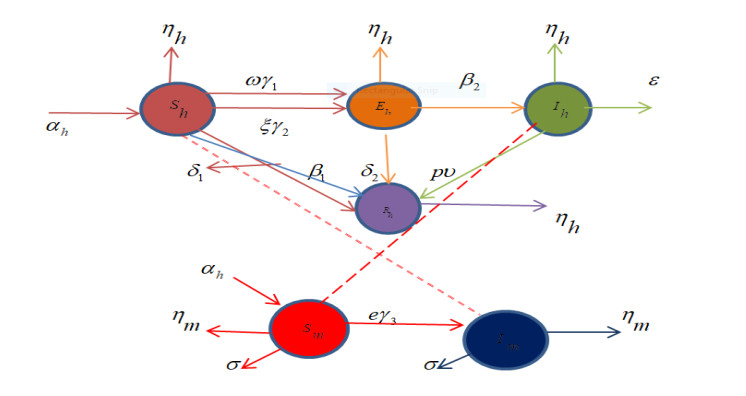
High energy dependence on fossil fuels and an increase in greenhouse gas emissions are factors that highlight the need for alternative energy sources. Photovoltaic technology is a strong candidate that uses the most abundant resource, solar energy, but what makes its wide use difficult is the high cost of the commercially available devices. Thus, research has been devoted to developing new low-cost photovoltaic systems that are easier to manufacture with high efficiency and durability, such as the third-generation solar cells. According to this study, organic solar cells (OPV) with polymers in the active layers are more prominent concerning power conversion efficiency associated with durability, resulting in great research interest. Furthermore, polymer solar cells are easier to process and can be manufactured on a large scale achieving high efficiencies and stability. This review aims to raise the state of the art about these solar cells, discourse their architectures, current developments on polymer structures, and most relevant challenges for OPV devices, as a search for increased efficiency and stability.
Citation: Taihana Paula, Maria de Fatima Marques. Recent advances in polymer structures for organic solar cells: A review[J]. AIMS Energy, 2022, 10(1): 149-176. doi: 10.3934/energy.2022009
[1] | Lamya Almaghamsi, Aeshah Alghamdi, Abdeljabbar Ghanmi . Existence of solution for a Langevin equation involving the ψ-Hilfer fractional derivative: A variational approach. AIMS Mathematics, 2025, 10(1): 534-550. doi: 10.3934/math.2025024 |
[2] | Arjumand Seemab, Mujeeb ur Rehman, Jehad Alzabut, Yassine Adjabi, Mohammed S. Abdo . Langevin equation with nonlocal boundary conditions involving a ψ-Caputo fractional operators of different orders. AIMS Mathematics, 2021, 6(7): 6749-6780. doi: 10.3934/math.2021397 |
[3] | Sotiris K. Ntouyas, Bashir Ahmad, Jessada Tariboon . Nonlocal integro-multistrip-multipoint boundary value problems for ¯ψ∗-Hilfer proportional fractional differential equations and inclusions. AIMS Mathematics, 2023, 8(6): 14086-14110. doi: 10.3934/math.2023720 |
[4] | Karim Guida, Lahcen Ibnelazyz, Khalid Hilal, Said Melliani . Existence and uniqueness results for sequential ψ-Hilfer fractional pantograph differential equations with mixed nonlocal boundary conditions. AIMS Mathematics, 2021, 6(8): 8239-8255. doi: 10.3934/math.2021477 |
[5] | Sunisa Theswan, Sotiris K. Ntouyas, Jessada Tariboon . Coupled systems of ψ-Hilfer generalized proportional fractional nonlocal mixed boundary value problems. AIMS Mathematics, 2023, 8(9): 22009-22036. doi: 10.3934/math.20231122 |
[6] | Thabet Abdeljawad, Pshtiwan Othman Mohammed, Hari Mohan Srivastava, Eman Al-Sarairah, Artion Kashuri, Kamsing Nonlaopon . Some novel existence and uniqueness results for the Hilfer fractional integro-differential equations with non-instantaneous impulsive multi-point boundary conditions and their application. AIMS Mathematics, 2023, 8(2): 3469-3483. doi: 10.3934/math.2023177 |
[7] | Zohreh Heydarpour, Maryam Naderi Parizi, Rahimeh Ghorbnian, Mehran Ghaderi, Shahram Rezapour, Amir Mosavi . A study on a special case of the Sturm-Liouville equation using the Mittag-Leffler function and a new type of contraction. AIMS Mathematics, 2022, 7(10): 18253-18279. doi: 10.3934/math.20221004 |
[8] | Weerawat Sudsutad, Chatthai Thaiprayoon, Sotiris K. Ntouyas . Existence and stability results for ψ-Hilfer fractional integro-differential equation with mixed nonlocal boundary conditions. AIMS Mathematics, 2021, 6(4): 4119-4141. doi: 10.3934/math.2021244 |
[9] | Zheng Kou, Saeed Kosari . On a generalization of fractional Langevin equation with boundary conditions. AIMS Mathematics, 2022, 7(1): 1333-1345. doi: 10.3934/math.2022079 |
[10] | Muath Awadalla, Manigandan Murugesan, Subramanian Muthaiah, Bundit Unyong, Ria H Egami . Existence results for a system of sequential differential equations with varying fractional orders via Hilfer-Hadamard sense. AIMS Mathematics, 2024, 9(4): 9926-9950. doi: 10.3934/math.2024486 |
High energy dependence on fossil fuels and an increase in greenhouse gas emissions are factors that highlight the need for alternative energy sources. Photovoltaic technology is a strong candidate that uses the most abundant resource, solar energy, but what makes its wide use difficult is the high cost of the commercially available devices. Thus, research has been devoted to developing new low-cost photovoltaic systems that are easier to manufacture with high efficiency and durability, such as the third-generation solar cells. According to this study, organic solar cells (OPV) with polymers in the active layers are more prominent concerning power conversion efficiency associated with durability, resulting in great research interest. Furthermore, polymer solar cells are easier to process and can be manufactured on a large scale achieving high efficiencies and stability. This review aims to raise the state of the art about these solar cells, discourse their architectures, current developments on polymer structures, and most relevant challenges for OPV devices, as a search for increased efficiency and stability.
There are many life-threatening diseases, out of which Malaria is one of them that is produced through the parasite known as Plasmodium that transmits to humans with the infected anopheles mosquito bite [1,2,3]. People with malaria are infected by the Protozoan parasite, which grows within the cells of red blood and spreads the illness. There are four Plasmodia species that can become the reason of infection, known as oval, falciparum, malaria, and vivax, while the two other most widespread Plasmodium species are falciparum and vivax [4]. Malaria affects lots of people each year, mainly in South Asia, Africa, and South America. It becomes the major reason of death and illness in various developing regions, specifically young children, and pregnant women. The main symptoms of malaria are fever, muscle pain, and headache. Malaria can progress promptly, possibly severe anemia, lung problems, and even cause death. There are various effective tactics that proposed the World Health Organization (WHO) to treat and prevent this dangerous disease. Some of them are indoor spraying, pesticide-treated bed nets, and some antimalarial medications. The vector control systems can be applied to lessen the poisoned mosquito population. In general, malaria is a substantial global health task, which needs a comprehensive approach for its cure by using the strategies of vector control and early identification for its treatment.
Malaria is a dynamic and complicated disease, which is considered difficult to present its model mathematically. The malaria parasite's life cycle is complex due to numerous phases of development both in mosquito vector and human host. These points are prompted by numerous features, e.g., mosquito actions, host immunity, and environmental environments that is used to make difficult the disease dynamics to predict and understand. The intricacy of the virus's spread dynamics is one of the main problems with malaria modelling. Malaria spread is influenced by numerous interrelated influences, e.g., the mosquito vector density, the host immunity degree, and the accessibility of efficient mediations. The relations between these aspects indicate the nonlinearity and hard to capture using the mathematical systems [5]. However, the mathematical malaria disease model based on the pesticides and medication (MDMPM) is presented, which is categorized in four human (susceptible, exposed, infected, recovered) and two vector (susceptible, infected) classes. The pathogen infects the population of host then the individuals are categorized into groups based on the parasites number and virus types. Kermack-McKendrick [6] proposed the mathematical SEIRSI model based on the population of human/vector groups. The nonlinear dynamics of the SEIRSI model is presented as [7]:
S′h(x)=αh+β1Rh(x)−γ1ψIh(x)Sh(x)−γ2ξIm(x)Sh(x)−(δ1+ηh)Sh(x),E′h(x)=γ2ξIm(x)Sh(x)+γ1ψIh(x)Sh(x)−(β2+δ2+ηh)Eh(x),I′h(x)=β2Eh(x)−(ϵ+pυ+ηh)Ih(x),R′h(x)=δ1Sh(x)+υpIh(x)+δ2Eh(x)−(β1+ηh)Rh(x),S′m(x)=αm−(σ+ηm)Sm(x)−γ3eSm(x)Ih(x),I′m(x)=γ3eIh(x)Sm(x)−(σ+ηm)Im(x). | (1) |
The compartmental illustration of the SEIRSI model based on the MDMPM is shown in Figure 1, while the description of each parameter is given in Table 1.
Parameters | Details |
β1 | Susceptible form of recovered individuals |
αm | Newborn mosquitoes transfer rate into Sm |
αh | Newborns ratio to enter in Sh |
γ1 | Transfer ratio of illness from one affected to susceptible people |
ψ | Transfusions rate of average blood with stable time in Ih and Sh |
β2 | Exposed to infected infection rate in people |
ξ | Infected mosquito susceptible bites using fixed time |
γ3 | Rate of Mosquitos infection |
γ2 | Disease transfer through infected (mosquito) to susceptible (individual) |
ηh | People natural rate of death |
δ2 | Exposed recovered population |
δ1 | Susceptible recovered population |
p | Rate of humans heal |
ε | Infected human death due to disease |
ηm | Mosquitos' natural death |
υ | Anti-malarial prescriptions |
e | Bites Sm using the infected humans |
σ | Expires sections using spraying for Sm and Im |
x | Time |
There are various deterministic and conventional numerical schemes that have been presented to solve the biological models, including the wavelet Bernstein scheme that has been presented to solve the epidemic SIR fractional kind of infectious disease model [8], Laplace method is applied for the fractional epidemic system of born vector disease [9], q-homotopy transform technique is proposed to solve the fractional Schistosomiasis disease model [10,11], a theoretic investigation is presented based on the impacts of vector-virus transmission mechanism [12], a collocation technique with shifted Chebyshev polynomials is applied to solve the fractional order diseased model of Rubella ailment [13], an analytical computational approach is provided for the inflammatory atherosclerosis disease system [14], the differential stomach and coronavirus models have been discussed numerically by applying the Adam method [15,16] and the design of a numerical method is presented for the maturing communication in population and epidemic models [17]. Some more deterministic procedures that have been applied to solve different dynamical models are presented in [18,19,20,21,22].
All mentioned schemes have their individual strength, and demerits, however the stochastic solvers have never been tested before for the nonlinear dynamics of the MDMPM. The current study is related to the numerical solutions of the MDMPM through the stochastic procedures using the scale conjugate gradient neural networks (SCJGNNs). In recent years, the stochastic computing paradigms have been proposed in various applications, e.g., eye surgery differential systems [23,24], food chain systems [25,26], smoking dynamical systems [27], models arising in biological applications, [28,29], thermal explosion singular systems [30,31], and many more [32,33,34,35,36,37,38,39,40,41,42,43]. Based on these noteworthy applications of neuro-computing paradigm, the authors are motivated to present the numerical solutions of the malaria disease model of pesticides and medication (MDMPM) with stochastic framework of scale conjugate gradient neural networks (SCJGNNs). The purpose of this work is to provide a stochastic framework based on the SCJGNNs for solving the mathematical model representing MDMPM dynamics.
The contributions, insights and innovative aspects of the current scheme is portrayed in terms of salient features as follows:
• A novel design of the stochastic framework via SCJGNNs is introduced/presented to get the numerical results/outcomes of the nonlinear dynamics of governing mathematical model of MDMPM.
• The capability and knacks of Runge-Kutta solver is exploited/developed to formulate the target datasets for different scenario of MDMPM system that are used for the testing, and training procedure of the networks via SCJGNNs.
• The exactness of the stochastic SCJGNNs is attained/verified/validated through the overlapping of the results with standard counterparts having very small magnitude of absolute error (AE).
• The reliable matching of the numerical results, a strong arrangement using the benchmark outputs along with consistent statistical performance indices further prove the importance/significance/role of the SCJGNNs to solve the variant of MDMPM models.
The remaining paper is presented as: Proposed SCJGNNs design is shown in Section 2, Sect 3 presents the numerical results, and the conclusions are discussed in the final section.
The current section is used to design the computing SCJGNNs structure to solve the MDMPM in two steps, including the detail of designed SCJGNNs performances along with its execution. The designed SCJGNNs is presented in Figure 2. In the 1st phase of Figure 2, the nonlinear mathematical system is illustrated, the stochastic methodology using the SCJGNNs is provided in the 2nd phase of Figure 2, while some results are presented in the last phase of Figure 2. The performances using the multi-layers network based on the structure of single neuron is illustrated in Figure 3.
The computing SCJGNNs approach for solving the MDMPM is executed using the command 'nftool' in software 'MATLAB', n-folded cross authentication, layers (output/hidden/input) structure, epochs are taken maximum 1000, fifteen hidden neurons, transfer function log-sigmoid, stopping standards, SCJG algorithm, tolerances, and step size. The label statics and input/target training are achieved using the basic outputs by taking the statics of validation (12%), testing (10%), and training (78%) for the MDMPM.
The designed computing SCJGNNs for the solutions of the MDMPM is performed by using 15 neurons, which have been validated through the best values of underfitting and overfitting based on the authorization and training at epochs 100, 86 and 69. For small neurons, the premature convergence (under fitting) problem is faced, while similar precision by using higher intricacy (overfitting issue) is noticed by taking higher neurons. The trials have been taken for the validation (12%), testing (10%), and training (78%) to solve the MDMPM. By taking a smaller data training, like 40% to 60%, the MSE values for testing 30% to 40% increase and generally poor presentations have been observed. On the other hand, if the training statics is almost designated > 90% and 5% for both testing and certification, the procedure's correctness improves marginally by choosing the label static, which presents the bias and targets input, but stochastic procedure's strength is not severely established for unbiased statics exclusive based targets information. The proposed values are performed on the input [0, 1] by applying the stochastic SCJGNNs procedure for the numerical performances of the MDMPM. The neuron structure using the input, output and hidden layers is shown in Figure 4.
The numerical solution for three different cases of the MDMPM by applying the computing procedures based on the SCJGNNs is presented in this section.
Case 1: Consider the values ψ=0.038, αh=0.027, γ1=0.020, ξ=0.13, β1=1730, γ2=0.010, δ1=0.001, ηh=0.0004, δ2=0.001, p=0.611, β2=0.001, ε=0.05, υ=0.01, e=0.022, αm=0.13, γ3=0.072, Sh(0)=0.01, Eh(0)=0.02, Ih(0)=0.03, Rh(0)=0.04, Sm(0)=0.05, and Im(0)=0.06 in system (1), which is shown as:
{S′h(x)=0.027+0.0014Rh(x)−(0.00076Ih(x)+0.0014+0.0013Im(x))Sh(x),(Sh)0=0.01,E′h(x)=(0.00076Ih(x)+0.0013Im(x))Sh(x)−0.0024Eh(x),(Eh)0=0.02,I′h(x)=−0.00752Ih(x)+0.001Eh(x),(Ih)0=0.03,R′h(x)=0.00611Ih(x)−0.00177Rh(x)+0.001Eh(x)+0.001Sh(x),(Rh)0=0.04,S′m(x)=0.13−0.05Sm(x)−0.001584Ih(x)Sm(x),(Sm)0=0.05,I′m(x)=0.001584Sm(x)Ih(x)−0.05Im(x),(Im)0=0.06. | (2) |
Case 2: Consider the parameter values ψ=0.038, αh=0.027, γ1=0.020, ξ=0.13, β1=1730, γ2=0.010, δ1=0.001, ηh=0.0004, δ2=0.001, p=0.611, β2=0.001, ε=0.05, υ=0.01, e=0.022, αm=0.13, γ3=0.072, Sh(0)=0.02, Eh(0)=0.03, Ih(0)=0.04, Rh(0)=0.05, Sm(0)=0.06, and Im(0)=0.07 in system (1) that is given as:
{S′h(x)=0.027+0.0014Rh(x)−(0.00076Ih(x)+0.0014+0.0013Im(x))Sh(x),(Sh)0=0.02,E′h(x)=(0.00076Ih(x)+0.0013Im(x))Sh(x)−0.0024Eh(x),(Eh)0=0.03,I′h(x)=−0.00752Ih(x)+0.001Eh(x),(Ih)0=0.04,R′h(x)=0.00611Ih(x)−0.00177Rh(x)+0.001Eh(x)+0.001Sh(x),(Rh)0=0.05,S′m(x)=0.13−0.05Sm(x)−0.001584Ih(x)Sm(x),(Sm)0=0.06,I′m(x)=0.001584Sm(x)Ih(x)−0.05Im(x),(Im)0=0.07. | (3) |
Case 3: Consider ψ=0.038, αh=0.027, γ1=0.020, ξ=0.13, β1=1730, γ2=0.010, δ1=0.001, ηh=0.0004, δ2=0.001, p=0.611, β2=0.001, ε=0.05, υ=0.01, e=0.022, αm=0.13, γ3=0.072, Sh(0)=0.03, Eh(0)=0.04, Ih(0)=0.05, Rh(0)=0.06, Sm(0)=0.07, and Im(0)=0.08 in system (1), given as:
{S′h(x)=0.027+0.0014Rh(x)−(0.00076Ih(x)+0.0014+0.0013Im(x))Sh(x),(Sh)0=0.03,E′h(x)=(0.00076Ih(x)+0.0013Im(x))Sh(x)−0.0024Eh(x),(Eh)0=0.04,I′h(x)=−0.00752Ih(x)+0.001Eh(x),(Ih)0=0.05,R′h(x)=0.00611Ih(x)−0.00177Rh(x)+0.001Eh(x)+0.001Sh(x),(Rh)0=0.06,S′m(x)=0.13−0.05Sm(x)−0.001584Ih(x)Sm(x),(Sm)0=0.07,I′m(x)=0.001584Sm(x)Ih(x)−0.05Im(x),(Im)0=0.08. | (4) |
The plots of the estimated results based on the numerical SCJGNNs procedure for solving the MDMPM are shown in Figures 5 to 9. Figure 5 presents the best validation measures and state transitions for the nonlinear dynamics of the MDMPM through the stochastic computing SCJGNNs scheme. The values of the mean square error (MSE) and the state of transition values are depicted in Figure 5. The computed performances of MSE using the best curves plots, certification, and training are provided in Figure 5(a-c) and the SoT measures are portrayed in Figure 5(d-f). The best solution performances of the MDMPM are shown at epochs 100, 86 and 69, which are found around 1.69774 ×10−07, 1.4324 × 10−07 and 3.5876 × 10−07. It indicates that convergence through the MSE is observed based on dataset of training, testing and certification. It is also observed that by updating the epochs, the authentications, training, and testing graphs lead to steady-state position up to 10-07. The gradient curves by applying the process of SCJGNNs to solve the MDMPM are calculated as 1.4161×10-06, 8.1804 × 10−07 and 2.9486 × 10−06. These graphic curves indicate the precision, exactness, and convergence by using the SCJGNNs procedure for the MDMPM. An error gradient presents the direction and magnitude that is calculated by the designed neural network for updating the weights in the proper amount/direction. In neural network fitting, the process of backpropagation is used to compute the loss function gradient. Mu is the used for the network's training for the momentum parameter, which includes the updated weight expressions to avoid the local minimum issues. It is observed that the network can stuck sometimes to the local minimum, which does not perform the convergence. In addition, Mu shows the control parameter based on that scheme that is used in the neural network's training.
Figure 6(a to c) presents the curves of fitting for the nonlinear MDMPM, which certifies the comparison of the results. Figure 6(d-f) signifies the error histogram (EH) values for the MDMPM by applying the computing SCJGNNs. The construction of EH is used to test the errors of the targeted and predicted values after the designed artificial neural network training. These errors present that how predicted measures contrast from the targeted values. These values based on the EH are obtained as 1.95 × 10−05, 8.64 × 10−05 and −5.90 × 10−06 for respective dynamics of the MDMPM. Figures 7 to 9 present the correlation values for the MDMPM using the SCJGNNs. These measures based on the determination coefficient R2 are found as 1 for each case of the MDMPM. The graphs of substantiation, testing, and training verify the correctness of the MDMPM using the numerical stochastic SCJGNNs procedure. The values of MSE convergence using the train, epochs, certification, backpropagation, test, and intricacy are tabulated in Table 2. The complexity measures using the SCJGNNs for the MDMPM are performed to authenticate the intricacy based on the network's training, i.e., epoch execution.
Case | MSE for instances of sampling | Gradient | Performance | Epoch | Time | ||
Test | validation | Train | |||||
I | 2.745×10−07 | 1.697 ×10−07 | 2.366×10−07 | 1.42×10−06 | 2.26×10−07 | 100 | 2 Sec |
II | 1.795×10−07 | 1.432×10−07 | 1.920×10−07 | 8.18×10−07 | 1.91×10−07 | 86 | 2 Sec |
III | 5.112×10−07 | 3.038×10−07 | 3.678×10−07 | 2.94×10−06 | 2.09×10−07 | 69 | 2 Sec |
Figures 10 and 11 present the comparison graphs and AE using the obtained and source statics for the MDMPM. It is demonstrated that the source and calculated outcomes of the MDMPM precisely coincided. Figure 10 represents the AE graphs for each category of the MDMPM. For the first class, the AE lies as 10−03 to 10−05 for case I and III and 10−02 to 10−04 for case II. These values for the 2nd category of the MDMPM are found around 10−05 to 10−06, 10−04 to 10−06 and 10−03 to 10−05 for cases I, II and III. The AE measures for the 3rd dynamics of the MDMPM lie as 10−04 to 10−07, 10−03 to 10−05 and 10−03 to 10−06 for respective cases. These values for the 4th class of the MDMPM are calculated as 10−03 to 10−05 for case I and for case II and III, these AE are found as 10−03 to 10−04. The AE values for the 5th and 6th dynamics are found as 10−03 to 10−05 for each case of the model. These reduceable AE measures provides the accuracy of the scheme for the MDMPM.
The stochastic numerical performances of the malaria disease using the pesticides and medication have been presented in this study. The populations-based host and vector are divided in the mathematical classification of the malaria using the pesticides and medication. Some conclusions of this work are reported as:
• The stochastic scale conjugate gradient neural network framework has been designed successfully for the numerical malaria disease model.
• The neural network procedure is presented by using fifteen neurons along with the log-sigmoid transfer function in the hidden layers to solve the model.
• The supervised neural networks process using the data as for validation 12%, testing 10%, and training 78% is presented for the numerical solutions of the system.
• The accuracy of the proposed stochastic scheme is examined through the overlapping of the achieved obtained and Runge-Kutta source solutions.
• The negligible performances of AE indicate the exactness of the computing stochastic approach.
• The consistency and trustworthiness of the designed procedure are assessed through the correlation, histogram curves, regression, and functional fitness process to solve the MDMPM.
• The perks/advantages of the proposed stochastic SCJGNNs procedure includes simplicity of the concept and understanding, straightforwardness in implementations and execution, provision of outcome on continuous domain, expandability and applicability.
The limitation/disadvantage of the stochastic SCJGNNs paradigm is the issue of trapping in local minima for scale conjugate gradient methodology while training of the weights of the networks, however, the said limitation is rectified by exploited heuristic global search methodologies in future by the interested readers. Additionally, in future, the designed SCJGNNs procedure can be explored/implemented for different applications of the nonlinear mathematical models arising in the field of computational fluid mechanics.
The authors declare that they have no conflict of interest
Manal Alqhtani: Conceptualization, methodology, validation, Writing-original draft preparation, writing-review; J.F. Gómez-Aguilar: Conceptualization, methodology, validation, investigation, Writing-original draft preparation, writing-review; Khaled M. Saad: Conceptualization, methodology, validation, writing-review and editing; Zulqurnain Sabir: Validation, investigation, Writing-original draft preparation, writing-review; Eduardo Pérez-Careta: Validation, formal analysis, investigation. All authors have read and agreed to the published version of the manuscript.
The authors are thankful to the Deanship of Scientific Research at Najran University for funding this work under the General Research Funding program grant code (NU/RG/SERC/12/22).
The authors declare they have not used Artificial Intelligence (AI) tools in the creation of this article.
[1] |
Ahmad KS, Naqvi SN, Jaffri SB (2021) Systematic review elucidating the generations and classifications of solar cells contributing towards environmental sustainability integration. Rev Inorg Chem 41: 21-39. https://doi.org/10.1515/revic-2020-0009 doi: 10.1515/revic-2020-0009
![]() |
[2] |
Sathiyan G, Siva G, Sivakumar EKT, et al. (2018) Synthesis and studies of carbazole-based donor polymer for organic solar cell applications. Colloid Polym Sci 296: 1193-1203. https://doi.org/10.1007/s00396-018-4337-4 doi: 10.1007/s00396-018-4337-4
![]() |
[3] |
Cui Y, Yao H, Gao B, et al. (2017) Fine-Tuned photoactive and interconnection layers for achieving over 13% efficiency in a fullerene-free tandem organic solar cell. J Am Chem Soc 139: 7302-7309. https://doi.org/10.1021/jacs.7b01493 doi: 10.1021/jacs.7b01493
![]() |
[4] |
Mutlu A, Can M, Tozlu C (2019) Performance improvement of organic solar cell via incorporation of donor type self-assembled interfacial monolayer. Thin Solid Films 685: 88-96. https://doi.org/10.1016/j.tsf.2019.05.064 doi: 10.1016/j.tsf.2019.05.064
![]() |
[5] |
Freudenberg J, Jänsch D, Hinkel F, et al. (2018) Immobilization strategies for organic semiconducting conjugated polymers. Chem Rev 118: 5598-5689. https://doi.org/10.1021/acs.chemrev.8b00063 doi: 10.1021/acs.chemrev.8b00063
![]() |
[6] |
Ragoussia M-E, Torres T (2015) New generation solar cells: Concepts, trends and perspectives. Chem Commun 51: 3957-3972. https://doi.org/10.1039/C4CC09888A doi: 10.1039/C4CC09888A
![]() |
[7] |
Majumder C, Rai A, Bose C (2018) Performance optimization of bulk heterojunction organic solar cell. Optik 157: 924-929. https://doi.org/10.1016/j.ijleo.2017.11.114 doi: 10.1016/j.ijleo.2017.11.114
![]() |
[8] |
Chen S, Yang S, Sun H, et al. (2017) Enhanced interfacial electron transfer of inverted perovskite solar cells by introduction of CoSe into the electron-transporting-layer. J Power Sources 353: 123-130. https://doi.org/10.1016/j.jpowsour.2017.03.144 doi: 10.1016/j.jpowsour.2017.03.144
![]() |
[9] |
Mabindisa R, Tambwe K, Mciteka L, et al. (2021) Organic nanostructured materials for sustainable application in next generation solar cells. Appl Sci 11: 11324. https://doi.org/10.3390/app112311324 doi: 10.3390/app112311324
![]() |
[10] |
Oh Kwon K, Uddin MA, Park J-H, et al. (2016) A high efficiency nonfullerene organic solar cell with optimized crystalline organizations. Adv Mater 28: 910-916. https://doi.org/10.1002/adma.201504091 doi: 10.1002/adma.201504091
![]() |
[11] |
Li Z, Zhu C, Yuan J, et al. (2022) Optimizing side chains on different nitrogen aromatic rings achieving 17% efficiency for organic photovoltaics. J Energy Chem 65: 173-178. https://doi.org/10.1016/j.jechem.2021.05.041 doi: 10.1016/j.jechem.2021.05.041
![]() |
[12] |
Wibowo FTA, Krishna NV, Sinaga S, et al. (2021) High-efficiency organic solar cells prepared using a halogen-free solution process. Cell Rep Phys Sci 2: 100517. https://doi.org/10.1016/j.xcrp.2021.100517 doi: 10.1016/j.xcrp.2021.100517
![]() |
[13] |
Bi P, Zhang S, Chen Z, et al. (2021) Reduced non-radiative charge recombination enables organic photovoltaic cell approaching 19% efficiency. Joule 5: 2408-2419. https://doi.org/10.1016/j.joule.2021.06.020 doi: 10.1016/j.joule.2021.06.020
![]() |
[14] |
Distler A, Brabec CJ, Egelhaaf H-J (2021) Organic photovoltaic modules with new world record efficiencies. Prog Photovoltaics: Res Appl 29: 24-31. https://doi.org/10.1002/pip.3336 doi: 10.1002/pip.3336
![]() |
[15] |
Ma L, Zhang S, Wang J, et al. (2020) Recent advances in non-fullerene organic solar cells: from lab to fab. Chem Commun 56: 14337. https://doi.org/10.1039/D0CC05528J doi: 10.1039/D0CC05528J
![]() |
[16] |
Yao C, Zhao J, Zhu Y, et al. (2020) Trifluoromethyl Group-Modified Non-Fullerene acceptor toward improved power conversion efficiency over 13% in polymer solar cells. ACS Appl Mater Interfaces 12: 11543-11550. https://doi.org/10.1021/acsami.9b20544 doi: 10.1021/acsami.9b20544
![]() |
[17] |
Yang Y (2021) The original design principles of the Y-Series nonfullerene acceptors, from Y1 to Y6. ACS Nano 15: 18679-18682. https://doi.org/10.1021/acsnano.1c10365 doi: 10.1021/acsnano.1c10365
![]() |
[18] |
Wagenpfahl A (2017) Mobility dependent recombination models for organic solar cells. J Phys: Condens Matter 29: 373001. https://doi.org/10.1088/1361-648X/aa7952 doi: 10.1088/1361-648X/aa7952
![]() |
[19] |
Xu B, Zheng Z, Zhao K, et al. (2016) A bifunctional interlayer material for modifying both the anode and cathode in highly efficient polymer solar cells. Adv Mater 28: 434-439. https://doi.org/10.1002/adma.201502989 doi: 10.1002/adma.201502989
![]() |
[20] |
Zheng Z, Hu Q, Zhang S, et al. (2018) A highly efficient non-fullerene organic solar cell with a fill factor over 0.80 enabled by a fine-tuned hole-transporting layer. Adv Mater 30: 1-9. https://doi.org/10.1002/adma.201801801 doi: 10.1002/adma.201801801
![]() |
[21] |
Doat O, Barboza BH, Batagin-Neto A, et al. (2021) Review: materials and modeling for organic photovoltaic devices. Polym Int 71: 6-25. https://doi.org/10.1002/pi.6280 doi: 10.1002/pi.6280
![]() |
[22] |
Zhao Y, Zhu Y, Cheng H-W, et al. (2021) A review on semitransparent solar cells for agricultural application. Mater Today Energy 22: 100852. https://doi.org/10.1016/j.mtener.2021.100852 doi: 10.1016/j.mtener.2021.100852
![]() |
[23] |
Bi P, Zhang S, Chen Z, et al. (2021) Reduced non-radiative charge recombination enables organic photovoltaic cell approaching 19% efficiency. Joule 5: 2408-2419. https://doi.org/10.1016/j.joule.2021.06.020 doi: 10.1016/j.joule.2021.06.020
![]() |
[24] |
Zuo L, Shi X, Jo SB, et al. (2018) Tackling energy loss for high‐efficiency organic solar cells with integrated multiple strategies. Adv Mater 30: 1706816. https://doi.org/10.1002/adma.201706816 doi: 10.1002/adma.201706816
![]() |
[25] |
Wilken S, Scheunemann D, Dahlström S, et al. (2021) How to reduce charge recombination in organic solar cells: There are still lessons to learn from P3HT:PCBM. Adv Electron Mater 7: 2001056. https://doi.org/10.1002/aelm.202001056 doi: 10.1002/aelm.202001056
![]() |
[26] |
Nakano K, Terado K, Kaji Y, et al. (2021) Reduction of electric current loss by Aggregation-Induced molecular alignment of a Non-Fullerene acceptor in organic photovoltaics. ACS Appl Mater Interfaces 13: 60299-60305. https://doi.org/10.1021/acsami.1c19275 doi: 10.1021/acsami.1c19275
![]() |
[27] |
Pugliese SN, Gallaher JK, Uddin MA, et al. (2022) Spectroscopic comparison of charge dynamics in fullerene and non-fullerene acceptor-based organic photovoltaic cells. J Mater Chem C. https://doi.org/10.1039/D1TC04800G doi: 10.1039/D1TC04800G
![]() |
[28] |
Zhao F, Zhang H, Zhang R, et al. (2020) Emerging approaches in enhancing the efficiency and stability in Non‐Fullerene organic solar cells. Adv Energy Mater 10: 2002746. https://doi.org/10.1002/aenm.202002746 doi: 10.1002/aenm.202002746
![]() |
[29] |
Zhu L, Zhang M, Zhong W, et al. (2021) Progress and prospects of the morphology of non-fullerene acceptor based high-efficiency organic solar cells. Energy Environ Sci 1: 4341-4357. https://doi.org/10.1039/D1EE01220G doi: 10.1039/D1EE01220G
![]() |
[30] |
Lin Y, Zhao F, Wu Y, et al. (2016) Mapping polymer donors toward high-efficiency fullerene free organic solar cells. Adv Mater 29: 1604155. https://doi.org/10.1002/adma.201604155 doi: 10.1002/adma.201604155
![]() |
[31] |
Chung HY, Park J-H, Cui J, et al. (2021) Influence of intramolecular charge-transfer characteristics of excitons on polaron generation at the Donor/Acceptor interface in polymer solar cells. J Phys Chem C 125: 18352-18361. https://doi.org/10.1021/acs.jpcc.1c05524 doi: 10.1021/acs.jpcc.1c05524
![]() |
[32] |
Etxebarria I, Ajuria J, Pacios R (2015) Solution-processable polymeric solar cells: A review on materials, strategies and cell architectures to overcome 10%. Org Electron: Phys, Mater, Appl 19: 34-60. https://doi.org/10.1016/j.orgel.2015.01.014 doi: 10.1016/j.orgel.2015.01.014
![]() |
[33] |
Zuo L, Yu J, Shi X, et al. (2017) High-Efficiency nonfullerene organic solar cells with a parallel tandem configuration. Adv Mater 29: 1702547. https://doi.org/10.1002/adma.201702547 doi: 10.1002/adma.201702547
![]() |
[34] |
Zheng NN, Wang ZF, Zhang K, et al. (2019) High-performance inverted polymer solar cells without an electron extraction layer via a one-step coating of cathode buffer and active layer. J Mater Chem A 7: 1429-1434. https://doi.org/10.1039/c8ta09763a doi: 10.1039/c8ta09763a
![]() |
[35] |
Pandey R, Lim JW, Kim JH, et al. (2018) Performance enhancement in organic photovoltaic solar cells using iridium (Ir) ultra-thin surface modifier (USM). Appl Surf Sci 444: 97-104. https://doi.org/10.1016/j.apsusc.2018.03.012 doi: 10.1016/j.apsusc.2018.03.012
![]() |
[36] |
Sun C, Pan F, Bin H, et al. (2018) A low cost and high performance polymer donor material for polymer solar cells. Nature Commun 9: 1-10. https://doi.org/10.1038/s41467-018-03207-x doi: 10.1038/s41467-018-03207-x
![]() |
[37] |
Shen W, Xiao M, Tang J, et al. (2015) Effective regulation of the micro-structure of thick P3HT: PC 71 BM film by the incorporation of ethyl benzenecarboxylate in toluene solution. RSC Adv 5: 47451-47457. https://doi.org/10.1039/C5RA06957B doi: 10.1039/C5RA06957B
![]() |
[38] |
Wang D, Wright M, Elumalai NK, et al. (2016) Stability of perovskite solar cells. Sol Energy Mater Sol Cells 147: 255-275. https://doi.org/10.1016/j.solmat.2015.12.025 doi: 10.1016/j.solmat.2015.12.025
![]() |
[39] |
Salem AMS, El-Sheikh SM, Harraz FA, et al. (2017) Inverted polymer solar cell based on MEH-PPV/PC 61 BM coupled with ZnO nanoparticles as electron transport layer. Appl Surf Sci 425: 156-163. https://doi.org/10.1016/j.apsusc.2017.06.322 doi: 10.1016/j.apsusc.2017.06.322
![]() |
[40] |
Ranganathan K, Wamwangi D, Coville NJ (2015) Plasmonic Ag nanoparticle interlayers for organic photovoltaic cells: An investigation of dielectric properties and light trapping. Sol Energy 118: 256-266. https://doi.org/10.1016/j.solener.2015.05.022 doi: 10.1016/j.solener.2015.05.022
![]() |
[41] |
Meyer J, Hamwi S, Kröger M, et al. (2012) Transition metal oxides for organic electronics: Energetics, device physics and applications. Adv Mater 24: 5408-5427. https://doi.org/10.1002/adma.201201630 doi: 10.1002/adma.201201630
![]() |
[42] | Nuramdhani I, Jose M, Samyn P, et al. (2019). Charge-Discharge characteristics of textile energy storage devices having different PEDOT: PSS ratios and conductive yarns configuration. Polymers 11: 345. https://doi.org/10.3390/polym11020345 |
[43] |
Mota IC, Santos BPS, Santos REPD, et al. (2021) Influence of reaction time on properties of regioregular poly(3-hexylthiophene) by the Grignard metathesis polymerization. J Therm Anal Calorim 2021: 1-26. https://doi.org/10.1007/s10973-021-10890-4 doi: 10.1007/s10973-021-10890-4
![]() |
[44] |
Ghosekar IC, Patil GC (2021) Review on performance analysis of P3HT:PCBM based bulk heterojunction organic solar cells. Semicond Sci Technol 36: 045005. https://doi.org/10.1088/1361-6641/abe21b doi: 10.1088/1361-6641/abe21b
![]() |
[45] |
Chen K-W, Lin L-Y, Li Y-H, et al. (2018) Fluorination effects of A-D-A-type small molecules on physical property and the performance of organic solar cell. Org Electron: Phy, Mater, Appl 52: 342-349. https://doi.org/10.1016/j.orgel.2017.11.021 doi: 10.1016/j.orgel.2017.11.021
![]() |
[46] |
Sathiyan G, Thangamuthu R, Sakthivel P (2016) Synthesis of carbazole-based copolymers containing carbazole-thiazolo[5, 4-:D] thiazole groups with different dopants and their fluorescence and electrical conductivity applications. RSC Adv 6: 69196-69205. https://doi.org/10.1039/C6RA08888K doi: 10.1039/C6RA08888K
![]() |
[47] |
Wang C, Liu F, Chen QM, et al. (2021) Benzothiadiazole-based conjugated polymers for organic solar cells. Chin J Polym Sci 39: 525-536. https://doi.org/10.1007/s10118-021-2537-8 doi: 10.1007/s10118-021-2537-8
![]() |
[48] |
Zhong W, Xiao J, Sun S, et al. (2016) Wide bandgap dithienobenzodithiophene-based π-conjugated polymers consisting of fluorinated benzotriazole and benzothiadiazole for polymer solar cells. J Mater Chem C 4: 4719-4727. https://doi.org/10.1039/C6TC00271D doi: 10.1039/C6TC00271D
![]() |
[49] |
Zhao Q, Qu J, He F (2020) Chlorination: An effective strategy for high-performance organic solar cells. Adv Sci 7: 2000509. https://doi.org/10.1002/advs.202000509 doi: 10.1002/advs.202000509
![]() |
[50] |
Chen W, Wu Y, Yue Y, et al. (2015) Efficient and stable large-area perovskite solar cells with inorganic charge extraction layers. Science 350: 944-948. https://doi.org/10.1126/science.aad1015 doi: 10.1126/science.aad1015
![]() |
[51] |
Li M, Gao K, Wan X, et al. (2017) Solution-processed organic tandem solar cells with power conversion efficiencies > 12%. Nature Photonics 11: 85-90. https://doi.org/10.1038/nphoton.2016.240 doi: 10.1038/nphoton.2016.240
![]() |
[52] |
Jo JW, Jung JW, Jung EH, et al. (2015) Fluorination on both D and A units in D-A type conjugated copolymers based on difluorobithiophene and benzothiadiazole for highly efficient polymer solar cells. Energy Environ Sci 8: 2427-2434. https://doi.org/10.1039/C5EE00855G doi: 10.1039/C5EE00855G
![]() |
[53] |
Chao P, Johner N, Zhong Xi, et al. (2019) Chlorination strategy on polymer donors toward efficient solar conversions. J Energy Chem 39: 208-216. https://doi.org/10.1016/j.jechem.2019.04.002 doi: 10.1016/j.jechem.2019.04.002
![]() |
[54] |
Zhou J, Zhang B, Geng Y, et al. (2021) Gradual chlorination at different positions of D-π-A copolymers based on benzodithiophene and isoindigo for organic solar cells. Mater Rep: Energy 1: 100065. https://doi.org/10.1016/j.matre.2021.100065. doi: 10.1016/j.matre.2021.100065
![]() |
[55] |
Dai T, Lei P, Zhang B, et al. (2021) Tricyclic or pentacyclic D units: Design of D−π-A-Type copolymers for high VOC organic photovoltaic cells. ACS Appl Mater Interfaces 13: 30756-30765. https://doi.org/10.1021/acsami.1c08487 doi: 10.1021/acsami.1c08487
![]() |
[56] |
Yan T, Bin H, Sun C, et al. (2018) Effect of Thieno[3, 2-b]thiophene π-bridge on photovoltaic performance of a D-A copolymer of alkoxy-benzodithiophene-alt-fluoro-benzotriazole. Org Electron 55: 106-111. https://doi.org/10.1016/j.orgel.2018.01.018 doi: 10.1016/j.orgel.2018.01.018
![]() |
[57] |
Bin H, Xiao L, Liu Y, et al. (2014) Effects of donor unit and p-Bridge on photovoltaic properties of D-A copolymers based on Benzo[1, 2-b:4, 5-c']-dithiophene-4, 8-dione acceptor unit. J Polym Sci Part A: Polym Chem 52: 1929-1940. https://doi.org/10.1002/pola.27209 doi: 10.1002/pola.27209
![]() |
[58] |
Akkuratov AV, Mühlbach S, Susarova DK, et al. (2017) Positive side of disorder: Statistical fluorene-carbazole-TTBTBTT terpolymers show improved optoelectronic and photovoltaic properties compared to the regioregular structures. Sol Energy Mater Sol Cells 160: 346-354. https://doi.org/10.1016/j.solmat.2016.10.039 doi: 10.1016/j.solmat.2016.10.039
![]() |
[59] |
Jiang X, Yang Y, Zhu J, et al. (2017) Constructing D-A copolymers based on thiophene-fused benzotriazole units containing different alkyl side-chains for non-fullerene polymer solar cells. J Mater Chem C 5: 8179-8186. https://doi.org/10.1039/C7TC02098H doi: 10.1039/C7TC02098H
![]() |
[60] |
Zhou P, Yang Y, Chen X, et al. (2017) Design of a thiophene-fused benzotriazole unit as an electron acceptor to build D-A copolymers for polymer solar cells. J Mater Chem C 5: 2951-2957. https://doi.org/10.1039/C7TC00083A doi: 10.1039/C7TC00083A
![]() |
[61] |
Jiang X, Wang J, Yang Y, et al. (2018) Fluorinated Thieno[2', 3':4, 5]benzo[1, 2‑d][1, 2, 3]triazole: New acceptor unit to construct polymer donors. ACS Omega 3: 13894-13901. https://doi.org/10.1021/acsomega.8b02053 doi: 10.1021/acsomega.8b02053
![]() |
[62] |
Chang C, Li W, Guo X, et al. (2018) A narrow-bandgap donor polymer for highly efficient as-cast non-fullerene polymer solar cells with a high open circuit voltage. Org Electron 58: 82-87. https://doi.org/10.1016/j.orgel.2018.04.001 doi: 10.1016/j.orgel.2018.04.001
![]() |
[63] |
Sun C, Pan F, Bin H, et al. (2018) A low cost and high performance polymer donor material for polymer solar cells. Nature Commun 9: 1-10. https://doi.org/10.1038/s41467-018-03207-x doi: 10.1038/s41467-018-03207-x
![]() |
[64] |
Fan B, Zhang D, Li M, et al. (2019) Achieving over 16% efficiency for single-junction organic solar cells. Sci China Chem 62: 746-752. https://doi.org/10.1007/s11426-019-9457-5 doi: 10.1007/s11426-019-9457-5
![]() |
[65] |
Xiong J, Jin K, Jiang Y, et al. (2019) Thiolactone copolymer donor gifts organic solar cells a 16.72% efficiency. Sci Bull 64: 1573-1576. https://doi.org/10.1016/j.scib.2019.10.002 doi: 10.1016/j.scib.2019.10.002
![]() |
[66] |
Liu Q, Jiang Y, Jin K, et al. (2020) 18% Efficiency organic solar cells. Sci Bull 65: 272-275. https://doi.org/10.1016/j.scib.2020.01.001 doi: 10.1016/j.scib.2020.01.001
![]() |
[67] | Matsuo Y, Hatano J, Kuwabara T, et al. (2012) Fullerene acceptor for improving open-circuit voltage in inverted organic photovoltaic devices without accompanying decrease in short-circuit current density. Appl Phys Lett, 100. https://doi.org/10.1063/1.3683469 |
[68] |
Zhao G, He Y, Li Y (2010) 6.5% Efficiency of polymer solar cells based on poly(3‐hexylthiophene) and Indene‐C60 bisadduct by device optimization. Adv Mater 22: 4355-4358. https://doi.org/10.1002/adma.201001339 doi: 10.1002/adma.201001339
![]() |
[69] |
Cai Y, Li Y, Wang R, et al. (2021) A Well-Mixed phase formed by two compatible Non-Fullerene acceptors enables ternary organic solar cells with efficiency over 18.6%. Adv Mater 33: 2101733. https://doi.org/10.1002/adma.202101733 doi: 10.1002/adma.202101733
![]() |
[70] |
Li M, Gao K, Wan X, et al. (2017) Solution-processed organic tandem solar cells with power conversion efficiencies > 12%. Nature Photonics 11: 85-90. https://doi.org/10.1038/nphoton.2016.240 doi: 10.1038/nphoton.2016.240
![]() |
[71] |
Chen X, Kan B, Kan Y, et al. (2020) As-Cast ternary organic solar cells based on an asymmetric Side-Chains featured acceptor with reduced voltage loss and 14.0% efficiency. Adv Funct Mater 30: 1909535. https://doi.org/10.1002/adfm.201909535 doi: 10.1002/adfm.201909535
![]() |
[72] |
Xiao L, Wu X, Ren G, et al. (2021) Highly efficient ternary solar cells with efficient förster resonance energy transfer for simultaneously enhanced photovoltaic parameters. Adv Funct Mater 31: 2105304. https://doi.org/10.1002/adfm.202105304 doi: 10.1002/adfm.202105304
![]() |
[73] |
Sharma R, Lee H, Seifrid M, et al. (2020) Performance enhancement of conjugated polymer-small molecule-non fullerene ternary organic solar cells by tuning recombination kinetics and molecular ordering. Sol Energy 201: 499-507. https://doi.org/10.1016/j.solener.2020.03.008 doi: 10.1016/j.solener.2020.03.008
![]() |
[74] |
Wan J, Zhang L, He Q, et al. (2020) High-Performance pseudoplanar heterojunction ternary organic solar cells with nonfullerene alloyed acceptor. Adv Funct Mater 30: 1909760. https://doi.org/10.1002/adfm.201909760 doi: 10.1002/adfm.201909760
![]() |
[75] |
Liu T, Guo Y, Yi Y, et al. (2016) Ternary organic solar cells based on two compatible nonfullerene acceptors with power conversion efficiency > 10%. Adv Mater 28: 10008-10015. https://doi.org/10.1002/adma.201602570 doi: 10.1002/adma.201602570
![]() |
[76] |
Kumari T, Lee SM, Yang C (2018) Cubic-Like bimolecular crystal evolution and over 12% efficiency in halogen-free ternary solar cells. Adv Funct Mater 28: 1707278. https://doi.org/10.1002/adfm.201707278 doi: 10.1002/adfm.201707278
![]() |
[77] |
Xie L, Yang C, Zhou R, et al. (2020) Ternary organic solar cells BasedonTwoNon-fullerene acceptors with complimentary absorption and balanced crystallinity. Chin J Chem 38: 935-940. https://doi.org/10.1002/cjoc.201900554 (2020) doi: 10.1002/cjoc.201900554(2020)
![]() |
[78] |
Xu R, Zhang K, Liu X, et al. (2018) Alkali Salt-Doped highly transparent and Thickness-Insensitive Electron-Transport layer for High-Performance polymer solar cell. ACS Appl Mater Interfaces 10: 1939-1947. https://doi.org/10.1021/acsami.7b17076 doi: 10.1021/acsami.7b17076
![]() |
[79] |
Singh A, Dey A, Das D, et al. (2016) Effect of dual cathode buffer layer on the charge carrier dynamics of rrP3HT: PCBM based bulk heterojunction solar cell. ACS Appl Mater Interfaces 8: 10904-10910. https://doi.org/10.1021/acsami.6b03102 doi: 10.1021/acsami.6b03102
![]() |
[80] |
Kageyama H, Kajii H, Ohmori Y, et al. (2011) MoO3 as a cathode buffer layer material for the improvement of planar pn-heterojunction organic solar cell performance. Appl Phys Express 4: 032301. https://doi.org/10.1143/APEX.4.032301 doi: 10.1143/APEX.4.032301
![]() |
[81] |
Sachdeva S, Kaur J, Sharma K, et al. (2018) Performance improvements of organic solar cell using dual cathode buffer layers. Curr Appl Phys 18: 1592-1599. https://doi.org/10.1016/j.cap.2018.10.009 doi: 10.1016/j.cap.2018.10.009
![]() |
[82] |
Yu X, Yu X, Zhang J, et al. (2015) Gradient Al-doped ZnO multi-buffer layers: Effect on the photovoltaic properties of organic solar cells. Mater Lett 161: 624-627. https://doi.org/10.1016/j.matlet.2015.09.017 doi: 10.1016/j.matlet.2015.09.017
![]() |
[83] |
Pandey R, Lim JW, Kim JH, et al. (2018) Performance enhancement in organic photovoltaic solar cells using iridium (Ir) ultra-thin surface modifier (USM). Appl Surf Sci 444: 97-104. https://doi.org/10.1016/j.apsusc.2018.03.012 doi: 10.1016/j.apsusc.2018.03.012
![]() |
1. | Ahmed Alsaedi, Bashir Ahmad, Afrah Assolami, Sotiris K. Ntouyas, On a nonlinear coupled system of differential equations involving Hilfer fractional derivative and Riemann-Liouville mixed operators with nonlocal integro-multi-point boundary conditions, 2022, 7, 2473-6988, 12718, 10.3934/math.2022704 | |
2. | M. Vellappandi, Venkatesan Govindaraj, José Vanterler da C. Sousa, Fractional optimal reachability problems with ψ ‐Hilfer fractional derivative , 2022, 45, 0170-4214, 6255, 10.1002/mma.8168 | |
3. | Fidel Meléndez-Vázquez, Guillermo Fernández-Anaya, Aldo Jonathan Muñóz-Vázquez, Eduardo Gamaliel Hernández-Martínez, Generalized conformable operators: Application to the design of nonlinear observers, 2021, 6, 2473-6988, 12952, 10.3934/math.2021749 | |
4. | Hamid Lmou, Khalid Hilal, Ahmed Kajouni, Serkan Araci, A New Result for ψ -Hilfer Fractional Pantograph-Type Langevin Equation and Inclusions, 2022, 2022, 2314-4785, 1, 10.1155/2022/2441628 | |
5. | Ravi P. Agarwal, Afrah Assolami, Ahmed Alsaedi, Bashir Ahmad, Existence Results and Ulam–Hyers Stability for a Fully Coupled System of Nonlinear Sequential Hilfer Fractional Differential Equations and Integro-Multistrip-Multipoint Boundary Conditions, 2022, 21, 1575-5460, 10.1007/s12346-022-00650-6 | |
6. | Abdulwasea Alkhazzan, Wadhah Al-Sadi, Varaporn Wattanakejorn, Hasib Khan, Thanin Sitthiwirattham, Sina Etemad, Shahram Rezapour, A new study on the existence and stability to a system of coupled higher-order nonlinear BVP of hybrid FDEs under the p-Laplacian operator, 2022, 7, 2473-6988, 14187, 10.3934/math.2022782 | |
7. | Sina Etemad, Iram Iqbal, Mohammad Esmael Samei, Shahram Rezapour, Jehad Alzabut, Weerawat Sudsutad, Izzet Goksel, Some inequalities on multi-functions for applying in the fractional Caputo–Hadamard jerk inclusion system, 2022, 2022, 1029-242X, 10.1186/s13660-022-02819-8 |
Parameters | Details |
β1 | Susceptible form of recovered individuals |
αm | Newborn mosquitoes transfer rate into Sm |
αh | Newborns ratio to enter in Sh |
γ1 | Transfer ratio of illness from one affected to susceptible people |
ψ | Transfusions rate of average blood with stable time in Ih and Sh |
β2 | Exposed to infected infection rate in people |
ξ | Infected mosquito susceptible bites using fixed time |
γ3 | Rate of Mosquitos infection |
γ2 | Disease transfer through infected (mosquito) to susceptible (individual) |
ηh | People natural rate of death |
δ2 | Exposed recovered population |
δ1 | Susceptible recovered population |
p | Rate of humans heal |
ε | Infected human death due to disease |
ηm | Mosquitos' natural death |
υ | Anti-malarial prescriptions |
e | Bites Sm using the infected humans |
σ | Expires sections using spraying for Sm and Im |
x | Time |
Case | MSE for instances of sampling | Gradient | Performance | Epoch | Time | ||
Test | validation | Train | |||||
I | 2.745×10−07 | 1.697 ×10−07 | 2.366×10−07 | 1.42×10−06 | 2.26×10−07 | 100 | 2 Sec |
II | 1.795×10−07 | 1.432×10−07 | 1.920×10−07 | 8.18×10−07 | 1.91×10−07 | 86 | 2 Sec |
III | 5.112×10−07 | 3.038×10−07 | 3.678×10−07 | 2.94×10−06 | 2.09×10−07 | 69 | 2 Sec |
Parameters | Details |
β1 | Susceptible form of recovered individuals |
αm | Newborn mosquitoes transfer rate into Sm |
αh | Newborns ratio to enter in Sh |
γ1 | Transfer ratio of illness from one affected to susceptible people |
ψ | Transfusions rate of average blood with stable time in Ih and Sh |
β2 | Exposed to infected infection rate in people |
ξ | Infected mosquito susceptible bites using fixed time |
γ3 | Rate of Mosquitos infection |
γ2 | Disease transfer through infected (mosquito) to susceptible (individual) |
ηh | People natural rate of death |
δ2 | Exposed recovered population |
δ1 | Susceptible recovered population |
p | Rate of humans heal |
ε | Infected human death due to disease |
ηm | Mosquitos' natural death |
υ | Anti-malarial prescriptions |
e | Bites Sm using the infected humans |
σ | Expires sections using spraying for Sm and Im |
x | Time |
Case | MSE for instances of sampling | Gradient | Performance | Epoch | Time | ||
Test | validation | Train | |||||
I | 2.745×10−07 | 1.697 ×10−07 | 2.366×10−07 | 1.42×10−06 | 2.26×10−07 | 100 | 2 Sec |
II | 1.795×10−07 | 1.432×10−07 | 1.920×10−07 | 8.18×10−07 | 1.91×10−07 | 86 | 2 Sec |
III | 5.112×10−07 | 3.038×10−07 | 3.678×10−07 | 2.94×10−06 | 2.09×10−07 | 69 | 2 Sec |