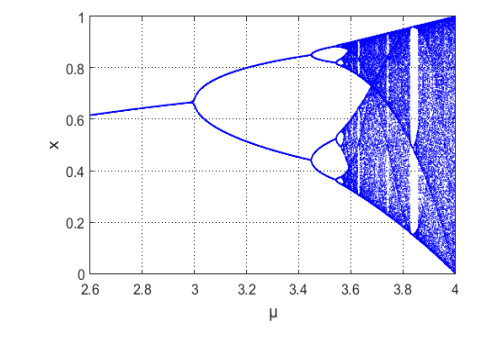
Nanofiber mats can be produced by electrospinning from diverse polymers and polymer blends as well as with embedded ceramics, metals, etc. The large surface-to-volume ratio makes such nanofiber mats a well-suited substrate for tissue engineering and other cell growth experiments. Cell growth, however, is not only influenced by the substrate morphology, but also by the sterilization process applied before the experiment as well as by the chemical composition of the fibers. A former study showed that cell growth and adhesion are supported by polyacrylonitrile/gelatin nanofiber mats, while both factors are strongly reduced on pure polyacrylonitrile (PAN) nanofibers. Here we report on the influence of different PAN blends on cell growth and adhesion. Our study shows that adding ZnO to the PAN spinning solution impedes cell growth, while addition of maltodextrin/pea protein or casein/gelatin supports cell growth and adhesion.
Citation: Daria Wehlage, Hannah Blattner, Al Mamun, Ines Kutzli, Elise Diestelhorst, Anke Rattenholl, Frank Gudermann, Dirk Lütkemeyer, Andrea Ehrmann. Cell growth on electrospun nanofiber mats from polyacrylonitrile (PAN) blends[J]. AIMS Bioengineering, 2020, 7(1): 43-54. doi: 10.3934/bioeng.2020004
[1] | Ziyu Jin, Ning Li . Diagnosis of each main coronary artery stenosis based on whale optimization algorithm and stacking model. Mathematical Biosciences and Engineering, 2022, 19(5): 4568-4591. doi: 10.3934/mbe.2022211 |
[2] | Chengkang Li, Ran Wei, Yishen Mao, Yi Guo, Ji Li, Yuanyuan Wang . Computer-aided differentiates benign from malignant IPMN and MCN with a novel feature selection algorithm. Mathematical Biosciences and Engineering, 2021, 18(4): 4743-4760. doi: 10.3934/mbe.2021241 |
[3] | Jun Gao, Qian Jiang, Bo Zhou, Daozheng Chen . Convolutional neural networks for computer-aided detection or diagnosis in medical image analysis: An overview. Mathematical Biosciences and Engineering, 2019, 16(6): 6536-6561. doi: 10.3934/mbe.2019326 |
[4] | Guanyu Wang . The Effects of Affinity Mediated Clonal Expansion of Premigrant Thymocytes on the Periphery T-Cell Repertoire. Mathematical Biosciences and Engineering, 2005, 2(1): 153-168. doi: 10.3934/mbe.2005.2.153 |
[5] | Yingjian Yang, Wei Li, Yingwei Guo, Nanrong Zeng, Shicong Wang, Ziran Chen, Yang Liu, Huai Chen, Wenxin Duan, Xian Li, Wei Zhao, Rongchang Chen, Yan Kang . Lung radiomics features for characterizing and classifying COPD stage based on feature combination strategy and multi-layer perceptron classifier. Mathematical Biosciences and Engineering, 2022, 19(8): 7826-7855. doi: 10.3934/mbe.2022366 |
[6] | Jiahao Zhang, Zhengming Gao, Suruo Li, Juan Zhao, Wenguang Song . Improved intelligent clonal optimizer based on adaptive parameter strategy. Mathematical Biosciences and Engineering, 2022, 19(10): 10275-10315. doi: 10.3934/mbe.2022481 |
[7] | Jingyao Liu, Qinghe Feng, Yu Miao, Wei He, Weili Shi, Zhengang Jiang . COVID-19 disease identification network based on weakly supervised feature selection. Mathematical Biosciences and Engineering, 2023, 20(5): 9327-9348. doi: 10.3934/mbe.2023409 |
[8] | Xiwen Qin, Shuang Zhang, Dongmei Yin, Dongxue Chen, Xiaogang Dong . Two-stage feature selection for classification of gene expression data based on an improved Salp Swarm Algorithm. Mathematical Biosciences and Engineering, 2022, 19(12): 13747-13781. doi: 10.3934/mbe.2022641 |
[9] | Binju Saju, Neethu Tressa, Rajesh Kumar Dhanaraj, Sumegh Tharewal, Jincy Chundamannil Mathew, Danilo Pelusi . Effective multi-class lungdisease classification using the hybridfeature engineering mechanism. Mathematical Biosciences and Engineering, 2023, 20(11): 20245-20273. doi: 10.3934/mbe.2023896 |
[10] | Kun Lan, Jianzhen Cheng, Jinyun Jiang, Xiaoliang Jiang, Qile Zhang . Modified UNet++ with atrous spatial pyramid pooling for blood cell image segmentation. Mathematical Biosciences and Engineering, 2023, 20(1): 1420-1433. doi: 10.3934/mbe.2023064 |
Nanofiber mats can be produced by electrospinning from diverse polymers and polymer blends as well as with embedded ceramics, metals, etc. The large surface-to-volume ratio makes such nanofiber mats a well-suited substrate for tissue engineering and other cell growth experiments. Cell growth, however, is not only influenced by the substrate morphology, but also by the sterilization process applied before the experiment as well as by the chemical composition of the fibers. A former study showed that cell growth and adhesion are supported by polyacrylonitrile/gelatin nanofiber mats, while both factors are strongly reduced on pure polyacrylonitrile (PAN) nanofibers. Here we report on the influence of different PAN blends on cell growth and adhesion. Our study shows that adding ZnO to the PAN spinning solution impedes cell growth, while addition of maltodextrin/pea protein or casein/gelatin supports cell growth and adhesion.
Lung cancer is one of the highest rates of morbidity and mortality in patients among the worldwide, and an effective approach for lung nodule detection and classification is very important for the early computer-aided diagnosis of lung cancer [1,2]. In this regard, the methods based on artificial intelligence have been proposed and developed, such as artificial neural network [3], evolutionary computation [4], and artificial immune system (AIS) [5]. Notably, AIS is inspired by human immune system, which has the characteristics of adaptability, robustness, homeostasis, memory, and immunity. Inspired by the human immune system, some new modes, algorithms, technologies and theoretical understandings were presented in the literation [6,7,8]. Feature selection is one of the most important tasks in many engineering problems. Importantly, AIS has a better performance in feature selection, which has the advantages of global optimization, parallel computing, noise tolerance, etc. It is widely applied in scientific computation and solving engineering problems of the existing literatures [8,9,10]. The feature selection algorithms based on immune-inspired have the advantage of parallel computing in multi-dimensional space.
Computer-aided lung cancer diagnosis mainly includes lung parenchyma extraction, the region of interest segmentation, the feature extraction and classification. In the image processing, feature selection and classification are important issues for the early detection of lung cancers [11,12,13,14]. In the past few years, many different models and approaches were designed for feature selection and classification, each with its own strengths and weaknesses. For instance, the method of kernel optimization was used for feature selection in the high-dimensional data, experimental results demonstrated the efficiency and accuracy [15]. The fuzzy clustering and hybrid intelligent methods were used for the diagnosis of pulmonary nodules [16]. In addition, Froz et al. [4] used an expert system for lung nodule classification, obtained an accuracy of 94.3% and a specificity of 94.78%. Lu et al. [5] used the genetic algorithm for feature selection, and support vector machine (SVM) for pulmonary nodule classification, obtained an accuracy of 99%. Similarly, Deep learning methods also have good performance in feature selection and classification. For example, the deep reinforcement learning method was adopted for lung nodule detection and obtained the accuracy of 64.4% [17]. In order to improve the accuracy of classification, the deep learning and swarm intelligence of computational intelligence techniques were used to develop types of nodule detection and classification, which achieved up to 93.71% accuracy [18]. In other respects, a novel convolutional neural networks with carefully designed loss function regularization was proposed for thyroid nodule detection in the ultrasound images, the proposed model can detect various types of thyroid nodules [19].
From the analysis of literature, it was observed that most of the studies thought that feature selection and classification were the independent processes, whereupon the feature selection and classification were done separately. Although the process is the local optimization, the final results are not global optimum. Actually, the processes are closely related and mutually influential, which could be performed simultaneously. In this regard, many methods have been proposed. For example, the genetic algorithm was used for feature selection and the SVM parameter optimization [20]. But genetic algorithm has an issue of the degradation phenomenon in the solving process of global optimal, which is easily premature convergence. Here, an immune algorithm can solve the problem by produces excellent antibodies through the memory function. However, immune algorithm also has some shortcomings that the initial population is insufficient diversity, and the variation scale is big or small. To solving these issues, tremendous research efforts have done in the past few years. For instance, Jordehi [21] introduced the chaotic strategies into the immune algorithm and designed to solve the global continuous optimization problems. Wu et al. [22] used the chaotic producers to replace the random generators and used the parallel mutation strategy for the hypermutation of antibodies. Liang et al. [23] used the logistic mapping to generate the initial antibody populations for the diversity of antibodies, etc. While most of the approaches thought that the value of a control factor of the chaotic motion is randomly. Recently, we found that it takes an appropriate value of the control factor will generate the exciting results.
In this work, we focused on improving the performance of immune clonal optimization algorithm for pulmonary nodule classification in chest CT images. Therefore, the method of intelligent immune clonal selection is proposed and developed by the population diversity and intelligent mutation. First, according to the mechanism and characteristics of chaotic motion, the proposed method utilizes the characteristics of chaotic motion and selects the control factor of optimal chaotic state, to generate the initial population with randomness and ergodicity. Second, the proposed method solved the singleness problem of the initial population of immune algorithm. Then based on the characteristics of the Gaussian mutation operator with a small scale, and Cauchy mutation operator with a big scale, the intelligent mutation strategy is developed, and the control factor of intelligent mutation is designed. Especially, the intelligent Gauss-Cauchy hybrid mutation operator is designed and implemented. Additionally, the Hessian information and multi-scale reverse LoG (Hessian-MRLoG) method were used to detect and locate the lesion area in the image preprocessing, and the candidate nodule areas are extracted by an algorithm of the lung nodule segmentation. Therefore, the computational amount of feature extraction and classification is greatly reduced, the data quality is improved and the computational complexity is reduced.
The remainder of this paper is organized as follows. Section 2 describes the method and approach of the proposed intelligent immune clonal for feature selection and parameter optimization. Section 3, the experimental results obtained by the proposed method. Lastly, Section 4 presents the final remarks of this work.
The chaotic mapping was adopted for antibody population initialization in the immune clonal selection algorithm. According to the theoretical study of population models, the logistic mapping model is described as follows:
xn+1=μxn(1−xn),n=1,2,... | (1) |
where μ is a regulation factor of the chaotic behavioral and μ > 0, x(n) is the chaotic variable and n is the number of iterations. Let the initial condition x0∈[0, 1], μ∈[0, 4], then the diagram of chaotic motion bifurcation is shown in Figure 1.
From Figure 1, if 0 < μ < 3, then the chaotic motion shows single-cycle sequence converges. If 3 < μ < 3.449, then the chaotic motion converges with a period of 2 times. If 3.449 < μ < 3.57, then the chaotic motion converges with a 4-fold period. If 3.57 < μ < 4, the chaotic motion comes into a chaotic state. If μ = 4, then the system enters a completely chaotic state. Based on the analysis, it is shown that a system evolves into chaotic state when the regulation factor 3.57 < μ ≤ 4. Therefore, let the initial conditions of x0∈[0, 1], μ∈[0, 4], and μ = 4, n = 200, then the random sequence is generated by the logistic model, which is shown in Figure 2.
Figure 2 shows the ergodicity of the logistic mapping with a suitable regulation factor, which was generated an initial antibody population with diversity. Thus, we utilize the characteristics of chaotic motion and select the regulation factor of optimal chaotic state, to generate an initial antibody population with randomness and ergodicity. It solved the singleness problem of the initial population generation of the immune clonal selection algorithm.
According to the literature [24], the combination of Gauss-Cauchy is written as:
fgc=k⋅(fgaussian(μ,σ)+fcauchy(x0,γ)) | (2) |
where k is an adjustment factor. fgaussian (μ, σ) is a Gaussian distribution function, where σ is the standard deviation and σ > 0, μ is the mathematical expectation. fcauchy (x0, γ) is a Cauchy distribution function, where x0 is the length setting of x-axis, γ is a window scale, and x∈(-∞, +∞). Let k = 1/2, μ = 0, σ = 1; x0 = 0, γ = 1, respectively, then the average distribution curve of Gauss-Cauchy combination is shown in Figure 3.
Therefore, the hybrid Gauss-Cauchy mutation operator is described as follows:
x′=x+k⋅(fgaussian(μ,σ)+fcauchy(x0,γ)) | (3) |
More importantly, we designed an intelligent strategy for the mutation, which is described below: In early phase, the mutation rate has the characteristics of large-scale variation; in the intermediate phase, the mutation rate has a middle-scale variation; in the late stage, the mutation rate has a small-scale variation. Thus, the mutation rate reduced gradually with the increase of evolutionary iterations. The mutation rate is designed by:
v=1−(t/tmax) | (4) |
where t is the current evolutionary iterations, tmax is the maximum iterations. The mutation rate of ν is a monotonically decreasing function, and ν∈[0, 1]. Consequently, the intelligent mutation operator (IMO) is given as:
x′=x+v⋅k⋅(fgaussian(μ,σ)+fcauchy(x0,γ)) | (5) |
where k is an adjustment factor, fgaussian (μ, σ) is a Gaussian distribution function, fcauchy (x0, γ) is a Cauchy distribution function. With the guidance of intelligent mutation strategy, the IMO not only has the local convergence characteristics of Gaussian mutation, but also the global convergence characteristics of Cauchy mutation.
The compound features were extracted in this work from three aspects, which include the intensity features, shape features, and texture features [25]. The character representation is listed in Table 1.Though the feature combination, 26 feature vectors were extracted for feature selection. Then the 26-dimensional feature space was constructed.
Classification | Feature name | Number | Formulas |
I.The intensity features (f1-f5) | Mean value | f1 | ∑Li=1[i⋅I(i)] |
Variance | f2 | ∑Li=1[(i−μ)2⋅I(i)] | |
Deviation | f3 | (1/σ2)⋅∑Li=1[(i−μ)3⋅I(i)] | |
Energy | f4 | ∑Li=1I2(i) | |
Gray-scale entropy | f5 | ∑Li=1[I(i)⋅log2I(i)] | |
II. The shape features (f6-f10) | Circularity | f6 | P02/S0 |
Compactness | f7 | 4π·Area/(Circumference)2 | |
Elongation | f8 | rmax / rmin | |
Convex degree | f9 | Area / Convex_Area | |
Rectangularity | f10 | Wr/ Lr | |
Compactness | f11 | rinside / routside | |
Moment | f12 | ∑Mi=1∑Nj=1[I2(i,j)/(1+|i−j|)] | |
III.Text features (f1-f14) | Angular second moment | f13 | ∑Ngi=1∑Ngi=1[p2(i,j)] |
Contrast | f14 | ∑Ngn=1n2{∑Ngi=1∑Ngj=1p(i,j)} | |
Correlation | f15 | (σxσy)−1⋅∑Ngi=1∑Ngi=1{(ij)p(i,j)−μxμy} | |
Variance | f16 | ∑Ngi=1∑Ngj=1{(i−μx)(i−μy)p(i,j)} | |
Inverse difference moment | f17 | ∑Ngi=1∑Ngj=1[1/(1+(i−j)2)⋅p(i,j)] | |
Sum average | f18 | ∑2Ngi=2(ipx+y(i)) | |
Sum variance | f19 | ∑2Ngi=2[(i−f8)2⋅px+y(i)] | |
Sum entropy | f20 | −∑2Ngi=2[px+y(i)⋅log(px+y(i))] | |
Entropy | f21 | −∑Ngi=1∑Ngj=1{p(i,j)⋅log(p(i,j))} | |
Difference variance | f22 | Var(∑Ngi=1∑Ngj=1p(i,j)) | |
Difference entropy | f23 | ∑Ngn=1{px−y(i)log(px−y(i))} | |
Information measures of correlation 1 | f24 | {−∑Ngi=1∑Nsj=1p(i,j)log(p(i,j))+∑Nsi=1∑Nsj=1p(i,j)log(∑Nsj=1p(i,j)⋅∑Nsi=1p(i,j))}⋅{max(∑Nsi=1p(i,j),∑Ngi=1p(i,j))}−1 | |
Information measures of correlation 2 | f25 | {1−exp(2∑Ngi=1∑Ngi=1(px(i)py(j)⋅log(px(i)py(j))+f9)}1/2 | |
Maximal correlation coefficient | f26 | (Second largest eigenvalue of Q)1/2, where Q(i,j)=∑k{p(i,k)p(j,k)/(px(i)py(j))} |
(1) Radial basis kernel function (RBF)
According to the study of lung nodule model in our previous study [26], we know that the intensity distribution of a lung nodule in the CT image, gradually increases from the periphery to the center. Therefore, this paper adopts RBF as the kernel function, which has a better performance on nonlinear mapping. A 2-dimensional (2D) Gaussian kernel function is represented by:
K(x,xi)=exp(−‖x−xi‖22σ2) | (6) |
Let γ = 1/(2σ2), then the Eq (6) is instead by a RBF
K(x,xi)=exp(−γ‖x−xi‖2) | (7) |
(2) Chromosome coding method
The antibody coding is the combining process of feature subsets and SVM parameters. An antibody is a result of the combination of feature subsets and SVM parameters. The synchronous optimization of the feature selection and SVM parameter is to find the optimal antibodies. It is noteworthy that an antibody has multiple gene positions, the number of gene positions is equal to the length of the coding string. In the chromosome coding, 1 is a dominant gene, which indicates the selected feature; 0 is a recessive gene, which indicates the unselected feature. In this work, the chromosome coding includes the penalty factor of C, the kernel parameter of r, and the feature set of f. While, the corresponding number of genes are represented by I, J and K, respectively. L denotes the string length, the encoded string is given by (xc1, xc2, …, xci, …, xcI; xγ1, xγ2, …, xγj, …, xγJ; xf1, xf2, …, xfk, …, xfK), and I + J + K = L. The chromosome encoding is shown in Figure 4.
From Figure 4, it should be noted that the first eight bits of (xc1, xc2, …, xci, …, xcI) are the coding of the penalty factor C, the following eight bits of (xγ1, xγ2, …, xγj, …, xγJ) are the coding of the kernel parameter γ, the last 26 bits of (xf1, xf2, …, xfk, …, xfK) are the coding of the feature set f.
(3) The affinity function
Affinity = SVM_Accuracy | (8) |
(4) Antibody selection and cloning
If the population size is N, the probability of antibody fitness is:
pfit=Fitness∑Ni=1Fitness(i) | (9) |
The number of clone is:
Nc(i)=Round(pfit⋅N/i) | (10) |
Additionally, the intelligent mutation was implemented by the proposed intelligent mutation operator. From the above analysis, the method of immune clonal optimization algorithm (ICOA) for parameter optimization in support vector machine (ICOA-SVM) was proposed and developed. The flowchart of the developed method is shown in Figure 5.
It should be noted that the implementation procedure can be obtained from the Figure 5. The input is the candidate feature set of F: F = {(xi, yi)∣x∈FnL}, y∈(1, 2, …, L), i = 1, …, n}. The output are the optimized parameters of (C, γ), and the selected feature subsets of f = {f1, f2, …, fk}, 1 ≤ k ≤ K. The implementation of the proposed algorithm includes the training and the testing stages. In the process of immune clonal optimization, the antibodies with high affinity were selected and cloned, and generated the offspring of Ab′(l) = {Ab(l), Ab1′(l), …, Abi′(l), …, Abn′(l)}, Abi′(l) = (xi1, xi2, xi(Nc-1)), Nc(i) = Round(pfit·N/i). Additionally, the others new individuals were generated by the low affinity antibodies though the mutation of Ab′ = Ab + 0.5·(1 - t/tmax) (fgaussian(0, 1) + fcauchy(0, 1)).
The proposed method was implemented in Matlab (R2015a; MathWorks, Inc., Natick, MA, USA) on a machine with Core i3 M 350 @ 2.27GHz, 6G RAM, 64-bit operating system, the CT images that we adopted were obtained from the international public LIDC database [27]. The 90 scans of CT images with the number of 652 lung nodules were randomly selected from the LIDC database. One scan has the number of 100~400 CT images. Thus, more than 20, 000 images were used in the experiments of this study.
There are several steps for the image processing. First, medical images are usually noisy, especially the low-dose spiral CT images, which mainly have the quantum noise, electronic noise, Gaussian noise, and speckle noise [28]. Therefore, a hybrid filtering strategy was developed, which used the median filter for the isolated noise reduction, and the multi-scale windowed Fourier filtering for the noise reduction [29]. Second, the multi-scale elliptic rolling-ball method, which was proposed and developed in our previous work [30], was used for pulmonary parenchyma extraction. Third, the Hessian-MRLoG method [2] was used for the detection and location of pulmonary nodule candidates, then the candidate nodule areas were extracted by an algorithm of the lung nodule segmentation [29]. Four, the features of the regions of interest (ROI) were extracted to construct the 42-dimensional coding space. Especially, there are 26-dimensional feature vectors in feature subsets, which include 5 gray features, 7 shape features, and 14 texture features. The experimental dataset was used to verify the effectiveness of the proposed method, and the experimental adjustment parameters of the proposed method were shown in Table 2.
Parameters | Name of the parameters | Value |
N | Population size | 60 |
tmax | Maximum iterations | 100 |
L | The length of chromosome genes | 34 |
m | The number of elites | 5 |
Nc_max | Maximum number of clones | 120 |
w | Mutation probability | 0.5 |
ξi | The relaxation variable | 0.5 |
k | The number of k-fold cross | 5 |
Especially, the penalty factor of C∈[0.001, 2.2 × 104], the kernel parameter of γ∈[1 × 10-5, 5]. The initial population was generated by the chaotic mapping. The feature subsets and SVM parameters were selected and optimized by the developed method of ICO. The optimal feature subsets and the best matching parameters were obtained by five times of 5-fold cross-validation. According to the number of selected feature dimensions, the optimal feature subsets and best match parameters are shown in Table 3.
Serial numbers | Parameters of SVM | Selected subsets of features | Accuracy |
1 | C = 3985, γ = 0.0432 | f6, f7, f3, f25, f9 | 0.920 |
2 | C = 6591, γ = 0.0332 | f6, f7, f4, f11, f9, f26, f14 | 0.965 |
3 | C = 6730, γ = 0.0436 | f6, f7, f4, f5, f8, f9, f26, f14 | 0.978 |
4 | C = 8390, γ = 0.0529 | f6, f7, f3, f5, f9, f26, f25, f2, f17, f14 | 0.940 |
5 | C = 8534, γ = 0.0623 | f6, f7, f3, f4, f5, f8, f9, f26, f25, f14, f11, f1, f18 | 0.935 |
In this experiment, the eight features of f6, f7, f4, f5, f8, f9, f26, and f14 constructing an optimal feature subset, and the corresponding parameters of SVM are C = 6730, γ = 0.0436, which obtained the highest accuracy of 97.8%. The experimental results revealed that the accuracy rate is not proportional to the dimensions of selected features. After ten times of 5-fold cross-validation, the relationship between the number of selected features and the classification accuracy is shown in Figure 6.
From Figure 6, the results indicate that it is not the more selected feature dimensions, the higher of the classification accuracy. Actually, it has a better match between the classification accuracy and the number of selected features. In this work, when the dimensions of selected features are 8, the accuracy of classification is the highest.
The combination of the two-classification problems can be represented by a two-class confusion matrix [26]. If the practical situation is a nodule, and the prediction result is also a nodule, then it belongs to the nodule, this situation is the true positive (TP). If the practical situation is a non-nodule, and the prediction result is a nodule, then it belongs to the non-nodule, this situation is the false positive (FP). If the practical situation is a nodule, and the prediction result is a non-nodule, then it belongs to the situation of false negative (FN). If the practical situation is a non-nodule, and the prediction result is a non-nodule, then it belongs to the situation of true negative (TN). Generally speaking, the performance of classification algorithms are commonly evaluated by the receiver operating characteristic (ROC) curve and its area under the curve (AUC) value. The evaluation indicators are shown in Table 4.
Indexes | Implication | Formulas |
Sensitivity | True positive rate | Sens = TP / (TP + FN) |
Specificity | True negative rate | Spec = TN / (TN + FP) |
Accuracy | Accuracy | Acc = (TP + TN) / (TP + TN + FP + FN) |
FPR | False positive rate | FPR = TP / (TP + FP) |
AUC | The area under the characteristic curve | AUC = ∑i{(1-3y(i) / 2-y(i-1) / 2) * (x(i)-x(i-1))} |
The bigger of the value of sensitivity, specificity, accuracy, and AUC, the better the performance of a classifier. Additionally, the lower the value of false positive rate (FPR), the better the performance of a classifier. In this work, at the same experimental conditions, we analyzed the properties of genetic algorithm (GA), immune clone selective algorithm (ICSA), and the proposed ICOA-SVM, respectively. Additionally, the approach of convolutional neural network (CNN) was used for the pulmonary nodule classification at the same experimental dataset. Especially, the initial learning rate is 0.001. After that, each generation of learning rate decays to 0.9 of the previous generation learning rate. After experiments, the experimental results were shown in Table 5.
Methods | Accuracy (%) | Sensitivity (%) | AUC (%) |
GA-SVM | 91.37 | 92.25 | 94.92 |
ICSA-SVM | 93.25 | 94.75 | 96.54 |
CNN | 93.13 | 92.50 | 96.64 |
The proposed | 97.87 | 98.75 | 99.09 |
From Table 5, the proposed method has the best performance among the four approaches. Therefore, the proposed method is superior to the mentioned approaches. Besides, to more clearly represent the classification performance, the ROC was plotted in Figure 7.
As can be seen from Figure 7, the area under the cure value of GA-SVM, ICSA-SVM, CNN, and the proposed are 94.92, 96.54, 96.64, and 99.09%, respectively. The proposed method has the biggest AUC value among the four approaches. According to its analysis, the proposed method is superior to the mentioned methods in the performance of lung nodule classification. Additionally, the performance of the proposed method was also compared with other related approaches, which reported in the literature in the last five years, as summarized in Table 6.
Literatures | Scans | Nodules | Accuracy (%) | Sensitivity (%) | FPs/scan | Dataset |
Lü et al. [3] | 50 | 435 | 84.6 | 88.9 | -- | Private |
Liu et al. [31] | 80 | 978 | 93.2 | 92.4 | 4.5 | LIDC |
Liu et al. [32] | 107 | 252 | -- | 89.4 | 2.0 | LIDC |
Kaya [33] | -- | 439 | 84.7 | 67.4 | -- | LIDC |
Khan et al. [34] | 84 | 103 | 97.5 | 98.9 | -- | LIDC |
Zhang et al. [35] | -- | 353 | 88.6 | 86.3 | -- | LIDC |
Jiang et al. [36] | -- | -- | 90.24 | 92.04 | 11.06 | LIDC |
Santos et al. [14] | 28 | 252 | -- | 90.6 | 1.17 | LIDC |
The proposed | 90 | 652 | 97.87 | 98.75 | 1.52 | LIDC |
The comparison reveals that the proposed method in this work has the highest sensitivity, while the approach reported in another study has the lowest FPR [14]. But, regarding the overall performance, the proposed method in this study has the best performance. Thus, the proposed method is superior to the traditional approaches. Additionally, the Hessian-MRLoG method was used to detect and locate the lesion area, then the candidate nodule areas were extracted by an algorithm of the lung nodule segmentation. As a result, the computational amount of feature extraction and classification is greatly reduced, the data quality is improved and the computational complexity is reduced. Therefore, this study gives a reference for a novel light-weight method of computer-aided diagnosis of pulmonary nodules.
Computer-aided pulmonary nodule diagnosis is an effective approach for the early detection of lung cancers. In this paper, an intelligent immune clonal optimization algorithm is proposed and developed to find the optimal feature subset and best match parameters of the classification algorithm, which makes the feature selection and classification be performed simultaneously. The initial population was generated by the randomness and ergodicity of the chaotic mapping. The singleness problem of the initial population of the immune clonal selection algorithm was solved in this work. The proposed method has the global and local convergence characteristics by the intelligent mutation operator. Therefore, a novel method based on an intelligent immune clonal optimization algorithm for pulmonary nodule classification was proposed and developed in this work. The proposed approaches of this work were used for the classification of 90 scans with 652 nodules, and the results revealed an accuracy of 97.87% with the 1.52 FPs/scan. Consequently, the experimental results demonstrated that the proposed approach can improve the accuracy and reduce the false positive rate in pulmonary nodule classification. This work has the potential for deployment in the early computer-aided diagnosis of lung cancer.
Supported by the grants from the National Natural Science Foundation of China (Grant No. 61673257, 61705127), the construction project of applied undergraduate of Shanghai for the major of electrical engineering and automation of SUES, and the doctoral training foundation of SUES (Grant No. 0232-A3-0100-21-0931).
No potential conflicts of interest were disclosed.
[1] |
Dizge N, Shaulsky E, Karanikola V (2019) Electrospun cellulose nanofibers for superhydrophobic and oleophobic membranes. J Membr Sci 590: 117271. doi: 10.1016/j.memsci.2019.117271
![]() |
[2] |
Pavlova ER, Bagrov DV, Monakhova KZ, et al. (2019) Tuning the properties of electrospun polylactide mats by ethanol treatment. Mater Des 181: 108061. doi: 10.1016/j.matdes.2019.108061
![]() |
[3] | Wang JN, Zhao WW, Wang B, et al. (2017) Multilevel-layer-structured polyamide 6/poly(trimethylene terephthalate) nanofibrous membranes for low-pressure air filtration. J Appl Pol Sci 134: 44716. |
[4] |
Cooper A, Oldinski R, Ma H Y, et al. (2013) Chitosan-based nanofibrous membranes for antibacterial filter applications. Carbohyd Polym 92: 254-259. doi: 10.1016/j.carbpol.2012.08.114
![]() |
[5] |
Banner J, Dautzenberg M, Feldhans T, et al. (2018) Water resistance and morphology of electrospun gelatine blended with citric acid and coconut oil. Tekstilec 61: 129-135. doi: 10.14502/Tekstilec2018.61.129-135
![]() |
[6] |
Grimmelsmann N, Homburg SV, Ehrmann A (2017) Electrospinning chitosan blends for nonwovens with morphologies between nanofiber mat and membrane. IOP Conf Series Mater Sci Eng 213: 012007. doi: 10.1088/1757-899X/213/1/012007
![]() |
[7] |
Wortmann M, Freese N, Sabantina L, et al. (2019) New polymers for needleless electrospinning from low-toxic solvents. Nanomater 9: 52. doi: 10.3390/nano9010052
![]() |
[8] |
Krasonu I, Tarassova E, Malmberg S, et al. (2019) Preparation of fibrous electrospun membranes with activated carbon filler. IOP Conf Series Mater Sci Eng 500: 012022. doi: 10.1088/1757-899X/500/1/012022
![]() |
[9] |
Plamus T, Savest N, Viirsalu M, et al. (2018) The effect of ionic liquids on the mechanical properties of electrospun polyacrylonitrile membranes. Polym Test 71: 335-343. doi: 10.1016/j.polymertesting.2018.09.003
![]() |
[10] |
Sabantina L, Mirasol JR, Cordero T, et al. (2018) Investigation of needleless electrospun PAN nanofiber mats. AIP Conf Proc 1952: 020085. doi: 10.1063/1.5032047
![]() |
[11] |
Wang JH, Cai C, Zhang ZJ, et al. (2020) Electrospun metal-organic frameworks with polyacrylonitrile as precursors to hierarchical porous carbon and composite nanofibers for adsorption and catalysis. Chemosphere 239: 124833. doi: 10.1016/j.chemosphere.2019.124833
![]() |
[12] |
de Oliveira JB, Guerrini LM, dos Santos Conejo L, et al. (2019) Viscoelastic evaluation of epoxy nanocomposite based on carbon nanofiber obtained from electrospinning processing. Polym Bull 76: 6063-6076. doi: 10.1007/s00289-019-02707-0
![]() |
[13] |
Trabelsi M, Mamun A, Klöcker M, et al. (2019) Increased mechanical properties of carbon nanofiber mats for possible medical applications. Fibers 7: 98. doi: 10.3390/fib7110098
![]() |
[14] |
Wang L, Zhang C, Gao F, et al. (2016) Needleless electrospinning for scaled-up production of ultrafine chitosan hybrid nanofibers used for air filtration. RSC Adv 6: 105988-105995. doi: 10.1039/C6RA24557A
![]() |
[15] |
Roche R, Yalcinkaya F (2018) Incorporation of PVDF nanofibre multilayers into functional structure for filtration applications. Nanomater 8: 771. doi: 10.3390/nano8100771
![]() |
[16] |
Lv D, Wang RX, Tang GS, et al. (2019) Ecofriendly electrospun membranes loaded with visible-light-responding nanoparticles for multifunctional usages: highly efficient air filtration, dye scavenging, and bactericidal activity. ACS Appl Mater Interfaces 11: 12880-12889. doi: 10.1021/acsami.9b01508
![]() |
[17] |
Fu QS, Lin G, Chen XD, et al. (2018) Mechanically reinforced PVdF/PMMA/SiO2 composite membrane and its electrochemical properties as a separator in lithium-ion batteries. Energy Technol 6: 144-152. doi: 10.1002/ente.201700347
![]() |
[18] |
Mamun A, Trabelsi M, Klöcker M, et al. (2019) Electrospun nanofiber mats with embedded non-sintered TiO2 for dye sensitized solar cells (DSSCs). Fibers 7: 60. doi: 10.3390/fib7070060
![]() |
[19] |
Xue YY, Guo X, Zhou HF, et al. (2019) Influence of beads-on-string on Na-Ion storage behavior in electrospun carbon nanofibers. Carbon 154: 219-229. doi: 10.1016/j.carbon.2019.08.003
![]() |
[20] |
Mamun A (2019) Review of possible applications of nanofibrous mats for wound dressings. Tekstilec 62: 89-100. doi: 10.14502/Tekstilec2019.62.89-100
![]() |
[21] |
Gao ST, Tang GS, Hua DW, et al. (2019) Stimuli-responsive bio-based polymeric systems and their applications. J Mater Chem B 7: 709-729. doi: 10.1039/C8TB02491J
![]() |
[22] |
Aljawish A, Muniglia L, Chevalot I (2016) Growth of human mesenchymal stem cells (MSCs) on films of enzymatically modified chitosan. Biotechnol Prog 32: 491-500. doi: 10.1002/btpr.2216
![]() |
[23] |
Muzzarelli RAA, EI Mehtedi M, Bottegoni C, et al. (2015) Genipin-crosslinked chitosan gels and scaffolds for tissue engineering and regeneration of cartilage and bone. Mar Drugs 13: 7314-7338. doi: 10.3390/md13127068
![]() |
[24] |
Klinkhammer K, Seiler N, Grafahrend D, et al. (2009) Deposition of electrospun fibers on reactive substrates for in vitro investigations. Tissue Eng Part C 15: 77-85. doi: 10.1089/ten.tec.2008.0324
![]() |
[25] |
Yoshida H, Klinkhammer K, Matsusaki M, et al. (2009) Disulfide-crosslinked electrospun poly(γ-glutamic acid) nonwovens as reduction-responsive scaffolds. Macromol Biosci 9: 568-574. doi: 10.1002/mabi.200800334
![]() |
[26] | Chatel A (2019) A brief history of adherent cell culture: where we come from and where we should go. BioProcess Int 17: 44-49. |
[27] | Whitford WG, Hardy JC, Cadwell JJS (2014) Single-use, continuous processing of primary stem cells. BioProcess Int 12: 26-32. |
[28] | Simon M (2015) Bioreactor design for adherent cell culture. The bolt-on bioreactor project, part 1: volumetric productivity. BioProcess Int 13: 28-33. |
[29] |
Allan SJ, De Bank PA, Ellis MJ (2019) Bioprocess design considerations for cultured meat production with a focus on the expansion bioreactor. Front Sus Food Syst 3: 44. doi: 10.3389/fsufs.2019.00044
![]() |
[30] | GE Healthcare Life Sciences (2013) Microcarrier Cell Culture, Principles and Methods.Available from: http://www.gelifesciences.co.kr/wp-content/uploads/2016/07/023.8_Microcarrier-Cell-Culture.pdf. |
[31] |
Lennaertz A, Knowles S, Drugmand JC, et al. (2013) Viral vector production in the integrity iCELLis single-use fixed-bed bioreactor, from bench-scale to industrial scale. BMC Proc 7: P59. doi: 10.1186/1753-6561-7-S6-P59
![]() |
[32] | Dohogne Y, Collignon F, Drugmand JC, et al. (2019) Scale-X bioreactor for viral vector production. Proof of concept for scalable HEK293 cell growth and adenovirus production, Univercell Application note.Available from: https://www.univercells.com/app/uploads/2019/05/scale-X%E2%84%A2-bioreactor-for-viral-production-Adeno_SFM.pdf. |
[33] |
Drugmand JC, Aghatos S, Schneider YJ, et al. (2007) Growth of mammalian and lepidopteram cells on BioNOC® II disks, a novel macroporous microcarrier. Cell Technology for Cell Products Heidelberg: Springer, 781-784. doi: 10.1007/978-1-4020-5476-1_143
![]() |
[34] |
Wehlage D, Blatter H, Sabantina L, et al. (2019) Sterilization of PAN/gelatin nanofibrous mats for cell growth. Tekstilec 62: 78-88. doi: 10.14502/Tekstilec2019.62.78-88
![]() |
[35] |
Ghasemi A, Imani R, Yousefzadeh M, et al. (2019) Studying the potential application of electrospun polyethylene terephthalate/graphene oxide nanofibers as electroconductive cardiac patc. Macromol Mater Eng 304: 1900187. doi: 10.1002/mame.201900187
![]() |
[36] |
Nekouian S, Sojoodi M, Nadri S (2019) Fabrication of conductive fibrous scaffold for photoreceptor differentiation of mesenchymal stem cell. J Cell Physiol 234: 15800-15808. doi: 10.1002/jcp.28238
![]() |
[37] |
Rahmani A, Nadri S, Kazemi HS, et al. (2019) Conductive electrospun scaffolds with electrical stimulation for neural differentiation of conjunctiva mesenchymal stem cells. Artif Organs 43: 780-790. doi: 10.1111/aor.13425
![]() |
[38] | Kutzli I, Beljo D, Gibis M, et al. (2019) Effect of maltodextrin dextrose equivalent on electrospinnability and glycation reaction of blends with pea protein isolate. Food Biophysics . |
[39] |
Diestelhorst E, Mance F, Mamun A, et al. (2020) Chemical and morphological modification of PAN nanofiber mats by addition of casein after electrospinning, stabilization and carbonization. Tekstilec 63: 38-49. doi: 10.14502/Tekstilec2020.63.38-49
![]() |
[40] |
Möller J, Korte K, Pörtner R, et al. (2018) Model-based identification of cell-cycle-dependent metabolism and putative autocrine effects in antibody producing CHO cell culture. Biotechnol Bioeng 115: 2996-3008. doi: 10.1002/bit.26828
![]() |
[41] |
Wippermann A, Rupp O, Brinkrolf K, et al. (2015) The DNA methylation landscape of Chinese hamster ovary (CHO) DP-12 cells. J Biotechnol 199: 38-46. doi: 10.1016/j.jbiotec.2015.02.014
![]() |
[42] |
Haredy AM, Nishizawa A, Honda K, et al. (2013) Improved antibody production in Chinese hamster ovary cells by ATF4 overexpression. Cytotechnology 65: 993-1002. doi: 10.1007/s10616-013-9631-x
![]() |
[43] |
Bazrafshan Z, Stylios GK (2018) Custom-built electrostatics and supplementary bonding in the design of reinforced Collagen-g-P (methyl methacrylate-co-ethyl acrylate)/nylon 66 core-shell fibers. J Mech Behav Biomed Mater 87: 19-29. doi: 10.1016/j.jmbbm.2018.07.002
![]() |
[44] |
Storck JL, Grothe T, Mamun A, et al. (2020) Orientation of electrospun magnetic nanofibers near conductive areas. Materials 13: 47. doi: 10.3390/ma13010047
![]() |
[45] |
Richter KN, Revelo NH, Seitz KJ, et al. (2018) Glyoxal as an alternative fixative to formaldehyde in immunostaining and super-resolution microscopy. EMBO J 37: 139-159. doi: 10.15252/embj.201695709
![]() |
[46] |
Huang LC, Lin W, Yagami M, et al. (2010) Validation of cell density and viability assays using Cedex automated cell counter. Biologicals 38: 393-400. doi: 10.1016/j.biologicals.2010.01.009
![]() |
[47] |
Sabantina L, Rodríguez-Cano MA, Klöcker M, et al. (2018) Fixing PAN nanofiber mats during stabilization for carbonization and creating novel metal/carbon composites. Polymers 10: 735. doi: 10.3390/polym10070735
![]() |
[48] |
Baek M, Kim MK, Cho HJ, et al. (2011) Factors influencing the cytotoxicity of zinc oxide nanoparticles: particle size and surface charge. J Phys Conf Ser 304: 012044. doi: 10.1088/1742-6596/304/1/012044
![]() |
[49] |
Sing S (2019) Zinc oxide nanoparticles impacts: cytotoxicity, genotoxicity, development toxicity, and neurotoxicity. Toxicol Mech Methods 29: 300-311. doi: 10.1080/15376516.2018.1553221
![]() |
[50] |
Kuebodeaux RE, Bernazzani P, Nguyen TTM (2018) Cytotoxic and membrane cholesterol effects of ultraviolet irradiation and zinc oxide nanoparticles on Chinese hamster ovary cells. Molecules 23: 2979. doi: 10.3390/molecules23112979
![]() |
[51] |
Zukiene R, Snitka V (2015) Zinc oxide nanoparticle and bovine serum albumin interaction andnanoparticles influence on cytotoxicity in vitro. Colloids Surf B 135: 316-323. doi: 10.1016/j.colsurfb.2015.07.054
![]() |
1. | Yudong Zhang, Juan Manuel Gorriz, Deepak Ranjan Nayak, Optimization Algorithms and Machine Learning Techniques in Medical Image Analysis, 2023, 20, 1551-0018, 5917, 10.3934/mbe.2023255 | |
2. | Wei Zhang, Lili An, Xiaojun Li, Feng Chen, Lidong Sun, Xunming Wang, Jie Cai, Adjustment method and energy consumption of centrifugal pump based on intelligent optimization algorithm, 2022, 8, 23524847, 12272, 10.1016/j.egyr.2022.09.031 | |
3. | Iftikhar Naseer, Sheeraz Akram, Tehreem Masood, Arfan Jaffar, Muhammad Adnan Khan, Amir Mosavi, Performance Analysis of State-of-the-Art CNN Architectures for LUNA16, 2022, 22, 1424-8220, 4426, 10.3390/s22124426 | |
4. | XiaoQing Zhang, GuangYu Wang, Shu-Guang Zhao, CapsNet-COVID19: Lung CT image classification method based on CapsNet model, 2022, 19, 1551-0018, 5055, 10.3934/mbe.2022236 | |
5. | Hafiz Muhammad Tayyab Khushi, Tehreem Masood, Arfan Jaffar, Sheeraz Akram, A Novel Approach to Classify Brain Tumor with an Effective Transfer Learning based Deep Learning Model, 2024, 67, 1678-4324, 10.1590/1678-4324-2024231137 | |
6. | Hafiz Muhammad Tayyab Khushi, Tehreem Masood, Arfan Jaffar, Sheeraz Akram, Sohail Masood Bhatti, Performance analysis ofstate‐of‐the‐art CNNarchitectures for brain tumour detection, 2024, 34, 0899-9457, 10.1002/ima.22949 | |
7. | Jintao Wang, Mao Qi, Zhenwu Xiang, Yi Tian, Dongbing Tong, SaraNet: Semantic aggregation reverse attention network for pulmonary nodule segmentation, 2024, 177, 00104825, 108674, 10.1016/j.compbiomed.2024.108674 | |
8. | Wenfeng Wang, Qi Mao, Yi Tian, Yan Zhang, Zhenwu Xiang, Lijia Ren, FMD-UNet: fine-grained feature squeeze and multiscale cascade dilated semantic aggregation dual-decoder UNet for COVID-19 lung infection segmentation from CT images, 2024, 10, 2057-1976, 055031, 10.1088/2057-1976/ad6f12 | |
9. | Tolga Hayit, Ali Endes, Fatma Hayit, The severity level classification of Fusarium wilt of chickpea by pre-trained deep learning models, 2023, 2239-7264, 10.1007/s42161-023-01520-z | |
10. | Hafiz Muhammad Tayyab Khushi, Tehreem Masood, Arfan Jaffar, Muhammad Rashid, Sheeraz Akram, Improved Multiclass Brain Tumor Detection via Customized Pretrained EfficientNetB7 Model, 2023, 11, 2169-3536, 117210, 10.1109/ACCESS.2023.3325883 | |
11. | Zhenwu Xiang, Qi Mao, Jintao Wang, Yi Tian, Yan Zhang, Wenfeng Wang, Dmbg-Net: Dilated multiresidual boundary guidance network for COVID-19 infection segmentation, 2023, 20, 1551-0018, 20135, 10.3934/mbe.2023892 | |
12. | 一展 刘, Classification of Pulmonary Nodules Based on 3D CMFF Deep Network Model, 2024, 13, 2324-8696, 588, 10.12677/MOS.2024.131057 | |
13. | Yan Zhang, Qi Mao, Yi Tian, Wenfeng Wang, Lijia Ren, Haibo Li, Dual-path information enhanced pyramid Unet for COVID-19 lung infection segmentation, 2025, 142, 09521976, 109977, 10.1016/j.engappai.2024.109977 | |
14. | Yi Tian, Qi Mao, Wenfeng Wang, Yan Zhang, Hierarchical agent transformer network for COVID-19 infection segmentation, 2025, 11, 2057-1976, 025055, 10.1088/2057-1976/adbafa |
Classification | Feature name | Number | Formulas |
I.The intensity features (f1-f5) | Mean value | f1 | ∑Li=1[i⋅I(i)] |
Variance | f2 | ∑Li=1[(i−μ)2⋅I(i)] | |
Deviation | f3 | (1/σ2)⋅∑Li=1[(i−μ)3⋅I(i)] | |
Energy | f4 | ∑Li=1I2(i) | |
Gray-scale entropy | f5 | ∑Li=1[I(i)⋅log2I(i)] | |
II. The shape features (f6-f10) | Circularity | f6 | P02/S0 |
Compactness | f7 | 4π·Area/(Circumference)2 | |
Elongation | f8 | rmax / rmin | |
Convex degree | f9 | Area / Convex_Area | |
Rectangularity | f10 | Wr/ Lr | |
Compactness | f11 | rinside / routside | |
Moment | f12 | ∑Mi=1∑Nj=1[I2(i,j)/(1+|i−j|)] | |
III.Text features (f1-f14) | Angular second moment | f13 | ∑Ngi=1∑Ngi=1[p2(i,j)] |
Contrast | f14 | ∑Ngn=1n2{∑Ngi=1∑Ngj=1p(i,j)} | |
Correlation | f15 | (σxσy)−1⋅∑Ngi=1∑Ngi=1{(ij)p(i,j)−μxμy} | |
Variance | f16 | ∑Ngi=1∑Ngj=1{(i−μx)(i−μy)p(i,j)} | |
Inverse difference moment | f17 | ∑Ngi=1∑Ngj=1[1/(1+(i−j)2)⋅p(i,j)] | |
Sum average | f18 | ∑2Ngi=2(ipx+y(i)) | |
Sum variance | f19 | ∑2Ngi=2[(i−f8)2⋅px+y(i)] | |
Sum entropy | f20 | −∑2Ngi=2[px+y(i)⋅log(px+y(i))] | |
Entropy | f21 | −∑Ngi=1∑Ngj=1{p(i,j)⋅log(p(i,j))} | |
Difference variance | f22 | Var(∑Ngi=1∑Ngj=1p(i,j)) | |
Difference entropy | f23 | ∑Ngn=1{px−y(i)log(px−y(i))} | |
Information measures of correlation 1 | f24 | {−∑Ngi=1∑Nsj=1p(i,j)log(p(i,j))+∑Nsi=1∑Nsj=1p(i,j)log(∑Nsj=1p(i,j)⋅∑Nsi=1p(i,j))}⋅{max(∑Nsi=1p(i,j),∑Ngi=1p(i,j))}−1 | |
Information measures of correlation 2 | f25 | {1−exp(2∑Ngi=1∑Ngi=1(px(i)py(j)⋅log(px(i)py(j))+f9)}1/2 | |
Maximal correlation coefficient | f26 | (Second largest eigenvalue of Q)1/2, where Q(i,j)=∑k{p(i,k)p(j,k)/(px(i)py(j))} |
Parameters | Name of the parameters | Value |
N | Population size | 60 |
tmax | Maximum iterations | 100 |
L | The length of chromosome genes | 34 |
m | The number of elites | 5 |
Nc_max | Maximum number of clones | 120 |
w | Mutation probability | 0.5 |
ξi | The relaxation variable | 0.5 |
k | The number of k-fold cross | 5 |
Serial numbers | Parameters of SVM | Selected subsets of features | Accuracy |
1 | C = 3985, γ = 0.0432 | f6, f7, f3, f25, f9 | 0.920 |
2 | C = 6591, γ = 0.0332 | f6, f7, f4, f11, f9, f26, f14 | 0.965 |
3 | C = 6730, γ = 0.0436 | f6, f7, f4, f5, f8, f9, f26, f14 | 0.978 |
4 | C = 8390, γ = 0.0529 | f6, f7, f3, f5, f9, f26, f25, f2, f17, f14 | 0.940 |
5 | C = 8534, γ = 0.0623 | f6, f7, f3, f4, f5, f8, f9, f26, f25, f14, f11, f1, f18 | 0.935 |
Indexes | Implication | Formulas |
Sensitivity | True positive rate | Sens = TP / (TP + FN) |
Specificity | True negative rate | Spec = TN / (TN + FP) |
Accuracy | Accuracy | Acc = (TP + TN) / (TP + TN + FP + FN) |
FPR | False positive rate | FPR = TP / (TP + FP) |
AUC | The area under the characteristic curve | AUC = ∑i{(1-3y(i) / 2-y(i-1) / 2) * (x(i)-x(i-1))} |
Methods | Accuracy (%) | Sensitivity (%) | AUC (%) |
GA-SVM | 91.37 | 92.25 | 94.92 |
ICSA-SVM | 93.25 | 94.75 | 96.54 |
CNN | 93.13 | 92.50 | 96.64 |
The proposed | 97.87 | 98.75 | 99.09 |
Literatures | Scans | Nodules | Accuracy (%) | Sensitivity (%) | FPs/scan | Dataset |
Lü et al. [3] | 50 | 435 | 84.6 | 88.9 | -- | Private |
Liu et al. [31] | 80 | 978 | 93.2 | 92.4 | 4.5 | LIDC |
Liu et al. [32] | 107 | 252 | -- | 89.4 | 2.0 | LIDC |
Kaya [33] | -- | 439 | 84.7 | 67.4 | -- | LIDC |
Khan et al. [34] | 84 | 103 | 97.5 | 98.9 | -- | LIDC |
Zhang et al. [35] | -- | 353 | 88.6 | 86.3 | -- | LIDC |
Jiang et al. [36] | -- | -- | 90.24 | 92.04 | 11.06 | LIDC |
Santos et al. [14] | 28 | 252 | -- | 90.6 | 1.17 | LIDC |
The proposed | 90 | 652 | 97.87 | 98.75 | 1.52 | LIDC |
Classification | Feature name | Number | Formulas |
I.The intensity features (f1-f5) | Mean value | f1 | ∑Li=1[i⋅I(i)] |
Variance | f2 | ∑Li=1[(i−μ)2⋅I(i)] | |
Deviation | f3 | (1/σ2)⋅∑Li=1[(i−μ)3⋅I(i)] | |
Energy | f4 | ∑Li=1I2(i) | |
Gray-scale entropy | f5 | ∑Li=1[I(i)⋅log2I(i)] | |
II. The shape features (f6-f10) | Circularity | f6 | P02/S0 |
Compactness | f7 | 4π·Area/(Circumference)2 | |
Elongation | f8 | rmax / rmin | |
Convex degree | f9 | Area / Convex_Area | |
Rectangularity | f10 | Wr/ Lr | |
Compactness | f11 | rinside / routside | |
Moment | f12 | ∑Mi=1∑Nj=1[I2(i,j)/(1+|i−j|)] | |
III.Text features (f1-f14) | Angular second moment | f13 | ∑Ngi=1∑Ngi=1[p2(i,j)] |
Contrast | f14 | ∑Ngn=1n2{∑Ngi=1∑Ngj=1p(i,j)} | |
Correlation | f15 | (σxσy)−1⋅∑Ngi=1∑Ngi=1{(ij)p(i,j)−μxμy} | |
Variance | f16 | ∑Ngi=1∑Ngj=1{(i−μx)(i−μy)p(i,j)} | |
Inverse difference moment | f17 | ∑Ngi=1∑Ngj=1[1/(1+(i−j)2)⋅p(i,j)] | |
Sum average | f18 | ∑2Ngi=2(ipx+y(i)) | |
Sum variance | f19 | ∑2Ngi=2[(i−f8)2⋅px+y(i)] | |
Sum entropy | f20 | −∑2Ngi=2[px+y(i)⋅log(px+y(i))] | |
Entropy | f21 | −∑Ngi=1∑Ngj=1{p(i,j)⋅log(p(i,j))} | |
Difference variance | f22 | Var(∑Ngi=1∑Ngj=1p(i,j)) | |
Difference entropy | f23 | ∑Ngn=1{px−y(i)log(px−y(i))} | |
Information measures of correlation 1 | f24 | {−∑Ngi=1∑Nsj=1p(i,j)log(p(i,j))+∑Nsi=1∑Nsj=1p(i,j)log(∑Nsj=1p(i,j)⋅∑Nsi=1p(i,j))}⋅{max(∑Nsi=1p(i,j),∑Ngi=1p(i,j))}−1 | |
Information measures of correlation 2 | f25 | {1−exp(2∑Ngi=1∑Ngi=1(px(i)py(j)⋅log(px(i)py(j))+f9)}1/2 | |
Maximal correlation coefficient | f26 | (Second largest eigenvalue of Q)1/2, where Q(i,j)=∑k{p(i,k)p(j,k)/(px(i)py(j))} |
Parameters | Name of the parameters | Value |
N | Population size | 60 |
tmax | Maximum iterations | 100 |
L | The length of chromosome genes | 34 |
m | The number of elites | 5 |
Nc_max | Maximum number of clones | 120 |
w | Mutation probability | 0.5 |
ξi | The relaxation variable | 0.5 |
k | The number of k-fold cross | 5 |
Serial numbers | Parameters of SVM | Selected subsets of features | Accuracy |
1 | C = 3985, γ = 0.0432 | f6, f7, f3, f25, f9 | 0.920 |
2 | C = 6591, γ = 0.0332 | f6, f7, f4, f11, f9, f26, f14 | 0.965 |
3 | C = 6730, γ = 0.0436 | f6, f7, f4, f5, f8, f9, f26, f14 | 0.978 |
4 | C = 8390, γ = 0.0529 | f6, f7, f3, f5, f9, f26, f25, f2, f17, f14 | 0.940 |
5 | C = 8534, γ = 0.0623 | f6, f7, f3, f4, f5, f8, f9, f26, f25, f14, f11, f1, f18 | 0.935 |
Indexes | Implication | Formulas |
Sensitivity | True positive rate | Sens = TP / (TP + FN) |
Specificity | True negative rate | Spec = TN / (TN + FP) |
Accuracy | Accuracy | Acc = (TP + TN) / (TP + TN + FP + FN) |
FPR | False positive rate | FPR = TP / (TP + FP) |
AUC | The area under the characteristic curve | AUC = ∑i{(1-3y(i) / 2-y(i-1) / 2) * (x(i)-x(i-1))} |
Methods | Accuracy (%) | Sensitivity (%) | AUC (%) |
GA-SVM | 91.37 | 92.25 | 94.92 |
ICSA-SVM | 93.25 | 94.75 | 96.54 |
CNN | 93.13 | 92.50 | 96.64 |
The proposed | 97.87 | 98.75 | 99.09 |
Literatures | Scans | Nodules | Accuracy (%) | Sensitivity (%) | FPs/scan | Dataset |
Lü et al. [3] | 50 | 435 | 84.6 | 88.9 | -- | Private |
Liu et al. [31] | 80 | 978 | 93.2 | 92.4 | 4.5 | LIDC |
Liu et al. [32] | 107 | 252 | -- | 89.4 | 2.0 | LIDC |
Kaya [33] | -- | 439 | 84.7 | 67.4 | -- | LIDC |
Khan et al. [34] | 84 | 103 | 97.5 | 98.9 | -- | LIDC |
Zhang et al. [35] | -- | 353 | 88.6 | 86.3 | -- | LIDC |
Jiang et al. [36] | -- | -- | 90.24 | 92.04 | 11.06 | LIDC |
Santos et al. [14] | 28 | 252 | -- | 90.6 | 1.17 | LIDC |
The proposed | 90 | 652 | 97.87 | 98.75 | 1.52 | LIDC |