors but also discerning the fundamental elements governing their equilibrium. Interestingly, the solution form of some solvable type difference equations and systems can even be expressed in terms of well-known integer sequences such as Fibonacci numbers, Lucas numbers, generalized Fibonacci numbers, etc. Our aim in this paper is to show that system (1.1) is solvable by deriving its closed-form formulas elegantly and to describe the behavior and periodicity of well-defined solutions to system (1.1). One of the reasons that makes this study interesting is that the solution of system (1.1) is expressed in terms of co-balancing numbers. This investigation delves into the fascinating interplay between global stability and co-balancing numbers within the solutions of rational difference equations. As mathematical structures intimately linked to equilibrium states, co-balancing numbers offer a unique lens through which we can interpret and analyze the stability of the system. The specific number 7 holds significance in our study due to its relevance to the underlying mathematical framework and the properties of the system under investigation. However, we acknowledge that the rationale behind the selection of this particular number is that the solution of system (1.1) is expressed in terms of co-balancing numbers, enabling us to obtain real, non-complex solutions. Numerous researchers have dedicated their efforts to unraveling the qualitative nuances of solutions to rational difference equations, exploring aspects such as global attractivity, boundedness, and periodicity, shedding light on the rich and multifaceted nature of nonlinear mathematical models. For instance, Ghezal [21] investigated the convergence of positive solutions to a rational system of 4(k+1)−order difference equations given by:
∀n≥0,ξn+1=ξn−(4k+3)1+ψn−kψn−(2k+1)ψn−(3k+2),ψn+1=ψn−(4k+3)1+ξn−kξn−(2k+1)ξn−(3k+2), |
where k is a fixed positive integer. El-sayed [22] contributed to the exploration by investigating the behavior of solutions to the nonlinear difference equation:
∀n≥0, ξn+1=αξn−1+βξnξn−1γξn+δξn−1. |
In [23], Ghezal et al. explored the solvability of a bilinear system of difference equations with coefficients dependent on the Jacobsthal sequence. They studied the local stability of the positive solutions. Simșek et al. [24] investigated the solution of the following difference equation
∀n≥0, ξn+1=ξn−171+ξn−5ξn−11. |
In [25], the solution forms for the following nonlinear difference equations were elucidated:
∀n≥0, ξn+1=1±1+ξn. |
Additionally, Zhang et al. [26] conducted an examination on the boundedness and global asymptotic stability of positive solutions within the system of nonlinear difference equations
∀n≥0,ξn+1=α+1ψn+1−p,ψn+1=α+ψnξn+1−rψn+1−s, |
where s is a fixed positive integer. Furthermore, Zhang et al. [27] delved into the dynamics of a system governed by the nonlinear difference equations
∀n≥0,ξn+1=ξn−2β+ψnψn−1ψn−2,ψn+1=ψn−2α+ξnξn−1ξn−2. |
In a demonstration by Okumuș [28], it was shown that the following two-dimensional system of difference equations can be successfully solved:
∀n≥0,ξn+1=±11+ψn(±1+ξn−1),ψn+1=±11+ξn(±1−ψn−1). |
Our paper provides a comprehensive investigation into the local and global stability of a system of rational difference equations, elucidating its connection to co-balancing numbers. This work distinguishes itself through its thorough exploration of the intricate dynamics of mathematical models and their stability properties, with a particular emphasis on the broader implications of global stability. By delving into the role of co-balancing numbers, the paper reveals their significance in achieving equilibrium within the solutions of rational difference equations, thereby enriching our understanding of dynamic systems. In comparison to existing works such as [28] which focus on investigating the solutions, stability characteristics, and asymptotic behavior of rational difference equations associated with Tribonacci numbers, our paper broadens the scope to examine the broader implications of global stability and its connection to co-balancing numbers. This broader perspective offers a more comprehensive understanding of the dynamics and equilibrium properties of dynamic systems. Moreover, while [26,27] concentrate on studying the behavior and stability of specific systems of rational difference equations, our paper extends this analysis to explore the broader concept of global stability and its relationship with co-balancing numbers. Additionally, while [25] and [23] delve into the solutions and local stability of specific types of difference equations associated with Fibonacci and Jacobsthal sequences, respectively, our paper broadens the scope to examine the broader implications of global stability and its connection to co-balancing numbers. This extension enhances our understanding of the stability properties of rational difference equations and offers valuable insights into the factors influencing their equilibrium behavior.
Recent advancements in computational methods for solving nonlinear evolution equations and partial differential equations (PDEs) have led to the development of innovative techniques such as the bilinear residual network method and the bilinear neural network method. While these methods primarily focus on obtaining exact analytical solutions for nonlinear PDEs, their underlying principles and computational frameworks share similarities with approaches used to solve systems of difference equations. Both systems of difference equations and PDEs describe the evolution of dynamic systems over discrete or continuous domains, respectively, and often exhibit nonlinear behavior. The computational methods proposed in the referenced works [29,30,31,32] leverage neural network architectures and advanced mathematical techniques to tackle the complexities inherent in solving nonlinear equations. Although the specific applications discussed in these references pertain to PDEs, the principles and methodologies underlying these methods can be adapted and extended to address systems of difference equations. By leveraging the flexibility and efficiency of neural networks and tensor-based approaches, researchers can explore new avenues for solving systems of difference equations, advancing our understanding of discrete dynamical systems and their behaviors. Therefore, while the referenced works focus on PDEs, their methodologies and computational frameworks hold promise for addressing challenges in systems of difference equations and related areas of research.
This paper not only contributes to the academic understanding of mathematical modeling but also provides valuable insights for practitioners in fields where dynamic systems play a crucial role. Through a synthesis of theoretical exploration and illustrative examples, we aim to elucidate the profound implications of global stability and co-balancing numbers in the realm of rational difference equations. For further insights into the system of rational difference equations and related results, researchers can explore the extensive body of work referenced in Abo-Zeid [33], Elsayed et al. [34,35,36], Kara [37], Berkal and Abo-Zeid [38], as well as the contributions by Ghezal and Zemmouri [39,40,41]. These references offer valuable insights into various aspects of rational difference equations, including global behavior, solution structures, periodicity, and stability properties. Additionally, Kara's investigation [37] provides further exploration into the dynamics of exponential-form difference equation systems.
We begin by recalling co-balancing numbers as defined in [42,43], which will be instrumental in our analysis of the solution to the system of difference equations (1.1).
Definition 2.1. A positive integer n is termed a balancing number if
1+2+⋯+(n−1)=(n+1)+(n+2)+⋯+(n+r), | (2.1) |
for some r∈N. Here, r is referred to as the balancer corresponding to the balancing number n. [44] demonstrated in a joint study that the balancing numbers satisfy the following recurrence relation:
Bn+1=6Bn−Bn−1, n≥1, |
with B0=0 and B1=1. The Binet formula is Bn=(τn−κn)/(τ−κ) for all n≥0, where κ=3−2√2 and τ=3+2√2.
Definition 2.2. The quotient of two consecutive terms of balancing numbers tends to a, that is, limBn+1/Bn=τ.
Definition 2.3. After a slight modification to (2.1), we call a natural number n a co-balancing number if it satisfies:
1+2+⋯+n=(n+1)+(n+2)+⋯+(n+r), | (2.2) |
and if the pair (n,r)∈ N is a solution to (2.2), then n is called the co-balancing number and r is termed the co-balancer associated with n.
Definition 2.4. The co-balancing sequence is defined by (bn) for n∈N, where
bn+1=6bn−bn−1+2, n≥2, |
with b1=0 and b2=2. The Binet formula for bn is given by bn=−1/2+(τn−1/2−κn−1/2)/(τ−κ) for all n≥0.
Our exploration of the solutions for system (1.1) involves a nuanced analysis of two distinct cases: one where s=0, and another where s>0.
When s=0, system (1.1) takes on the following form
∀n≥0,ξn+1=17−ψn(7−ξn−1),ψn+1=17−ξn(7−ψn−1). | (3.1) |
In this context, we endeavor to derive closed-form solutions for system (3.1). To achieve this, we utilize the following change of variables, denoted by
∀n,ξn=vn−1un,ψn=un−1vn, | (3.2) |
which transforms system (3.1) into its equivalent counterpart:
∀n≥0,{(vnun+1)−1=7−un−1vn(7−vn−2un−1)(unvn+1)−1=7−vn−1un(7−un−2vn−1)⇕∀n≥0,un+1=7vn−7un−1+vn−2,vn+1=7un−7vn−1+un−2. | (3.3) |
To further simplify the last system, we sum and subtract the two equations and introduce a new variable transformation:
∀n, xn+1=un+1+vn+1 and yn+1=un+1−vn+1. | (3.4) |
Therefore, we arrive at an equivalent system given by: ∀n≥0,
xn+1=7xn−7xn−1+xn−2, | (3.5) |
yn+1=−7yn−7yn−1−yn−2. | (3.6) |
Through this, we can deduce closed-form expressions as outlined in the following three Lemmas.
Lemma 3.1. Consider the linear difference equation (3.5) with initial values x−2, x−1, and x0∈R. The general solution {xn,n≥−2} is delineated by the following expression:
∀n,(κ−1)2xn=−κ(x−2+x0)+(κ2+1)x−1+κ2(1κ+1(x−2+κx0)−x−1)Bn+1+(1−κκ+1x−2+(κ−τ)x−1+τ−κ2κ+1x0)Bn−(1κ+1(κx−2+x0)−x−1)Bn−1, |
where (Bn,n≥1) represents the sequence of balancing numbers.
Proof. We commence the proof by employing the characteristic polynomial of Eq (3.5):
λ3−7λ2+7λ−1=(λ−1)(λ−κ)(λ−τ)=0. |
The roots of this equation are λ1=1, λ2=κ, λ3=τ. Consequently, the closed form of the general solution for difference equation (3.5) is expressed as:
∀n≥−2, xn=θ1+θ2κn+θ3τn. |
Here, x−2, x−1, and x0 denote initial values satisfying:
x0=θ1+θ2+θ3,x−1=θ1+τθ2+κθ3,x−2=θ1+τ2θ2+κ2θ3. |
By solving the standard system and conducting subsequent calculations, we obtain:
(κ−1)2θ1=−κ(x−2+x0)+(κ2+1)x−1,(κ−1)2θ2=κ2κ+1(x−2+κx0)−κ2x−1,(κ−1)2θ3=1κ+1(κx−2+x0)−x−1. |
Hence, we derive the following expression:
∀n, (κ−1)2xn=−κ(x−2+x0)+(κ2+1)x−1+κ2(1κ+1(x−2+κx0)−x−1)κn+(1κ+1(κx−2+x0)−x−1)τn, |
and after further calculations and simplification, we arrive at:
∀n, (κ−1)2xn=−κ(x−2+x0)+(κ2+1)x−1+κ2(1κ+1(x−2+κx0)−x−1)Bn+1+(1−κκ+1x−2+(κ−τ)x−1+τ−κ2κ+1x0)Bn−(1κ+1(κx−2+x0)−x−1)Bn−1. |
The lemma is thereby proven.
Lemma 3.2. Consider the linear difference equation (3.6) with initial values y−2, y−1, and y0∈R. The general solution {yn,n≥−2} can be succinctly expressed as:
∀n,(κ−1)2(−1)nyn=−κ(y−2+y0)−(κ2+1)y−1+κ2(1κ+1(y−2+κy0)+y−1)Bn+1+(1−κκ+1y−2+(τ−κ)y−1+τ−κ2κ+1y0)Bn−(1κ+1(κy−2+y0)+y−1)Bn−1. |
Proof. To establish the solution for the difference equation (3.5), we begin by utilizing its characteristic polynomial:
λ3+7λ2+7λ+1=(λ+1)(λ+κ)(λ+τ)=0. |
The roots of this polynomial are denoted as λ1=−1, λ2=−κ, λ3=−τ, then the closed form of the general solution for this difference equation is expressed as:
∀n≥−2, (−1)nyn=ϑ1+ϑ2κn+ϑ3τn. |
Here, y−2, y−1, and y0 are defined by the standard system:
y0=ϑ1+ϑ2+ϑ3,y−1=−ϑ1−τϑ2−κϑ3,y−2=ϑ1+τ2ϑ2+κ2ϑ3. |
Upon solving the standard system and conducting subsequent calculations, the coefficients ϑ1, ϑ2 and ϑ3 are determined as follows:
(κ−1)2ϑ1=−κ(y−2+y0)−(κ2+1)y−1.(κ−1)2ϑ2=κ2κ+1(y−2+κy0)+κ2y−1.(κ−1)2ϑ3=1κ+1(κy−2+y0)+y−1. |
Consequently, the final expression for yn is given by:
∀n, (κ−1)2(−1)nyn=−κ(y−2+y0)−(κ2+1)y−1+κ2(1κ+1(y−2+κy0)+y−1)κn+(1κ+1(κy−2+y0)+y−1)τn. |
Further simplification yields the key result:
∀n, (κ−1)2(−1)nyn=−κ(y−2+y0)−(κ2+1)y−1+κ2(1κ+1(y−2+κy0)+y−1)Bn+1+(1−κκ+1y−2+(τ−κ)y−1+τ−κ2κ+1y0)Bn−(1κ+1(κy−2+y0)+y−1)Bn−1. |
Thus, the lemma is effectively proven.
Lemma 3.3. Consider the system of linear difference equations (3.3) with initial values u−2, u−1, u0, v−2, v−1, and v0∈R. The general solution {(un,vn),n≥−2} can be succinctly expressed as:
∀n,(κ−1)2u2n=−κ(u−2+u0)+(κ2+1)v−1+κ2(1κ+1(u−2+κu0)−v−1)B2n+1+(1−κκ+1u−2+(κ−τ)v−1+τ−κ2κ+1u0)B2n−(1κ+1(κu−2+u0)−v−1)B2n−1, |
∀n,(κ−1)2u2n+1=−κ(v−2+v0)+(κ2+1)u−1+κ2(1κ+1(v−2+κv0)−u−1)B2n+2+(1−κκ+1v−2+(κ−τ)u−1+τ−κ2κ+1v0)B2n+1−(1κ+1(κv−2+v0)−u−1)B2n, |
∀n,(κ−1)2v2n=−κ(v−2+v0)+(κ2+1)u−1+κ2(1κ+1(v−2+κv0)−u−1)B2n+1+(1−κκ+1v−2+(κ−τ)u−1+τ−κ2κ+1v0)B2n−(1κ+1(κv−2+v0)−u−1)B2n−1, |
∀n,(κ−1)2v2n+1=−κ(u−2+u0)+(κ2+1)v−1+κ2(1κ+1(u−2+κu0)−v−1)B2n+2+(1−κκ+1u−2+(κ−τ)v−1+τ−κ2κ+1u0)B2n+1−(1κ+1(κu−2+u0)−v−1)B2n. |
Proof. Through the equivalent linear systems (3.5) and (3.6) and by employing the conversely defined change of variables (3.4), un+1 is represented as (xn+1+yn+1)/2 and vn+1 is represented as (xn+1−yn+1)/2. The subsequent application of Lemmas 3.1 and 3.2 allows us to deduce the closed-form solution for system (3.3) as:
∀n, 2(κ−1)2un=−κ((x−2+(−1)ny−2)+(x0+(−1)ny0))+(κ2+1)(x−1+(−1)n+1y−1)+κ2(1κ+1((x−2+(−1)ny−2)+κ(x0+(−1)ny0))−(x−1−(−1)ny−1))Bn+1+(1−κκ+1(x−2+(−1)ny−2)+(κ−τ)(x−1−(−1)ny−1)+τ−κ2κ+1(x0+(−1)ny0))Bn−(1κ+1(κ(x−2+(−1)ny−2)+(x0+(−1)ny0))−(x−1+(−1)n+1y−1))Bn−1, |
∀n, 2(κ−1)2vn=κ(((−1)ny−2−x−2)+((−1)ny0−x0))+(κ2+1)(x−1+(−1)ny−1)+κ2(1κ+1(((−1)n+1y−2+x−2)+κ((−1)n+1y0+x0))+((−1)n+1y−1−x−1))Bn+1+(1−κκ+1(x−2+(−1)n+1y−2)+(κ−τ)(x−1−(−1)n+1y−1)+τ−κ2κ+1(x0+(−1)n+1y0))Bn+(1κ+1(κ((−1)ny−2−x−2)+((−1)ny0−x0))+(x−1+(−1)ny−1))Bn−1. |
By applying the variable substitution defined in (3.4) once again, the desired results can be obtained. Hence, the lemma is effectively demonstrated.
Below, we derive a main result specific to this subsection and present it in the form of a theorem.
Theorem 3.1. Consider the system of nonlinear difference equations (3.1) with initial values ξ−1, ξ0, ψ−1, and ψ0∈R. The general solution {(ξn,ψn),n≥−1} can be succinctly expressed as:
∀n,ξ2n={−κ(ξ0ψ−1+1)+(κ2+1)ξ0+κ2(1κ+1(ξ0ψ−1+κ)−ξ0)B2n+(1−κκ+1ξ0ψ−1+(κ−τ)ξ0+τ−κ2κ+1)B2n−1−(1κ+1(κξ0ψ−1+1)−ξ0)B2n−2}×{−κ(ξ0ψ−1+1)+(κ2+1)ξ0+κ2(1κ+1(ξ0ψ−1+κ)−ξ0)B2n+1+(1−κκ+1ξ0ψ−1+(κ−τ)ξ0+τ−κ2κ+1)B2n−(1κ+1(κξ0ψ−1+1)−ξ0)B2n−1}−1, |
∀n,ξ2n+1={−κ(ψ0ξ−1+1)+(κ2+1)ψ0+κ2(1κ+1(ψ0ξ−1+κ)−ψ0)B2n+1+(1−κκ+1ψ0ξ−1+(κ−τ)ψ0+τ−κ2κ+1)B2n−(1κ+1(κψ0ξ−1+1)−ψ0)B2n−1}×{−κ(ψ0ξ−1+1)+(κ2+1)ψ0+κ2(1κ+1(ψ0ξ−1+κ)−ψ0)B2n+2+(1−κκ+1ψ0ξ−1+(κ−τ)ψ0+τ−κ2κ+1)B2n+1−(1κ+1(κψ0ξ−1+1)−ψ0)B2n}−1, |
∀n,ψ2n={−κ(ψ0ξ−1+1)+(κ2+1)ψ0+κ2(1κ+1(ψ0ξ−1+κ)−ψ0)B2n+(1−κκ+1ψ0ξ−1+(κ−τ)ψ0+τ−κ2κ+1)B2n−1−(1κ+1(κψ0ξ−1+1)−ψ0)B2n−2}×{−κ(ψ0ξ−1+1)+(κ2+1)ψ0+κ2(1κ+1(ψ0ξ−1+κ)−ψ0)B2n+1+(1−κκ+1ψ0ξ−1+(κ−τ)ψ0+τ−κ2κ+1)B2n−(1κ+1(κψ0ξ−1+1)−ψ0)B2n−1)−1, |
∀n,ψ2n+1={−κ(ξ0ψ−1+1)+(κ2+1)ξ0+κ2(1κ+1(ξ0ψ−1+κ)−ξ0)B2n+1+(1−κκ+1ξ0ψ−1+(κ−τ)ξ0+τ−κ2κ+1)B2n−(1κ+1(κξ0ψ−1+1)−ξ0)B2n−1}×{−κ(ξ0ψ−1+1)+(κ2+1)ξ0+κ2(1κ+1(ξ0ψ−1+κ)−ξ0)B2n+2+(1−κκ+1ξ0ψ−1+(κ−τ)ξ0+τ−κ2κ+1)B2n+1−(1κ+1(κξ0ψ−1+1)−ξ0)B2n}−1. |
Proof. The proof of this theorem relies on Lemma 3.2 and employs the change of variables (3.2). Consequently, we establish the following relationships denoted as
∀n,ξ2n=v2n−1u2n,ξ2n+1=v2nu2n+1,ψ2n=u2n−1v2n,ψ2n+1=u2nv2n+1, |
then, for all n,
∀n, ξ2n={−κ(u−2+u0)+(κ2+1)v−1+κ2(1κ+1(u−2+κu0)−v−1)B2n+(1−κκ+1u−2+(κ−τ)v−1+τ−κ2κ+1u0)B2n−1−(1κ+1(κu−2+u0)−v−1)B2n−2}×{−κ(u−2+u0)+(κ2+1)v−1+κ2(1κ+1(u−2+κu0)−v−1)B2n+1+(1−κκ+1u−2+(κ−τ)v−1+τ−κ2κ+1u0)B2n−(1κ+1(κu−2+u0)−v−1)B2n−1}−1={−κ(ξ0ψ−1+1)+(κ2+1)ξ0+κ2(1κ+1(ξ0ψ−1+κ)−ξ0)B2n+(1−κκ+1ξ0ψ−1+(κ−τ)ξ0+τ−κ2κ+1)B2n−1−(1κ+1(κξ0ψ−1+1)−ξ0)B2n−2}×{−κ(ξ0ψ−1+1)+(κ2+1)ξ0+κ2(1κ+1(ξ0ψ−1+κ)−ξ0)B2n+1+(1−κκ+1ξ0ψ−1+(κ−τ)ξ0+τ−κ2κ+1)B2n−(1κ+1(κξ0ψ−1+1)−ξ0)B2n−1}−1. |
The remaining details are straightforward and omitted for brevity.
Example 3.1. Consider the system (3.1) with initial values ξ−1=1.50, ξ0=−1.20, ψ−1=−1.10, and ψ0=1.20. Figure 1 presents a graphical depiction of the sustained dynamics within this system.
For a 2(s+1)-order system with s>0, our aim is to deduce closed-form solutions for system (1.1). This system is viewed as an extension of system (3.1), represented as follows for all n≥0:
ξ(s+1)(n+1)−m=17−ψ(s+1)n−m(7−ξ(s+1)(n−1)−m), ψ(s+1)(n+1)−m=17−ξ(s+1)n−m(7−ψ(s+1)(n−1)−m), |
for m∈{0,1,…,s} and n∈N. Introducing the notations: Φ(s)n(m)=ξ(s+1)n−m, Ψ(s)n(m)=ψ(s+1)n−m, for m∈{0,1,…,s} and n≥0, we can derive (s+1)−systems analogous to system (3.1),
∀n≥0, Φ(s)n+1(m)=17−Ψ(s)n(m)(7−Φ(s)n−1(m)), Ψ(s)n+1(m)=17−Φ(s)n(m)(7−Ψ(s)n−1(m)), |
for m∈{0,1,…,s}. Based on this discussion, we present the first main result.
Theorem 3.2. Consider the system of nonlinear difference equations (1.1) with initial values ξ−v∈R and ψ−v∈R, v∈{0,1,…,2s+1}. The general solution {(ξn,ψn),n} can be succinctly expressed as: for m∈{0,1,…,s},
∀n,ξ(s+1)(2n)−m={−κ(ξ−mψ−(s+1)−m+1)+(κ2+1)ξ−m+κ2(1κ+1(ξ−mψ−(s+1)−m+κ)−ξ−m)B2n+(1−κκ+1ξ−mψ−(s+1)−m+(κ−τ)ξ−m+τ−κ2κ+1)B2n−1−(1κ+1(κξ−mψ−(s+1)−m+1)−ξ−m)B2n−2}×{−κ(ξ−mψ−(s+1)−m+1)+(κ2+1)ξ−m+κ2(1κ+1(ξ−mψ−(s+1)−m+κ)−ξ−m)B2n+1+(1−κκ+1ξ−mψ−(s+1)−m+(κ−τ)ξ−m+τ−κ2κ+1)B2n−(1κ+1(κξ−mψ−(s+1)−m+1)−ξ−m)B2n−1}−1, |
∀n,ξ(s+1)(2n+1)−m={−κ(ψ−mξ−(s+1)−m+1)+(κ2+1)ψ−m+κ2(1κ+1(ψ−mξ−(s+1)−m+κ)−ψ−m)B2n+1+(1−κκ+1ψ−mξ−(s+1)−m+(κ−τ)ψ−m+τ−κ2κ+1)B2n−(1κ+1(κψ−mξ−(s+1)−m+1)−ψ−m)B2n−1}×{−κ(ψ−mξ−(s+1)−m+1)+(κ2+1)ψ−m+κ2(1κ+1(ψ−mξ−(s+1)−m+κ)−ψ−m)B2n+2+(1−κκ+1ψ−mξ−(s+1)−m+(κ−τ)ψ−m+τ−κ2κ+1)B2n+1−(1κ+1(κψ−mξ−(s+1)−m+1)−ψ−m)B2n}−1, |
∀n,ψ(s+1)(2n)−m={−κ(ψ−mξ−(s+1)−m+1)+(κ2+1)ψ−m+κ2(1κ+1(ψ−mξ−(s+1)−m+κ)−ψ−m)B2n+(1−κκ+1ψ−mξ−(s+1)−m+(κ−τ)ψ−m+τ−κ2κ+1)B2n−1−(1κ+1(κψ−mξ−(s+1)−m+1)−ψ−m)B2n−2}×{−κ(ψ−mξ−(s+1)−m+1)+(κ2+1)ψ−m+κ2(1κ+1(ψ−mξ−(s+1)−m+κ)−ψ−m)B2n+1+(1−κκ+1ψ−mξ−(s+1)−m+(κ−τ)ψ−m+τ−κ2κ+1)B2n−(1κ+1(κψ−mξ−(s+1)−m+1)−ψ−m)B2n−1}, |
∀n,ψ(s+1)(2n+1)−m={−κ(ξ−mψ−(s+1)−m+1)+(κ2+1)ξ−m+κ2(1κ+1(ξ−mψ−(s+1)−m+κ)−ξ−m)B2n+1+(1−κκ+1ξ−mψ−(s+1)−m+(κ−τ)ξ−m+τ−κ2κ+1)B2n−(1κ+1(κξ−mψ−(s+1)−m+1)−ξ−m)B2n−1}×{−κ(ξ−mψ−(s+1)−m+1)+(κ2+1)ξ−m+κ2(1κ+1(ξ−mψ−(s+1)−m+κ)−ξ−m)B2n+2+(1−κκ+1ξ−mψ−(s+1)−m+(κ−τ)ξ−m+τ−κ2κ+1)B2n+1−(1κ+1(κξ−mψ−(s+1)−m+1)−ξ−m)B2n}−1. |
Proof. Utilizing the (s+1)−systems analogous to system (3.1) and applying Theorem 3.1, we conclude the proof of Theorem 3.2.
In the preceding outcome, the solutions of system (1.1) were represented using a balancing sequence, whereas the next result was articulated using a co-balancing sequence.
Corollary 3.1. Consider the system of nonlinear difference equations (1.1) with initial values ξ−v∈R, and ψ−v∈R, v∈{0,1,…,2s+1}. The general solution {(ξn,ψn),n} can be succinctly expressed as: for m∈{0,1,…,s},
∀n,ξ(s+1)(2n)−m={−2κ(ξ−mψ−(s+1)−m+1)+2(κ2+1)ξ−m+κ2(1κ+1(ξ−mψ−(s+1)−m+κ)−ξ−m)b2n+1+(1−κ−κ2κ+1ξ−mψ−(s+1)−m+(κ2+κ−τ)ξ−m+τ−κ2−κ3κ+1)b2n−(1κ+1ξ−mψ−(s+1)−m+(κ−τ−1)ξ−m+τ−κ2+1κ+1)b2n−1+(1κ+1(κξ−mψ−(s+1)−m+1)−ξ−m)b2n−2}×{−2κ(ξ−mψ−(s+1)−m+1)+2(κ2+1)ξ−m+κ2(1κ+1(ξ−mψ−(s+1)−m+κ)−ξ−m)b2n+2+(1−κ−κ2κ+1ξ−mψ−(s+1)−m+(κ2+κ−τ)ξ−m+τ−κ2−κ3κ+1)b2n+1−(1κ+1ξ−mψ−(s+1)−m+(κ−τ−1)ξ−m+τ−κ2+1κ+1)b2n+(1κ+1(κξ−mψ−(s+1)−m+1)−ξ−m)b2n−1}−1, |
∀n,ξ(s+1)(2n+1)−m={−2κ(ψ−mξ−(s+1)−m+1)+2(κ2+1)ψ−m+κ2(1κ+1(ψ−mξ−(s+1)−m+κ)−ψ−m)b2n+2+(1−κ−κ2κ+1ψ−mξ−(s+1)−m+(κ2+κ−τ)ψ−m+τ−κ2−κ3κ+1)b2n+1−(1κ+1ψ−mξ−(s+1)−m+(κ−τ−1)ψ−m+τ−κ2+1κ+1)b2n+(1κ+1(κψ−mξ−(s+1)−m+1)−ψ−m)b2n−1}×{−2κ(ψ−mξ−(s+1)−m+1)+2(κ2+1)ψ−m+κ2(1κ+1(ψ−mξ−(s+1)−m+κ)−ψ−m)b2n+3+(1−κκ2κ+1ψ−mξ−(s+1)−m+(κ2+κ−τ)ψ−m+τ−κ2−κ3κ+1)b2n+2−(1κ+1ψ−mξ−(s+1)−m+(κ−τ−1)ψ−m+τ−κ2+1κ+1)b2n+1+(1κ+1(κψ−mξ−(s+1)−m+1)−ψ−m)b2n}−1, |
∀n,ψ(s+1)(2n)−m={−2κ(ψ−mξ−(s+1)−m+1)+2(κ2+1)ψ−m+κ2(1κ+1(ψ−mξ−(s+1)−m+κ)−ψ−m)b2n+1+(1−κ−κ2κ+1ψ−mξ−(s+1)−m+(κ2+κ−τ)ψ−m+τ−κ2−κ3κ+1)b2n−(1κ+1ψ−mξ−(s+1)−m+(κ−τ−1)ψ−m+τ−κ2+1κ+1)b2n−1+(1κ+1(κψ−mξ−(s+1)−m+1)−ψ−m)b2n−2}×{−2κ(ψ−mξ−(s+1)−m+1)+2(κ2+1)ψ−m+κ2(1κ+1(ψ−mξ−(s+1)−m+κ)−ψ−m)b2n+2+(1−κ−κ2κ+1ψ−mξ−(s+1)−m+(κ2+κ−τ)ψ−m+τ−κ2−κ3κ+1)b2n+1−(1κ+1ψ−mξ−(s+1)−m+(κ−τ−1)ψ−m+τ−κ2+1κ+1)b2n+(1κ+1(κψ−mξ−(s+1)−m+1)−ψ−m)b2n−1}−1, |
∀n,ψ(s+1)(2n+1)−m={−2κ(ξ−mψ−(s+1)−m+1)+2(κ2+1)ξ−m+κ2(1κ+1(ξ−mψ−(s+1)−m+κ)−ξ−m)b2n+2+(1−κ−κ2κ+1ξ−mψ−(s+1)−m+(κ2+κ−τ)ξ−m+τ−κ2−κ3κ+1)b2n+1−(1κ+1ξ−mψ−(s+1)−m+(κ−τ−1)ξ−m+τ−κ2+1κ+1)b2n+(1κ+1(κξ−mψ−(s+1)−m+1)−ξ−m)b2n−1}×{−2κ(ξ−mψ−(s+1)−m+1)+2(κ2+1)ξ−m+κ2(1κ+1(ξ−mψ−(s+1)−m+κ)−ξ−m)b2n+3+(1−κ−κ2κ+1ξ−mψ−(s+1)−m+(κ2+κ−τ)ξ−m+τ−κ2−κ3κ+1)b2n+2−(1κ+1ξ−mψ−(s+1)−m+(κ−τ−1)ξ−m+τ−κ2+1κ+1)b2n+1+(1κ+1(κξ−mψ−(s+1)−m+1)−ξ−m)b2n}−1, |
where (bn,n≥1) represents the sequence of co-balancing numbers.
Proof. The proof of this corollary establishes the following relation between balancing and co-balancing numbers: 2Bn=bn+1−bn. Further details have been omitted.
Remark 3.1. The general solution to the system of nonlinear difference equations (1.1) with initial values ξ−v∈R and ψ−v∈R, v∈{0,1,…,2s+1} is of paramount importance. The general solution {(ξn,ψn), n} can be succinctly expressed as follows: for m∈{0,1,…,s}, for every n, ξ(s+1)(2n)−m, ξ(s+1)(2n+1)−m, ψ(s+1)(2n)−m, and ψ(s+1)(2n+1)−m can be expressed using the proposed formulas, where (Bn) and (bn) represent balancing and cobalancing numbers. This remark illustrates the method by which solutions to the complex system of difference equations can be accurately expressed, highlighting the importance of employing co-balancing numbers in this context.
Corollary 3.2. Consider the following nonlinear difference equation
∀n≥0,ξn+1=17−ξn−s(7−ξn−2s−1), |
where s is a fixed positive integer with initial values ξ−v∈R, v∈{0,1,…,2s+1}. The general solution {ξn,n} can be succinctly expressed as: for m∈{0,1,…,s},
∀n,ξ(s+1)(2n)−m={−κ(ξ−mξ−(s+1)−m+1)+(κ2+1)ξ−m+κ2(1κ+1(ξ−mξ−(s+1)−m+κ)−ξ−m)B2n+(1−κκ+1ξ−mξ−(s+1)−m+(κ−τ)ξ−m+τ−κ2κ+1)B2n−1−(1κ+1(κξ−mξ−(s+1)−m+1)−ξ−m)B2n−2}×{−κ(ξ−mξ−(s+1)−m+1)+(κ2+1)ξ−m+κ2(1κ+1(ξ−mξ−(s+1)−m+κ)−ξ−m)B2n+1+(1−κκ+1ξ−mξ−(s+1)−m+(κ−τ)ξ−m+τ−κ2κ+1)B2n−(1κ+1(κξ−mξ−(s+1)−m+1)−ξ−m)B2n−1}−1, |
∀n,ξ(s+1)(2n+1)−m={−κ(ξ−mξ−(s+1)−m+1)+(κ2+1)ξ−m+κ2(1κ+1(ξ−mξ−(s+1)−m+κ)−ξ−m)B2n+1+(1−κκ+1ξ−mξ−(s+1)−m+(κ−τ)ξ−m+τ−κ2κ+1)B2n−(1κ+1(κξ−mξ−(s+1)−m+1)−ξ−m)B2n−1}×{−κ(ξ−mξ−(s+1)−m+1)+(κ2+1)ξ−m+κ2(1κ+1(ξ−mξ−(s+1)−m+κ)−ξ−m)B2n+2+(1−κκ+1ξ−mξ−(s+1)−m+(κ−τ)ξ−m+τ−κ2κ+1)B2n+1−(1κ+1(κξ−mξ−(s+1)−m+1)−ξ−m)B2n}−1. |
Proof. The proof of the general solution for the nonlinear difference equation is derived from Theorem 3.2 when ξ−v=ψ−v for v∈{0,1,…,2s+1}.
Example 3.2. Consider the system (1.1) with s=1 and initial values ξ−4=1.50, ξ−3=1.20, ξ−2=0.30, ξ−1=0.40, ξ0=0.50, ψ−4=−1.10, ψ−3=−1.20, ψ−2=−1.30, ψ−1=−1.40, and ψ0=−1.50. Figure 2 presents a graphical depiction of the sustained dynamics within this system.
Example 3.3. Consider the system (1.1) with s=2 and initial values ξ−v=1−√v and ψ−v=−1.2exp(v)+2, v∈{0,1,…,6}. Figure 3 presents a graphical depiction of the sustained dynamics within this system.
In this subsection, we first determine the equilibrium points and then proceed to analyze the local and global stability of the solutions of system (1.1). The system has three positive equilibrium points:
Λ_1=(¯ξ1,¯ψ1)=(1,1), Λ_2=(¯ξ2,¯ψ2)=κ(1,1)and Λ_3=(¯ξ3,¯ψ3)=τ(1,1), |
which are solutions to the standard system:
¯ξ=17−¯ψ(7−¯ξ),¯ψ=17−¯ξ(7−¯ψ). |
The following theorem provides information on the local stability of the equilibrium point Λ_2 of system (1.1).
Theorem 3.3. The positive equilibrium point Λ_2 of system (1.1) is locally asymptotically stable.
Proof. In order to analyze the local stability of system (1.1) around the equilibrium point Λ_2, we employ linearized equations. Let ∀n≥0, ξ_n+1=Γ(ξ_n), where ξ_n:=(x_′n,y_′n)′, x_n=(x(1)n,x(2)n,…,x(2s+2)n)′, y_n=(y(1)n,y(2)n,…,y(2s+2)n)′,
{x(1)n=ξn,x(2)n=ξn−1,…,x(2l+2)n=ξn−2s−1y(1)n=ψn,y(2)n=ψn−1,…,y(2l+2)n=ψn−2s−1. |
The mapping Γ is defined as:
Γ:[0,+∞)4(s+1)⟶[0,+∞)4(s+1)Z=(x(1),…,x(2s+2),y(1),…,y(2s+2))⟼Γ(Z)=(Γ1(Z),x(2),…,x(2s+2),Γ2(Z),y(2),…,y(2s+2)) |
where
Γ1(Z)=17−y(s+1)(7−x(2s+2)), Γ2(Z)=17−x(s+1)(7−y(2s+2)). |
The partial derivatives of Γ1(.) (resp., Γ2(.)) with respect to its components are given by:
{∂∂y(s+1)Γ1(Z)=7−x(2s+2)(7−y(s+1)(7−x(2s+2)))2, ∂∂x(2s+2)Γ1(Z)=y(s+1)(7−y(s+1)(7−x(2s+2)))2∂∂x(s+1)Γ2(Z)=7−y(2s+2)(7−x(s+1)(7−y(2s+2)))2, ∂∂y(2s+2)Γ2(Z)=x(s+1)(7−x(s+1)(7−y(2s+2)))2. |
Given the symbols introduced earlier, we can express the linearized system around the equilibrium point Λ_2 as: Γ(ξ_n)=χsξ_n, where χs is the stability matrix defined as:
χs=(00⋯000⋯0κ(κ2−7κ+7)210⋯000⋯0001⋯000⋯00⋮⋮⋱⋱⋱⋱⋱⋮⋮00⋯000⋯1000⋯07−κ(κ2−7κ+7)20⋯0000⋯000⋯0000⋯000⋯00⋮⋮⋱⋱⋱⋱⋱⋮⋮00⋯000⋯0000⋯07−κ(κ2−7κ+7)20⋯0000⋯000⋯0000⋯000⋯00⋮⋮⋱⋱⋱⋱⋱⋮⋮00⋯000⋯0000⋯000⋯0κ(κ2−7κ+7)210⋯000⋯0001⋯000⋯00⋮⋮⋱⋱⋱⋱⋱⋮⋮00⋯000⋯10). |
Upon performing initial computations, we find that the characteristic polynomial of χs is given by:
Pχs(λ)=|χs−λI4(s+1)×4(s+1)|=(λ2s+2)2−(7−κ)2(κ2−7κ+7)4λ2s+2−κ2(κ2−7κ+7)4, |
where I4(s+1)×4(s+1) is the identity matrix of size 4(s+1)×4(s+1). By analyzing the characteristic polynomial, we can determine the eigenvalues of χs. After conducting meticulous calculations, we ascertain that the roots of χs satisfy λ2s+2=(√17−4)(70√2−99) or λ2s+2=(√17+4)(99−70√2). This implies that all the moduli of every eigenvalue of χs are less than one. Therefore, the positive equilibrium point Λ_2 of system (1.1) is concluded to be locally asymptotically stable.
Theorem 3.4. Consider the system of nonlinear difference equations (1.1) with initial values ξ−v∈R, and ψ−v∈R, v∈{0,1,…,2s+1}.
1) Every positive solution {(ξn,ψn),n} of this system converges to the equilibrium point Λ_2 as n⟶∞.
2) The positive equilibrium point Λ_2 of system (1.1) is globally asymptotically stable.
Proof. (ⅰ) Let {(ξn,ψn), n} be a positive solution of system (1.1). We are interested in calculating the limit of the sequences (ξ(s+1)(2n)−m), (ξ(s+1)(2n+1)−m), (ψ(s+1)(2n)−m), and(ψ(s+1)(2n+1)−m), for m∈{0,1,…,s}. To proceed with this calculation, let's start by analyzing the behavior of ξ(s+1)(2n)−m as n approaches infinity.
limnξ(s+1)(2n)−m=limn{(−κ(ξ−mψ−(s+1)−m+1)+(κ2+1)ξ−m)1B2n+1+κ2(1κ+1(ξ−mψ−(s+1)−m+κ)−ξ−m)B2nB2n+1+(1−κκ+1ξ−mψ−(s+1)−m+(κ−τ)ξ−m+τ−κ2κ+1)B2n−1B2n+1−(1κ+1(κξ−mψ−(s+1)−m+1)−ξ−m)B2n−2B2n+1}×{(−κ(ξ−mψ−(s+1)−m+1)+(κ2+1)ξ−m)1B2n+1+κ2(1κ+1(ξ−mψ−(s+1)−m+κ)−ξ−m)+(1−κκ+1ξ−mψ−(s+1)−m+(κ−τ)ξ−m+τ−κ2κ+1)B2nB2n+1−(1κ+1(κξ−mψ−(s+1)−m+1)−ξ−m)B2n−1B2n+1}−1. |
From the given limits: limnB2nB2n+1=κ, limnB2n−1B2n+1=κ2, limnB2n−2B2n+1=κ3, and limn1B2n+1=0, we get
limnξ(s+1)(2n)−m={κ3(1κ+1(ξ−mψ−(s+1)−m+κ)−ξ−m)+κ2(1−κκ+1ξ−mψ−(s+1)−m+(κ−τ)ξ−m+τ−κ2κ+1)−κ3(1κ+1(κξ−mψ−(s+1)−m+1)−ξ−m)}×{κ2(1κ+1(ξ−mψ−(s+1)−m+κ)−ξ−m)+κ(1−κκ+1ξ−mψ−(s+1)−m+(κ−τ)ξ−m+τ−κ2κ+1)−κ2(1κ+1(κξ−mψ−(s+1)−m+1)−ξ−m)}−1=κ, |
for m∈{0,1,…,s}. The same approach can be applied to (ξ(s+1)(2n+1)−m), (ψ(s+1)(2n)−m), and(ψ(s+1)(2n+1)−m), for m∈{0,1,…,s}.
(ⅱ) The proof of the second assertion follows directly from Theorem 3.3 and the first assertion.
Remark 3.2. Throughout the examples in this paper, Figures 1–3 demonstrate that the equilibrium point Λ_2 of system (1.1) is globally asymptotically stable.
This study provided a comprehensive exploration of the dynamics of a system of rational difference equations, investigating both local and global stability aspects. The findings underscored the intricate relationship between stability properties and co-balancing numbers, revealing their significant impact on achieving equilibrium within the solutions of rational difference equations. By examining the role of co-balancing numbers, we gained insights into the interplay between global stability and co-balancing numbers, enhancing our understanding of dynamic systems' mathematical structures. Moreover, this article serves as a valuable resource for mathematicians, researchers, and scholars interested in the intersection of global stability and co-balancing sequences within rational difference equations. The consistently demonstrated global asymptotic stability of the equilibrium point, supported by examples and figures throughout the paper, underscores the significance of the findings. Further research in this direction holds promise for uncovering additional nuances in the intricate relationship between stability and co-balancing numbers within mathematical modeling.
In the realm of integrable systems, the quest for analytical solutions to nonlinear PDEs has long been a challenge due to the absence of a universal method. However, recent breakthroughs in symbolic computation, particularly through the application of neural networks proposed by Zhang et al. [45,46,47,48], have opened a new avenue for the analytical treatment of nonlinear PDEs. These innovative approaches pave the way for symbolic computation to tackle the complexities of nonlinear PDEs, laying the groundwork for a universal method for obtaining analytical expressions. While the focus of these works is primarily on obtaining exact solutions for PDEs, the underlying methodologies and principles can inspire new ideas for future work. A potential open problem that arises from this research is the exploration of applying similar computational techniques to systems of difference equations. By adapting the neural network architectures and symbolic computation methods employed in solving nonlinear PDEs, researchers can investigate the feasibility of obtaining analytical solutions for systems of difference equations, thus advancing our understanding of discrete dynamical systems and their behaviors. This interdisciplinary approach holds promise for addressing longstanding challenges in the field and opens avenues for further exploration at the intersection of computational mathematics and dynamical systems theory.
The authors declare they have not used Artificial Intelligence (AI) tools in the creation of this article.
The authors extend their appreciation to the Deanship of Scientific Research, Vice Presidency for Graduate Studies and Scientific Research at King Faisal University, Saudi Arabia, for financial support under the annual funding track [5964].
The authors declare there is no conflicts of interest.
[1] | A. M. Kalasic, O. K. Vidovic, Aging and health: priorities of the World Health Organization for the decade of healthy aging 2020-2030, Ageing Human Rights, (2018), 67. |
[2] |
M. T. Tull, K. A. Edmonds, K. M. Scamaldo, J. R. Richmond, J. P. Rose, K. L. Gratz, Psychological outcomes associated with stay-at-home orders and the perceived impact of COVID-19 on daily life, Psychiatry Res., 289 (2020), 113098. https://doi.org/10.1016/j.psychres.2020.113098 doi: 10.1016/j.psychres.2020.113098
![]() |
[3] |
W. Gardner, D. States, N. Bagley, The coronavirus and the risks to the elderly in long-term care. J. Aging Soc. Policy, 32 (2020), 310-315. https://doi.org/10.1080/08959420.2020.1750543 doi: 10.1080/08959420.2020.1750543
![]() |
[4] | Covid2019 coronavirus disease, Retrieved on: 26 December 2021, Available from: https://www.worldometers.info/coronavirus/. |
[5] |
G. Wu, P. Yang, Y. Xie, H. C. Woodruff, X. Rao, J. Guiot, et al., Development of a clinical decision support system for severity risk prediction and triage of COVID-19 patients at hospital admission: an international multicentre study, Eur. Respir. J., 56 (2020), 2001104. https://doi.org/10.1183/13993003.01104-2020 doi: 10.1183/13993003.01104-2020
![]() |
[6] |
M. Mittelbrunn, G. Kroemer, Hallmarks of T cell aging, Nat. Immunol., 22 (2021), 687-698. https://doi.org/10.1038/s41590-021-00927-z doi: 10.1038/s41590-021-00927-z
![]() |
[7] |
M. Jiang, Y. Guo, Q. Luo, Z. Huang, R. Zhao, S. Liu, et al., T-Cell subset counts in peripheral blood can be used as discriminatory biomarkers for diagnosis and severity prediction of coronavirus disease 2019, J. Infect. Dis., 222 (2020), 198-202. https://doi.org/10.1093/infdis/jiaa252 doi: 10.1093/infdis/jiaa252
![]() |
[8] |
H. Zhang, X. Wang, Z. Fu, M. Luo, Z. Zhang, K. Zhang, et al., Potential factors for prediction of disease severity of COVID-19 patients, medRxiv, 2020. https://doi.org/10.1101/2020.03.20.20039818 doi: 10.1101/2020.03.20.20039818
![]() |
[9] |
C. Zhang, L. Qin, K. Li, Q. Wang, Y. Zhao, B. Xu, et al., A novel scoring system for prediction of disease severity in COVID-19, Front. Cell. Infect. Microbiol., 10 (2020), 318. https://doi.org/10.3389/fcimb.2020.00318 doi: 10.3389/fcimb.2020.00318
![]() |
[10] |
B. K. Patterson, J. Guevara-Coto, R. Yogendra, E. B. Francisco, E. Long, A. Pise, et al., Immune-based prediction of COVID-19 severity and chronicity decoded using machine learning, Front. Immunol., 12 (2021), 700782. https://doi.org/10.3389/fimmu.2021.700782 doi: 10.3389/fimmu.2021.700782
![]() |
[11] | S. Yasar, C. Colak, S. Yologlu, Artificial intelligence-based prediction of Covid-19 severity on the results of protein profiling, Comput. Methods Program Biomed., 202 (2021), 105996. https://doi.org/10.1016/j.cmpb.2021.105996 |
[12] |
T. D. Bennett, R. A. Moffitt, J. G. Hajagos, B. Amor, A. Anand, M. M. Bissell, et al., Clinical characterization and prediction of clinical severity of SARS-CoV-2 infection among US adults using data from the US national COVID cohort collaborative, JAMA Netw. Open, 4 (2021), e2116901. https://doi.org/10.1001/jamanetworkopen.2021.16901 doi: 10.1001/jamanetworkopen.2021.16901
![]() |
[13] |
M. Balbi, A. Caroli, A. Corsi, G. Milanese, A. Surace, F. Di Marco, et al., Chest X-ray for predicting mortality and the need for ventilatory support in COVID-19 patients presenting to the emergency department, Eur. Radiol., 31 (2021), 1999-2012. https://doi.org/10.1007/s00330-020-07270-1 doi: 10.1007/s00330-020-07270-1
![]() |
[14] |
R. Fusco, R. Grassi, V. Granata, S. V. Setola, F. Grassi, D. Cozzi, et al., Artificial intelligence and COVID-19 using Chest CT scan and Chest X-ray images: Machine learning and deep learning approaches for diagnosis and treatment, J. Pers. Med., 11 (2021), 993. https://doi.org/10.3390/jpm11100993 doi: 10.3390/jpm11100993
![]() |
[15] |
F. Shan, Y. Gao, J. Wang, W. Shi, N. Shi, M. Han, et al., Abnormal lung quantification in chest CT images of COVID-19 patients with deep learning and its application to severity prediction, Med. Phys., 48 (2021), 1633-1645. https://doi.org/10.1002/mp.14609 doi: 10.1002/mp.14609
![]() |
[16] |
Y. Z. Feng, S. Liu, Z. Y. Cheng, J. C. Quiroz, D. Rezazadegan, P. Chen, et al., Severity assessment and progression prediction of COVID-19 patients based on the LesionEncoder framework and chest CT, Information, 12 (2021), 471. https://doi.org/10.3390/info12110471 doi: 10.3390/info12110471
![]() |
[17] |
A. G. Dastider, F. Sadik, S. A. Fattah, An integrated autoencoder-based hybrid CNN-LSTM model for COVID-19 severity prediction from lung ultrasound, Comput. Biol. Med., 132 (2021), 104296. https://doi.org/10.1016/j.compbiomed.2021.104296 doi: 10.1016/j.compbiomed.2021.104296
![]() |
[18] | N. Alballa, I. Al-Turaiki, Machine learning approaches in COVID-19 diagnosis, mortality, and severity risk prediction: A review, Inform. Med. Unlocked, 24 (2021), 100564. https://doi.org/10.1016/j.imu.2021.100564 |
[19] |
Z. Car, S. B. Šegota, N. Anđelić, I. Lorencin, V. Mrzljak, Modeling the spread of COVID-19 infection using a multilayer perceptron, Comput. Math. Methods Med., 29 (2020), 5714714. https://doi.org/10.1155/2020/5714714 doi: 10.1155/2020/5714714
![]() |
[20] |
A. Blagojević, T. Šušteršič, I. Lorencin, S. B. Šegota, N. Anđelić, D. Milovanović, et al., Artificial intelligence approach towards assessment of condition of COVID-19 patients-Identification of predictive biomarkers associated with severity of clinical condition and disease progression, Comput. Biol. Med., 138 (2021), 104869. https://doi.org/10.1016/j.compbiomed.2021.104869 doi: 10.1016/j.compbiomed.2021.104869
![]() |
[21] |
N. Anđelić, S. B. Šegota, I. Lorencin, V. Mrzljak, Z. Car, Estimation of COVID-19 epidemic curves using genetic programming algorithm, Health Informatics J., 27 (2021), 1460458220976728. https://doi.org/10.1177/1460458220976728 doi: 10.1177/1460458220976728
![]() |
[22] |
C. Iwendi, A. K. Bashir, A. Peshkar, R. Sujatha, J. M. Chatterjee, S. Pasupuleti, et al., COVID-19 patient health prediction using boosted random forest algorithm, Front. Public Health, 8 (2020), 357. https://doi.org/10.3389/fpubh.2020.00357 doi: 10.3389/fpubh.2020.00357
![]() |
[23] |
R. K. Zimmerman, M. P. Nowalk, T. Bear, R. Taber, K. S. Clarke, T. M. Sax, et al., Proposed clinical indicators for efficient screening and testing for COVID-19 infection using Classification and Regression Trees (CART) analysis, Hum. Vaccin. Immunother., 17 (2021), 1109-1112. https://doi.org/10.1080/21645515.2020.1822135 doi: 10.1080/21645515.2020.1822135
![]() |
[24] |
K. K. A. Ghany, H. M. Zawbaa, H. M. Sabri, COVID-19 prediction using LSTM algorithm: GCC case study, Inform. Med. Unlocked, 23 (2021), 100566. https://doi.org/10.1016/j.imu.2021.100566 doi: 10.1016/j.imu.2021.100566
![]() |
[25] |
L. J. Muhammad, M. Islam, S. S. Usman, S. I. Ayon, Predictive data mining models for novel coronavirus (COVID-19) infected patients' recovery, SN Comput. Sci., 1 (2020), 1-7. https://doi.org/10.1007/s42979-020-00216-w doi: 10.1007/s42979-020-00216-w
![]() |
[26] | S. K. Bandyopadhyay, S. Dutta, Machine learning approach for confirmation of COVID-19 cases: positive, negative, death and release, medRxiv, 2020. https://doi.org/10.1101/2020.03.25.20043505 |
[27] |
F. De Felice, A. Polimeni, Coronavirus disease (COVID-19): a machine learning bibliometric analysis, In Vivo, 34 (2020), 1613-1617. https://doi.org/10.21873/invivo.11951 doi: 10.21873/invivo.11951
![]() |
[28] |
S. Kushwaha, S. Bahl, A. K. Bagha, K. S. Parmar, M. Javaid, A. Haleem, et al., Significant applications of machine learning for COVID-19 pandemic, J. Ind. Integr. Manag., 5 (2020), 453-479. https://doi.org/10.1142/S2424862220500268 doi: 10.1142/S2424862220500268
![]() |
[29] |
N. S. Punn, S. K. Sonbhadra, S. Agarwal, COVID-19 epidemic analysis using machine learning and deep learning algorithms, MedRxiv, 2020. https://doi.org/10.1101/2020.04.08.20057679 doi: 10.1101/2020.04.08.20057679
![]() |
[30] | Kaggle Datasets, Retrieved on: 26 December 26 2021, Available from: https://www.kaggle.com/search?q=dataset+cd4+covid, |
[31] |
L. Yan, H. T. Zhang, J. Goncalves, Y. Xiao, M. Wang, Y. Guo, et al., An interpretable mortality prediction model for COVID-19 patients, Nat. Mach. Intell., 2 (2020), 283-288. https://doi.org/10.1038/s42256-020-0180-7 doi: 10.1038/s42256-020-0180-7
![]() |
[32] |
A. Trickey, M. T. May, P. Schommers, J. Tate, S. M. Ingle, J. L. Guest, et al., CD4: CD8 ratio and CD8 count as prognostic markers for mortality in human immunodeficiency virus-infected patients on antiretroviral therapy: the Antiretroviral Therapy Cohort Collaboration (ART-CC), Clin. Infect. Dis., 65 (2017), 959-966. https://doi.org/10.1093/cid/cix466 doi: 10.1093/cid/cix466
![]() |
[33] |
N. Hasan, A methodological approach for predicting COVID-19 epidemic using EEMD-ANN hybrid model, Internet Things, 11 (2020), 100228. https://doi.org/10.1016/j.iot.2020.100228 doi: 10.1016/j.iot.2020.100228
![]() |
[34] |
H. M. Gomes, J. P. Barddal, F. Enembreck, A. Bifet, A survey on ensemble learning for data stream classification, ACM Comput. Surveys, 50 (2017), 23. https://doi.org/10.1145/3054925 doi: 10.1145/3054925
![]() |
[35] |
S. Dˇzeroski, B. Zenko, Is combining classifiers with stacking better than selecting the best one?, Mach. Learn., 54 (2004), 255-273. https://doi.org/10.1023/B:MACH.0000015881.36452.6e doi: 10.1023/B:MACH.0000015881.36452.6e
![]() |
[36] |
O. Sagi, L. Rokach, Ensemble learning: A survey, WIREs Data Mining Knowl. Discov., 8 (2018), e1249. https://doi.org/10.1002/widm.1249 doi: 10.1002/widm.1249
![]() |
[37] | The all relevant feature selection using random forest MB Kursa, preprint, arXiv: 1106.5112. |
[38] |
G. Chandrashekar, F. Sahin, A survey on feature selection methods. Comput. Electr.l Eng., 40 (2014), 16-28. https://doi.org/10.1016/j.compeleceng.2013.11.024 doi: 10.1016/j.compeleceng.2013.11.024
![]() |
[39] | A. Bommert, X. Sun, B. Bischl, J. Rahnenführer, M. Lang, Benchmark for filter methods for feature selection in high-dimensional classification data. Comput. Stat. Data Anal., 143 (2020), 106839. https://doi.org/10.1016/j.csda.2019.106839 |
[40] |
B. Venkatesh, J. Anuradha, A review of feature selection and its methods, Cybern. Inform. Technol., 19 (2019), 3-26. https://doi.org/10.2478/cait-2019-0001 doi: 10.2478/cait-2019-0001
![]() |
[41] |
L. N. Sanchez-Pinto, L. R. Venable, J. Fahrenbach, M. M. Churpek, Comparison of variable selection methods for clinical predictive modeling, Int. J. Med. Inform., 116 (2018), 10-17. https://doi.org/10.1016/j.ijmedinf.2018.05.006 doi: 10.1016/j.ijmedinf.2018.05.006
![]() |
[42] |
M. Azmi, G. C. Runger, A. Berrado, Interpretable regularized class association rules algorithm for classification in a categorical data space, Inform. Sci., 483 (2019), 313-331. https://doi.org/10.1016/j.ins.2019.01.047 doi: 10.1016/j.ins.2019.01.047
![]() |
[43] | F. Thabtah, P. Cowling, Y. Peng, MCAR: multi-class classification based on association rule. In The 3rd ACS/IEEE International Conference on Computer Systems and Applications, (2005), 33. https://doi.org/10.1109/AICCSA.2005.1387030 |
[44] | K. Mittal, G. Aggarwal, P. Mahajan, A comparative study of association rule mining techniques and predictive mining approaches for association classification, I. J. Adv. Res. Comput. Sci., 8 (2017). |
[45] | J. Allaire, RStudio: integrated development environment for R, Boston MA, 770 (2012), 165-171. |
[46] | W. Gardner, R. Maliki, S. M. Cutts, B. W. Muir, D. Ballabio, D. A. Winkler, et al., Self-organizing map and relational perspective mapping for the accurate visualization of high-dimensional hyperspectral data, Anal. Chem., 92 (15), 10450-10459. https://doi.org/10.1021/acs.analchem.0c00986 |
[47] |
I. Izonin, R. Tkachenko, N. Shahovska, N. Lotoshynska, The additive input-doubling method based on the SVR with nonlinear kernels: small data approach, Symmetry, 13 (2021), 612. https://doi.org/10.3390/sym13040612 doi: 10.3390/sym13040612
![]() |
[48] |
I. Izonin, R. Tkachenko, I. Droniuk, P. Tkachenko, M. Gregus, M. Rashkevych, Predictive modeling based on small data in clinical medicine: RBF-based additive input-doubling method, Math. Biosci. Eng., 31 (2021), 2599. https://doi.org/10.3934/mbe.2021132 doi: 10.3934/mbe.2021132
![]() |
1. | Hashem Althagafi, Ahmed Ghezal, Solving a system of nonlinear difference equations with bilinear dynamics, 2024, 9, 2473-6988, 34067, 10.3934/math.20241624 |