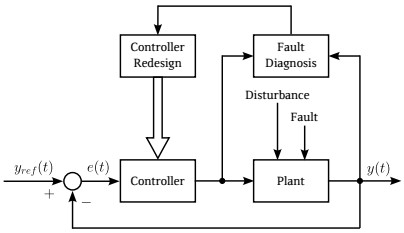
The fault-tolerant control problem of a hydraulic servo actuator in the presence of actuator faults is studied utilizing adaptive dynamic programming. This task is challenging because of unknown system dynamics, uncertain disturbances or unmeasurable system states of such highly nonlinear systems in real applications. The aim is to achieve asymptotic tracking and actuator faults compensation by minimizing some predefined performance index. The discrete-time algebraic Riccati equation is iteratively solved by the adaptive dynamic programming approach. For practical reasons, adaptive dynamic programming techniques and fault compensation are integrated to iteratively compute an approximated optimal fault-tolerant control using real-time input/output data without any a priori knowledge of the system dynamics and unmeasurable states. As a result, a fault-tolerant control of hydraulic servo actuator is then designed based on adaptive dynamic programming via output feedback. Also, the convergence analysis of a data-driven fault-tolerant control is theoretically shown as well. Finally, intensive simulation results are given to prove the validity and merits of the developed data-driven fault-tolerant control strategy.
Citation: Vladimir Stojanovic. Fault-tolerant control of a hydraulic servo actuator via adaptive dynamic programming[J]. Mathematical Modelling and Control, 2023, 3(3): 181-191. doi: 10.3934/mmc.2023016
[1] | Vladimir Djordjevic, Ljubisa Dubonjic, Marcelo Menezes Morato, Dragan Prsic, Vladimir Stojanovic . Sensor fault estimation for hydraulic servo actuator based on sliding mode observer. Mathematical Modelling and Control, 2022, 2(1): 34-43. doi: 10.3934/mmc.2022005 |
[2] | Weiwei Han, Zhipeng Zhang, Chengyi Xia . Modeling and analysis of networked finite state machine subject to random communication losses. Mathematical Modelling and Control, 2023, 3(1): 50-60. doi: 10.3934/mmc.2023005 |
[3] | Lusong Ding, Weiwei Sun . Neuro-adaptive finite-time control of fractional-order nonlinear systems with multiple objective constraints. Mathematical Modelling and Control, 2023, 3(4): 355-369. doi: 10.3934/mmc.2023029 |
[4] | Naveen Kumar, Km Shelly Chaudhary . Position tracking control of nonholonomic mobile robots via $ H_\infty $-based adaptive fractional-order sliding mode controller. Mathematical Modelling and Control, 2025, 5(1): 121-130. doi: 10.3934/mmc.2025009 |
[5] | Hongli Lyu, Yanan Lyu, Yongchao Gao, Heng Qian, Shan Du . MIMO fuzzy adaptive control systems based on fuzzy semi-tensor product. Mathematical Modelling and Control, 2023, 3(4): 316-330. doi: 10.3934/mmc.2023026 |
[6] | S. Y. Tchoumi, Y. Kouakep-Tchaptchie, D. J. Fotsa-Mbogne, J. C. Kamgang, J. M. Tchuenche . Optimal control of a malaria model with long-lasting insecticide-treated nets. Mathematical Modelling and Control, 2021, 1(4): 188-207. doi: 10.3934/mmc.2021018 |
[7] | M. Haripriya, A. Manivannan, S. Dhanasekar, S. Lakshmanan . Finite-time synchronization of delayed complex dynamical networks via sampled-data controller. Mathematical Modelling and Control, 2025, 5(1): 73-84. doi: 10.3934/mmc.2025006 |
[8] | Monica Veronica Crankson, Olusegun Olotu, Ayodeji Sunday Afolabi, Afeez Abidemi . Modeling the vaccination control of bacterial meningitis transmission dynamics: a case study. Mathematical Modelling and Control, 2023, 3(4): 416-434. doi: 10.3934/mmc.2023033 |
[9] | Yuyang Zhao, Yang Liu . Output controllability and observability of mix-valued logic control networks. Mathematical Modelling and Control, 2021, 1(3): 145-156. doi: 10.3934/mmc.2021013 |
[10] | Yanchao He, Yuzhen Bai . Finite-time stability and applications of positive switched linear delayed impulsive systems. Mathematical Modelling and Control, 2024, 4(2): 178-194. doi: 10.3934/mmc.2024016 |
The fault-tolerant control problem of a hydraulic servo actuator in the presence of actuator faults is studied utilizing adaptive dynamic programming. This task is challenging because of unknown system dynamics, uncertain disturbances or unmeasurable system states of such highly nonlinear systems in real applications. The aim is to achieve asymptotic tracking and actuator faults compensation by minimizing some predefined performance index. The discrete-time algebraic Riccati equation is iteratively solved by the adaptive dynamic programming approach. For practical reasons, adaptive dynamic programming techniques and fault compensation are integrated to iteratively compute an approximated optimal fault-tolerant control using real-time input/output data without any a priori knowledge of the system dynamics and unmeasurable states. As a result, a fault-tolerant control of hydraulic servo actuator is then designed based on adaptive dynamic programming via output feedback. Also, the convergence analysis of a data-driven fault-tolerant control is theoretically shown as well. Finally, intensive simulation results are given to prove the validity and merits of the developed data-driven fault-tolerant control strategy.
As the core of the contemporary control theory, optimal control has received wide attention from researchers and manufacturers during the last few decades. Werbos [1] first suggested ADP, a valuable approximation method, as an appropriate tool for solving optimal control tasks in nonlinear systems. In the past few years, many ADP-based methods have been well developed for discrete-time nonlinear systems [2,3,4], data-based control systems [5], intelligent systems [6,7] and unknown nonlinear systems subject to various constraints [8,9] or faults with the actuator [10,11].
With the rapid development of modern technologies, systems are becoming more and more complex and extensive. For this reason, the need for controlling complex systems has been increasing lately. As a result, unavoidable system faults can affect product quality, damage equipment or harm humans. It is well known that in existing engineering systems, the system components, such as actuators and sensors, may be severely damaged due to sudden faults that occur individually or simultaneously during operation, causing severe disasters [12,13]. From all types of faults, actuator faults significantly contribute to reducing control system performance. In addition, the actuators may suffer from faults during long-term operations owing to hardware ageing and other factors. Therefore, it is crucial to develop FTC approaches to handle such faults and keep the acceptable performance of the control system [14].
It is well known that there are passive and active FTC schemes. Passive FTC is a robust control technique in terms of a priori given set of faults. Passive FTC consists of two parts: a feedback control, which is exclusively controlled by a performance controller, while uncertainties of the model and different disturbances are controlled by the controller's robustness. Hence, passive FTC schemes are entirely restricted when handling significant faults. In contrast to that, active FTC schemes have a more vital ability to tolerate faults, which accomplish stability and requested performances by actively tuning control techniques according to the decision of a fault diagnosis unit. There are various methods for operating active FTCs, such as fault accommodation, fault compensation or fault reconfiguration. FTC has extensive usage and crucial importance in different research areas, particulary in the intelligent manufacturing industry. FTC is a progressive regulation method that provides secured work of the system in the case of component or parameter faults [15,16,17]. The general structure of the data-driven FTC systems is shown in Figure 1.
Recently, data-driven FTC has become one of the most vibrant research areas. A new online fault detection and isolation strategy based on the multi-model concept for aircraft jet engines is considered in [18], in which the linearization of nonlinear dynamics was performed on a set of linear models [19]. The fault detection and isolation problem of nonlinear systems using a symbolic-based linear multi-model concept is discussed in [20]. The intelligent optimal control strategy for unknown discrete-time nonlinear systems is discussed in [21,22]. This control strategy is ADP based iterative algorithm [23,24] belonging to machine learning for solving the appropriate Hamilton-Jacobi-Bellman (HJB) equation, which is very complicated to solve directly.
Many ADP based FTC approaches have been developed in recent years, in which actuator faults represent the most frequent faults. To operate with actuator gain and bias faults, the ADP method is employed to create a sliding-mode FTC algorithm [25]. In [26], a guaranteed cost FTC technique based on actor-critic networks is studied for highly complicated nonlinear systems with actuator efficiency loss. Estimated actuator faults from a fault observer is developed in [27] to derive FTC scheme for a class of nonlinear systems based on ADP. An adaptive fuzzy FTC method is developed in [28] for a strict-feedback nonlinear system.
A large number of hydraulic servo actuator (HSA) driven machines often operate with large loads in harsh and mostly outdoor environments. External influences affect that the HSA parameters cannot be determined accurately. As a result of unknown dynamics, high nonlinearities, unmeasurable states and high-quality control of HSA has always been a challenge in research. Moreover, changes in operating conditions during work, such as oil temperature, bulk modulus, fluctuating supply pressure and pipe volume, will lead to parameter changes that reduce existing control performance. The mentioned facts make challenging problems in achieving high-quality HSA control, which is unachievable without knowledge of the exact HSA model [29,30].
ADP is an efficient method for achieving high HSA optimal controller performance by relying on adaptive control, optimal control and reinforcement learning [22,31,32,33,34]. ADP is a data-driven control approach that can ensure the stability of a feedback-controlled system [31]. In the presence of unknown system dynamics and unmeasurable states, ADP approaches based on measured input/output data from linear systems, also known as output feedback, are of significant importance. One key advantage of using output feedback approaches is that no understanding of HSA dynamics is required. This indirect method creates a series of suboptimal controllers for an unknown HSA model, which with further iterations lead to the optimal control strategy. Since nonlinear systems may be accurately described by linear models with online estimated dynamics, this increases the practical value of control strategies [35,36]. Furthermore, measuring the entire HSA state vector directly is not practical in actual implementations and would need very costly instruments for measuring. Therefore, it is more practical when using control algorithms that employ state reconstruction approaches instead of taking direct measurements of the states [31].
ADP-based FTC for HSA is recommended as a result of the discussions above. The controller learns to account for unknown HSA dynamics, disturbances and faults in order to provide expected performances from the measured input/output data. ADP, state reconstruction and output feedback are used to iteratively solve FTC. After identifying the unknown HSA model, the algebraic Riccati equation (ARE) can be solved iteratively. To fulfill the criteria of the persistent excitation condition, some exploration noise should be introduced to control input to assure consistency of approximations and get unique solutions in every iteration step [37]. Application of ADP-based control methods makes data collecting for the discrete-time HSA model simpler than for the continuous-time model. As a result, the discretized HSA model's state vector may be reconstructed using the collected input and output data, allowing the implementation of ADP-based FTC control.
Here, a real-time fault compensation procedure based on ADP to enable optimal control of faulty HSA system is considered in this paper. When actuator faults occur, a ADP-based control algorithm may be biased or incapable of performing optimal control. This issue is fixed by reconfiguring the ADP controller using fault compensation. The Lyapunov theory provides a reliable guarantee for a closed-loop control system with actuator faults. Despite the classical ADP approach, the control action is no longer necessary in the proposed control procedure, which efficiently decreases the computational burden. The approach under consideration is divided into two parts: an ADP-based optimal control and a real-time fault compensation. Thus, it can be very suitably and easily applied to solve fault-tolerant control problems.
The remaining parts of this paper are arranged as follows. Section 2 discusses the difficulty of modeling HSA with uncertain dynamics. In Section 3, the FTC based on ADP is presented. The results of the simulation demonstrate the applicability and efficacy of the ADP-based FTC for the HSA in the face of total uncertainty in the model in Section 4. The final observations are provided in Section 5.
The HSA under consideration comprises of a servo valve and a hydraulic cylinder, see Figure 2. Va, pa, Aa and Vb, pb, Ab are fluid volume, fluid pressure and effective area of the head and road piston side, respectively. Internal and external leakage flow are qLi, qLe, forward and return flows are qa, qb, respectively. Disturbance force is denoted as Fext, load spring gradient is Ke, while βe is bulk modulus of the fluid. Supply and tank pressures are pS and p0, respectively. Based on the component dynamics of the HSA, which consists of the dynamics of the motion of the piston, the dynamics of the pressure in the cylinder, and the dynamics of the servo valve, an analysis of the characteristics of the HSA can be derived. The HSA model is clearly built on complicated nonlinear equations that depend on a variety of factors that are impossible to identify precisely [30].
If the state and input variables are defined as
x(t)=[x1(t)x2(t)x3(t)x4(t)]T≜[y(t)˙y(t)pa(t)pb(t)]T, | (2.1) |
u(t)=xv(t), | (2.2) |
the HSA's nonlinear continuous-time dynamics may be described in state-space terms as follows
˙x(t)=f(x(t))+g(x(t),u(t))+h(t),y(t)=η(x(t)), | (2.3) |
in which the state dynamics is f(x(t)), the input function is g(x(t),u(t)), output function η(x(t))=x1(t), and disturbance function h(t), piston displacement is y, xv is the spool valve displacement, mt is total mass which consists of piston mass mp and payload mass m.
This nonlinear HSA model will also be treated as a linearized model whose parameters were acquired experimentally at various operating points [30]. The mathematical equations are now more appropriately represented by means of the load pressure
pL=pa−αpb | (2.4) |
leading to fewer complicated dynamic equations. Applying a new state vector [x1(t)x2(t)x3(t)]T≜[y(t)˙y(t)pL(t)]T helps us to represent the HSA in a more succinct manner. The following is the reduced order's linearized continuous-time description.
˙x(t)=Ax(t)+Bu(t), | (2.5) |
y(t)=Cx(t), | (2.6) |
where A=[0100−BCmtAamt0−KdKp], B=[00Kx]T, C=[100], in which BC represents viscous friction coefficient, while Kd, Kp and Kx are hydraulic cylinder gain, pressure and valve sensitivity coefficients, respectively, for more details see [30].
Let us consider the linear model of HSA with actuator faults as follows
˙x(t)=Ax(t)+B(u(t)−fa(t)), | (3.1) |
y(t)=Cx(t), | (3.2) |
where x(t)∈Rn, u(t)∈Rm, y(t)∈Rr, fa(t)∈Rm, represent the system state, the control input, the output, the unknown actuator fault, respectively. A∈Rn×n, B∈Rn×m, C∈Rr×n are unknown system matrices, assuming that (A,B) is controllable and (A,C) is observable.
For HSA system (3.1)-(3.2) with fa(t)=0 the performance function may be written as follows:
J(x0)=∫∞0[yT(τ)Qy(τ)+uT(τ)Ru(τ)]dτ, | (3.3) |
where x0∈Rn is an initial state, Q=QT≥0, R=RT>0, with (A,Q1/2C) observable.
The objective of the design is to create a linear optimal control as follows
u=−K∗x, | (3.4) |
which minimizes performance index (3.3), in which the optimal feedback gain matrix K∗ is
K∗=R−1BTP∗, | (3.5) |
where P∗=(P∗)T>0 is a symmetric solution to the well-known algebraic Riccati equation (ARE) [38]
ATP∗+P∗A+CTQC−P∗BR−1BTP∗=0. | (3.6) |
It should be noted that this optimal control design is mainly applicable to low-order simple linear systems. In fact, for high order large-scale systems, it is usually difficult to directly solve P∗ from (3.6), which is nonlinear in P.
Also, for practical implementation of the control system, it is easier to realize the data acquisition for the discrete-time systems compared with continuous-time systems. Consequently, we transform the continuous-time HSA into the following discrete-time HSA as follows
xk+1=Adxk+Bduk, | (3.7) |
yk=Cxk, | (3.8) |
in which Ad=eAh, Bd=∫h0(eAτdτ)B, where h>0 is a specific sampling period, assuming ωh=2π/h is non-pathological sampling frequency, whose existence is well known [39]. In other words, the controllability and observability of the original continuous-time HSA system is kept after discretization. Namely, if the state, input, and output vector at the sampled instant kh are xk, uk, yk, respectively, then (Ad,C) and (Ad,Q1/2C) are observable while (Ad,Bd) is controllable.
The ADP-based controller for HSA system is divided into three components: state reconstruction, critic, and actor. The state reconstruction establishes a link between the input and output data and HSA states solving the optimal control of HSA with unknown dynamics. The controller's critic component is designed to estimate the control strategy based on the input/output data. In order to enhance its effectiveness, the controller learns online. At last, the better control strategy is implemented by the actor.
Minimizing (3.3) can be approximated using the PI technique if the HSA system is fault-free (i.e., fa=0). The control goal is to provide a fault compensation control strategy for systems (3.1)-(3.2) that experience actuator faults in order to stabilize the closed-loop system.
Further, the performance index for discretized system (3.7)-(3.8) is:
Jd(x0)=∞∑j=0yTjQdyj+uTjRduj, | (3.9) |
where Qd=Qh and Rd=Rh. The optimal control low minimizing (3.9) is
uk=−K∗dxk, | (3.10) |
where discrete optimal feedback gain matrix is K∗d=(R+BTdP∗dBd)−1BTdP∗dAd, in which P∗d is the unique symmetric positive definite solution to
ATdP∗dAd−P∗d+CTQC−ATdP∗dBdK∗d=0. | (3.11) |
Since equation (3.11) is nonlinear in P∗d, it is difficult to directly solve P∗d for high order large scale systems. Nevertheless, many efficient algorithms have been developed to numerically approximate the solution of (3.11). One of these algorithms was developed by Hewer [40], and is introduced in the form of Lemma 3.1.
Lemma 3.1. Let K0∈Rm×n be any stability feedback gain matrix and let Pj be the symmetric positive definite solution of the Lyapunov equation
(Ad−BdKj)TPj(Ad−BdKj)+CTQdC+KTjRdKj=0, | (3.12) |
where Kj, j=1,2,… can be updated as follows
Kj=(R+BTdPj−1Bd)−1BTdPj−1Ad. | (3.13) |
Then, it holds
1. Ad−BdKj is a stability matrix;
2. P∗d≤Pj+1≤Pj;
3. limj→∞Kj=K∗d, limj→∞Pj=P∗d.
The solution to the nonlinear equation (3.11) is numerically approximated by iteratively solving Lyapunov equations (3.12), which are linear in Pj, and recursively updating the control low Kj by (3.13) [40]. The sequences {Pj}∞j=0 and {Kj}∞j=0, calculated by this method, converge to P∗d and K∗d, respectively. Also, a Schur matrix is denoted as Ad−BdKj for j=0,1,…. It should be noted that the method by Hewers is an offline algorithm which is dependent on system characteristics. To apply this method online for the HSA model (3.7)-(3.8), it will be constructed an output feedback ADP-based control method, which does not reliant on knowing of HSA matrices.
Remark 3.1. For Hurwitz feedback matrix A−BK, K∈Rm×n is called stabilizing feedback gain matrix for a linear system ˙x=Ax+Bu.
Inspired by [41,42], HSA model (3.7)-(3.8) may be developed employing inputs and outputs sequences on the time horizon [k−N,k−1] In the form of
xk=ANdxk−N+V(N)ˉuk−1,k−N,ˉyk−1,k−N=U(N)xk−N+T(N)ˉuk−1,k−N, | (3.14) |
where
ˉuk−1,k−N=[uTk−1uTk−2…uTk−N]T,ˉyk−1,k−N=[yTk−1yTk−2…yTk−N]T,V(N)=[BdAdBd…AN−1dBd],U(N)=[(CAN−1d)T(CAd)T…CT]T,T(N)=[0CBdCAdBd⋯CAN−2dBd00CBd⋯CAN−3dBd⋮⋮⋱⋱⋮00⋯0CBd00⋯00], |
and the observability index is N=max(ρu,ρv) in which ρu is the minimum integer which guarantees that U(ρu) is of full column rank and ρv is the minimal integer which assures that V(ρv) is of full row rank [41]. As a consequence, U(N) has a left inverse that is expressed as U+(N)=[UT(N)U(N)]−1UT(N). An ADP-based controller with output feedback can be used to deal with the optimal control issue of HSA. State reconstruction (3.14) is an essential part of this method, which uniqueness is expressed in the form of Lemma 3.2 [43].
Lemma 3.2. If the system's observability and controllability requirements (3.7)-(3.8) are satisfied, the states of HSA are uniquely obtained as a result of measurable inputs and outputs as shown below
xk=Θzk, | (3.15) |
where Θ=[MuMy] is full row rank, Mu=V(N)−MyT(N), My=ANdU+(N), zk=[ˉuTk−1,k−NˉyTk−1,k−N]T∈Rq, in which q=N[dim(u)+dim(y)].
According to (3.12)-(3.13), an online output feedback learning approach may be implemented in the form of u∗k=−¯Kdzk, resulting in a suboptimal feature of the closed-loop system. The discrete-time model (3.7) is shown below
xk+1=Ajxk+Bd(Kjxk+uk), | (3.16) |
where Aj=Ad−BdKj. Setting ˉKj=KjΘ and ˉPj=ΘTPjΘ, from (3.12) and (3.16) it can be obtained
zTk+1ˉPjzk+1−zTkˉPjzk=ϕ1Kvec(ˉH1j)+ϕ2Kvec(ˉH2j)−(yTkQyk+zTkˉKTjRˉKjzk), | (3.17) |
in which ˉH1j=BTdˉPjBd, ˉH2j=BTdˉPjAdΘ, ϕ1K=uTk⊗uTk−(zTk⊗zTk)(ˉKTj⊗ˉKTj), ϕ2K=2[(zTk⊗zTk)(Iq⊗ˉKTj)+(zTk⊗uTk)].
The rank condition written in the form of Lemma 3.3 ensures the convergence of the online output feedback learning control [42,44].
Lemma 3.3. Let us suppose that for a sufficiently large s∈Z+ it holds:
rank(Γ)=(dim(u)+dim(z))(dim(u)+dim(z)+1)/2, | (3.18) |
where
Γ=[ηk0⊗ηk0ηk1⊗ηk1⋯ηks⊗ηks], | (3.19) |
where k0<k1<⋯<ks∈Z+ and ηkj=[uTkj,zTkj]T, j=¯0,s, then (ˉPj,ˉH1j,ˉH2j) can be uniquely solved based on ˉKj and measurable online data during the period k∈[k0,ks]. Further, ˉKj+1 is obtained as follows
ˉKj+1=(R+ˉH1j)−1ˉH2j. | (3.20) |
To fulfill the condition of persistent excitation, some exploration noise ek must be included in the input signal without influencing the convergence of the online learning phase [45,46]. Applying the experimental design theory, a sum of sine waves with different frequencies is added to the input during the learning phase, which enables persistent excitation. Note that (3.17) is called policy evaluation, which is used to uniquely solve ˉPj, and (3.20) is policy improvement (PI), which is used to update control gain ˉKj+1. It should be noticed that solving (3.17) rather than (3.12), completely removes the initial need for precise knowledge of HSA dynamics. With the formulation for zk in mind, we can see that the control policy ˆuk=−¯K∗kzk involves exclusively the previous measurement of inputs and outputs.
Control law is designed as uk=ˆuk+ˆfa, where ˆfa is the fault compensation term suggested to prevent performance decline, which can be represented as ˆfa,k+1=la(2ˆukR−xTˆB))T. This fault approximation is employed to online compensate the effects of the actual actuator faults.
Consider the linear HSA (2.5)-(2.6) with actuator fault fa(t):
˙x(t)=Ax(t)+B(t)(u(t)−fa(t)), | (4.1) |
y(t)=Cx(t). | (4.2) |
The term fa(t) denotes an unknown additive actuator fault as:
fa(t)={0,t≤7 s,3,7 s≤t≤11 s,0,11 s≤t≤14 s,1.5,⋅sin(0.1π⋅(t−14))14 s≤t≤16 s,0,16 s≤t≤20 s, | (4.3) |
for the purpose of simulation. A comparative view of actual and estimated actuator fault, made up of a step and sine-type faults, is shown in Figure 3.
The weight matrices Q and R are set to be identity matrices, the observability index is N=3 and the initial state vector is x0=[−5,0,5] in order to illustrate the effectiveness of the proposed ADP-based control of HSA while 10−4 is chosen as the convergence threshold ε.
To illustrate the efficacy of the proposed ADP based FTC, the system input and output as well as system states of HSA, without the fault compensation are shown in Figure 4 and Figure 5.
As a result of the unknown actuator fault, the control law uk cannot provide system stability. It is clear that for good trajectory tracking, online compensation of the actuator fault must be included in the control low.
The system input and output of HSA as well as the system states under ADP-based control with fault compensation, are shown in Figure 6 and Figure 7. It is evident that the system states quickly reach equilibrium once a fault arises, which is used to learn the fault online.
In spite of the existence of system uncertainties and measurement noises, the tracking capabilities are good. It can be seen that despite the presence of system uncertainties and measurement noises, the tracking performances are good.
Finally, it can be shown that the designed controller has good flexibility and robustness. In order to further quantify the results of the comparison of the fault-tolerant controller using adaptive dynamic programming versus the ADP-based controller without fault compensation, the following three performance indices are given:
MSE=1NN∑i=1(yd(i)−y(i))2,IAE=N∑i=1|yd(i)−y(i)|,ITAE=N∑i=1|yd(i)−y(i)|i, | (4.4) |
The index MSE is used to evaluate the tracking accuracy. The index IAE can evaluate the overshoot of the whole process of the system. The index ITAE is employed to evaluate the speed of the transient response. The numerical results of these three indices are shown in Table 1.
Performance indices | ADP-based FTC | ADP-based controller |
MSE | 0.004 | 21.254 |
IAE | 12.548 | 486.018 |
ITAE | 914.515 | 6.163⋅104 |
For MSE, the proposed fault-tolerant controller based on ADP is about 99.98% lower than the ADP-based controller without fault compensation. For IAE, the proposed fault-tolerant controller based on ADP control is about 97.42% lower than the ADP-based controller without fault compensation. Moreover, for ITAE, the proposed fault-tolerant controller based on ADP is about 98.52% lower than the ADP-based controller without fault compensation. As a result, it can be obtained from the three indices in Table 1 that the proposed scheme can achieve better tracking performance with smaller tracking error, smaller overshoot and faster response than the ADP-based controller without fault compensation.
We develop a new data-driven FTC scheme for HSA with completely unknown dynamics and the presence of actuator faults based on ADP with an enhanced performance index. The provided FTC strategy has the primary benefit of avoiding knowledge of full system dynamics and faults using output feedback, the state reconstruction method and adaptive dynamic programming, which is extremely significant in real-world settings. As a result, data-driven FTC for discretized HSA was iteratively developed, and very efficiently ensures online solutions to control HSAs, which are regarded as highly nonlinear dynamic systems. Future research may involve event-triggered ADP-based control for industrial systems in order to reduce the interaction between the controller and the actuators.
The authors declare they have not used Artificial Intelligence (AI) tools in the creation of this article.
This research has been supported in part by the Serbian Ministry of Education, Science and Technological Development (451-03-47/2023-01/200108).
The authors declare that there are no conflicts of interest in this paper.
[1] | P. Werbos, Handbook of intelligent control: Neural, fuzzy, and adaptive approaches, In: White DA, Sofge DA, editors. Approximate dynamic programming for real-time control and neural modeling, New York: Van Nostrand Reinhold, 1992,493–525. |
[2] |
Q. Wei, D. Liu, H. Lin, Value iteration adaptive dynamic programming for optimal control of discrete-time nonlinear systems, IEEE Trans. Cybern., 46 (2016), 840–853. https://doi.org/10.1109/TCYB.2015.2492242 doi: 10.1109/TCYB.2015.2492242
![]() |
[3] |
X. Yang, D. Liu, Q. Wei, D. Wang, Guaranteed cost neural tracking control for a class of uncertain nonlinear systems using adaptive dynamic programming, Neurocomputing, 198 (2016), 80–90. https://doi.org/10.1016/j.neucom.2015.08.119 doi: 10.1016/j.neucom.2015.08.119
![]() |
[4] |
L. Dong, X. Zhong, C. Sun, H. He, Adaptive event-triggered control based on heuristic dynamic programming for nonlinear discrete-time systems, IEEE Trans. Neural Networks Learn. Syst., 28 (2016), 1594–1605. https://doi.org/10.1109/TNNLS.2016.2541020 doi: 10.1109/TNNLS.2016.2541020
![]() |
[5] |
Q. Sun, C. Zhang, N. Jiang, J. Yu, L. Xu, Data-driven nonlinear near-optimal regulation based on multi-dimensional Taylor network dynamic programming, IEEE Access, 8 (2020), 36476–36484. https://doi.org/10.1109/ACCESS.2020.2975391 doi: 10.1109/ACCESS.2020.2975391
![]() |
[6] |
Y. Jiang, Z. Jiang, Robust adaptive dynamic programming for large-scale systems with an application to multimachine power systems, IEEE T. CIRCUITS-II, 59 (2012), 693–697. https://doi.org/10.1109/TCSII.2012.2213353 doi: 10.1109/TCSII.2012.2213353
![]() |
[7] |
B. Zhao, D. Liu, C. Luo, Reinforcement learning-based optimal stabilization for unknown nonlinear systems subject to inputs with uncertain constraints, IEEE Trans. Neural Networks Learn. Syst., 31 (2020), 4330–4340. https://doi.org/10.1109/TNNLS.2019.2954983 doi: 10.1109/TNNLS.2019.2954983
![]() |
[8] |
D. Wang, D. Liu, H. Li, B. Luo, H. Ma, An approximate optimal control approach for robust stabilization of a class of discrete-time nonlinear systems with uncertainties, IEEE Trans. Syst. Man Cybern. Syst., 46 (2016), 713–717. https://doi.org/10.1109/TSMC.2015.2466191 doi: 10.1109/TSMC.2015.2466191
![]() |
[9] |
X. Yang, D. Liu, D. Wang, Reinforcement learning for adaptive optimal control of unknown continuous-time nonlinear systems with input constraints, Int. J. Control, 87 (2014), 553–566. https://doi.org/10.1080/00207179.2013.848292 doi: 10.1080/00207179.2013.848292
![]() |
[10] | H. Xia, B. Zhao, F. Zhou, B. Dong, G. Liu, Y. Li, Fault tolerant control for reconfigurable manipulators based on adaptive dynamic programming with an improved performance index function, In: 2016 12th World Congress on Intelligent Control and Automation (WCICA), Guilin, China. New Jersey: IEEE; (2016), 2273–2278. https://doi.org/10.1109/WCICA.2016.7578344 |
[11] |
X. Xiao, X. Li, Adaptive dynamic programming method-based synchronisation control of a class of complex dynamical networks with unknown dynamics and actuator faults, IET Control Theory Appl., 12 (2018), 291–298. https://doi.org/ 10.1049/iet-cta.2017.0845 doi: 10.1049/iet-cta.2017.0845
![]() |
[12] |
C. Chen, K. Xie, F.L. Lewis, S. Xie, A. Davoudi, Fully distributed resilience for adaptive exponential synchronization of heterogeneous multiagent systems against actuator faults, IEEE Trans. Automat. Contr., 64 (2019), 3347–3354. https://doi.org/10.1109/TAC.2018.2881148 doi: 10.1109/TAC.2018.2881148
![]() |
[13] |
C. Xie, G. Yang, Approximate guaranteed cost fault-tolerant control of unknown nonlinear systems with time-varying actuator faults, Nonlinear Dyn., 83 (2016), 269–282. https://doi.org/10.1007/s11071-015-2324-6 doi: 10.1007/s11071-015-2324-6
![]() |
[14] |
G. Dong, H. Li, H. Ma, R. Lu, Finite-time consensus tracking neural network FTC of multi-agent systems, IEEE Trans. Neural Networks Learn. Syst., 32 (2021), 653–662. https://doi.org/10.1109/TNNLS.2020.2978898 doi: 10.1109/TNNLS.2020.2978898
![]() |
[15] |
M. Benosman, K.Y. Lum, Passive actuators' fault-tolerant control for affine nonlinear systems, IEEE Trans. Control Syst. Technol., 18 (2010), 152–163. https://doi.org/10.1109/TCST.2008.2009641 doi: 10.1109/TCST.2008.2009641
![]() |
[16] |
V. Djordjevic, V. Stojanovic, D. Prsic, L. J. Dubonjic, M. M. Morato, Observer-based fault estimation in steer-by-wire vehicle, Engineering Today, 1 (2022), 7–17. https://doi.org/10.5937/engtoday2201007D doi: 10.5937/engtoday2201007D
![]() |
[17] |
W. Guo, A. Qiu, C. Wen, Active actuator fault tolerant control based on generalized internal model control and performance compensation, IEEE Access, 7 (2019), 175514–175521. https://doi.org/10.1109/ACCESS.2019.2957824 doi: 10.1109/ACCESS.2019.2957824
![]() |
[18] | Y. Fu, T. Chai, Nonlinear Adaptive Decoupling Control Based on Neural Networks and Multiple Models, International Journal of Innovative Computing, Information and Control, 8 (2012), 1867–1878. |
[19] |
N. Meskin, E. Naderi, K. Khorasani, A multiple model-based approach for fault diagnosis of jet engines, IEEE Trans. Control Syst. Technol., 21 (2011), 254–262. https://doi.org/10.1109/TCST.2011.2177981 doi: 10.1109/TCST.2011.2177981
![]() |
[20] | Z. Abbasfard, A. Baniamerian, K. Khorasani, Fault diagnosis of gas turbine engines: A symbolic multiple model approach, In: European Control Conference 2014, Strasbourg, France. New Jersey: IEEE; 2014,944–951. https://doi.org/10.1109/ECC.2014.6862358 |
[21] | D. Liu, D. Wang, D. Zhao, Adaptive dynamic programming for optimal control of unknown nonlinear discrete-time systems, In: IEEE Symposium on Adaptive Dynamic Programming and Reinforcement Learning 2011, Paris, France. New Jersey: IEEE; 2011,242–249. https://doi.org/10.1109/ADPRL.2011.5967357 |
[22] |
J. J. Murray, C. J. Cox, G. G. Lendaris, R. Saeks, Adaptive dynamic programming, IEEE Trans. Syst. Man Cybern. Syst., 32 (2002), 140–153. https://doi.org/10.1109/TSMCC.2002.801727 doi: 10.1109/TSMCC.2002.801727
![]() |
[23] |
F. Wang, H. Zhang, D. Liu, Adaptive dynamic programming: An introduction, IEEE Comput. Intell. Mag., 4 (2009), 39–47. https://doi.org/10.1109/MCI.2009.932261 doi: 10.1109/MCI.2009.932261
![]() |
[24] | Z. Jiang, Y. Jiang, Robust adaptive dynamic programming: recent results and applications, In: Chinese Control Conference 2013: Proceedings of the 32nd Chinese Control Conference, Xi'an, China. New Jersey: IEEE; 2013,968–973. |
[25] |
Q. Fan, G. Yang, Adaptive fault-tolerant control for affine non-linear systems based on approximate dynamic programming, IET Control Theory Appl., 10 (2016), 655–663. https://doi.org/10.1049/iet-cta.2015.1081 doi: 10.1049/iet-cta.2015.1081
![]() |
[26] |
D. Ye, T. Song, Decentralized reliable guaranteed cost control for large-scale nonlinear systems using actor-critic network, Neurocomputing, 320 (2018), 121–128. https://doi.org/10.1016/j.neucom.2018.09.029 doi: 10.1016/j.neucom.2018.09.029
![]() |
[27] |
B. Zhao, D. Liu, Y. Li, Observer based adaptive dynamic programming for fault tolerant control of a class of nonlinear systems, INFORM. SCIENCES, 384 (2017), 21–33. https://doi.org/10.1016/j.ins.2016.12.016 doi: 10.1016/j.ins.2016.12.016
![]() |
[28] |
Y. Li, K. Sun, S. Tong, Observer-based adaptive fuzzy fault-tolerant optimal control for SISO nonlinear systems, IEEE Trans. Cybern., 49 (2019), 649–661. https://doi.org/ 10.1109/TCYB.2017.2785801 doi: 10.1109/TCYB.2017.2785801
![]() |
[29] |
V. Stojanovic, N. Nedic, D. Prsic, L. J. Dubonjic, V. Djordjevic, Application of cuckoo search algorithm to constrained control problem of a parallel robot platform, Int. J. Adv. Manuf. Technol., 87 (2016), 2497–2507. https://doi.org/10.1007/s00170-016-8627-z doi: 10.1007/s00170-016-8627-z
![]() |
[30] | M. Jelali, A. Kroll, Hydraulic servo-systems: Modelling, identification and control, 1st ed., London: Springer, 2002. https://doi.org/10.1007/978-1-4471-0099-7 |
[31] | F. L. Lewis, D. Liu, Reinforcement learning and approximate dynamic programming for feedback control, 1st ed., New Jersey: John Wiley & Sons, 2013. |
[32] | P. J. Werbos, Beyond regression: New tools for prediction and analysis in the behavioral sciences, PhD thesis: Harvard University; 1974. |
[33] |
W. Gao, Z. Jiang, Learning-based adaptive optimal tracking control of strict-feedback nonlinear systems, IEEE Trans. Neural Networks Learn. Syst., 29 (2018), 2614–2624. https://doi.org/10.1109/TNNLS.2017.2761718 doi: 10.1109/TNNLS.2017.2761718
![]() |
[34] |
T. Bian, Z. Jiang, Value iteration and adaptive dynamic programming for data-driven adaptive optimal control design, AUTOMATICA, 71 (2016), 348–360. https://doi.org/10.1016/j.automatica.2016.05.003 doi: 10.1016/j.automatica.2016.05.003
![]() |
[35] | M. Tomás-Rodríguez, S. P. Banks, Linear, time-varying approximations to nonlinear dynamical systems: with applications in control and optimization, 1st ed., London: Springer, 2010. https://doi.org/10.1007/978-1-84996-101-1 |
[36] |
V. Stojanovic, D. Prsic, Robust identification for fault detection in the presence of non-Gaussian noises: Application to hydraulic servo drives, Nonlinear Dyn., 100 (2020), 2299–2313. https://doi.org/10.1007/s11071-020-05616-4 doi: 10.1007/s11071-020-05616-4
![]() |
[37] |
C. R. Rojas, J. C. Aguero, J. S. Welsh, G. C. Goodwin, A. Feuer, Robustness in experiment design, IEEE Trans. Automat. Contr., 57 (2011), 860–874. https://doi.org/10.1109/TAC.2011.2166294 doi: 10.1109/TAC.2011.2166294
![]() |
[38] | F. L. Lewis, D. Vrabie, V. L. Syrmos, Optimal control, 3rd ed. New Jersey: John Wiley & Sons; 2012. |
[39] | T. Chen, B. A. Francis, Optimal sampled-data control systems, 1st ed. London: Springer; 2012. |
[40] |
G. Hewer, An iterative technique for the computation of the steady state gains for the discrete optimal regulator, IEEE Trans. Automat. Contr., 16 (1971), 382–384. https://doi.org/10.1109/TAC.1971.1099755 doi: 10.1109/TAC.1971.1099755
![]() |
[41] |
F. L. Lewis, K. G. Vamvoudakis, Reinforcement learning for partially observable dynamic processes: Adaptive dynamic programming using measured output data, IEEE Trans. Syst. Man Cybern. Syst., 41 (2011), 14–25. https://doi.org/10.1109/TSMCB.2010.2043839 doi: 10.1109/TSMCB.2010.2043839
![]() |
[42] | W. Gao, Y. Jiang, Z. Jiang, T. Chai, Adaptive and optimal output feedback control of linear systems: An adaptive dynamic programming approach, In: World Congress on Intelligent Control and Automation 2014: Proceeding of the 11th World Congress on Intelligent Control and Automation; 2014 Jun 29- Jul 4; Shenyang, China. New Jersey: IEEE; 2014. pp. 2085–2090. https://doi.org/10.1109/WCICA.2014.7053043 |
[43] | W. Aangenent, D. Kostic, B. de Jager, R. VandeMolengraft, M. Steinbuch, Data-based optimal control, In: Foster JA, Lutton E, Miller J, Ryan C, Tettamanzi AG, editors. American Control Conference 2005: Proceedings of the 2005, American Control Conference, 2005; 2005 Apr 3-5; City, Country. Berlin: Publisher; 2005. pp. 1460–1465. https://doi.org/10.1109/ACC.2005.1470171 |
[44] | K. J. Åström, B. Wittenmark, Adaptive control, 2nd ed. New York: Dover Publications, 2008. |
[45] |
K. G. Vamvoudakis, F. L. Lewis, Multi-player non-zero-sum games: Online adaptive learning solution of coupled Hamilton–Jacobi equations, AUTOMATICA, 47 (2011), 1556–1569. https://doi.org/10.1016/j.automatica.2011.03.005 doi: 10.1016/j.automatica.2011.03.005
![]() |
[46] |
H. Xu, S. Jagannathan, F. L. Lewis, Stochastic optimal control of unknown linear networked control system in the presence of random delays and packet losses, AUTOMATICA, 48 (2012), 1017–1030. https://doi.org/10.1016/j.automatica.2012.03.007 doi: 10.1016/j.automatica.2012.03.007
![]() |
1. | Maria Letizia Corradini, Resilience enhancement to loss of actuator effectiveness in a Model-Free Adaptive framework, 2024, 361, 00160032, 106957, 10.1016/j.jfranklin.2024.106957 | |
2. | Zhiwen Fang, Jiaxin Zhang, Linhu Yao, Hongwei Wang, Hongli Wang, Improved spectral amplitude modulation for tacholess estimation of rotation speed, 2024, 236, 02632241, 115056, 10.1016/j.measurement.2024.115056 | |
3. | Rasananda Muduli, Debashisha Jena, Tukaram Moger, Automatic generation control of is-landed micro-grid using integral reinforcement learning-based adaptive optimal control strategy, 2024, 0948-7921, 10.1007/s00202-024-02648-6 | |
4. | Deshuai Zheng, Jin Yan, Tao Xue, Yong Liu, State-Dependent Maximum Entropy Reinforcement Learning for Robot Long-Horizon Task Learning, 2024, 110, 0921-0296, 10.1007/s10846-024-02049-8 | |
5. | Chienchang Chen, Hongjie Dai, Chunghong Lee, Tunghsien Hsieh, Weicheng Hung, Wenyuh Jywe, The development of thermal error compensation on CNC machine tools by combining ridge parameter selection and backward elimination procedure, 2024, 130, 0268-3768, 2423, 10.1007/s00170-023-12778-z | |
6. | Heqiang Tian, Jinchang An, Hongqiang Ma, Bin Tian, Trajectory Planning and Adaptive Fuzzy PID Control for Precision in Robotic Vertebral Plate Cutting: Addressing Force Dynamics and Deformation Challenges, 2024, 110, 1573-0409, 10.1007/s10846-024-02199-9 | |
7. | Rui Shao, Mingle Zhou, Min Li, Delong Han, Gang Li, TD-Net:tiny defect detection network for industrial products, 2024, 10, 2199-4536, 3943, 10.1007/s40747-024-01362-x | |
8. | Li Zhu, Wei Liu, Hanzhong Tan, Tao Hu, A novel Bayesian optimization prediction framework for four-axis industrial robot joint motion state, 2024, 10, 2199-4536, 4867, 10.1007/s40747-024-01425-z | |
9. | Keshab Patra, Arpita Sinha, Anirban Guha, Online Capability Based Task Allocation of Cooperative Manipulators, 2024, 110, 0921-0296, 10.1007/s10846-024-02050-1 | |
10. | Yanzhe Wang, Qian Yang, Weiwei Qu, A collision-free transition path planning method for placement robots in complex environments, 2024, 10, 2199-4536, 8481, 10.1007/s40747-024-01585-y | |
11. | Ziwen Gu, Yatao Shen, Zijian Wang, Jiayi Qiu, Wenmei Li, Chun Huang, Yaqun Jiang, Peng Li, Load forecasting model considering dynamic coupling relationships using structured dynamic-inner latent variables and broad learning system, 2024, 133, 09521976, 108180, 10.1016/j.engappai.2024.108180 | |
12. | Yong Peng, Junjie Zeng, Yue Hu, Qi Fang, Quanjun Yin, Reinforcement learning from suboptimal demonstrations based on Reward Relabeling, 2024, 255, 09574174, 124580, 10.1016/j.eswa.2024.124580 | |
13. | Gianluca Manduca, Gaspare Santaera, Marco Miraglia, Godfried Jansen Van Vuuren, Paolo Dario, Cesare Stefanini, Donato Romano, A Bioinspired Control Strategy Ensures Maneuverability and Adaptability for Dynamic Environments in an Underactuated Robotic Fish, 2024, 110, 1573-0409, 10.1007/s10846-024-02080-9 | |
14. | Yihui Hu, Shaopeng Hu, Xiaoyan Li, Shengli Cao, Huanchao Du, Dan Li, Supervisor synthesis for asynchronous diagnosability enforcement in labeled Petri nets, 2024, 677, 00200255, 120907, 10.1016/j.ins.2024.120907 | |
15. | Xiangbo Xu, Bo Ran, Nan Jiang, Lei Xu, Pan Huan, Xianwei Zhang, Zhe Li, A systematic review of ultrasonic techniques for defects detection in construction and building materials, 2024, 226, 02632241, 114181, 10.1016/j.measurement.2024.114181 | |
16. | Xinyi He, Chang Liu, Xiaodi Li, A practical leader–follower hybrid control scheme for wheeled mobile robots, 2024, 184, 09600779, 114954, 10.1016/j.chaos.2024.114954 | |
17. | Bolin Liao, Cheng Hua, Qian Xu, Xinwei Cao, Shuai Li, Inter-robot management via neighboring robot sensing and measurement using a zeroing neural dynamics approach, 2024, 244, 09574174, 122938, 10.1016/j.eswa.2023.122938 | |
18. | Ye Bai, Sheng-Jen Hsieh, A hybrid method using FABRIK and custom ANN in solving inverse kinematic for generic serial robot manipulator, 2024, 130, 0268-3768, 4883, 10.1007/s00170-023-12928-3 | |
19. | Yunjun Zheng, Jinchuan Zheng, Ke Shao, Han Zhao, Zhihong Man, Zhe Sun, Adaptive fuzzy sliding mode control of uncertain nonholonomic wheeled mobile robot with external disturbance and actuator saturation, 2024, 663, 00200255, 120303, 10.1016/j.ins.2024.120303 | |
20. | Adam Zielonka, Andrzej Sikora, Marcin Woźniak, Fuzzy rules intelligent car real-time diagnostic system, 2024, 135, 09521976, 108648, 10.1016/j.engappai.2024.108648 | |
21. | Gang Zou, Min Xia, Liudong Zhang, Zhen Lei, Zhiqiang Peng, Jun Liu, Intelligent identification of power grid parameters based on dynamic weighting, 2024, 135, 09521976, 108822, 10.1016/j.engappai.2024.108822 | |
22. | Tae Jong Choi, Nikhil Pachauri, Adaptive search space for stochastic opposition-based learning in differential evolution, 2024, 300, 09507051, 112172, 10.1016/j.knosys.2024.112172 | |
23. | Wei He, Xiang Wang, Mohammad Masoud Namazi, Wangping Zhou, Josep M. Guerrero, A reduced-order adaptive state observer for DC–DC converters with unknown constant power load, 2024, 143, 09670661, 105785, 10.1016/j.conengprac.2023.105785 | |
24. | Dezheng Hua, Xinhua Liu, Grzegorz M. Królczyk, Weihua Li, Darius Andriukaitis, Janusz Gołdasz, Zhixiong Li, A new industrially magnetic capsule MedRobot integrated with smart motion controller, 2024, 133, 0268-3768, 4561, 10.1007/s00170-024-13986-x | |
25. | S. P. Karthi, K. Kavitha, Detecting and Classifying Parametric Faults in Analog Circuits Using an Optimized Attention Neural Networks, 2024, 43, 0278-081X, 5401, 10.1007/s00034-024-02722-1 | |
26. | David Biagioni, Xiangyu Zhang, Christiane Adcock, Michael Sinner, Peter Graf, Jennifer King, Comparative analysis of grid-interactive building control algorithms: From model-based to learning-based approaches, 2024, 133, 09521976, 108498, 10.1016/j.engappai.2024.108498 | |
27. | Sijia Gu, Fei Zhu, BAGAIL: Multi-modal imitation learning from imbalanced demonstrations, 2024, 174, 08936080, 106251, 10.1016/j.neunet.2024.106251 | |
28. | Aijun Yin, Zhaoyi Sun, Junlin Zhou, Hypergraph construction using Multi-Sensor for helicopter Tail-Drive system fault diagnosis, 2024, 231, 02632241, 114586, 10.1016/j.measurement.2024.114586 | |
29. | Zishuo Dong, Xu Li, Feng Luan, Lingming Meng, Jingguo Ding, Dianhua Zhang, Fusion of theory and data-driven model in hot plate rolling: A case study of rolling force prediction, 2024, 245, 09574174, 123047, 10.1016/j.eswa.2023.123047 | |
30. | Swami Nath Maurya, Win-Jet Luo, Bivas Panigrahi, Prateek Negi, Pei-Tang Wang, Input attribute optimization for thermal deformation of machine-tool spindles using artificial intelligence, 2024, 0956-5515, 10.1007/s10845-024-02350-1 | |
31. | Jiaxiang Luo, Bozhe Xu, Xiangyang Li, Zhannan Yao, Autonomous Cooperative Hunting with Rule-Based and Self-Learning Control for Multiagent Systems, 2024, 110, 1573-0409, 10.1007/s10846-024-02177-1 | |
32. | Mohammadreza Izadi, Reza Faieghi, High-gain disturbance observer for robust trajectory tracking of quadrotors, 2024, 145, 09670661, 105854, 10.1016/j.conengprac.2024.105854 | |
33. | Hoijo Jeong, Jinyoung Suk, Seungkeun Kim, Control of quadrotor UAV using variable disturbance observer-based strategy, 2024, 150, 09670661, 105990, 10.1016/j.conengprac.2024.105990 | |
34. | Zhonglin Lin, Yufeng Li, Qiang Qian, Feng Huang, Xinglong Zhang, Tianhong Zhang, Weixiong Wang, Construction of an improved semi-physical simulation system for UAV with integrated energy-consumption prediction model and its evaluation of the path planning algorithms in mountainous scenery, 2024, 197, 03608352, 110601, 10.1016/j.cie.2024.110601 | |
35. | Lingli Cui, Zhichao Jiang, Dongdong Liu, Huaqing Wang, A novel adaptive generalized domain data fusion-driven kernel sparse representation classification method for intelligent bearing fault diagnosis, 2024, 247, 09574174, 123225, 10.1016/j.eswa.2024.123225 | |
36. | Jintao Hu, Min Chen, Hailong Tang, Jiyuan Zhang, An adversarial transfer learning method based on domain distribution prediction for aero-engine fault diagnosis, 2024, 133, 09521976, 108287, 10.1016/j.engappai.2024.108287 | |
37. | Haipeng Wang, Application of new features based on artificial intelligent robot technology in medium-scale urban design pedigree and intelligent management and control, 2024, 22, 26673053, 200379, 10.1016/j.iswa.2024.200379 | |
38. | Sajad Rafiee, Mohammadrasoul Kankashvar, Hossein Bolandi, Output feedback fault-tolerant Q-learning for discrete-time linear systems with actuator faults, 2024, 138, 09521976, 109355, 10.1016/j.engappai.2024.109355 | |
39. | Yuan Wang, Zhenbin Du, Yanming Wu, Pseudo-partial-derivative information-driven adaptive fault-tolerant tracking control for discrete-time systems, 2024, 10, 2199-4536, 2531, 10.1007/s40747-023-01280-4 | |
40. | Bo Dong, Zhendong Ding, Tianjiao An, Yiming Cui, Xinye Zhu, Integral reinforcement learning-based event-triggered optimal tracking control for modular robot manipulators via non-zero-sum game, 2024, 35, 0957-0233, 096205, 10.1088/1361-6501/ad50f8 | |
41. | Yongwei Zhang, Shunchao Zhang, Integral sliding mode-based event-triggered optimal fault tolerant tracking control of continuous-time nonlinear systems, 2024, 79, 09473580, 101021, 10.1016/j.ejcon.2024.101021 | |
42. | Zhiqiang Zhang, Yuxiang Shen, Shuiqing Xu, Unsupervised learning of part-based representations using sparsity optimized auto-encoder for machinery fault diagnosis, 2024, 145, 09670661, 105871, 10.1016/j.conengprac.2024.105871 | |
43. | Weimin Zhai, Weiming Fu, Jiahu Qin, Qichao Ma, Yu Kang, A novel approach based on spatio-temporal attention and multi-scale modeling for mechanical failure prediction, 2024, 147, 09670661, 105938, 10.1016/j.conengprac.2024.105938 | |
44. | Ning Liu, Shangkun Liu, Wei-Min Zheng, PPSO and Bayesian game for intrusion detection in WSN from a macro perspective, 2024, 10, 2199-4536, 7645, 10.1007/s40747-024-01553-6 | |
45. | Aditi Gupta, Adeiza J. Onumanyi, Satyadev Ahlawat, Yamuna Prasad, Virendra Singh, Adnan M. Abu-Mahfouz, DAT: A robust Discriminant Analysis-based Test of unimodality for unknown input distributions, 2024, 182, 01678655, 125, 10.1016/j.patrec.2024.04.021 | |
46. | Ruben Kins, Christian Möbitz, Thomas Gries, Towards autonomous learning and optimisation in textile production: data-driven simulation approach for optimiser validation, 2024, 0956-5515, 10.1007/s10845-024-02405-3 | |
47. | Zhuoran Li, Kazem Chamran, Mustafa Muwafak Alobaedy, Muhammad Aman Sheikh, Tahir Siddiqui, Abdul Ahad, An Optimization on 2D-SLAM Map Construction Algorithm Based on LiDAR, 2024, 110, 1573-0409, 10.1007/s10846-024-02123-1 | |
48. | Linhui Zhao, Xin Zhao, Huaiyu Li, Rack force fault tolerance estimation of steer-by-wire system under different resolver faults based on sensor flows, 2024, 147, 09670661, 105941, 10.1016/j.conengprac.2024.105941 | |
49. | Alaaddin Aydın, Selda Güney, Fault detection system for paper cup machine based on real-time image processing, 2024, 133, 09521976, 108070, 10.1016/j.engappai.2024.108070 | |
50. | Tin-Chih Toly Chen, Yu-Cheng Wang, Evaluating innovative future robotic applications in manufacturing using a fuzzy collaborative intelligence approach, 2024, 130, 0268-3768, 6027, 10.1007/s00170-024-13046-4 | |
51. | Ying Li, Lijie Zhang, Siyuan Liu, Xiangfeng Wang, Chenghang Sun, Pengfei Liang, Xiaoming Yuan, A novel interpretable semi-supervised graph learning model for intelligent fault diagnosis of hydraulic pumps, 2024, 305, 09507051, 112598, 10.1016/j.knosys.2024.112598 | |
52. | Ding Wang, Hongyu Ma, Junfei Qiao, Multilayer adaptive critic design with digital twin for data-driven optimal tracking control and industrial applications, 2024, 133, 09521976, 108228, 10.1016/j.engappai.2024.108228 | |
53. | De-Yu Weng, Jun-Wei Zhu, Qi Xuan, Dirichlet probability navigated fault detection via key-group memory auto-encoder under non-stationary working conditions, 2024, 670, 00200255, 120635, 10.1016/j.ins.2024.120635 | |
54. | Onuchukwu Godwin Chike, Norhayati Ahmad, Wan Fahmin Faiz Wan Ali, Neural network prediction of thermal field spatiotemporal evolution during additive manufacturing: an overview, 2024, 134, 0268-3768, 2107, 10.1007/s00170-024-14256-6 | |
55. | Marcelo Luis Ruiz-Rodríguez, Sylvain Kubler, Jérémy Robert, Yves Le Traon, Dynamic maintenance scheduling approach under uncertainty: Comparison between reinforcement learning, genetic algorithm simheuristic, dispatching rules, 2024, 248, 09574174, 123404, 10.1016/j.eswa.2024.123404 | |
56. | Rafael A. Cordeiro, José R. Azinheira, Alexandra Moutinho, A pair of two-stage Kalman filters to detect and isolate failures in aircraft with redundant actuators, 2024, 147, 09670661, 105909, 10.1016/j.conengprac.2024.105909 | |
57. | Ziyuan Wang, Chengzhu Wang, Yonggang Li, Variational autoencoder based on knowledge sharing and correlation weighting for process-quality concurrent fault detection, 2024, 133, 09521976, 108051, 10.1016/j.engappai.2024.108051 | |
58. | Junting Lin, Xiaohui Qiu, Maolin Li, Tracking interval control for urban rail trains based on safe reinforcement learning, 2024, 137, 09521976, 109226, 10.1016/j.engappai.2024.109226 | |
59. | Xiaofeng Wang, Jihong Yan, Deep learning based multi-source heterogeneous information fusion framework for online monitoring of surface quality in milling process, 2024, 133, 09521976, 108043, 10.1016/j.engappai.2024.108043 | |
60. | Zhangbao Xu, Jialin Ren, Xin Zhou, Jianyong Yao, Adaptive prescribed performance output feedback control for full-state-constrained DC motors subject to uncertainties and input saturation, 2024, 77, 09473580, 100961, 10.1016/j.ejcon.2024.100961 | |
61. | Wenle Wang, Qilong Li, Fuwen Zhu, Association rules combined fuzzy decision quality control technology in intelligent manufacturing, 2024, 21, 26673053, 200331, 10.1016/j.iswa.2024.200331 | |
62. | Yanxiang Feng, Sida Ren, Xiaoling Li, Ye Cao, Yikang Yang, Robust supervisory control for automated manufacturing systems with unreliable resources by analyzing reachable state space, 2024, 664, 00200255, 120258, 10.1016/j.ins.2024.120258 | |
63. | Ding Wang, Hongyu Ma, Jin Ren, Ning Gao, Junfei Qiao, Adaptive critic design with weight allocation for intelligent learning control of wastewater treatment plants, 2024, 133, 09521976, 108284, 10.1016/j.engappai.2024.108284 | |
64. | Yuzhu Huang, Zhaoyan Zhang, Xiong Yang, Backstepping based neural H∞ optimal tracking control for nonlinear state constrained systems with input delay and disturbances, 2024, 595, 09252312, 127869, 10.1016/j.neucom.2024.127869 | |
65. | Sheng Huang, Intelligent device recognition of internet of things based on machine learning, 2024, 22, 26673053, 200368, 10.1016/j.iswa.2024.200368 | |
66. | Youness Boutyour, Abdellah Idrissi, Dynamic confidence-based constraint adjustment in distributional constrained policy optimization: enhancing supply chain management through adaptive reinforcement learning, 2024, 0956-5515, 10.1007/s10845-024-02492-2 | |
67. | Huiling Wang, Jun Sun, Xiaofeng Gu, Zunhao Hu, Chao Zhou, Weakly-supervised auto-encoder via energy regularization and soft multi-label learning on k labeled samples, 2024, 585, 09252312, 127596, 10.1016/j.neucom.2024.127596 | |
68. | Abhishek Kumar, Sumit Patil, Ahmed Kovacevic, Sathiskumar Anusuya Ponnusami, Performance prediction and Bayesian optimization of screw compressors using Gaussian Process Regression, 2024, 133, 09521976, 108270, 10.1016/j.engappai.2024.108270 | |
69. | Arindam Sarkar, Moirangthem Marjit Singh, Hanjabam Saratchandra Sharma, Artificial recurrent neural network coordinated secured transmission towards safeguarding confidentiality in smart Industrial Internet of Things, 2024, 1868-8071, 10.1007/s13042-024-02310-4 | |
70. | Maham Zafar, Kashif Kifayat, Ammara Gul, Usman Tahir, Sarah Abu Ghazalah, Complex networks after centrality-based attacks and defense, 2024, 10, 2199-4536, 3907, 10.1007/s40747-024-01354-x | |
71. | Haoming Zou, Guoshan Zhang, Zhiguo Yan, Wanquan Liu, Dynamic event-triggered finite-horizon robust suboptimal control of multi-player systems with input disturbances, 2025, 611, 09252312, 128665, 10.1016/j.neucom.2024.128665 | |
72. | Jue Wang, Huihui Pan, Weichao Sun, Event-Triggered Adaptive Output Constraint Tracking Control of Uncertain MIMO Nonlinear Systems With Sensor and Actuator Faults, 2024, 21, 1545-5955, 6774, 10.1109/TASE.2023.3330966 | |
73. | Siwei Qiao, Xinghua Liu, Dianhui Wang, Shuzhi Sam Ge, Security concern and fuzzy output sliding mode load frequency control of power systems, 2024, 676, 00200255, 120793, 10.1016/j.ins.2024.120793 | |
74. | Yingqiang Liu, Zheng Chen, Bin Yao, Online Optimization-Based Time-Optimal Adaptive Robust Control of Linear Motors With Input and State Constraints, 2024, 29, 1083-4435, 3157, 10.1109/TMECH.2024.3404821 | |
75. | Julio Yuzo Yassuda, Cristiano Marcos Agulhari, Emerson Ravazzi Pires da Silva, Sampled-data robust control of a 2-DoF helicopter modeled using a quasi-LPV framework, 2024, 145, 09670661, 105870, 10.1016/j.conengprac.2024.105870 | |
76. | Kazuki Takahashi, Tomoki Fukai, Yutaka Sakai, Takashi Takekawa, Goal-oriented inference of environment from redundant observations, 2024, 174, 08936080, 106246, 10.1016/j.neunet.2024.106246 | |
77. | Youming Wang, Lisha Chen, A multi-scale spatial–temporal capsule network based on sequence encoding for bearing fault diagnosis, 2024, 10, 2199-4536, 6189, 10.1007/s40747-024-01462-8 | |
78. | Wenxin Dai, Xue Zhou, Zhigang Sun, Guofu Zhai, Series alternating current arc fault detection method based on relative position matrix and deep convolutional neural network, 2024, 136, 09521976, 108874, 10.1016/j.engappai.2024.108874 | |
79. | Peng Wu, Gongye Yu, Qianqian Yu, Pengqi Wang, Yongming Han, Bo Ma, An adaptive few-shot fault diagnosis method based on virtual samples generated by fault characteristics of rotating machines, 2024, 136, 09521976, 109017, 10.1016/j.engappai.2024.109017 | |
80. | Aleksandra Loskutova, Daniel Roozbahani, Marjan Alizadeh, Heikki Handroos, Design and Development of a Robust Control Platform for a 3-Finger Robotic Gripper Using EMG-Derived Hand Muscle Signals in NI LabVIEW, 2024, 110, 1573-0409, 10.1007/s10846-024-02160-w | |
81. | Zhenguo Zhang, Tianhao Ma, Yadan Zhao, Shuai Yu, Fan Zhou, Adaptive dynamic programming-based multi-fault tolerant control of reconfigurable manipulator with input constraint, 2024, 10, 2199-4536, 8341, 10.1007/s40747-024-01550-9 | |
82. | Zigang Deng, Hongfu Shi, Yihao Chen, Zhihao Ke, Le Liang, Xin Liu, Kaiwen Li, Weihua Zhang, A cost-effective linear propulsion system featuring PMEDW for HTS maglev vehicle: design, implementation, and dynamic test, 2025, 240, 02632241, 115618, 10.1016/j.measurement.2024.115618 | |
83. | Xin Zhang, Tao Yang, Hao Long, Haiyang Shi, Jiaxu Wang, Laihao Yang, Fisher discrimination multiple kernel dictionary learning for robust identification of nonlinear features in machinery health monitoring, 2024, 677, 00200255, 120862, 10.1016/j.ins.2024.120862 | |
84. | Tayyab Ashfaq, Sidra Mumtaz, Saghir Ahmad, Basharat Ullah, Fahad R. Albogamy, Automatic Generation Control in Renewables-Integrated Multi-Area Power Systems: A Comparative Control Analysis, 2024, 16, 2071-1050, 5735, 10.3390/su16135735 | |
85. | Zaid Allal, Hassan N. Noura, Flavien Vernier, Ola Salman, Khaled Chahine, Wind turbine fault detection and identification using a two-tier machine learning framework, 2024, 22, 26673053, 200372, 10.1016/j.iswa.2024.200372 | |
86. | Zitao Chen, Kairui Chen, Ruizhi Tang, Optimal synchronization with L2-gain performance: An adaptive dynamic programming approach, 2024, 179, 08936080, 106566, 10.1016/j.neunet.2024.106566 | |
87. | Camilo Andrés Gonzalez Olier, Heriberto Enrique Maury Ramírez, Leidy Milena Mora Higuera, A novel methodology for the selection of the optimal velocity profile for planned point-to-point trajectories in 1-DoF manipulators, 2024, 134, 0268-3768, 2051, 10.1007/s00170-024-14141-2 | |
88. | Qingzong Li, Pingyu Jiang, Jianwei Wang, Maolin Yang, Yuqian Yang, A kind of intelligent dynamic industrial event knowledge graph and its application in process stability evaluation, 2024, 0956-5515, 10.1007/s10845-024-02325-2 | |
89. | Chunhua Zhao, Baoping Tang, Lei Deng, Yi Huang, Hao Tan, Global probability distribution structure-sparsity filter pruning for edge fault diagnosis in resource constrained wireless sensor networks, 2024, 133, 09521976, 108319, 10.1016/j.engappai.2024.108319 | |
90. | Caio Filipe de Lima Munguba, Alvaro Antonio Villa Ochoa, Gustavo de Novaes Pires Leite, Alexandre Carlos Araújo da Costa, José Ângelo Peixoto da Costa, Frederico Duarte de Menezes, Evandro Pedro Alves de Mendonça, Leonardo José de Petribú Brennand, Olga de Castro Vilela, Marrison Gabriel Guedes de Souza, Fault detection framework in wind turbine pitch systems using machine learning: Development, validation, and results, 2024, 138, 09521976, 109307, 10.1016/j.engappai.2024.109307 | |
91. | Jianying Li, Hailong Yang, Hui Ji, Characterization of Two-Cylinder Parallel Electro-hydraulic Force/Position Synchronization Based on RBF Fuzzy Neural Network Control, 2024, 1562-2479, 10.1007/s40815-024-01846-5 | |
92. | Shanke Li, Kun Peng, Fei Hui, Ziqi Li, Cheng Wei, Wenbo Wang, A Decision-Making Approach for Complex Unsignalized Intersection by Deep Reinforcement Learning, 2024, 73, 0018-9545, 16134, 10.1109/TVT.2024.3408917 | |
93. | Zuoyi Chen, Hong-Zhong Huang, Jun Wu, Yuanhang Wang, Zero-faulty sample machinery fault detection via relation network with out-of-distribution data augmentation, 2025, 141, 09521976, 109753, 10.1016/j.engappai.2024.109753 | |
94. | Ghofrane Benarfa, Ali Amamou, Sousso Kelouwani, Marie Hébert, Lotfi Zeghmi, Samir Jemei, Online health-aware energy management strategy of a fuel cell hybrid autonomous mobile robot under startup–shutdown condition, 2025, 266, 09574174, 125943, 10.1016/j.eswa.2024.125943 | |
95. | Roland Bolboacă, Piroska Haller, Bela Genge, Feature analysis and ensemble-based fault detection techniques for nonlinear systems, 2024, 0941-0643, 10.1007/s00521-024-10551-1 | |
96. | Zhenchao Hu, Jinwei Chen, Yuanfu Li, Jinzhi Lu, Huisheng Zhang, Dimitris Kiritsis, Product complexity management enabled by a model-based approach, 2024, 03608352, 110776, 10.1016/j.cie.2024.110776 | |
97. | Xiaokang Wang, Siqi Jiang, Xinghan Li, Mozhu Wang, Causal discovery and fault diagnosis based on mixed data types for system reliability modeling, 2025, 11, 2199-4536, 10.1007/s40747-024-01740-5 | |
98. | Yan-Lin He, Zi-Yang Lu, Qun-Xiong Zhu, Novel Manifold Autoencoder for Industrial Process Fault Diagnosis, 2025, 21, 1551-3203, 858, 10.1109/TII.2024.3465597 | |
99. | Rasananda Muduli, Debashisha Jena, Tukaram Moger, Application of Reinforcement Learning-Based Adaptive PID Controller for Automatic Generation Control of Multi-Area Power System, 2025, 22, 1545-5955, 1057, 10.1109/TASE.2024.3359219 | |
100. | Tuan Anh Nguyen, Development of a Novel Integrated Control Strategy for Automotive Electric Power Steering Systems, 2025, 22, 1545-5955, 926, 10.1109/TASE.2024.3356509 | |
101. | Suhuan Zhang, Fanglai Zhu, Xufeng Ling, Event-triggered UIO-based security control for discrete-time systems under deception attacks, 2025, 00200255, 121902, 10.1016/j.ins.2025.121902 | |
102. | Lindokuhle J. Mpanza, Jimoh O. Pedro, Comparing passive and active fault-tolerant control of swashplate actuator faults in a medium-scale rotorcraft unmanned aerial vehicle with experimental validation, 2025, 145, 09521976, 110163, 10.1016/j.engappai.2025.110163 | |
103. | Hean Hua, Yaonan Wang, Hang Zhong, Hui Zhang, Yongchun Fang, A Novel Guided Deep Reinforcement Learning Tracking Control Strategy for Multirotors, 2025, 22, 1545-5955, 2062, 10.1109/TASE.2024.3374752 | |
104. | Guhui Li, Zidong Wang, Xingzhen Bai, Zhongyi Zhao, Hongli Dong, Event-Triggered Set-Membership Filtering for Active Power Distribution Systems Under Fading Channels: A Zonotope-Based Approach, 2025, 22, 1545-5955, 1139, 10.1109/TASE.2024.3360600 | |
105. | Ding Wang, Jiangyu Wang, Derong Liu, Junfei Qiao, General multi-step value iteration for optimal learning control, 2025, 175, 00051098, 112168, 10.1016/j.automatica.2025.112168 | |
106. | Shuangyi Hu, Qiang Chen, Xuemei Ren, Shubo Wang, Adaptive Predefined-Time Synchronization and Tracking Control for Multimotor Driving Servo Systems, 2025, 30, 1083-4435, 494, 10.1109/TMECH.2024.3398695 | |
107. | Zhikai Yao, Xianglong Liang, Shuping Wang, Jianyong Yao, Model-Data Hybrid Driven Control of Hydraulic Euler–Lagrange Systems, 2025, 30, 1083-4435, 131, 10.1109/TMECH.2024.3390129 | |
108. | Zheng Lian, Zhijie Zhou, Changhua Hu, You Cao, Shuaiwen Tang, Yijie Sun, Transparent Fault Diagnosis for Complex Equipment Considering Expert Reliability Based on Belief Rule Base and Linguistic Z-Number, 2025, 22, 1545-5955, 3887, 10.1109/TASE.2024.3402099 | |
109. | Jiaxin Li, Maosong Cheng, Yongbo Wei, Zhimin Dai, FPGA implementation of edge-side motor fault diagnosis using a Kalman filter-based empirical mode decomposition algorithm, 2025, 159, 09670661, 106312, 10.1016/j.conengprac.2025.106312 | |
110. | Tobias Demmer, Daniel Lichte, Riccardo Patriarca, Kai-Dietrich Wolf, Infrastructure Resilience and Cybernetics: A Dead-Time Controller Method to Managing Disruptions, 2025, 09518320, 111066, 10.1016/j.ress.2025.111066 | |
111. | Haojun Zhong, Zhenlei Wang, Yuzhe Hao, A goal-conditioned offline reinforcement learning algorithm and its application to quad-rotors, 2025, 152, 09521976, 110678, 10.1016/j.engappai.2025.110678 | |
112. | Chujian Zeng, Bo Zhao, Derong Liu, Improved cost function-based fault tolerant control for nonlinear systems with simultaneous faults, 2025, 638, 09252312, 130125, 10.1016/j.neucom.2025.130125 | |
113. | Bingchang Hou, Yu Wang, Dong Wang, Cycle-embedded sparsity measures as a generalized objective function of impulsive mode decomposition for impulsive fault component extraction, 2025, 231, 08883270, 112566, 10.1016/j.ymssp.2025.112566 | |
114. | Mohammadrasoul Kankashvar, Sajad Rafiee, Hossein Bolandi, Fault-tolerant Q-learning for discrete-time linear systems with actuator and sensor faults using input-output measured data, 2025, 27731863, 100259, 10.1016/j.fraope.2025.100259 |
Performance indices | ADP-based FTC | ADP-based controller |
MSE | 0.004 | 21.254 |
IAE | 12.548 | 486.018 |
ITAE | 914.515 | 6.163⋅104 |