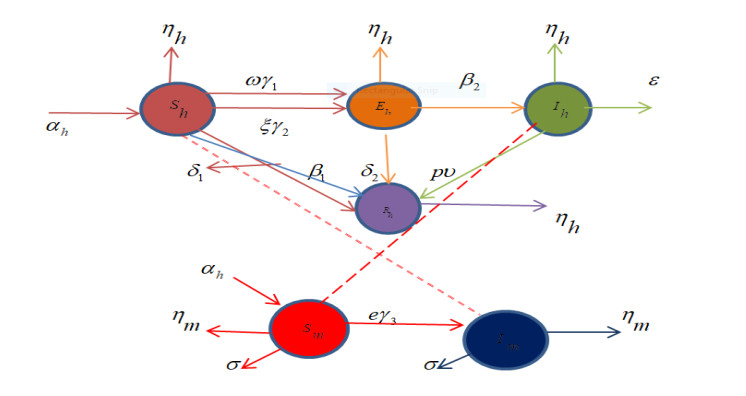
The field of neuroscience has been significantly impacted by the emergence of artificial intelligence (AI), particularly language models like ChatGPT. ChatGPT, developed by OpenAI, is a powerful conversational AI tool with the ability to communicate in multiple languages and process vast amounts of data. The commentary explores the significant impact of ChatGPT on the field of neuroscience, emphasizing its potential contributions, challenges, and ethical considerations. ChatGPT has shown promise in various aspects of neuroscience research, including hypothesis generation, data analysis, literature review, collaboration, and education. However, it is not without limitations, particularly in terms of accuracy, potential bias, and ethical concerns. The commentary highlights the potential applications of ChatGPT in the context of child and adolescent mental health, where it could revolutionize assessment and treatment processes. By analyzing text from young patients, ChatGPT can identify patterns related to mental health issues, enhancing diagnostic accuracy and treatment planning. It can also improve communication between patients and healthcare professionals, offering real-time insights and educational resources. While ChatGPT presents exciting opportunities, the commentary acknowledges the need for careful oversight and control to address privacy concerns, biases, and potential misuse. Ethical considerations surrounding the model's impact on emotions, behavior, and biases require ongoing scrutiny and safeguards. In conclusion, ChatGPT offers transformative potential in neuroscience and mental health, but it must be harnessed responsibly, with a focus on ethical considerations and scientific rigor to ensure its positive impact on research and clinical practice.
Citation: Arosh S. Perera Molligoda Arachchige, Kamel Chebaro, Alice J. M. Jelmoni. Advances in large language models: ChatGPT expands the horizons of neuroscience[J]. STEM Education, 2023, 3(4): 263-272. doi: 10.3934/steme.2023016
[1] | Lamya Almaghamsi, Aeshah Alghamdi, Abdeljabbar Ghanmi . Existence of solution for a Langevin equation involving the ψ-Hilfer fractional derivative: A variational approach. AIMS Mathematics, 2025, 10(1): 534-550. doi: 10.3934/math.2025024 |
[2] | Arjumand Seemab, Mujeeb ur Rehman, Jehad Alzabut, Yassine Adjabi, Mohammed S. Abdo . Langevin equation with nonlocal boundary conditions involving a ψ-Caputo fractional operators of different orders. AIMS Mathematics, 2021, 6(7): 6749-6780. doi: 10.3934/math.2021397 |
[3] | Sotiris K. Ntouyas, Bashir Ahmad, Jessada Tariboon . Nonlocal integro-multistrip-multipoint boundary value problems for ¯ψ∗-Hilfer proportional fractional differential equations and inclusions. AIMS Mathematics, 2023, 8(6): 14086-14110. doi: 10.3934/math.2023720 |
[4] | Karim Guida, Lahcen Ibnelazyz, Khalid Hilal, Said Melliani . Existence and uniqueness results for sequential ψ-Hilfer fractional pantograph differential equations with mixed nonlocal boundary conditions. AIMS Mathematics, 2021, 6(8): 8239-8255. doi: 10.3934/math.2021477 |
[5] | Sunisa Theswan, Sotiris K. Ntouyas, Jessada Tariboon . Coupled systems of ψ-Hilfer generalized proportional fractional nonlocal mixed boundary value problems. AIMS Mathematics, 2023, 8(9): 22009-22036. doi: 10.3934/math.20231122 |
[6] | Thabet Abdeljawad, Pshtiwan Othman Mohammed, Hari Mohan Srivastava, Eman Al-Sarairah, Artion Kashuri, Kamsing Nonlaopon . Some novel existence and uniqueness results for the Hilfer fractional integro-differential equations with non-instantaneous impulsive multi-point boundary conditions and their application. AIMS Mathematics, 2023, 8(2): 3469-3483. doi: 10.3934/math.2023177 |
[7] | Zohreh Heydarpour, Maryam Naderi Parizi, Rahimeh Ghorbnian, Mehran Ghaderi, Shahram Rezapour, Amir Mosavi . A study on a special case of the Sturm-Liouville equation using the Mittag-Leffler function and a new type of contraction. AIMS Mathematics, 2022, 7(10): 18253-18279. doi: 10.3934/math.20221004 |
[8] | Weerawat Sudsutad, Chatthai Thaiprayoon, Sotiris K. Ntouyas . Existence and stability results for ψ-Hilfer fractional integro-differential equation with mixed nonlocal boundary conditions. AIMS Mathematics, 2021, 6(4): 4119-4141. doi: 10.3934/math.2021244 |
[9] | Zheng Kou, Saeed Kosari . On a generalization of fractional Langevin equation with boundary conditions. AIMS Mathematics, 2022, 7(1): 1333-1345. doi: 10.3934/math.2022079 |
[10] | Muath Awadalla, Manigandan Murugesan, Subramanian Muthaiah, Bundit Unyong, Ria H Egami . Existence results for a system of sequential differential equations with varying fractional orders via Hilfer-Hadamard sense. AIMS Mathematics, 2024, 9(4): 9926-9950. doi: 10.3934/math.2024486 |
The field of neuroscience has been significantly impacted by the emergence of artificial intelligence (AI), particularly language models like ChatGPT. ChatGPT, developed by OpenAI, is a powerful conversational AI tool with the ability to communicate in multiple languages and process vast amounts of data. The commentary explores the significant impact of ChatGPT on the field of neuroscience, emphasizing its potential contributions, challenges, and ethical considerations. ChatGPT has shown promise in various aspects of neuroscience research, including hypothesis generation, data analysis, literature review, collaboration, and education. However, it is not without limitations, particularly in terms of accuracy, potential bias, and ethical concerns. The commentary highlights the potential applications of ChatGPT in the context of child and adolescent mental health, where it could revolutionize assessment and treatment processes. By analyzing text from young patients, ChatGPT can identify patterns related to mental health issues, enhancing diagnostic accuracy and treatment planning. It can also improve communication between patients and healthcare professionals, offering real-time insights and educational resources. While ChatGPT presents exciting opportunities, the commentary acknowledges the need for careful oversight and control to address privacy concerns, biases, and potential misuse. Ethical considerations surrounding the model's impact on emotions, behavior, and biases require ongoing scrutiny and safeguards. In conclusion, ChatGPT offers transformative potential in neuroscience and mental health, but it must be harnessed responsibly, with a focus on ethical considerations and scientific rigor to ensure its positive impact on research and clinical practice.
There are many life-threatening diseases, out of which Malaria is one of them that is produced through the parasite known as Plasmodium that transmits to humans with the infected anopheles mosquito bite [1,2,3]. People with malaria are infected by the Protozoan parasite, which grows within the cells of red blood and spreads the illness. There are four Plasmodia species that can become the reason of infection, known as oval, falciparum, malaria, and vivax, while the two other most widespread Plasmodium species are falciparum and vivax [4]. Malaria affects lots of people each year, mainly in South Asia, Africa, and South America. It becomes the major reason of death and illness in various developing regions, specifically young children, and pregnant women. The main symptoms of malaria are fever, muscle pain, and headache. Malaria can progress promptly, possibly severe anemia, lung problems, and even cause death. There are various effective tactics that proposed the World Health Organization (WHO) to treat and prevent this dangerous disease. Some of them are indoor spraying, pesticide-treated bed nets, and some antimalarial medications. The vector control systems can be applied to lessen the poisoned mosquito population. In general, malaria is a substantial global health task, which needs a comprehensive approach for its cure by using the strategies of vector control and early identification for its treatment.
Malaria is a dynamic and complicated disease, which is considered difficult to present its model mathematically. The malaria parasite's life cycle is complex due to numerous phases of development both in mosquito vector and human host. These points are prompted by numerous features, e.g., mosquito actions, host immunity, and environmental environments that is used to make difficult the disease dynamics to predict and understand. The intricacy of the virus's spread dynamics is one of the main problems with malaria modelling. Malaria spread is influenced by numerous interrelated influences, e.g., the mosquito vector density, the host immunity degree, and the accessibility of efficient mediations. The relations between these aspects indicate the nonlinearity and hard to capture using the mathematical systems [5]. However, the mathematical malaria disease model based on the pesticides and medication (MDMPM) is presented, which is categorized in four human (susceptible, exposed, infected, recovered) and two vector (susceptible, infected) classes. The pathogen infects the population of host then the individuals are categorized into groups based on the parasites number and virus types. Kermack-McKendrick [6] proposed the mathematical SEIRSI model based on the population of human/vector groups. The nonlinear dynamics of the SEIRSI model is presented as [7]:
S′h(x)=αh+β1Rh(x)−γ1ψIh(x)Sh(x)−γ2ξIm(x)Sh(x)−(δ1+ηh)Sh(x),E′h(x)=γ2ξIm(x)Sh(x)+γ1ψIh(x)Sh(x)−(β2+δ2+ηh)Eh(x),I′h(x)=β2Eh(x)−(ϵ+pυ+ηh)Ih(x),R′h(x)=δ1Sh(x)+υpIh(x)+δ2Eh(x)−(β1+ηh)Rh(x),S′m(x)=αm−(σ+ηm)Sm(x)−γ3eSm(x)Ih(x),I′m(x)=γ3eIh(x)Sm(x)−(σ+ηm)Im(x). | (1) |
The compartmental illustration of the SEIRSI model based on the MDMPM is shown in Figure 1, while the description of each parameter is given in Table 1.
Parameters | Details |
β1 | Susceptible form of recovered individuals |
αm | Newborn mosquitoes transfer rate into Sm |
αh | Newborns ratio to enter in Sh |
γ1 | Transfer ratio of illness from one affected to susceptible people |
ψ | Transfusions rate of average blood with stable time in Ih and Sh |
β2 | Exposed to infected infection rate in people |
ξ | Infected mosquito susceptible bites using fixed time |
γ3 | Rate of Mosquitos infection |
γ2 | Disease transfer through infected (mosquito) to susceptible (individual) |
ηh | People natural rate of death |
δ2 | Exposed recovered population |
δ1 | Susceptible recovered population |
p | Rate of humans heal |
ε | Infected human death due to disease |
ηm | Mosquitos' natural death |
υ | Anti-malarial prescriptions |
e | Bites Sm using the infected humans |
σ | Expires sections using spraying for Sm and Im |
x | Time |
There are various deterministic and conventional numerical schemes that have been presented to solve the biological models, including the wavelet Bernstein scheme that has been presented to solve the epidemic SIR fractional kind of infectious disease model [8], Laplace method is applied for the fractional epidemic system of born vector disease [9], q-homotopy transform technique is proposed to solve the fractional Schistosomiasis disease model [10,11], a theoretic investigation is presented based on the impacts of vector-virus transmission mechanism [12], a collocation technique with shifted Chebyshev polynomials is applied to solve the fractional order diseased model of Rubella ailment [13], an analytical computational approach is provided for the inflammatory atherosclerosis disease system [14], the differential stomach and coronavirus models have been discussed numerically by applying the Adam method [15,16] and the design of a numerical method is presented for the maturing communication in population and epidemic models [17]. Some more deterministic procedures that have been applied to solve different dynamical models are presented in [18,19,20,21,22].
All mentioned schemes have their individual strength, and demerits, however the stochastic solvers have never been tested before for the nonlinear dynamics of the MDMPM. The current study is related to the numerical solutions of the MDMPM through the stochastic procedures using the scale conjugate gradient neural networks (SCJGNNs). In recent years, the stochastic computing paradigms have been proposed in various applications, e.g., eye surgery differential systems [23,24], food chain systems [25,26], smoking dynamical systems [27], models arising in biological applications, [28,29], thermal explosion singular systems [30,31], and many more [32,33,34,35,36,37,38,39,40,41,42,43]. Based on these noteworthy applications of neuro-computing paradigm, the authors are motivated to present the numerical solutions of the malaria disease model of pesticides and medication (MDMPM) with stochastic framework of scale conjugate gradient neural networks (SCJGNNs). The purpose of this work is to provide a stochastic framework based on the SCJGNNs for solving the mathematical model representing MDMPM dynamics.
The contributions, insights and innovative aspects of the current scheme is portrayed in terms of salient features as follows:
• A novel design of the stochastic framework via SCJGNNs is introduced/presented to get the numerical results/outcomes of the nonlinear dynamics of governing mathematical model of MDMPM.
• The capability and knacks of Runge-Kutta solver is exploited/developed to formulate the target datasets for different scenario of MDMPM system that are used for the testing, and training procedure of the networks via SCJGNNs.
• The exactness of the stochastic SCJGNNs is attained/verified/validated through the overlapping of the results with standard counterparts having very small magnitude of absolute error (AE).
• The reliable matching of the numerical results, a strong arrangement using the benchmark outputs along with consistent statistical performance indices further prove the importance/significance/role of the SCJGNNs to solve the variant of MDMPM models.
The remaining paper is presented as: Proposed SCJGNNs design is shown in Section 2, Sect 3 presents the numerical results, and the conclusions are discussed in the final section.
The current section is used to design the computing SCJGNNs structure to solve the MDMPM in two steps, including the detail of designed SCJGNNs performances along with its execution. The designed SCJGNNs is presented in Figure 2. In the 1st phase of Figure 2, the nonlinear mathematical system is illustrated, the stochastic methodology using the SCJGNNs is provided in the 2nd phase of Figure 2, while some results are presented in the last phase of Figure 2. The performances using the multi-layers network based on the structure of single neuron is illustrated in Figure 3.
The computing SCJGNNs approach for solving the MDMPM is executed using the command 'nftool' in software 'MATLAB', n-folded cross authentication, layers (output/hidden/input) structure, epochs are taken maximum 1000, fifteen hidden neurons, transfer function log-sigmoid, stopping standards, SCJG algorithm, tolerances, and step size. The label statics and input/target training are achieved using the basic outputs by taking the statics of validation (12%), testing (10%), and training (78%) for the MDMPM.
The designed computing SCJGNNs for the solutions of the MDMPM is performed by using 15 neurons, which have been validated through the best values of underfitting and overfitting based on the authorization and training at epochs 100, 86 and 69. For small neurons, the premature convergence (under fitting) problem is faced, while similar precision by using higher intricacy (overfitting issue) is noticed by taking higher neurons. The trials have been taken for the validation (12%), testing (10%), and training (78%) to solve the MDMPM. By taking a smaller data training, like 40% to 60%, the MSE values for testing 30% to 40% increase and generally poor presentations have been observed. On the other hand, if the training statics is almost designated > 90% and 5% for both testing and certification, the procedure's correctness improves marginally by choosing the label static, which presents the bias and targets input, but stochastic procedure's strength is not severely established for unbiased statics exclusive based targets information. The proposed values are performed on the input [0, 1] by applying the stochastic SCJGNNs procedure for the numerical performances of the MDMPM. The neuron structure using the input, output and hidden layers is shown in Figure 4.
The numerical solution for three different cases of the MDMPM by applying the computing procedures based on the SCJGNNs is presented in this section.
Case 1: Consider the values ψ=0.038, αh=0.027, γ1=0.020, ξ=0.13, β1=1730, γ2=0.010, δ1=0.001, ηh=0.0004, δ2=0.001, p=0.611, β2=0.001, ε=0.05, υ=0.01, e=0.022, αm=0.13, γ3=0.072, Sh(0)=0.01, Eh(0)=0.02, Ih(0)=0.03, Rh(0)=0.04, Sm(0)=0.05, and Im(0)=0.06 in system (1), which is shown as:
{S′h(x)=0.027+0.0014Rh(x)−(0.00076Ih(x)+0.0014+0.0013Im(x))Sh(x),(Sh)0=0.01,E′h(x)=(0.00076Ih(x)+0.0013Im(x))Sh(x)−0.0024Eh(x),(Eh)0=0.02,I′h(x)=−0.00752Ih(x)+0.001Eh(x),(Ih)0=0.03,R′h(x)=0.00611Ih(x)−0.00177Rh(x)+0.001Eh(x)+0.001Sh(x),(Rh)0=0.04,S′m(x)=0.13−0.05Sm(x)−0.001584Ih(x)Sm(x),(Sm)0=0.05,I′m(x)=0.001584Sm(x)Ih(x)−0.05Im(x),(Im)0=0.06. | (2) |
Case 2: Consider the parameter values ψ=0.038, αh=0.027, γ1=0.020, ξ=0.13, β1=1730, γ2=0.010, δ1=0.001, ηh=0.0004, δ2=0.001, p=0.611, β2=0.001, ε=0.05, υ=0.01, e=0.022, αm=0.13, γ3=0.072, Sh(0)=0.02, Eh(0)=0.03, Ih(0)=0.04, Rh(0)=0.05, Sm(0)=0.06, and Im(0)=0.07 in system (1) that is given as:
{S′h(x)=0.027+0.0014Rh(x)−(0.00076Ih(x)+0.0014+0.0013Im(x))Sh(x),(Sh)0=0.02,E′h(x)=(0.00076Ih(x)+0.0013Im(x))Sh(x)−0.0024Eh(x),(Eh)0=0.03,I′h(x)=−0.00752Ih(x)+0.001Eh(x),(Ih)0=0.04,R′h(x)=0.00611Ih(x)−0.00177Rh(x)+0.001Eh(x)+0.001Sh(x),(Rh)0=0.05,S′m(x)=0.13−0.05Sm(x)−0.001584Ih(x)Sm(x),(Sm)0=0.06,I′m(x)=0.001584Sm(x)Ih(x)−0.05Im(x),(Im)0=0.07. | (3) |
Case 3: Consider ψ=0.038, αh=0.027, γ1=0.020, ξ=0.13, β1=1730, γ2=0.010, δ1=0.001, ηh=0.0004, δ2=0.001, p=0.611, β2=0.001, ε=0.05, υ=0.01, e=0.022, αm=0.13, γ3=0.072, Sh(0)=0.03, Eh(0)=0.04, Ih(0)=0.05, Rh(0)=0.06, Sm(0)=0.07, and Im(0)=0.08 in system (1), given as:
{S′h(x)=0.027+0.0014Rh(x)−(0.00076Ih(x)+0.0014+0.0013Im(x))Sh(x),(Sh)0=0.03,E′h(x)=(0.00076Ih(x)+0.0013Im(x))Sh(x)−0.0024Eh(x),(Eh)0=0.04,I′h(x)=−0.00752Ih(x)+0.001Eh(x),(Ih)0=0.05,R′h(x)=0.00611Ih(x)−0.00177Rh(x)+0.001Eh(x)+0.001Sh(x),(Rh)0=0.06,S′m(x)=0.13−0.05Sm(x)−0.001584Ih(x)Sm(x),(Sm)0=0.07,I′m(x)=0.001584Sm(x)Ih(x)−0.05Im(x),(Im)0=0.08. | (4) |
The plots of the estimated results based on the numerical SCJGNNs procedure for solving the MDMPM are shown in Figures 5 to 9. Figure 5 presents the best validation measures and state transitions for the nonlinear dynamics of the MDMPM through the stochastic computing SCJGNNs scheme. The values of the mean square error (MSE) and the state of transition values are depicted in Figure 5. The computed performances of MSE using the best curves plots, certification, and training are provided in Figure 5(a-c) and the SoT measures are portrayed in Figure 5(d-f). The best solution performances of the MDMPM are shown at epochs 100, 86 and 69, which are found around 1.69774 ×10−07, 1.4324 × 10−07 and 3.5876 × 10−07. It indicates that convergence through the MSE is observed based on dataset of training, testing and certification. It is also observed that by updating the epochs, the authentications, training, and testing graphs lead to steady-state position up to 10-07. The gradient curves by applying the process of SCJGNNs to solve the MDMPM are calculated as 1.4161×10-06, 8.1804 × 10−07 and 2.9486 × 10−06. These graphic curves indicate the precision, exactness, and convergence by using the SCJGNNs procedure for the MDMPM. An error gradient presents the direction and magnitude that is calculated by the designed neural network for updating the weights in the proper amount/direction. In neural network fitting, the process of backpropagation is used to compute the loss function gradient. Mu is the used for the network's training for the momentum parameter, which includes the updated weight expressions to avoid the local minimum issues. It is observed that the network can stuck sometimes to the local minimum, which does not perform the convergence. In addition, Mu shows the control parameter based on that scheme that is used in the neural network's training.
Figure 6(a to c) presents the curves of fitting for the nonlinear MDMPM, which certifies the comparison of the results. Figure 6(d-f) signifies the error histogram (EH) values for the MDMPM by applying the computing SCJGNNs. The construction of EH is used to test the errors of the targeted and predicted values after the designed artificial neural network training. These errors present that how predicted measures contrast from the targeted values. These values based on the EH are obtained as 1.95 × 10−05, 8.64 × 10−05 and −5.90 × 10−06 for respective dynamics of the MDMPM. Figures 7 to 9 present the correlation values for the MDMPM using the SCJGNNs. These measures based on the determination coefficient R2 are found as 1 for each case of the MDMPM. The graphs of substantiation, testing, and training verify the correctness of the MDMPM using the numerical stochastic SCJGNNs procedure. The values of MSE convergence using the train, epochs, certification, backpropagation, test, and intricacy are tabulated in Table 2. The complexity measures using the SCJGNNs for the MDMPM are performed to authenticate the intricacy based on the network's training, i.e., epoch execution.
Case | MSE for instances of sampling | Gradient | Performance | Epoch | Time | ||
Test | validation | Train | |||||
I | 2.745×10−07 | 1.697 ×10−07 | 2.366×10−07 | 1.42×10−06 | 2.26×10−07 | 100 | 2 Sec |
II | 1.795×10−07 | 1.432×10−07 | 1.920×10−07 | 8.18×10−07 | 1.91×10−07 | 86 | 2 Sec |
III | 5.112×10−07 | 3.038×10−07 | 3.678×10−07 | 2.94×10−06 | 2.09×10−07 | 69 | 2 Sec |
Figures 10 and 11 present the comparison graphs and AE using the obtained and source statics for the MDMPM. It is demonstrated that the source and calculated outcomes of the MDMPM precisely coincided. Figure 10 represents the AE graphs for each category of the MDMPM. For the first class, the AE lies as 10−03 to 10−05 for case I and III and 10−02 to 10−04 for case II. These values for the 2nd category of the MDMPM are found around 10−05 to 10−06, 10−04 to 10−06 and 10−03 to 10−05 for cases I, II and III. The AE measures for the 3rd dynamics of the MDMPM lie as 10−04 to 10−07, 10−03 to 10−05 and 10−03 to 10−06 for respective cases. These values for the 4th class of the MDMPM are calculated as 10−03 to 10−05 for case I and for case II and III, these AE are found as 10−03 to 10−04. The AE values for the 5th and 6th dynamics are found as 10−03 to 10−05 for each case of the model. These reduceable AE measures provides the accuracy of the scheme for the MDMPM.
The stochastic numerical performances of the malaria disease using the pesticides and medication have been presented in this study. The populations-based host and vector are divided in the mathematical classification of the malaria using the pesticides and medication. Some conclusions of this work are reported as:
• The stochastic scale conjugate gradient neural network framework has been designed successfully for the numerical malaria disease model.
• The neural network procedure is presented by using fifteen neurons along with the log-sigmoid transfer function in the hidden layers to solve the model.
• The supervised neural networks process using the data as for validation 12%, testing 10%, and training 78% is presented for the numerical solutions of the system.
• The accuracy of the proposed stochastic scheme is examined through the overlapping of the achieved obtained and Runge-Kutta source solutions.
• The negligible performances of AE indicate the exactness of the computing stochastic approach.
• The consistency and trustworthiness of the designed procedure are assessed through the correlation, histogram curves, regression, and functional fitness process to solve the MDMPM.
• The perks/advantages of the proposed stochastic SCJGNNs procedure includes simplicity of the concept and understanding, straightforwardness in implementations and execution, provision of outcome on continuous domain, expandability and applicability.
The limitation/disadvantage of the stochastic SCJGNNs paradigm is the issue of trapping in local minima for scale conjugate gradient methodology while training of the weights of the networks, however, the said limitation is rectified by exploited heuristic global search methodologies in future by the interested readers. Additionally, in future, the designed SCJGNNs procedure can be explored/implemented for different applications of the nonlinear mathematical models arising in the field of computational fluid mechanics.
The authors declare that they have no conflict of interest
Manal Alqhtani: Conceptualization, methodology, validation, Writing-original draft preparation, writing-review; J.F. Gómez-Aguilar: Conceptualization, methodology, validation, investigation, Writing-original draft preparation, writing-review; Khaled M. Saad: Conceptualization, methodology, validation, writing-review and editing; Zulqurnain Sabir: Validation, investigation, Writing-original draft preparation, writing-review; Eduardo Pérez-Careta: Validation, formal analysis, investigation. All authors have read and agreed to the published version of the manuscript.
The authors are thankful to the Deanship of Scientific Research at Najran University for funding this work under the General Research Funding program grant code (NU/RG/SERC/12/22).
The authors declare they have not used Artificial Intelligence (AI) tools in the creation of this article.
[1] | The Evolution of Neuroscience, 2019. Retrieved from: https://www.kentscientific.com/blog/the-evolution-of-neuroscience/ |
[2] |
Zukotynski, K., Gaudet, V., Uribe, C.F., Mathotaarachchi, S., Smith, K.C., Rosa-Neto, P., et al., Machine learning in nuclear medicine: part 2-neural networks and clinical aspects. Journal of Nuclear Medicine, 2021, 62(1): 22–29. DOI: 10.2967/jnumed.119.231837 doi: 10.2967/jnumed.119.231837
![]() |
[3] | Radford, A., Wu, J., Child, R., Luan, D., Amodei, D. and Sutskever, I., Language models are unsupervised multitask learners. OpenAI blog, 2019, 1(8): 9. |
[4] |
Cifarelli, C.P. and Sheehan, J.P., Large language model artificial intelligence: the current state and future of ChatGPT in neuro-oncology publishing. Journal of Neuro-Oncology, 2023,163: 473–474. DOI: 10.1007/s11060-023-04336-0 doi: 10.1007/s11060-023-04336-0
![]() |
[5] |
Chen, T.C., Kaminski, E., Koduri, L., Singer, A., Singer, J., Couldwell, M., et al., Chat GPT as a Neuro-Score Calculator: Analysis of a Large Language Model's Performance on Various Neurological Exam Grading Scales. World neurosurgery, 2023,179: e342–e347. DOI: 10.1016/j.wneu.2023.08.088 doi: 10.1016/j.wneu.2023.08.088
![]() |
[6] |
Madadi, Y., Delsoz, M., Lao, P.A., Fong, J.W., Hollingsworth, T.J., Kahook, M.Y., et al., ChatGPT Assisting Diagnosis of Neuro-ophthalmology Diseases Based on Case Reports. medRxiv, 2023. DOI: 10.1101/2023.09.13.23295508 doi: 10.1101/2023.09.13.23295508
![]() |
[7] |
Sohail, S.S., Farhat, F., Himeur, Y., Nadeem, M., Madsen, D.Ø., Singh, Y., et al., The Future of GPT: A Taxonomy of Existing ChatGPT Research, Current Challenges, and Possible Future Directions. Current Challenges, and Possible Future Directions, 2023. DOI: 10.2139/ssrn.4413921 doi: 10.2139/ssrn.4413921
![]() |
[8] | ChatGPT. Retrieved from: https://openai.com/chatgpt |
[9] |
Perera Molligoda Arachchige, A.S., ChatGPT in nuclear medicine and radiology: reply to Laudicella et al.. Clin Transl Imaging, 2023, 11: 505–506. DOI: 10.1007/s40336-023-00579-z doi: 10.1007/s40336-023-00579-z
![]() |
[10] |
Arachchige, A.S.P.M., Early applications of ChatGPT in medical practice, education and research. Clinical medicine, 2023, 23(4): 429–430. DOI: 10.7861/clinmed.Let.23.4.2 doi: 10.7861/clinmed.Let.23.4.2
![]() |
[11] |
Perera Molligoda Arachchige, A.S. and Stomeo, N., Exploring the Opportunities and Challenges of ChatGPT in Academic Writing: Reply to Bom et al.. Nuclear medicine and molecular imaging, 2023, 57(5): 213–214. DOI: 10.1007/s13139-023-00816-3 doi: 10.1007/s13139-023-00816-3
![]() |
[12] |
Kung, T.H., Cheatham, M., Medenilla, A., Sillos, C., De Leon, L., Elepaño, C., et al., Performance of ChatGPT on USMLE: Potential for AI-assisted medical education using large language models. PLOS digital health, 2023, 2(2): e0000198. DOI: 10.1371/journal.pdig.0000198 doi: 10.1371/journal.pdig.0000198
![]() |
[13] |
Perera Molligoda Arachchige, A.S., New Horizons: The Potential Role of OpenAI's ChatGPT in Clinical Radiology. Journal of the American College of Radiology, 2023. DOI: 10.1016/j.jacr.2023.06.028 doi: 10.1016/j.jacr.2023.06.028
![]() |
[14] |
Perera Molligoda Arachchige, A.S. and Svet, A., Integrating artificial intelligence into radiology practice: undergraduate students' perspective. European journal of nuclear medicine and molecular imaging, 2021, 48: 4133–4135. DOI: 10.1007/s00259-021-05558-y doi: 10.1007/s00259-021-05558-y
![]() |
[15] | ChatGPT Passes Radiology Board Exam. RSNA, 2023. Retrieved from: https://www.rsna.org/news/2023/may/chatgpt-passes-board-exam |
[16] |
Perera Molligoda Arachchige, A.S., Large language models (LLM) and ChatGPT: a medical student perspective. European journal of nuclear medicine and molecular imaging, 2023, 50(8): 2248–2249. DOI: 10.1007/s00259-023-06227-y doi: 10.1007/s00259-023-06227-y
![]() |
[17] |
Giannos, P., Evaluating the limits of AI in medical specialisation: ChatGPT's performance on the UK Neurology Specialty Certificate Examination. BMJ neurology open, 2023, 5(1): e000451. DOI: 10.1136/bmjno-2023-000451 doi: 10.1136/bmjno-2023-000451
![]() |
[18] |
Yilmaz, R. and Yilmaz, F.G., Augmented intelligence in programming learning: Examining student views on the use of ChatGPT for programming learning. Computers in Human Behavior: Artificial Humans, 2023, 1(2): 100005. DOI: 10.1016/j.chbah.2023.100005. doi: 10.1016/j.chbah.2023.100005
![]() |
[19] |
Yilmaz, R. and Yilmaz, F.G., The effect of generative artificial intelligence (AI)-based tool use on students' computational thinking skills, programming self-efficacy and motivation. Computers and Education: Artificial Intelligence, 2023, 4: 100147. DOI: 10.1016/j.caeai.2023.100147 doi: 10.1016/j.caeai.2023.100147
![]() |
[20] |
Yilmaz, R., Yurdugül, H., Yilmaz, F.G., Şahi̇n, M., Sulak, S., Aydin, F., et al., Smart MOOC integrated with intelligent tutoring: A system architecture and framework model proposal. Computers and Education: Artificial Intelligence, 2022, 3: 100092. DOI: 10.1016/j.caeai.2022.100092 doi: 10.1016/j.caeai.2022.100092
![]() |
[21] | Yılmaz, R. and Yılmaz, F.G.K., Examining student satisfaction with the use of smart mooc, 2022. Retrieved from: https://acikerisim.bartin.edu.tr/handle/11772/6929 |
[22] | Yılmaz, R. and Yılmaz, F.G.K., Investigation of students' self-regulation skills, motivation and disorientation in smart mooc, 2022. Retrieved from: https://acikerisim.bartin.edu.tr/handle/11772/6932 |
[23] |
Imran, N., Hashmi, A. and Imran, A., Chat-GPT: Opportunities and Challenges in Child Mental Healthcare. Pakistan Journal of Medical Sciences, 2023, 39(4). DOI: 10.12669/pjms.39.4.81187. doi: 10.12669/pjms.39.4.81187
![]() |
[24] |
Sohail, S.S., Madsen, D.Ø., Himeur, Y. and Ashraf, M., Using ChatGPT to navigate ambivalent and contradictory research findings on artificial intelligence. Frontiers in Artificial Intelligence, 2023, 6: 1195797. DOI: 10.3389/frai.2023.1195797 doi: 10.3389/frai.2023.1195797
![]() |
[25] |
Cheng, S.W., Chang, C.W., Chang, W.J., Wang, H.W., Liang, C.S., Kishimoto, T., et al., The now and future of ChatGPT and GPT in psychiatry. Psychiatry and clinical neurosciences, 2023, 77(11): 592–596. DOI: 10.1111/pcn.13588 doi: 10.1111/pcn.13588
![]() |
[26] |
Madadi, Y., Delsoz, M., Lao, P.A., Fong, J.W., Hollingsworth, T.J., Kahook, M.Y., et al., ChatGPT Assisting Diagnosis of Neuro-ophthalmology Diseases Based on Case Reports. medRxiv, 2023. DOI: 10.1101/2023.09.13.23295508 doi: 10.1101/2023.09.13.23295508
![]() |
[27] | Haemmerli, J., Sveikata, L., Nouri, A., May, A., Egervari, K., Freyschlag, C., et al., ChatGPT in glioma patient adjuvant therapy decision making: ready to assume the role of a doctor in the tumour board? medRxiv, 2023, 2023-03. DOI: 10.1101/2023.03.19.23287452 |
[28] | McCallum, S., ChatGPT banned in Italy over privacy concerns. Retrieved from: https://www.bbc.com/news/technology-65139406 |
[29] | Yılmaz, R. and Yılmaz, F.G.K., Investigation of student views on data privacy and ethical use of data in smart learning environments, 2022. Retrieved from: https://acikerisim.bartin.edu.tr/handle/11772/6931 |
[30] |
Perera Molligoda Arachchige, A.S. and Stomeo, N., Controversies surrounding AI-based reporting systems in echocardiography. Journal of echocardiography, 2023, 1–2. DOI: 10.1007/s12574-023-00620-0 doi: 10.1007/s12574-023-00620-0
![]() |
1. | Ahmed Alsaedi, Bashir Ahmad, Afrah Assolami, Sotiris K. Ntouyas, On a nonlinear coupled system of differential equations involving Hilfer fractional derivative and Riemann-Liouville mixed operators with nonlocal integro-multi-point boundary conditions, 2022, 7, 2473-6988, 12718, 10.3934/math.2022704 | |
2. | M. Vellappandi, Venkatesan Govindaraj, José Vanterler da C. Sousa, Fractional optimal reachability problems with ψ ‐Hilfer fractional derivative , 2022, 45, 0170-4214, 6255, 10.1002/mma.8168 | |
3. | Fidel Meléndez-Vázquez, Guillermo Fernández-Anaya, Aldo Jonathan Muñóz-Vázquez, Eduardo Gamaliel Hernández-Martínez, Generalized conformable operators: Application to the design of nonlinear observers, 2021, 6, 2473-6988, 12952, 10.3934/math.2021749 | |
4. | Hamid Lmou, Khalid Hilal, Ahmed Kajouni, Serkan Araci, A New Result for ψ -Hilfer Fractional Pantograph-Type Langevin Equation and Inclusions, 2022, 2022, 2314-4785, 1, 10.1155/2022/2441628 | |
5. | Ravi P. Agarwal, Afrah Assolami, Ahmed Alsaedi, Bashir Ahmad, Existence Results and Ulam–Hyers Stability for a Fully Coupled System of Nonlinear Sequential Hilfer Fractional Differential Equations and Integro-Multistrip-Multipoint Boundary Conditions, 2022, 21, 1575-5460, 10.1007/s12346-022-00650-6 | |
6. | Abdulwasea Alkhazzan, Wadhah Al-Sadi, Varaporn Wattanakejorn, Hasib Khan, Thanin Sitthiwirattham, Sina Etemad, Shahram Rezapour, A new study on the existence and stability to a system of coupled higher-order nonlinear BVP of hybrid FDEs under the p-Laplacian operator, 2022, 7, 2473-6988, 14187, 10.3934/math.2022782 | |
7. | Sina Etemad, Iram Iqbal, Mohammad Esmael Samei, Shahram Rezapour, Jehad Alzabut, Weerawat Sudsutad, Izzet Goksel, Some inequalities on multi-functions for applying in the fractional Caputo–Hadamard jerk inclusion system, 2022, 2022, 1029-242X, 10.1186/s13660-022-02819-8 |
Parameters | Details |
β1 | Susceptible form of recovered individuals |
αm | Newborn mosquitoes transfer rate into Sm |
αh | Newborns ratio to enter in Sh |
γ1 | Transfer ratio of illness from one affected to susceptible people |
ψ | Transfusions rate of average blood with stable time in Ih and Sh |
β2 | Exposed to infected infection rate in people |
ξ | Infected mosquito susceptible bites using fixed time |
γ3 | Rate of Mosquitos infection |
γ2 | Disease transfer through infected (mosquito) to susceptible (individual) |
ηh | People natural rate of death |
δ2 | Exposed recovered population |
δ1 | Susceptible recovered population |
p | Rate of humans heal |
ε | Infected human death due to disease |
ηm | Mosquitos' natural death |
υ | Anti-malarial prescriptions |
e | Bites Sm using the infected humans |
σ | Expires sections using spraying for Sm and Im |
x | Time |
Case | MSE for instances of sampling | Gradient | Performance | Epoch | Time | ||
Test | validation | Train | |||||
I | 2.745×10−07 | 1.697 ×10−07 | 2.366×10−07 | 1.42×10−06 | 2.26×10−07 | 100 | 2 Sec |
II | 1.795×10−07 | 1.432×10−07 | 1.920×10−07 | 8.18×10−07 | 1.91×10−07 | 86 | 2 Sec |
III | 5.112×10−07 | 3.038×10−07 | 3.678×10−07 | 2.94×10−06 | 2.09×10−07 | 69 | 2 Sec |
Parameters | Details |
β1 | Susceptible form of recovered individuals |
αm | Newborn mosquitoes transfer rate into Sm |
αh | Newborns ratio to enter in Sh |
γ1 | Transfer ratio of illness from one affected to susceptible people |
ψ | Transfusions rate of average blood with stable time in Ih and Sh |
β2 | Exposed to infected infection rate in people |
ξ | Infected mosquito susceptible bites using fixed time |
γ3 | Rate of Mosquitos infection |
γ2 | Disease transfer through infected (mosquito) to susceptible (individual) |
ηh | People natural rate of death |
δ2 | Exposed recovered population |
δ1 | Susceptible recovered population |
p | Rate of humans heal |
ε | Infected human death due to disease |
ηm | Mosquitos' natural death |
υ | Anti-malarial prescriptions |
e | Bites Sm using the infected humans |
σ | Expires sections using spraying for Sm and Im |
x | Time |
Case | MSE for instances of sampling | Gradient | Performance | Epoch | Time | ||
Test | validation | Train | |||||
I | 2.745×10−07 | 1.697 ×10−07 | 2.366×10−07 | 1.42×10−06 | 2.26×10−07 | 100 | 2 Sec |
II | 1.795×10−07 | 1.432×10−07 | 1.920×10−07 | 8.18×10−07 | 1.91×10−07 | 86 | 2 Sec |
III | 5.112×10−07 | 3.038×10−07 | 3.678×10−07 | 2.94×10−06 | 2.09×10−07 | 69 | 2 Sec |