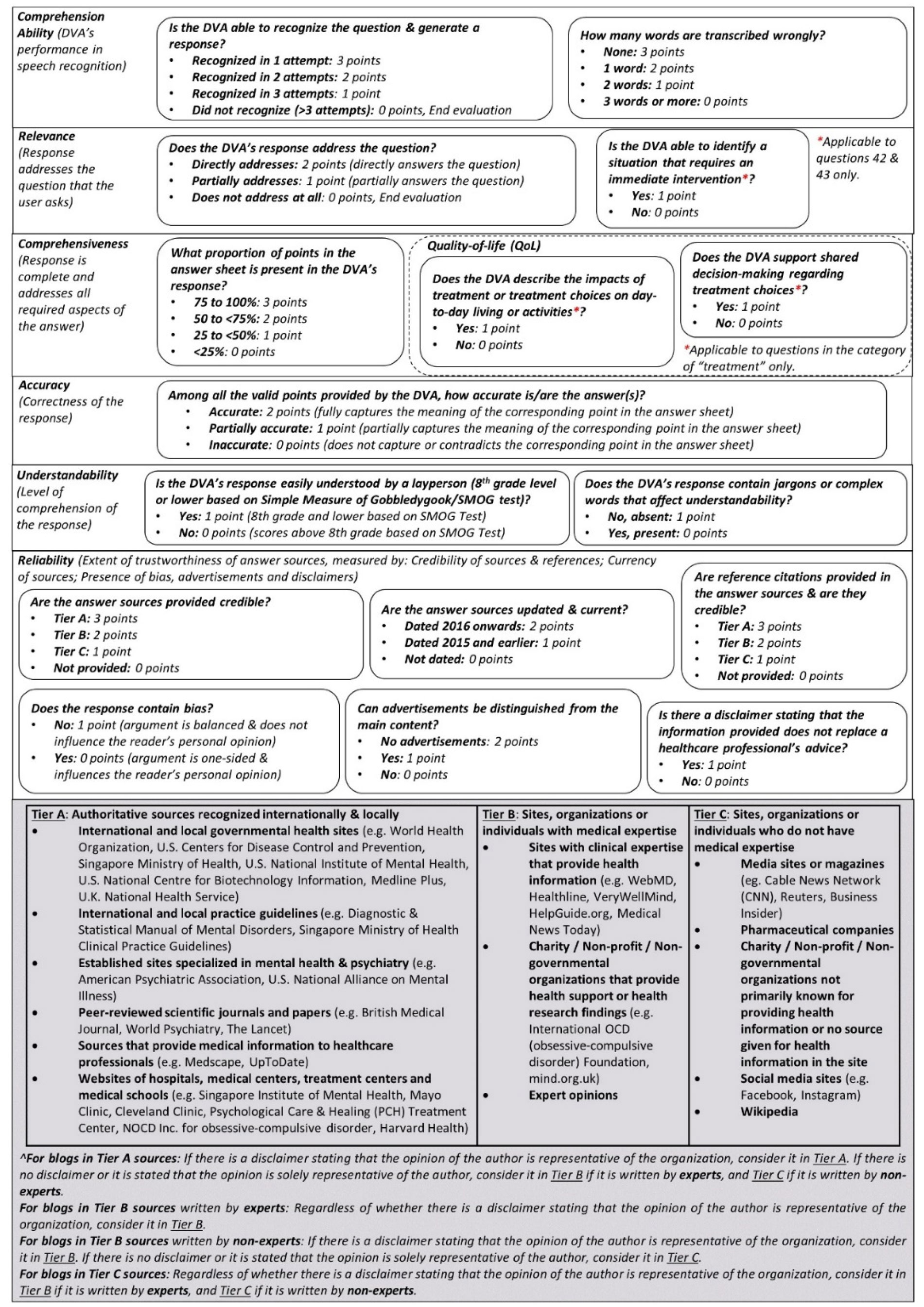
Malaria is a serious health problem in Africa, and the ongoing COVID-19 pandemic has affected the implementation of key malaria control interventions. This jeopardizes the gains made in malaria. As a result, a new co-infection model of COVID-19 and malaria is constructed, and the role of vaccination in COVID-19-malaria co-infection is analyzed. The existence and stability of the equilibria of each single infection are first studied by their respective basic reproduction numbers. When the basic reproduction numbers RC0 and RM0 are both below unity, the COVID-19-malaria-free equilibrium is locally asymptotically stable. Sensitivity analysis reveals that the main parameters affecting the spread of diseases are their respective disease transmission rate and vaccine efficacy. Further, we introduce the effect of vaccination rate and efficacy on controlling the co-infected population. It also shows that under the condition of a low recovery rate caused by the shortage of medical resources, improving the vaccination rate and effectiveness of vaccines has a positive impact on suppressing diseases. The model is then extended into an optimal control system by introducing prevention and treatment measures for COVID-19 and malaria. The results suggest that applying each strategy alone can reduce the scale of co-infection, but strategy A increases the number of malaria cases and strategy B prolongs the period of COVID-19 infection. Measures to control COVID-19 must be combined with efforts to ensure malaria control is maintained.
Citation: Yaxin Ren, Yakui Xue. Modeling and optimal control of COVID-19 and malaria co-infection based on vaccination[J]. Mathematical Modelling and Control, 2024, 4(3): 316-335. doi: 10.3934/mmc.2024026
[1] | Hongyong Zhao, Qianjin Zhang, Linhe Zhu . The spatial dynamics of a zebrafish model with cross-diffusions. Mathematical Biosciences and Engineering, 2017, 14(4): 1035-1054. doi: 10.3934/mbe.2017054 |
[2] | Xiaomei Bao, Canrong Tian . Turing patterns in a networked vegetation model. Mathematical Biosciences and Engineering, 2024, 21(11): 7601-7620. doi: 10.3934/mbe.2024334 |
[3] | Nazanin Zaker, Christina A. Cobbold, Frithjof Lutscher . The effect of landscape fragmentation on Turing-pattern formation. Mathematical Biosciences and Engineering, 2022, 19(3): 2506-2537. doi: 10.3934/mbe.2022116 |
[4] | Jichun Li, Gaihui Guo, Hailong Yuan . Nonlocal delay gives rise to vegetation patterns in a vegetation-sand model. Mathematical Biosciences and Engineering, 2024, 21(3): 4521-4553. doi: 10.3934/mbe.2024200 |
[5] | Tingting Ma, Xinzhu Meng . Global analysis and Hopf-bifurcation in a cross-diffusion prey-predator system with fear effect and predator cannibalism. Mathematical Biosciences and Engineering, 2022, 19(6): 6040-6071. doi: 10.3934/mbe.2022282 |
[6] | Mingzhu Qu, Chunrui Zhang, Xingjian Wang . Analysis of dynamic properties on forest restoration-population pressure model. Mathematical Biosciences and Engineering, 2020, 17(4): 3567-3581. doi: 10.3934/mbe.2020201 |
[7] | Maya Mincheva, Gheorghe Craciun . Graph-theoretic conditions for zero-eigenvalue Turing instability in general chemical reaction networks. Mathematical Biosciences and Engineering, 2013, 10(4): 1207-1226. doi: 10.3934/mbe.2013.10.1207 |
[8] | Swadesh Pal, Malay Banerjee, Vitaly Volpert . Spatio-temporal Bazykin’s model with space-time nonlocality. Mathematical Biosciences and Engineering, 2020, 17(5): 4801-4824. doi: 10.3934/mbe.2020262 |
[9] | Yue Xing, Weihua Jiang, Xun Cao . Multi-stable and spatiotemporal staggered patterns in a predator-prey model with predator-taxis and delay. Mathematical Biosciences and Engineering, 2023, 20(10): 18413-18444. doi: 10.3934/mbe.2023818 |
[10] | Yongli Cai, Malay Banerjee, Yun Kang, Weiming Wang . Spatiotemporal complexity in a predator--prey model with weak Allee effects. Mathematical Biosciences and Engineering, 2014, 11(6): 1247-1274. doi: 10.3934/mbe.2014.11.1247 |
Malaria is a serious health problem in Africa, and the ongoing COVID-19 pandemic has affected the implementation of key malaria control interventions. This jeopardizes the gains made in malaria. As a result, a new co-infection model of COVID-19 and malaria is constructed, and the role of vaccination in COVID-19-malaria co-infection is analyzed. The existence and stability of the equilibria of each single infection are first studied by their respective basic reproduction numbers. When the basic reproduction numbers RC0 and RM0 are both below unity, the COVID-19-malaria-free equilibrium is locally asymptotically stable. Sensitivity analysis reveals that the main parameters affecting the spread of diseases are their respective disease transmission rate and vaccine efficacy. Further, we introduce the effect of vaccination rate and efficacy on controlling the co-infected population. It also shows that under the condition of a low recovery rate caused by the shortage of medical resources, improving the vaccination rate and effectiveness of vaccines has a positive impact on suppressing diseases. The model is then extended into an optimal control system by introducing prevention and treatment measures for COVID-19 and malaria. The results suggest that applying each strategy alone can reduce the scale of co-infection, but strategy A increases the number of malaria cases and strategy B prolongs the period of COVID-19 infection. Measures to control COVID-19 must be combined with efforts to ensure malaria control is maintained.
Globally, the number of persons suffering from mental health disorders is on the rise [1]. In 2015, an estimated 322 million people were living with depression worldwide [1]. With the recent COVID-19 pandemic, mental well-being was further challenged with fears of contracting an infection [2] and feelings of isolation [3]. Mental health conditions have been associated with stigma in society, causing an individual to perceive oneself as unacceptable [4],[5]. The impact of stigma often results in a reduced likelihood of seeking treatment [4],[6],[7]. In 2018, a USA survey reported that people suffering from depression were increasingly turning to the Internet for mental health-related support [8]. Among them, 90% had researched mental health information online, while 75% had accessed others' health stories through blogs, podcasts and videos [8]. Thus, it is not uncommon that many tend to opt for online support environments, including support groups and social media channels [5],[8].
In recent years, digital voice assistants (DVAs) have been increasingly adopted as digital health tools with the purpose of providing information regarding health-related queries for various health conditions, including minor ailments [9], postpartum depression [10], vaccinations [11],[12], cancer screening [13] and smoking cessation advice [14]. Smartphone-based DVAs, such as Apple Siri and Google Assistant, have been particularly popular [15]. According to Google, 27% of Internet searches in 2018 came from using the voice search feature on smartphones [16], with this trend posited to grow. The artificial intelligence (AI) component in DVAs enables voice recognition and responses in natural language [10],[17], thereby enabling these DVAs to participate in two-way conversations with users [18]. Given the growing popularity of using DVAs to search for online health information [8], it is crucial that DVAs are able to provide relevant, appropriate and easy-to understand responses to queries by users in relation to mental health literacy, such as symptom recognition, information sources, awareness of causes and risks and an understanding of treatment types [19],[20]. While there are quality assessment tools that evaluate the quality of online health information, such as the Health-on-the-Net Code (HONcode) [21], DISCERN [22] and Quality Evaluation Scoring Tool (QUEST) [23], from our knowledge, there are no existing ones for the purpose of assessing DVAs. On the other hand, studies that have evaluated the quality of information provided by DVAs [9]–[14] have not focused on mental health conditions.
As we move into a post-pandemic world, it is crucial that public mental health should not be ignored [24]. There is a need to evaluate the quality of information provided by DVAs in the mental health domain. Studies have suggested that providing useful and comprehensive online information about mental health conditions in a user-friendly way can help consumers gain a better understanding of the disease, which in turn can help prevent and/or reduce the severity of the mental health disorder [25]. Furthermore, providing high-quality information online on mental health conditions can potentially reduce the stigma and prejudice attached to these disorders [25]. With the increased popularity of consumers performing health information searches through DVAs, it is crucial that DVAs are able to provide high-quality information on mental health conditions through their responses. Our hypothesis is that DVAs are able to provide responses that are relevant, appropriate and easy-to understand in relation to mental health queries. Thus, the primary objective of this study was to evaluate the quality of DVA responses to mental health-related queries by using an in-house-developed quality assessment rubric. In this study, DVAs are defined as inanimate programs enhanced with AI that interact with human users using speech commands. These are different from other technologies such as chatbots [26] or automated telephone-response systems [27],[28].
In this study, the quality of DVAs was defined as the degree of excellence to which a DVA could fulfill the needs of mental health-related queries [29]. This definition was represented by six quality domains: comprehension ability, relevance, comprehensiveness, accuracy, understandability and reliability. The quality domains were adapted from tools evaluating the quality of online health information or sources. The relevance domain was adapted from the DISCERN [22] and CRAAP (currency, relevance, authority, accuracy and purpose) [30],[31] tools. The accuracy and reliability domains were adapted from DISCERN [22], CRAAP [30],[31] and HONcode [21]. In addition, the reliability domain was also adapted from the Ensuring Quality Information for Patients (EQIP) tool [32], LIDA Minervation validation instrument [33], QUEST [23] and Quality Component Scoring System [34]. The comprehensiveness domain was adapted from DISCERN and EQIP [22],[32], and understandability was adapted from EQIP and LIDA [32],[33].
The quality domains evaluated three aspects of DVA quality: the DVAs themselves (comprehension ability), the DVAs' responses (relevance, comprehensiveness, accuracy and understandability) and the answer sources provided by the DVAs (reliability) (Figure 1). The composite score for all domains added up to a maximum of 32 points. All DVA responses were classified into four types: verbal response only, web response only, verbal and web response and no response. “Verbal response only” referred to a short verbal text that directly answered the question without providing a link. Conversely, a “web response only” referred to a link without any verbal explanation provided. A “verbal and web response” consisted of both the aforementioned parts in a single response. If the DVA did not provide any responses, it would be classified as “no response”. Since understandability was evaluated for both the verbal and web responses, in cases where the DVA only provided one type of response, the composite score would be 30 points instead.
The DVA's comprehension ability was assessed based on its ability to accurately recognize and transcribe the question posed to it. Relevance of the DVA's responses was assessed based on whether the response had adequately addressed the question. For two questions, the DVAs were evaluated for their ability to successfully refer to a contact point in cases requiring immediate intervention. Comprehensiveness was assessed based on whether the DVA's response was complete and fulfilled all of the points in the answer sheet. In addition, two quality-of-life (QoL) criteria assessed whether the DVA described impacts of treatment or treatment choices on day-to-day living or activities, and whether it supported shared decision-making regarding treatment choices. Accuracy assessed whether each point in the DVA's response correctly matched the corresponding point in the answer sheet. Understandability was assessed based on whether a layman would easily understand the DVA response according to the Simple Measure of Gobbledygook (SMOG) readability test [35],[36], and whether it contained medical jargon/complex words. Lastly, the reliability of answer sources provided by the DVAs was evaluated based on six criteria: credibility of the sources and reference citations, how current/updated were the sources, presence/absence of bias and advertisements and whether there was a disclaimer stating that the information provided did not replace a healthcare professional's advice. All DVA responses were evaluated regardless of whether they were verbal or web responses.
A total of 66 questions on mental well-being and mental health conditions were compiled and categorized into five categories: general mental health, depression, anxiety, obsessive-compulsive disorder (OCD) and bipolar disorder. These conditions were chosen due to their rising prevalence in global and local data [1],[37]. Besides the section on general mental health, questions in the other sections on the specific mental health conditions were classified into three subcategories: disease state, symptoms and treatment (Appendix 1).
Questions and answers were sourced primarily from the American Psychiatric Association [38], National Institute of Mental Health [39], Medline Plus [40], World Health Organization [41], USA Centers for Disease Control and Prevention [42], Mayo Clinic [43], Cleveland Clinic [44], National Alliance on Mental Illness [45], Anxiety and Depression Association of America [46] and the International Obsessive-Compulsive Disorder Foundation [47]. In addition, questions were also sourced from AnswerThePublic [48] with the following keywords: “mental health”, “depression”, “anxiety”, “OCD” (obsessive-compulsive disorder) and “bipolar disorder”. Answers were also compiled from established clinical guidelines, including the Diagnostic and Statistical Manual of Mental Disorders, 5th edition (DSM-5) [49] and the Singapore Ministry of Health Clinical Practice Guidelines [50]. The questions and answers were reviewed by three reviewers (VC, WLL, KY). Any differences in opinions were resolved through discussions until consensus was reached. Two reviewers (JC and LL) pilot-tested half of the questions to ensure that the evaluation rubric could be applied across different questions. Their feedback was used to refine the rubric for the actual evaluation.
Four smartphone DVAs were employed for evaluation: Apple Siri, Samsung Bixby, Google Assistant and Amazon Alexa. Siri and Google Assistant were accessed by using an iPhone 6 (iOS14.7.1), while Bixby and Alexa were accessed by using a Samsung Galaxy Note 9 (OS10). All questions were posed to the DVAs in English by native English speakers—in the same order and in the exact way that the questions were phrased in Appendix 1. The evaluations and scoring were done independently on the same devices by three evaluators in a quiet room at their homes: VC (female), LSK (male) and AP (female). Each evaluator would ask all 66 questions to one DVA in one sitting. However, they would pose the questions to a different DVA in a separate sitting (i.e., four separate sessions). If the DVA was unable to capture the question and generate a response after three repeated attempts, the evaluation would end and no points would be awarded. Each evaluator completed the evaluation of all four DVAs within a week, after which, the devices were transferred to the next evaluator, who would then evaluate the DVAs on the same devices over the next consecutive week. As such, all evaluations were completed within 3 weeks. The search and internet histories for the individual DVAs were reset before and after each round of evaluation. The location function was turned on as the DVAs were evaluated for their ability to refer to a contact point. If the DVA provided more than one web link, the first web link was taken for evaluation.
Descriptive statistics (numbers and percentages) were employed to report the types of responses, proportion of successful responses and sources cited by the DVAs. The quality scores were calculated for each mental health category (general mental health, depression, anxiety, OCD, bipolar disorder) and question subcategory (disease state, symptoms, treatment), as well as for each quality domain (comprehension ability, relevance, comprehensiveness, accuracy, understandability, reliability, overall quality), by dividing the sum of points awarded for each DVA against the maximum possible number of points in each mental health category, question subcategory and quality domain (Equation 1). This calculation was also performed across all questions to generate a composite quality score. All quality scores were converted to percentages and reported as medians and interquartile ranges (IQRs). All results were taken as averages of the three evaluators.
All statistical analyses were performed at a significance level of 0.05 by using the Statistical Package for Social Sciences (SPSS) software (version 27). Normality tests, including Shapiro-Wilk tests (n < 50) and Kolmogorov-Smirnov tests (n ≥ 50) were conducted before Kruskal-Wallis tests were applied to compare the results across all four DVAs. Post-hoc analyses using Wilcoxon rank sum tests with Bonferroni adjustments were subsequently performed for each possible pairwise comparison among the DVAs. Wilcoxon rank sum testing was also used to compare the understandability of verbal and web responses. Inter-rater reliability was calculated by using the intraclass correlation coefficient (ICC) [51] based on a mean rating of three evaluators, absolute agreement, a two-way mixed-effects model and a 95% confidence interval (95% CI).
The majority of the responses by Siri were web responses (72.7%), while verbal responses formed the major proportion of responses by Alexa (62.1%) (Table 1). The largest proportion of responses from Google Assistant consisted of both verbal and web responses (78.8%). However, Bixby had a comparable distribution of verbal responses only (36.4%) and verbal and web responses (42.4%).
Number of responses (%), N = 66 a |
||||
Apple Siri | Samsung Bixby | Google Assistant | Amazon Alexa | |
Types of responses by DVAs | ||||
Verbal response only b | 6 (9.1) | 24 (36.4) | 1 (1.5) | 41 (62.1) |
Web response only c | 48 (72.7) | 14 (21.2) | 13 (19.7) | 0 (0) |
Verbal and web response d | 11 (16.7) | 28 (42.4) | 32 (78.8) | 24 (36.4) |
No response | 1 (1.5) | 0 (0) | 0 (0) | 1 (1.5) |
Proportion of successful responses | ||||
Questions that were recognized e | 63 (95.5) | 46 (69.7) | 66 (100.0) | 60 (90.9) |
Relevant responses | 47 (71.2) | 38 (57.6) | 66 (100.0) | 44 (66.7) |
Proportion of sources provided in DVA responses | ||||
Tier A | 13 (19.7) | 19 (28.8) | 36 (54.5) | 20 (30.3) |
Tier B | 19 (28.8) | 18 (27.3) | 18 (27.3) | 8 (12.1) |
Tier C | 15 (22.7) | 1 (1.5) | 6 (9.1) | 15 (22.7) |
No sources provided, or sources that could not be evaluated | 19 (28.8) | 28 (42.4) | 6 (9.1) | 23 (34.8) |
Note: a Results were taken from the average of three evaluators. b A short verbal text that directly answered the question without providing a link. c A link was provided in response to the question without a verbal explanation. d Both a verbal explanation and a link were present in the response. e These were questions that were captured on the smartphone screen and induced a response by the DVA. Responses such as “I'm not sure I understood that” were classified as the DVA not recognizing the question.
The proportion of responses that were successfully recognized varied across the DVAs. Responses were deemed to be recognized successfully if the questions were captured on the smartphone screen and a response was provided by the DVA. If the DVA provided a response like “I'm not sure I understood that”, its response would be classified as not being recognized. Similarly, if the DVA provided a response that was relevant to the question, it would be classified as such. For the proportion of questions that were recognized, Google Assistant performed the best (100%), followed by Siri (95.5%), Alexa (90.9%) and Bixby (69.7%). The proportion of relevant responses followed the same trend, with Google Assistant performing the best (100%) and Bixby performing the worst (57.6%).
In terms of the credibility of the sources provided, Google Assistant (54.5%) and Siri (19.7%) had the highest and lowest proportions of Tier A sources, respectively. Over a quarter of the sources by Siri (28.8%), Bixby (27.3%) and Google Assistant (27.3%) were Tier B, while Siri and Alexa had the largest proportions of Tier C sources (22.7% each).
Across all 66 questions (Table 2), Google Assistant had the highest median composite quality score (78.9%) among the DVAs, while Alexa had the lowest median composite score (64.5%). Siri (83.9%), Bixby (87.7%) and Google Assistant (87.4%) scored the best for questions on depression, in contrast to Alexa (72.3%), which scored the best for OCD questions. Alexa scored significantly lower (63.0%, p < 0.001) than all other DVAs for questions on depression, and significantly lower (60.5%) than Bixby (75.9%, p < 0.001) and Google Assistant (76.4%, p = 0.004) for questions on anxiety. On the other hand, Bixby scored significantly lower than all other DVAs for questions on general mental health and OCD (0%, p < 0.001 each). Additionally, Siri scored significantly lower than Google Assistant for questions on OCD (61.7% versus 78.4%, p = 0.002).
Among the question subcategories, Siri (71.7%) and Google Assistant (80.5%) scored the best for questions on disease state, as compared to questions on symptoms and treatment (Table 2). On the other hand, Bixby had similar scores across all three subcategories of disease state, symptoms and treatment. In contrast, Alexa scored the highest for questions on symptoms (71.5%), but its score in the treatment subcategory (57.3%) was significantly lower than those of Bixby (78.3%, p < 0.001) and Google Assistant (77.3%, p < 0.001). Furthermore, Alexa's scores were also significantly lower than Google Assistant for questions in the subcategory of disease state (69.6% versus 80.5%, p = 0.004).
Classification of Questions | Median Quality Scores of DVAs [% (IQR)] |
p-values* | |||
Apple Siri | Samsung Bixby | Google Assistant | Amazon Alexa | ||
Across all questions | 70.4 (60.9–79.3) | 72.8 (0–81.6) | 78.9 (73.9–85.2) | 64.5 (57.7–76.7) | <0.001 |
Mental Health Categories | |||||
General mental health | 77.1 (71.3–85.2) | 0 (0–16.7) | 80.5 (76.4–89.1) | 70.7 (57.1–79.7) | <0.001 |
Depression | 83.9 (76.0–86.9) | 87.7 (83.6–89.3) | 87.4 (79.4–88.7) | 63.0 (61.4–72.1) | <0.001 |
Anxiety | 71.8 (67.2–87.6) | 75.9 (71.3–80.7) | 76.4 (69.8–83.6) | 60.5 (42.5–68.4) | 0.006 |
Obsessive-compulsive disorder | 61.7 (53.7–69.8) | 0 (0–29.6) | 78.4 (73.6–85.7) | 72.3 (59.1–80.0) | <0.001 |
Bipolar disorder | 66.4 (44.4–70.4) | 77.5 (71.3–81.6) | 75.9 (70.1–81.6) | 63.0 (48.2–80.5) | 0.004 |
Question Subcategories | |||||
Disease state | 71.7 (66.9–79.0) | 71.6 (28.2–83.3) | 80.5 (73.8–84.8) | 69.6 (62.5–80.2) | 0.031 |
Symptoms | 66.7 (53.9–83.1) | 76.7 (25.0–80.9) | 77.5 (70.7–86.0) | 71.5 (57.7–80.4) | 0.239 |
Treatment | 60.5 (49.4–74.2) | 78.3 (63.0–85.0) | 77.3 (69.6–84.3) | 57.3 (30.6–62.1) | <0.001 |
Note: *Kruskal-Wallis test was performed among all the four DVAs with statistical significance defined as p < 0.05. Post-hoc analyses using the Wilcoxon rank sum test with Bonferroni adjustment were performed for each possible pairwise comparison among the DVAs, with statistical significance defined as p < 0.00833.
Across all quality domains, Google Assistant scored the highest while Alexa scored the lowest (Table 3). In terms of comprehension ability, Google Assistant scored significantly higher (100%, p < 0.001) than the other DVAs. In addition, Alexa (100%) scored significantly higher than Siri (88.9%, p < 0.001) and Bixby (94.5%, p = 0.03) in this domain. Google Assistant (100%) and Bixby (100%) also scored significantly higher than Siri (66.7%) and Alexa (75.0%) in terms of relevance. Only Google Assistant was successful in identifying situations that required immediate intervention from one evaluator (16.7%).
Alexa scored the worst among all DVAs in terms of comprehensiveness (22.2%, p < 0.001) and reliability (58.3%, p < 0.001). In addition, Alexa also performed the poorest when evaluated against the QoL criteria (10.0%), as compared to Bixby, which performed the best (76.7%). In contrast, Google Assistant scored the best (77.8%) in terms of comprehensiveness, but it had similar reliability scores as Bixby (75.0% each). In terms of accuracy, Alexa scored the lowest among the DVAs (75.0% versus 100% for other DVAs, p = 0.003). However, all DVAs had similar scores for understandability (50.0% each). The understandability of verbal responses was significantly lower than that of web responses (33.3% versus 50.0%, p = 0.004). Inter-rater reliability ranged from moderate to good for both the overall quality and the individual quality domains (Table 3).
Quality Domains | Median Quality Scores of DVAs [% (IQR)] |
p-value* | Intraclass Correlation Coefficient [ICC (95% CI)] a | |||
Apple Siri | Samsung Bixby | Google Assistant | Amazon Alexa | |||
Comprehen-sion ability | 88.9 (70.8–100) | 94.5 (0–100) | 100 (100–100) | 100 (88.9–100) | <0.001 | 0.892 (0.868–0.913) |
Relevance | 66.7 (50.0–100) | 100 (66.7–100) | 100 (83.3–100) | 75.0 (33.3–100) | <0.001 | 0.753 (0.691–0.804) |
Comprehen-siveness | 66.7 (44.4–83.3) | 66.7 (55.6–88.9) | 77.8 (55.6–88.9) | 22.2 (0–66.7) | <0.001 | 0.747 (0.660–0.812) |
Accuracy | 100 (75.0–100) | 100 (83.3–100) | 100 (83.3–100) | 75.0 (50.0–100) | 0.003 | 0.691 (0.593–0.769) |
Understand-ability | 50.0 (25.0–75.0) | 50.0 (33.3–68.8) | 50.0 (33.3–66.7) | 50.0 (25.0–75.0) | 0.724 | 0.672 (0.513–0.775) |
Reliability | 72.9 (63.2–83.3) | 75.0 (63.9–84.3) | 75.0 (66.7–84.3) | 58.3 (49.1–63.9) | <0.001 | 0.896 (0.863–0.922) |
Overall quality | 70.4 (60.9–79.3) | 72.8 (0–81.6) | 78.9 (73.9–85.2) | 64.5 (57.7–76.7) | <0.001 | 0.848 (0.813–0.877) |
Note: * Kruskal-Wallis test was performed among all four DVAs with statistical significance defined as p < 0.05. Post-hoc analyses using the Wilcoxon rank sum test with Bonferroni adjustment were performed for each possible pairwise comparison among the DVAs, with statistical significance defined as p < 0.00833. a ICC values and their 95% CIs were calculated using the SPSS platform based on the mean rating of three evaluators, absolute agreement and a two-way mixed-effects model. ICC values indicate moderate-to-good inter-rater reliability.
In relation to our hypothesis, this study has shown that DVAs are able to provide relevant and appropriate responses to mental health-related queries. However, the understandability of their responses was relatively low. Furthermore, not all DVAs fared the same in terms of the different quality domains, and they also varied across the various mental health conditions. Overall, Google Assistant performed the best among all DVAs, suggesting that it was able to comprehend the queries and provide responses that were relevant and accurate across the various mental health categories. In comparison, Bixby fared the worst in terms of responding to questions on general mental health and OCD. On the other hand, Alexa's responses were the least comprehensive and reliable across all questions, as well as in the categories of depression, anxiety and bipolar disorder.
All DVAs performed well in terms of comprehension ability. This result was similar to a study by Yang and colleagues, who investigated the abilities of Siri, Google Assistant, Alexa and Cortana in terms of responding to questions on postpartum depression [10]. In their study, all DVAs performed well in terms of recognizing the postpartum depression questions, with scores ranging from 79% (Alexa) to 100% (Siri and Google Assistant). However, in our study, Siri and Bixby performed poorer than Google Assistant and Alexa. For Bixby, a quarter of the questions posed (27.3%, n = 18/66) were scored as 0%. In particular, Bixby often transcribed “OCD” as “o CD” (two separate words), resulting in a large proportion of questions failing to be recognized. In addition, while Bixby could accurately transcribe questions on general mental health, it could not generate responses for many of these questions (80%, n = 8/10) and frequently answered with “I'm not sure I understood that”. We postulate that our observations could be due to Bixby's primary design intent, which was to assist users in operating the phone via voice commands, rather than provide accurate responses to questions, as in the case of other DVAs [52]. On the other hand, while Siri could successfully capture all questions, it was penalized for transcribing errors. Siri tended to cut off the user before the entire question was posed, resulting in incomplete prompts being captured on the screen. Examples included “Can depression...” and “What is the difference between...”, when the entire questions that were meant to be asked were “Can depression be genetic?” and “What is the difference between normal behavior and OCD?”. respectively.
In regard to relevance, Siri and Alexa performed more poorly than Google Assistant and Bixby due to the irrelevant responses provided. For example, Siri responded with answers about medications when the question posed was “How are anxiety disorders diagnosed?” Similarly, Alexa responded with the effects of bipolar disorder to the question of “Who does bipolar disorder affect?” When the DVAs were evaluated for their ability to refer cases that required immediate intervention, only Google Assistant managed to respond appropriately to one evaluator. Interestingly, our observations differed from a study by Kocaballi and colleagues [53], who reported that Siri scored the highest for safety-critical prompts when compared to Google Assistant, Bixby and Alexa. In another study by Miner et al. [17], even though Google Now and Samsung S Voice (predecessor of Bixby) [54] managed to recognize queries on suicide as a cause for concern, Google Now did not recognize the cause for concern for queries on depression, while the responses from S Voice varied, with the cause of concern being recognized only in some instances. Nonetheless, the authors of both studies agreed that there was an inconsistency in the responses of the DVAs and that their abilities to recognize causes for concern should improve. It is unclear whether the inability of DVAs to respond to queries appropriately is due to system failure, a failure of the natural language understanding, a misrecognized prompt, the DVA being unable to find a response or the DVA deliberately not responding to particular types of queries [53]. However, we agree with Kocaballi and colleagues and advocate that the DVAs' capabilities should be made more transparent to users so that it can improve user experience and reduce confusion.
For comprehensiveness, Alexa performed the worst among the DVAs. It also scored significantly lower than Bixby and Google Assistant in terms of accuracy. In contrast, Alexa performed well in terms of comprehension ability, suggesting that, even though it could comprehend the questions being posed, it did not provide comprehensive and accurate responses. Our findings were consistent with a study by Alagha and Helbing, who evaluated the quality of responses to questions on vaccines by Google Assistant, Siri and Alexa [11]. In their study, the authors indicated that Alexa lacked in its ability to process health queries and generate responses from high-quality sources. Furthermore, in our study, Alexa performed significantly poorer than the other DVAs in terms of reliability. One reason was its tendency to only provide verbal responses, such as “Here's something I found on Mayo Clinic”, while the other DVAs provided specific links to webpages. In addition, Alexa provided invalid links to “reference.com”, which could not be accessed on several occasions. Our observations were also in line with the DVA vaccine information study by Alagha and Helbing [11], who reported that Google Assistant and Siri were more capable of directing the user to authoritative sources than Alexa, which did not provide answers from the same sources as the other DVAs. Hence, our recommendation is to supplement Alexa's responses to mental health queries with those of another DVA or other external resources so that any lack of or discrepancies in health-related information provided can be identified by the user.
There was a significant difference between the understandability of verbal responses versus web responses. Verbal responses were less easily understood, as according to the SMOG readability test, and contained more jargon than web responses. However, both types of responses also scored poorly, indicating that the responses of the DVAs to mental health queries are less likely to be understood by a layperson. Our results concurred with a study assessing the readability of online health information, which showed that, among 12 health conditions, the information on dementia and anxiety were the hardest to read [55]. As the understandability of health-related information is important to raise one's awareness and knowledge of mental health issues and self-care, we advocate that the information provided by DVAs should be complemented with other information online and shared between the patient and caregiver (or someone whom the patient trusts) in a close and private setting that is comfortable for the patient.
Across the mental health conditions, Siri, Bixby and Google Assistant scored the highest for questions on depression. Our results were similar to the study by Miner et al., which investigated the responses of Siri, Google Now, S Voice and Cortana to questions on depression [17]. In their study, the DVAs were generally able to recognize prompts, but they were not able to refer the user to a depression helpline. On the contrary, a study by Kocaballi et al. showed that DVAs had the lowest ratio of appropriate responses to mental health prompts, including those of depression [53]. Even though there have been studies investigating the quality of conversational agents on mental health conditions [56],[57], these studies focused on other types of conversational agents, such as chatbots and mobile apps, instead of DVAs. To the best of our knowledge, there is a paucity of studies that explore the quality of DVAs in relation to mental health conditions, especially OCD and bipolar disorder. While Google Assistant seems to be one of the top two DVAs that can potentially be recommended for queries on OCD and bipolar disorder (Figure 2), its ability to answer questions on these two conditions may not be as well established as that for general mental health and depression queries. Interestingly, Siri did not perform as well on either of these mental health conditions. As such, we recommend Apple users who seek information about OCD and/or bipolar disorder from Siri to supplement their responses with other online resources from Google Assistant or Google searches. In any case, our study presents new insight into the quality of DVAs across the span of these four mental health conditions—depression, anxiety, OCD and bipolar disorder.
The main limitation of this study is that we were only able to evaluate a subset of four DVAs and four mental health conditions. Therefore, our results might not be representative of the DVAs' performances for other mental health conditions, nor of the quality of other DVAs (e.g., Google Home Mini and Microsoft Cortana). Furthermore, as the location function of the DVAs were switched on during our evaluations, the search results might have been adapted to the local context, and minor variations could exist depending on the country and location of the user. Studies have shown that the responses of DVAs provided to the same questions can differ [17],[58]. Although the qualitative responses of the DVAs were not compared in this study, we tried to minimize this variability by having each evaluator use the same devices for their evaluations. In order to account for the variations in evaluation scores of the same DVA response by the different evaluators, we calculated the ICC values for each quality domain (Table 3) to determine the inter-rater reliability; our results indicated moderate-to-good reliability. Similarly, inter-rater reliability for the overall quality scores of the DVAs was good. Nonetheless, we acknowledge that this bias may exist in the DVA responses, and our study results should be interpreted with this limitation in mind. In addition, our evaluation protocol might not be reflective of real-life usage of DVAs by the layperson. In our study, when the question posed to the DVAs was not recognized on the first attempt, there would be two more attempts made before the evaluation ended. However, in real-life, users might forgo repeatedly asking the same question multiple times if they encountered an unsuccessful response on their first try. Next, due to time limitations, only the first web link provided by the DVAs was evaluated in this study, but, in reality, users might access other links as well if more than one link was provided by the DVAs. Lastly, our results only provide the quality of the DVAs in a snapshot of time. With advancements in voice recognition technologies, natural language processing and other AI-based algorithms, we expect that the quality of the DVAs will also improve over time. As such, we advise caution when extrapolating the results of this study to other DVAs, other countries/states, other mental health conditions or over time.
Overall, Google Assistant performed the best in terms of responding to mental health-related queries, while Alexa performed the worst. In terms of specific mental health conditions, Bixby performed the worst for questions on general mental health and OCD. While the comprehension abilities of the DVAs were generally good, our study showed that the DVAs had differing performances in the domains of relevance, comprehensiveness, accuracy and reliability. Moreover, the responses of the DVAs generally lacked in understandability. Based on our quality evaluations, we have provided a DVA recommendation list that users can potentially consider for the different mental health conditions (Figure 2). While Google Assistant generally works well across all of the included mental health conditions, Siri and Bixby can also be used for depression and anxiety. On the other hand, Alexa and Bixby may potentially be used for OCD and bipolar disorder, respectively. However, when depending on the DVA responses to their mental health-related queries, we caution the general public to supplement the information provided by the DVAs with other online information from authoritative healthcare organizations, and to always seek the help and advice of a healthcare professional when managing their mental health condition(s). In light of many organizations adapting to the post-pandemic world, future research should focus on other types of mental health conditions (e.g., stress) in patients, caregivers and healthcare professionals resulting from specific circumstances, such as workplace disruptions, loss of healthcare services and the accumulation of new job roles as healthcare undergoes a major digital transformation worldwide. In addition, further research can also be done to evaluate other types of DVAs' performance for mental health conditions that are relevant to the researchers' communities.
[1] |
L. Wang, Z. D. Teng, T. L. Zhang, Threshold dynamics of a malaria transmission model in periodic environment, Commun. Nonlinear Sci. Numer. Simul., 18 (2013), 1288–1303. https://doi.org/10.1016/j.cnsns.2012.09.007 doi: 10.1016/j.cnsns.2012.09.007
![]() |
[2] |
Z. Mukandavire, A. B. Gumel, W. Garira, J. M. Tchuenche, Mathematical analysis of a model for HIV-malaria co-infection, Math. Biosci. Eng., 6 (2009), 333–362. https://doi.org/10.3934/mbe.2009.6.333 doi: 10.3934/mbe.2009.6.333
![]() |
[3] |
X. R. Dong, X. Zhang, M. Y. Wang, L. W. Gu, J. Li, M. X. Gong, Heparin-decorated nanostructured lipid carriers of artemether-protoporphyrin IX-transferrin combination for therapy of malaria, Int. J. Pharm., 605 (2021), 120813. https://doi.org/10.1016/j.ijpharm.2021.120813 doi: 10.1016/j.ijpharm.2021.120813
![]() |
[4] |
L. Xue, C. A. Manore, P. Thongsripong, J. M. Hyman, Two-sex mosquito model for the persistence of Wolbachia, J. Biol. Dyn., 11 (2017), 216–237. https://doi.org/10.1080/17513758.2016.1229051 doi: 10.1080/17513758.2016.1229051
![]() |
[5] |
P. Chanda-Kapata, N. Kapata, A. Zumla, COVID-19 and malaria: a symptom screening challenge for malaria endemic countries, Int. J. Infect. Dis., 94 (2020), 151–153. https://doi.org/10.1016/j.ijid.2020.04.007 doi: 10.1016/j.ijid.2020.04.007
![]() |
[6] |
J. Nachega, M. Seydi, A. Zumla, The late arrival of coronavirus disease 2019 (COVID-19) in Africa: mitigating pan-continental spread, Clin. Infect. Dis., 71 (2020), 875–878. https://doi.org/10.1093/cid/ciaa353 doi: 10.1093/cid/ciaa353
![]() |
[7] |
J. Hopman, B. Allegranzi, S. Mehtar, Managing COVID-19 in low- and middle-income countries, JAMA, 323 (2020), 1549–1550. https://doi.org/10.1001/jama.2020.4169 doi: 10.1001/jama.2020.4169
![]() |
[8] |
J. R. Gutman, N. W. Lucchi, P. T. Cantey, L. C Steinhardt, A. M Samuels, M. L. Kamb, et al., Malaria and parasitic neglected tropical diseases: potential syndemics with COVID-19? Amer. J. Trop. Med. Hyg., 103 (2020), 572–577. https://doi.org/10.4269/ajtmh.20-0516 doi: 10.4269/ajtmh.20-0516
![]() |
[9] |
C. Y. Chiang, A. E. Sony, Tackling the threat of COVID-19 in Africa: an urgent need for practical planning, Int. J. Tuberc. Lung. Dis., 24 (2020), 541–542. https://doi.org/10.5588/ijtld.20.0192 doi: 10.5588/ijtld.20.0192
![]() |
[10] |
M. Gilbert, G. Pullano, F. Pinotti, E. Valdano, C. Poletto, P. Y. Boëlle, et al., Preparedness and vulnerability of African countries against importations of COVID-19: a modelling study, Lancet, 395 (2020), 871–877. https://doi.org/10.1016/S0140-6736(20)30411-6 doi: 10.1016/S0140-6736(20)30411-6
![]() |
[11] |
V. Quaresima, M. M. Naldini, D. M. Cirillo, The prospects for the SARS-CoV-2 pandemic in Africa, EMBO Mol. Med., 12 (2020), e12488. https://doi.org/10.15252/emmm.202012488 doi: 10.15252/emmm.202012488
![]() |
[12] |
M. Majumder, P. K. Tiwari, S. Pal, Impact of nonlinear infection rate on HIV/AIDS considering prevalence-dependent awareness, Math. Methods Appl. Sci., 46 (2023), 3821–3848. https://doi.org/10.1002/mma.8723 doi: 10.1002/mma.8723
![]() |
[13] |
S. Y. Tchoumi, M. L. Diagne, H. Rwezaura, J. M. Tchuenche, Malaria and COVID-19 co-dynamics: a mathematical model and optimal control, Appl. Math. Model., 99 (2021), 294–327. https://doi.org/10.1016/j.apm.2021.06.016 doi: 10.1016/j.apm.2021.06.016
![]() |
[14] |
M. M. Ojo, E. F. D. Goufo, The impact of COVID-19 on a malaria dominated region: a mathematical analysis and simulations, Alex. Eng. J., 65 (2023), 23–39. https://doi.org/10.1016/j.aej.2022.09.045 doi: 10.1016/j.aej.2022.09.045
![]() |
[15] |
B. Yang, Z. H. Yu, Y. L. Cai, The impact of vaccination on the spread of COVID-19: studying by a mathematical model, Phys. A, 590 (2022), 126717. https://doi.org/10.1016/j.physa.2021.126717 doi: 10.1016/j.physa.2021.126717
![]() |
[16] |
B. J. Nath, K. Dehingia, V. N. Mishra, Y. M. Chu, H. K. Sarmah, Mathematical analysis of a within-host model of SARS-CoV-2, Adv. Differ. Equations, 2021 (2021), 13. https://doi.org/10.1186/s13662-021-03276-1 doi: 10.1186/s13662-021-03276-1
![]() |
[17] |
M. A. Rasheed, S. Raza, A. Zohaib, M. I. Riaz, A. Amin, M. Awais, et al., Immunoinformatics based prediction of recombinant multi-epitope vaccine for the control and prevention of SARS-CoV-2, Alex. Eng. J., 60 (2021), 3087–3097. https://doi.org/10.1016/j.aej.2021.01.046 doi: 10.1016/j.aej.2021.01.046
![]() |
[18] |
Z. H. Shen, Y. M. Chu, M. A. Khan, S. Muhammad, O. A. Al-Hartomy, M. Higazy, Mathematical modeling and optimal control of the COVID-19 dynamics, Results Phys., 31 (2021), 105028. https://doi.org/10.1016/j.rinp.2021.105028 doi: 10.1016/j.rinp.2021.105028
![]() |
[19] |
Y. M. Chu, A. Ali, M. A. Khan, S. Islam, S. Ullah, Dynamics of fractional order COVID-19 model with a case study of Saudi Arabia, Results Phys., 21 (2021), 103787. https://doi.org/10.1016/j.rinp.2020.103787 doi: 10.1016/j.rinp.2020.103787
![]() |
[20] |
Y. M. Chu, M. F. Yassen, I. Ahmad, P. Sunthrayuth, M. A. Khan, A fractional SARS-CoV-2 model with Atangana-Baleanu derivative: application to fourth wave, Fractals, 30 (2022), 2240210. https://doi.org/10.1142/S0218348X22402101 doi: 10.1142/S0218348X22402101
![]() |
[21] |
P. Pandey, Y. M. Chu, J. F. Gmez-Aguilar, H. Jahanshahi, A. A. Aly, A novel fractional mathematical model of COVID-19 epidemic considering quarantine and latent time, Results Phys., 26 (2021), 104286. https://doi.org/10.1016/j.rinp.2021.104286 doi: 10.1016/j.rinp.2021.104286
![]() |
[22] |
A. Omame, H. Rwezaura, M. L. Diagne, COVID-19 and dengue co-infection in Brazil: optimal control and cost-effectiveness analysis, Eur. Phys. J. Plus, 136 (2021), 1090. https://doi.org/10.1140/epjp/s13360-021-02030-6 doi: 10.1140/epjp/s13360-021-02030-6
![]() |
[23] |
A. Omame, M. E. Isah, M. Abbas, A fractional order model for dual variants of COVID-19 and HIV co-infection via Atangana-Baleanu derivative, Alex. Eng. J., 61 (2022), 9715–9731. https://doi.org/10.1016/j.aej.2022.03.013 doi: 10.1016/j.aej.2022.03.013
![]() |
[24] |
N. Ringa, M. L. Diagne, H. Rwezaura, A. Omame, S. Y. Tchoumi, J. M. Tchuenche, HIV and COVID-19 co-infection: a mathematical model and optimal control, Inf. Med. Unlocked, 31 (2022), 100978. https://doi.org/10.1016/j.imu.2022.100978 doi: 10.1016/j.imu.2022.100978
![]() |
[25] |
I. M. Hezam, A. Foul, A. Alrasheedi, A dynamic optimal control model for COVID-19 and cholera co-infection in Yemen, Adv. Differ. Equations, 2021 (2021), 108. https://doi.org/10.1186/s13662-021-03271-6 doi: 10.1186/s13662-021-03271-6
![]() |
[26] |
W. Y. Shen, Y. M. Chu, M. ur Rahman, I. Mahariq, A. Zeb, Mathematical analysis of HBV and HCV co-infection model under nonsingular fractional order derivative, Results Phys., 28 (2021), 104582. https://doi.org/10.1016/j.rinp.2021.104582 doi: 10.1016/j.rinp.2021.104582
![]() |
[27] |
M. Majumder, P. K. Tiwari, S. Pal, Impact of saturated treatments on HIV-TB dual epidemic as a consequence of COVID-19: optimal control with awareness and treatment, Nonlinear Dyn., 109 (2022), 143–176. https://doi.org/10.1007/s11071-022-07395-6 doi: 10.1007/s11071-022-07395-6
![]() |
[28] |
J. M. Mutua, F. B. Wang, N. K. Vaidya, Modeling malaria and typhoid fever co-infection dynamics, Math. Biosci., 264 (2015), 128–144. https://doi.org/10.1016/j.mbs.2015.03.014 doi: 10.1016/j.mbs.2015.03.014
![]() |
[29] |
A. Omame, M. Abbas, C. P. Onyenegecha, A fractional-order model for COVID-19 and tuberculosis co-infection using Atangana-Baleanu derivative, Chaos Solitons Fract., 153 (2021), 111486. https://doi.org/10.1016/j.chaos.2021.111486 doi: 10.1016/j.chaos.2021.111486
![]() |
[30] |
A. Omame, M. Abbas, C. P. Onyenegecha, A fractional order model for the co-interaction of COVID-19 and hepatitis B virus, Results Phys., 37 (2022), 105498. https://doi.org/10.1016/j.rinp.2022.105498 doi: 10.1016/j.rinp.2022.105498
![]() |
[31] |
B. E. Bassey, J. U. Atsu, Global stability analysis of the role of multi-therapies and non-pharmaceutical treatment protocols for COVID-19 pandemic, Chaos Solitons Fract., 143 (2021), 110574. https://doi.org/10.1016/j.chaos.2020.110574 doi: 10.1016/j.chaos.2020.110574
![]() |
[32] |
A. S. Bhadauria, R. Pathak, M. Chaudhary, A SIQ mathematical model on COVID-19 investigating the lockdown effect, Infect. Dis. Modell., 6 (2021), 244–257. https://doi.org/10.1016/j.idm.2020.12.010 doi: 10.1016/j.idm.2020.12.010
![]() |
[33] | J. Couras, I. Area, J. J. Nieto, C. J. Silva, D. F. M. Torres, Optimal control of vaccination and plasma transfusion with potential usefulness for COVID-19, In: P. Agarwal, J. J. Nieto, M. Ruzhansky, D. F. M. Torres, Analysis of infectious disease problems (COVID-19) and their global impact, Springer, 2021. https://doi.org/10.1007/978-981-16-2450-6_23 |
[34] |
P. Wintachai, K. Prathom, Stability analysis of SEIR model related to efficiency of vaccines for COVID-19 situation, Heliyon, 7 (2021), e06812. https://doi.org/10.1016/j.heliyon.2021.e06812 doi: 10.1016/j.heliyon.2021.e06812
![]() |
[35] | S. Berkane, I. Harizi, A. Tayebi, Modeling the effect of population-wide vaccination on the evolution of COVID-19 epidemic in Canada, medRxiv, 2021. https://doi.org/10.1101/2021.02.05.21250572 |
[36] |
M. A. Acuña-Zegarra, S. Díaz-Infante, D. Baca-Carrasco, D. Olmos-Liceaga, COVID-19 optimal vaccination policies: a modeling study on efficacy, natural and vaccine-induced immunity responses, Math. Biosci., 337 (2021), 108614. https://doi.org/10.1016/j.mbs.2021.108614 doi: 10.1016/j.mbs.2021.108614
![]() |
[37] |
B. Buonomo, R. D. Marca, A. d'Onofrio, M. Groppi, A behavioural modelling approach to assess the impact of COVID-19 vaccine hesitancy, J. Theor. Biol., 534 (2022), 110973. https://doi.org/10.1016/j.jtbi.2021.110973 doi: 10.1016/j.jtbi.2021.110973
![]() |
[38] |
A. Fridman, R. Gershon, A. Gneezy, COVID-19 and vaccine hesitancy: a longitudinal study, Plos One, 16 (2021), e0250123. https://doi.org/10.1371/journal.pone.0250123 doi: 10.1371/journal.pone.0250123
![]() |
[39] |
N. Gul, R. Bilal, E. A. Algehyne, The dynamics of fractional order Hepatitis B virus model with asymptomatic carriers, Alex. Eng. J., 60 (2021), 3945–3955. https://doi.org/10.1016/j.aej.2021.02.057 doi: 10.1016/j.aej.2021.02.057
![]() |
[40] |
F. B. Agusto, Optimal isolation control strategies and cost-effectiveness analysis of a two-strain avian influenza model, Biosystems, 113 (2013), 155–164. https://doi.org/10.1016/j.biosystems.2013.06.004 doi: 10.1016/j.biosystems.2013.06.004
![]() |
[41] |
P. van den Driessche, J. Watmough, Reproduction numbers and sub-threshold endemic equilibria for compartmental models of disease transmission, Math. Biosci., 180 (2002), 29–48. https://doi.org/10.1016/S0025-5564(02)00108-6 doi: 10.1016/S0025-5564(02)00108-6
![]() |
[42] | J. P. L. Salle, The stability of dynamical systems, SIAM, 1976. |
[43] |
L. Xue, H. Y. Zhang, W. Sun, C. Scoglio, Transmission dynamics of multi-strain dengue virus with cross-immunity, Appl. Math. Comput., 392 (2021), 125742. https://doi.org/10.1016/j.amc.2020.125742 doi: 10.1016/j.amc.2020.125742
![]() |
[44] | L. Pontryagin, V. Boltyanskii, R. Gamkrelidze, E. Mishchenko, The mathematical theory of optimal control process, 4 Eds., Routledge, 1962. |
Number of responses (%), N = 66 a |
||||
Apple Siri | Samsung Bixby | Google Assistant | Amazon Alexa | |
Types of responses by DVAs | ||||
Verbal response only b | 6 (9.1) | 24 (36.4) | 1 (1.5) | 41 (62.1) |
Web response only c | 48 (72.7) | 14 (21.2) | 13 (19.7) | 0 (0) |
Verbal and web response d | 11 (16.7) | 28 (42.4) | 32 (78.8) | 24 (36.4) |
No response | 1 (1.5) | 0 (0) | 0 (0) | 1 (1.5) |
Proportion of successful responses | ||||
Questions that were recognized e | 63 (95.5) | 46 (69.7) | 66 (100.0) | 60 (90.9) |
Relevant responses | 47 (71.2) | 38 (57.6) | 66 (100.0) | 44 (66.7) |
Proportion of sources provided in DVA responses | ||||
Tier A | 13 (19.7) | 19 (28.8) | 36 (54.5) | 20 (30.3) |
Tier B | 19 (28.8) | 18 (27.3) | 18 (27.3) | 8 (12.1) |
Tier C | 15 (22.7) | 1 (1.5) | 6 (9.1) | 15 (22.7) |
No sources provided, or sources that could not be evaluated | 19 (28.8) | 28 (42.4) | 6 (9.1) | 23 (34.8) |
Note: a Results were taken from the average of three evaluators. b A short verbal text that directly answered the question without providing a link. c A link was provided in response to the question without a verbal explanation. d Both a verbal explanation and a link were present in the response. e These were questions that were captured on the smartphone screen and induced a response by the DVA. Responses such as “I'm not sure I understood that” were classified as the DVA not recognizing the question.
Classification of Questions | Median Quality Scores of DVAs [% (IQR)] |
p-values* | |||
Apple Siri | Samsung Bixby | Google Assistant | Amazon Alexa | ||
Across all questions | 70.4 (60.9–79.3) | 72.8 (0–81.6) | 78.9 (73.9–85.2) | 64.5 (57.7–76.7) | <0.001 |
Mental Health Categories | |||||
General mental health | 77.1 (71.3–85.2) | 0 (0–16.7) | 80.5 (76.4–89.1) | 70.7 (57.1–79.7) | <0.001 |
Depression | 83.9 (76.0–86.9) | 87.7 (83.6–89.3) | 87.4 (79.4–88.7) | 63.0 (61.4–72.1) | <0.001 |
Anxiety | 71.8 (67.2–87.6) | 75.9 (71.3–80.7) | 76.4 (69.8–83.6) | 60.5 (42.5–68.4) | 0.006 |
Obsessive-compulsive disorder | 61.7 (53.7–69.8) | 0 (0–29.6) | 78.4 (73.6–85.7) | 72.3 (59.1–80.0) | <0.001 |
Bipolar disorder | 66.4 (44.4–70.4) | 77.5 (71.3–81.6) | 75.9 (70.1–81.6) | 63.0 (48.2–80.5) | 0.004 |
Question Subcategories | |||||
Disease state | 71.7 (66.9–79.0) | 71.6 (28.2–83.3) | 80.5 (73.8–84.8) | 69.6 (62.5–80.2) | 0.031 |
Symptoms | 66.7 (53.9–83.1) | 76.7 (25.0–80.9) | 77.5 (70.7–86.0) | 71.5 (57.7–80.4) | 0.239 |
Treatment | 60.5 (49.4–74.2) | 78.3 (63.0–85.0) | 77.3 (69.6–84.3) | 57.3 (30.6–62.1) | <0.001 |
Note: *Kruskal-Wallis test was performed among all the four DVAs with statistical significance defined as p < 0.05. Post-hoc analyses using the Wilcoxon rank sum test with Bonferroni adjustment were performed for each possible pairwise comparison among the DVAs, with statistical significance defined as p < 0.00833.
Quality Domains | Median Quality Scores of DVAs [% (IQR)] |
p-value* | Intraclass Correlation Coefficient [ICC (95% CI)] a | |||
Apple Siri | Samsung Bixby | Google Assistant | Amazon Alexa | |||
Comprehen-sion ability | 88.9 (70.8–100) | 94.5 (0–100) | 100 (100–100) | 100 (88.9–100) | <0.001 | 0.892 (0.868–0.913) |
Relevance | 66.7 (50.0–100) | 100 (66.7–100) | 100 (83.3–100) | 75.0 (33.3–100) | <0.001 | 0.753 (0.691–0.804) |
Comprehen-siveness | 66.7 (44.4–83.3) | 66.7 (55.6–88.9) | 77.8 (55.6–88.9) | 22.2 (0–66.7) | <0.001 | 0.747 (0.660–0.812) |
Accuracy | 100 (75.0–100) | 100 (83.3–100) | 100 (83.3–100) | 75.0 (50.0–100) | 0.003 | 0.691 (0.593–0.769) |
Understand-ability | 50.0 (25.0–75.0) | 50.0 (33.3–68.8) | 50.0 (33.3–66.7) | 50.0 (25.0–75.0) | 0.724 | 0.672 (0.513–0.775) |
Reliability | 72.9 (63.2–83.3) | 75.0 (63.9–84.3) | 75.0 (66.7–84.3) | 58.3 (49.1–63.9) | <0.001 | 0.896 (0.863–0.922) |
Overall quality | 70.4 (60.9–79.3) | 72.8 (0–81.6) | 78.9 (73.9–85.2) | 64.5 (57.7–76.7) | <0.001 | 0.848 (0.813–0.877) |
Note: * Kruskal-Wallis test was performed among all four DVAs with statistical significance defined as p < 0.05. Post-hoc analyses using the Wilcoxon rank sum test with Bonferroni adjustment were performed for each possible pairwise comparison among the DVAs, with statistical significance defined as p < 0.00833. a ICC values and their 95% CIs were calculated using the SPSS platform based on the mean rating of three evaluators, absolute agreement and a two-way mixed-effects model. ICC values indicate moderate-to-good inter-rater reliability.
Number of responses (%), N = 66 a |
||||
Apple Siri | Samsung Bixby | Google Assistant | Amazon Alexa | |
Types of responses by DVAs | ||||
Verbal response only b | 6 (9.1) | 24 (36.4) | 1 (1.5) | 41 (62.1) |
Web response only c | 48 (72.7) | 14 (21.2) | 13 (19.7) | 0 (0) |
Verbal and web response d | 11 (16.7) | 28 (42.4) | 32 (78.8) | 24 (36.4) |
No response | 1 (1.5) | 0 (0) | 0 (0) | 1 (1.5) |
Proportion of successful responses | ||||
Questions that were recognized e | 63 (95.5) | 46 (69.7) | 66 (100.0) | 60 (90.9) |
Relevant responses | 47 (71.2) | 38 (57.6) | 66 (100.0) | 44 (66.7) |
Proportion of sources provided in DVA responses | ||||
Tier A | 13 (19.7) | 19 (28.8) | 36 (54.5) | 20 (30.3) |
Tier B | 19 (28.8) | 18 (27.3) | 18 (27.3) | 8 (12.1) |
Tier C | 15 (22.7) | 1 (1.5) | 6 (9.1) | 15 (22.7) |
No sources provided, or sources that could not be evaluated | 19 (28.8) | 28 (42.4) | 6 (9.1) | 23 (34.8) |
Classification of Questions | Median Quality Scores of DVAs [% (IQR)] |
p-values* | |||
Apple Siri | Samsung Bixby | Google Assistant | Amazon Alexa | ||
Across all questions | 70.4 (60.9–79.3) | 72.8 (0–81.6) | 78.9 (73.9–85.2) | 64.5 (57.7–76.7) | <0.001 |
Mental Health Categories | |||||
General mental health | 77.1 (71.3–85.2) | 0 (0–16.7) | 80.5 (76.4–89.1) | 70.7 (57.1–79.7) | <0.001 |
Depression | 83.9 (76.0–86.9) | 87.7 (83.6–89.3) | 87.4 (79.4–88.7) | 63.0 (61.4–72.1) | <0.001 |
Anxiety | 71.8 (67.2–87.6) | 75.9 (71.3–80.7) | 76.4 (69.8–83.6) | 60.5 (42.5–68.4) | 0.006 |
Obsessive-compulsive disorder | 61.7 (53.7–69.8) | 0 (0–29.6) | 78.4 (73.6–85.7) | 72.3 (59.1–80.0) | <0.001 |
Bipolar disorder | 66.4 (44.4–70.4) | 77.5 (71.3–81.6) | 75.9 (70.1–81.6) | 63.0 (48.2–80.5) | 0.004 |
Question Subcategories | |||||
Disease state | 71.7 (66.9–79.0) | 71.6 (28.2–83.3) | 80.5 (73.8–84.8) | 69.6 (62.5–80.2) | 0.031 |
Symptoms | 66.7 (53.9–83.1) | 76.7 (25.0–80.9) | 77.5 (70.7–86.0) | 71.5 (57.7–80.4) | 0.239 |
Treatment | 60.5 (49.4–74.2) | 78.3 (63.0–85.0) | 77.3 (69.6–84.3) | 57.3 (30.6–62.1) | <0.001 |
Quality Domains | Median Quality Scores of DVAs [% (IQR)] |
p-value* | Intraclass Correlation Coefficient [ICC (95% CI)] a | |||
Apple Siri | Samsung Bixby | Google Assistant | Amazon Alexa | |||
Comprehen-sion ability | 88.9 (70.8–100) | 94.5 (0–100) | 100 (100–100) | 100 (88.9–100) | <0.001 | 0.892 (0.868–0.913) |
Relevance | 66.7 (50.0–100) | 100 (66.7–100) | 100 (83.3–100) | 75.0 (33.3–100) | <0.001 | 0.753 (0.691–0.804) |
Comprehen-siveness | 66.7 (44.4–83.3) | 66.7 (55.6–88.9) | 77.8 (55.6–88.9) | 22.2 (0–66.7) | <0.001 | 0.747 (0.660–0.812) |
Accuracy | 100 (75.0–100) | 100 (83.3–100) | 100 (83.3–100) | 75.0 (50.0–100) | 0.003 | 0.691 (0.593–0.769) |
Understand-ability | 50.0 (25.0–75.0) | 50.0 (33.3–68.8) | 50.0 (33.3–66.7) | 50.0 (25.0–75.0) | 0.724 | 0.672 (0.513–0.775) |
Reliability | 72.9 (63.2–83.3) | 75.0 (63.9–84.3) | 75.0 (66.7–84.3) | 58.3 (49.1–63.9) | <0.001 | 0.896 (0.863–0.922) |
Overall quality | 70.4 (60.9–79.3) | 72.8 (0–81.6) | 78.9 (73.9–85.2) | 64.5 (57.7–76.7) | <0.001 | 0.848 (0.813–0.877) |