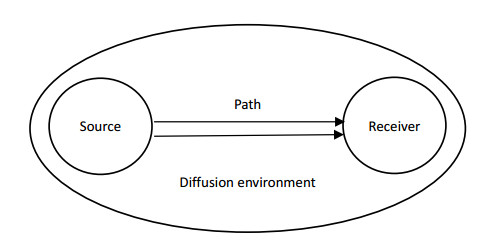
By constructing a technological innovation diffusion (TID) index system of the manufacturing industry with 15 indexes from four dimensions of diffusion source, diffusion path, diffusion receiver, and diffusion environment, this paper measures the TID in the manufacturing industry and analyzes its distribution characteristics from the perspective of industry and region from 2005 to 2018 by using the entropy weight method and the dynamic multi-indicator projection pursuit (PP-IPM) method. The results show that the TID in China's manufacturing industry has a good development trend in the sample period, and the diffusion resources and paths have a relatively greater impact on TID; the absorptive capacity of the receiver also has a significant impact, while the impact of the diffusion environment is relatively small. During the sample period, at the sector level, a pattern has formed in which transportation equipment manufacturing, electrical machinery and equipment manufacturing, and communication equipment, computer and electronic equipment manufacturing are the sources of diffusion, gradually spreading to other sectors according to the degree of industrial relevance. At the regional level, the diffusion pattern is that Guangdong, Jiangsu, Zhejiang, Shandong, Beijing, and Shanghai are the sources and diffuse to the surrounding and central and western regions successively.
Citation: Mengxin Wang, Lang Li, Hanyong Lan. The measurement and analysis of technological innovation diffusion in China's manufacturing industry[J]. National Accounting Review, 2021, 3(4): 452-471. doi: 10.3934/NAR.2021024
[1] | Ming He, Barnabé Walheer . Technology intensity and ownership in the Chinese manufacturing industry: A labor productivity decomposition approach. National Accounting Review, 2020, 2(2): 110-137. doi: 10.3934/NAR.2020007 |
[2] | Shuang Luo, Wenting Lei, Peng Hou . Impact of artificial intelligence technology innovation on total factor productivity: an empirical study based on provincial panel data in China. National Accounting Review, 2024, 6(2): 172-194. doi: 10.3934/NAR.2024008 |
[3] | Guido Ferrari, Yanyun Zhao, José Mondéjar Jiménez, Luca Secondi . The economy of Tuscany in the post Covid-19 era: struggling with energy crisis and inflation. What to do to resume the journey?1. National Accounting Review, 2024, 6(1): 1-26. doi: 10.3934/NAR.2024001 |
[4] | Jinhui Zhu, Zhenghui Li . Can digital financial inclusion effectively stimulate technological Innovation of agricultural enterprises?—A case study on China. National Accounting Review, 2021, 3(4): 398-421. doi: 10.3934/NAR.2021021 |
[5] | Tinghui Li, Xue Li . Does structural deceleration happen in China? Evidence from the effect of industrial structure on economic growth quality. National Accounting Review, 2020, 2(2): 155-173. doi: 10.3934/NAR.2020009 |
[6] | Yanting Xu, Tinghui Li . Measuring digital economy in China. National Accounting Review, 2022, 4(3): 251-272. doi: 10.3934/NAR.2022015 |
[7] | Jinhong Wang, Yanting Xu . Factors influencing the transition of China's economic growth momentum. National Accounting Review, 2024, 6(2): 220-244. doi: 10.3934/NAR.2024010 |
[8] | Sylvie Kotíková . Spillover effects: A challenging public interest to measure. National Accounting Review, 2023, 5(4): 373-404. doi: 10.3934/NAR.2023022 |
[9] | Kewei Ma, Guido Ferrari, Zichuan Mi . SAM-based analysis of China’s economic system and measurement of the effects of a VAT rate cut after the tax reform. National Accounting Review, 2020, 2(1): 26-52. doi: 10.3934/NAR.2020002 |
[10] | Jiehua Ma, Zhenghui Li . Measuring China's urban digital economy. National Accounting Review, 2022, 4(4): 329-361. doi: 10.3934/NAR.2022019 |
By constructing a technological innovation diffusion (TID) index system of the manufacturing industry with 15 indexes from four dimensions of diffusion source, diffusion path, diffusion receiver, and diffusion environment, this paper measures the TID in the manufacturing industry and analyzes its distribution characteristics from the perspective of industry and region from 2005 to 2018 by using the entropy weight method and the dynamic multi-indicator projection pursuit (PP-IPM) method. The results show that the TID in China's manufacturing industry has a good development trend in the sample period, and the diffusion resources and paths have a relatively greater impact on TID; the absorptive capacity of the receiver also has a significant impact, while the impact of the diffusion environment is relatively small. During the sample period, at the sector level, a pattern has formed in which transportation equipment manufacturing, electrical machinery and equipment manufacturing, and communication equipment, computer and electronic equipment manufacturing are the sources of diffusion, gradually spreading to other sectors according to the degree of industrial relevance. At the regional level, the diffusion pattern is that Guangdong, Jiangsu, Zhejiang, Shandong, Beijing, and Shanghai are the sources and diffuse to the surrounding and central and western regions successively.
The diffusion of technological innovation is the process that technological innovation is adopted by its users through specific channels. In the process of technological innovation diffusion (TID), the users of new technology not only get the technology accumulation but also obtain technological progress through the digestion, absorption, and production of new technology, further promoting the diffusion of technological innovation. Therefore, whether technological innovation can eventually become the source of social and technological progress depends largely on the diffusion of technological innovation. Studies have shown that the diffusion effect of technological innovation is influenced by factors such as time and geographical distance (Comin et al., 2012; Ertur et al., 2017; Santacreu, 2019).
In recent years, with the development of industrial agglomeration, association, and integration, the diffusion of technological innovation among industries is becoming increasingly important (Hacking et al., 2019; Lee et al., 2016; Lyu et al., 2017). At present, great differences exist in the technological innovation level and the independent innovation ability among sectors in China's manufacturing industry. Industries with relatively leading technology and a high degree of innovation, such as information and communication, information technology, and intelligent equipment, have produced technology diffusion effect to a large extent, and foreign advanced technologies introduced through channels like foreign direct investment and international trade also have diffusion effects. Enough attention should be paid to the diffusion of these advanced technologies, especially when China's economic development turns to high-quality development. Research and analysis of the diffusion situation of technological innovation in the manufacturing industry are of great practical significance for improving the scientific and technological innovation strength and optimizing and upgrading the industry.
The research on the diffusion of technological innovation mainly focuses on the evolution process, influencing factors, simulation, and prediction, among which the simulation and prediction of TID is the focus of research. In the early stage, scholars mainly used mathematical models like differential dynamics, jump-diffusion model and Markov chain to simulate the process and speed change of innovation diffusion over time. Laciana and Rovere (2011) simulated the dynamic diffusion process of technological innovation and found that the new technological diffusion rate accelerates when the differences between individuals become larger. With the deepening of research, some scholars have studied the spatial spillover process of TID from the spatial perspective, such as Cheng and Shen (2018), who studied the spatial technological diffusion effect spatial-temporal characteristics at the provincial level in China. In recent years, some scholars have focused on simulating the complex network structure and path characteristics of TID from the perspective of complex networks. For example, Cao et al. (2018) simulated TID by using the evolutionary game of complex networks under different network topology structures such as small-world and scale-free; Yu et al. (2020) revealed the complexity of TID from the perspective of multiple networks. The above research studies the diffusion process of technological innovation from different perspectives and believes that technological diffusion can be a communication process, a substitution process, a learning process, a spatial process, a game process, or a network evolution process.
Few studies focus on measuring TID in the manufacturing industry, and most scholars mainly take single indicators such as technology market turnover, technology license, and patent introduction as alternative variables for the TID. Xu et al. (2013) used the technology transaction volume to measure the technology diffusion and studied the impact of the technology diffusion in Beijing and Shanghai on the economic growth of China's various regions. Dechezleprêtre et al. (2015) studied the transnational diffusion of new technologies under environmental control by measuring the flow of new technologies from source countries to recipient countries using patents. Lv et al. (2020) studied whether the differentiation phenomenon of industrial technology groups affects the diffusion of industrial innovation by measuring the innovation diffusion with the number of patents cited by other patentees. Although the above-mentioned studies measure the diffusion of technological innovation from different aspects, there are significant differences in the diffusion results measured by various alternative variables; thus, it has certain limitations to measure the diffusion of technological innovation only by a single indicator. Nelson (2009) adopted the direct citation patents, secondary citation patents, patent licenses, and publication citations generated by the recombinant DNA technology as the measurement indicators of innovation diffusion, respectively, and they found that the direct citation patents greatly underestimated the degree of technological diffusion compared with the indicators such as patent licenses and publication citations. Huang et al. (2011) compared innovation diffusion indicators such as patent citation, technology licenses, and publication citations, and they found that different indicators had different roles in different stages of innovation diffusion, and a single indicator failed to capture the overall situation of innovation diffusion fully. Some scholars also focus on the diffusion of technological innovation in some industries, including agriculture and green environmental protection industries. Yu et al. (2018) comprehensively measured the environment of Chinese agricultural technology diffusion from the perspectives of nature, society, science and technology, economy and policy. Grafström (2017) confirmed the existence of TID among countries in the wind power field in Europe by using patent data. Hötte (2020) found that technological changes can accelerate the development of green technology innovation and diffusion under the continuous development of absorption capacity. Cole and Fernando (2021) evaluated the diffusion and sustainability of mobile-phone-based agricultural advice technology in Indian cotton farmers.
In general, the existing research has some reference significance for measuring the TID in the manufacturing industry, but there is also some space to be explored. First, the existing research focuses on the diffusion environment of technological innovation, and little research deeply discusses the actual measurement and application of the index system of TID. Even if the measurement of TID is carried out, it mainly focuses on the regional perspective and lacks the analysis of TID of various sectors from the industry perspective. Secondly, the subject of TID involves the spillover capacity of the diffusion source and the absorption capacity of the receiver. The existing research mainly builds the diffusion index system from the perspective of the spillover capacity, and the absorption capacity of the receiver has not been fully reflected in the index system. The main contributions of this paper are as follows. First, considering the absorptive capacity of the receiver, this paper constructs a TID index system, including the absorptive capacity of the receiver and the spillover capacity of diffusion sources. Second, this paper focuses on the manufacturing industry and measures the diffusion innovation in the manufacturing industry from the perspectives of sector and region, and the distribution characteristics are also analyzed.
TID plays an essential role in the dissemination of new technologies. TID is a process in which technological innovation spreads from the source of diffusion to the receiver of technological innovation, generally from the sector or region with a higher technological level to that with a lower technological level. An effective diffusion process of technology innovation can affect the overall improvement of the technology level of the industry or region to a large extent. Based on Rogers' innovation diffusion theory (Rogers, 2003), a complete TID system includes four essential components: diffusion source, diffusion path, diffusion receiver, and diffusion environment that influence the whole diffusion system. The interrelationship of the components can be illustrated in Figure 1.
For the diffusion system shown in Figure 1, the diffusion source is the origin of technological innovation (Rogers, 2003), and it is the diffusion side with more advanced or superior technology. The diffusion source generally needs to have a high technical level and resource conditions for innovation diffusion, such as sufficient human resources reserves and patent technology. It can be an enterprise with advanced technology, an enterprise group, or a specific region. The diffusion receiver refers to the party that accepts advanced or superior technology, and it is the target of innovative diffusion. It can be a specific enterprise, an enterprise group, or a certain region. Compared with the diffusion source, the technical level of the diffusion receiver should be relatively low, forming a certain technical potential difference with the diffusion source so as to promote the effective diffusion of technological innovation (Jan, 2002). At the same time, the receiver should also have certain primary conditions, such as certain technical reserves and human resources level and suitable infrastructure conditions, which can be regarded as a technological absorption capacity of the receiver. Only when the receiver has a specific technological absorption capacity can it effectively undertake the technological spillover of the diffusion source, promoting the diffusion of technological innovation (Cohen and Levinthal, 1990). In the cycle of identification, absorption, application and re-innovation of new technologies, not only the receiver will improve its own technical level but also the superior technologies formed by re-innovation will further promote the diffusion of innovation. The diffusion path is the method, tool, or carrier through which technological innovation spreads from the diffusion source to the receiver. In the context of economic globalization, the scope of diffusion can be global. Diffusion tools or carriers include various forms of technology transfer, trade, and investment. The diffusion environment is the sum of various factors that play a comprehensive effect on the diffusion system (Wang et al., 2003). In general, an effective diffusion system should have a good diffusion environment to serve technological diffusion effectively. Whether the diffusion environment is conducive to innovation diffusion can be reflected in the diffusion speed. The faster the diffusion speed is, the better the diffusion environment is; conversely, the slower the diffusion speed, the worse the diffusion environment.
Based on the above analysis on the technology innovation diffusion system, comprehensively considering various factors of diffusion source, diffusion receiver, diffusion path, and diffusion environment, this paper constructs a TID index system including the above four dimensions to measure the comprehensive situation of TID.
The diffusion source provides diffusion resources, which is fundamentally characterized by a strong technical level and a variety of innovation resources for diffusion. In selecting indicators, this paper starts from two aspects. First, innovation activities of enterprises mainly come from purposeful research and development (R & D), which requires human resources and material capital investment. This paper selects the full-time equivalent of R & D personnel and the expenditure of R & D instruments and equipment to reflect it. Considering that not all enterprises carry out R & D activities, this paper adopts the number of industrial enterprises with R & D activities to reflect the sustainability of technological innovation. Second, the most direct manifestation of corporate R & D activities is the new product development. Given the periodicity of technology research and development, this paper uses the number of new product development projects and the number of effective invention patents to reflect the technological innovation in the R & D stage and the successful stage, respectively.
There are many paths for TID. Technology transfer, investment, and trade may be the path for TID. Given the availability of data, this paper mainly considers two aspects. One is the direct technology innovation diffusion path, that is, the technology diffusion by the transfer of non-patented technology or patent ownership, among which the non-patented technology transfer is generally through the technology market transactions, so this paper chooses the technology output amount in the technology market to reflect it, while the patent ownership transfer is directly reflected by the transfer of patent rights and license income. The other is the indirect path of TID, that is, the sales of new products drive the diffusion of technological innovation. For manufacturers with strong technological innovation ability, a high technical level is implied in their new products, which will spread to the product buyer (diffusion receiver) indirectly. This paper reflects the indirect diffusion of technological innovation through the indicator of new product sales income. The higher the income from new product sales, the more indirect technology innovation diffusion.
Based on the aforementioned theoretical analysis, the absorption capacity is mainly considered in terms of diffusion receiver. The size of the absorption capacity will affect the diffusion effect of the new technology, and the receiver needs a good absorption capacity to identify and absorb the introduced new technology. First, this paper uses "technology inflow amount (10,000 yuan) in the technology market" to reflect the market recognition of the receiver. The more the inflow amount, the more the market recognizes the absorption capacity of the receiver. Indicators such as "technology digestion and absorption expenditure (10,000 yuan)" and "technology transformation expenditure (10,000 yuan)" are adopted to reflect the receiver's efforts to improve its technology level. The more these two inputs are, the stronger the receiver's absorption capacity is.
In the diffusion system, the quality of the diffusion environment is mainly reflected in the diffusion speed. The faster the diffusion speed, the more continuous the emergence and diffusion of new technologies, indicating that labor productivity between industries or regions has increased rapidly. This paper selects indicators like the technology output growth rate, the patent licensing growth rate, the new product sales growth rate, and the current asset turnover rate to reflect the diffusion environment. The higher the rates of these indicators, the better the diffusion environment for technological innovation. Seven of the value indicators with the unit of "10,000 yuan" in Table 1 are based on the current year price. When processing data later with the model, the influence of the price factor is eliminated by standardizing the data.
Diffusion system | Dimension | Indicator | Unit |
Technological innovation diffusion (TID) | Diffusion source (Diffusion resources) | R & D personnel full-time equivalent | person-year |
R & D instruments & equipment expenditures | 10,000 yuan | ||
Industrial enterprises with R & D activities | Unit | ||
Number of new product development projects | Item | ||
Number of effective invention patents | Piece | ||
Diffusion path | Technology output amount of the technology market | 10,000 yuan | |
Patent ownership transfer and licensing income | 10,000 yuan | ||
Sales income of new products | 10,000 yuan | ||
Diffusion receiver (Absorption capacity) | Technology inflow amount in the technology market | 10,000 yuan | |
Technology digestion and absorption expenditure | 10,000 yuan | ||
Technological transformation expenditure | 10,000 yuan | ||
Diffusion environment | Technology output growth rate | % | |
Patent licensing growth rate | % | ||
New product sales growth rate | % | ||
the turnover rate of current assets | % |
The main purpose of this paper is to measure the TID in various manufacturing sectors and regions in China. The sample interval determined according to available data is from 2005 to 2018. At the regional level, it involves 31 provinces and cities (excluding Hong Kong, Macao, and Taiwan), and at the industry level, it is selected and determined according to the industry classification standard of the national economy. Within the time scope considered in this paper, China has implemented three industry classification standards, namely, National Economic Industry Classification in 2002, 2011, and 2017. Considering the industry classification standard in 2011 involving the longest time range of this study and based on the 2011 standard as a benchmark, this paper appropriately adjusts and merges the industry classification, in which the non-metallic mineral products sector is merged into other manufacturing sectors, and the automobile manufacturing sector, railway, ship, aerospace, and other transportation equipment manufacturing sector are merged into the transportation equipment manufacturing sector. After adjustment, 27 manufacturing sectors are obtained. Table 2 shows specific manufacturing sectors and categories.
Order number | Sector | Code in this study | Order number | Sector | Code in this study |
1 | Agricultural and sideline food | Z1 | 15 | Pharmaceutical manufacturing | Z15 |
processing sector | 16 | Chemical fiber manufacturing | Z16 | ||
2 | Food manufacturing | Z2 | 17 | Rubber and Plastic Products | Z17 |
3 | Wine, beverage, and refined tea | Z3 | sector | ||
manufacturing sector | 18 | Ferrous metal smelting and | Z18 | ||
4 | Tobacco sector | Z4 | rolling processing sector | ||
5 | Textile sector | Z5 | 19 | Non-ferrous metal smelting and | Z19 |
6 | Textile and clothing sector | Z6 | rolling processing sector | ||
7 | Leather, fur, feathers and their | Z7 | 20 | Metal products sector | Z20 |
products and shoe-making sector | 21 | General equipment | Z21 | ||
8 | Wood processing and wood, | Z8 | manufacturing | ||
bamboo, rattan, brown, grass | 22 | Special equipment | Z22 | ||
products sector | manufacturing | ||||
9 | Furniture manufacturing | Z9 | 23 | Transportation equipment | Z23 |
10 | Paper making and paper products | Z10 | manufacturing | ||
sector | 24 | Electrical machinery and | Z24 | ||
11 | Printing industry and recording | Z11 | equipment manufacturing | ||
media replication sector | 25 | Computers, communications, | Z25 | ||
12 | Culture, education, industrial | Z12 | and other electronic equipment | ||
beauty, sports and entertainment | manufacturing sector | ||||
goods manufacturing sector | 26 | Instrument manufacturing | Z26 | ||
13 | Oil processing, coking, and nuclear | Z13 | 27 | Other manufacturing sector | Z27 |
fuel processing sector | |||||
14 | Chemical raw materials and | Z14 | |||
chemical products manufacturing | |||||
sector |
According to the index system shown in Table 1, the corresponding data of 27 manufacturing sectors and 31 provinces and cities are collected and sorted. Relevant data are obtained from China Industrial Statistical Yearbook, China Science and Technology Statistics Yearbook, China Regional Innovation Capacity Monitoring Report, China Technology Market Report, and the EPS database. Due to the change of statistical caliber, the index data after 2010 are the data of industrial enterprises above designated size, while the data of 2010 and previous years are of large and medium-sized industrial enterprises1. In order to ensure the comparability of the data, this paper adjusts the data before 2010 according to the total assets ratio of large and medium-sized industrial enterprises in the corresponding year to obtain the data of industrial enterprises above the designated size in the corresponding year. In addition, the moving average method is used to deal with the missing data of very few indicators.
1Since 2011, the statistical scope of industrial enterprise data in the China Yearbook of Science and Technology Statistics has been adjusted from large and medium-sized industrial enterprises to industrial enterprises above designated size. Some special years have expanded the scope of the survey due to the economic census or R&D resource inventory, and industrial data above the designated size are also available, such as the year 2004, 2008 and 2009.
Since the 1960s, some scholars have characterized the diffusion situation of technological innovation by referring to the diffusion model in communication, and gradually a series of TID models have been formed represented by the S curve diffusion model, the gravity model, and the maximum entropy model. In this paper, the dynamic projection pursuit clustering method commonly used in analyzing high-dimensional data is used to measure TID. Projection Pursuit (PP) is a statistical method for processing and analyzing high-dimensional data. It can project high-dimensional data onto low-dimensional (1–3 dimensional) subspace to find projections that reflect the structure or features of original high-dimensional data so as to achieve the purpose of studying and analyzing high-dimensional data (Friedman and Tukey, 1974). An ideal point method based on projection pursuit for dynamic multiple indicators (PP-IPM) improves the PP method (Jin et al., 2004). Its advantage is that it can be used to evaluate nonlinear, non-normal and other high-dimensional data, and the results of optimal projection have high accuracy, good robustness, and strong anti-interference ability (Lyu et al., 2017). This paper studies the TID in China's manufacturing industry from 2005 to 2018 and among regions, involving 15 indicators in each of 27 sectors and 31 provinces and municipalities. The data set for research is characterized by multiple dimensions and high complexity, and the PP-IPM method is suitable for the measurement.
In addition, this study involves 15 metrics, and the determination of the weights has a large impact on the study results. To ensure the scientific weight setting, the entropy method is used to determine the weights. The entropy method is an objective empowerment method that determines the index weight based on the information provided by the observed values of each index (Jin and Li, 2018). It can avoid the one-sided nature of the subjective empowerment method and ensure the objectivity and accuracy of the measurement results.
Suppose that the measurement evaluation system has m years, n objects, and k evaluation indexes; Tα(α=1,2,⋯,m), indicating year α; Yi(i=1,2,⋯,n), representing object i, and assuming z(i) are valid solutions; Pj(j=1,2,⋯,k), representing evaluation index j; xaij represents the index value j of object i in the year a. The basic steps of determining index weight by using entropy method and then combining the PP-IPM method to build a model for measuring TID are as follows.
For a positive index:
baij=xaij−xminxmax−xmin | (1) |
For a reverse index:
baij=xmax−xaijxmax−xmin | (2) |
where baij represents the index value j of index i in year a after the dimensionless treatment. xmin is the minimum of index j, and xmax is the maximum of index j. The normalized panel data matrix is expressed as B=(B1,B2,⋯Bn)T, where, Bi=(baij)mk.
1. Calculate the entropy of index j
Ej=−k1m∑a=1n∑i=1PaijlnPaij | (3) |
where k1=1ln(mn), Paij=baij∑ma=1∑ni=1baij.
2. Calculate the weight of index j by the entropy method
w1j=Dj∑kj=1Dj | (4) |
where Dj=1−Ej
1. Generate the positive and negative ideal matrices
According to the normalized matrix B, a positive ideal matrix B+=(b+aj)mk and a negative ideal matrix B−=(b−aj)mk are generated, where b+aj=max(baij), b−aj=min(baij).
2. Construct the projection pursuit function
The PP method is used to combine the normalized matrix B, the positive ideal matrix B+, and the negative ideal matrix B− to obtain a projection direction vector c=(p1,p2,⋯,pj,⋯,pk,q1,q2,⋯,qa,⋯,qm), including indexes and time weights, thus obtaining the one-dimensional projection value z(i):
z(i)={∑ma=1qa[∑kj=1pj(baij−b−aj)2]}1/2{∑ma=1qa[∑kj=1pj(baij−b+aj)2]}1/2+{∑ma=1qa[∑kj=1pj(baij−b−aj)2]}1/2 | (5) |
where, pj is the weight of index j; qa is the weight of year a; z(i) represents the Euclidean distance or relative proximity of the evaluation object Yi with B+ and B−, that is, it is closest to B+ and farthest from B−. According to the principle of scattering projection values as far as possible, the objective function is constructed:
Q(c)=Sz=√∑ni(z(i)−Ez)2n | (6) |
where, Sz is the standard deviation of z(i); Ez represents the mean of the sequence {z(i)|i=1,2,⋯,n}.
3. Solve the nonlinear optimization projection pursuit objective function with constraints
When the sample panel data matrix is given, the projection objective function Q(c) varies only with the projection direction vector c. Therefore, the essence of solving the optimal projection direction is to maximize the objective function of nonlinear projection with constraints:
{maxQ(c)st.k∑j=1pj=1m∑a=1qa=1pj>0,qa>0 | (7) |
It is difficult to solve the optimal projection vector of the aforementioned nonlinear programming problem using conventional methods. Thus, this paper adopts the accelerated genetic algorithm (RAGA) to simulate the law of survival of the fittest in the process of biological evolution and the mechanism of chromosome information exchange within the population. The best projection vector is obtained:
c∗=(p∗1,p∗2,⋯,p∗k,q∗1,q∗2,⋯,q∗m) | (8) |
where, w2=(p∗1,p∗2,⋯,p∗k) is the index weight vector; namely, the weight of index j is w2J=p∗J, and the time weight vector is q∗=(q∗1,q∗2,⋯,q∗m).
1. Calculate the comprehensive weight of index j
wj=12w1j+12w2j | (9) |
2. Calculate the TID value of object i in year a
Sai=12S1ai+12S2ai | (10) |
where, S1ai=∑kj=1w1jbaij, S2ai=z(i)∗, and z(i)∗ is the value obtained by substituting the best projection c∗ into the formula z(i). Sai refers to the TID value of object i in year a. The value combines the information of the diffusion source, diffusion path, receiver and diffusion environment of object i in year a, reflecting the comprehensive situation of TID. At the same time, the data used in the calculation have been dimensionless, and the obtained values can be compared among different sectors and regions. The larger the value, the more prominent the TID of the analyzed object in the corresponding year.
Based on the sector and region data of China's manufacturing industry, combined with the above-established models, this section uses MATLAB to measure the TID. Firstly, the weight of each index can be obtained (see Table 3). The analysis of the weight of each indicator shows the relative importance of each indicator and the impact on TID.
Diffusion system | Dimension | Weight of each dimension | Indicator | Weight of each index | ||
Sector level | Region level | Sector level | Region level | |||
technological innovation diffusion | Diffusion source (Diffusion resources) | 0.4058 | 0.4710 | R & D personnel full-time equivalent | 0.0794 | 0.0915 |
R & D instruments & equipment expenditures | 0.0755 | 0.0944 | ||||
Industrial enterprises with R & D activities | 0.0781 | 0.1143 | ||||
Number of new product development projects | 0.0855 | 0.0805 | ||||
Number of effective invention patents | 0.0873 | 0.0903 | ||||
Diffusion path | 0.2421 | 0.2206 | Technology output amount of the technology market | 0.0712 | 0.0733 | |
Patent ownership transfer and licensing income | 0.0759 | 0.0639 | ||||
Sales income of new products | 0.0949 | 0.0833 | ||||
Diffusion receiver (Absorbing capacity) | 0.2219 | 0.1580 | Technology inflow amount in technology market | 0.0849 | 0.0633 | |
Technology digestion and absorption expenditure | 0.0583 | 0.0564 | ||||
Technological transformation expenditure | 0.0786 | 0.0382 | ||||
Diffusion environment (Diffusion speed) | 0.1303 | 0.1505 | Technology output growth rate | 0.0262 | 0.0617 | |
Patent licensing growth rate | 0.0453 | 0.0320 | ||||
New product sales growth rate | 0.0349 | 0.0470 | ||||
turnover rate of current assets | 0.0238 | 0.0099 | ||||
Dimension average weight | 0.2500 | 0.2500 | Index average weight | 0.0667 | 0.0667 |
As shown in Table 3, from the weights of the four dimensions of the diffusion system, we can see that the comprehensive weight of the diffusion source exceeds 40%, no matter at the sector level or the regional level, significantly exceeding the average weight and those of the other three dimensions, indicating that the richness of the diffusion resources plays a fundamental important role in the process of TID. The weight of the diffusion path is slightly lower than the average weight at both the sector and the region levels, but it also exceeds 22%, which is another crucial factor affecting the diffusion of technological innovation. The weight of the diffusion receiver is significantly lower than that of the diffusion source, which is equivalent to that of the diffusion path at the sector level, but significantly lower than that of the diffusion path at the region level, indicating that the importance of the absorption capacity of the receiver is still behind that of the diffusion source and diffusion path, and even more backward at the regional level. The comprehensive weight of the diffusion environment is the lowest in the four dimensions at both the sector and region levels, indicating that the diffusion environment has a relatively small impact on TID.
The weights of the specific indicators under each dimension are similar to the comprehensive weights of the four dimensions. The five indicators under the diffusion source dimension are significantly higher than other indicators, indicating that indicators of the diffusion source are the most important ones affecting TID. Of the three indicators under the diffusion path dimension, only one indicator is slightly below the average weight at the regional level, and the other indicators are all above the average weight, indicating that the diffusion path is also an important index affecting the diffusion effect. The effects of the three absorption capacity indicators of the diffusion receiver are differentiated. The weights of the three indicators at the sector level are equivalent to or above the average weight and significantly higher than those at the regional level. The four indicators under the diffusion environment dimension are all significantly lower than the weight of other dimensions.
The above index weight analysis shows that whether from the comprehensive weight of the four dimensions of the diffusion system or the weight of each specific indicator, the diffusion resource of the diffusion source is the most important factor affecting the TID; the diffusion path also has great influence; the role of the absorption capacity of the receiver is slightly less than that of the diffusion path, and it's significantly higher at the sector level; the impact of the diffusion environment is relatively small.
Based on the above-mentioned methods and industry data, this sub-section calculates the values of the TID in 27 sectors of the manufacturing industry from 2005 to 2018 using the MATLAB software. To facilitate analysis and discussion, this sub-section takes 27 sectors as the object and takes the TID values of each year as variables to conduct Q-type clustering, where the Euclidean square distance is adopted for the distance between variables, and the Sum of Squares of Deviations (Ward) method is adopted between categories. After cluster analysis, the 27 sectors are grouped into three categories according to the distance between samples (see Table 4 and Figure 2). Among them, the distance between samples within each category is relatively small, and the distance between samples of different categories is relatively large.
Category | Code | Sector | Category | Code | Sector |
The first | Z23 | Transportation equipment | The third | Z2 | Food manufacturing |
manufacturing | Z3 | Wine, beverage, and refined tea | |||
Z24 | Electrical machinery and equipment | manufacturing sector | |||
manufacturing | Z4 | Tobacco sector | |||
Z25 | Computers, communications, and | Z6 | Textile and clothing sector | ||
other electronic equipment | Z7 | Leather, fur, feathers and their | |||
manufacturing | products and shoe-making sector | ||||
The second | Z1 | Agricultural and sideline food | Z8 | Wood processing and wood, | |
processing sector | bamboo, rattan, brown, grass | ||||
Z5 | Textile sector | products sector | |||
Z14 | Chemical raw materials and chemical | Z9 | Furniture manufacturing | ||
products manufacturing sector | Z10 | Paper making and paper products | |||
Z15 | Pharmaceutical manufacturing | sector | |||
Z17 | Rubber and Plastic Products sector | Z11 | Printing industry and recording | ||
Z18 | Ferrous metal smelting and rolling | media replication sector | |||
processing sector | Z12 | Culture, education, industrial | |||
Z19 | Non-ferrous metal smelting and | beauty, sports and entertainment | |||
rolling processing sector | goods manufacturing sector | ||||
Z20 | Metal products sector | Z13 | Oil processing, coking, and nuclear | ||
Z21 | General equipment manufacturing | fuel processing sector | |||
Z22 | Special equipment manufacturing | Z16 | Chemical fiber manufacturing | ||
Z26 | Instrument manufacturing | Z27 | Other manufacturing sector |
It can be seen from Figure 2 that the total amount of innovation diffusion in China's manufacturing industry showed a rapid rise during the research period, increasing from 2.09 in 2005 to 7.73 in 2018, an increase of 2.70 times. In terms of the total amount, the second category contributes the largest diffusion, followed by the first category, and the third category has the smallest contribution. From the perspective of the average, the average of all sectors shows a relatively stable growth trend; the average of the first category shows a fluctuating growth trend; the second category also has a relatively obvious growth trend, while the third category grows slightly with little change. In terms of the time trend, both the total amount and the average amount can be roughly divided into three development stages: 2005–2010, 2011–2015, 2016 and later. From 2005 to 2010, the total amount and the average value increased steadily; From 2011 to 2015, the total amount had a noticeable jump, while the average diffusion values of the first and second categories showed inevitable fluctuations, especially the first category; Since 2016, both the total amount and the average values increase steadily at a high level. Due to the different number of sectors contained in the three categories, there are great differences in the diffusion characteristics.
The first category has the characteristics of high technological level and large diffusion amount. This category includes three sectors: transportation equipment manufacturing, electrical machinery and equipment manufacturing, and computers, communications, and other electronic equipment manufacturing. Due to the prominent spillover effect, absorption effect, and diffusion speed, their TID presents a continuous rapid rise trend over time. These sectors have the characteristics of high technological level and high diffusion amount. They are technology-intensive sectors, with many fields in the industry leading the world in terms of the technological level, such as high-speed railway, power facilities, and 5G network equipment. Sectors in this category also have the largest technological diffusion during the sample period. The calculation results show that the average diffusion of this category increased from 0.1632 in 2005 to 0.9440 in 2018, with an increase of 4.78 times and an annual growth rate of 14.45%. In 2018, the diffusion of technological innovation of the three sectors in this category ranged from 0.81-1.19, much higher than other categories. Most of these sectors belong to technology-intensive industries with high technological innovation levels and strong penetration ability to other industries, so the TID grows rapidly.
The second category has the characteristics of moderate technological level and diffusion amount. This category includes 11 sectors (see Table 4 for details), including traditional sectors such as agricultural and sideline food processing and textile sectors and sectors with relatively high technological levels such as pharmaceutical manufacturing, general equipment manufacturing, and special equipment manufacturing. On the whole, such sectors are strongly related to sectors of the first category, but their scientific and technological innovation is significantly lower than those of the first category, and the TID is also relatively moderate. The calculation results show that the diffusion average of sectors in this category increased from 0.0829 in 2005 to 0.3336 in 2018, an increase of 3.02 times, with an average annual growth rate of 11.30%. In 2018, the TID of 11 sectors in this category was between 0.18 and 0.58, significantly lower than that of the first category but higher than that of the third category.
The third category has the characteristics of relatively low technological level and diffusion amount. This category includes 13 sectors (see Table 4 for details), which are mainly of the traditional light industry, such as food, beverage, textile shoes and hats, as well as resource mining sectors such as wood processing, oil mining and processing, having a low industrial association with sectors of the first category. These sectors all belong to the traditional industry in China with relatively low technological content, limited technological innovation development potential, and vulnerability to fluctuations in the economic environment, resulting in slow technological innovation. Mainly because they receive relatively low technological diffusion from other sectors.
On the whole, during the sample period, the TID of the manufacturing industry has formed a pattern in which sectors of the first category (transportation equipment manufacturing, electrical machinery and equipment manufacturing and communication equipment, computer and electronic equipment manufacturing) are taken as the diffusion source and gradually spread to the sectors of the second and third categories of according to the degree of industrial relevance, driving the overall improvement of the technological innovation level. The technological innovation level in the sectors of the second category is moderate, and there is a certain degree of diffusion while absorbing new technologies. Sectors in the third category mainly absorb the diffusion from other categories, and the degree of outward technology diffusion is low.
According to the data and index weights, the TID in 31 regions of China from 2005 to 2018 (except Hong Kong, Macao, and Taiwan) is calculated respectively by using the MATLAB software. To facilitate the analysis, this subsection takes 31 provinces as the object and takes the TID in each year from 2005 to 2018 as the variable for Q-type clustering, in which the distance between variables adopts Euclidean square distance, and the clustering between categories adopts the sum of squares of deviation (Ward) method. After clustering analysis, 31 provinces are divided into three categories according to the distance between samples (see Table 5 and Figure 3). Among them, the distance between samples within each category is relatively small, and the distance between samples of different categories is relatively large.
Category | Code | Province or municipality | Category | Code | Province or municipality |
The first | P1 | Jiangsu | The second | P17 | Sichuan |
P2 | Zhejiang | P18 | Shaanxi | ||
P3 | Shandong | The third | P19 | Shanxi | |
P4 | Guangdong | P20 | Inner Mongolia | ||
The second | P5 | Beijing | P21 | Jilin | |
P6 | Tianjin | P22 | Heilongjiang | ||
P7 | Hebei | P23 | Guangxi | ||
P8 | Liaoning | P24 | Hainan | ||
P9 | Shanghai | P25 | Guizhou | ||
P10 | Anhui | P26 | Yunnan | ||
P11 | Fujian | P27 | Xizang | ||
P12 | Jiangxi | P28 | Gansu | ||
P13 | Henan | P29 | Qinghai | ||
P14 | Hubei | P30 | Ningxia | ||
P15 | Hunan | P31 | Xinjiang | ||
P16 | Chongqing |
As can be seen from Figure 3, the total amount of TID in 31 provinces (municipalities) increased from 1.67 in 2005 to 7.54 in 2018, an increase of 3.52 times, with an average annual growth of 12.31%. The diffusion gap among the three categories of provinces is large. The growth trend of the provinces in the first and second categories is obvious, while that of the provinces in the third category is relatively flat, with little change during the sample period. In terms of the average, the average of the first category is significantly higher than the second and third categories; the second category is comparable to the national average, while the third category is the lowest and has little change during the sample period. Due to the differences in the number of provinces included in the three categories, there are also significant differences in diffusion characteristics.
Provinces in the first category have the characteristics of rapid growth in technological diffusion. They have a high diffusion of manufacturing technological innovation and a rapid growth rate. There are four provinces in this category, including Jiangsu, Zhejiang, Shandong, and Guangdong. The total amount of diffusion increased from 0.49 in 2005 to 3.59 in 2018, increasing 6.31 times, with an average annual growth of 16.54%. In 2018, the diffusion of various provinces was between 0.49 and 1.22, with the highest being Guangdong 1.22 and the lowest being Shandong 0.49. The four provinces are far higher than the others in total amount, average, and growth rate of diffusion. They have developed economies and technology, high degrees of innovation, and active innovation activities, and they are the main output and export places of high-tech products in China. The diffusion of their technological innovation has played a leading and driving role in the development of science and technology in other regions.
Provinces in the second category have the characteristics of steady growth. This category includes 14 provinces, consisting of 4 municipalities directly under the central government (Beijing, Shanghai, Tianjin, Chongqing)2, 3 coastal provinces (Liaoning, Hebei, Fujian), 5 Yangtze River Delta and central provinces (Anhui, Jiangxi, Henan, Hubei, Hunan), and 2 western-central provinces (Sichuan, Shaanxi). The total amount of diffusion in these provinces increased from 0.80 in 2005 to 3.25 in 2018, increasing 3.06 times, with an average annual growth of 11.39%. In 2018, the diffusion of these provinces ranged from 0.15 to 0.36, with Shanghai being the highest and Shaanxi the lowest. These provinces have good economic strength, a good technological innovation environment, and location conditions. Although there are certain fluctuations in the diffusion growth rate of manufacturing technological innovation, the overall trend is stable. These provinces also include two first-tier cities, Beijing and Shanghai. In terms of the city, they have the strongest strength of science and technology innovation, but compared with the four provinces in the first category, they are still slightly smaller, so they are classified into the second category, but both the total amount and speed of diffusion are significantly higher than those similar provinces. The performance of Anhui, Hubei, Hunan, and other provinces is also very eye-catching. These provinces are located in the Yangtze River Basin, around the scientific and technological innovation center cities such as Shanghai and Wuhan, so the level and diffusion of technological innovation are very prominent. In addition, it should be noted that as a municipality directly under the central government, Tianjin's total diffusion increased significantly in 2011 and peaked in 2014, but then began to decline slowly, and the decline was very obvious after 2016. In the context of the integration of Beijing, Tianjin, and Hebei, enough attention should be paid to how to identify the development orientation of Tianjin and reverse the negative trend in future development.
2 From the perspective of cities, Shanghai and Beijing are undoubtedly the cities with the strongest economic and technological innovation in China, but they are also provincial-level units themselves. From a provincial perspective, Jiangsu, Zhejiang, Shandong, and Guangdong are more extensive and contain more cities. Therefore, the total amount of technological innovation and diffusion in Shanghai and Beijing still lags behind that in the above-mentioned provinces and falls into the second category.
Provinces in the third category have the characteristics of slow development. This category includes 13 provinces, including Shanxi, Inner Mongolia, Jilin, Heilongjiang, Guangxi, Hainan, Guizhou, Yunnan, Tibet, Gansu, Qinghai, Ningxia, and Xinjiang, all of which are in western China except Hainan. The total amount of diffusion in these provinces increased from 0.37 in 2005 to 0.69 in 2018, increasing 0.84 times, with an average annual growth of 4.82%. In 2018, the diffusion of each province ranged from 0.024 to 0.087, far lower than those of the above two categories. As the only eastern province, Hainan's industrial structure is mainly dominated by tertiary industries such as agriculture and tourism, and the manufacturing industry is not developed, so the situation of TID is poor. Other provinces are located in the inland areas of Western China, with a normal economic development level, weak industrial foundation, low level of technological innovation, and slow diffusion speed.
In general, the TID in China's manufacturing industry has formed a pattern with Guangdong, Jiangsu, Zhejiang, Shandong, Beijing, and Shanghai as the sources of diffusion, spreading to the second-category provinces and regions, and then further to the third-category provinces, which leads to the overall improvement of the technological level. As the source of diffusion, all provinces are in the eastern coastal areas, with the most developed economy and science and technology in China. In terms of the total amount and speed of TID, it also reflects the characteristics that the above diffusion source provinces are the highest, the eastern and central regions and western core provinces are the second, and the western region is the worst.
The measurement and analysis of the TID in the manufacturing industry are conducive to understanding the diffusion situation and distribution characteristics of technological innovation in various sectors and regions in China so as to provide countermeasures and suggestions for improving the overall scientific and technological innovation strength of China's manufacturing industry. Based on the existing research, this paper constructs the index system of the TID in the manufacturing industry from the perspective of absorption ability and applies the entropy method combined with the PP-IPM method to measure the technological diffusion of 27 manufacturing sectors and 31 provinces from 2005 to 2018, obtaining the following conclusions and implications:
First, the TID in the manufacturing industry can be measured from four dimensions: diffusion source, diffusion path, diffusion receiver (absorption capacity), and diffusion environment. Among them, the diffusion resources of the diffusion source mainly reflect the ability of the innovation subject to diffuse new technologies, while the absorption ability of the diffusion receiver reflects the degree of innovation subject digesting and absorbing new technologies. The measurement system with the two as the core, supplemented by the diffusion path and the diffusion environment, can fully reflect the TID system. According to the calculation results, the weights of the four dimensions are sorted from large to small as diffusion source (diffusion resource), diffusion path, diffusion receiver (absorption capacity), and diffusion environment.
Second, in terms of specific sectors, during the sample period, a pattern has formed in which sectors like the transportation equipment manufacturing, the electrical machinery and equipment manufacturing and equipment and communication equipment, and the computer and electronic equipment manufacturing are the diffusion sources, which gradually diffuse to sectors of the second and the third categories according to the industry correlation degree, driving the overall improvement of the technological innovation level. Sectors of the second category not only absorb new technologies but also diffuse to a certain extent. Sectors of the third category mainly absorb the diffusion from other categories, and the degree of outward technology diffusion is low. As the diffusion source, the sectors are capital and technology-intensive, with high technical level and strong market competitiveness, and they are also of the high-tech industry that China has focused on developing in recent years. Promoting the innovation level of these sectors, maintaining their competitive advantages, and then enhancing their diffusion ability are the core links to promote the technological innovation level of China's manufacturing industry.
Third, from the regional perspective, during the sample period, Guangdong, Jiangsu, Zhejiang, Shandong, Beijing and Shanghai are the sources of diffusion, successively diffuse technology to neighboring provinces and the Yangtze River basin provinces, and then further to other central and western provinces. As the sources of diffusion, these provinces and municipalities are the most developed economic and technological regions in China. The improvement of economic and technological strength is a prerequisite for the diffusion of technological innovation. Therefore, to further accelerate the reform and the opening up, promote the further development of the economy and science and technology of the coastal provinces, and enhance the technological innovation ability and diffusion ability are important ways to improve the science and technology level in other regions of China.
This research was funded by the Chinese National Funding of Social Sciences, grant number 18ATJ002 and 17BTJ016.
The authors declare no conflict of interest in this paper.
[1] | Cao Y, He D, Hong W, et al. (2017) The Statistic Measurement Study on the Comprehensive Competitiveness in Chinese Provincial Forestry Based on the Projection Pursuit Model. Forestry Econ 39: 75–80. |
[2] | Cao X, Zhang LP, Liu GW (2018) Research on Dynamic mechanisms of Innovation Diffusion and Its Simulation Based on the Social Network Structure. Oper Res Manage 27: 149–156. |
[3] | Cheng SH, Shen LH (2018) Spatio-temporal Evolution of Chinese Technology Spatial Diffusion Effect Based on Provincial Data. Econ Manage 32: 31–39. |
[4] |
Cohen WM, Levinthal DA (1990) Absorptive capacity: A new perspective on learning and innovation. Adm Sci Q 35: 128–152. doi: 10.2307/2393553
![]() |
[5] |
Cole SA, Fernando AN (2021) Mobileizing Agricultural Advice Technology Adoption Diffusion and Sustainability. Econ J 131: 192–219. doi: 10.1093/ej/ueaa084
![]() |
[6] | Comin D, Dmitriev M, Rossi-Hansberg E (2012) The spatial diffusion of technology. Nat Bur Econ Res. |
[7] |
Dechezleprêtre A, Neumayer E, Perkins R (2015) Environmental regulation and the cross-border diffusion of new technology: Evidence from automobile patents. Res Policy 44: 244–257. doi: 10.1016/j.respol.2014.07.017
![]() |
[8] |
Ertur C, Musolesi A (2017) Weak and Strong Cross-Sectional Dependence: A Panel Data Analysis of International Technology Diffusion. J Appl Econom 32: 477–503. doi: 10.1002/jae.2538
![]() |
[9] |
Friedman JH, Tukey JW (1974) A Projection Pursuit Algorithm for Exploratory Data Analysis. IEEE Trans Comput 23: 881–890. doi: 10.1109/T-C.1974.224051
![]() |
[10] |
Grafström J, Lindman Å (2017) Invention, innovation and diffusion in the European wind power sector. Technol Forecasting Soc Change 114: 179–191. doi: 10.1016/j.techfore.2016.08.008
![]() |
[11] |
Hacking N, Pearson P, Eames M (2019) Mapping innovation and diffusion of hydrogen fuel cell technologies: Evidence from the UK's hydrogen fuel cell technological innovation system, 1954–2012. Int J Hydrogen Energ 44: 29805–29848. doi: 10.1016/j.ijhydene.2019.09.137
![]() |
[12] | Hötte K (2020) How to accelerate green technology diffusion? Directed technological change in the presence of coevolving absorptive capacity. Energy Econ 85. |
[13] | Huang ZQ, Zhang GH (2010) On the Measurement Indicators of Innovation Diffusion and Knowledge Spillover-Patent Citation, Technology Licensing and Publication Citation. Sci Technol Progress Policy 27: 124–126. |
[14] |
Jan F, Bart V (2002) Technology-gaps, Innovation-diffusion and Transformation: An Evolutionary Interpretation. Res Policy 31: 1291–1304. doi: 10.1016/S0048-7333(02)00064-1
![]() |
[15] | Jin H, Li RJ (2018) Governance Performance Evaluation in Beijing-Tianjin-Hebei Region: Combination Evaluation Method. J Hebei Univ Technol 10: 7–12. |
[16] | Jin JL, Wang SJ, Wei YM (2004) Projection Pursuit Model for Dynamic Multiple Attribute Decision Problems. Chinese J Manage Sci 12: 64–67. |
[17] |
Laciana CE, Rovere SL (2011) Ising-like agent-based technology diffusion model: Adoption patterns vs. seeding strategies. Physica A 390: 1139–1149. doi: 10.1016/j.physa.2010.11.006
![]() |
[18] |
Lee H, Kim N, Kwak K, et al. (2016) Diffusion Patterns in Convergence among High-Technology Industries: A Co-Occurrence-Based Analysis of Newspaper Article Data. Sustainability 8: 1029. doi: 10.3390/su8101029
![]() |
[19] | Lv YB, Nie JW, Liu QS (2020) The Impact of Industrial Technology Grouping on Innovation Diffusion. Res Manage 41: 78–88. |
[20] |
Lyu Y, Liu Q, He B, et al. (2017) Structural embeddedness and innovation diffusion: the moderating role of industrial technology grouping. Scientometrics 111: 889–916. doi: 10.1007/s11192-017-2320-3
![]() |
[21] |
Nelson AJ (2009) Measuring knowledge spillovers: What patents, licenses and publications reveal about innovation diffusion. Res Policy 38: 994–1005. doi: 10.1016/j.respol.2009.01.023
![]() |
[22] | Rogers EM (2003) Diffusion of Innovations. Free Press. |
[23] | Santacreu AM (2019) International Technology Licensing, Intellectual Property Rights, and Tax Havens. FRB St. Louis Working Paper. |
[24] | Wang WD, Fergola P, Tenneriello C (2003) Innovation diffusion model in patch environment. Appl Math Comput 134: 51–67. |
[25] | Xu Z, Jiao XH, Zhu GL (2013) The technology diffusion of national heart cities and regional economic growth-taking Beijing and Shanghai as example. Sci Res Manage 34: 16–23. |
[26] | Yu DK, Peng J, Tu GP (2018) The Evaluation of Agricultural Technology Diffusion environment of China Regions. Math Pract Theory 48: 43–57. |
[27] |
Yu Q, Yu Z, Ma D (2020) A multiplex network perspective of innovation diffusion: An information-behavior framework. IEEE Access 8: 36427–36440. doi: 10.1109/ACCESS.2020.2975357
![]() |
1. | Zexia Zhao, Peiqiong Wang, The impact of managerial competence on corporate carbon performance: An empirical study based on Chinese heavy polluters, 2023, 11, 2296-598X, 10.3389/fenrg.2023.1130339 | |
2. | Lingfu Zhang, Yongfang Dou, Hailing Wang, Green supply chain management, risk-taking, and corporate value—Dual regulation effect based on technological innovation capability and supply chain concentration, 2023, 11, 2296-665X, 10.3389/fenvs.2023.1096349 | |
3. | Yanhong Feng, Qingqing Hu, Heterogeneity and spillover effects of carbon emission trading on green innovation, 2023, 20, 1551-0018, 6468, 10.3934/mbe.2023279 | |
4. | Yue Liu, Chunying Ma, Zhehao Huang, Can the digital economy improve green total factor productivity? An empirical study based on Chinese urban data, 2023, 20, 1551-0018, 6866, 10.3934/mbe.2023296 | |
5. | Shiwen Fu, Jianguo Yuan, Deyun Xiao, Zhiqiang Chen, Gaorong Yang, Research on environmental regulation, environmental protection tax, and earnings management, 2023, 11, 2296-665X, 10.3389/fenvs.2023.1085144 | |
6. | Li Xu, Ping Guo, Guoqin Pan, Effects of inter-industry agglomeration on environmental pollution: Evidence from China, 2023, 20, 1551-0018, 7113, 10.3934/mbe.2023307 | |
7. | Yue Liu, Jinzhi Liu, Lichang Zhang, Enterprise financialization and R&D innovation: A case study of listed companies in China, 2023, 31, 2688-1594, 2447, 10.3934/era.2023124 | |
8. | Xinmiao Zhou, Hongyuan Lu, Shengchao Ye, The information transmission and risk contagion effect between green bond market and government bond market in China, 2023, 11, 2296-665X, 10.3389/fenvs.2023.1091203 | |
9. | Shucheng Liu, Peijin Wu, The impact of high-tech industrial agglomeration on China’s green innovation efficiency: A spatial econometric analysis, 2023, 11, 2296-665X, 10.3389/fenvs.2023.1167918 | |
10. | Huwei Wen, Jiayi Shi, Peng Lu, Can Green Technology Innovation Reduce the Operational Risks of Energy-Intensive Enterprises?, 2023, 11, 2079-8954, 194, 10.3390/systems11040194 | |
11. | Shen Zhong, Yu’an Fang, The Impact of Electricity Consumption on Green Total Factor Productivity: Evidence from Chinese Provinces, 2024, 1868-7873, 10.1007/s13132-024-01949-2 | |
12. | Kingsley E. Dogah, Presley K. Wesseh, Samuel Adomako, Green credit policy, technological innovation, and corporate financial performance: Evidence from the energy industry, 2024, 0964-4733, 10.1002/bse.4041 | |
13. | Ping Zhou, Jin Xiang, Decai Tang, Jiannan Li, Bright Obuobi, María del Carmen Valls Martínez, Intelligence level evaluation and influencing factors analysis of equipment manufacturing industry in the Yangtze River Delta, 2024, 19, 1932-6203, e0299119, 10.1371/journal.pone.0299119 | |
14. | Yue Liu, Liming Chen, Han Luo, Yuzhao Liu, Yixian Wen, The impact of intellectual property rights protection on green innovation: A quasi-natural experiment based on the pilot policy of the Chinese intellectual property court, 2024, 21, 1551-0018, 2587, 10.3934/mbe.2024114 | |
15. | Xiaoli Hao, Shufang Wen, Jianing Zhu, Haitao Wu, Yu Hao, Can business managerial capacity improve green innovation in different industries? Evidence from Chinese listed companies, 2024, 33, 0964-4733, 2600, 10.1002/bse.3600 |
Diffusion system | Dimension | Indicator | Unit |
Technological innovation diffusion (TID) | Diffusion source (Diffusion resources) | R & D personnel full-time equivalent | person-year |
R & D instruments & equipment expenditures | 10,000 yuan | ||
Industrial enterprises with R & D activities | Unit | ||
Number of new product development projects | Item | ||
Number of effective invention patents | Piece | ||
Diffusion path | Technology output amount of the technology market | 10,000 yuan | |
Patent ownership transfer and licensing income | 10,000 yuan | ||
Sales income of new products | 10,000 yuan | ||
Diffusion receiver (Absorption capacity) | Technology inflow amount in the technology market | 10,000 yuan | |
Technology digestion and absorption expenditure | 10,000 yuan | ||
Technological transformation expenditure | 10,000 yuan | ||
Diffusion environment | Technology output growth rate | % | |
Patent licensing growth rate | % | ||
New product sales growth rate | % | ||
the turnover rate of current assets | % |
Order number | Sector | Code in this study | Order number | Sector | Code in this study |
1 | Agricultural and sideline food | Z1 | 15 | Pharmaceutical manufacturing | Z15 |
processing sector | 16 | Chemical fiber manufacturing | Z16 | ||
2 | Food manufacturing | Z2 | 17 | Rubber and Plastic Products | Z17 |
3 | Wine, beverage, and refined tea | Z3 | sector | ||
manufacturing sector | 18 | Ferrous metal smelting and | Z18 | ||
4 | Tobacco sector | Z4 | rolling processing sector | ||
5 | Textile sector | Z5 | 19 | Non-ferrous metal smelting and | Z19 |
6 | Textile and clothing sector | Z6 | rolling processing sector | ||
7 | Leather, fur, feathers and their | Z7 | 20 | Metal products sector | Z20 |
products and shoe-making sector | 21 | General equipment | Z21 | ||
8 | Wood processing and wood, | Z8 | manufacturing | ||
bamboo, rattan, brown, grass | 22 | Special equipment | Z22 | ||
products sector | manufacturing | ||||
9 | Furniture manufacturing | Z9 | 23 | Transportation equipment | Z23 |
10 | Paper making and paper products | Z10 | manufacturing | ||
sector | 24 | Electrical machinery and | Z24 | ||
11 | Printing industry and recording | Z11 | equipment manufacturing | ||
media replication sector | 25 | Computers, communications, | Z25 | ||
12 | Culture, education, industrial | Z12 | and other electronic equipment | ||
beauty, sports and entertainment | manufacturing sector | ||||
goods manufacturing sector | 26 | Instrument manufacturing | Z26 | ||
13 | Oil processing, coking, and nuclear | Z13 | 27 | Other manufacturing sector | Z27 |
fuel processing sector | |||||
14 | Chemical raw materials and | Z14 | |||
chemical products manufacturing | |||||
sector |
Diffusion system | Dimension | Weight of each dimension | Indicator | Weight of each index | ||
Sector level | Region level | Sector level | Region level | |||
technological innovation diffusion | Diffusion source (Diffusion resources) | 0.4058 | 0.4710 | R & D personnel full-time equivalent | 0.0794 | 0.0915 |
R & D instruments & equipment expenditures | 0.0755 | 0.0944 | ||||
Industrial enterprises with R & D activities | 0.0781 | 0.1143 | ||||
Number of new product development projects | 0.0855 | 0.0805 | ||||
Number of effective invention patents | 0.0873 | 0.0903 | ||||
Diffusion path | 0.2421 | 0.2206 | Technology output amount of the technology market | 0.0712 | 0.0733 | |
Patent ownership transfer and licensing income | 0.0759 | 0.0639 | ||||
Sales income of new products | 0.0949 | 0.0833 | ||||
Diffusion receiver (Absorbing capacity) | 0.2219 | 0.1580 | Technology inflow amount in technology market | 0.0849 | 0.0633 | |
Technology digestion and absorption expenditure | 0.0583 | 0.0564 | ||||
Technological transformation expenditure | 0.0786 | 0.0382 | ||||
Diffusion environment (Diffusion speed) | 0.1303 | 0.1505 | Technology output growth rate | 0.0262 | 0.0617 | |
Patent licensing growth rate | 0.0453 | 0.0320 | ||||
New product sales growth rate | 0.0349 | 0.0470 | ||||
turnover rate of current assets | 0.0238 | 0.0099 | ||||
Dimension average weight | 0.2500 | 0.2500 | Index average weight | 0.0667 | 0.0667 |
Category | Code | Sector | Category | Code | Sector |
The first | Z23 | Transportation equipment | The third | Z2 | Food manufacturing |
manufacturing | Z3 | Wine, beverage, and refined tea | |||
Z24 | Electrical machinery and equipment | manufacturing sector | |||
manufacturing | Z4 | Tobacco sector | |||
Z25 | Computers, communications, and | Z6 | Textile and clothing sector | ||
other electronic equipment | Z7 | Leather, fur, feathers and their | |||
manufacturing | products and shoe-making sector | ||||
The second | Z1 | Agricultural and sideline food | Z8 | Wood processing and wood, | |
processing sector | bamboo, rattan, brown, grass | ||||
Z5 | Textile sector | products sector | |||
Z14 | Chemical raw materials and chemical | Z9 | Furniture manufacturing | ||
products manufacturing sector | Z10 | Paper making and paper products | |||
Z15 | Pharmaceutical manufacturing | sector | |||
Z17 | Rubber and Plastic Products sector | Z11 | Printing industry and recording | ||
Z18 | Ferrous metal smelting and rolling | media replication sector | |||
processing sector | Z12 | Culture, education, industrial | |||
Z19 | Non-ferrous metal smelting and | beauty, sports and entertainment | |||
rolling processing sector | goods manufacturing sector | ||||
Z20 | Metal products sector | Z13 | Oil processing, coking, and nuclear | ||
Z21 | General equipment manufacturing | fuel processing sector | |||
Z22 | Special equipment manufacturing | Z16 | Chemical fiber manufacturing | ||
Z26 | Instrument manufacturing | Z27 | Other manufacturing sector |
Category | Code | Province or municipality | Category | Code | Province or municipality |
The first | P1 | Jiangsu | The second | P17 | Sichuan |
P2 | Zhejiang | P18 | Shaanxi | ||
P3 | Shandong | The third | P19 | Shanxi | |
P4 | Guangdong | P20 | Inner Mongolia | ||
The second | P5 | Beijing | P21 | Jilin | |
P6 | Tianjin | P22 | Heilongjiang | ||
P7 | Hebei | P23 | Guangxi | ||
P8 | Liaoning | P24 | Hainan | ||
P9 | Shanghai | P25 | Guizhou | ||
P10 | Anhui | P26 | Yunnan | ||
P11 | Fujian | P27 | Xizang | ||
P12 | Jiangxi | P28 | Gansu | ||
P13 | Henan | P29 | Qinghai | ||
P14 | Hubei | P30 | Ningxia | ||
P15 | Hunan | P31 | Xinjiang | ||
P16 | Chongqing |
Diffusion system | Dimension | Indicator | Unit |
Technological innovation diffusion (TID) | Diffusion source (Diffusion resources) | R & D personnel full-time equivalent | person-year |
R & D instruments & equipment expenditures | 10,000 yuan | ||
Industrial enterprises with R & D activities | Unit | ||
Number of new product development projects | Item | ||
Number of effective invention patents | Piece | ||
Diffusion path | Technology output amount of the technology market | 10,000 yuan | |
Patent ownership transfer and licensing income | 10,000 yuan | ||
Sales income of new products | 10,000 yuan | ||
Diffusion receiver (Absorption capacity) | Technology inflow amount in the technology market | 10,000 yuan | |
Technology digestion and absorption expenditure | 10,000 yuan | ||
Technological transformation expenditure | 10,000 yuan | ||
Diffusion environment | Technology output growth rate | % | |
Patent licensing growth rate | % | ||
New product sales growth rate | % | ||
the turnover rate of current assets | % |
Order number | Sector | Code in this study | Order number | Sector | Code in this study |
1 | Agricultural and sideline food | Z1 | 15 | Pharmaceutical manufacturing | Z15 |
processing sector | 16 | Chemical fiber manufacturing | Z16 | ||
2 | Food manufacturing | Z2 | 17 | Rubber and Plastic Products | Z17 |
3 | Wine, beverage, and refined tea | Z3 | sector | ||
manufacturing sector | 18 | Ferrous metal smelting and | Z18 | ||
4 | Tobacco sector | Z4 | rolling processing sector | ||
5 | Textile sector | Z5 | 19 | Non-ferrous metal smelting and | Z19 |
6 | Textile and clothing sector | Z6 | rolling processing sector | ||
7 | Leather, fur, feathers and their | Z7 | 20 | Metal products sector | Z20 |
products and shoe-making sector | 21 | General equipment | Z21 | ||
8 | Wood processing and wood, | Z8 | manufacturing | ||
bamboo, rattan, brown, grass | 22 | Special equipment | Z22 | ||
products sector | manufacturing | ||||
9 | Furniture manufacturing | Z9 | 23 | Transportation equipment | Z23 |
10 | Paper making and paper products | Z10 | manufacturing | ||
sector | 24 | Electrical machinery and | Z24 | ||
11 | Printing industry and recording | Z11 | equipment manufacturing | ||
media replication sector | 25 | Computers, communications, | Z25 | ||
12 | Culture, education, industrial | Z12 | and other electronic equipment | ||
beauty, sports and entertainment | manufacturing sector | ||||
goods manufacturing sector | 26 | Instrument manufacturing | Z26 | ||
13 | Oil processing, coking, and nuclear | Z13 | 27 | Other manufacturing sector | Z27 |
fuel processing sector | |||||
14 | Chemical raw materials and | Z14 | |||
chemical products manufacturing | |||||
sector |
Diffusion system | Dimension | Weight of each dimension | Indicator | Weight of each index | ||
Sector level | Region level | Sector level | Region level | |||
technological innovation diffusion | Diffusion source (Diffusion resources) | 0.4058 | 0.4710 | R & D personnel full-time equivalent | 0.0794 | 0.0915 |
R & D instruments & equipment expenditures | 0.0755 | 0.0944 | ||||
Industrial enterprises with R & D activities | 0.0781 | 0.1143 | ||||
Number of new product development projects | 0.0855 | 0.0805 | ||||
Number of effective invention patents | 0.0873 | 0.0903 | ||||
Diffusion path | 0.2421 | 0.2206 | Technology output amount of the technology market | 0.0712 | 0.0733 | |
Patent ownership transfer and licensing income | 0.0759 | 0.0639 | ||||
Sales income of new products | 0.0949 | 0.0833 | ||||
Diffusion receiver (Absorbing capacity) | 0.2219 | 0.1580 | Technology inflow amount in technology market | 0.0849 | 0.0633 | |
Technology digestion and absorption expenditure | 0.0583 | 0.0564 | ||||
Technological transformation expenditure | 0.0786 | 0.0382 | ||||
Diffusion environment (Diffusion speed) | 0.1303 | 0.1505 | Technology output growth rate | 0.0262 | 0.0617 | |
Patent licensing growth rate | 0.0453 | 0.0320 | ||||
New product sales growth rate | 0.0349 | 0.0470 | ||||
turnover rate of current assets | 0.0238 | 0.0099 | ||||
Dimension average weight | 0.2500 | 0.2500 | Index average weight | 0.0667 | 0.0667 |
Category | Code | Sector | Category | Code | Sector |
The first | Z23 | Transportation equipment | The third | Z2 | Food manufacturing |
manufacturing | Z3 | Wine, beverage, and refined tea | |||
Z24 | Electrical machinery and equipment | manufacturing sector | |||
manufacturing | Z4 | Tobacco sector | |||
Z25 | Computers, communications, and | Z6 | Textile and clothing sector | ||
other electronic equipment | Z7 | Leather, fur, feathers and their | |||
manufacturing | products and shoe-making sector | ||||
The second | Z1 | Agricultural and sideline food | Z8 | Wood processing and wood, | |
processing sector | bamboo, rattan, brown, grass | ||||
Z5 | Textile sector | products sector | |||
Z14 | Chemical raw materials and chemical | Z9 | Furniture manufacturing | ||
products manufacturing sector | Z10 | Paper making and paper products | |||
Z15 | Pharmaceutical manufacturing | sector | |||
Z17 | Rubber and Plastic Products sector | Z11 | Printing industry and recording | ||
Z18 | Ferrous metal smelting and rolling | media replication sector | |||
processing sector | Z12 | Culture, education, industrial | |||
Z19 | Non-ferrous metal smelting and | beauty, sports and entertainment | |||
rolling processing sector | goods manufacturing sector | ||||
Z20 | Metal products sector | Z13 | Oil processing, coking, and nuclear | ||
Z21 | General equipment manufacturing | fuel processing sector | |||
Z22 | Special equipment manufacturing | Z16 | Chemical fiber manufacturing | ||
Z26 | Instrument manufacturing | Z27 | Other manufacturing sector |
Category | Code | Province or municipality | Category | Code | Province or municipality |
The first | P1 | Jiangsu | The second | P17 | Sichuan |
P2 | Zhejiang | P18 | Shaanxi | ||
P3 | Shandong | The third | P19 | Shanxi | |
P4 | Guangdong | P20 | Inner Mongolia | ||
The second | P5 | Beijing | P21 | Jilin | |
P6 | Tianjin | P22 | Heilongjiang | ||
P7 | Hebei | P23 | Guangxi | ||
P8 | Liaoning | P24 | Hainan | ||
P9 | Shanghai | P25 | Guizhou | ||
P10 | Anhui | P26 | Yunnan | ||
P11 | Fujian | P27 | Xizang | ||
P12 | Jiangxi | P28 | Gansu | ||
P13 | Henan | P29 | Qinghai | ||
P14 | Hubei | P30 | Ningxia | ||
P15 | Hunan | P31 | Xinjiang | ||
P16 | Chongqing |