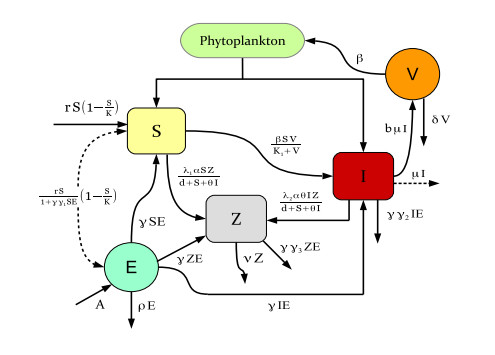
Citation: Saswati Biswas, Pankaj Kumar Tiwari, Yun Kang, Samares Pal. Effects of zooplankton selectivity on phytoplankton in an ecosystem affected by free-viruses and environmental toxins[J]. Mathematical Biosciences and Engineering, 2020, 17(2): 1272-1317. doi: 10.3934/mbe.2020065
[1] | He Liu, Chuanjun Dai, Hengguo Yu, Qing Guo, Jianbing Li, Aimin Hao, Jun Kikuchi, Min Zhao . Dynamics induced by environmental stochasticity in a phytoplankton-zooplankton system with toxic phytoplankton. Mathematical Biosciences and Engineering, 2021, 18(4): 4101-4126. doi: 10.3934/mbe.2021206 |
[2] | Juan Li, Yongzhong Song, Hui Wan, Huaiping Zhu . Dynamical analysis of a toxin-producing phytoplankton-zooplankton model with refuge. Mathematical Biosciences and Engineering, 2017, 14(2): 529-557. doi: 10.3934/mbe.2017032 |
[3] | Xinyou Meng, Jie Li . Stability and Hopf bifurcation analysis of a delayed phytoplankton-zooplankton model with Allee effect and linear harvesting. Mathematical Biosciences and Engineering, 2020, 17(3): 1973-2002. doi: 10.3934/mbe.2020105 |
[4] | Jean-Jacques Kengwoung-Keumo . Dynamics of two phytoplankton populations under predation. Mathematical Biosciences and Engineering, 2014, 11(6): 1319-1336. doi: 10.3934/mbe.2014.11.1319 |
[5] | Dongmei Wu, Hao Wang, Sanling Yuan . Stochastic sensitivity analysis of noise-induced transitions in a predator-prey model with environmental toxins. Mathematical Biosciences and Engineering, 2019, 16(4): 2141-2153. doi: 10.3934/mbe.2019104 |
[6] | Yueping Dong, Jianlu Ren, Qihua Huang . Dynamics of a toxin-mediated aquatic population model with delayed toxic responses. Mathematical Biosciences and Engineering, 2020, 17(5): 5907-5924. doi: 10.3934/mbe.2020315 |
[7] | Hong Yang . Global dynamics of a diffusive phytoplankton-zooplankton model with toxic substances effect and delay. Mathematical Biosciences and Engineering, 2022, 19(7): 6712-6730. doi: 10.3934/mbe.2022316 |
[8] | Xin Zhao, Lijun Wang, Pankaj Kumar Tiwari, He Liu, Yi Wang, Jianbing Li, Min Zhao, Chuanjun Dai, Qing Guo . Investigation of a nutrient-plankton model with stochastic fluctuation and impulsive control. Mathematical Biosciences and Engineering, 2023, 20(8): 15496-15523. doi: 10.3934/mbe.2023692 |
[9] | Wenjie Yang, Qianqian Zheng, Jianwei Shen, Linan Guan . Bifurcation and pattern dynamics in the nutrient-plankton network. Mathematical Biosciences and Engineering, 2023, 20(12): 21337-21358. doi: 10.3934/mbe.2023944 |
[10] | Qihua Huang, Hao Wang . A toxin-mediated size-structured population model: Finite difference approximation and well-posedness. Mathematical Biosciences and Engineering, 2016, 13(4): 697-722. doi: 10.3934/mbe.2016015 |
After the pioneering work of Anderson and May [1], research on predator-prey-parasite system has been explored extensively [2,3,4]. It is now well documented that predator-prey-virus models exhibit more complex and interesting dynamical behaviors than the conventional ecological or epidemiological models [5]. Parasites play a prominent role in trophic interactions and strongly influence the food web characteristics. It can also manipulate the potential of its host and affect the abundance of a predator [6]. A number of studies have demonstrated that the predators tend to forage for prey with high parasite burden [7]. The different factors regulating the intricate planktonic food web are, namely, selective predation [8], nutrient inflow [9], grazing by higher predators [10], etc. Zooplankton are capable of discriminatory feeding [11], and its food selectivity criteria vary to a large extent [12]. Zooplankton can selectively ingest its food depending on a myriad of factors, namely size [13], digestibility [8], toxicity [14], availability [15], and nutrition value [16] of food. A number of studies have documented that Calanoid copepods can discriminate between toxic and nontoxic dinoflagellates [17], noxious and innoxious blue-greens [18], and live and dead algae of the same species [19].
Marine viruses have been recognized to have a decisive lead in altering the physiological and biochemical compositions of their hosts [20,21]. During viral infection, the host-cell characteristics such as cell size, the diffused infochemicals and the cell lipid membrane properties are changed, which influence the feeding behavior as well as the growth rate of zooplankton [22,23]. Emiliania huxleyi are extremely abundant marine phytoplankton species of the cocolithophore group which are frequently found in most of the marine ecosystems. Under favourable environmental conditions, E. huxleyi outburst into large seasonal blooms [24]. Gaint physcodnaviruses (E. huxleyi viruses, EhVs) are known to infect and lyse E. huxleyi cells and to be highly associated with the regulation of populations and the termination of blooms [25]. It has been documented that the exposure of E. huxleyi to EhVs causes more production of DMS, DMSP compared to normal bloom conditions [26,27]. Some studies have proposed that the infochemicals released from the infected cells act as an activated chemical signal which triggers the selective feeding behavior of the grazer zooplankton [23,28,29,27]. The role of viral infection in the producer trophic level of marine ecosystems has been investigated by several authors [30,31,32,33]. Some model based studies explicitly considered the role of free-viruses in eco-epidemiological system [6,34], assuming that both the healthy and infected phytoplankton are equally preferable for predation.
Pollutants have a potentially hazardous effect on marine ecosystem. Pollution may arise from different sources, the most well known are influx of domestic sewage, industrial waste, agricultural run-off etc. The environmental toxins affect the plankton communities at different levels − abundance, growth strategies, dominance and succession patterns. During predation these toxins are passed onto higher trophic level in the food chain in a cascading manner. The contact rate between environmental toxins and marine organisms is mainly dependent on their distribution pattern and behavioral attributes in the ecosystems [35]. Environmental toxins cause an implicit suppression in the growth of phytoplankton cells among a wide range of phytoplankton population [36,37]. In some recent studies, this growth suppression behavior of environmental toxins have been incorporated mathematically [38,39]. It has been shown that environmental toxin-induced growth suppression of phytoplankton population can lead to a destabilized system. However, the depletion of environmental toxins from the aquatic system plays a crucial role to stabilize the system. Yu et al. [40] have studied a stochastic phytoplankton-zooplankton model by considering toxin-producing phytoplankton and Markov switching in an impulsive polluted environment; they found that environmental fluctuations and exogenous toxicant input have a great influence on the survival fate of plankton.
Recently, Bairagi and Adak [41] have studied the predator-prey dynamics, where the prey species are susceptible to infection and predators feed upon both the susceptible and infected prey with some preference. Their findings depict that understanding the consequences of predator's selectivity or preference may play a pivotal role to shape the community structure and ecological properties involving parasites. By dividing the entire phytoplankton population of a pelagic ecosystem into preferred and nonpreferred phytoplankton, Bairagi et al. [42] have shown that the selective predation of zooplankton and nutritional value of phytoplankton strongly impact on the complex plankton dynamics, including the bloom phenomenon. In the present investigation, we have captured the idea of selectivity tendency of grazer zooplankton on susceptible phytoplankton cells in the presence of infected ones, for the very first time. The objective of our study is to investigate the role of selectivity behavior on the persistence and dominance of healthy phytoplankton cells. We would also like to investigate the combined effects of free-viruses and environmental toxins on the dynamics of phytoplankton-zooplankton in the system, and how the selectivity behavior of zooplankton regulate the dynamics. The above ecological questions are answered by the model analysis and numerical simulations.
Pollution of freshwater and marine systems by anthropogenic sources has become a concern over the last several decades. Organic (e.g. triazine herbicides) [43] and inorganic compounds (e.g. heavy metals) [44] both may have harmful effects to the organisms. For example, photosynthesis of the green algae Selenastrum capricornutum is inhibited due to pollutants originating from industrial and recreational sources [45]. In a marine planktonic community comprising mainly diatoms and herbivorous copepods in a low silicate, elevated copper environment [46], copper harms diatoms. At low concentrations, the herbicide triazine also affects primary producers directly by inhibiting photosynthesis, while effects on subsequent trophic levels only would be indirect [46]. Contamination of water bodies with insecticides such as carbaryl, azadirachtin, or cypermetrin increases the death rate of zooplankton.
In phytoplankton population, viruses are not transmitted from infected phytoplankton to susceptible phytoplankton by contact. Usually, virus particles attach to the host cell and release its genetic material into the cell. The virus then uses the host cells machinery to replicate its own genetic material. Once replication has been completed, the virus particles burst out of the host cell into the extracellular space resulting in the death of the host cell. After escaped from the host cell, virus is ready to infect other susceptible phytoplankton. This kind of transmission converts directly a part of phytoplankton population to an infected class [6]. Moreover, in the absence of viral infection, primary consumers like copepods, access over E. huxleyi and other phytoplankton species at random but in the presence of viral infection zooplankton exhibit some sort of grazing preferences [23,27].
Let S, I and Z be the concentrations of susceptible phytoplankton, infected phytoplankton and zooplankton in an aquatic ecosystem, and let V and E be the concentrations of free-viruses and environmental toxins in the same system. We have the following assumptions:
1. In the absence of free-viruses V, environmental toxins E and the grazer zooplankton Z, the susceptible phytoplankton S grow logistically as dSdt=rS(1−SK) with r being the intrinsic growth rate and K being the carrying capacity. The environmental toxins E affects the growth rate of S, and it reduces to r1+γγ1SE, where γ is the contact rate of environmental toxins with phytoplankton and zooplankton, and γ1 is the inhibition of the susceptible phytoplankton growth rate due to environmental toxins.
2. The susceptible phytoplankton S, becomes infected by direct contact with free viruses, V, living in the system following a disc function βSVK1+V with the transmission parameter β and the half saturation constant K1. In the presence of free-viruses, V, the susceptible phytoplankton S is consumed by the zooplankton Z with the rate of αSd+S+θI. The parameter α is the predation rate of the zooplankton Z; d is the half saturation constant; and θ measures the selectivity/preference of the zooplankton to infected phytoplankton over the susceptible one or vice-versa. If θ>1, then zooplankton prefer infected phytoplankton, θ=1 implies equal preference to susceptible and infected phytoplankton, and 0<θ<1 means that zooplankton prefer susceptible phytoplankton.
3. It is assumed that the infected phytoplankton, I, do not reproduce [47,48], and they are removed by cell lysis before having the capability of reproducing [49]. The infected phytoplankton do not compete for resource with susceptible phytoplankton, S. The infected phytoplankton, I, is consumed by the zooplankton, Z, with the rate of αθId+S+θI. The infected phytoplankton is assumed to die at a constant rate μ with an additional mortality rate γγ2E due to the environmental toxins, E, in the aquatic system.
4. The zooplankton population increase on consumption of susceptible and infected phytoplankton; experience natural death at a constant rate ν, and additional death due to presence of environmental toxins in the system, γγ3ZE.
5. Let b be the virus replication factor through the infected phytoplankton I, i.e., lysis of infected phytoplankton, on the average, producing b virus particles (b≫1). The decay rate of virus is assumed to be constant, δ. The virus is removed through the infection of susceptible phytoplankton at the rate βSVK1+V.
6. The environmental toxins E has a constant input rate A and a depletion rate of ρ. The additional removal rates are due to being absorbed by both phytoplankton and zooplankton through their contact at a rate γ [38,40].
The schematic diagram for the interplay among all the considered dynamical variables is depicted in Figure 1. Based on these ecological assumptions, we have the following system of nonlinear differential equations,
dSdt=rS1+γγ1SE(1−SK)−βSVK1+V−αSZd+S+θI,dIdt=βSVK1+V−αθIZd+S+θI−γγ2IE−μI,dZdt=αZ(λ1S+λ2θI)d+S+θI−νZ−γγ3ZE,dVdt=bμI−βSVK1+V−δV,dEdt=A−γ(S+I+Z)E−ρE. | (2.1) |
The parameters involved in system (2.1) are positive constants. System (2.1) is to be analyzed with the following initial conditions,
S(0)>0, I(0)>0, Z(0)>0, V(0)>0, E(0)>0. | (2.2) |
We assume 0<λ2≤λ1<1, i.e., the per capita contribution of infected phytoplankton to the growth of zooplankton never exceeds that of the susceptible phytoplankton. Biological meanings of parameters in the system (2.1) is given in Table 1.
Parameters | Descriptions | Values | Units |
r | Intrinsic growth rate of susceptible phytoplankton | 1.2 | 1/day |
K | Carrying capacity of susceptible phytoplankton | 10 | cells/L |
β | Force of infection | 0.45 | 1/day |
K1 | Half saturation constant | 3 | cells/L |
α | Capture rate of susceptible phytoplankton | 0.18 | 1/day |
θ | Selectivity/preference of the zooplankton | 0.15 | — |
d | Half saturation constant | 5 | cells/L |
λ1 | Reproductive gain of zooplankton from susceptible phytoplankton | 0.75 | — |
λ2 | Reproductive gain of zooplankton from infected phytoplankton | 0.72 | — |
μ | Death rate of infected phytoplankton | 0.2 | 1/day |
ν | Mortality rate of zooplankton (natural and due to fish predation) | 0.012 | 1/day |
b | Virus replication factor | 40 | — |
δ | Decay rate of free-virus | 0.23 | 1/day |
A | Input rate of environmental toxins in the system | 6 | μg/L/day |
γ | Contact rate between environmental toxins with phytoplankton and zooplankton | 0.15 | L/cell/day |
γ1 | Inhibition of growth rate of susceptible phytoplankton due to environmental toxins | 0.1 | day L/μg |
γ2 | Death rate of infected phytoplankton due to environmental toxins | 0.02 | cell/μg |
γ3 | Death rate of zooplankton due to environmental toxins | 0.05 | cell/μg |
ρ | Depletion rate of environmental toxins | 0.25 | 1/day |
In theoretical ecology, boundedness of a system implies that the system is well behaved. Boundedness of the solutions entails that none of the interacting populations grow exponentially for a long-time interval. The abundance of each population is bounded due to limited resource. Moreover, permanence of a system means the long-term survival of all populations of the system, no matter what the initial populations are. From mathematical point of view, permanence of a system means that strictly positive solutions do not have omega limit points on the boundary of the non-negative cone.
We have the following theorem.
Theorem 3.1. Every solution of system (2.1) with initial conditions (2.2) exists and unique in some interval [0,κ), where S(t)>0, I(t)>0, Z(t)>0, V(t)>0, E(t)>0, ∀t≥0. Further, all non-negative solutions of system (2.1) that start in R5+ are uniformly bounded, and the feasible region for system (2.1) is given by the following set
Ω={(S,I,Z,V,E): 0≤S+I+Z+νbμV≤M, 0≤E≤Em}. |
Further, if the following inequalities are satisfied:
rK1d>(1+γγ1KEm)(βdVm+αK1Zm),βSa>(αθZmd+γγ2Em+μ)Im,α(λ1Sa+λ2θIa)d+K+θIm>ν+γγ3Em, | (3.1) |
then system (2.1) is permanent. The quantities M, Em, Sa, Im, Zm and Vm are defined in the proof.
For proof of this theorem, see Appendix A.
From the conditions in (3.1), we note that the susceptible phytoplankton may disappear from the aquatic system for very high values of force of infection or its contact rate with environmental toxins; the infected phytoplankton and hence the free-viruses may extinct for very low values of the force of infection or for very high values of its contact rate with environmental toxins; the zooplankton may extinct for very high values of its natural mortality or death rate due to environmental toxins.
In the absence of free-viruses and environmental toxins, system (2.1) reduces to,
dSdt=rS(1−SK)−αSZd+S,dZdt=λ1αSZd+S−νZ, | (3.2) |
whose dynamics have been well studied [50]. Here, we summarize its dynamics as follows. System (3.2) has the three feasible equilibria.
1. The plankton-free equilibrium point ˜e0=(0,0) is always a saddle.
2. If K(λ1α−ν)<dν, then global stability at the zooplankton-free equilibrium ˜e1=(K,0).
3. If K(λ1α−ν)>dν and K<d(λ1α+ν)λ1α−ν, then global stability at the coexistence equilibrium ˜e∗=(˜S∗,˜Z∗), where
˜S∗=dνλ1α−ν, ˜Z∗=λ1rd{K(λ1α−ν)−dν}K(λ1α−ν)2. |
4. If K(λ1α−ν)>dν and K>d(λ1α+ν)λ1α−ν, then globally stable and unique limit cycle around the coexistence equilibrium ˜e∗=(˜S∗,˜Z∗).
In the absence of free-viruses in the aquatic system, model (2.1) reduces to,
dSdt=rS1+γγ1SE(1−SK)−αSZd+S,dZdt=λ1αSZd+S−νZ−γγ3ZE,dEdt=A−γ(S+Z)E−ρE. | (3.3) |
Our model (3.3) differs from the models of [38,39] in the sense that here zooplankton are assumed to be affected by environmental toxins. In [38], the interaction between phytoplankton and zooplankton is assumed to be modified Beddington type. The effect of toxin liberation by phytoplankton on zooplankton and predation of zooplankton by fish are considered in [39]. Yu et al. [40] studied a stochastic model by considering that environmental toxins increase the death rate of phytoplankton as well as zooplankton.
System (3.3) has the following three non-negative equilibria:
1. The plankton-free equilibrium ˆe0=(0,0,A/ρ), always feasible.
2. The zooplankton-free equilibrium ˆe1=(K,0,A/(γK+ρ)), always feasible.
3. The coexistence equilibrium ˆe∗=(ˆS∗,ˆZ∗,ˆE∗), where
ˆZ∗=1γ[γγ3A(d+ˆS∗)(λ1α−ν)ˆS∗−νd−(ρ+γˆS∗)], ˆE∗=Aρ+γ(ˆS∗+ˆZ∗) |
and ˆS∗ is given by positive root of the following equation:
r1+γγ1SˆE∗(1−SK)−αˆZ∗d+S=0. | (3.4) |
Regarding local stability of equilibria of the system (3.3), we have the following theorem.
Theorem 3.2. 1. The equilibrium ˆe0 is always unstable.
2. The equilibrium ˆe1 is always stable.
3. The equilibrium ˆe∗, if feasible, is locally asymptotically stable if and only if the following conditions hold,
ˆA1>0, ˆA3>0, ˆA1ˆA2−ˆA3>0. | (3.5) |
For proof of this theorem, see Appendix B.
In the absence of environmental toxins in the aquatic system, model (2.1) reduces to,
dSdt=rS(1−SK)−βSVK1+V−αSZd+S+θI,dIdt=βSVK1+V−αθIZd+S+θI−μI,dZdt=αZ(λ1S+λ2θI)d+S+θI−νZ,dVdt=bμI−βSVK1+V−δV. | (3.6) |
In [6], authors assumed that both the healthy and infected phytoplankton are equally preferable for predation. They showed that virus replication factor and half saturation constant of viral infection play an important role in persistence of infection in the system. They also observed that for low infection rate, the virus cannot persist in the system and the system becomes infection-free. If the force of infection increases then above a threshold value, all the species coexist and the system becomes endemic. However, for very large values of force of infection, the zooplankton population extinct from the system. The preference behavior of predator over susceptible prey is considered in [41]; the explicit role of parasites is not taken into account. In [42], the authors showed that the phytoplankton-zooplankton interaction is very complex and the plankton dynamics strongly depends on the selective predation of zooplankton.
System (3.6) exhibits five non-negative equilibria given as,
1. The population-free equilibrium ¯e0=(0,0,0,0), which is always feasible.
2. The equilibrium with only susceptible phytoplankton ¯e1=(K,0,0,0), which is always feasible.
3. The disease-free equilibrium ¯e2=(¯S2,0,¯Z2,0), where ¯S2=˜S∗ and ¯Z2=˜Z∗. The equilibrium ¯e2 is feasible provided K(λ1α−ν)>dν.
4. The zooplankton-free equilibrium ¯e3=(¯S3,¯I3,0,¯V3), where ¯S3=Kr(r−β¯V3K1+¯V3), ¯I3=Kβ¯V3μr(K1+¯V3)(1−β¯V3K1+¯V3) and ¯V3 is positive root of the quadratic,
¯a2V2+¯a1V+¯a0=0, | (3.7) |
where
¯a2=rδ, ¯a1=2rK1δ−βK(b−1)(r−β), ¯a0=rK1{δK1−βK(b−1)}. |
As ¯a2>0, equation (3.7) has exactly one positive root if ¯a0<0. A necessary condition for ¯S3 to be positive is rK1+(r−β)¯V3>0. Thus, the equilibrium ¯e3 is feasible provided,
rK1+(r−β)¯V3>0, δK1−βK(b−1)<0. | (3.8) |
In case, the latter is not satisfied, then equation (3.7) has either two or no positive roots.
5. The coexistence equilibrium ¯e∗=(¯S∗,¯I∗,¯Z∗,¯V∗). In equilibrium ¯e∗, we have
¯S∗=1λ1α−ν[νd−θ(λ2α−ν)¯V∗[δ(K1+¯V∗)(λ1α−ν)+νdβ¯V∗]bμ(K1+¯V∗)(λ1α−ν)+θβ¯V∗(λ2α−ν)],¯I∗=¯V∗[δ(K1+¯V∗)(λ1α−ν)+νdβ¯V∗]bμ(K1+¯V∗)(λ1α−ν)+θβ¯V∗(λ2α−ν), ¯Z∗=d+¯S∗+θ¯I∗αθ¯I∗[β¯S∗¯V∗K1+¯V∗−μ¯I∗] | (3.9) |
and ¯V∗ is positive root of the equation
r(1−¯S∗K)−βVK1+V−α¯Z∗d+¯S∗+θ¯I∗=0. | (3.10) |
Regarding local stability of equilibria of the system (3.6), we have the following theorem.
Theorem 3.3. 1. The equilibrium ¯e0 is always unstable.
2. The equilibrium ¯e1 is locally asymptotically stable if
δK1−βK(b−1)>0, Kλ1α−ν(K+d)<0. | (3.11) |
3. The equilibrium ¯e2, if feasible, is locally asymptotically stable if and only if the following conditions are satisfied,
¯C1>0, ¯C4>0, ¯C1¯C2−¯C3>0, ¯C3(¯C1¯C2−¯C3)−¯C21¯C4>0. | (3.12) |
4. The equilibrium ¯e3, if feasible, is locally asymptotically stable provided,
¯B1>0, ¯B3>0, ¯B1¯B2−¯B3>0, α(λ1¯S3+λ2θ¯I3)d+¯S3+θ¯I3−ν<0. | (3.13) |
5. The equilibrium ¯e∗, if feasible, is locally asymptotically stable if and only if the following conditions are satisfied,
¯A1>0, ¯A4>0, ¯A1¯A2−¯A3>0, ¯A3(¯A1¯A2−¯A3)−¯A21¯A4>0. | (3.14) |
For proof of this theorem, see Appendix C.
System (2.1) exhibits the following five non-negative equilibria:
1. The equilibrium e0=(0,0,0,0,A/ρ), which is always feasible.
2. The equilibrium e1=(K,0,0,0,A/(γK+ρ)), which is always feasible.
3. The equilibrium e2=(S2,0,Z2,0,E2), where S2=ˆS∗, Z2=ˆZ∗ and E2=ˆE∗.
4. The equilibrium e3=(S3,I3,0,V3,E3), where
I3=1bμ[δV3+βS3V3K1+V3], E3=Aρ+γ{S3+1bμ(δV3+βS3V3K1+V3)}, |
S3 and V3 are positive solutions of the following isoclines:
r(1−S/K)[ρ+γ{S+1bμ(δV+βSVK1+V)}]ρ+γ{S+1bμ(δV+βSVK1+V)+Aγ1S}−βVK1+V=0,βSVK1+V−1bμ(δV+βSVK1+V)[μ+Aγγ2ρ+γ{S+1bμ(δV+βSVK1+V)}]=0. |
5. The equilibrium e∗=(S∗,I∗,Z∗,V∗,E∗), where
E∗=1γγ3[α{λ1S∗+λ2θbμ(δV∗+βS∗V∗K1+V∗)}d+S∗+θbμ(δV∗+βS∗V∗K1+V∗)−ν], I∗=1bμ(δV∗+βS∗V∗K1+V∗),Z∗=1γ[γγ3A{d+S∗+θbμ(δV∗+βS∗V∗K1+V∗)}S∗(αλ1−ν)−νd+θ(αλ2−ν)bμ(δV∗+βS∗V∗K1+V∗)−ρ−γ{S∗+1bμ(δV∗+βS∗V∗K1+V∗)}], |
S∗ and V∗ are positive solutions of the following isoclines:
r1+γγ1SE∗(1−SK)−βV∗K1+V∗−αZ∗d+S+θI∗=0, | (3.15) |
βSVK1+V−αθI∗Z∗d+S+θI∗−γγ2I∗E∗−μI∗=0. | (3.16) |
Regarding local stability of equilibria of the system (2.1), we have the following theorem.
Theorem 3.4. 1. The equilibrium e0 is always unstable.
2. The equilibrium e1 is locally asymptotically stable provided,
ν+γγ3AγK+ρ>αλ1Kd+K, (μ+γγ2AγK+ρ)(δ+βKK1)>bβμKK1. | (3.17) |
3. The equilibrium e2, if feasible, is locally asymptotically stable if and only if the following conditions hold,
A5>0, A1A2−A3>0, A3(A1A2−A3)−A1(A1A4−A5)>0,A4{A3(A1A2−A3)−A1(A1A4−A5)}−A5{A2(A1A2−A3)−(A1A4−A5)}>0. | (3.18) |
4. The equilibrium e3, if feasible, is locally asymptotically stable if and only if the following conditions hold,
B5>0, B1B2−B3>0, B3(B1B2−B3)−B1(B1B4−B5)>0,B4{B3(B1B2−B3)−B1(B1B4−B5)}−B5{B2(B1B2−B3)−(B1B4−B5)}>0. | (3.19) |
5. The equilibrium e∗, if feasible, is locally asymptotically stable if and only if the following conditions hold,
C5>0, C1C2−C3>0, C3(C1C2−C3)−C1(C1C4−C5)>0,C4{C3(C1C2−C3)−C1(C1C4−C5)}−C5{C2(C1C2−C3)−(C1C4−C5)}>0. | (3.20) |
For proof of this theorem, see Appendix D.
In the context of a biological system, bifurcations describe how the system dynamics may qualitatively drastically change if a parameter varies. A Hopf bifurcation is a critical point where a system's stability switches and a periodic solution arises. We consider here some parameters: selectivity/preference of zooplankton (θ), force of infection (β), growth suppression of susceptible phytoplankton due to environmental toxins (γ1) and carrying capacity of susceptible phytoplankton (K) as bifurcation parameters. Analytically we investigate for the possibility of Hopf bifurcation from the coexistence equilibrium e∗ by taking the selectivity/preference of zooplankton (θ) as bifurcation parameter, keeping other parameters fixed.
Regarding Hopf bifurcation of the system (2.1) around equilibrium e∗ with respect to the parameter θ, we have the following theorem.
Theorem 3.5. When the intensity of selectivity (θ) exceeds a critical value, the system (2.1) enters into Hopf-bifurcation around the coexistence equilibrium e∗. The necessary and sufficient conditions for the occurrence of Hopf bifurcation are that there exists θ=θ∗ such that
1. ψ(θ∗)≡{C3(θ∗)−C1(θ∗)C2(θ∗)}{C5(θ∗)C2(θ∗)−C3(θ∗)C4(θ∗)}−{C5(θ∗)−C1(θ∗)C4(θ∗)}2=0.
2. C1(θ∗)>0, C1(θ∗)C2(θ∗)−C3(θ∗)>0, C3(θ∗)−C1(θ∗)ω∗0>0, ω∗0=C5(θ∗)−C1(θ∗)C4(θ∗)C3(θ∗)−C1(θ∗)C2(θ∗)>0.
3. dψ(θ)dθ|θ=θ∗=0.
For proof of this theorem, see Appendix E.
In Table 2, we listed the equilibria of the systems (2.1), (3.2), (3.3) and (3.6), and the conditions for their stability.
Models | Equilibria | Conditions for LAS |
(3.2) | ˜e0=(0,0)) | Always unstable |
˜e1=(K,0) | K(λ1α−ν)<dν | |
˜e∗=(˜S∗,˜Z∗) | K(λ1α−ν)>dν, K<d(λ1α+ν)λ1α−ν | |
(3.3) | ˆe0=(0,0,A/ρ) | Always unstable |
ˆe1=(K,0,A/(γK+ρ)) | Always stable | |
ˆe∗=(ˆS∗,ˆZ∗,ˆE∗) | Conditions in (3.5) | |
(3.6) | ¯e0=(0,0,0,0) | Always unstable |
¯e1=(K,0,0,0) | Conditions in (3.11) | |
¯e2=(¯S2,0,¯Z2,0) | Conditions in (3.12) | |
¯e3=(¯S3,¯I3,0,¯V3) | Conditions in (3.13) | |
¯e∗=(¯S∗,¯I∗,¯Z∗,¯V∗) | Conditions in (3.14) | |
(2.1) | e0=(0,0,0,0,A/ρ) | Always unstable |
e1=(K,0,0,0,A/(γK+ρ)) | Conditions in (3.17) | |
e2=(S2,0,Z2,0,E2) | Conditions in (3.18) | |
e3=(S3,I3,0,V3,E3) | Conditions in (3.19) | |
e∗=(S∗,I∗,Z∗,V∗,E∗) | Conditions in (3.20) |
Here, we report the simulations to investigate the behavior of system (2.1), performed using the Matlab variable step Runge-Kutta solver ode45. The set of parameter values are chosen within the range prescribed in various previous literature sources [6,34,38,39,41,42], and are given in Table 1. Unless it is mentioned, the values of parameters used for numerical simulations are the same as in Table 1. In Table 3, we listed the equilibria of the systems (2.1), (3.2), (3.3) and (3.6), and the corresponding eigenvalues of the system's Jacobian. In Table 3, we choose parameter values in such a way that coexistence equilibrium of each system is stable. For system (2.1), we choose parameter values as in Table 1, while for other systems, the changed parameters are: α=0.03 in system (3.2); α=0.057 in system (3.3); α=0.057 and θ=0.15 in system (3.6). From the table, we note that the system (3.3) has two coexistence equilibria: ˆe∗1 and ˆe∗2, of which the former is stable and the latter is unstable. Also, the equilibria ˆe1 and ˆe∗1 are simultaneously stable, thus the system (3.3) shows bistability behavior between these two equilibria. Bistability is a phenomenon where the system can converge to two different equilibria in the same parametric region based on the variation of the initial conditions.
Models | Equilibria | Eigenvalues of the system's Jacobian |
(3.2) | ˜e0=(0,0)) | 1.1999, −0.0120 |
˜e1=(10,0) | −1.1999, 0.0030 | |
˜e∗=(5.7142,183.6734) | −0.4043, −0.0071 | |
(3.3) | ˆe0=(0,0,24) | −0.2500, 1.1999, −0.1920 |
ˆe1=(10,0,3.4285)) | −1.7500, −0.7924, −0.0092 | |
ˆe∗1=(2.5599,116.9310,0.3301) | −0.0066±0.0911i, −18.1762 | |
ˆe∗2=(9.6963,7.1492,2.1607) | −2.7882, −0.8680, 0.0057 | |
(3.6) | ¯e0=(0,0,0,0) | 1.1999, −0.2300, −0.2000, −0.0120 |
¯e1=(10,0,0,0) | −1.1999, 2.5825, −4.5125, 0.0165 | |
¯e2=(1.9512,0,117.7870,0) | 0.0184±0.0894i, 1.0989, −1.9665 | |
¯e3=(6.2735,14.0269,0,475.6954) | −0.7533, −0.2313, −0.1981, 0.0145 | |
¯e∗=(1.6059,2.4375,70.1090,81.7521) | −0.3601, −0.1733, −0.0194±0.0687i | |
(2.1) | e0=(0,0,0,0,24) | −0.2500, 1.1999, −0.1920, −0.2300, −0.2719 |
e1=(10,0,0,0,3.4285) | −1.7500, −0.7924, −0.0377, −4.5166, 2.5763 | |
e2=(0.8666,0,35.2363,0,1.0590) | 0.0886, −0.0583, −5.6732, −1.3824, 0.6571 | |
e3=(5.6319,12.2052,0,413.5891,2.0508) | −2.9498, −0.6434, −0.2289, −0.1814, 0.0273 | |
e∗=(0.8860,1.2285,21.9939,41.1149,1.5518) | −3.8772, −0.3882, −0.1071±0.0316i, −0.0290 |
First, we investigate the dynamics of the system (2.1) in the absence of free viruses and environmental toxins i.e., subsystem (3.2), Figure 2. We observe that for system (3.2), the coexistence equilibrium ˜e∗ is stable at α=0.03 but the stability is lost on increasing the values of α or K. Moreover, on decreasing the values of λ1, the coexistence equilibrium ˜e∗ losses its stability and the zooplankton-free equilibrium ˜e1 appears in the system. We observe that the system (3.2) is oscillatory at α=0.057, while the introduction of environmental toxins in system (i.e., system (3.3)) makes it stable (Figure 3(a)), but for higher values of input rate of environmental toxins (A=20.5), the system settles to zooplankton-free steady state (Figure 3(b)). Next, we see bistability behavior of the system (3.3) in Figure 4(a). Here, blue region stands for stability domain for the equilibrium ˆe1, and red region stands for stability domain for the equilibria ˆe1 and ˆe∗1. We observe that the system (3.3) shows bistability between the zooplankton-free equilibrium ˆe1 and the coexistence equilibrium ˆe∗1 for large input rate of environmental toxins in the aquatic system. Thus, high input rate of environmental toxins leads to extinction of zooplankton or persistence of all the populations depending on the initial conditions. We also plot basin of attractions to confirm bistability behavior of the system. In Figure 4(b), magenta region represents the basin of attraction for the equilibrium ˆe1, and the green region represents the basin of attraction for the equilibrium ˆe∗1. Next, we observe the dynamics of system (3.6), i.e., the system with free-viruses but without environmental toxins. We observe that the introduction of free-viruses drives the unstable equilibrium of S−Z system to stable equilibrium of S−I−Z−V system, Figure 5. On increasing the preference of zooplankton on infected phytoplankton, system (3.6) shows limit cycle oscillation around the disease-free equilibrium, ¯e2 (see Figure 6). Next, we observe the behavior of system (3.6) by varying the force of infection, β (see Figure 7). We find that for low values of force of infection, the system is oscillatory around the disease-free equilibrium, but the oscillation is around the coexistence equilibrium on increasing the values of force of infection; further increase in the values of force of infection results in stable coexistence of all the populations.
We employed a global sensitivity analysis to assess the impact of uncertainty and the sensitivity of the outcomes of the numerical simulations to variations in each parameter of the model (2.1) using Latin Hypercube Sampling (LHS) and partial rank correlation coefficients (PRCC) [51,52]. To generate the LHS matrices, we assume that all the model parameters are uniformly distributed. We performed PRCC analyses for three different time points in order to investigate which parameters consistently influence free-viruses and environmental toxins in time. Nonlinear and monotone relationships are observed for the free-viruses and environmental toxins with the input parameters of the model (2.1), which is a prerequisite for computing PRCCs. Then, simulations of the model per LHS run were carried out, using the baseline values tabulated in Table 4 and the ranges as 25% from the baseline values (in either direction). The indexes are evaluated at the time points 200,400 and 600 days. For each of the three time points, denoted by 1, 2 and 3, PRCC results are shown in Figs. 8(a) & 8(b) for free-viruses and environmental toxins, respectively. We observe that the PRCC values of free-viruses decrease with time for the parameters, α, d, ν, γ1 and γ3 while for the environmental toxins, the PRCC values decrease for the parameters β and θ, and increases for the parameter A, indicating that these parameters consistently influence free-viruses/environmental toxins in the system; for other parameters there is no trend of either increasing or decreasing in the PRCC's values.
Parameters | Baseline values | Minimum values | Maximum values |
r | 1.2 | 0.9000 | 1.5000 |
K | 10 | 7.5000 | 12.5000 |
β | 0.45 | 0.3375 | 0.5626 |
K1 | 3 | 2.2500 | 3.7500 |
α | 0.18 | 0.1350 | 0.2250 |
θ | 0.15 | 0.1125 | 0.1875 |
d | 5 | 3.7500 | 6.2500 |
λ1 | 0.75 | 0.5625 | 0.9375 |
λ2 | 0.72 | 0.5400 | 0.9000 |
μ | 0.2 | 0.1500 | 0.2500 |
ν | 0.012 | 0.0090 | 0.0150 |
b | 40 | 30.0000 | 50.0000 |
δ | 0.23 | 0.1725 | 0.2875 |
A | 6 | 4.5000 | 7.5000 |
γ | 0.15 | 0.1125 | 0.1875 |
γ1 | 0.1 | 0.0750 | 0.1250 |
γ2 | 0.02 | 0.0150 | 0.0250 |
γ3 | 0.05 | 0.0375 | 0.0625 |
ρ | 0.25 | 0.1875 | 0.3125 |
We discuss the Hopf bifurcation near the coexistence equilibrium e∗ on varying the intensity of selectivity (θ), inhibition of phytoplankton growth due to environmental toxins (γ1), force of infection (β), and carrying capacity of susceptible phytoplankton (K). For the set of parameter values in Table 1, system (2.1) shows stable coexistence of all the populations (see Figure 9) but at θ=1.8, we observe that the system shows limit cycle oscillations around the coexistence equilibrium (see Figure 10). To get a clear view, we vary θ from 0 to 6 and draw bifurcation diagram of system (2.1) with respect to θ, Figure 11. We observe that for low values of θ, the system shows stable coexistence of all the populations, but shows limit cycle oscillations around the coexistence equilibrium e∗ after a critical value of θ; further increase in the values of θ leads to instability of the infection-free equilibrium e2. Next, we draw the bifurcation diagram of the system (2.1) by varying the parameter γ1 in the interval [0, 0.2], Figure 12. We get that for very low values of γ1, the solution trajectories are oscillating around the coexistence equilibrium but the solution trajectories become stable after a threshold value of γ1. Now, we vary the force of infection (β) in the interval [0, 1] and draw bifurcation diagram of the system (2.1), Figure 13. We observe that for very low values of β, the infection-free equilibrium e2 is unstable, but increase in the values of β results in the instability of the coexistence equilibrium, e∗. The equilibrium e∗ becomes stable for higher values of β. For very large values of force of infection, the system settles to the zooplankton-free steady state. Next, by varying the values of K in the interval $ [5,15] $, we plot bifurcation diagram of the system (2.1), Figure 14. We observe that the stable equilibrium becomes unstable with high-amplitude oscillations with increasing values of K. It is apparent from Figure 15 that for large values of input rate of environmental toxins, the zooplankton-free equilibrium, e3, is stable.
Next, we investigate how the stability of the system changes on varying two parameters at a time: (θ,A) and (θ,β), Figure 16. For this reason, we vary θ and A, and plot stability regions in (θ,A) plane, Figure 16(a). Here, regions green, red, blue, black and magenta correspond to zooplankton-free, stable coexistence, unstable coexistence, unstable infection-free, and stable infection-free domain, respectively. For low values of θ (θ<1) and high values of A, we get a zooplankton-free system. By increasing the values of θ, while keeping A at low values, the infection-free equilibrium is unstable while for large values of both θ and A, the infection-free equilibrium is stable. For low values of θ, the coexistence equilibrium is stable while for moderate values of θ, the coexistence equilibrium is unstable. It is apparent from Figure 16(b) that on increasing the values of force of infection, the system becomes zooplankton-free irrespective of the preference of zooplankton for phytoplankton. For low values of β, on increasing θ, the infection-free equilibrium is stable while on increasing the values of θ and moderate values of β, the coexistence equilibrium is stable.
Now, we see the effects of replication factor of free-viruses and input rate of environmental toxins in the aquatic system on the equilibrium abundances of all the densities for the systems (2.1), (3.2), (3.3) and (3.6), Figure 17. The comparisons between equilibrium levels of all the populations for different values of virus replication factor (b) and input rate of environmental toxins (A) are given in Table 5. We observe that the susceptible phytoplankton is at high equilibrium level if there is no viruses in the system and input rate of toxins is very low; while the susceptible phytoplankton is at low level in the absence of toxins but high value of virus replication factor. The infected phytoplankton is at high equilibrium level if virus replication factor is high, and becomes zero in the absence of free-viruses. The zooplankton population is maximum when the system is free of viruses and toxins but becomes very low in their presence. High values of replication factor of viruses and input rate of toxins maintained the high levels of these two environmental degrading agents. Next, we see the effect of two controllable parameters, decay rate of free-viruses and depletion rate of environmental toxins, on the equilibrium levels of variables in system (2.1), Figure 18. We see that zooplankton are at higher density for high values of these two rate parameters in comparison to the case when these parameters are at low values. Opposite situations occur for other variables of the system. Further, we see the percentage increase/decrease in system's variables by varying the decay rate of free-viruses (δ) and depletion rate of environmental toxins (ρ) in the interval [0.01, 0.99], Figure 19. From the figure, it is clear that the concentration of zooplankton changes significantly on increasing the decay rate of free-viruses and depletion rate of environmental toxins; the free-viruses are highly sensitive to its death rate.
State variables | Comparison |
Susceptible phytoplankton (S) | ˆS∗>˜S∗>S∗>¯S∗ |
Infected phytoplankton (I) | I∗>¯I∗>ˆI∗=˜I∗=0 |
Zooplankton (Z) | ˜Z∗>ˆZ∗>¯Z∗>Z∗ |
Free-viruses (V) | V∗>¯V∗>ˆV∗=˜V∗=0 |
Environmental toxins (E) | E∗>˜E∗>¯E∗=˜E∗=0 |
In this paper, we proposed a model that incorporates zooplankton's selectivity on susceptible phytoplankton in the presence of infective ones, and seen the combined effects of marine viruses and environmental toxins on the dynamics of phytoplankton-zooplankton interactions. We studied the dynamics of full as well as submodels. We observed that in the absence of free-viruses and environmental toxins in the ecosystem, the system is stable for low values of capture rate of susceptible phytoplankton by zooplankton and carrying capacity of susceptible phytoplankton. But, increase in either of these two parameters, drives the system to an oscillatory state. If the reproductive gain of zooplankton on consumption of susceptible phytoplankton is very low, then the system will become zooplankton-free. We got that the introduction of environmental toxins in the system makes it stable if the system with susceptible phytoplankton and zooplankton is already oscillatory. Moreover, high input rate of environmental toxins results in the extinction of zooplankton population, which is not reported in the previous studies [38,39]. The extinction of zooplankton may be due to the fact that environmental toxins increase their death rate. Previously, the destabilizing effects of input rate of environmental toxins and its contact rate with phytoplankton, and the stabilizing effects of depletion rate of environmental toxins have been reported [38,39]. Also, we observed that the presence of free-viruses in the ecosystem can stabilize an unstable equilibrium of the S−Z system. But, increase in the preference of zooplankton on infected phytoplankton results in the oscillation of the system around disease-free steady state, which was not obtained in the previous study [6]. We found that for low values of force of infection, the system is oscillatory around the disease-free equilibrium, but the oscillation is around the coexistence equilibrium on increasing the values of force of infection; further increase in the values of force of infection results in stable coexistence of all the populations.
For system (2.1), we observed that for low values of preference of zooplankton on infected phytoplankton, the coexistence equilibrium is stable, but the coexistence equilibrium becomes unstable after a threshold value of preference of zooplankton; further increase in the values of preference of zooplankton on infected phytoplankton leads to instability of the infection-free equilibrium. Note that selectivity behavior of zooplankton was not considered in the previous study [6]. On increasing the values of the parameter related to inhibition of growth rate of susceptible phytoplankton due to environmental toxins, the system get stabilized. It is noted that for very low values of force of infection, the infection-free equilibrium is unstable but increase in the values of force of infection leads to the instability of the coexistence equilibrium; for higher values of force of infection a stable coexistence of all the populations is observed. Moreover, the ecosystem becomes zooplankton-free for very high values of force of infection. We observed that the stable equilibrium becomes unstable with increasing the carrying capacity of susceptible phytoplankton, i.e., increasing nutrient supply, which supports the existing hypothesis of the paradox of enrichment [53].
The results of sensitivity analysis suggest that a strategy that reduces the parameters having positive PRCC values with either free-viruses or environmental toxins will adequately reduce the density of phytoplankton in the system. Furthermore, a strategy that increases the parameters having negative PRCC values with free-viruses (environmental toxins) will be effective in curtailing free-viruses (environmental toxins) in the system. Identification of these key parameters is important to the formulation of effective control strategies necessary for combating free-viruses and environmental toxins in the aquatic system. Comparison of the equilibrium values of variables in full and subsystems shows that the replication factor of free-viruses and input rate of environmental toxins in the ecosystems can be used as biological controls to combat the reductions in zooplankton and healthy phytoplankton populations. As different parameters show important effects in reducing the concentration of zooplankton, from modeling and analysis perspective, we can conclude that the concentration of zooplankton can be maintained by increasing the decay rate of free-viruses and depletion rate of environmental toxins. For the control of infected phytoplankton in the system, it is imperative to decrease free-viruses from that system by using antiviral reagents [54,55]. Bioremediation technology could be very useful to convert the toxigenic compounds to nontoxic products without further disruption to the local environment, which will enhance the persistence and stability of the populations [56]. Although environmental toxins are thought not to be entering marine ecosystems in large quantities yet, experimental evidence reveals the fact that phytoplankton populations are highly vulnerable to damage from environmental toxins [57]. Sometimes, for high concentration of environmental toxins, complete growth inhibition in phytoplankton was observed [58]. So, there might be a possibility of the population crash. But, it can be controlled through the higher depletion rate of environmental toxins. Regulating the depletion rate of environmental toxins could be an effective control strategy to prevent the crash of the aquatic food web system, which could be achieved with suitable human activities such as safe use of pesticides, chemical toxins, awareness among human etc.
The authors thank the associate editor and the anonymous reviewers for valuable comments, which contributed to the improvement in the presentation of the paper. The research work of Saswati Biswas is supported by Council of Scientific and Industrial Research, Government of India, New Delhi in the form of Senior Research Fellowship (Ref. No. 20/12/2015(ⅱ)EU-V). Pankaj Kumar Tiwari is thankful to University Grants Commissions, New Delhi, India for providing financial support in form of D. S. Kothari post-doctoral fellowship (No.F.4-2/2006 (BSR)/MA/17-18/0021). The work of Yun Kang is partially supported by NSF-DMS (1313312 & 1716802); NSF-IOS/DMS (1558127), DARPA (ASC-SIM Ⅱ), and The James S. McDonnell Foundation 21st Century Science Initiative in Studying Complex Systems Scholar Award (UHC Scholar Award 220020472).
The authors declare that there is no conflict of interest in this paper.
[1] | R. M. Anderson and R. M. May, The invasion, persistence, and spread of infectious diseases within animal and plant communities, Philos. Trans. R. Soc. Lond. B, 314 (1986), 533-570. |
[2] | J. Chattopadhyay and N. Bairagi, Pelicans at risk in Salton Sea - an eco-epidemiological model, Ecol. Model., 136 (2001), 103-112. |
[3] | Y. Xiao and L. Chen, Modelling and analysis of a predator-prey model with disease in the prey, Math. Biosci., 171 (2001), 59-82. |
[4] | E. Venturino, Epidemics in predator-prey models: Disease in the predators, IMA J. Math. Appl. Med. Biol., 19 (2002), 185-205. |
[5] | K. Hadeler and H.I. Freedman, Predator-prey population with parasite infection, J. Math. Biol., 27 (1989), 609-631. |
[6] | S. Samanta, R. Dhar, J. Pal, et al., Effect of enrichment on plankton dynamics where phytoplankton can be infected from free viruses, Nonlinear Studies, 20 (2013), 223-236. |
[7] | K. D. Lafferty and A. K. Morris, Altered behaviour of parasitized killfish increases susceptibility to predation by bird final hosts, Ecology, 77 (1996), 1390-1397. |
[8] | W. R. DeMott, Optimal foraging theory as a predictor of chemically mediated food selection by suspension-feeding copepods, Limnol. Oceanogr., 34 (1989), 140-154. |
[9] | M. A. Leibold, Biodiversity and nutrient enrichment in pond plankton communities, Evol. Ecol. Res., 1 (1999), 73-95. |
[10] | J. L. Brooks and S. I. Dodson, Predation, body size, and composition of plankton, Science, 150 (1965), 28-35. |
[11] | A. Mitra, C. Castellanib, W. C. Gentleman, et al., Bridging the gap between marine biogeochemical and fisheries sciences; configuring the zooplankton link, Prog. Oceanogr., 129 (2014), 176-199. |
[12] | M. D. Troch, M. Grego, V. A. Chepurnov, et al., Food patch size, food concentration and grazing efficiency of the harpacticoid Paramphiascella fulvofasciata (Crustacea, Copepoda), J. Exp. Mar. Biol. Ecol., 343 (2007), 210-216. |
[13] | W. R. DeMott, Discrimination between algae and artificial particles by freshwater and marine copepods, Limnol. Oceanogr., 33 (1988), 397-408. |
[14] | J. Pal, S. Bhattacharya and J. Chattopadhyay, Does predator go for size selection or preferential toxic-nontoxic species under limited resource?, OJBS, 10 (2010), 11-16. |
[15] | N. Aberle, H. Hillebrand, J. Grey, et al., Selectivity and competitive interactions between two benthic invertebrate grazers (Asellus aquaticus and Potamopyrgus antipodarum): An experimental study using 13C-and 15N-labelled diatoms, Freshwater Biol., 50 (2005), 369-379. |
[16] | M. G. Danielsdottir, M. T. Brett, G. B. Arhonditsis, Phytoplankton food quality control of planktonic food web processes, Hydrobiologia, 589 (2007), 29-41. |
[17] |
M. Huntley, P. Sykes, S. Rohan, et al., Chemically-mediated rejection of dinoflagellate prey by the copepods Calanus pacificus and Paracalanus parvus: mechanism, occurrence and significance, Mar. Ecol. Prog. Ser., 28 (1986), 105-120. doi: 10.3354/meps028105
![]() |
[18] | R. S. Fulton III and H.W. Paerl, Effects of colonial morphology on zooplankton utilization of algal resources during blue-green algal (Microcystis aeruginosa) blooms, Limnol. Oceanogr. 32 (1987), 634-644. |
[19] | G. A. Paffenhofer and K. B. Van Sant, The feeding response of a marine planktonic copepod to quantity and quality of particles, Mar. Ecol. Prog. Ser. 27 (1985), 55-65. |
[20] | C. Evans, D. W. Pond and W. H. Wilson, Changes in Emiliania huxleyi fatty acid profiles during infection with E. huxleyi virus 86: physiological and ecological implications, Aquat. Microb. Ecol., 55 (2009), 219-228. |
[21] | G. Bratbak, J. K. Egge and M. Heldal, Viral mortality of the marine alga Emiliania huxleyi (Haptophyceae) and termination of algal blooms, Mar. Ecol. Prog. Ser., 93 (1993), 39-48. |
[22] | C. Evans and W.H. Wilson, Preferential grazing of Oxyrrhis marina on virus infected Emiliania huxleyi, Limnol. Oceanogr., 53 (2008), 2035-2040. |
[23] |
A. Vermont, J. M. Martnez, J. D. Waller, et al., Virus infection of Emiliania huxleyi deters grazing by the copepod Acartia tonsa, J. Plankton Res., 38 (2016), 1194-1205. doi: 10.1093/plankt/fbw064
![]() |
[24] |
D. W. Townsend, M. D. Keller, P. M. Holligan, et al., Blooms of the coccolithophore Emiliania huxleyi with respect to hydrography in the Gulf of Maine, Cont. Shelf Res., 14 (1994), 979-1000. doi: 10.1016/0278-4343(94)90060-4
![]() |
[25] | W. H. Wilson, G. A. Tarran, D. Schroeder, et al., Isolation of viruses responsible for the demise of an Emiliania huxleyi bloom in the English Channel, J. Mar. Biol. Assoc. U.K., 82 (2002), 369-377. |
[26] | C. Evans, S. V. Kadner, L. J. Darroch, et al., The relative significance of viral lysis and microzooplankton grazing as pathways of dimethylsulfoniopropionate (DMSP) cleavage: An Emiliania huxleyi culture study, Limnol. Oceanogr., 52 (2007), 1036-1045. |
[27] |
S. Strom, G. Wolfe, J. Holmes, et al., Chemical defense in the microplankton I: Feeding and growth rates of heterophic protists on the DMS-producing phytoplankter Emiliania huxleyi, Limnol. Oceanogr., 48 (2003), 217-229. doi: 10.4319/lo.2003.48.1.0217
![]() |
[28] | G. V. Wolfe and M. Steinke, Grazing-activated production of dimethyl sulfide (DMS) by two clones of Emiliania huxleyi, Limnol. Oceanogr., 41 (1996), 1151-1160. |
[29] | M. Steinke, G. Malin and P. S. Liss, Trophic interaction in the sea: an ecological role for climate relevant volatiles? J. Phycol., 38 (2002), 630-630. |
[30] | E. Beretta and Y. Kuang, Modeling and analysis of a marine bacteriophage infection, Math. Biosci., 149 (1998), 57-76. |
[31] | E. Beltrami and T.O. Carroll, Modeling the role of viral disease in recurrent phytoplankton blooms, J. Math. Biol., 32 (1994), 857-863. |
[32] | S. Gakkhar and K. Negi, A mathematical model for viral infection in toxin producing phytoplankton and zooplankton system, Appl. Math. Comp., 179 (2006), 301-313. |
[33] | J. Chattopadhyay and S. Pal, Viral infection on phytoplankton-zooplankton system: A mathematical model, Ecol. Model., 151 (2002), 15-28. |
[34] |
N. Bairagi, P. K. Roy, R. R. Sarkar, et al., Virus replication factor may be a controlling agent for obtaining disease-free system in a multi-species eco-epidemiological system, J. Biol. Syst., 13 (2005), 245-259. doi: 10.1142/S0218339005001501
![]() |
[35] | J. Labille and J. Brant, Stability of nanoparticles in water, Nanomedicine, 5 (2010), 985-998. |
[36] | A. J. Miao, K. A. Schwehr, C. Xu, et al., The algal toxicity of silver engineered nanoparticles and detoxification by exopolymeric substances, Environ. Pollut., 157 (2009), 3034-3041. |
[37] | R. J. Miller, S. Bennett, A. A. Keller, et al., TiO2 nanoparticles are phototoxic to marine phytoplankton, PLoS ONE, 7 (2012), e30321. |
[38] | S. Rana, S. Samanta, S.Bhattacharya, et al., The effect of nanoparticles on plankton dynamics: A mathematical model, BioSystems, 127 (2015), 28-41. |
[39] | P. Panja, S. K. Mondal and D. K. Jana, Effects of toxicants on phytoplankton-zooplankton-fish dynamics and harvesting, Chaos Solit. Fract., 104 (2017), 389-399. |
[40] | X. Yu, S. Yuan and T. Zhang, Survival and ergodicity of a stochastic phytoplankton-zooplankton model with toxin-producing phytoplankton in an impulsive polluted environment, Appl. Math. Comp., 347 (2019), 249-264. |
[41] | N. Bairagi and D. Adak, Complex dynamics of a predator-prey-parasite system: An interplay among infection rate, predator's reproductive gain and preference, Ecol. Compl., 22 (2015), 1-12. |
[42] | N. Bairagi, S. Saha, S. Chaudhuri, et al., Zooplankton selectivity and nutritional value of phytoplankton influences a rich variety of dynamics in a plankton population model, Phy. Rev. E, 99 (2019), 012406. |
[43] | K. Bester, H. Hhnerfuss, U. Brockmann, et al., Biological effects of triazine herbicide contamination on marine phytoplankton, Arch. Environ. Contam. Toxicol., 29 (1995), 277-283. |
[44] | R. C. Antweiler, C. J. Patton and H. E. Taylor, Nutrients, in chemical data for water samples collected during four upriver cruises on the Mississippi river between New Orleans, Louisiana, and Minneapolis, Minnesota, May 1990-April 1992, J.A. Moody, ed., U.S. Geological Survey Open-File Report, 94-523 (1995), 89-125. |
[45] | U.S. Environmental Protection Agency Great Lakes National Program Office Significant Activities Report. http://www.epa.gov/glnpo/aoc/waukegan.html. |
[46] | J. R. Rueter, S. W. Chisholm and F. Morel, Effects of copper toxicity on silicon acid uptake and growth in Thalassiosira pseudonana, J. Phycol. 17 (1981) 270-278. |
[47] | J. C. Holmes and W. M. Bethel, Modification of intermediate host behavior by parasites, In: Canning, E.V., Wright, C.A. (Eds.), Behavioral Aspects of Parasite Transmission. Suppl. I to Zool. f. Linnean Soc., 51 (1972), 123-149. |
[48] | K. D. Lafferty, Foraging on prey that are modified by parasites, Am. Nat., 140 (1992), 854-867. |
[49] | W. D. Hamilton, R. Axelrod and R. Tanese, Sexual reproduction as an adaptation to resist parasite: a review, Proc. Natl. Acad. Sci. USA, 87 (1990), 3566-3573. |
[50] | H. L. Smith, The Rosenzweig-Macarthur predator-prey model, https://math.la.asu.edu/halsmith/Rosenzweig.pdf. |
[51] | S. M. Blower and H. Dowlatabadi, Sensitivity and uncertainty analysis of complex models of disease transmission: An HIV model, as an example, Int. Stat. Rev., 62 (1994), 229-243. |
[52] | S. Marino, I. B. Hogue, C. J. Ray, et al., A methodology for performing global uncertainty and sensitivity analysis in systems biology, J. Theor. Biol., 254 (2008), 178-196. |
[53] | M. L. Rosenzweig, Paradox of enrichment: destabilization of exploitation ecosystems in ecological time, Science, 171 (1971), 385. |
[54] | A. C. Baudoux, A. Noordeloos, M. Veldhuis, et al., Virally induced mortality of Phaeocystis globosa during two spring blooms in temperate coastal waters, Aquat. Microb. Ecol., 44 (2006), 207-217. |
[55] | C. Evans, S. D. Archer, J. Stphan, et al., Direct estimates of the contribution of viral lysis and microzooplankton grazing to the decline of a Micromonas spp. population, Aquat. Microb. Ecol., 30 (2003), 207-219. |
[56] | A. Chhater, H. Purohit, R. Shanker, et al., Bacterial consortia for crude oil spill remediation, Wat. Sci. Technol., 34 (1996), 187-193. |
[57] | B. D. Duval, UV from sunlight excites nanoparticles to kill phytoplankton in lab setting, (2012) http://earthsky.org/human-world/uv-from-sunlight-excites-nanoparticles-to-kill-phytoplankton-in-lab-setting/. |
[58] | A. Miao, X. Y. Zhang, Z. Luo, et al., Zinc oxide-engineered nanoparticles: dissolution and toxicity to marine phytoplankton, Environ. Toxicol. Chem., 29 (2010), 2814-2822. |
[59] | G. Birkhoff and G. C. Rota, Ordinary Differential Equations, 4th edn. John Wiley & Sons, New York (1989). |
[60] | J. K. Hale, Introduction to Functional Differential Equations, vol. 99, Springer, Berlin (1993). |
[61] | X. Yang, L. Chen and J. Chen, Permanence and positive periodic solution for the single-species nonautonomous delay diffusive models, Comput. Math. Appl., 32 (1996), 109-116. |
[62] | V. Lakshmikantham, S. Leela and A. A. Martynyuk, Stability Analysis of Nonlinear Systems, Marcel Dekker, Inc., New York/Basel (1989). |
[63] | G. Gandolfo, Economic Dynamics, Springer, New York, USA (1996). |
1. | Arindam Mandal, Pankaj Kumar Tiwari, Samares Pal, Impact of awareness on environmental toxins affecting plankton dynamics: a mathematical implication, 2020, 1598-5865, 10.1007/s12190-020-01441-5 | |
2. | Arindam Mandal, Saswati Biswas, Samares Pal, Toxicity-mediated regime shifts in a contaminated nutrient–plankton system, 2023, 33, 1054-1500, 023106, 10.1063/5.0122206 | |
3. | Saswati Biswas, Pankaj Kumar Tiwari, Samares Pal, Effects of toxicity and zooplankton selectivity on plankton dynamics under seasonal patterns of viruses with time delay, 2022, 45, 0170-4214, 585, 10.1002/mma.7799 | |
4. | Vladimir G. Dvoretsky, Marina P. Venger, Anastasya V. Vashchenko, Tatyana M. Maksimovskaya, Tatyana G. Ishkulova, Veronika V. Vodopianova, Pelagic Bacteria and Viruses in a High Arctic Region: Environmental Control in the Autumn Period, 2022, 11, 2079-7737, 845, 10.3390/biology11060845 | |
5. | Sourav Kumar Sasmal, Yasuhiro Takeuchi, Editorial: Mathematical Modeling to Solve the Problems in Life Sciences, 2020, 17, 1551-0018, 2967, 10.3934/mbe.2020167 | |
6. | Xiaomei Feng, Yuan Miao, Shulin Sun, Lei Wang, Dynamic Behaviors of a Stochastic Eco-Epidemiological Model for Viral Infection in the Toxin-Producing Phytoplankton and Zooplankton System, 2022, 10, 2227-7390, 1218, 10.3390/math10081218 | |
7. | SASANKA SHEKHAR MAITY, PANKAJ KUMAR TIWARI, SAMARES PAL, AN ECOEPIDEMIC SEASONALLY FORCED MODEL FOR THE COMBINED EFFECTS OF FEAR, ADDITIONAL FOODS AND SELECTIVE PREDATION, 2022, 30, 0218-3390, 285, 10.1142/S0218339022500103 | |
8. | Vikas Kumar, Bulti Pramanick, Impact of nanoparticles on the dynamics of a Crowley–Martin type phytoplankton–zooplankton interaction model, 2022, 8, 26667207, 100139, 10.1016/j.rico.2022.100139 | |
9. | Subarna Roy, Pankaj Kumar Tiwari, Himadri Nayak, Maia Martcheva, Effects of fear, refuge and hunting cooperation in a seasonally forced eco-epidemic model with selective predation, 2022, 137, 2190-5444, 10.1140/epjp/s13360-022-02751-2 | |
10. | SASANKA SHEKHAR MAITY, PANKAJ KUMAR TIWARI, ZHISHENG SHUAI, SAMARES PAL, ROLE OF SPACE IN AN ECO-EPIDEMIC PREDATOR-PREY SYSTEM WITH THE EFFECT OF FEAR AND SELECTIVE PREDATION, 2023, 31, 0218-3390, 883, 10.1142/S0218339023500316 | |
11. | Sasanka Shekhar Maity, Pankaj Kumar Tiwari, Samares Pal, Comprehensive Analysis of Deterministic and Stochastic Eco-Epidemic Models Incorporating Fear, Refuge, Supplementary Resources, and Selective Predation Effects, 2024, 191, 0167-8019, 10.1007/s10440-024-00654-1 | |
12. | Saswati Biswas, Arindam Mandal, Samares Pal, Catastrophic and noncatastrophic population crashes in a bitrophic system with dynamic additional food provision to cooperative predators, 2024, 109, 2470-0045, 10.1103/PhysRevE.109.024224 | |
13. | Sasanka Shekhar Maity, Pankaj Kumar Tiwari, Nanda Das, Samares Pal, 2023, Chapter 12, 978-3-031-33049-0, 197, 10.1007/978-3-031-33050-6_12 | |
14. | Arindam Mandal, Nazmul Sk, Saswati Biswas, Nutrient enrichment and phytoplankton toxicity influence a diversity of complex dynamics in a fear-induced plankton-fish model, 2024, 578, 00225193, 111698, 10.1016/j.jtbi.2023.111698 | |
15. | Tiancai Liao, Stochastic dynamics of a plankton model with zooplankton selectivity and nutritional value of phytoplankton, 2024, 70, 1598-5865, 251, 10.1007/s12190-023-01959-4 | |
16. | Arindam Mandal, Saswati Biswas, Pankaj Kumar Tiwari, Samares Pal, Seasonal refuge patterns of phytoplankton trigger irregular bloom events in a contaminated environment, 2024, 14, 2045-2322, 10.1038/s41598-024-66578-w | |
17. | Conghui Zhang, Jin Lu, Maoxing Liu, Hanzhi Zhang, Stable patterns with jump-discontinuity for a phytoplankton–zooplankton system with both Allee and fear effect, 2025, 472, 01672789, 134481, 10.1016/j.physd.2024.134481 | |
18. | Soumita Sen, Suddhyashil Sarkar, Samares Pal, Comparative studies on a zooplankton-fish model subjected to infection in zooplankton with varying rates of disease transmission, 2024, 27731863, 100194, 10.1016/j.fraope.2024.100194 | |
19. | Ravikant Singh, Archana Ojha, Pankaj Kumar Tiwari, Nilesh Kumar Thakur, Yun Kang, Impact of time delay in a plankton–fish system with nonlinear harvesting and external toxicity, 2025, 18, 1793-5245, 10.1142/S1793524523500961 | |
20. | Nada A. Almuallem, Miled El Hajji, How Can Viruses Affect the Growth of Zooplankton on Phytoplankton in a Chemostat?, 2025, 13, 2227-7390, 1192, 10.3390/math13071192 |
Parameters | Descriptions | Values | Units |
r | Intrinsic growth rate of susceptible phytoplankton | 1.2 | 1/day |
K | Carrying capacity of susceptible phytoplankton | 10 | cells/L |
β | Force of infection | 0.45 | 1/day |
K1 | Half saturation constant | 3 | cells/L |
α | Capture rate of susceptible phytoplankton | 0.18 | 1/day |
θ | Selectivity/preference of the zooplankton | 0.15 | — |
d | Half saturation constant | 5 | cells/L |
λ1 | Reproductive gain of zooplankton from susceptible phytoplankton | 0.75 | — |
λ2 | Reproductive gain of zooplankton from infected phytoplankton | 0.72 | — |
μ | Death rate of infected phytoplankton | 0.2 | 1/day |
ν | Mortality rate of zooplankton (natural and due to fish predation) | 0.012 | 1/day |
b | Virus replication factor | 40 | — |
δ | Decay rate of free-virus | 0.23 | 1/day |
A | Input rate of environmental toxins in the system | 6 | μg/L/day |
γ | Contact rate between environmental toxins with phytoplankton and zooplankton | 0.15 | L/cell/day |
γ1 | Inhibition of growth rate of susceptible phytoplankton due to environmental toxins | 0.1 | day L/μg |
γ2 | Death rate of infected phytoplankton due to environmental toxins | 0.02 | cell/μg |
γ3 | Death rate of zooplankton due to environmental toxins | 0.05 | cell/μg |
ρ | Depletion rate of environmental toxins | 0.25 | 1/day |
Models | Equilibria | Conditions for LAS |
(3.2) | ˜e0=(0,0)) | Always unstable |
˜e1=(K,0) | K(λ1α−ν)<dν | |
˜e∗=(˜S∗,˜Z∗) | K(λ1α−ν)>dν, K<d(λ1α+ν)λ1α−ν | |
(3.3) | ˆe0=(0,0,A/ρ) | Always unstable |
ˆe1=(K,0,A/(γK+ρ)) | Always stable | |
ˆe∗=(ˆS∗,ˆZ∗,ˆE∗) | Conditions in (3.5) | |
(3.6) | ¯e0=(0,0,0,0) | Always unstable |
¯e1=(K,0,0,0) | Conditions in (3.11) | |
¯e2=(¯S2,0,¯Z2,0) | Conditions in (3.12) | |
¯e3=(¯S3,¯I3,0,¯V3) | Conditions in (3.13) | |
¯e∗=(¯S∗,¯I∗,¯Z∗,¯V∗) | Conditions in (3.14) | |
(2.1) | e0=(0,0,0,0,A/ρ) | Always unstable |
e1=(K,0,0,0,A/(γK+ρ)) | Conditions in (3.17) | |
e2=(S2,0,Z2,0,E2) | Conditions in (3.18) | |
e3=(S3,I3,0,V3,E3) | Conditions in (3.19) | |
e∗=(S∗,I∗,Z∗,V∗,E∗) | Conditions in (3.20) |
Models | Equilibria | Eigenvalues of the system's Jacobian |
(3.2) | ˜e0=(0,0)) | 1.1999, −0.0120 |
˜e1=(10,0) | −1.1999, 0.0030 | |
˜e∗=(5.7142,183.6734) | −0.4043, −0.0071 | |
(3.3) | ˆe0=(0,0,24) | −0.2500, 1.1999, −0.1920 |
ˆe1=(10,0,3.4285)) | −1.7500, −0.7924, −0.0092 | |
ˆe∗1=(2.5599,116.9310,0.3301) | −0.0066±0.0911i, −18.1762 | |
ˆe∗2=(9.6963,7.1492,2.1607) | −2.7882, −0.8680, 0.0057 | |
(3.6) | ¯e0=(0,0,0,0) | 1.1999, −0.2300, −0.2000, −0.0120 |
¯e1=(10,0,0,0) | −1.1999, 2.5825, −4.5125, 0.0165 | |
¯e2=(1.9512,0,117.7870,0) | 0.0184±0.0894i, 1.0989, −1.9665 | |
¯e3=(6.2735,14.0269,0,475.6954) | −0.7533, −0.2313, −0.1981, 0.0145 | |
¯e∗=(1.6059,2.4375,70.1090,81.7521) | −0.3601, −0.1733, −0.0194±0.0687i | |
(2.1) | e0=(0,0,0,0,24) | −0.2500, 1.1999, −0.1920, −0.2300, −0.2719 |
e1=(10,0,0,0,3.4285) | −1.7500, −0.7924, −0.0377, −4.5166, 2.5763 | |
e2=(0.8666,0,35.2363,0,1.0590) | 0.0886, −0.0583, −5.6732, −1.3824, 0.6571 | |
e3=(5.6319,12.2052,0,413.5891,2.0508) | −2.9498, −0.6434, −0.2289, −0.1814, 0.0273 | |
e∗=(0.8860,1.2285,21.9939,41.1149,1.5518) | −3.8772, −0.3882, −0.1071±0.0316i, −0.0290 |
Parameters | Baseline values | Minimum values | Maximum values |
r | 1.2 | 0.9000 | 1.5000 |
K | 10 | 7.5000 | 12.5000 |
β | 0.45 | 0.3375 | 0.5626 |
K1 | 3 | 2.2500 | 3.7500 |
α | 0.18 | 0.1350 | 0.2250 |
θ | 0.15 | 0.1125 | 0.1875 |
d | 5 | 3.7500 | 6.2500 |
λ1 | 0.75 | 0.5625 | 0.9375 |
λ2 | 0.72 | 0.5400 | 0.9000 |
μ | 0.2 | 0.1500 | 0.2500 |
ν | 0.012 | 0.0090 | 0.0150 |
b | 40 | 30.0000 | 50.0000 |
δ | 0.23 | 0.1725 | 0.2875 |
A | 6 | 4.5000 | 7.5000 |
γ | 0.15 | 0.1125 | 0.1875 |
γ1 | 0.1 | 0.0750 | 0.1250 |
γ2 | 0.02 | 0.0150 | 0.0250 |
γ3 | 0.05 | 0.0375 | 0.0625 |
ρ | 0.25 | 0.1875 | 0.3125 |
State variables | Comparison |
Susceptible phytoplankton (S) | ˆS∗>˜S∗>S∗>¯S∗ |
Infected phytoplankton (I) | I∗>¯I∗>ˆI∗=˜I∗=0 |
Zooplankton (Z) | ˜Z∗>ˆZ∗>¯Z∗>Z∗ |
Free-viruses (V) | V∗>¯V∗>ˆV∗=˜V∗=0 |
Environmental toxins (E) | E∗>˜E∗>¯E∗=˜E∗=0 |
Parameters | Descriptions | Values | Units |
r | Intrinsic growth rate of susceptible phytoplankton | 1.2 | 1/day |
K | Carrying capacity of susceptible phytoplankton | 10 | cells/L |
β | Force of infection | 0.45 | 1/day |
K1 | Half saturation constant | 3 | cells/L |
α | Capture rate of susceptible phytoplankton | 0.18 | 1/day |
θ | Selectivity/preference of the zooplankton | 0.15 | — |
d | Half saturation constant | 5 | cells/L |
λ1 | Reproductive gain of zooplankton from susceptible phytoplankton | 0.75 | — |
λ2 | Reproductive gain of zooplankton from infected phytoplankton | 0.72 | — |
μ | Death rate of infected phytoplankton | 0.2 | 1/day |
ν | Mortality rate of zooplankton (natural and due to fish predation) | 0.012 | 1/day |
b | Virus replication factor | 40 | — |
δ | Decay rate of free-virus | 0.23 | 1/day |
A | Input rate of environmental toxins in the system | 6 | μg/L/day |
γ | Contact rate between environmental toxins with phytoplankton and zooplankton | 0.15 | L/cell/day |
γ1 | Inhibition of growth rate of susceptible phytoplankton due to environmental toxins | 0.1 | day L/μg |
γ2 | Death rate of infected phytoplankton due to environmental toxins | 0.02 | cell/μg |
γ3 | Death rate of zooplankton due to environmental toxins | 0.05 | cell/μg |
ρ | Depletion rate of environmental toxins | 0.25 | 1/day |
Models | Equilibria | Conditions for LAS |
(3.2) | ˜e0=(0,0)) | Always unstable |
˜e1=(K,0) | K(λ1α−ν)<dν | |
˜e∗=(˜S∗,˜Z∗) | K(λ1α−ν)>dν, K<d(λ1α+ν)λ1α−ν | |
(3.3) | ˆe0=(0,0,A/ρ) | Always unstable |
ˆe1=(K,0,A/(γK+ρ)) | Always stable | |
ˆe∗=(ˆS∗,ˆZ∗,ˆE∗) | Conditions in (3.5) | |
(3.6) | ¯e0=(0,0,0,0) | Always unstable |
¯e1=(K,0,0,0) | Conditions in (3.11) | |
¯e2=(¯S2,0,¯Z2,0) | Conditions in (3.12) | |
¯e3=(¯S3,¯I3,0,¯V3) | Conditions in (3.13) | |
¯e∗=(¯S∗,¯I∗,¯Z∗,¯V∗) | Conditions in (3.14) | |
(2.1) | e0=(0,0,0,0,A/ρ) | Always unstable |
e1=(K,0,0,0,A/(γK+ρ)) | Conditions in (3.17) | |
e2=(S2,0,Z2,0,E2) | Conditions in (3.18) | |
e3=(S3,I3,0,V3,E3) | Conditions in (3.19) | |
e∗=(S∗,I∗,Z∗,V∗,E∗) | Conditions in (3.20) |
Models | Equilibria | Eigenvalues of the system's Jacobian |
(3.2) | ˜e0=(0,0)) | 1.1999, −0.0120 |
˜e1=(10,0) | −1.1999, 0.0030 | |
˜e∗=(5.7142,183.6734) | −0.4043, −0.0071 | |
(3.3) | ˆe0=(0,0,24) | −0.2500, 1.1999, −0.1920 |
ˆe1=(10,0,3.4285)) | −1.7500, −0.7924, −0.0092 | |
ˆe∗1=(2.5599,116.9310,0.3301) | −0.0066±0.0911i, −18.1762 | |
ˆe∗2=(9.6963,7.1492,2.1607) | −2.7882, −0.8680, 0.0057 | |
(3.6) | ¯e0=(0,0,0,0) | 1.1999, −0.2300, −0.2000, −0.0120 |
¯e1=(10,0,0,0) | −1.1999, 2.5825, −4.5125, 0.0165 | |
¯e2=(1.9512,0,117.7870,0) | 0.0184±0.0894i, 1.0989, −1.9665 | |
¯e3=(6.2735,14.0269,0,475.6954) | −0.7533, −0.2313, −0.1981, 0.0145 | |
¯e∗=(1.6059,2.4375,70.1090,81.7521) | −0.3601, −0.1733, −0.0194±0.0687i | |
(2.1) | e0=(0,0,0,0,24) | −0.2500, 1.1999, −0.1920, −0.2300, −0.2719 |
e1=(10,0,0,0,3.4285) | −1.7500, −0.7924, −0.0377, −4.5166, 2.5763 | |
e2=(0.8666,0,35.2363,0,1.0590) | 0.0886, −0.0583, −5.6732, −1.3824, 0.6571 | |
e3=(5.6319,12.2052,0,413.5891,2.0508) | −2.9498, −0.6434, −0.2289, −0.1814, 0.0273 | |
e∗=(0.8860,1.2285,21.9939,41.1149,1.5518) | −3.8772, −0.3882, −0.1071±0.0316i, −0.0290 |
Parameters | Baseline values | Minimum values | Maximum values |
r | 1.2 | 0.9000 | 1.5000 |
K | 10 | 7.5000 | 12.5000 |
β | 0.45 | 0.3375 | 0.5626 |
K1 | 3 | 2.2500 | 3.7500 |
α | 0.18 | 0.1350 | 0.2250 |
θ | 0.15 | 0.1125 | 0.1875 |
d | 5 | 3.7500 | 6.2500 |
λ1 | 0.75 | 0.5625 | 0.9375 |
λ2 | 0.72 | 0.5400 | 0.9000 |
μ | 0.2 | 0.1500 | 0.2500 |
ν | 0.012 | 0.0090 | 0.0150 |
b | 40 | 30.0000 | 50.0000 |
δ | 0.23 | 0.1725 | 0.2875 |
A | 6 | 4.5000 | 7.5000 |
γ | 0.15 | 0.1125 | 0.1875 |
γ1 | 0.1 | 0.0750 | 0.1250 |
γ2 | 0.02 | 0.0150 | 0.0250 |
γ3 | 0.05 | 0.0375 | 0.0625 |
ρ | 0.25 | 0.1875 | 0.3125 |
State variables | Comparison |
Susceptible phytoplankton (S) | ˆS∗>˜S∗>S∗>¯S∗ |
Infected phytoplankton (I) | I∗>¯I∗>ˆI∗=˜I∗=0 |
Zooplankton (Z) | ˜Z∗>ˆZ∗>¯Z∗>Z∗ |
Free-viruses (V) | V∗>¯V∗>ˆV∗=˜V∗=0 |
Environmental toxins (E) | E∗>˜E∗>¯E∗=˜E∗=0 |