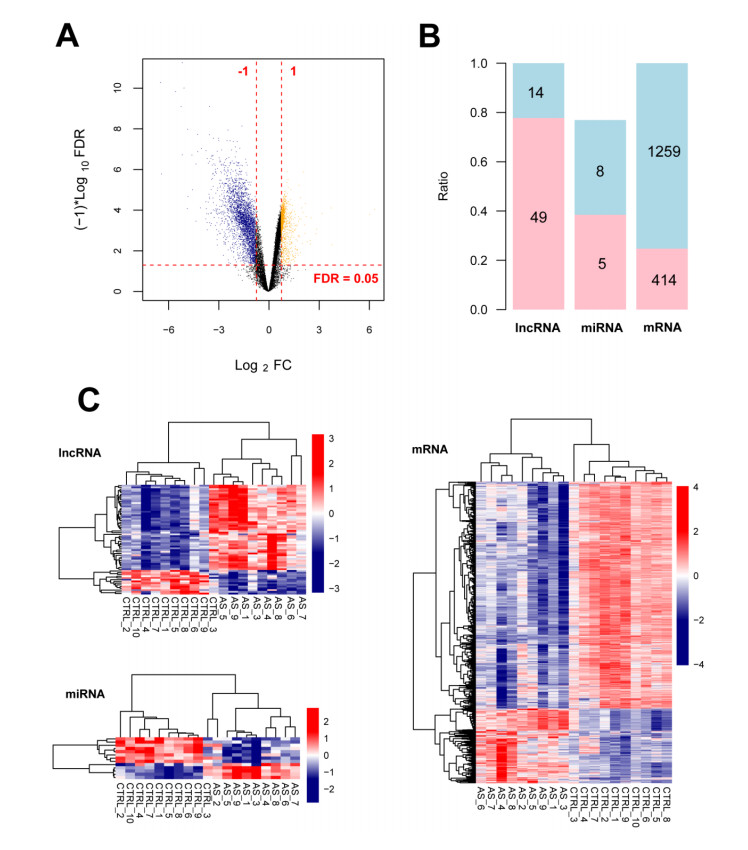
This study aimed to analyze the potential genes associated with immune cell infiltration in atherosclerosis (AS).
Gene expression profile data (GSE57691) of human arterial tissue samples were downloaded, and differentially expressed RNAs (DERNAs; long-noncoding RNA [lncRNAs], microRNAs [miRNAs], and messenger RNAs [mRNAs]) in AS vs. control groups were selected. Based on genome-wide expression levels, the proportion of infiltrating immune cells in each sample was assessed. Genes associated with immune infiltration were selected, and subjected to Gene Ontology (GO) and Kyoto Encyclopedia of Genes and Genomes (KEGG) enrichment analyses. Finally, a competing endogenous RNA (ceRNA) network was constructed, and the genes in the network were subjected to functional analyses.
A total of 1749 DERNAs meeting the thresholds were screened, including 1673 DEmRNAs, 63 DElncRNAs, and 13 DEmiRNAs. The proportions of B cells, CD4+ T cells, and CD8+ T cells were significantly different between the two groups. In total, 341 immune-associated genes such as HBB, FCN1, IL1B, CXCL8, RPS27A, CCN3, CTSZ, and SERPINA3 were obtained that were enriched in 70 significantly related GO biological processes (such as immune response) and 15 KEGG pathways (such as chemokine signaling pathway). A ceRNA network, including 33 lncRNAs, 11 miRNAs, and 216 mRNAs, was established.
Genes such as FCN1, IL1B, and SERPINA3 may be involved in immune cell infiltration and may play important roles in AS progression via ceRNA regulation.
Citation: Xiaodong Xia, Manman Wang, Jiao Li, Qiang Chen, Heng Jin, Xue Liang, Lijun Wang. Identification of potential genes associated with immune cell infiltration in atherosclerosis[J]. Mathematical Biosciences and Engineering, 2021, 18(3): 2230-2242. doi: 10.3934/mbe.2021112
[1] | Jie Chen, Jinggui Chen, Bo Sun, Jianghong Wu, Chunyan Du . Integrative analysis of immune microenvironment-related CeRNA regulatory axis in gastric cancer. Mathematical Biosciences and Engineering, 2020, 17(4): 3953-3971. doi: 10.3934/mbe.2020219 |
[2] | Yun-xiang Li, Shi-ming Wang, Chen-quan Li . Four-lncRNA immune prognostic signature for triple-negative breast cancer. Mathematical Biosciences and Engineering, 2021, 18(4): 3939-3956. doi: 10.3934/mbe.2021197 |
[3] | Jianpei Hu, Zengnan Mo . Dissection of tumor antigens and immune landscape in clear cell renal cell carcinoma: Preconditions for development and precision medicine of mRNA vaccine. Mathematical Biosciences and Engineering, 2023, 20(2): 2157-2182. doi: 10.3934/mbe.2023100 |
[4] | Xuesi Chen, Qijun Zhang, Qin Zhang . Predicting potential biomarkers and immune infiltration characteristics in heart failure. Mathematical Biosciences and Engineering, 2022, 19(9): 8671-8688. doi: 10.3934/mbe.2022402 |
[5] | Jie Wang, Md. Nazim Uddin, Rehana Akter, Yun Wu . Contribution of endothelial cell-derived transcriptomes to the colon cancer based on bioinformatics analysis. Mathematical Biosciences and Engineering, 2021, 18(6): 7280-7300. doi: 10.3934/mbe.2021360 |
[6] | Shengjue Xiao, Yufei Zhou, Ailin Liu, Qi Wu, Yue Hu, Jie Liu, Hong Zhu, Ting Yin, Defeng Pan . Uncovering potential novel biomarkers and immune infiltration characteristics in persistent atrial fibrillation using integrated bioinformatics analysis. Mathematical Biosciences and Engineering, 2021, 18(4): 4696-4712. doi: 10.3934/mbe.2021238 |
[7] | Rongxing Qin, Lijuan Huang, Wei Xu, Qingchun Qin, Xiaojun Liang, Xinyu Lai, Xiaoying Huang, Minshan Xie, Li Chen . Identification of disulfidptosis-related genes and analysis of immune infiltration characteristics in ischemic strokes. Mathematical Biosciences and Engineering, 2023, 20(10): 18939-18959. doi: 10.3934/mbe.2023838 |
[8] | Yuedan Wang, Jinke Huang, Jiaqi Zhang, Fengyun Wang, Xudong Tang . Identifying biomarkers associated with the diagnosis of ulcerative colitis via bioinformatics and machine learning. Mathematical Biosciences and Engineering, 2023, 20(6): 10741-10756. doi: 10.3934/mbe.2023476 |
[9] | Jun Wang, Mingzhi Gong, Zhenggang Xiong, Yangyang Zhao, Deguo Xing . Immune-related prognostic genes signatures in the tumor microenvironment of sarcoma. Mathematical Biosciences and Engineering, 2021, 18(3): 2243-2257. doi: 10.3934/mbe.2021113 |
[10] | Chenyang Jiang, Weidong Jiang . AGTR1, PLTP, and SCG2 associated with immune genes and immune cell infiltration in calcific aortic valve stenosis: analysis from integrated bioinformatics and machine learning. Mathematical Biosciences and Engineering, 2022, 19(4): 3787-3802. doi: 10.3934/mbe.2022174 |
This study aimed to analyze the potential genes associated with immune cell infiltration in atherosclerosis (AS).
Gene expression profile data (GSE57691) of human arterial tissue samples were downloaded, and differentially expressed RNAs (DERNAs; long-noncoding RNA [lncRNAs], microRNAs [miRNAs], and messenger RNAs [mRNAs]) in AS vs. control groups were selected. Based on genome-wide expression levels, the proportion of infiltrating immune cells in each sample was assessed. Genes associated with immune infiltration were selected, and subjected to Gene Ontology (GO) and Kyoto Encyclopedia of Genes and Genomes (KEGG) enrichment analyses. Finally, a competing endogenous RNA (ceRNA) network was constructed, and the genes in the network were subjected to functional analyses.
A total of 1749 DERNAs meeting the thresholds were screened, including 1673 DEmRNAs, 63 DElncRNAs, and 13 DEmiRNAs. The proportions of B cells, CD4+ T cells, and CD8+ T cells were significantly different between the two groups. In total, 341 immune-associated genes such as HBB, FCN1, IL1B, CXCL8, RPS27A, CCN3, CTSZ, and SERPINA3 were obtained that were enriched in 70 significantly related GO biological processes (such as immune response) and 15 KEGG pathways (such as chemokine signaling pathway). A ceRNA network, including 33 lncRNAs, 11 miRNAs, and 216 mRNAs, was established.
Genes such as FCN1, IL1B, and SERPINA3 may be involved in immune cell infiltration and may play important roles in AS progression via ceRNA regulation.
Atherosclerosis (AS) is a progressive, chronic, lipid-driven inflammatory disease of the arterial wall and an underlying cause of most cardiovascular diseases [1]. Many factors contribute to the accumulation of atheromatous plaques in the arterial wall, such as hypercholesterolemia, hypertension, diabetes mellitus, obesity, and smoking [2]. Despite the development of preventive strategies targeting classical risk factors, AS remains a major cause of morbidity and mortality worldwide [3]. At present, the lack of timely detection and effective control has led to ineffective control of the occurrence and development of atherosclerotic stenosis and vulnerable plaques [4]. Therefore, it is imperative to explore the accurate molecular markers and potential pathogenesis to contribute to the diagnosis and treatment of atherosclerotic diseases.
AS is a chronic inflammatory disease that involves infiltration of immune cells such as neutrophils, macrophages, T cells, and B cells, into the inner layer of vessel walls [5]. The role of immune cells in AS has been widely studied. For instance, a previous study reported T cell recruitment at an early stage in the development of atherosclerotic lesions [6]. In the presence of low-density lipoprotein, chemoattractants and adhesion molecules are expressed in the intima, leading to T cell infiltration into the plaques [7]. The function of B cell subsets in atherogenesis is controversial, as it has both atherogenic and atheroprotective properties [8]. Although numerous studies have reported the underlying pathogenesis of immune cell infiltration in AS, only a few studies have analyzed the potential genes associated with immune cell infiltration in AS [9].
In this study, we downloaded the AS-associated gene expression profile dataset (GSE57691) from the NCBI Gene Expression Omnibus (GEO) database and analyzed the proportion of immune cells and immune infiltration-related genes via bioinformatic analysis. A competing endogenous RNA (ceRNA) network was subsequently constructed to explore the potential regulatory mechanisms of immune-related genes in AS. Our results provide evidence of the involvement of the genes related to immune cell infiltration in the occurrence and development of AS.
On June 16, 2020, the gene expression profile data (GSE57691 [10]) of human arterial tissue samples were downloaded from the NCBI GEO [11] (https://www.ncbi.nlm.nih.gov/) database. This dataset contained 68 samples, and only 19 samples related to AS were selected for analysis, including nine samples of arterial occlusion and 10 samples from healthy controls. The clinical information of these 19 AS-related samples is shown in Supplementary Table 1. The test platform was an Illumina HumanHT-12 V4.0 expression beadchip.
The detailed platform annotation information (probe corresponding RNA type and symbol) of the Illumina HumanHT-12 V4.0 expression beadchip was downloaded from the ENSEMBL database (https://asia.ensembl.org/index.html). Based on the long-noncoding RNA (lncRNA), microRNA (miRNA), and messenger RNA (mRNA) annotation information included in the HUGO Gene Nomenclature Committee (HGNC) [12] database (http://www.genenames.org/), the lncRNAs, miRNAs, and mRNAs in the expression profile dataset were annotated.
The limma version 3.34.7 [13] in R3.4.1 language was subsequently used to screen significantly DERNAs (lncRNA, miRNA, and mRNA) between the AS and the control comparison groups, and false discovery rate (FDR) < 0.05 and |log2 fold change (FC)| > 1 were selected as the thresholds. According to the expression levels of these identified DERNAs in samples, bidirectional hierarchical clustering based on the Pearson correlation algorithm was conducted using R3.4.1 pheatmap version 1.0.8 (https://cran.r-project.org/web/packages/pheatmap/index.html) [14].
Each gene expression profile dataset was uploaded to estimate the proportion of immune and cancer cells (EPIC, https://gfellerlab.shinyapps.io/EPIC_1-1/) [15], and the proportion of immune-infiltrating cells in each sample was assessed based on genome-wide expression levels. EPIC can analyze the infiltration rate of the following seven immune cells based on the expression data: B cells, CD4+ T cells, CD8+ T cells, endothelial cells, macrophages, natural killer cells, and other immune cells.
Using the cor function in R3.4.1, the Pearson's correlation coefficient between the significant DEmRNAs and the immune-infiltrating cell types in the sample was calculated, and significant DEmRNAs closely associated with immune infiltration were identified. In addition, the biological processes related to immunity were searched from the AmiGO 2 database (http://amigo.geneontology.org/amigo) with "immune" as the keyword, and the genes associated with immune-related biological processes were downloaded. Meanwhile, the Kyoto Encyclopedia of Genes and Genomes (KEGG) database (https://www.kegg.jp/) was used to download all pathways associated with "immune." Next, the intersection sections between DEmRNAs and immune function-related genes were selected for further analyses, including Gene Ontology (GO) and KEGG enrichment analyses (criteria for significance screening: p value < 0.05) using DAVID 6.8 (https://david.ncifcrf.gov/) [16,17].
The connection relationships between DElncRNA and DEmiRNAs were searched from the DIANA-LncBasev2 database [18] (http://carolina.imis.athena-innovation.gr/diana_tools/web/index.php?r=lncbasev2%2Findex-experimental), and only the connection pairs with opposite DE direction were retained to construct DElncRNA-DEmiRNA connection network. Thereafter, the starBase Version 2.0 database [19] (http://starbase.sysu.edu.cn/) that integrates the target gene prediction information from TargetScan, PicTar, RNA22, PITA, and miRanda databases was used to search for the target genes of DEmiRNAs. We selected the regulatory relationships included in at least one of the databases as DEmiRNA-target gene pairs. Then, the DEmRNAs were mapped to the target genes, and the miRNA and mRNA pairs that were negatively correlated in expression levels were retained to build a DEmiRNA-DEmRNA regulatory relationship.
Finally, DElncRNA-DEmiRNA and DEmiRNA-DEmRNA connection networks were synthesized, and a ceRNA network comprising DElncRNAs, DEmiRNAs, and DEmRNAs was proposed and visualized using Cytoscape version 3.6.1 (https://cytoscape.org/) [20]. DAVID 6.8 was used for GO biological process and KEGG pathway enrichment analyses of these genes in the ceRNA regulatory network. A P value less than 0.05 was selected as the threshold for screening significant enrichment.
In the dataset, 17861 mRNAs, 902 lncRNAs, and 46 miRNAs were annotated. For the AS versus control comparison groups in the dataset, a total of 1749 DERNAs meeting the thresholds were screened, including 1673 mRNAs (1259 downregulated and 414 upregulated), 63 lncRNAs (14 downregulated and 49 upregulated), and 13 miRNAs (8 downregulated and 5 upregulated). The volcano plot is shown in Figure 1A, and the distribution of DElncRNAs, DEmiRNAs, and DEmRNAs is displayed in Figure 1B. The bidirectional hierarchical clustering heatmaps based on the expression level of DERNAs are shown in Figure 1C, which infers that the three types of RNAs could effectively differentiate the AS samples from the healthy control samples.
EPIC was used to quantitatively evaluate the types of immune-infiltrating cells in each sample, as shown in Figure 2A. We used the intergroup T test to compare each type of immune-infiltrating cells in the AS and control groups. As shown in Figure 2B, the proportions of B cells, CD4+ T cells, and CD8+ T cells were significantly different between the two groups.
The correlation coefficients between the three types of immune-infiltrating cells and DEmRNAs were calculated, and only those genes with absolute values of correlation coefficients greater than 0.2 were retained. Finally, 1614 genes were identified. In total, 3020 unique genes related to immune function and 817 unique genes related to immune pathways were downloaded from the database. As shown in Figure 3, 582 intersection genes were observed, which were further compared with 1614 genes above to obtain 341 intersection genes, including HBB, FCN1, IL1B, CXCL8, RPS27A, CCN3, CTSZ, and SERPINA3. The overlapping genes were subjected to GO biological process and KEGG signal pathway enrichment analyses, and a total of 70 significantly related GO biological processes (such as immune response, positive regulation of molecular function, and response to wounding) and 15 KEGG pathways (such as chemokine signaling pathway, leukocyte transendothelial migration, and proteasome) were observed (Figure 4).
After searching the DIANA-LncBasev2 database, 89 DElncRNA-DEmiRNA pairs were obtained. By mapping the 314 immune-related genes to the regulatory target genes of the DEmiRNAs, 409 DEmiRNA-DEmRNA pairs were identified. By integrating DElncRNA-DEmiRNA and DEmiRNA-DEmRNA interaction pairs, a ceRNA network was constructed that included 33 lncRNAs (SNHG10, MBNL1-AS1, PKN2-AS1, LINC01165, MIAT, etc.), 11 miRNAs (miR-6764, miR-6514, miR-1226, miR-7-1, etc.), and 216 mRNAs (e.g., FCN1, SERPINA3, and IL1B) (Figure 5). GO and KEGG enrichment analyses showed that these mRNAs in the ceRNA network were significantly enriched in immune response, positive regulation of molecular function, positive regulation of catalytic activity, response to wounding, and chemotaxis, as well as pathways of chemokine signaling pathway, leukocyte transendothelial migration, regulation of actin cytoskeleton, cytokine-cytokine receptor interaction, and T cell receptor signaling pathway (Figure 6).
AS is a chronic inflammatory disorder of the arteries, characterized by subendothelial accumulation of immune cells, extracellular matrix deposition, and cholesterol [21]. In this study, we focused on immune cell infiltration in AS and identified 341 immune-related genes. Based on these genes and the identified DEmiRNAs and DElncRNAs, a ceRNA network comprising 33 lncRNAs, 11 miRNAs, and 216 mRNAs was constructed, and the mRNAs in this network were found to be significantly enriched in some immune-associated functions and pathways.
In comparison with the healthy condition, AS can contribute to the change in cell types in the arterial tissue, which expresses different species of RNA, and T and B cells in the media of the vascular wall play important roles during the development of atherosclerotic processes [22]. Our results showed that B cells, CD4+ T cells, and CD8+ T cells were significantly differently expressed between the two groups, which further indicated their important role in AS. During AS, T cells can recognize self-antigens and induce humoral immunity driven by B cells [23], leading to the destruction of active immune privileges in the media. CD4+ T cells are the main T cell type at all stages of lesion development. It has been reported that local immune responses dominated by CD4+ T cells occur at all stages of AS [24]. Additionally, abundant CD8+ T cell infiltrates have been identified in atherosclerotic lesions of the carotid artery [25]. However, in contrast to CD4+ T cells, no AS-related antigens that may activate CD8+ T cells have been found [26]. Based on our results, we speculate that AS progression may be closely associated with changes in B cells, CD4+ T cells, and CD8+ T cells.
To investigate the underlying molecular mechanisms of AS, immune cell infiltration-related genes were analyzed. A total of 341 immune infiltration-related genes were identified. By combining the obtained DEmiRNAs and DElncRNAs, a ceRNA network was established to investigate the potential regulatory mechanism in AS. FCN1 (ficolin 1) had the highest |log2 FC| among the immune-related upregulated genes in the network. FCN1 is involved in the complement lectin pathway and is elevated in patients with Takayasu arteritis [27]. Abnormal expression of FCN1 has been suggested as a potential target for coronary artery diseases [28]. To our knowledge, the role of FCN1 in AS has not been well investigated. In this study, FCN1 was enriched in immune response-associated GO function, which further demonstrated the important function of the immune system in AS. Further, FCN1 is involved in the SNHG10-miR-6764-FCN1 axis. We speculate that SNHG10 may function as a ceRNA to promote AS progression by upregulating FCN1.
IL1B (interleukin 1 beta) also had a higher log2 FC among the immune-related genes in the ceRNA network, and was enriched in the cytokine-cytokine receptor interaction pathway. IL1B, one of the first recognized cytokines, is a critical driving factor of local and systemic immune responses in atherosclerotic cardiovascular disease [29]. A study suggested that IL1B can promote a pro-atherogenic phenotypic shift in vascular smooth muscle cells by downregulating the expression of smooth muscle markers and inducing the expression of pro-inflammatory cytokines [30]. In the constructed ceRNA network, IL1B was found to be regulated by miR-7-1 in the ceRNA relationship of PKN2-AS1-miR-7-1-IL1B. We speculate that PKN2-AS1 may serve as a ceRNA to facilitate AS by regulating IL1B expression.
SERPINA3 (serpin family A member 3) had the highest |log2 FC| among the immune-related downregulated genes in the network, and was regulated by miR-6514. It was involved in the ceRNA axis of SERPINA3-miR-6514-MBNL1-AS1. SERPINA3 belongs to the serpin superfamily and plays an important role in vessel wall biology [31]. The plasma SERPINA3 level is elevated in acute myocardial infarction as compared with healthy controls [32]. Importantly, SERPINA3 is expressed in the medial smooth muscle cells and endothelial cells of human atherosclerotic lesions [33]. Further, it can regulate immune cells via elastase and cathepsin G regulation [31,34], indicating its role in immunity.
However, this study has some limitations. The number of samples is small, necessitating further studies with larger sample sizes to verify this conclusion. In addition, the immune cell-specific or non-specific biomarkers associated with AS should be further explored, and experimental studies on human and mouse models should be carried out to confirm our findings.
In conclusion, our study identified 1673 DEmRNAs, 63 DElncRNAs, and 13 DEmiRNAs between AS patients and healthy controls, and 341 immune-related genes. B cells, CD4+ T cells, and CD8+ T cells were found to be closely related to AS progression. A ceRNA regulatory network comprising 33 lncRNAs, 11 miRNAs, and 216 mRNAs was proposed, and immune-related genes such as FCN1, IL1B, and SERPINA3 were thought to serve as potential risk markers of atherosclerotic immune cell infiltration. These genes may promote AS progression via ceRNA regulation. Our findings will help improve the understanding of the occurrence and development of AS and explain novel underlying pathological molecular mechanisms for AS.
This study was supported by the National Natural Science Foundation of China Youth Science Foundation Project (No. 81700304), the Scientific Research Fund Project of Key Laboratory of the Second Hospital of Tianjin Medical University (No. 2019ZDSYS11), the Foundation of Tianjin Union Medical Center (No. 2017YJ006), and Peking Union Medical Foundation - Ruiyi Emergency Medical Research Fund (No. R2018006), the National Natural Science Foundation of China (No. 82072222), and the Fundamental Research Funds for the Central Universities, China (No.3332019127). These funders have no role in this study.
The authors declare that they have no conflict of interest.
[1] | A. Gistera, G. K. Hansson, The immunology of atherosclerosis, Nat. Rev. Nephrol., 13 (2017), 368-380. |
[2] | I. Gyárfás, M. Keltai, Y. Salim, Effect of potentially modifiable risk factors associated with myocardial infarction in 52 countries (the interheart study): Case-control study, Orvosi. Hetil., 147 (2006), 675-686. |
[3] |
M. Nus, Z. Mallat, Immune-mediated mechanisms of atherosclerosis and implications for the clinic, Expert Rev. Clin. Immunol., 12 (2016), 1217-1237. doi: 10.1080/1744666X.2016.1195686
![]() |
[4] |
L. Saba, T. Saam, H. R. Jäger, C. Yuan, T. S. Hatsukami, D. Saloner, et al., Imaging biomarkers of vulnerable carotid plaques for stroke risk prediction and their potential clinical implications, Lancet Neurol., 18 (2019), 559-572. doi: 10.1016/S1474-4422(19)30035-3
![]() |
[5] |
D. Baptista, F. Mach, K. J. Brandt, Follicular regulatory T cell in atherosclerosis, J. Leukoc. Biol., 104 (2018), 925-930. doi: 10.1002/JLB.MR1117-469R
![]() |
[6] | T. Shimokama, S. Haraoka, T. Watanabe, Immunohistochemical and ultrastructural demonstration of the lymphocyte-macrophage interaction in human aortic intima, Mod. pathol. Offic. J. United States Canadian Acad. Pathol., 4 (1991), 101-107. |
[7] |
A. Hermansson, D. F. Ketelhuth, D. Strodthoff, M. Wurm, E. M. Hansson, A. Nicoletti, et al., Inhibition of T cell response to native low-density lipoprotein reduces atherosclerosis, J. Exp. Med., 207 (2010), 1081-1093. doi: 10.1084/jem.20092243
![]() |
[8] |
D. Tsiantoulas, A. P. Sage, Z. Mallat, C. J. Binder, Targeting B cells in atherosclerosis: closing the gap from bench to bedside, Arterioscler, Thromb., Vasc. Biol., 35 (2015), 296-302. doi: 10.1161/ATVBAHA.114.303569
![]() |
[9] |
J. Xu, Y. Yang, Potential genes and pathways along with immune cells infiltration in the progression of atherosclerosis identified via microarray gene expression dataset re-analysis, Vascular, 28 (2020), 643-654. doi: 10.1177/1708538120922700
![]() |
[10] |
E. Biros, G. Gabel, C. S. Moran, C. Schreurs, J. H. N. Lindeman, P. J. Walker, et al., Differential gene expression in human abdominal aortic aneurysm and aortic occlusive disease, Oncotarget, 6 (2015), 12984-12996. doi: 10.18632/oncotarget.3848
![]() |
[11] |
T. Barrett, S. E. Wilhite, P. Ledoux, C. Evangelista, I. F. Kim, M. Tomashevsky, et al., NCBI GEO: Archive for functional genomics data sets-update, Nucleic Acids Res., 41 (2012), 991-995. doi: 10.1093/nar/gks1193
![]() |
[12] |
M. W. Wright, A short guide to long non-coding RNA gene nomenclature, Hum. Genomics, 8 (2014), 7. doi: 10.1186/1479-7364-8-7
![]() |
[13] |
M. E. Ritchie, B. Phipson, D. Wu, Y. Hu, C. W. Law, W. Shi, et al., Limma powers differential expression analyses for RNA-sequencing and microarray studies, Nucleic Acids Res., 43 (2015), e47. doi: 10.1093/nar/gkv007
![]() |
[14] |
L. Wang, C. Cao, Q. Ma, Q. Zeng, H. Wang, Z. Cheng, et al., RNA-seq analyses of multiple meristems of soybean: novel and alternative transcripts, evolutionary and functional implications, BMC Plant Biol., 14 (2014), 169-169. doi: 10.1186/1471-2229-14-169
![]() |
[15] | J. Racle, K. De Jonge, P. Baumgaertner, D. E. Speiser, D. Gfeller, Simultaneous enumeration of cancer and immune cell types from bulk tumor gene expression data, eLife, 13 (2017), e26476. |
[16] |
D. Huang, B. T. Sherman, R. A. Lempicki, Systematic and integrative analysis of large gene lists using DAVID bioinformatics resources, Nat. Protoc., 4 (2009), 44-57. doi: 10.1038/nprot.2008.211
![]() |
[17] |
L. Salmena, L. Poliseno, Y. Tay, L. Kats, P. P. Pandolfi, A ceRNA hypothesis: The rosetta stone of a hidden rna language?, Cell, 146 (2011), 353-358. doi: 10.1016/j.cell.2011.07.014
![]() |
[18] |
M. D. Paraskevopoulou, I. S. Vlachos, D. Karagkouni, G. Georgakilas, I. Kanellos, T. Vergoulis, et al., DIANA-LncBase v2: Indexing microRNA targets on non-coding transcripts, Nucleic Acids Res., 44 (2016), 231-238. doi: 10.1093/nar/gkv1270
![]() |
[19] | J. Li, S. Liu, H. Zhou, L. Qu, J. Yang, StarBase v2.0: decoding miRNA-ceRNA, miRNA-ncRNA and protein-RNA interaction networks from large-scale CLIP-Seq data, Nucleic Acids Res., 42 (2014), 92-97. |
[20] |
P. Shannon, A. Markiel, O. Ozier, N. S. Baliga, J. T. Wang, D. Ramage, et al., Cytoscape: A software environment for integrated models of biomolecular interaction networks, Genome Res., 13 (2003), 2498-2504. doi: 10.1101/gr.1239303
![]() |
[21] |
V. Vianahuete, J. J. Fuster, Potential therapeutic value of interleukin 1b-targeted strategies in atherosclerotic cardiovascular disease, Rev. Esp. Cardiol., 72 (2019), 760-766. doi: 10.1016/j.recesp.2019.02.021
![]() |
[22] |
R. Zorcpleskovic, A. Pleskovic, O. Vraspirporenta, M. Zorc, A. Milutinovic, Immune cells and vasa vasorum in the tunica media of atherosclerotic coronary arteries, Bosn. J. Basic Med. Sci., 18 (2018), 240-245. doi: 10.17305/bjbms.2018.2951
![]() |
[23] |
D. A. Chistiakov, A. N. Orekhov, Y. V. Bobryshev, Immune-inflammatory responses in atherosclerosis: Role of an adaptive immunity mainly driven by T and B cells, Immunobiology, 221 (2016), 1014-1033. doi: 10.1016/j.imbio.2016.05.010
![]() |
[24] | X. Zhou, S. Stemme, G. K. Hansson, Evidence for a local immune response in atherosclerosis, CD4+ T cells infiltrate lesions of apolipoprotein-E-deficient mice, Am. J. pathol., 149 (1996), 359. |
[25] |
C. Cochain, M. Koch, S. M. Chaudhari, M. Busch, J. Pelisek, L. Boon, et al., CD8+ T cells regulate monopoiesis and circulating Ly6C-high monocyte levels in atherosclerosis in mice, Circ. Res., 117 (2015), 244-253. doi: 10.1161/CIRCRESAHA.117.304611
![]() |
[26] |
T. Kimura, K. Tse, A. Sette, K. Ley, Vaccination to modulate atherosclerosis, Autoimmunity, 48 (2015), 152-160. doi: 10.3109/08916934.2014.1003641
![]() |
[27] |
M. Katayama, K. Ota, N. Nagimiura, N. Ohno, N. Yabuta, H. Nojima, et al., Ficolin-1 is a promising therapeutic target for autoimmune diseases, Int. Immunol., 31 (2019), 23-32. doi: 10.1093/intimm/dxy056
![]() |
[28] |
S. J. Catarino, F. A. Andrade, A. B. W. Boldt, L. Guilherme, I. J. Messias-Reason, Sickening or healing the heart? The association of ficolin-1 and rheumatic fever, Front. Immunol., 9 (2018), 3009. doi: 10.3389/fimmu.2018.03009
![]() |
[29] |
P. Libby, Interleukin-1 beta as a target for atherosclerosis therapy: Biological basis of cantos and beyond, J. Am. Coll. Cardiol., 70 (2017), 2278-2289. doi: 10.1016/j.jacc.2017.09.028
![]() |
[30] |
M. R. Alexander, M. Murgai, C. W. Moehle, G. K. Owens, Interleukin-1β modulates smooth muscle cell phenotype to a distinct inflammatory state relative to PDGF-DD via NF-κB-dependent mechanisms, Physiol. Genom., 44 (2012), 417-429. doi: 10.1152/physiolgenomics.00160.2011
![]() |
[31] |
V. Sorokin, C. C. Woo, Role of Serpina3 in vascular biology, Int. J. Cardiol., 304 (2020), 154-155. doi: 10.1016/j.ijcard.2019.12.030
![]() |
[32] |
L. Zhao, M. Zheng, Z. Guo, K. Li, Y. Liu, M. Chen, et al., Circulating Serpina3 levels predict the major adverse cardiac events in patients with myocardial infarction, Int. J. Cardiol., 300 (2020), 34-38. doi: 10.1016/j.ijcard.2019.08.034
![]() |
[33] |
D. Wagsater, D. X. Johansson, V. Fontaine, E. Vorkapic, A. Backlund, A. Razuvaev, et al., Serine protease inhibitor A3 in atherosclerosis and aneurysm disease, Int. J. Mol. Med., 30 (2012), 288-294. doi: 10.3892/ijmm.2012.994
![]() |
[34] |
A. J. Horvath, J. A. Irving, J. Rossjohn, R. H. Law, S. P. Bottomley, N. S. Quinsey, et al., The murine orthologue of human antichymotrypsin: a structural paradigm for clade A3 serpins, J. Biol. Chem., 280 (2005), 43168-43178. doi: 10.1074/jbc.M505598200
![]() |
1. | Anjana Soman, S. Asha Nair, Unfolding the cascade of SERPINA3: Inflammation to cancer, 2022, 1877, 0304419X, 188760, 10.1016/j.bbcan.2022.188760 | |
2. | Wei Xiong, Shiyan Feng, Hong Wang, Song Qing, Yong Yang, Yanhua Zhao, Zhongbo Zeng, Jian Gong, Identification of candidate genes and pathways in limonin-mediated cardiac repair after myocardial infarction, 2021, 142, 07533322, 112088, 10.1016/j.biopha.2021.112088 | |
3. | Shuaifei Pan, Yue Li, Haokun He, Shuguang Cheng, Jiang Li, Janak L. Pathak, Identification of ferroptosis, necroptosis, and pyroptosis-associated genes in periodontitis-affected human periodontal tissue using integrated bioinformatic analysis, 2023, 13, 1663-9812, 10.3389/fphar.2022.1098851 | |
4. | Yihong Yin, Zhaohong Xie, Dong Chen, Hao Guo, Min Han, Zhengyu Zhu, Jianzhong Bi, Integrated investigation of DNA methylation, gene expression and immune cell population revealed immune cell infiltration associated with atherosclerotic plaque formation, 2022, 15, 1755-8794, 10.1186/s12920-022-01259-z | |
5. | Ruoyu Dong, Jikuan Li, Guangwei Jiang, Ning Han, Yaochao Zhang, Xiaoming Shi, Novel immune cell infiltration-related biomarkers in atherosclerosis diagnosis, 2023, 11, 2167-8359, e15341, 10.7717/peerj.15341 | |
6. | Xiaocheng Li, Ming Qiao, Yan Zhou, Yan Peng, Gang Wen, Chenchen Xie, Yamei Zhang, Modulating the RPS27A/PSMD12/NF-κB pathway to control immune response in mouse brain ischemia-reperfusion injury, 2024, 30, 1528-3658, 10.1186/s10020-024-00870-3 |