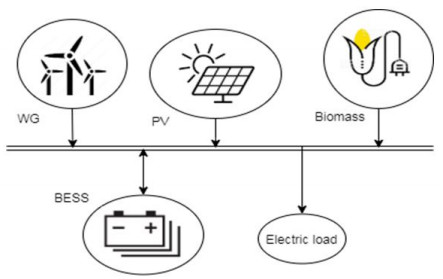
Black Americans (AA) face a confluence of challenges when seeking care including unaffordable costs, negative experiences with providers, racism, and distrust in the healthcare system. This study utilized linear regressions and mediation analysis to explore the interconnectedness of these challenges within a community-based sample of 313 AA women aged 45 and older. Approximately 23% of participants reported affordability problems, while 44% had a negative experience with a provider. In the initial linear regression model excluding perceived racism, higher levels of distrust were observed among women reporting affordability problems (β = 2.66; p = 0.003) or negative experiences with a healthcare provider (β = 3.02; p = <0.001). However, upon including perceived racism in the model, it emerged as a significant predictor of distrust (β = 0.81; p = < 0.001), attenuating the relationships between affordability and distrust (β = 1.74; p = 0.030) and negative experience with a provider and distrust (β = 1.79; p = 0.009). Mediation analysis indicated that perceived racism mediated approximately 35% and 41% of the relationships between affordability and distrust and negative experience with a provider and distrust, respectively. These findings underscore the critical imperative of addressing racism in the efforts to mitigate racial disparities in healthcare. Future research should explore the applicability of these findings to other marginalized populations.
Citation: Jacqueline Wiltshire, Carla Jackie Sampson, Echu Liu, Myra Michelle DeBose, Paul I Musey, Keith Elder. Affordability, negative experiences, perceived racism, and health care system distrust among black American women aged 45 and over[J]. AIMS Public Health, 2024, 11(4): 1030-1048. doi: 10.3934/publichealth.2024053
[1] | Qinghua Liu, Jing Wang, Keying Song . Displacement identification of oil storage tank and calibration of tank capacity table model based on indicator function integral. Mathematical Biosciences and Engineering, 2024, 21(2): 1872-1883. doi: 10.3934/mbe.2024082 |
[2] | Ping Zhou, Yuting Zhang, Yunlei Yu, Weijia Cai, Guangquan Zhou . 3D shape measurement based on structured light field imaging. Mathematical Biosciences and Engineering, 2020, 17(1): 654-668. doi: 10.3934/mbe.2020034 |
[3] | Alessia Andò, Simone De Reggi, Davide Liessi, Francesca Scarabel . A pseudospectral method for investigating the stability of linear population models with two physiological structures. Mathematical Biosciences and Engineering, 2023, 20(3): 4493-4515. doi: 10.3934/mbe.2023208 |
[4] | Mohamed S. Eliwa, Buthaynah T. Alhumaidan, Raghad N. Alqefari . A discrete mixed distribution: Statistical and reliability properties with applications to model COVID-19 data in various countries. Mathematical Biosciences and Engineering, 2023, 20(5): 7859-7881. doi: 10.3934/mbe.2023340 |
[5] | Pratik Purohit, Prasun K. Roy . Interaction between spatial perception and temporal perception enables preservation of cause-effect relationship: Visual psychophysics and neuronal dynamics. Mathematical Biosciences and Engineering, 2023, 20(5): 9101-9134. doi: 10.3934/mbe.2023400 |
[6] | Jin Li . Linear barycentric rational collocation method to solve plane elasticity problems. Mathematical Biosciences and Engineering, 2023, 20(5): 8337-8357. doi: 10.3934/mbe.2023365 |
[7] | Kelum Gajamannage, Erik M. Bollt . Detecting phase transitions in collective behavior using manifold's curvature. Mathematical Biosciences and Engineering, 2017, 14(2): 437-453. doi: 10.3934/mbe.2017027 |
[8] | Maysaa Al Qurashi, Saima Rashid, Sobia Sultana, Hijaz Ahmad, Khaled A. Gepreel . New formulation for discrete dynamical type inequalities via h-discrete fractional operator pertaining to nonsingular kernel. Mathematical Biosciences and Engineering, 2021, 18(2): 1794-1812. doi: 10.3934/mbe.2021093 |
[9] | Muhammad Bilal Khan, Pshtiwan Othman Mohammed, Muhammad Aslam Noor, Khadijah M. Abualnaja . Fuzzy integral inequalities on coordinates of convex fuzzy interval-valued functions. Mathematical Biosciences and Engineering, 2021, 18(5): 6552-6580. doi: 10.3934/mbe.2021325 |
[10] | Ke Bi, Yue Tan, Ke Cheng, Qingfang Chen, Yuanquan Wang . Sequential shape similarity for active contour based left ventricle segmentation in cardiac cine MR image. Mathematical Biosciences and Engineering, 2022, 19(2): 1591-1608. doi: 10.3934/mbe.2022074 |
Black Americans (AA) face a confluence of challenges when seeking care including unaffordable costs, negative experiences with providers, racism, and distrust in the healthcare system. This study utilized linear regressions and mediation analysis to explore the interconnectedness of these challenges within a community-based sample of 313 AA women aged 45 and older. Approximately 23% of participants reported affordability problems, while 44% had a negative experience with a provider. In the initial linear regression model excluding perceived racism, higher levels of distrust were observed among women reporting affordability problems (β = 2.66; p = 0.003) or negative experiences with a healthcare provider (β = 3.02; p = <0.001). However, upon including perceived racism in the model, it emerged as a significant predictor of distrust (β = 0.81; p = < 0.001), attenuating the relationships between affordability and distrust (β = 1.74; p = 0.030) and negative experience with a provider and distrust (β = 1.79; p = 0.009). Mediation analysis indicated that perceived racism mediated approximately 35% and 41% of the relationships between affordability and distrust and negative experience with a provider and distrust, respectively. These findings underscore the critical imperative of addressing racism in the efforts to mitigate racial disparities in healthcare. Future research should explore the applicability of these findings to other marginalized populations.
In Egypt, many new projects are being performed in various areas, such as those in the new valley of the western desert and those in Egypt's northern coastal borders. These projects will help in the horizontal transferring of people from the cramped Nile valley to other uninhabited different locations to reduce the metropolis's crowdedness as approximately 95% of the country's population live along the sides of the Nile river and inside the Nile Delta [1]. Egypt's electricity consumption grew rapidly in the last few years as a result of economic growth. Still, it remains a challenge for Egypt to provide stable electricity access to rural areas. The population augmentation and the new towns' establishment are presenting an extreme burden on the electrical network. The Egyptian government is trying these days to turn to renewable energy sources to tackle fossil fuel shortage. Egyptian economy balance would be threatened without depending on renewable sources as it will be a great importer of fossil fuels. Integrating renewable energy sources to power systems and microgrids establishment are the best options for handling the electricity crises and decreasing the burden on the grid [2].
Microgrid systems transfer the conventional power system with a single power supply and radial network into a new distribution system with multi-power sources. It can be categorized as isolated or grid-connected, relying on the connection with the public grid [3]. In isolated microgrids, it is essential for each microgrid to self satisfy its load requirements as it is considered a small power system with its own generation, transmission, control and distribution [4]. Renewable energy system is a suitable cheap technological approach for rural electrification. It stimulates the economy and communities to enhance their standards. Small-scale distributed renewable generation systems are considered these days as a vital solution for energy problems [5].
Globally, the total renewable power capacity reached 2195 GW. Renewable energy sector employed around 10.3 million people (directly and indirectly), and approximately $ 280 billion were spent on new investments [6]. Almost 145 countries have implemented policies to promote clean energy technologies over the last few decades. According to the reports of BP Energy (2020), renewable energy (including biofuels) recorded a large increment in energy consumption of (3.2 EJ) in 2019. Egypt has committed to increasing its dependency on sustainable energy technologies. According to the international renewable energy agency's (IRENA) reports, the total combined renewable capacity to the grid was represented by 3.7 gigawatts (GW) in 2018. This amount is composed of 2.8 GW from hydropower and about 0.9 GW from solar and wind power. The Egyptian government aims to have 20% of its electricity from renewable energies by 2022 and 42% in 2035 [7]. Egypt has abundant chances to achieve these goals, as it enjoys plenty of renewable energy resources, including hydropower, wind, solar and biomass. Egypt has a significant potential for investments in solar energy sector because it is one of the Sunbelt countries. Besides, its sunlight period is between 3500 and 4500 hr/year with around 12–30 MJ/m2/day [8]. Egypt is also encouraging wind farms establishment. Its average wind speed is about 10.5 m/s for average 100-meter hub heights.
Hybrid renewable generation offers more flexibility and lower cost alternatives compared to relying upon one source. Countries with a wealth of sunlight radiation, strong potential of bio-degradable waste and a decent wind energy supply can handle the electricity access for far areas. By considering the sustainable energy sources uncertainties (solar, wind, etc.), battery energy storage systems (BESS) and other reservoirs like biogas energy sources are nominated to be integrated with renewable sources to ensure uninterrupted electricity and power security [9].
Biomass is a renewable energy source that can be easily transformed into electricity through the gasification process. Compared with fossil fuel technologies, biomass technology has many environmental and economic advantages. High-capacity biomass gasification units are the best choice because of their investment efficiency and effectiveness. Small-scale biomass gasification is vital in providing electricity for localized usage where the low electrification level and the unstable transmission network [10]. Rice straw is an attractive biomass solid waste due to its availability in rural areas and its high calorific value. Rice is one of the most common crops in Egypt. Open-field burning is the main environmental issue of rice farming in most countries. Farmers have a limited time to clear their fields after the harvest ends. They burn the rice straw to start planting seeds for the next season. This behaviour has many impacts on carbon dioxide (CO2) emissions, which is the main combustion product that creates dark clouds, and plays a major role in children's exacerbating asthma. Managing the straw after rice harvest is a critical problem these days, and one of the appropriate solutions is using it as a fuel in gasification-electricity procedures [11,12].
Many authors have studied the optimum operation of hybrid microgrids, including renewable energy sources. Reference [13] studied the microgrid's short-term economic and environmental dispatch problem using enhanced quantum particle swarm optimization (QPSO) to improve the units' performance that helps to minimize polluting gas emissions. In reference [14], a detailed comparison of three different DC microgrids has been held (one grid-powered based on conventional fuel generation, and the other two off-grid based on solar energy generation). The comparison aimed to highlight the most notable positive and negative aspects regarding technical efficiency, economic and environmental impacts. In reference [15], a detailed literature review of the current microgrid's sizing methods was presented. The surveyed methods were split into two major groups of cost-based and non-cost-based sizing methods. Reference [16] provides a comprehensive overview of the most widely used tools for designing HRESs. It also explains how social factors such as job development and social acceptance improve such software packages' capabilities. Renewable energy integration to replace diesel power generation is projected to be cost-effective for off-grids and substantially reduces greenhouse gas emissions. Hybrid systems are highly recommended for isolated microgrids to guarantee reliability overcome battery problems [17]. In reference [18], the authors explored the possibility and the benefits of merging renewable energy technologies such as solar and wind with traditional power generation systems like diesel and natural gas sources. In [19], the optimum generation capacity and storage for a hybrid (PV/wind/BESS) system based on instantaneous wind speed variations are introduced to maintain system reliability and reduce the investment cost. In reference [20], optimal sizing of PV/diesel/battery system is researched, including V2G. In reference [21], PV/WG isolated microgrid is sized using the critical month by algorithmic optimization techniques and Homer Pro. In reference [22], Grasshopper Optimization Algorithm (GOA) is used in optimal microgrid sizing to supply energy demand reliably based on the deficiency of power supply probability (DPSP) and the cost of energy (COE). Energy storage systems act as a tool for handling peak load demand as well as boosting the gains from the thermal power plant while enhancing the system stability and reliability [23]. Authors in ref [24] studied the integration of BESS in efficient rural electrification. BESS' optimum sizing guarantees the system reliability and extends battery life which affects the replacement cost. In reference [25] general parallel genetic algorithm is used to maximize the technical benefits for the distribution system by optimally integrating PV and energy storage systems.
Biomass is a valuable resource that supplements other renewable sources if it is used in electricity generation. It has zero emissions if combined with technologies for carbon capture and storage [26]. Biomass gasification is a low-cost tool for providing electricity to off-grid rural communities [27]. For the Sharjah town's optimal electrification, micropower optimization methods are used to design a solar/biomass hybrid renewable energy-based microgrid [28]. Reference [29] used rice husk as the basis of energy for paddy parboiling and drying, also studied the worked gasifier's energy, exergy analysis and the gas-fired boiler equipment. Large-scale biomass to hydrogen technology is one of the brightest future technology in the sustainable energy sector [30]. Gasification technology is viewed as an effective thermochemical process either to substitute fossil fuel in existing diesel engines or to produce electricity using gas engines [31]. This technique enables the implementation of electrification programs in rural areas and improves grid quality. Reference [32] has studied the combustion of rice straw to obtain energy and its related problems in China. Roughly 3.1 million tons/year of rice straw in Egypt are projected to be disposed of by burning directly in the open air, so the recycling is an urgent necessity. Reference [33] investigated rice straw's ability for energy production in Egypt. The simulation also illustrated the rice straw power plant's efficiency to determine the required amount of energy, minimize fossil fuels reliance and greenhouse gas emissions reduction.
On the other hand, RESs have been shown to have short-term fluctuations, and their energy profiles are not entirely predictable. Using information from nearby substations improves prediction accuracy and reduced data imputation errors [34]. Uncertainty modelling outlines renewable sources' fluctuations. Reference [35] showed the impacts of load and wind uncertainties in increasing the cost by 22%. In reference [36], the stochastic energy operation management is studied for grid-connected MG, including WT/PV/Micro-Turbine/Fuel Cell and BESS. Scenario-based strategies convert the stochastic problem to several deterministic problems. Monte-Carlo method is a suitable method for scenarios generation [37]. Genetic algorithm and Monte-Carlo method are used in optimal BESS sizing, taking into consideration the wind power uncertainty due to wind speed fluctuation in reference [38]. Optimal design and operation for isolated microgrids are discussed in reference [39], considering solar energy generation's stochastic behaviour. In reference [40], The point estimate method (PEM) is used to view the uncertainty linked to wind and solar powers. Reference [41] studied the consequences of renewable sources, market price and load uncertainties in optimal scheduling of a grid-connected microgrid over a 24-hour horizon, using the modified bat algorithm (MBA). The probability density functions are used in modelling renewable energy sources uncertainty. In reference [42], a multi-scenario optimization model based on the minimum life cycle cost is applied using the general algebraic modelling system (GAMS) to choose the best siting, sizing, and type of renewable sources in the distribution system.
The power sector is essential to Egypt's socioeconomic growth and lowering poverty goals [43]. Depending on the previous literature, this paper presents a renewable energy study for rural communities electrification without grid connections. A comparison between different combinations of sources is also presented, using PV modules, wind, and biogas generators. The main contribution of the proposed scheme in this manuscript depending on all the above, are as follows:
1. An optimal sizing approach of a real new residential area in Egypt using renewable energy sources is proposed.
2. Uncertainty of renewable energy methodology is considered with a complete study for its effects on the optimal sizing and cost schemes.
3. The bio-gasification generation is implemented to deepen its effects on the environment and power system generation.
4. Environmental effects of rice straw recycling through biomass gasification are introduced.
5. Three different operation mechanisms are introduced according to the availability of power conversion sources.
6. Nine case studies with detailed analysis are presented to confirm their used renewable generation robustness and effectiveness.
7. A comparison of two optimization algorithms is held to ensure the system's feasibility.
The frame of the remaining sections is written as follows; in section 2, the system is described while the objective functions are discussed in section 3. In section 4, the system constraints are introduced. Different optimization techniques are discussed in section 5. The system uncertainties are illustrated in section 6. Also, the simulation and comparison between nine optimized scenarios are shown in section 7. The results are discussed in section 8, while the conclusion and future work are then held in section 9.
The proposed system configuration with the available power generation units is presented in Figure 1. The system's operation is categorized into three different operation mechanisms depending on the used units and the storage system availability. The first category uses renewable energies without using batteries. The load generation mismatch is faced through biomass generation. The second category uses a combination of renewable energy sources, BESS, and biomass generation to ensure full system reliability. In the third category, the system consists of renewable energy sources with batteries and without biomass generation availability. Different scenarios of each category are studied in this paper, taking into consideration the system constraints to reach the optimal cost and reliable configuration of each scenario. Also, a comparison between scenarios is held to show the economic feasibility and the environmental response of each system. Figure 2 shows the mechanisms of each category. The system components are discussed in the following paragraphs.
Photovoltaic (PV) is the simplest energy technology to convert solar energy into electricity. It features easy installation, high reliability and low maintenance cost. PV solar modules' power depends mainly on environmental and meteorological data like solar radiation and the daily sun existence duration. The instantaneous generated output power of a PV system (Ppv(t)) with respect to the incident solar irradiance Id(t) in (Wh/m2) can be calculated by using [44]:
Ppv(t)=ηpv∗Spv∗Id(t)Id,Stc | (2.1) |
Where ηpv is PV's efficiency, Spv and Id,Stc are the rated capacity of the PV in kW and solar radiation at the standard test condition, respectively.
The instantaneous wind generator's (WG) output power depends on the wind speed at a specified hub height. The output power of a WG can be described by using the function described in Eq (2.2):
{Swt∗w3−w3cinw3r−w3cot(wcin≤w≤wr)Swt(wr≤w≤wcot)0(w<wcinorw>wr)} | (2.2) |
Where Swt is the rated power capacity of the WG. wcin, wr and wcot are the cut-in, rated and cut-out wind speed, respectively [45].
Biomass gasification is the method of transforming solid bio-waste into an ignitable gas mixture composed mainly of carbon monoxide, oxygen, methane, hydrogen, etc. It can be used for thermal purposes or as a fuel for mechanical or electric power generation in internal combustion engines.
The power of biomass depends mainly on the calorific value and the available biomass amount [46]:
Pbg=Totalbiomassavailable(Ton/yr)∗1000∗CVbm∗ηbm365∗860∗Operatinghours/day | (2.3) |
The biogas operating and maintenance cost includes two parts (fixed and variable cost), depending on the proposed power and the used fuel amount:
OMbg,npv=θ1bg∗Pbg∗N∑j=1(1+μbg1+ir)j+θ2bg∗PWyrbg∗N∑j=1(1+μbg1+ir)j | (2.4) |
The amount of biogas fuel cost is described in Eq (2.5):
Fbg,npv=θ3bg∗BFyrr∗N∑j=1(1+μbg1+ir)j | (2.5) |
The capital and salvage values of biomass unit are described in Eqs (2.6) and (2.7):
Cbg=γbg∗Pbg | (2.6) |
SVbg,npv=λbg∗Pbg∗(1+δ1+ir)N | (2.7) |
Where CVbm is the biomass calorific valve, ηbm is the overall conversion efficiency, θ1bg is the annual fixed operation and maintenance cost ($/kW/year), Pbg is the power produce by biogas generator, ir is the interest rate, μbg is the escalation rate, θ2bg is the variable operation and maintenance cost ($/kWh), PWyrbg is the annual working power of biogas generator (kWh/year), θ3bg is the biomass fuel cost ($/ton), BFyrr is the annual required biomass fuel (ton/year), γbg is the initial cost of biogas system ($/kW), λbg is the resale price of the system ($/kW), and δ is the inflation rate [47].
Battery energy storage systems are used to match the generated energy with the consumed energy and enhance the system's energy balance by storing the energy from different sources such as solar and/or wind generators. For example, excess energy due to high levels of wind speeds or solar radiation will be stored in batteries. This stored energy will be released for smoothing the injected power into the system, especially in low renewable energy capabilities [48].
The charging equation:
SOC(t)=SOC(t−1)∗(1−σ)+(Pg(t)−Pl(t)ηinv)∗ηbc | (2.8) |
The discharging equation:
SOC(t)=SOC(t−1)∗(1−σ)+(Pl(t)ηinv−Pg(t))/ηbd | (2.9) |
Eqs (2.10) and (2.11) describe the capital and replacement cost values of BESS:
CBat=NBat∗αBat | (2.10) |
Rnpv,bat=NBat∗αbat∗∑j=5,10,15(1+μbat1+ir)j | (2.11) |
Where SOC is the state of charge, σ is the hourly rate of self-discharge, Pl(t) is the load demand, Pg(t) is the generated power, NBat is the number of battery units, ηbc is the charging efficiency, ηbd is the discharging efficiency, μbat is the escalation rate, αbat is the cost of battery set in $ and ηinv is the inverter efficiency [49].
The optimum system sizing's objective functions are the minimization of load-generation mismatch (loss of power supply LPS) and the total system cost minimization. The first objective function is described in the following equation:
F1=min:(LPS(t))=min:(|Pl(t)−(Pg(t))|) | (3.1) |
If the system uses BESS, the first objective function will be expressed as :
F1=min:(LPS(t))=min:(|Pl(t)−(Pg(t)+SOC(t−1)−SOC(t))|) | (3.2) |
Where Pg(t) and Pl(t) are the summation of the generated power and load power, respectively.
The total cost minimization is the second objective function:
F2=min:(cost)=min:∑Ck+OMk+Rk−SVk∀k∈PV,W,Bat,Bio | (3.3) |
Where Ck is the capital cost of component k, OMk is the operating and maintenance cost of component k, Rk is the replacement cost, and SVk is the salvage cost of each component.
For optimal sizing and achieving all objective functions, the system is subjected to some restrictions.
The limits constraints of maximum and minimum power values of each component are represented in Eqs (4.1)–(4.4):
0≤PPV≤PPv,max | (4.1) |
0≤PW≤PW,max | (4.2) |
0≤Pbio≤Pbio,max | (4.3) |
0≤NBat≤NBat,max | (4.4) |
Battery's SOC defines the remaining power and reflects battery effectiveness. At any time, the value of SOC has to be within the specified limits (20% and 80% for most control strategies) to prevent batteries' damage, secure battery, avoid over-discharging, and increase battery life-time [50,51]. The state of charge is controlled within limits for also dealing with the sudden fluctuations and changes in renewable energies, especially for deterministic scenarios [52].
SOCmin≤SOC(t)≤SOCmax | (4.5) |
where
SOCmin=0.2∗NBat∗Cb | (4.6) |
SOCmax=0.8∗NBat∗Cb | (4.7) |
The total generated power by the available generating units of each scenario, such as WG, PV, biogas generator and ESS should always meet the load demand requirements in all considered scenarios. Eq (4.8) shows the sum of available PV and WG powers at each time, depending on their availability in each scenario configuration. Eqs (4.9)–(4.11) show the power balance constraint of each category.
Pgr(t)=Pw(t)+PPV(t) | (4.8) |
For category (A):
Pgr(t)+Pbio(t)=PL(t) | (4.9) |
For category (B):
Pgr(t)+Pbio(t)+PdisBESS(t)−PchBESS(t)=PL(t) | (4.10) |
For category (C):
Pgr(t)+PdisBESS(t)−PchBESS(t)=PL(t) | (4.11) |
Multi-objective optimization methods are used in this paper to consider all potential trade-offs between different objective functions, which are normally conflicting. Two optimization algorithms are discussed in this paper to find the optimum solution. Each algorithm uses the available input data (load demand profile, generating components, available resources and weather data) to achieve all the system's objectives. Optimal system configuration determines optimal system size, net present cost, total capital cost and the corresponding CO2 reduction. The architecture of the optimization tool is illustrated in Figure 3.
Multi-objective genetic algorithm is a meta-heuristic mechanism motivated by the natural selection technique, which is a part of larger classes of evolutionary algorithms. Genetic algorithms are widely used and biologically inspired by developers to produce high-quality optimization and search prospects, like mutation, crossover and selection.
MOGA utilizes a weighted sum of various objective functions in the selection stage and merges them into a scalar fitness function. The design characteristic of the various objective functions weights are not specified and randomly changed through each selection. Thus the search orientation in this algorithm is not fixed.
At each generation over the process of MOGA, an empirical series of pareto optimal solutions are stored and updated. Also, a certain number of solutions are picked at random from the series. Those solutions are considered elite individuals. The elite mechanism has the benefit of preserving the diversity of each population [53].
The block diagram of the proposed MOGA algorithm is shown in Figure 4, and it is described below:
● Stage 1 (Initialization): generate an initial population.
● Stage 2 (Evaluation): calculate the values of the objective functions for the created population.
● Stage 3 (Selection): use random weights to determine each population's fitness value, then pick a pair of strings from the existing population.
● Stage 4 (Crossover and Mutation): a crossover strategy is implemented for each chosen pair to produce a new population via the crossover process. After that, the mutation process is carried out.
● Stage 5 (Elitist): delete some strings of created strings haphazardly and substitute them with elite strings picked at random from temporary optimal pareto solutions.
● Stage 6 (Termination): if the stopping requirement is not satisfied, go to Stage 2.
● Stage 7 (Optimal Solution): the MOGA suggests the preferable options.
The ε−multi-objective genetic algorithm depends on the vision of ε−dominance to test the optimisation's stored results in the A(t) archive. ε−pareto set (x∗) is produced, and it seeks to reach the optimal pareto front F(x∗) with bounded memory sources. Furthermore, It continuously changes the pareto front boundaries and avoids any loss of the solutions belonging to the front ends. The objective space is sectioned to a particular number of boxes Nbox which can be calculated from:
Nbox=Fmaxi−Fminiε,Fmaxi=maxF(x)i,Fmini=minF(x)i,∀x∈X∗ | (5.1) |
where ε is the box's width and i∈ [1, 2....., N].
Each box can be filled with only one solution, which helps to preserve the divergence of F(x∗). For a specific solution, the boxi(x) is defined by:
boxi(x)=Fi(x)−FminiFmaxi−Fmini∗Nbox | (5.2) |
A solution with x1ε-dominates x2 where x1<εx2, when and only if
(box(x1)<box(x2)∨(box(x1)=box(x2)andx1<x2) | (5.3) |
Thus, a set x∗∈x is ε-pareto when and only if
∀x1,x2∈x∗,x1≠x2,box(x1)≠box(x2)∧box(x1)>εbox(x2) | (5.4) |
A(t) is always updated by ε-dominant solutions which do not share the same box. The remaining solution always occupies the center of the box to accomplish the prevention of solutions belonging to neighboring boxes and increase the solution variety [54,55]. ε's goal is to reach X∗ with as many solutions as possible to adequately characterize the pareto front, which is bounded by:
Nsmax=ΠNi=1Nboxi+1Nmaxbox+1,Nmaxbox=max[Nboxi] | (5.5) |
Three populations are generated To ensure solution variance. P(t) is the main population, Q(t) is the auxiliary population, and A(t) represents the archive of the best solutions. The proposed algorithm's principal steps are as follows:
● Step 1: Begin with creating empty A(t).
● Step 2: Initializing the base population at random P(0).
● Step 3: Calculate the corresponding function value P(t).
● Step 4: Test the applicable values to be included in A(t).
● Step 5: Generate Q(t) by using two random individuals from P(t) and A(t).
● Step 6: Calculate the corresponding function value for each individual in Q(t).
● Step 7: According to the objective space location, test which individual can be added to A(t).
● Step 8: Update P(t) with individuals from G(t) by randomly comparing each value of G(t) with selected individuals of P(t).
● Step 9: Eventually, the pareto front's smart characterisation is formed by individuals from A(t).
Because of weather effects on renewable energy, it became increasingly difficult to guarantee the system's reliability. Therefore, it is necessary to solve this issue by introducing PV and wind sources' uncertainty that primarily depend on weather conditions. Monte-Carlo Simulation (MCS) or Monte-Carlo approach provides an appropriate solution to quantitative issues by carrying out statistical sampling procedures.
A variety of random configurations may be used in this case to produce data and samples to reflect the solar radiation and wind speed variation. Each input is independently sampled to construct a random sequence {xi}Ni=1 from Ud(0, 1) with estimated mean of [56]:
¯x=1NN∑i=1xi | (6.1) |
The general approach of solving stochastic formulations is to transform stochastic models into deterministic problems. Monte Carlo approach succeeds in representing uncertainties by inputs sampling and defining them through the probabilities distribution functions [57,58].
MCS is conducted to generate a finite number of possible scenarios based on the probability distributions for all stochastic parameters [59], as shown in Figure 5. The microgrid sizing procedures with MCS used the same parameters of the deterministic problem formulation and can be described as follows [38]:
● Step 1: Initialize algorithm parameters.
● Step 2: Apply Monte-Carlo simulation techniques to produce plentiful different scenarios with the same probability.
● Step 3: In each scenario, the optimum size is calculated by operating strategy.
● Step 4: Test the constraint satisfaction to create the next generation and jump to step 5.
● Step 5: Repeat step 2 to 4 till the specific iteration step is completed, or other termination conditions are met.
Figure 6 shows fifty uncertain-based scenarios for wind speed and solar radiation.
Two optimization techniques are applied for nine scenarios of the microgrid to ensure load satisfactions. The comparison between these techniques is shown in Table 1. For comparison, the same systems are also studied in deterministic and stochastic cases to show the uncertainties effects in selecting the appropriate capacities of generating units, storage systems, and proper biomass generation implementation. Table 2 shows the stochastic results. The economic and technical specifications of the proposed system's components are listed in Table 3. In this work, the optimization and Monte Carlo simulation have been coded in MATLAB software.
Cost | PV | WG | No of | Pbiomass | ||
($) | (KW) | (KW) | Batteries | (max) KW | ||
Category A | ||||||
Scenario 1 | MOGA | 1.787∗108 | 100.072 | 0 | 0 | 321.810 |
(PV+Biomass) | ϵ−MOGA | 1.789∗108 | 99.883 | 0 | 0 | 328.254 |
Scenario 2 | MOGA | 1.539∗108 | 0 | 705.943 | 0 | 277.906 |
(WG+Biomass) | ϵ−MOGA | 1.540∗108 | 0 | 705.770 | 0 | 278.426 |
Scenario 3 | MOGA | 1.480∗108 | 21.118 | 678.794 | 0 | 279.669 |
(PV+WG+Biomass) | ϵ−MOGA | 1.479∗108 | 20.052 | 684.906 | 0 | 280.355 |
Category B | ||||||
Scenario 4 | MOGA | 3.455∗107 | 462.114 | 0 | 23 | 94.150 |
(PV+Biomass+BESS) | ϵ−MOGA | 2.647∗107 | 488.195 | 0 | 22 | 328.917 |
Scenario 5 | MOGA | 6.649∗107 | 0 | 1883.090 | 14 | 95.797 |
(WG+Biomass+BESS) | ϵ−MOGA | 2.953∗107 | 0 | 2297.645 | 14 | 303.548 |
Scenario 6 | MOGA | 6.030∗107 | 413.147 | 292.798 | 24 | 88.689 |
(PV+WG+Biomass+BESS) | ϵ− MOGA | 3.236∗107 | 0 | 2500 | 17 | 330 |
Category C | ||||||
Scenario 7 | MOGA | 4.040∗106 | 535.060 | 0 | 22 | 0 |
(PV+BESS) | ϵ−MOGA | 3.359∗106 | 474.495 | 0 | 18 | 0 |
Scenario 8 | MOGA | 9.397∗106 | 0 | 2469.141 | 13 | 0 |
(WG+BESS) | ϵ−MOGA | 9.397∗106 | 0 | 2469.182 | 13 | 0 |
Scenario 9 | MOGA | 5.599∗106 | 562.579 | 272.012 | 27 | 0 |
(PV+WG+BESS) | ϵ−MOGA | 5.204∗106 | 634.020 | 0 | 29 | 0 |
Configuration | Cost | PV | WG | No of | Pbiomass | |
($) | (KW) | (KW) | Batteries | (max) KW | ||
Category A | ||||||
Scenario 1 | PV+Biomass | 1.911∗108 | 77.534 | 0 | 0 | 322.528 |
Scenario 2 | WG+Biomass | 1.933∗108 | 0 | 317.317 | 0 | 329.821 |
Scenario 3 | PV+WG+Biomass | 1.890∗108 | 42.377 | 182.208 | 0 | 320.965 |
Category B | ||||||
Scenario 4 | PV+Biomass+BESS | 8.979∗107 | 501.204 | 0 | 33 | 191.922 |
Scenario 5 | WG+Biomass+BESS | 1.246∗108 | 0 | 1698.970 | 30 | 155.320 |
Scenario 6 | PV+WG+Biomass+BESS | 7.533∗107 | 458.217 | 701.070 | 42 | 103.754 |
Category C | ||||||
Scenario 7 | PV+BESS | 4.327∗106 | 504.755 | 0 | 25 | 0 |
Scenario 8 | WG+BESS | 1.007∗107 | 0 | 2166.951 | 25 | 0 |
Scenario 9 | PV+WG+BESS | 7.912∗106 | 773.901 | 9.108 | 47 | 0 |
Component and Economic Specification | ||
Discount rate (r) | 5% | |
Escalation rate | 7% | |
PV Module | ||
Investment cost | 1690 | $/KW |
Maintenance | 26 | $/KW/yr |
(PV) reduction factor | 84% | |
lifetime | 25 | years |
Wind Generator | ||
Investment cost | 2030 | $/KW |
Operating and Maintenance | 75 | $/KW/yr |
lifetime | 25 | years |
Cut-in wind speed | 4 | m/s |
Cut-out wind speed | 25 | m/s |
Rated wind speed | 14.5 | m/s |
Shear coefficient | 0.14 | |
Turbine hub height | 50 | m |
BESS | ||
Investment cost | 200 | $/KWh |
Replacement cost | 200 | $/KWh |
Self discharge rate | 0.01% | |
Efficiency | 90% | |
Life time | 5 | years |
Biomass generator | ||
Investment cost(γbg) | 6200 | $/KWh |
Annual fixed operation and maintenance cost of biomass system(θ1bg) | 0.05*γbg | $/Kw/year |
Variable operation and maintenance cost of biogas system(θ2bg) | 0.0042 | $/Kw/year |
Biomass fuel cost of biogas system(θ2bg) | 35 | ($/t/year) |
Biomass system resale price(λbg) | 0.3*γbg | ($/Kw) |
Electrical conversion efficiency(ηbm) | 0.21 |
In this category, there is no storage system. All available generated power is used directly to fulfil load requirements. Three combinations of generating units are studied in this category. Scenario (1) consists of PV with biomass, while scenario (2) consists of WG with biomass, and scenario (3) consists of a combination of PV and WG with biomass generator.
Figures 7–9 show the generation load mismatch and the energy share of each generating unit for each scenario. The total cost of each scenario is plotted in Figure 10 to show the comparison between scenarios and show the percentages of capital cost with running cost.
Category (B) represents batteries and biomass generation's participation in the same configuration with other renewable sources. Scenario (4) consists of PV with biomass and BESS units. Scenario (5) integrates batteries with WG and biomass generators. Scenario (6) represents the share of all available system components (PV, WG, BESS and biomass).
Figures 11–13 show the generation load mismatch and each generating unit's energy share for scenarios 4–6, respectively. Figure 14 shows the total system cost for each of category B' scenarios.
This category is considered the most simple and economical category, but it has the lowest environmental concern of CO2 emissions and rice straw problems. In this kind of systems, there is no existence of biomass technology. The power generation depends mainly on a combination of PV and WG. Figures 15–17 show the generation load mismatch and each generating unit's energy share for scenarios 7–9, respectively. The capital cost and operating with maintenance cost of this category are shown in Figure 18.
In all scenarios, the load is met with optimal system size using two multi-optimization techniques. It is found that the results of the two optimization techniques have some similarities in some cases and differences in other scenarios. Thus, the simulation results for the nine scenarios confirm the following features:
1. Scenario 7 is the cheapest scenario between all discussed scenarios by a total system cost of $ 4.040 million with a PV capacity of 535.060 kWh and a total number of 22 battery sets. In contrast, PV without BESS scenario is not recommended because it is the highest cost scenario. It does not use the available capabilities of solar energy due to the lack of energy storage systems. However, it would be applicable in case of applying load demand control strategies.
2. ε-MOGA gives almost nearly equal results as MOGA for category A and C, but it gives different results for category B. It uses biomass generators with high capacity in category B, but it is used for a limited no of hours during the day, which causes the low cost of fuel and low corresponding total cost. Although the results of ε-MOGA for category B seems to be better than MOGA, it is not accepted as biomass generator is planned to be used for a limited number of hours during the day. It can not be used as a backup generator because biomass units take time to be inserted to the system.
3. Without using BESS as in category A, the amount of biomass share is very high. It reaches 81% in scenario 1 as the PV is the only generating system with biomass. Besides, PV does not work for more than thirteen hours of the all-day hours, as seen in Figure 7a. Introducing wind generators in category (A) is noticed to be more dependable as it exists during all-day hours. Although wind speed is not high at all times, its existence is better than solar existence. Biomass share is reduced to around 69% in scenario 2 and 3, which reduces the cost and increases the share of other generating units like WG in scenario (2) and both PV and WG in Scenario 3.
4. Category (B) is considered a mix between category (A) and (C). Both BESS and biomass are considered in this category. It is the most reliable category as BESS enhances the system performance in fluctuated weather conditions. In addition, bio-gas can be considered a backup generation in case of load increase or other generating unit outages. In this category, the percentage of biomass's share is decreased, and the percentage share of other renewable sources is increased. Wind generation share is about 75% and 50% in scenarios 5 and 6, respectively. While PV energy share is about 86% and 35% in Scenarios 4 and 5. Biomass energy share in scenario 4 is the same as scenario 6 by a value of 14%. This percentage increases in the case of PV absence, as in scenario 5.
5. From deterministic results, PV and wind power capacities increased by the integration of BESS. By comparing category A with category B, PV increased by (3.6%) from scenario 1 to scenario 4. Also, WG capacity increased by (1.6%) from scenario 2 to scenario 4. In scenario 3, BESS integration increases PV capacity by (392.029 kWh) and decreases WG capacity by (386.004 kWh) as noticed from scenario 6's results.
6. From shown results in table 1, if BESS replaces biomass units in category A as in Category C, the cost of scenarios 1–3 will be decreased by ($ 174.66 million), ($ 144.503 million) and ($ 142.401 million) respectively. Removing biomass generators from category B reduces the total system cost and increases other renewable sources' share. By comparing scenario 4 with 7 and 5 with 8, PV power increased by (72.9 kWh), and wind power increased by (586.051 kWh). Also, by comparing scenario 6 with scenario 9, PV power is increased, and WG power is decreased like what happened in comparing scenarios 3 and 6.
7. PV energy share represents 100% in scenario 7, and WG represents the same amount in scenario 8. It is found that category c is the most economically effective category as it recorded the least cost between other categories with about total cost of ($ 4.040 million), ($ 9.397 million) and ($ 5.599 million) for scenarios 7–9, respectively.
8. In scenario 9, the power share of PV is nine times the power share of wind. In the case of no storage, it is not economical to use a high PV amount.
9. When comparing scenario 7 with scenario 8, PV introduction is better than WG, with a cost reduction of about (57%). In comparison, (PV+WG+BESS) scenario is noticed to have ($ 1.559 million) over cost compared to scenario 7.
10. Biomass generation cost is high due to the high operating cost, including fuel, operating and maintenance costs. The high cost of Categories A and B illustrates the high use of biomass generation, as shown in Figures 10 and 14. Differently, the capital cost in category C's scenarios represents a significant percentage of the total system's cost, including the installation and establishment of the system, as seen in Figure 18.
11. (PV + BESS) is the best economical choice for sizing, but it is still unreliable if the weather changes as there is only a single generating source. In contrast, applying (PV with Biomass) is the highest cost scenario due to the storage system absence, so all power is fed at night from the biomass system, which is costly.
12. Biomass is an expensive choice for electricity generation, but it is an environmentally friendly choice that increases system reliability, especially in critical situations. It can be used as a backup in case of generation shortage. It is not economically recommended to use biomass generators alone without other generation sources. Hybrid renewable energy is the best energy choice for planning isolated microgrid as it leans on different types of sources. Moreover, renewable sources with storage systems are considered the best reliable option.
13. Using biomass for electrical generation is not effective only as a power source. It is considered a recycling process to get rid of unwanted waste. Rice straw using as biomass fuel helps in different environmental trends like clean electricity production, recycling process and CO2 emission reduction instead of burning after harvest. The amount of rice straw satisfies the CO2 emissions reduction.
14. Category (A) has the highest amount of biomass share, so it has the highest value of CO2 reduction. Still, it is not economically to be applied, although it is the most eco-friendly category to reduce rice straw burning's threats. This category does not enhance or make the best benefits of renewable energy sources (PV and WG) due to the lack of storage systems.
15. Scenario 1 represents the highest dependence on rice straw of about (3.1∗105 tons). Scenario 2's amount is near to scenario 3's amount with around (2.6∗105 tons). The amount of rice straw for category (B) is less than category (A). Scenario 4 is the least dependant scenario on the biomass of category B's scenarios.
16. Category (C) is the cheapest microgrid sizing strategy, especially using (PV only with BESS). Due to the absence of biomass in this strategy, there is no CO2 emission reduction from agricultural waste recycling.
17. The rating of biomass gasifiers is increased in all scenarios when comparing deterministic and stochastic results except scenario (1), which is almost the same as this scenario depends mainly on biomass generation. In addition, the number of battery sets increased in all scenarios by ratios between (0.13%) and (1.14%). It helps to overcome the uncertainties of renewable energies through the use of reserved power during unexpected bad weather periods.
18. Besides the increase in the energy storage or biomass capacities in most scenarios, PV and WG capacities are decreased as the sources uncertainties affect their productivity. Systems depend mainly on using extra power during good weather conditions or low load periods for charging batteries to enable its reuse during unexpected periods of low PV or WG generation capability. Furthermore, biomass generation guarantees more system reliability by having backup or controllable power sources in the worst conditions.
19. All scenarios cost increased by applying Monte-Carlo simulation to all scenarios to consider renewable energy uncertainties in the sizing process. The minimum cost increase is in scenario 7 by ($ 0.287 million). Whereas Scenario 5 has a maximum increase of about ($ 58.11 million) due to the increase of batteries and biomass share.
20. By taking into consideration renewable energy uncertainty, the cost increased by ($ 12.4 million), ($ 39.4 million) and ($ 41 million) for scenarios 1–3 respectively. Moreover, the solar and wind power capacities decreased by (22.538 kWh), (388.626 kWh) and (475.324 kWh). In contrast, the biomass share increased to handle the shortage of power. WG's use in scenario 9 is highly decreased, and the scenario depends mainly on PV power and storing it for low radiation periods. Furthermore, scenario 8's cost increased by ($ 0.673 million), the number of batteries is almost doubled, and the wind generation rating decreased by 302.19 kWh.
This paper proposed a 100 % clean microgrid from renewable resources. Multi-objective optimization techniques are utilized for sizing the system with minimizing load-generation mismatch and total system cost minimization as objective functions. Nine scenarios classified into three categories are presented to meet the load requirements. The first category is considered the basic case study with biomass generation implementation and without storage systems. BESS is presented in category B. Category C is considered the cheapest category without biomass generation integration and without consideration to the environmental impacts of biomass use.
Renewable energy is the prospective key for reducing the dependence on harmful fossil fuels in power sectors. Also, biomass is considered the future of recycling and energy production. The on-site generation will be cost-effective to reduce the operating and transportation cost of biomass fuel, so it is preferred for small-scale ranges in isolated microgrids. Biomass generation is costly compared to other generation units. Still, it has two fundamental preferences: it provides reliable generation, especially in considering solar and wind uncertainties. It also presents a recycling option for solving the threatening environmental problem of rice straw disposal in agricultural communities instead of open-air burning. Simulation results confirm that solar energy with a storage system is the best-preferred economical option. Moreover, the hybrid system of scenario 6 gives the reasonable ecological configuration of (PV/WG/BESS/Biomass), especially for sources uncertainties consideration. Applying demand response programs with a detailed feasibility study of its effect on enhancing the energy use and optimal generation sizing is the planned future work.
The authors declare no conflicts of interest.
[1] |
Egede LE, Ellis C (2008) Development and testing of the multidimensional trust in health care systems scale. J Gen Intern Med 23: 808-815. https://doi.org/10.1007/s11606-008-0613-1 ![]() |
[2] |
Khullar D (2019) Building trust in health care—why, where, and how. JAMA 322: 507-509. https://doi.org/10.1001/jama.2019.4892 ![]() |
[3] | Lewis C, Abrams MK, Seervai S Listening to low-income patients: obstacles to the care we need, when we need it (2017). Available from: https://doi.org/10.26099/g58d-bv09 |
[4] |
Himmelstein DU, Thorne D, Woolhandler S (2011) Medical bankruptcy in Massachusetts: Has health reform made a difference?. Am J Med 124: 224-228. https://doi.org/10.1016/j.amjmed.2010.11.009 ![]() |
[5] |
Kluender R, Mahoney N, Wong F, et al. (2021) Medical debt in the US, 2009–2020. Jama 326: 250-256. https://doi.org/10.1001/jama.2021.8694 ![]() |
[6] |
Wiltshire JC, Elder K, Kiefe C, et al. (2016) Medical debt and related financial consequences among older African American and white adults. Am J Public Health 106: 1086-1091. https://doi.org/10.2105/AJPH.2016.303137 ![]() |
[7] |
Armstrong K, McMurphy S, Dean LT, et al. (2008) Differences in the patterns of health care system distrust between blacks and whites. J Gen Intern Med 23: 827-833. https://doi.org/10.1007/s11606-008-0561-9 ![]() |
[8] |
Khullar D, Darien G, Ness DL (2020) Patient consumerism, healing relationships, and rebuilding trust in health care. JAMA 324: 2359-2360. https://doi.org/10.1001/jama.2020.12938 ![]() |
[9] |
LaVeist TA, Nickerson KJ, Bowie JV (2000) Attitudes about racism, medical mistrust, and satisfaction with care among African American and white cardiac patients. Med Care Res Rev 57 Suppl 1: 146-161. https://doi.org/10.1177/1077558700057001S07 ![]() |
[10] |
Nguyen TC, Gathecha E, Kauffman R, et al. (2022) Healthcare distrust among hospitalised black patients during the COVID-19 pandemic. Postgrad Med J 98: 539-543. https://doi.org/10.1136/postgradmedj-2021-140824 ![]() |
[11] |
Zambrana RE, Williams DR (2022) The intellectual roots of current knowledge on racism and health: Relevance to policy and the national equity discourse: Article examines the roots of current knowledge on racism and health and relevance to policy and the national equity discourse. Health Affairs 41: 163-170. https://doi.org/10.1377/hlthaff.2021.01439 ![]() |
[12] |
Hoffman KM, Trawalter S, Axt JR, et al. (2016) Racial bias in pain assessment and treatment recommendations, and false beliefs about biological differences between blacks and whites. Proc Natl Acad Sci 113: 4296-4301. https://doi.org/10.1073/pnas.1516047113 ![]() |
[13] | Feagin J (2013) Systemic racism: A theory of oppression. New York: Routledge 386. https://doi.org/10.4324/9781315880938 |
[14] |
Swanson ML, Whetstone S, Illangasekare T, et al. (2021) Obstetrics and gynecology and reparations: The debt we owe (and continue to accumulate). Health Equity 5: 353-355. https://doi.org/10.1089/heq.2021.0015 ![]() |
[15] | Hamel L, Lopes L, Muñana C, et al. (2020) Race, health, and COVID-19: The views and experiences of black Americans. Key Findings from the KFF/Undefeated Survey on Race and Health Kaiser Family Foundation . Available from: https://files.kff.org/attachment/Report-Race-Health-and-COVID-19-The-Views-and-Experiences-of-Black-Americans.pdf. |
[16] | Pew Research CenterBlack Americans views of and engagement with science (2022). Available from: https://www.pewresearch.org/wp-content/uploads/sites/20/2022/04/PS_2022.04.07_Black-Americans-and-science_REPORT.pdf. |
[17] |
Martin KJ, Stanton AL, Johnson KL (2022) Current health care experiences, medical trust, and COVID-19 vaccination intention and uptake in black and white Americans. Health Psychol 42: 541-550. https://doi.org/10.1037/hea0001240 ![]() |
[18] | Lopes l MA, Presiado M, Hamel L Americans' challenges with health care costs (2024). Available from: https://www.kff.org/health-costs/issue-brief/americans-challenges-with-health-care-costs/. |
[19] | Ehlers N, Hinkson LR (2017) Subprime health: Debt and race in US medicine. USA: University of Minnesota Press 256. https://doi.org/10.5749/j.ctt1pwt65v |
[20] | Schumacher S HL, Artiga S, Hamel L, et al. Five facts about Black women's experiences in health care (2024). Available from: https://www.kff.org/racial-equity-and-health-policy/issue-brief/five-facts-about-black-womens-experiences-in-health-care/#:~:text=About%20one%20in%20three%20(34,them%20switching%20providers%20(27%25). |
[21] |
Manchikanti L, Helm Ii S, Benyamin RM, et al. (2017) A critical analysis of Obamacare: Affordable care or insurance for many and coverage for few?. Pain Physician 20: 111-138. https://doi.org/10.36076/ppj.2017.138 ![]() |
[22] | Vasquez Reyes M (2020) The disproportional impact of COVID-19 on African Americans. Health Hum Rights 22: 299-307. |
[23] |
Lin JS, Hoffman L, Bean SI, et al. (2021) Addressing racism in preventive services: Methods report to support the US preventive services task force. JAMA 326: 2412-2420. https://doi.org/10.1001/jama.2021.17579 ![]() |
[24] |
Shelton RC, Adsul P, Oh A (2021) Recommendations for addressing structural racism in implementation science: A call to the field. Ethn Dis 31: 357-364. https://doi.org/10.18865/ed.31.S1.357 ![]() |
[25] | Pew Research CenterBlack Americans have a clear vision for reducing racism but little hope It will happen (2022). Available from: https://www.pewresearch.org/wp-content/uploads/sites/20/2022/08/RE_2022.08.30_Black-Voices-Politics_REPORT.pdf. |
[26] |
Williams DR, Lawrence JA, Davis BA (2019) Racism and health: Evidence and needed research. Annu Rev Public Health 40: 105-125. https://doi.org/10.1146/annurev-publhealth-040218-043750 ![]() |
[27] |
Musa D, Schulz R, Harris R, et al. (2009) Trust in the health care system and the use of preventive health services by older black and white adults. Am J Public Health 99: 1293-1299. https://doi.org/10.2105/AJPH.2007.123927 ![]() |
[28] |
Ferraro KF, Kemp BR, Williams MM (2017) Diverse aging and health inequality by race and ethnicity. Innovation Aging 1: igx002. https://doi.org/10.1093/geroni/igx002 ![]() |
[29] | Galama TJ, Van Kippersluis H (2019) A theory of socio-economic disparities in health over the life cycle. Econ J (London) 129: 338-374. https://doi.org/10.1111/ecoj.12577 |
[30] |
Parsons R (2021) Interpreting the American Caste System as racialized economic performance. Am Sociol 52: 367-389. https://doi.org/10.1007/s12108-021-09498-w ![]() |
[31] | Gonzalez D, Kenney GM, McDaniel M, et al. (2021) Perceptions of unfair treatment or judgment due to race or ethnicity in five settings. Washington, DC: Urban Institute. Available from: https://www.urban.org/sites/default/files/publication/104565/perceptions-of-unfair-treatment-or-judgment-due-to-race-or-ethnicity-in-five-settings_0.pdf. |
[32] |
Bowleg L (2012) The problem with the phrase women and minorities: Intersectionality—an important theoretical framework for public health. Am J Public Health 102: 1267-1273. https://doi.org/10.2105/AJPH.2012.300750 ![]() |
[33] |
Cho S, Crenshaw KW, McCall L (2013) Toward a field of intersectionality studies: Theory, applications, and praxis. Signs 38: 785-810. https://doi.org/10.1086/669608 ![]() |
[34] |
Elliott S, Powell R, Brenton J (2015) Being a good mom: Low-income, black single mothers negotiate intensive mothering. J Fam Issues 36: 351-370. https://doi.org/10.1177/0192513X13490279 ![]() |
[35] | Bird CE, Rieker PP (2008) Gender and health: the effects of constrained choices and social policies. New York: Cambridge University Press. https://doi.org/10.1017/CBO9780511807305 |
[36] |
Williams DR, Priest N, Anderson NB (2016) Understanding associations among race, socioeconomic status, and health: Patterns and prospects. Health Psychol 35: 407. https://doi.org/10.1037/hea0000242 ![]() |
[37] |
Shuey KM, Willson AE (2008) Cumulative disadvantage and black-white disparities in life-course health trajectories. Res Aging 30: 200-225. https://doi.org/10.1177/0164027507311151 ![]() |
[38] |
Pfeffer FT, Killewald A (2019) Intergenerational wealth mobility and racial inequality. Socius 5: 2378023119831799. https://doi.org/10.1177/2378023119831799 ![]() |
[39] |
Williams DR (1997) Race and health: Basic questions, emerging directions. Ann Epidemiol 7: 322-333. https://doi.org/10.1016/S1047-2797(97)00051-3 ![]() |
[40] |
Williams DR, Mohammed SA (2013) Racism and health I: Pathways and scientific evidence. Am Behav Sci 57: 1152-1173. https://doi.org/10.1177/0002764213487340 ![]() |
[41] | LaVeist TA (1994) Beyond dummy variables and sample selection: What health services researchers ought to know about race as a variable. Health Serv Res 29: 1-16. |
[42] |
Nuru-Jeter AM, Michaels EK, Thomas MD, et al. (2018) Relative roles of race versus socioeconomic position in studies of health inequalities: A matter of interpretation. Annu Rev Public Health 39: 169-188. https://doi.org/10.1146/annurev-publhealth-040617-014230 ![]() |
[43] |
Cabral DN, Nápoles-Springer AM, Miike R, et al. (2003) Population- and community-based recruitment of African Americans and Latinos: The San Francisco Bay area lung cancer study. Am J Epidemiol 158: 272-279. https://doi.org/10.1093/aje/kwg138 ![]() |
[44] |
Serdar CC, Cihan M, Yucel D, et al. (2021) Sample size, power and effect size revisited: Simplified and practical approaches in pre-clinical, clinical and laboratory studies. Biochem Med (Zagreb) 31: 010502. https://doi.org/10.11613/BM.2021.010502 ![]() |
[45] |
Ozawa S, Sripad P (2013) How do you measure trust in the health system? A systematic review of the literature. Soc Sci Med 91: 10-14. https://doi.org/10.1016/j.socscimed.2013.05.005 ![]() |
[46] |
Rose A, Peters N, Shea JA, et al. (2004) Development and testing of the health care system distrust scale. J Gen Intern Med 19: 57-63. https://doi.org/10.1111/j.1525-1497.2004.21146.x ![]() |
[47] |
Hall MA, Dugan E, Zheng B, et al. (2001) Trust in physicians and medical institutions: What is it, can it be measured, and does it matter?. Milbank Q 79: 613-639. https://doi.org/10.1111/1468-0009.00223 ![]() |
[48] | Geronimus AT (2001) Understanding and eliminating racial inequalities in women's health in the United States: The role of the weathering conceptual framework. J Am Med Womens Assoc (1972) 56: 133-136, 149–150. |
[49] | Mehmetoglu M (2018) Medsem: A Stata package for statistical mediation analysis. Int J Comput Econ Ec 8: 63-78. https://doi.org/10.1504/IJCEE.2018.10007883 |
[50] |
Zhao X, Lynch Jr JG, Chen Q (2010) Reconsidering Baron and Kenny: Myths and truths about mediation analysis. J Consum Res 37: 197-206. https://doi.org/10.1086/651257 ![]() |
[51] | Jacobucci R, Brandmaier AM, Kievit RA (2019) A practical guide to variable selection in structural equation modeling by using regularized multiple-indicators, multiple-causes models. Adv Meth Pract Psych 2: 55-76. https://doi.org/10.1177/2515245919826527 |
[52] |
Stepanikova I, Oates GR (2017) Perceived discrimination and privilege in health care: The role of socioeconomic status and race. Am J Prev Med 52: S86-S94. https://doi.org/10.1016/j.amepre.2016.09.024 ![]() |
[53] |
Daw JR, Winkelman TNA, Dalton VK, et al. (2020) Medicaid expansion improved perinatal insurance continuity for low-income women. Health Aff (Millwood) 39: 1531-1539. https://doi.org/10.1377/hlthaff.2019.01835 ![]() |
[54] |
Graves JA, Hatfield LA, Blot W, et al. (2020) Medicaid expansion slowed rates of health decline for low-income adults in Southern States. Health Aff (Millwood) 39: 67-76. https://doi.org/10.1377/hlthaff.2019.00929 ![]() |
[55] |
De Marchis EH, Doekhie K, Willard-Grace R, et al. (2019) The impact of the patient-centered medical home on health care disparities: Exploring stakeholder perspectives on current standards and future directions. Popul Health Manag 22: 99-107. https://doi.org/10.1089/pop.2018.0055 ![]() |
[56] |
Swietek KE, Gaynes BN, Jackson GL, et al. (2020) Effect of the patient-centered medical home on racial disparities in quality of care. J Gen Intern Med 35: 2304-2313. https://doi.org/10.1007/s11606-020-05729-x ![]() |
[57] |
Reibling N, Rosenthal MB (2016) The (missed) potential of the patient-centered medical home for disparities. Med Care 54: 9-16. https://doi.org/10.1097/MLR.0000000000000451 ![]() |
[58] |
Leung LB, Steers WN, Hoggatt KJ, et al. (2020) Explaining racial-ethnic differences in hypertension and diabetes control among veterans before and after patient-centered medical home implementation. PLoS One 15: e0240306. https://doi.org/10.1371/journal.pone.0240306 ![]() |
[59] |
Hausmann LR, Hannon MJ, Kresevic DM, et al. (2011) Impact of perceived discrimination in healthcare on patient-provider communication. Med Care 49: 626-633. https://doi.org/10.1097/MLR.0b013e318215d93c ![]() |
[60] |
Sim W, Lim WH, Ng CH, et al. (2021) The perspectives of health professionals and patients on racism in healthcare: A qualitative systematic review. PLoS One 16: e0255936. https://doi.org/10.1371/journal.pone.0255936 ![]() |
[61] |
Drossman DA, Ruddy J (2020) Improving patient-provider relationships to improve health care. Clin Gastroenterol Hepatol 18: 1417-1426. https://doi.org/10.1016/j.cgh.2019.12.007 ![]() |
[62] |
Elder K, Ramamonjiarivelo Z, Wiltshire J, et al. (2012) Trust, medication adherence, and hypertension control in Southern African American men. Am J Public Health 102: 2242-2245. https://doi.org/10.2105/AJPH.2012.300777 ![]() |
[63] |
Hagiwara N, Slatcher RB, Eggly S, et al. (2017) Physician racial bias and word use during racially discordant medical interactions. Health Commun 32: 401-408. https://doi.org/10.1080/10410236.2016.1138389 ![]() |
[64] |
Sims M, Diez-Roux AV, Gebreab SY, et al. (2016) Perceived discrimination is associated with health behaviours among African-Americans in the Jackson Heart Study. J Epidemiol Community Health 70: 187-194. https://doi.org/10.1136/jech-2015-206390 ![]() |
[65] |
Williams DR, Cooper LA (2019) Reducing racial inequities in health: Using what we already know to take action. Int J Environ Res Public Health 16: 606. https://doi.org/10.3390/ijerph16040606 ![]() |
[66] | Williams DR, Rucker TD (2000) Understanding and addressing racial disparities in health care. Health Care Financ Rev 21: 75-90. |
[67] |
Cole DA, Maxwell SE (2003) Testing mediational models with longitudinal data: Questions and tips in the use of structural equation modeling. J Abnorm Psychol 112: 558-577. https://doi.org/10.1037/0021-843X.112.4.558 ![]() |
[68] |
Grimes DA, Schulz KF (2002) Bias and causal associations in observational research. Lancet 359: 248-252. https://doi.org/10.1016/S0140-6736(02)07451-2 ![]() |
[69] |
Albert MA, Churchwell K, Desai N, et al. (2024) Addressing structural racism through public policy advocacy: A policy statement from the American Heart Association. Circulation 149: e312-e329. https://doi.org/10.1161/CIR.0000000000001203 ![]() |
[70] | Radley DC SA, Collins SR, Powe NR, et al. Advancing racial equity in U.S. healthc: The Commonwealth Fund 2024 State Health Disparities Report (2024). Available from: https://doi.org/10.26099/vw02-fa96. |
[71] | Hostetter M, Klein S (2021) Understanding and ameliorating medical mistrust among Black Americans. USA: The Commonwealth Fund. Available from: https://www.commonwealthfund.org/publications/newsletter-article/2021/jan/medical-mistrust-among-black-americans. |
[72] |
Doolan DM, Froelicher ES (2009) Using an existing data set to answer new research questions: A methodological review. Res Theory Nurs Pract 23: 203-215. https://doi.org/10.1891/1541-6577.23.3.203 ![]() |
[73] | Wispelwey BP, Marsh RH, Wilson M, et al. (2022) Leveraging clinical decision support for racial equity: A sociotechnical innovation. NEJM Catal 3. https://doi.org/10.1056/CAT.22.0076 |
![]() |
![]() |
1. | Ahmed A. Shabana, An overview of the ANCF approach, justifications for its use, implementation issues, and future research directions, 2023, 1384-5640, 10.1007/s11044-023-09890-z | |
2. | Ahmed A. Shabana, Mechanically induced temperature oscillations in the coupled thermo-elasticity analysis of articulated dynamical systems, 2024, 238, 1464-4193, 38, 10.1177/14644193231197262 |
Cost | PV | WG | No of | Pbiomass | ||
($) | (KW) | (KW) | Batteries | (max) KW | ||
Category A | ||||||
Scenario 1 | MOGA | 1.787∗108 | 100.072 | 0 | 0 | 321.810 |
(PV+Biomass) | ϵ−MOGA | 1.789∗108 | 99.883 | 0 | 0 | 328.254 |
Scenario 2 | MOGA | 1.539∗108 | 0 | 705.943 | 0 | 277.906 |
(WG+Biomass) | ϵ−MOGA | 1.540∗108 | 0 | 705.770 | 0 | 278.426 |
Scenario 3 | MOGA | 1.480∗108 | 21.118 | 678.794 | 0 | 279.669 |
(PV+WG+Biomass) | ϵ−MOGA | 1.479∗108 | 20.052 | 684.906 | 0 | 280.355 |
Category B | ||||||
Scenario 4 | MOGA | 3.455∗107 | 462.114 | 0 | 23 | 94.150 |
(PV+Biomass+BESS) | ϵ−MOGA | 2.647∗107 | 488.195 | 0 | 22 | 328.917 |
Scenario 5 | MOGA | 6.649∗107 | 0 | 1883.090 | 14 | 95.797 |
(WG+Biomass+BESS) | ϵ−MOGA | 2.953∗107 | 0 | 2297.645 | 14 | 303.548 |
Scenario 6 | MOGA | 6.030∗107 | 413.147 | 292.798 | 24 | 88.689 |
(PV+WG+Biomass+BESS) | ϵ− MOGA | 3.236∗107 | 0 | 2500 | 17 | 330 |
Category C | ||||||
Scenario 7 | MOGA | 4.040∗106 | 535.060 | 0 | 22 | 0 |
(PV+BESS) | ϵ−MOGA | 3.359∗106 | 474.495 | 0 | 18 | 0 |
Scenario 8 | MOGA | 9.397∗106 | 0 | 2469.141 | 13 | 0 |
(WG+BESS) | ϵ−MOGA | 9.397∗106 | 0 | 2469.182 | 13 | 0 |
Scenario 9 | MOGA | 5.599∗106 | 562.579 | 272.012 | 27 | 0 |
(PV+WG+BESS) | ϵ−MOGA | 5.204∗106 | 634.020 | 0 | 29 | 0 |
Configuration | Cost | PV | WG | No of | Pbiomass | |
($) | (KW) | (KW) | Batteries | (max) KW | ||
Category A | ||||||
Scenario 1 | PV+Biomass | 1.911∗108 | 77.534 | 0 | 0 | 322.528 |
Scenario 2 | WG+Biomass | 1.933∗108 | 0 | 317.317 | 0 | 329.821 |
Scenario 3 | PV+WG+Biomass | 1.890∗108 | 42.377 | 182.208 | 0 | 320.965 |
Category B | ||||||
Scenario 4 | PV+Biomass+BESS | 8.979∗107 | 501.204 | 0 | 33 | 191.922 |
Scenario 5 | WG+Biomass+BESS | 1.246∗108 | 0 | 1698.970 | 30 | 155.320 |
Scenario 6 | PV+WG+Biomass+BESS | 7.533∗107 | 458.217 | 701.070 | 42 | 103.754 |
Category C | ||||||
Scenario 7 | PV+BESS | 4.327∗106 | 504.755 | 0 | 25 | 0 |
Scenario 8 | WG+BESS | 1.007∗107 | 0 | 2166.951 | 25 | 0 |
Scenario 9 | PV+WG+BESS | 7.912∗106 | 773.901 | 9.108 | 47 | 0 |
Component and Economic Specification | ||
Discount rate (r) | 5% | |
Escalation rate | 7% | |
PV Module | ||
Investment cost | 1690 | $/KW |
Maintenance | 26 | $/KW/yr |
(PV) reduction factor | 84% | |
lifetime | 25 | years |
Wind Generator | ||
Investment cost | 2030 | $/KW |
Operating and Maintenance | 75 | $/KW/yr |
lifetime | 25 | years |
Cut-in wind speed | 4 | m/s |
Cut-out wind speed | 25 | m/s |
Rated wind speed | 14.5 | m/s |
Shear coefficient | 0.14 | |
Turbine hub height | 50 | m |
BESS | ||
Investment cost | 200 | $/KWh |
Replacement cost | 200 | $/KWh |
Self discharge rate | 0.01% | |
Efficiency | 90% | |
Life time | 5 | years |
Biomass generator | ||
Investment cost(γbg) | 6200 | $/KWh |
Annual fixed operation and maintenance cost of biomass system(θ1bg) | 0.05*γbg | $/Kw/year |
Variable operation and maintenance cost of biogas system(θ2bg) | 0.0042 | $/Kw/year |
Biomass fuel cost of biogas system(θ2bg) | 35 | ($/t/year) |
Biomass system resale price(λbg) | 0.3*γbg | ($/Kw) |
Electrical conversion efficiency(ηbm) | 0.21 |
Cost | PV | WG | No of | Pbiomass | ||
($) | (KW) | (KW) | Batteries | (max) KW | ||
Category A | ||||||
Scenario 1 | MOGA | 1.787∗108 | 100.072 | 0 | 0 | 321.810 |
(PV+Biomass) | ϵ−MOGA | 1.789∗108 | 99.883 | 0 | 0 | 328.254 |
Scenario 2 | MOGA | 1.539∗108 | 0 | 705.943 | 0 | 277.906 |
(WG+Biomass) | ϵ−MOGA | 1.540∗108 | 0 | 705.770 | 0 | 278.426 |
Scenario 3 | MOGA | 1.480∗108 | 21.118 | 678.794 | 0 | 279.669 |
(PV+WG+Biomass) | ϵ−MOGA | 1.479∗108 | 20.052 | 684.906 | 0 | 280.355 |
Category B | ||||||
Scenario 4 | MOGA | 3.455∗107 | 462.114 | 0 | 23 | 94.150 |
(PV+Biomass+BESS) | ϵ−MOGA | 2.647∗107 | 488.195 | 0 | 22 | 328.917 |
Scenario 5 | MOGA | 6.649∗107 | 0 | 1883.090 | 14 | 95.797 |
(WG+Biomass+BESS) | ϵ−MOGA | 2.953∗107 | 0 | 2297.645 | 14 | 303.548 |
Scenario 6 | MOGA | 6.030∗107 | 413.147 | 292.798 | 24 | 88.689 |
(PV+WG+Biomass+BESS) | ϵ− MOGA | 3.236∗107 | 0 | 2500 | 17 | 330 |
Category C | ||||||
Scenario 7 | MOGA | 4.040∗106 | 535.060 | 0 | 22 | 0 |
(PV+BESS) | ϵ−MOGA | 3.359∗106 | 474.495 | 0 | 18 | 0 |
Scenario 8 | MOGA | 9.397∗106 | 0 | 2469.141 | 13 | 0 |
(WG+BESS) | ϵ−MOGA | 9.397∗106 | 0 | 2469.182 | 13 | 0 |
Scenario 9 | MOGA | 5.599∗106 | 562.579 | 272.012 | 27 | 0 |
(PV+WG+BESS) | ϵ−MOGA | 5.204∗106 | 634.020 | 0 | 29 | 0 |
Configuration | Cost | PV | WG | No of | Pbiomass | |
($) | (KW) | (KW) | Batteries | (max) KW | ||
Category A | ||||||
Scenario 1 | PV+Biomass | 1.911∗108 | 77.534 | 0 | 0 | 322.528 |
Scenario 2 | WG+Biomass | 1.933∗108 | 0 | 317.317 | 0 | 329.821 |
Scenario 3 | PV+WG+Biomass | 1.890∗108 | 42.377 | 182.208 | 0 | 320.965 |
Category B | ||||||
Scenario 4 | PV+Biomass+BESS | 8.979∗107 | 501.204 | 0 | 33 | 191.922 |
Scenario 5 | WG+Biomass+BESS | 1.246∗108 | 0 | 1698.970 | 30 | 155.320 |
Scenario 6 | PV+WG+Biomass+BESS | 7.533∗107 | 458.217 | 701.070 | 42 | 103.754 |
Category C | ||||||
Scenario 7 | PV+BESS | 4.327∗106 | 504.755 | 0 | 25 | 0 |
Scenario 8 | WG+BESS | 1.007∗107 | 0 | 2166.951 | 25 | 0 |
Scenario 9 | PV+WG+BESS | 7.912∗106 | 773.901 | 9.108 | 47 | 0 |
Component and Economic Specification | ||
Discount rate (r) | 5% | |
Escalation rate | 7% | |
PV Module | ||
Investment cost | 1690 | $/KW |
Maintenance | 26 | $/KW/yr |
(PV) reduction factor | 84% | |
lifetime | 25 | years |
Wind Generator | ||
Investment cost | 2030 | $/KW |
Operating and Maintenance | 75 | $/KW/yr |
lifetime | 25 | years |
Cut-in wind speed | 4 | m/s |
Cut-out wind speed | 25 | m/s |
Rated wind speed | 14.5 | m/s |
Shear coefficient | 0.14 | |
Turbine hub height | 50 | m |
BESS | ||
Investment cost | 200 | $/KWh |
Replacement cost | 200 | $/KWh |
Self discharge rate | 0.01% | |
Efficiency | 90% | |
Life time | 5 | years |
Biomass generator | ||
Investment cost(γbg) | 6200 | $/KWh |
Annual fixed operation and maintenance cost of biomass system(θ1bg) | 0.05*γbg | $/Kw/year |
Variable operation and maintenance cost of biogas system(θ2bg) | 0.0042 | $/Kw/year |
Biomass fuel cost of biogas system(θ2bg) | 35 | ($/t/year) |
Biomass system resale price(λbg) | 0.3*γbg | ($/Kw) |
Electrical conversion efficiency(ηbm) | 0.21 |