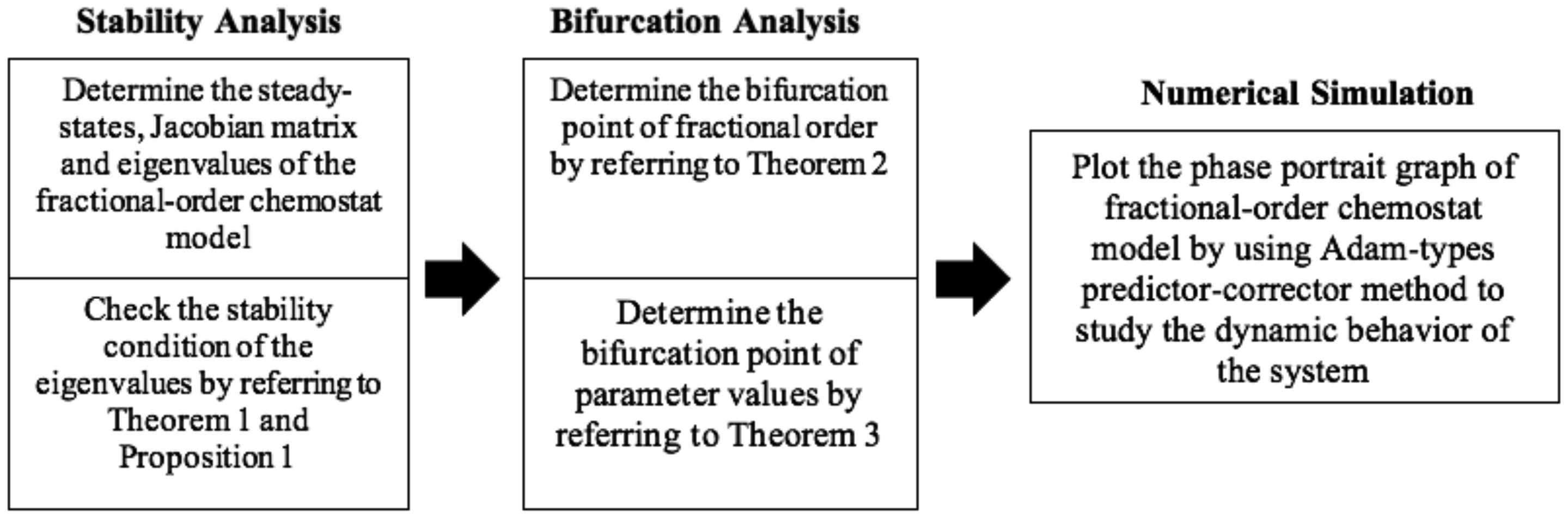
The continuous increase of energy consumption resulted in the unavoidable increase in demand for renewable energy (RE) investment projects in recent years. Although the necessity of developing alternative energy sources is clear, the government cannot afford the huge investment in RE investment projects without private sector participation. Therefore, analyzing the decision-making procedure from the investor's point of view is essential to improve this process. Numerous studies in the literature developed various multi-criteria decision-making approaches using expert's judgments to provide informed decisions on RE investment projects. While prior efforts are valuable, accounting heterogeneity impact with regard to experts' background and knowledge on results has not been examined. Therefore, this study aims to develop a modified decision-making approach in RE projects using an analytical hierarchy process to: (1) provide a comprehensive review of investors criteria in RE projects; (2) evaluate how the level of expertise of experts in RE subject has an impact on the achieved common solution; and (3) determine the best RE alternative in different scenarios. Then, Iran, as a case study is selected to illustrate the model practicability. The results indicate that those who have higher expertise in the subject are more concerned about the "consumption market", and "government supportive policies". Whereas economic factors remain the most challenging criteria in less expert participation views. Both groups chose 'wind energy' as the best alternative energy source for investment based on current Iran's energy market. It is anticipated that the developed methodology and its results can be used by (1) government and public agencies to understand the investors' concerns; (2) investors to make a more-informed risk-based decision in RE projects or other complex decision-making projects.
Citation: Abdolmajid Erfani, Mehdi Tavakolan, Ali Hassandokht Mashhadi, Pouria Mohammadi. Heterogeneous or homogeneous? A modified decision-making approach in renewable energy investment projects[J]. AIMS Energy, 2021, 9(3): 558-580. doi: 10.3934/energy.2021027
[1] | Shaimaa A. M. Abdelmohsen, D. Sh. Mohamed, Haifa A. Alyousef, M. R. Gorji, Amr M. S. Mahdy . Mathematical modeling for solving fractional model cancer bosom malignant growth. AIMS Biophysics, 2023, 10(3): 263-280. doi: 10.3934/biophy.2023018 |
[2] | Mati ur Rahman, Mehmet Yavuz, Muhammad Arfan, Adnan Sami . Theoretical and numerical investigation of a modified ABC fractional operator for the spread of polio under the effect of vaccination. AIMS Biophysics, 2024, 11(1): 97-120. doi: 10.3934/biophy.2024007 |
[3] | Yasir Nadeem Anjam, Mehmet Yavuz, Mati ur Rahman, Amna Batool . Analysis of a fractional pollution model in a system of three interconnecting lakes. AIMS Biophysics, 2023, 10(2): 220-240. doi: 10.3934/biophy.2023014 |
[4] | Marco Menale, Bruno Carbonaro . The mathematical analysis towards the dependence on the initial data for a discrete thermostatted kinetic framework for biological systems composed of interacting entities. AIMS Biophysics, 2020, 7(3): 204-218. doi: 10.3934/biophy.2020016 |
[5] | Mehmet Yavuz, Kübra Akyüz, Naime Büşra Bayraktar, Feyza Nur Özdemir . Hepatitis-B disease modelling of fractional order and parameter calibration using real data from the USA. AIMS Biophysics, 2024, 11(3): 378-402. doi: 10.3934/biophy.2024021 |
[6] | Larisa A. Krasnobaeva, Ludmila V. Yakushevich . On the dimensionless model of the transcription bubble dynamics. AIMS Biophysics, 2023, 10(2): 205-219. doi: 10.3934/biophy.2023013 |
[7] | Carlo Bianca . Differential equations frameworks and models for the physics of biological systems. AIMS Biophysics, 2024, 11(2): 234-238. doi: 10.3934/biophy.2024013 |
[8] | Mohammed Alabedalhadi, Mohammed Shqair, Ibrahim Saleh . Analysis and analytical simulation for a biophysical fractional diffusive cancer model with virotherapy using the Caputo operator. AIMS Biophysics, 2023, 10(4): 503-522. doi: 10.3934/biophy.2023028 |
[9] | Bertrand R. Caré, Pierre-Emmanuel Emeriau, Ruggero Cortini, Jean-Marc Victor . Chromatin epigenomic domain folding: size matters. AIMS Biophysics, 2015, 2(4): 517-530. doi: 10.3934/biophy.2015.4.517 |
[10] | David Gosselin, Maxime Huet, Myriam Cubizolles, David Rabaud, Naceur Belgacem, Didier Chaussy, Jean Berthier . Viscoelastic capillary flow: the case of whole blood. AIMS Biophysics, 2016, 3(3): 340-357. doi: 10.3934/biophy.2016.3.340 |
The continuous increase of energy consumption resulted in the unavoidable increase in demand for renewable energy (RE) investment projects in recent years. Although the necessity of developing alternative energy sources is clear, the government cannot afford the huge investment in RE investment projects without private sector participation. Therefore, analyzing the decision-making procedure from the investor's point of view is essential to improve this process. Numerous studies in the literature developed various multi-criteria decision-making approaches using expert's judgments to provide informed decisions on RE investment projects. While prior efforts are valuable, accounting heterogeneity impact with regard to experts' background and knowledge on results has not been examined. Therefore, this study aims to develop a modified decision-making approach in RE projects using an analytical hierarchy process to: (1) provide a comprehensive review of investors criteria in RE projects; (2) evaluate how the level of expertise of experts in RE subject has an impact on the achieved common solution; and (3) determine the best RE alternative in different scenarios. Then, Iran, as a case study is selected to illustrate the model practicability. The results indicate that those who have higher expertise in the subject are more concerned about the "consumption market", and "government supportive policies". Whereas economic factors remain the most challenging criteria in less expert participation views. Both groups chose 'wind energy' as the best alternative energy source for investment based on current Iran's energy market. It is anticipated that the developed methodology and its results can be used by (1) government and public agencies to understand the investors' concerns; (2) investors to make a more-informed risk-based decision in RE projects or other complex decision-making projects.
Numerous mathematical models have been established to predict and study the biological system. In the past four decades, there have been far-reaching research on improving cell mass production in chemical reactors [1]. The chemostat model is used to understand the mechanism of cell mass growth in a chemostat. A chemostat is an apparatus for continuous culture that contains bacterial populations. It can be used to investigate the cell mass production under controlled conditions. This reactor provides a dynamic system for population studies and is suitable to be used in a laboratory. A substrate is continuously added into the reactor containing the cell mass, which grows by consuming the substrate that enters through the inflow chamber. Meanwhile, the mixture of cell mass and substrate is continuously harvested from the reactor through the outflow chamber. The dynamics in the chemostat can be investigated by using the chemostat model [2].
Ordinary differential equations (ODEs) are commonly used for modelling biological systems. However, most biological systems behaviour has memory effects, and ODEs usually neglect such effects. The fractional-order differential equations (FDEs) are taken into account when describing the behaviour of the systems' equations. A FDEs is a generalisation of the ODEs to random nonlinear order [3]. This equation is more effective because of its good memory, among other advantages [4]–[8]. The errors occuring from the disregarded parameters when modelling of phenomena in real-life also can be reduced. FDEs are also used to efficiently replicate the real nature of various systems in the field of engineering and sciences [9]. In the past few decades, FDEs have been used in biological systems for various studies [5], [6], [10]–[17].
Since great strides in the study of FDEs have been developed, the dynamics in the chemostat can be investigated using the mathematical model of the chemostat in the form of FDEs. Moreover, there have been few studies on the expansion of the chemostat model with fractional-order theory. Thus, we deepen and complete the analysis on the integer-order chemostat model with fractional-order theory and discuss the stability of the equilibrium points of the fractional-order chemostat model. Next, the bifurcation analysis for the fractional-order chemostat model is conducted to identify the bifurcation point that can change the stability of the system. The analysis identifies the values of the fractional-order and the system parameters to ensure the operation of the chemostat is well-controlled.
Recently, there are many approaches to define fractional operators such as by Caputo, Riemann-Liouville, Hadamard, and Grunwald-Letnikov [3]. However, Caputo is often used due to its convenience in various applications [18]. Caputo is also useful to encounter an obstacle where the initial condition is done in the differential of integer-order [19]. In this paper, we applied Caputo derivative to define the system of fractional-order. The Caputo derivative for the left-hand side is defined as
where Г denotes the function of gamma, n is an integer, where
The Adams-type predictor-corrector method is one of the technique that have been proposed for fractional-order differential equations [19]–[21]. The Adams-type predictor-corrector method is a analysis of numerical algorithm that involves two basics steps: predictor and corrector. The predictor formula can be described as
meanwhile the corrector formula can be determined by
The predictor-corrector method is also called as the PECE (Predict, Evaluate, Correct, Evaluate) method [22]. The procedure of the predictor-corrector method can be explained as follows
(i) Calculate the predictor step,
(ii) Evaluate
(iii) Calculate the corrector step,
(iv) Evaluate
The procedure repeatedly predicts and corrects the value until the corrected value becomes a converged number [21]. This method is able to maintain the stability of the properties and has good accuracy. Moreover, this method also has lower computational cost than other methods [23]. The algorithm of the Adams-type predictor-corrector method proposed by [22] is shown in Appendix.
The conditions of stability for integer-order differential equations and fractional-order differential equations are different. Both systems could have the same steady-state points but different stability conditions [24], [25]. The stability condition for fractional-order differential equations can be stated by Theorem 1 and the Routh-Hurwitz stability condition as described by Proposition 1.
Theorem 1
Proposition 1
or
The stability theorem on the fractional-order systems and fractional Routh-Hurwitz stability conditions are introduced to analyze the stability of the model. The fractional Routh-Hurwitz stability conditions is specifically introduced for the eigenvalues of the Jacobian matrix that obtained in quadratic form. The proof of this proposition is shown in Appendix.
Bifurcation can be defined as any sudden change that occurs while a parameter value is varied in the differential equation system and it has a significant influence on the solution [27]. An unstable steady-state may becomes stable and vice versa. A slight changes in the parameter value may change the system's stability. Despite the steady-state point and the eigenvalues of the system of fractional-order are similar as the system of integer-order, the discriminant method used for the stability of the steady-state point is different. Accordingly, the Hopf bifurcation condition in the fractional-order system is slightly different as compared with the integer-order system.
Fractional order α can be selected as the bifurcation parameter in a fractional-order system, but this is not allowed in an integer-order system. The existence of Hopf bifurcation can be stated as in Theorem 2.
Theorem 2
(i) 1. The characteristic equation of chemostat system has a pair of complex conjugate roots,
(ii) Critical value
(iii)
Proof. Condition (i) is not easy to obtain due to the selected parameter's value. However, this condition can be managed under some confined conditions. In fact, the washout steady-state solution of the chemostat model has two negative real roots. The remaining two roots depend on the characteristic of the polynomial from the no-washout steady-state solution.
Condition (ii) can be satisfied with the existence of critical value α* and when
The integer system required p = 0 for the bifurcation's operating condition. For the fractional-order system, the operating condition of the system will change into
In studying the dynamic process of chemostat, the parameters such as
Theorem 3
(i) The characteristic equation of chemostat system has a pair of complex conjugate roots,
(ii) Critical value
(iii)
Proof. This theorem can be proved in the same way as Theorem 2. Therefore, condition (i) can be guaranteed. Condition (ii) can be satisfied with the existence of critical value
For condition (iii), the condition of
Firstly, determine the steady-states, Jacobian matrix and eigenvalues of the fractional-order chemostat model. The stability properties of the fractional-order chemostat model were estimated by using the stability and bifurcation analyses with FDEs by referring to Theorem 1 and Proposition 1. Then, determine the bifurcation point of fractional-order by referring to Theorem 2 and determine the bifurcation point of parameter values by referring to Theorem 3. Next, plot the phase portrait of fractional-order chemostat model by using Adam-types predictor-corrector method to study the dynamic behaviour of the system. Figure 1 depicts the flowchart of this research. This flowchart can be applied to all problems with suitable parameter values.
An integer-order chemostat model that considered a variable yield coefficient and the Monod growth model from [1] is studied in this section. The chemostat system can be written as
with the initial value of
Let Eq. (3.2) equal to zero in order to find the steady-state solutions
By solving Eq. (3.4), the following solutions are obtained
From
Hence, the solutions of steady-state for the chemostat model are
(i) Washout:
(ii) No Washout:
where
The steady-state solutions are physically meaningful if their components are positive. Therefore,
The solution of steady-state in Equation (3.8) represents the washout situation, where the cell mass is wholly removed from the reactor and where the substrate concentration is at the same stock as in the beginning. This state must always be unstable in order to ensure that the cell mass is able to grow in the chemostat. This is because the cell mass will be continuously removed from the chemostat if the washout steady-state is stable. The Jacobian matrix for the washout steady-state solution can be written as
The eigenvalues of this matrix are
The eigenvalues in
The eigenvalues of the Jacobian matrix in terms of the characteristic polynomial are
where
and
The eigenvalues of the no-washout steady-state solution were evaluated with Routh-Hurwitz condition in Proposition 1. Based on the eigenvalues in Eq. (3.15) and by referring to the study by [5], the eigenvalues' condition can be simplified as the following two cases
(i) If b>0 or equivalent to
(ii) If b<0 or equivalent to
Then, if
The parameter values of the fractional-order chemostat model are provided in Table 1. The initial substrate concentration, S0 and ρ were assumed as non-negative values to ensure that the steady-state solutions were physically meaningful. The stability diagram of the steady-state solutions is plotted in Figure 2.
Parameters | Description | Values | Units |
k | Saturation constant | 1.75 | gl−1 |
Q | Dilution rate | 0.02 | l2gr−1 |
µ | Maximum growth rate | 0.3 | h−1 |
γ | Constant in yield coefficient | 0.01 | – |
β | Constant in yield coefficient | 5.25 | lg−1 |
S0 | Input concentration of substrate | 1 | gl−1 |
The washout steady-state solution is stable if Q > 0 and
The steady-state solutions of the fractional-order chemostat model for the parameter values given in Table 1 are
(i) Washout:
(ii) No Washout:
The eigenvalues obtained from the washout steady-state solution are
and the eigenvalues from the no-washout steady-state solution are
Based on Eq. (3.22) to Eq. (3.25), these satisfied the first condition of Hopf bifurcation in Theorem 2. There exists a pair of complex conjugate roots and the other eigenvalues are negative real roots. The transversality condition as the third condition is also satisfied. The eigenvalues of the washout steady-state solution based on the chemostat system is not imaginary, and so there is no existence of Hopf bifurcation in the washout steady-state solution. According to Theorem 2, the critical value of the fractional-order as stated in the second condition can be obtained as
where
Value of p and q are obtained from
The running state of the fractional-order chemostat system when fractional order α at the Hopf bifurcation point is shown. The fractional-order chemostat system changed its stability once Hopf bifurcation occurred. Therefore, we conjecture that the system of fractional-order chemostat may be lost or gain its stability when the fractional order α is less than the Hopf bifurcation point, or
The initial concentration of the substrate, S0, was chosen as the control parameter, while fractional order α was fixed. The solutions of steady-state of the fractional-order chemostat model with S0 as the control parameter are
(i) Washout:
(ii) No Washout:
The eigenvalues obtained from the washout steady-state solution are
and the eigenvalues from the no-washout steady-state solution are
These satisfied the first condition of Hopf bifurcation in Theorem 3. There exist a pair of complex conjugate roots in terms of S0, and the other eigenvalues were negative real roots in terms of S0. The transversality condition as the third condition is also satisfied. According to Theorem 3, the critical value of the fractional order as stated in the second condition can be obtained as follows
By referring to the study by [18], Eq. (3.37) can also be calculated as
From the calculations, the critical value of the initial concentration of the substrate is
The change in the running state when the value of the initial substrate concentration passes through the Hopf bifurcation point is shown. The stability of the fractional-order chemostat system changed once Hopf bifurcation occurred. In
The Hopf bifurcation points of system of fractional-order chemostat and system of integer-order chemostat are different.
The stability analysis of the fractional-order chemostat model was conducted based on the stability theory of FDEs. The integer-order chemostat model was extended to the FDEs. There are two steady-state solutions obtained, which are washout and no-washout steady-state solutions. The Hopf bifurcation of the order of α occured at the solutions of steady-state when the Hopf bifurcation conditions is fulfilled. The results show that the increasing or decreasing the value of α may stabilise the unstable state of the chemostat system. Therefore, the running state of the fractional-order chemostat system is affected by the value of α. The Hopf bifurcation of the initial concentration of the substrate, S0, also occurred when the Hopf bifurcation condition is fulfilled. As the evidence from the phase portrait plots, increase the value of the initial substrate concentration may destabilise the stable state of the chemostat system. The value of the initial substrate should remain at
[1] |
Heravi G, Salehi MM, Rostami M (2020) Identifying cost-optimal options for a typical residential nearly zero energy building's design in developing countries. Clean Technol Environ Policy 22: 2107-2128. doi: 10.1007/s10098-020-01962-4
![]() |
[2] |
Hansen K, Breyer C, Lund H (2019) Status and perspectives on 100% renewable energy systems. Energy 175: 471-480. doi: 10.1016/j.energy.2019.03.092
![]() |
[3] |
Houri Jafari H, Vakili A, Eshraghi H, et al. (2016) Energy planning and policy making; The case study of Iran. Energy Sources, Part B: Econ, Plann, Policy 11: 682-689. doi: 10.1080/15567249.2012.741186
![]() |
[4] |
Gabbar HA, Eldessouky A, Runge J (2016) Evaluation of renewable energy deployment scenarios for building energy management. AIMS Energy 4: 742-761. doi: 10.3934/energy.2016.5.742
![]() |
[5] |
Saeed T, Tularam GA (2017) Relations between fossil fuel returns and climate change variables using canonical correlation analysis. Energy Sources, Part B: Econ, Plann, Policy 12: 675-684. doi: 10.1080/15567249.2016.1265615
![]() |
[6] |
Kim SB, Cho JH (2014) A study on forecasting green infrastructure construction market. KSCE J Civ Eng 18: 430-443. doi: 10.1007/s12205-014-0189-8
![]() |
[7] |
Hailu AD, Kumsa DK (2021) Ethiopia renewable energy potentials and current state. AIMS Energy 9: 1-14. doi: 10.3934/energy.2021001
![]() |
[8] |
Kahia M, Jebli MB, Belloumi M (2019) Analysis of the impact of renewable energy consumption and economic growth on carbon dioxide emissions in 12 MENA countries. Clean Technol Environ Policy 21: 871-885. doi: 10.1007/s10098-019-01676-2
![]() |
[9] | Alizadeh R, Maknoon R, Majidpour M (2014) Clean Development Mechanism, a bridge to mitigate the Greenhouse Gasses: is it broken in Iran. 13th International Conference on Clean Energy (ICCE): 399-404. |
[10] | Asutosh AT, Woo J, Kouhirostami M, et al. (2020) Renewable energy industry trends and its contributions to the development of energy resilience in an era of accelerating climate change. Int J Energy Power Eng 14: 233-240. |
[11] |
Brockway PE, Owen A, Brand-Correa LI, et al. (2019) Estimation of global final-stage energy-return-on-investment for fossil fuels with comparison to renewable energy sources. Nat Energy 4: 612-621. doi: 10.1038/s41560-019-0425-z
![]() |
[12] |
Suárez-Eiroa B, Fernández E, Méndez-Martínez G, et al. (2019) Operational principles of circular economy for sustainable development: Linking theory and practice. J Cleaner Prod 214: 952-961. doi: 10.1016/j.jclepro.2018.12.271
![]() |
[13] |
Kirchherr J, Piscicelli L, Bour R, et al. (2018) Barriers to the circular economy: evidence from the European Union (EU). Ecol Econ 150: 264-272. doi: 10.1016/j.ecolecon.2018.04.028
![]() |
[14] |
Okafor C, Madu C, Ajaero C, et al. (2021) Moving beyond fossil fuel in an oil-exporting and emerging economy: Paradigm shift. AIMS Energy 9: 379-413. doi: 10.3934/energy.2021020
![]() |
[15] | International Energy Agency. Data and statistics, 2018. Available from: https://www.iea.org/data-and-statistics/. |
[16] |
Falcone PM, Morone P, Sica E (2018) Greening of the financial system and fuelling a sustainability transition: A discursive approach to assess landscape pressures on the Italian financial system. Technol Forecast Soc Change 127: 23-37. doi: 10.1016/j.techfore.2017.05.020
![]() |
[17] |
Gielen D, Boshell F, Saygin D, et al. (2019) The role of renewable energy in the global energy transformation. Energy Strategy Rev 24: 38-50. doi: 10.1016/j.esr.2019.01.006
![]() |
[18] |
Fadly D (2019) Low-carbon transition: Private sector investment in renewable energy projects in developing countries. World Dev 122: 552-569. doi: 10.1016/j.worlddev.2019.06.015
![]() |
[19] |
Moriarty P, Honnery D (2018) Energy policy and economics under climate change. AIMS Energy 6: 272-290. doi: 10.3934/energy.2018.2.272
![]() |
[20] |
Yang X, He L, Xia Y, et al. (2019) Effect of government subsidies on renewable energy investments: The threshold effect. Energy Policy 132: 156-166. doi: 10.1016/j.enpol.2019.05.039
![]() |
[21] |
Ndiritu SW, Engola MK (2020) The effectiveness of feed-in-tariff policy in promoting power generation from renewable energy in Kenya. Renewable Energy 161: 593-605. doi: 10.1016/j.renene.2020.07.082
![]() |
[22] | Erfani A, Tavakolan M (2020) Risk evaluation model of wind energy investment projects using modified fuzzy group decision-making and monte carlo simulation. Arthaniti: J Econ Theory Pract, 0976747920963222. |
[23] |
Echeverri-Martínez R, Alfonso-Morales W, Caicedo-Bravo EF (2020) A methodological Decision-Making support for the planning, design and operation of smart grid projects. AIMS Energy 8: 627-651. doi: 10.3934/energy.2020.4.627
![]() |
[24] |
Lee HC, Chang CT (2018). Comparative analysis of MCDM methods for ranking renewable energy sources in Taiwan. Renewable Sustainable Energy Rev 92: 883-896. doi: 10.1016/j.rser.2018.05.007
![]() |
[25] | Monzer N, Fayek AR, Lourenzutti R, et al. (2019) Aggregation-based framework for construction risk assessment with heterogeneous groups of experts. J Constr Eng Manage, 145. |
[26] |
Perez IJ, Cabrerizo FJ, Alonso S, et al. (2013) A new consensus model for group decision making problems with non-homogeneous experts. IEEE Trans Syst, Man, Cybern: Syst 44: 494-498. doi: 10.1109/TSMC.2013.2259155
![]() |
[27] | Zheng Y (2018) Identifying Dominators and Followers in Group Decision Making based on The Personality Traits. IUI Workshops. Available from: http://ceur-ws.org/Vol-2068/humanize3.pdf. |
[28] |
Morente-Molinera JA, Wu X, Morfeq A, et al. (2020). A novel multi-criteria group decision-making method for heterogeneous and dynamic contexts using multi-granular fuzzy linguistic modelling and consensus measures. Inf Fusion 53: 240-250. doi: 10.1016/j.inffus.2019.06.028
![]() |
[29] |
Alizadeh R, Soltanisehat L, Lund PD, et al. (2020). Improving renewable energy policy planning and decision-making through a hybrid MCDM method. Energy Policy 137: 111-174. doi: 10.1016/j.enpol.2019.111174
![]() |
[30] |
Gündoğdu FK, Kahraman C (2020) A novel spherical fuzzy analytic hierarchy process and its renewable energy application. Soft Comput 24: 4607-4621. doi: 10.1007/s00500-019-04222-w
![]() |
[31] |
Zarnegar M (2018) Renewable energy utilization in Iran. Energy Sources, Part A: Recovery, Util, Environ Eff 40: 765-771. doi: 10.1080/15567036.2018.1457741
![]() |
[32] |
Thang VV, Ha T (2019) Optimal siting and sizing of renewable sources in distribution system planning based on life cycle cost and considering uncertainties. AIMS Energy 7: 211-226. doi: 10.3934/energy.2019.2.211
![]() |
[33] | Alizadeh R, Beiragh RG, Soltanisehat L, et al. (2020) Performance evaluation of complex electricity generation systems: A dynamic network-based data envelopment analysis approach. Energy Econ, 91. |
[34] | Alizadeh R, Lund PD, Soltanisehat L (2020) Outlook on biofuels in future studies: A systematic literature review. Renewable Sustainable Energy Rev, 134. |
[35] |
Alizadeh R, Allen JK, Mistree F (2020) Managing computational complexity using surrogate models: a critical review. Res Eng Des 31: 275-298. doi: 10.1007/s00163-020-00336-7
![]() |
[36] | Bou-Rabee M, Sulaiman SA, Saleh MS, et al. (2017) Using artificial neural networks to estimate solar radiation in Kuwait. Renewable Sustainable Energy Rev 72: 434-438. |
[37] |
Teimourian A, Bahrami A, Teimourian H, et al. (2020) Assessment of wind energy potential in the southeastern province of Iran. Energy Sources, Part A: Recovery, Util, Environ Eff 42: 329-343. doi: 10.1080/15567036.2019.1587079
![]() |
[38] | Çakmakçı BA, Hüner E (2020) Evaluation of wind energy potential: a case study. Energy Sources, Part A: Recovery, Util, Environ Eff, 1-19. |
[39] |
Azizkhani M, Vakili A, Noorollahi Y, et al. (2017) Potential survey of photovoltaic power plants using Analytical Hierarchy Process (AHP) method in Iran. Renewable Sustainable Energy Rev 75: 1198-1206. doi: 10.1016/j.rser.2016.11.103
![]() |
[40] |
Díaz-Cuevas P, Domínguez-Bravo J, Prieto-Campos A (2019) Integrating MCDM and GIS for renewable energy spatial models: assessing the individual and combined potential for wind, solar and biomass energy in Southern Spain. Clean Technol Environ Policy 21: 1855-1869. doi: 10.1007/s10098-019-01754-5
![]() |
[41] |
Yun TS, Lee JS, Lee SC, et al. (2011) Geotechnical issues related to renewable energy. KSCE J Civ Eng 15: 635-642. doi: 10.1007/s12205-011-0004-8
![]() |
[42] |
Liu X, Xu Y, Herrera F (2019). Consensus model for large-scale group decision making based on fuzzy preference relation with self-confidence: Detecting and managing overconfidence behaviors. Inf Fusion 52: 245-256. doi: 10.1016/j.inffus.2019.03.001
![]() |
[43] |
Heravi G, Fathi M, Faeghi S (2017) Multi-criteria group decision-making method for optimal selection of sustainable industrial building options focused on petrochemical projects. J Cleaner Prod 142: 2999-3013. doi: 10.1016/j.jclepro.2016.10.168
![]() |
[44] |
Dranka GG, Cunha J, de Lima JD, et al. (2020) Economic evaluation methodologies for renewable energy projects. AIMS Energy 8: 339-364. doi: 10.3934/energy.2020.2.339
![]() |
[45] |
Boran FE, Boran K, Dizdar E (2012) A fuzzy multi criteria decision making to evaluate energy policy based on an information axiom: a case study in Turkey. Energy Sources, Part B: Econ, Plann, Policy 7: 230-240. doi: 10.1080/15567240902839294
![]() |
[46] |
Celiktas MS, Kocar G (2010) From potential forecast to foresight of Turkey's renewable energy with Delphi approach. Energy 35: 1973-1980. doi: 10.1016/j.energy.2010.01.012
![]() |
[47] | Kul C, Zhang L, Solangi YA (2020) Assessing the Renewable Energy Investment Risk Factors for Sustainable Development in Turkey. J Cleaner Prod, 124164. |
[48] | Hsu CC, Sandford BA (2007) The Delphi technique: making sense of consensus. Pract Assess, Res, Eval 12: 10. |
[49] |
Kabak M, Dağdeviren M, Burmaoğlu S (2016) A hybrid SWOT-FANP model for energy policy making in Turkey. Energy Sources, Part B: Econ, Plann, Policy 11: 487-495. doi: 10.1080/15567249.2012.673692
![]() |
[50] |
Aslani A, Naaranoja M, Zakeri B (2012) The prime criteria for private sector participation in renewable energy investment in the Middle East (case study: Iran). Renewable Sustainable Energy Rev 16: 1977-1987. doi: 10.1016/j.rser.2011.12.015
![]() |
[51] | Aslani A, Feng B (2014) Investment prioritization in renewable energy resources with consideration to the investment criteria in Iran. Distrib Gener Altern Energy J 29: 7-26. |
[52] | Erfani A, Tavakolan M (2019) Challenges in renewable energy investment projects in Iran: A review of the main Criteria and Risks. 3rd International Conference on Applied Researches in Structural Engineering and Construction Management, 1-10. |
[53] |
Boran FE (2018) A new approach for evaluation of renewable energy resources: A case of Turkey. Energy Sources, Part B: Econ, Plann, Policy 13: 196-204. doi: 10.1080/15567249.2017.1423414
![]() |
[54] |
Ozorhon B, Batmaz A, Caglayan S (2018) Generating a framework to facilitate decision making in renewable energy investments. Renewable Sustainable Energy Rev 95: 217-226. doi: 10.1016/j.rser.2018.07.035
![]() |
[55] |
Cavallaro F, Ciraolo L (2005) A multicriteria approach to evaluate wind energy plants on an Italian island. Energy Policy 33: 235-244. doi: 10.1016/S0301-4215(03)00228-3
![]() |
[56] |
Wang JJ, Jing YY, Zhang CF, et al. (2009) Review on multi-criteria decision analysis aid in sustainable energy decision-making. Renewable Sustainable Energy Rev 13: 2263-2278. doi: 10.1016/j.rser.2009.06.021
![]() |
[57] |
Kaya T, Kahraman C (2010) Multicriteria renewable energy planning using an integrated fuzzy VIKOR & AHP methodology: The case of Istanbul. Energy 35: 2517-2527. doi: 10.1016/j.energy.2010.02.051
![]() |
[58] |
Şengül Ü, Eren M, Shiraz SE, et al. (2015) Fuzzy TOPSIS method for ranking renewable energy supply systems in Turkey. Renewable Energy 75: 617-625. doi: 10.1016/j.renene.2014.10.045
![]() |
[59] |
Strantzali E, Aravossis K (2016) Decision making in renewable energy investments: A review. Renewable Sustainable Energy Rev 55: 885-898. doi: 10.1016/j.rser.2015.11.021
![]() |
[60] |
Al Garni H, Kassem A, Awasthi A, et al. (2016) A multicriteria decision making approach for evaluating renewable power generation sources in Saudi Arabia. Sustainable Energy Technol Assess 16: 137-150. doi: 10.1016/j.seta.2016.05.006
![]() |
[61] |
Balin A, Baraçli H (2017) A fuzzy multi-criteria decision-making methodology based upon the interval type-2 fuzzy sets for evaluating renewable energy alternatives in Turkey. Technol Econ Dev Econ 23: 742-763. doi: 10.3846/20294913.2015.1056276
![]() |
[62] |
Çolak M, Kaya İ (2017) Prioritization of renewable energy alternatives by using an integrated fuzzy MCDM model: A real case application for Turkey. Renewable Sustainable Energy Rev 80: 840-853. doi: 10.1016/j.rser.2017.05.194
![]() |
[63] |
Wu Y, Li L, Song Z, et al. (2019) Risk assessment on offshore photovoltaic power generation projects in China based on a fuzzy analysis framework. J Cleaner Prod 215: 46-62. doi: 10.1016/j.jclepro.2019.01.024
![]() |
[64] |
Solangi YA, Tan Q, Mirjat NH, et al. (2019) An integrated Delphi-AHP and fuzzy TOPSIS approach toward ranking and selection of renewable energy resources in Pakistan. Processes 7: 118. doi: 10.3390/pr7020118
![]() |
[65] | Karakaş E (2019) Evaluation of renewable energy alternatives for turkey via modified fuzzy ahp. Available from: http://zbw.eu/econis-archiv/bitstream/11159/3155/1/1667513583.pdf. |
[66] |
Peng HG, Shen KW, He SS, et al. (2019) Investment risk evaluation for new energy resources: An integrated decision support model based on regret theory and ELECTRE III. Energy Convers Manage 183: 332-348. doi: 10.1016/j.enconman.2019.01.015
![]() |
[67] | Saaty TL (2008) Decision making with the analytic hierarchy process. Int J Serv Sci 1: 83-98. |
[68] |
Kamaruzzaman SN, Lou ECW, Wong PF, et al. (2018) Developing weighting system for refurbishment building assessment scheme in Malaysia through analytic hierarchy process (AHP) approach. Energy Policy 112: 280-290. doi: 10.1016/j.enpol.2017.10.023
![]() |
[69] | Saaty TL (1977) A scaling method for priorities in hierarchical structures. J Math Psychol 15: 234-281. |
[70] | Saaty TL (1990) Multicriteria decision making: the analytic hierarchy process: planning, priority setting resource allocation. |
[71] |
Katal F, Fazelpour F (2018) Multi-criteria evaluation and priority analysis of different types of existing power plants in Iran: An optimized energy planning system. Renewable Energy 120: 163-177. doi: 10.1016/j.renene.2017.12.061
![]() |
[72] |
Erdogan SA, Šaparauskas J, Turskis Z (2019) A multi-criteria decision-making model to choose the best option for sustainable construction management. Sustainability 11: 2239. doi: 10.3390/su11082239
![]() |
[73] | Feili H, Ahmadian P, Rabiei E, et al. (2018) Ranking of suitable renewable energy location using AHP method and scoring systems with sustainable development perspective. 6th International Conference on Economics, Management, Engineering Science and Art, 1-8. |
[74] | OPEC, Share of world crude oil reserves (2018) Available from: https://www.opec.org/opec_web/en/data_graphs/330.htm. |
[75] |
Mollahosseini A, Hosseini SA, Jabbari M, et al. (2017) Renewable energy management and market in Iran: A holistic review on current state and future demands. Renewable Sustainable Energy Rev 80: 774-788. doi: 10.1016/j.rser.2017.05.236
![]() |
1. | Xiaomeng Ma, Zhanbing Bai, Sujing Sun, Stability and bifurcation control for a fractional-order chemostat model with time delays and incommensurate orders, 2022, 20, 1551-0018, 437, 10.3934/mbe.2023020 |
Parameters | Description | Values | Units |
k | Saturation constant | 1.75 | gl−1 |
Q | Dilution rate | 0.02 | l2gr−1 |
µ | Maximum growth rate | 0.3 | h−1 |
γ | Constant in yield coefficient | 0.01 | – |
β | Constant in yield coefficient | 5.25 | lg−1 |
S0 | Input concentration of substrate | 1 | gl−1 |