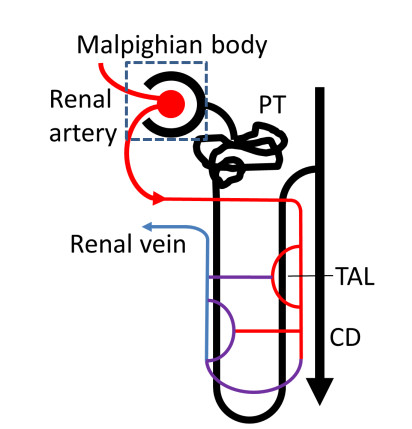
Citation: Niksa Praljak, Shawn D. Ryan, Andrew Resnick. Pulsatile flow through idealized renal tubules: Fluid-structure interaction and dynamic pathologies[J]. Mathematical Biosciences and Engineering, 2020, 17(2): 1787-1807. doi: 10.3934/mbe.2020094
[1] | Nerion Zekaj, Shawn D. Ryan, Andrew Resnick . Fluid-structure interaction modelling of neighboring tubes with primary cilium analysis. Mathematical Biosciences and Engineering, 2023, 20(2): 3677-3699. doi: 10.3934/mbe.2023172 |
[2] | Benchawan Wiwatanapataphee, Yong Hong Wu, Thanongchai Siriapisith, Buraskorn Nuntadilok . Effect of branchings on blood flow in the system of human coronary arteries. Mathematical Biosciences and Engineering, 2012, 9(1): 199-214. doi: 10.3934/mbe.2012.9.199 |
[3] | B. Wiwatanapataphee, D. Poltem, Yong Hong Wu, Y. Lenbury . Simulation of Pulsatile Flow of Blood in Stenosed Coronary Artery Bypass with Graft. Mathematical Biosciences and Engineering, 2006, 3(2): 371-383. doi: 10.3934/mbe.2006.3.371 |
[4] | Sangita Swapnasrita, Joost C de Vries, Carl M. Öberg, Aurélie MF Carlier, Karin GF Gerritsen . Computational modeling of peritoneal dialysis: An overview. Mathematical Biosciences and Engineering, 2025, 22(2): 431-476. doi: 10.3934/mbe.2025017 |
[5] | Aftab Ahmed, Javed I. Siddique . The effect of magnetic field on flow induced-deformation in absorbing porous tissues. Mathematical Biosciences and Engineering, 2019, 16(2): 603-618. doi: 10.3934/mbe.2019029 |
[6] | Huu Thuan Nguyen, Tu Anh Do, Benoît Cosson . Numerical simulation of submerged flow bridge scour under dam-break flow using multi-phase SPH method. Mathematical Biosciences and Engineering, 2019, 16(5): 5395-5418. doi: 10.3934/mbe.2019269 |
[7] | Zhangli Peng, Andrew Resnick, Y.-N. Young . Primary cilium: a paradigm for integrating mathematical modeling with experiments and numerical simulations in mechanobiology. Mathematical Biosciences and Engineering, 2021, 18(2): 1215-1237. doi: 10.3934/mbe.2021066 |
[8] | Oualid Kafi, Nader El Khatib, Jorge Tiago, Adélia Sequeira . Numerical simulations of a 3D fluid-structure interaction model for blood flow in an atherosclerotic artery. Mathematical Biosciences and Engineering, 2017, 14(1): 179-193. doi: 10.3934/mbe.2017012 |
[9] | Ziqiang Cheng, Jin Wang . Modeling epidemic flow with fluid dynamics. Mathematical Biosciences and Engineering, 2022, 19(8): 8334-8360. doi: 10.3934/mbe.2022388 |
[10] | Annalisa Quaini, Sunčica Čanić, David Paniagua . Numerical characterization of hemodynamics conditions near aortic valve after implantation of left ventricular assist device. Mathematical Biosciences and Engineering, 2011, 8(3): 785-806. doi: 10.3934/mbe.2011.8.785 |
Time-dependent flow in liquid-filled elastic tubes is a longstanding object of research with broad applicability [1,2,3]; yet there is still much to be understood. In biology, a major application is flow within organ systems (e.g., cardiovascular, liver and kidney). One major barrier to kidney flow research is the lack of ability to perform in vivo flow measurements through a living renal tubule. For a brief review of the literature see [4]. The so-called internal biofluiddynamics [5] has been heavily studied by a large community of scientists and engineers [6,7]; however there remains a need to develop a multiscale mathematical model to better predict and understand the relationship between applied fluid stresses and resultant tissue mechanotransduction mechanisms. Fluid flow has been shown to have significant biological implications regarding salt and water homeostasis [8,9] and tubular structure itself [10,11,12,13]. Importantly, a cellular structure, the primary cilium, is hypothesized to function as a flow sensor [14,15] and cilia have been implicated in a variety of physiological and pathophysicological conditions [4]. Indeed, we are interested in the relationship between fluid flow stimulation of primary cilia and regulation of transepithelial salt and water transport.
While the study of internal biofluiddynamics can be traced back to Galen and Harvey [16,17], the quantitative study of pulsatile viscous flow within elastic tubes originates with Womersley [18,19,20]. This work has been extended to include semi-permeable tubes [21,22,23] which can be used to model fluid and solute reabsorption, important metrics that parameterize kidney function.
As considered here (Figure 1), kidney anatomy consists of approximately 1 ×106 independent non-branching nephrons, each beginning with a malpighian body through which blood plasma enters via the glomerular filter and ending with the collecting tubule, which empties into the collecting duct, renal pelvis, and ureter. Nephron walls are single-cell thick, and as the fluid ultrafiltrate moves through a nephron, most >99% of the fluid is reabsorbed back into the blood. Current in silico models of renal flow exist for the proximal tubule [24,25], and some in silico models exist for the Loop of Henle [26,27,28] and collecting duct [29]. Our work presented here is a crucial step in modeling the dynamics of interior renal flow. In this study, we focus only on accurately modeling the distal nephron (specifically, the cortical collecting duct) rather than the entire nephron. Broadly speaking, our 'future work' includes modeling the entire nephron by 1) incorporation of unsteady pressure perturbations, 2) incorporation of solute and water reabsorption and 3) to remove simplified cylindrical geometry assumptions. The effects of renal flow on primary cilia can be performed within the laboratory under well-controlled conditions [30,31], but the field suffers from a lack of knowledge about what fluid flow conditions are actually present, especially deep within the medulla.
A survey of available literature reveals a few salient facts regarding time-dependent fluid flow within a nephron. In [32], clear evidence of pulsatile flow within a living rat proximal tubule is presented via live cell microscopy of an oscillating primary cilium. The oscillation frequency, 4.5Hz, is correlated with the heart rate of anesthetized rats, and furthermore, proximal tubule pulsatile flow ceased at death. In [33], a mathematical model of the thick ascending limb demonstrated that compliant (elastic) tubule walls act to intensify harmonics; the driving frequency chosen was 30mHz and the output flow rate contained harmonics at 60 and 90mHz.
Two recent reports provide additional relevant information. In [34], a model of a nephron tree consisting of 22 interacting nephrons and connecting vasculature demonstrated both the synchronization of tubular pressure oscillations as well as both quasiperiodic and chaotic pressure perturbations within a single component nephron. In [35], a detailed computational model of a single complete nephron was developed, providing predictions of Glomerular Filtration Rate (GFR) and volume flow rate that are in reasonable agreement with experimental measurements.
Here, we investigate the dynamic behavior of an elastic cylindrical tube filled with an incompressible Newtonian fluid driven by pulsatile pressure variations using a macroscopic multiscale mathematical model. Solutions of the governing differential equations were obtained numerically, using COMSOL Multiphysics® [36] finite-element analysis packages (e.g., Fluid-Structure Interaction). Results presented here examine the effect of varying the driving frequency on the flow over a range of wall compliances. Throughout this report, we assume the elastic tube to be impermeable. This approximation is most valid for the distal nephron (collecting tubule and collecting duct), segments that are responsible for the final "fine tuning" of salt and water resabsorption, approximately 0.5%.
Furthermore, since we aimed to interrogate distal nephron dynamics, an adequate assumption in modeling the renal tubular structure is non-porous walls. However, within future studies focused in modeling excretion and reabsorption at the Loop of Henle, porous wall incorporation becomes imperative. Porous walls within cylindrical tubules are comparatively different to non-porous walls. In particular through a dispersion coefficient study, earlier work found that solute transport due to flow of a non-Newtonian Ostwald-de Waele fluid can be perturbed by porous walls compared to non-porous walls [37]. Also, the Loop of Henle creates an area of high urea concentration through implementations of electrolyte pumps, specifically producing an efficient process to reabsorb water and create a concentrated urine for excretion. Early work [38] presented an analytical model for transport of a chemical species within a cylindrical tube coupled to a porous medium, potentially creating a feasible basis for future modeling of the Loop of Henle.
One of the aims of the work presented here is to investigate the fluid-structure interaction within a renal tubule (Figure 2) and identify possible flow regimes of interest: Resonant interactions, for example. One particularly intriguing concept is dynamic pathology [39], where the source of disease is not genetic, but rather a consequence of altered system dynamics due to chronic accumulated changes in system parameters (e.g., wall compliance).
This manuscript is ordered as follows. First, the development of the fluid-structure equations that model the distal nephron is presented. Secondly, the numerical computation and validation is analyzed and verified by investigating grid stability and solution accuracy. Then, the results from the numerical simulation are presented. Finally, our conclusions are discussed.
We modeled a fluid-filled renal tubule as a combination of a homogeneous, incompressible, Newtonian fluid (with viscosity 'μ') and a circular cylindrical isotropic elastic tube with radius 'r'. This simple model can be readily extended to incorporate transmural flow corresponding to salt and water reabsorption by semi-permeable tube walls; tubule-tubule interactions via the extracellular viscoelastic medium [40,41]; and orthotropic modeling of the tube wall [42]. Flow is laminar and generated via the pulse, so we use the time-dependent Navier-Stokes momentum equations for the fluid, an elastic solid model for the tube wall, and analyze fluid-structure interaction via a FSI module in COMSOL®.
One important simplification is that the flow velocity does not have an azimuthal component; a second important simplification considered here is that bulk fluid properties (velocity 'u(t)', density 'ρf', pressure 'p(t)') vary continuously in space and time. However, we must separately consider the fluid velocity and density at fluid-solid interfaces. This is due to the fact that the stress tensor is related to the velocity gradient, and the velocity must not have discontinuities at the fluid-solid interface (no-slip boundary condition [43]). We expect that the no-slip boundary condition must be relaxed when, for example, our model incorporates wall permeability [44]. Similarly, we expect that axisymmetry may be broken if there are neighboring tubules that can mechanically interact via the interstitium.
Understanding the temporal evolution of the fluid-wall interplay requires use of governing equations that characterize the dynamics of the renal tubule. Using parameter values relevant to renal physiology, we wish to model pulsatile flow of an incompressible viscous Newtonian fluid within an axisymmetric tube with varying pulsation frequencies and structural elasticities. Futhermore, our reference configuration is illustrated on the top row in Figure 2 and the coordinate system implement for modeling is cylindrical coordinates (z,r,θ). The fluid viscosity and density are denoted by μf and ρf. The fluid flow in the renal tubule is described by the incompressible Navier-Stokes equations for the velocity field u = (uz, ur) in the spatial (deformed) moving coordinate system:
ρf∂u∂t−∇⋅[−pI+μf(∇u+(∇u)T)]+ρf((u−um)⋅∇)u=F,−∇⋅u=0 | (2.1) |
In the above equations I, F and um = (uz, ur)m represents the diagonal matrix, external volume force affecting the fluid, and the coordinate system velocity; in particular, we are assuming that no external forces are acting on our single renal tubule system − hence F = 0. In future studies, we plan to incorporate neighboring tubules since nephrons are closely compacted in a mammalian kidney − thus F ≠ 0.
We will define Ξ = Ξ(z, r) as the original location of a material particle. The variable Ξ represents the coordinate system of the solid structure throughout the deformation history; in other words, the coordinate system is known as the material frame. As the particle moves at a certain time t, we can represent the particle's position with the spatial coordinate system (i.e., spatial frame) as ξ = ξ(Ξ, t). By definition, Ξ is fixed to the body, while ξ is fixed in space. The displacement vector is v(Ξ, t); therefore, we can use the displacement vector as a transformation between the material to the spatial frame by ξ = Ξ + v(Ξ, t). This expression only holds true for local changes in the shape of the material. The deformation gradient Γ becomes
Γ=∂ξ∂Ξ=I+∂v∂Ξ | (2.2) |
where Γ represents a Jacobian matrix of the transformation between the material and spatial frame. Thus, the determinant of the deformation gradient is X = det(Γ). In addition, determinant of the deformation gradient can be expressed as the volume factor X = dVdVo which provides valuable info into the volume change during deformations.
For an arbitrary undeformed material volume Vo, the differential momentum balance equation for the solid body is
ρo∂2v∂t2=fV+∇Ξ⋅PT | (2.3) |
where fV and P represents force per deformed volume (i.e., volumetric force) and first Piola-Kirchhoff stress tensor. Futhermore, we will introduce several known first Piola-Kirchhoff known relations:
P=XσΓ−T=τΓ−T | (2.4) |
P=ΓS=∂ξ∂ΞS=(I+∇v)S. | (2.5) |
In the above equations, σ and τ represents the Cauchy stress tensor and the Kirchhoff stress tensor. In Eq (2.4), we have a relation between stress tensors, while Eq (2.5) expresses a direct relation between the first and second Piola-Kirchoff stress tensor; therefore, we can finally express Eq (2.3) with a momentum balance equation description for a linear elastic material with the constitutive relation S = S(Λ) by substituting Eq (2.5) into Eq (2.3):
ρo∂2v∂t2=fV+∇Ξ⋅(I+∇v)S(Λ). | (2.6) |
The variable Λ is the Green-Lagrange strain tensor where Λij = 12(∂Ξjvi + ∂Ξivj + ∂Ξjvk∂Ξivk) in Einstein notation and ∂Ξ ≡ ∂∂Ξ for convenience. In addition, we can always change between perspectives by rewriting the displacement vector as Ξ(ξ, t) = ξ - v(ξ, t). Equation (2.6) is important as it governs the linear elastic kinematics for the tubule wall, and the relation gives a closed system of equations for the displacement vector. For fluid-structure interplay, COMSOL handles the interactions using Arbitrary Lagrangian-Eulerian (ALE) formulation.
The ALE formulation involves using two different frames (e.g., Eulerian and Lagrangian) to describe the system. In our case, the solid domain will be formulated through the Lagrangian approach, while the fluid domain will be governed by an Eulerian framework. The fluid interacts with a tubule wall through pressure and viscous forces along the interacting boundary. In particular, the renal tubules are relatively compliant, so the load from the fluid causes a deformation which can lead to altered flow patterns. For this interplay, the constitutive relations between the fluid domain and wall domain at the interface are:
u=∂v∂t | (2.7) |
(σ⋅n)fluid=(σ⋅n)wall. | (2.8) |
The above equations demands for equating between fluid-wall velocities and stresses at the interacting boundary through the fluid velocity, displacement vector, Cauchy stress tensor, and the normal vector.
At the tubule inlet, a pulsatile pressure profile is applied and at the outlet all discharged fluid is considered 'absorbed', meaning it exits the simulation volume. Following [39], we express the time-varying component of pressure p(t) as a sum of steady and oscillatory components. Unfortunately, nomenclature varies within the literature when discussing the steady component (e.g., average, mean, or steady) of pulsatile flow; throughout our analysis, we will define the time-independent pressure component at the inlet as Po. In addition, we define the oscillatory component as Pθ. Before we introduce fully developed pulsatile flow (FDPF), we ramp the inlet pressure until it reaches Po with a smooth ramp function (continuous second derivative smoothing) defined in COMSOL®. When the fluid, solid, and mesh variables (see Numerical Computation) are fully computed, we then introduce the oscillatory component Pθ. This allowed us to transition from an equilibrium state to a steady state by introducing FDPF with elastic deformations determined after ramping has completed. We initially prescribed flow velocity, wall displacement, and mesh displacement variables as zero at t = 0 since P(t)=0. Furthermore, the inlet pressure P(t) can be described as
P(t)=Po+Pθ={Poψ(t)t=[0−0.1] secondsPo(1+αsin(ωt))t≥0.1 seconds | (2.9) |
where ψ(t), α and ω represents the ramping step function, flow amplitude, and flow angular frequency. Phase 2 (i.e., t≥0.1) in Eq (2.9) is illustrated on Figure 3. Throughout our analysis, we focused on fluid-structure interplay during FDPF. Applying the boundary condition that all discharged fluid is absorbed, we set the outlet pressure boundary condition to zero.
We begin by considering a single driving frequency set equal to the pulse (ω/2π = 1Hz for humans, ω/2π = 7Hz for mice). The assumed boundary condition on fluid elements at the tube wall, the "no-slip" boundary condition u(r=Rwall)*, requires the fluid to have zero relative velocity at the tube wall. Although detailed measurements to establish or refute the no-slip condition within renal tubules have not been performed, we assume this boundary condition holds. We note that the no-slip boundary condition is violated when transmural flow exists, models of semi-permeable walls require violation of both the no-slip boundary condition and the conservation of mass [7,44]. Additionally, we prescribed weakly coupled displacement along the axial dimension. This constraint is feasible since in vivo, tubule axial length is fixed while tubule radius is not. This constraint should be removed when modeling nephrogenesis.
*Rwall is a fixed radius during static flow; however, the tubular wall will experience deformations leading to many other possible zero relative velocities.
Unlike rigid tubes where fluid velocity can only increase when the driving pressure increases, an elastic tube can radially deform in response to driving pressure (Figure 2), resulting in the exchange of energy between fluid (kinetic energy) and wall (elastic energy). This phenomenon results in qualitatively different flow dynamics as compared to flow within a rigid tube. One difference is the propagation of pressure waves: In a rigid tube, pressure waves propagate with infinite velocity. Another difference is the existence of a non-zero radial fluid velocity component in elastic tubes.
The stress-strain equations of elasticity govern deformations of the tube wall. In general, the strain has three independent components and the stress tensor has six independent components. In our model, we are most interested in radial strains and axial shear stresses, shown schematically in Figure 4. However, there exists no reported values of the magnitude value of stress found in a singular renal tubule, so we instead focused on analyzing deformation influence on fluid flow and vice versa.
Fortunately, there do exist some well-measured parameters for the system under consideration. In addition to summaries provided in [4], these include: The single-nephron glomerular filtration rate (SNGFR) [45], fluid density and viscosity [46,47], tube diameter and wall compliance [48], and driving pressure dynamics [49]. Values for transmural pressure gradients and permeabilities can be found in [50,51,52]. Representative values of relevant parameters are provided in Table 1. We do wish to note that of all the parameters on the list, SNGFR may show the most variability over the entire nephron, beginning at approximately 100nl/min at the entrance and, due to water and solute absorption, drops along the nephron length. The value used here, then, is only applicable to the cortical collecting duct.
Parameter name | Symbol | Representative value | Reference(s) |
Inner radius of tube | |
|
[48] |
Wall thickness | |
|
[48] |
Young's Modulus of tube wall | |
|
[54] |
Poisson ratio of tube wall | |
|
|
Density of wall material | |
|
[53] |
Density of fluid | |
|
[46] |
Dynamic viscosity of fluid | |
|
[47] |
SNGFR | |
|
[45] |
Time-averaged pressure | |
|
[49] |
Length of tube segment | |
|
[54] |
Shear stress exerted on wall | |
to be calculated | N/A |
Here we implement a finite element analysis incorporating the coupled partial differential equations (PDE) system developed in this work in COMSOL® to compute stable solutions. Advantages of this modeling approach include accurate representations of complex geometries, explicit inclusion of material parameters and the ability to capture local effects (e.g., resonant interactions).
Our analysis presented throughout this study involved mesh: Normal. This mesh was chosen over other meshes based on results in Table 2 and low computational cost. A total number of 7019 elements with an average element quality 0.866 and an element area ratio of 0.06102. The mesh area was 1.333×10−9m2. The mesh contains 477 elements along the edges; in particular, the total number of boundary quadrilateral layers at the interface is 2. We fixed the maximum and minimum element size to 6.7×10−6m and 3×10−8m with a maximum element growth rate at 1.3.
Mesh | Triangles | Quads | Vertices | Solution Time | Peak |
Extra Coarse | |||||
Normal | |||||
Finer | |||||
Extremely Fine |
During the solver process, we initially ramped the pressure gradient from zero to the steady pressure component (Po). Then as we approached t=0.1, we added the oscillatory flow component (Pθ) to initiate fully developed pulsatile flow. The initial ramping had no influence on the fluid-structure interplay analysis since we analyzed the data between t=[0.1−end]. Furthermore, the initial ramping had no physical influence since we introduced an oscillatory component at the exact time that the pressure gradient reached the steady component value. The advantage of ramping between t=[0−0.1] was to prescribe initial conditions to zero for the fluid, solid, and mesh variables.
We used the PARDISO solver with Nested dissection multithread for the preordering algorithm. For our approach to solve the FSI problem in COMSOL®, we used the fully coupled method which involves simultaneous solution of flow and wall fields. We implemented the Constant (Newton) nonlinear methods with a damping factor of 1. In addition, our numerical convergence was based on a tolerance termination technique. This technique contained a maximum number of 4 iterations and a tolerance factor of 0.01. The solvers and methods described above were solved on flow, solid, and moving mesh variables. Lastly, we implemented Matlab R2018b for data post-processing. In this section, computational grid independence and validation of the numerical results will be presented.
We studied four different meshes labeled Extra Coarse, Normal, Finer, and Extremely Fine in order to analyze the grid independence of the numerical solutions, see Figure 5 and Table 2. These four meshes are labeled in order from most finely discretized grid elements to least finely discretized grid elements. The number of grid elements are listed in Table 2. Figure 5 illustrated the same fluid-structure interface region. The red border enclosed the interacting boundary which is made up of quadrilateral elements rather than traditional triangular elements since quadrilateral elements are preferred for high magnitude loads and displacements. We demonstrated with increasing total number of mesh elements would lead to improved solution accuracy. Importantly for model verification, the solution accuracy eventually converged as we increased total number of meshes elements, scene in Figure 6.
As discussed in the previous section, quadrilateral elements are better suited for deformations [55], so they are generated along the interacting boundary. Shear and normal forces from the fluid act on the wall which deforms the tube structure over time. Triangular elements are used to decrease computational time in areas where there is no fluid-wall interplay, e.g., centered at r=0. We carry out verification studies focused on both fluid and solid dynamics (e.g., radial velocities, axial velocities, wall stresses and deformations) at different locations and times. This allows for the discovery of possible numerical instabilities as the mesh is refined. However, no divergence or mesh dependency was found.
The results from our studies verify numerical stability by showing improved accuracy for the solutions as the quantity of elements increase, as compared to analytical results when appropriate (e.g., Hagen-Poiseuille Flow). In particular, validations were obtained by using idealized base cases (e.g., rigid tube and steady flow) to obtain any evidence of numerical instability, none were seen. No result from structure or fluid outputs showed any solution divergence or mesh dependency during pulsating flow-wall interplay.
In the present work, the interaction of pulsatile flow is simulated through a single idealized renal tubule located within the renal medulla. Our effort is motivated by a significant difficulty in obtaining in vivo fluid flow conditions within a living kidney. Although several experimental approaches have been conducted with varying degrees of success [56,57], measurements were limited to flow within tubules located near the kidney surface, the renal cortex. Our in-silico model provides high-confidence simulations which can be used to study the flow-wall dynamics in the interior kidney, for example the renal medulla. In addition, known perturbed analytical solutions can be retrieved for limiting cases. We present results investigating potential sources of pathology in a manner not possible with current clinical diagnostic modalities.
We present results of the effects of pulsatile flow on tubule structure and conversely, the effect of tubule wall compliance on the flow. The relevance of these results is the identification of mechanical properties of the tubule wall and fluid flow (i.e., wall compliance, pulsatile frequency and waveform amplitude) that can lead to testable hypotheses regarding progressive pathologies (e.g., Chronic Kidney Disease (CKD), hypertension and Polycystic Kidney Disease) in the kidney. These hypotheses will be phrased in terms of progressive changes in wall and flow parameters, as opposed to specific genetic mutations.
In Figure 7, the driving frequency and amplitude were varied and the tubule expansion computed. The radial percentage change was parameterized by R%=1−⟨Rwall⟩−RwallRwall × 100 along the interacting boundary. The notation ⟨ ⟩ represents the average value, for example Rwall is the unstrained tubule radius while ⟨Rwall⟩ represents the average strained radius along the tubule segment. R% is a convenient parameter used to investigate how the oscillatory component of flow strains the wall as compared to static flow. In addition, R% is an effective parameter describing the accessible fluid volume contained within the tubule via the expression Vfluid=2πR%L.
We present evidence that our model correctly captures pulsatile flow effects on the wall strain in Figure 7a. This figure shows the expansion of the tube segment as a function of ω from the flow. Unsurprisingly, we find that low frequencies ω≪1 rad/s approximate steady flow. Our model verifies that ΔP=32μL⟨uz⟩D2, and so in this limiting case, Hagen-Poiseuille is an adequate approximation to model renal fluid flow. ΔP, μ, L, ⟨uz⟩, D represent the pressure gradient, fluid viscosity, tube length, averaged axial velocity, and tube diameter. However in most human scenarios, pulsatile angular frequency fluctuations around α=2π, implying that Hagen-Poiseuille is not an adequate approximation for realistic tubular physiology. In addition, we fix ω=π rad/s and computed R% as a function of α (i.e., the amplitude waveform) in Figure 7b. This figure demonstrates that there is a linear relationship between the pulse amplitude and wall strain.
We present evidence for resonance-type coupling behavior between flow and wall strain using physiologically relevant parameters in Figure 8. Here, we plot the time-averaged value of R% as a function of frequency, both as linear-linear plot and a linear-log plot to more clearly demonstrate that the average radial deformation varies with driving frequency and has a maximum value for a particular driving frequency. Qualitatively, the average radial deformation appears to have the same spectral response as a damped driven oscillator. Interestingly, the peak value of R% does not display the same resonance-type behavior (Table 3).
f Hz at α = 0.1 | mean(R%) | max(R%) |
0.001 | 11.45 | 11.47 |
0.01 | 11.48 | 11.50 |
0.1 | 11.70 | 11.94 |
1 | 11.97 | 12.71 |
2 | 11.46 | 12.69 |
π | 11.42 | 12.71 |
1.5π | 11.29 | 12.71 |
It is important to note that our results were obtained for a single, isolated, tubule. In reality, there is considerable internephron coupling that must be accounted for- both contact-type elastic mechanical coupling and viscous coupling via the interstitial fluid. It has been shown that coupled nephrons and nephron trees can generate irregular oscillations and complex power spectra [58,59]. Other studies suggest that internephron coupling may prompt synchronization, quasiperiodicty, and perhaps chaos in a nephron tree [60,61]. Internephron coupling is beyond the scope of this report, which is simply focused on creating a validated model. Our model can be expanded to incorporate hydro-elasto-mechanical internephron coupling and that is one goal of future modeling efforts.
Regardless, our results demonstrate a method to explore how varying flow parameters (e.g., amplitude and frequency) can significantly alter the strain dynamics of nephrons. Our model shows that there is a relationship between flow waveform and wall strain. Moreover, this knowledge can provide insight into understanding more complex geometries, for example that found in nephron trees.
To evaluate the effects of wall compliance on the dynamical variables related to pulsatile flow of incompressible Newtonian fluid inside the elastic renal tubule, the wall elastic modulus was varied (e.g., E = 3×104, 1×105, 1×108 Pa) to model physiologically realistic tubule wall properties [54]. The importance of this parameter study is to investigate, for example, tubular atrophy or tubular fibrosis. Tubular atrophy is a general term that describes tissue phenotypes due to chronic tubular injuries, resulting is the tubular basement membrane becoming increasingly stiff. Altered wall stiffness also occurs in other physiological systems, notably the vasculature (arteriosclerosis), pulmonary (cystic fibrosis) and liver (biliary fibrosis).
A numerical study was conducted by varying the compliance of the wall structure when all other boundary conditions and properties of the fluid and tubule wall remained unchanged. As can be seen in Figure 9, as the elastic modulus increases from 3×104 to 1×108 Pa, the maximum values of axial velocity decreases. Furthermore, in the limiting case E ≫ 3×104 Pa the wall acts as a rigid tubule since radial expansion is negligible. By increasing the elastic modulus, the rigidity of walls increases and the radial deformation decreases; the radial displacement is shown in Figures 9 and 10.
In Figure 11 and Table 4, the peak axial velocity and volume flow rate dramatically decrease as flexibility of tubular wall decreases and reaches an asymptotic value. This asymptote represents the limiting case - rigid walls. As seen in the figures, this demonstrates an important interplay between wall stiffness and fluid transport rates.
ENm2 | peak(uz(t=1.25s)) cms | peak(uz(t=1.5s)) cms | Q(t=1.25) μlmin | Q(t=1.5) μlmin |
3×104 | 2.2996 | 2.0716 | 44.1770 | 38.8567 |
5×104 | 2.1439 | 1.9310 | 37.0092 | 33.0431 |
7×104 | 2.0704 | 1.8707 | 34.4082 | 31.0496 |
1×105 | 2.0105 | 1.8244 | 32.3964 | 29.3401 |
3×105 | 1.9293 | 1.7549 | 29.4193 | 26.8891 |
5×105 | 1.9077 | 1.7451 | 28.9257 | 26.4890 |
7×105 | 1.8993 | 1.7325 | 28.6464 | 26.2376 |
3×106 | 1.8822 | 1.7214 | 28.2334 | 25.8702 |
5×106 | 1.8801 | 1.7200 | 28.2701 | 25.8012 |
7×106 | 1.8792 | 1.7195 | 28.2450 | 25.7830 |
1×107 | 1.8786 | 1.7191 | 28.2246 | 25.7695 |
5×107 | 1.8775 | 1.7183 | 28.1887 | 25.7439 |
1×108 | 1.8774 | 1.7182 | 28.1845 | 25.7406 |
1×109 | 1.8772 | 1.7181 | 28.1809 | 25.7376 |
In addition, as the elastic stiffness of the renal tubules decreases, the kidney requires more power to pump renal fluid through the nephron. Hence, the system could experience structural degeneration and increased system pressure (e.g., hypertension). Similarly, a reduction of renal tubule elasticity leads to the development of Chronic Kidney Disease. Therefore, the efficiency of kidney function decreases with decreasing elasticity which supports the notion of dynamic pathology.
In this paper, kidney flow biomechanical environments corresponding to pathophysiological regimes were investigated by examining fluid-structure interactions and solving partial differential equations in Arbitrary Lagrangian-Eulerian formalism using finite element modeling techniques. In addition, analysis of fluid and structure parameter studies as a model for several pathologies was performed. Although we present an imperative approach within investigating renal dynamics, our numerical simulations are limited to modeling the distal nephron. Within future studies, we plan to model entire nephron dynamics by incorporating unsteady pressure perturbations, reabsorption and releasing the axisymmetric geometry assumption. Our conclusions are summarized as:
(1) Our model shows that a better understanding of driving pressure waveforms (both frequencies and amplitudes) involved deep within the medulla is needed as elastohydrodynamic coupling between neighboring nephrons could strongly modify fluid throughput.
(2) In the case of driving pressure frequency influence on distal tubular wall dynamics, our model shows support in approximating renal flow as Hagen-Posiuille in the limiting case ω≪π. Our model demonstrates that there exists a ω that maximizes radial expansion during local time scales.
(3) From the results of flow in stiffening distal convoluted tubules, the peak axial velocity monotonically decreases as the compliance decreases. Our investigation provides important links between biomechanical environments and possible kidney function failures, demonstrating that fluid volume flow rates decrease as the structural parameters of the wall transition to pathological values.
(4) Our model provides the ability to analyze local tissue stresses in damaged or degenerated nephrons. For many chronic diseases, tissue damage accumulates over long periods of time; our model allows us to improve understanding of the progressive damage due to hypertension and diabetes.
(5) Since detailed knowledge about the actual renal fluid flows experienced by cilia in a living kidney is largely lacking, this research effort provides important information regarding design [4] of perfused tissue and organ experiments.
All authors declare no conflicts of interest in this paper.
[1] | I. Mnassri, A. El Baroudi, Vibrational frequency analysis of finite elastic tube filled with compressible viscous fluid, Acta Mech. Solida Sin., 30 (2017), 435-444. |
[2] | R. M. Terrill, An exact solution for flow in a porous pipe, Z. Angew. Math. Phys., 33 (1982), 547-552. |
[3] | O. San, A. E. Staples, Dynamics of pulsatile flows through elastic microtubes, Int. J. Appl. Mech., 4 (2012). |
[4] | S. Nag, A. Resnick, Biophysics and biofluid dynamics of primary cilia: Evidence for and against the flow-sensing function, Am. J. Physiol. Renal Physiol., 313 (2017), 706-720. |
[5] | M. J. Lighthill, Mathematical Biofluiddynamics, Society for Industrial and Applied Mathematics, Philadelphia, 1975. |
[6] | A. El Baroudi, F. Razafimahery, L. Rakotomanana, Fluid-structure interaction within three-dimensional models of an idealized arterial wall, Int. J. Eng. Sci., 84 (2014), 113-126. |
[7] | M. Zamir, The Physics of Pulsatile Flow, Springer-Verlag, New York, 2000. |
[8] | P. D. Cabral, J. L. Garvin, Luminal flow regulates no and o2(-) along the nephron, Am. J. Physiol. Renal Physiol., 300 (2011), 1047-1053. |
[9] | L. M. Satlin, S. Sheng, C. B. Woda, T. R. Kleyman, Epithelial Na(+) channels are regulated by flow, Am. J. Physiol. Renal Physiol., 280 (2001), 1010-1018. |
[10] | M. Essig, G. Friedlander, Tubular shear stress and phenotype of renal proximal tubular cells, J. Am. Soc. Nephrol, 14 (2003), S33-35. |
[11] | J. B. Freund, J. G. Goetz, K. L. Hill, J. Vermot, Fluid flows and forces in development: Functions, features and biophysical principles, Development, 139 (2012), 1229-1245. |
[12] | F. Kotsis, R. Nitschke, M. Doerken, G. WalzE, W, Kuehn, Flow modulates centriole movements in tubular epithelial cells, Pflugers Arch, 456 (2008), 1025-1035. |
[13] | A. B. Maunsbach, G. H. Giebisch, B. A. Stanton, Effects of flow rate on proximal tubule ultrastructure, Am. J. Physiol., 253 (1987), 582-587. |
[14] | H. A. Praetorius, K. R. Spring, Removal of the mdck cell primary cilium abolishes flow sensing, J. Membr. Biol., 191 (2002), 69-76. |
[15] | H. A. Praetorius, K. R. Spring, The renal cell primary cilium functions as a flow sensor, Curr. Opin. Nephrol Hypertens, 12 (2003), 517-520. |
[16] | D. J. Furley, J. S. Wilkie, Galen on Respiration and the Arteries: an Edition with English Translation and Commentary of De usu Respirationis, An in Arteriis Natura Sanguis Contineatur, De usu Pulsum, and De Causis Respirationis, Princeton University Press, Guildford, 1984. |
[17] | W. Harvey, On the Motion of the Heart and Blood in Animals; and on the Circulation of the Blood; and on the Generation of Animals, William Benton, Chicago, 1952. |
[18] | J. R. Womersley, Method for the calculation of velocity, rate of flow and viscous drag in arteries when the pressure gradient is known, J. Physiol., 127 (1955), 553-563. |
[19] | J. R. Womersley, Xxiv. oscillatory motion of a viscous liquid in a thin-walled elastic tubei: The linear approximation for long waves, London, Edinburgh, Dublin Philos. Mag. J. Sci., 46 (1955), 199-221. |
[20] | J. Womersley, An elastic tube theory of pulse transmission and oscillatory flow in mammalian arteries, 1957. |
[21] | R. I. Macey, Pressure flow patterns in a cylinder with reabsorbing walls, Bull. Math. Biophy., 25 (1963), 1-9. |
[22] | E. A. Marshall, E. A. Trowbridge, Flow of a newtonian fluid through a permeable tube - application to proximal renal tubule, Bull. Math. Bio., 36 (1974), 457-476. |
[23] | C. Pozrikidis, Stokes flow through a permeable tube, Arch. Appl. Mech., 80 (2010), 323-333. |
[24] | A. M. Weinstein, Nonequilibrium thermodynamic model of the rat proximal tubule epithelium, Biophys. J., 44 (1983), 153-70. |
[25] | A. M. Weinstein, A mathematical model of the rat proximal tubule, Am. J. Physiol., 250 (1986), 860-873. |
[26] | A. M. Weinstein, A mathematical model of rat ascending henle limb. i. cotransporter function, Am. J. Physiol. Renal Physiol., 298 (2010), 512-524. |
[27] | A. M. Weinstein, A mathematical model of rat ascending henle limb. iii. tubular function, Am. J. Physiol. Renal Physiol., 298 (2010), 543-556. |
[28] | A. M. Weinstein, T. A. Krahn, A mathematical model of rat ascending henle limb. ii. epithelial function, Am. J. Physiol. Renal Physiol., 298 (2010), 525-542. |
[29] | A. M. Weinstein, A mathematical model of rat collecting duct. i. flow effects on transport and urinary acidification, Am. J. Physiol. Renal Physiol., 283 (2002), 1237-1251. |
[30] | M. E. Downs, A. M. Nguyen, F. A. Herzog, D. A. Hoey, C. R. Jacobs, An experimental and computational analysis of primary cilia deflection under fluid flow, Comput. Methods Biomech. Biomed. Eng., 17 (2014), 2-10. |
[31] | W. Liu, S. Xu, C. Woda, P. Kim, S. Weinbaum, L. M. Satlin, Effect of flow and stretch on the [Ca2+] i response of principal and intercalated cells in cortical collecting duct, Am. J. Physiol. Renal Physiol., 285 (2003), 998-1012. |
[32] | A. K. O'Connor, E. B. Malarkey, N. F. Berbari, M. J. Croyle, C. J. Haycraft, P. D. Bell, et al., An inducible ciliagfp mouse model for in vivo visualization and analysis of cilia in live tissue, Cilia, 2 (2013), 8. |
[33] | A. T. Layton, L. C. Moore, H. E. Layton, Signal transduction in a compliant thick ascending limb, Am. J. Physiol. Renal Physiol., 302 (2012), 1188-1202. |
[34] | D. J. Marsh, O. V. Sosnovtseva, E. Mosekilde, N. H. Holstein-Rathlou, Vascular coupling induces synchronization, quasiperiodicity, and chaos in a nephron tree, Chaos, 17 (2007), 015114. |
[35] | A. T. Layton, H. E. Layton, A computational model of epithelial solute and water transport along a human nephron, PLoS Comput. Biol., 15 (2019), e1006108. |
[36] | COMSOL Multiphysics® v. 5.4. www.comsol.com. COMSOL AB, Stockholm, Sweden. |
[37] | M. Dejam, Dispersion in non-newtonian fluid flows in a conduit with porous walls, Chem. Eng. Sci., 189 (2018), 296-310. |
[38] | M. Dejam, H. Hassanzadeh, Z. X. Chen, Shear dispersion in combined pressure-driven and electro-osmotic flows in a channel with porous walls, Chem. Eng. Sci., 137 (2015), 205-215. |
[39] | M. Zamir, Hemo-Dynamics, Springer International Publishing, 2015. |
[40] | A. T. Layton, Modeling transport and flow regulatory mechanisms of the kidney, ISRN Biomath., 2012 (2012). |
[41] | A. T. Layton, L. C. Moore, H. E. Layton, Multistable dynamics mediated by tubuloglomerular feedback in a model of coupled nephrons, Bull. Math. Bio., 71 (2009). |
[42] | H. B. Atabek, Wave propagation through a viscous fluid contained in a tethered, initially stresses, orthotropic elastic tube, Biophys. J., 8 (1968), 626-649. |
[43] | M. A. Day, The no-slip condition of fluid dynamics, Erkenntnis, 33 (1990), 285-296. |
[44] | B. J. Cox, J. M. Hill, Flow through a circular tube with a permeable navier slip boundary, Nanoscale Res. Lett., 6 (2011), 389. |
[45] | L. N. Reinking, B. Schmidt-Nielsen, Peristaltic flow of urine in the renal capillary collecting ducts of hamsters, Kidney Int., 20 (1981), 55-60. |
[46] | M. Pradella, R. M. Dorizzi, F. Rigolin, Relative density of urine: Methods and clinical significance, Crit. Rev. Clin. Lab. Sci., 26 (1988), 195-242. |
[47] | B. Vahidi, N. Fatouraee, A. Imanparast, A. N. Moghadam, A mathematical simulation of the ureter: Effects of the model parameters on ureteral pressure/flow relations, J. Biomech. Eng., 133 (2011), 031004. |
[48] | S. Cortell, F. J. Gennari, M. Davidman, W. H. Bossert, W. B. Schwartz, A definition of proximal and distal tubular compliance. Practical and theoretical implications, J. Clin. Invest., 52 (1973), 2330-2339. |
[49] | N. H. Holstein-Rathlou, D. J. Marsh, Oscillations of tubular pressure, flow, and distal chloride concentration in rats, Am. J. Physiol., 256 (1989), 1007-1014. |
[50] | E. Gonzalez, P. Carpi-Medina, G. Whittembury, Cell osmotic water permeability of isolated rabbit proximal straight tubules, Am. J. Physiol., 242 (1982), 321-330. |
[51] | E. Frömter, C. Mller, T. Wick, Permeability properties of the proximal tubular epithelium of the rat kidney studied with electrophysiological methods, Electrophysiol. Epithelial Cells, (1971), 119-146. |
[52] | J. A. Schafer, Transepithelial osmolality differences, hydraulic conductivities, and volume absorption in the proximal tubule, Annu. Rev. Physiol., 52 (1990), 709-726. |
[53] | J. Howard, Mechanics of Motor Proteins and the Cytoskeleton, Sinauer, 2001. |
[54] | T. Sakai, D. A. Craig, A. S. Wexler, D. J. Marsh, Fluid waves in renal tubules, Biophys. J., 50 (1986), 805-813. |
[55] | M. Mahran, A. ELsabbagh, H. Negm, A comparison between different finite elements for elastic and aero-elastic analyses, J. Adv. Res., 8 (2017), 635-648. |
[56] | R. Carrisoza-Gaytan, Y. Liu, D. Flores, C. Else, H. G. Lee, G. Rhodes, et al., Effects of biomechanical forces on signaling in the cortical collecting duct (ccd), Am. J. Physiol. Renal Physiol., 307 (2014), 195-204. |
[57] | J. J. Kang, I. Toma, A. Sipos, F. McCulloch, J. Peti-Peterdi, Quantitative imaging of basic functions in renal (patho) physiology, Am. J. Physiol. Renal Physiol., 291 (2006), 495-502. |
[58] | A. T. Layton, L. C. Moore, H. E. Layton, Multistability in tubuloglomerular feedback and spectral complexity in spontaneously hypertensive rats, Am. J. Physiol. Renal Physiol., 291 (2006) 79-97. |
[59] | E. B. Pitman, R. M. Zaritski, K. J. Kesseler, L. C. Moore, H. E. Layton, Feedback-mediated dynamics in two coupled nephrons, Bul. Math. Bio., 66 (2004), 1463-1492. |
[60] | J. L. Laugesen, E. Mosekilde, N.-H. Holstein-Rathlou, Synchronization of period-doubling oscillations in vascular coupled nephrons, Chaos: Interdiscip. J. Nonlinear Sci., 21 (2011), 033128. |
[61] | D. J. Marsh, O. V. Sosnovtseva, E. Mosekilde, N. H. Holstein-Rathlou, Vascular coupling induces synchronization, quasiperiodicity, and chaos in a nephron tree, Chaos: Interdiscip. J. Nonlinear Sci., 17 (2007), 015114. |
1. | Nerion Zekaj, Shawn D. Ryan, Andrew Resnick, Fluid-structure interaction modelling of neighboring tubes with primary cilium analysis, 2022, 20, 1551-0018, 3677, 10.3934/mbe.2023172 | |
2. | Marta Ortega-Martinez, Vanessa Gutierrez-Davila, Esthefania Gutierrez-Arenas, Alberto Niderhauser-Garcia, Ricardo M. Cerda-Flores, Gilberto Jaramillo-Rangel, The Convoluted Tubules of the Nephron Must Be Considered Elliptical, and Not Circular, in Stereological Studies of the Kidney, 2021, 46, 1420-4096, 229, 10.1159/000515051 | |
3. | Shuo Li, Sohail Ahmad, Kashif Ali, Ahmed M. Hassan, Waleed Hamali, Wasim Jamshed, A mathematical approach for modeling the blood flow containing nanoparticles by employing the Buongiorno’s model, 2023, 12, 2191-9097, 10.1515/ntrev-2023-0139 |
Parameter name | Symbol | Representative value | Reference(s) |
Inner radius of tube | |
|
[48] |
Wall thickness | |
|
[48] |
Young's Modulus of tube wall | |
|
[54] |
Poisson ratio of tube wall | |
|
|
Density of wall material | |
|
[53] |
Density of fluid | |
|
[46] |
Dynamic viscosity of fluid | |
|
[47] |
SNGFR | |
|
[45] |
Time-averaged pressure | |
|
[49] |
Length of tube segment | |
|
[54] |
Shear stress exerted on wall | |
to be calculated | N/A |
Mesh | Triangles | Quads | Vertices | Solution Time | Peak |
Extra Coarse | |||||
Normal | |||||
Finer | |||||
Extremely Fine |
f Hz at α = 0.1 | mean(R%) | max(R%) |
0.001 | 11.45 | 11.47 |
0.01 | 11.48 | 11.50 |
0.1 | 11.70 | 11.94 |
1 | 11.97 | 12.71 |
2 | 11.46 | 12.69 |
π | 11.42 | 12.71 |
1.5π | 11.29 | 12.71 |
ENm2 | peak(uz(t=1.25s)) cms | peak(uz(t=1.5s)) cms | Q(t=1.25) μlmin | Q(t=1.5) μlmin |
3×104 | 2.2996 | 2.0716 | 44.1770 | 38.8567 |
5×104 | 2.1439 | 1.9310 | 37.0092 | 33.0431 |
7×104 | 2.0704 | 1.8707 | 34.4082 | 31.0496 |
1×105 | 2.0105 | 1.8244 | 32.3964 | 29.3401 |
3×105 | 1.9293 | 1.7549 | 29.4193 | 26.8891 |
5×105 | 1.9077 | 1.7451 | 28.9257 | 26.4890 |
7×105 | 1.8993 | 1.7325 | 28.6464 | 26.2376 |
3×106 | 1.8822 | 1.7214 | 28.2334 | 25.8702 |
5×106 | 1.8801 | 1.7200 | 28.2701 | 25.8012 |
7×106 | 1.8792 | 1.7195 | 28.2450 | 25.7830 |
1×107 | 1.8786 | 1.7191 | 28.2246 | 25.7695 |
5×107 | 1.8775 | 1.7183 | 28.1887 | 25.7439 |
1×108 | 1.8774 | 1.7182 | 28.1845 | 25.7406 |
1×109 | 1.8772 | 1.7181 | 28.1809 | 25.7376 |
Parameter name | Symbol | Representative value | Reference(s) |
Inner radius of tube | |
|
[48] |
Wall thickness | |
|
[48] |
Young's Modulus of tube wall | |
|
[54] |
Poisson ratio of tube wall | |
|
|
Density of wall material | |
|
[53] |
Density of fluid | |
|
[46] |
Dynamic viscosity of fluid | |
|
[47] |
SNGFR | |
|
[45] |
Time-averaged pressure | |
|
[49] |
Length of tube segment | |
|
[54] |
Shear stress exerted on wall | |
to be calculated | N/A |
Mesh | Triangles | Quads | Vertices | Solution Time | Peak |
Extra Coarse | |||||
Normal | |||||
Finer | |||||
Extremely Fine |
f Hz at α = 0.1 | mean(R%) | max(R%) |
0.001 | 11.45 | 11.47 |
0.01 | 11.48 | 11.50 |
0.1 | 11.70 | 11.94 |
1 | 11.97 | 12.71 |
2 | 11.46 | 12.69 |
π | 11.42 | 12.71 |
1.5π | 11.29 | 12.71 |
ENm2 | peak(uz(t=1.25s)) cms | peak(uz(t=1.5s)) cms | Q(t=1.25) μlmin | Q(t=1.5) μlmin |
3×104 | 2.2996 | 2.0716 | 44.1770 | 38.8567 |
5×104 | 2.1439 | 1.9310 | 37.0092 | 33.0431 |
7×104 | 2.0704 | 1.8707 | 34.4082 | 31.0496 |
1×105 | 2.0105 | 1.8244 | 32.3964 | 29.3401 |
3×105 | 1.9293 | 1.7549 | 29.4193 | 26.8891 |
5×105 | 1.9077 | 1.7451 | 28.9257 | 26.4890 |
7×105 | 1.8993 | 1.7325 | 28.6464 | 26.2376 |
3×106 | 1.8822 | 1.7214 | 28.2334 | 25.8702 |
5×106 | 1.8801 | 1.7200 | 28.2701 | 25.8012 |
7×106 | 1.8792 | 1.7195 | 28.2450 | 25.7830 |
1×107 | 1.8786 | 1.7191 | 28.2246 | 25.7695 |
5×107 | 1.8775 | 1.7183 | 28.1887 | 25.7439 |
1×108 | 1.8774 | 1.7182 | 28.1845 | 25.7406 |
1×109 | 1.8772 | 1.7181 | 28.1809 | 25.7376 |