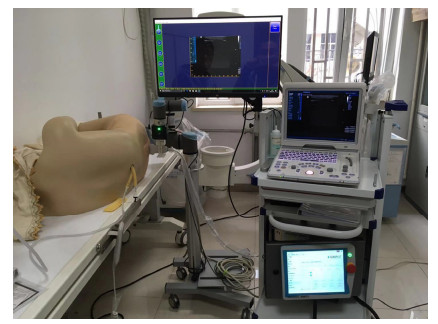
Listeria monocytogenes is a concern in seafood and its food processing environment (FPE). Several outbreaks globally have been linked to various types of seafood. Genetic profiling of L. monocytogenes is valuable to track bacterial contamination throughout the FPE and in understanding persistence mechanisms, with limited studies from South Africa. Forty-six L. monocytogenes isolates from origins: Fish/seafood products (n = 32) (salmon, smoked trout, fresh hake, oysters), the FPE (n = 6), and clinical (n = 8) were included in this study. Lineage typing, antibiotic susceptibility testing, and screening for two genes (bcrABC and emrC) conferring sanitizer tolerance was conducted. The seafood and FPE isolates originated from seven different factories processing various seafood products with undetermined origin. All clinical isolates were categorized as lineage I, and seafood and FPE isolates were mostly categorized into lineage II (p < 0.01). Seafood and FPE isolates (53%) carried the bcrABC gene cassette and one fish isolate, the emrC gene. A subset, n = 24, was grouped into serotypes, sequence types (STs), and clonal complexes (CCs) with whole genome sequencing (WGS). Eight CCs and ten STs were identified. All clinical isolates belonged to serogroup 4b, hypervirulent CC1. CC121 was the most prevalent in isolates from food and the FPE. All isolates carried Listeria pathogenicity islands (LIPI) 1 and 2. LIPI-3 and LIPI-4 were found in certain isolates. We identified genetic determinants linked to enhanced survival in the FPE, including stress survival islets (SSI) and genes conferring tolerance to sanitizers. SSI-1 was found in 44% isolates from seafood and the FPE. SSI-2 was found in all the ST121 seafood isolates. Isolates (42%) harbored transposon Tn1688_qac (ermC), conferring tolerance to quaternary ammonium compounds. Five plasmids were identified in 13 isolates from seafood and the FPE. This is the first One Health study reporting on L. monocytogenes genetic diversity, virulence and resistance profiles from various types of seafood and its FPE in South Africa.
Citation: Karlene Lambrechts, Pieter Gouws, Diane Rip. Genetic diversity of Listeria monocytogenes from seafood products, its processing environment, and clinical origin in the Western Cape, South Africa using whole genome sequencing[J]. AIMS Microbiology, 2024, 10(3): 608-643. doi: 10.3934/microbiol.2024029
[1] | Xiaoming Liu, Zhonghan Liu, Yaqin Pan, Yufeng Huang, Desheng Wu, Zhaoyu Ba . Full-endoscopic transforaminal procedure to treat the single-level adjacent segment disease after posterior lumbar spine fusion: 1–2 years follow-up. Mathematical Biosciences and Engineering, 2019, 16(6): 7829-7838. doi: 10.3934/mbe.2019393 |
[2] | Wei-wei Jiang, Guang-quan Zhou, Ka-Lee Lai, Song-yu Hu, Qing-yu Gao, Xiao-yan Wang, Yong-ping Zheng . A fast 3-D ultrasound projection imaging method for scoliosis assessment. Mathematical Biosciences and Engineering, 2019, 16(3): 1067-1081. doi: 10.3934/mbe.2019051 |
[3] | Kai Cheng, Lixia Li, Yanmin Du, Jiangtao Wang, Zhenghua Chen, Jian Liu, Xiangsheng Zhang, Lin Dong, Yuanyuan Shen, Zhenlin Yang . A systematic review of image-guided, surgical robot-assisted percutaneous puncture: Challenges and benefits. Mathematical Biosciences and Engineering, 2023, 20(5): 8375-8399. doi: 10.3934/mbe.2023367 |
[4] | Wei-wei Jiang, Xin-xin Zhong, Guang-quan Zhou, Qiu Guan, Yong-ping Zheng, Sheng-yong Chen . An automatic measurement method of spinal curvature on ultrasound coronal images in adolescent idiopathic scoliosis. Mathematical Biosciences and Engineering, 2020, 17(1): 776-788. doi: 10.3934/mbe.2020040 |
[5] | Biao Cai, Qing Xu, Cheng Yang, Yi Lu, Cheng Ge, Zhichao Wang, Kai Liu, Xubin Qiu, Shan Chang . Spine MRI image segmentation method based on ASPP and U-Net network. Mathematical Biosciences and Engineering, 2023, 20(9): 15999-16014. doi: 10.3934/mbe.2023713 |
[6] | Yongde Zhang, Qihang Yuan, Hafiz Muhammad Muzzammil, Guoqiang Gao, Yong Xu . Image-guided prostate biopsy robots: A review. Mathematical Biosciences and Engineering, 2023, 20(8): 15135-15166. doi: 10.3934/mbe.2023678 |
[7] | Won Man Park, Young Joon Kim, Shaobai Wang, Yoon Hyuk Kim, Guoan Li . Investigation of lumbar spine biomechanics using global convergence optimization and constant loading path methods. Mathematical Biosciences and Engineering, 2020, 17(4): 2970-2983. doi: 10.3934/mbe.2020168 |
[8] | Liwei Deng, Zhen Liu, Tao Zhang, Zhe Yan . Study of visual SLAM methods in minimally invasive surgery. Mathematical Biosciences and Engineering, 2023, 20(3): 4388-4402. doi: 10.3934/mbe.2023203 |
[9] | Tong Sun, Xin Zeng, Penghui Hao, Chien Ting Chin, Mian Chen, Jiejie Yan, Ming Dai, Haoming Lin, Siping Chen, Xin Chen . Optimization of multi-angle Magneto-Acousto-Electrical Tomography (MAET) based on a numerical method. Mathematical Biosciences and Engineering, 2020, 17(4): 2864-2880. doi: 10.3934/mbe.2020161 |
[10] | Yali Ouyang, Zhuhuang Zhou, Weiwei Wu, Jin Tian, Feng Xu, Shuicai Wu, Po-Hsiang Tsui . A review of ultrasound detection methods for breast microcalcification. Mathematical Biosciences and Engineering, 2019, 16(4): 1761-1785. doi: 10.3934/mbe.2019085 |
Listeria monocytogenes is a concern in seafood and its food processing environment (FPE). Several outbreaks globally have been linked to various types of seafood. Genetic profiling of L. monocytogenes is valuable to track bacterial contamination throughout the FPE and in understanding persistence mechanisms, with limited studies from South Africa. Forty-six L. monocytogenes isolates from origins: Fish/seafood products (n = 32) (salmon, smoked trout, fresh hake, oysters), the FPE (n = 6), and clinical (n = 8) were included in this study. Lineage typing, antibiotic susceptibility testing, and screening for two genes (bcrABC and emrC) conferring sanitizer tolerance was conducted. The seafood and FPE isolates originated from seven different factories processing various seafood products with undetermined origin. All clinical isolates were categorized as lineage I, and seafood and FPE isolates were mostly categorized into lineage II (p < 0.01). Seafood and FPE isolates (53%) carried the bcrABC gene cassette and one fish isolate, the emrC gene. A subset, n = 24, was grouped into serotypes, sequence types (STs), and clonal complexes (CCs) with whole genome sequencing (WGS). Eight CCs and ten STs were identified. All clinical isolates belonged to serogroup 4b, hypervirulent CC1. CC121 was the most prevalent in isolates from food and the FPE. All isolates carried Listeria pathogenicity islands (LIPI) 1 and 2. LIPI-3 and LIPI-4 were found in certain isolates. We identified genetic determinants linked to enhanced survival in the FPE, including stress survival islets (SSI) and genes conferring tolerance to sanitizers. SSI-1 was found in 44% isolates from seafood and the FPE. SSI-2 was found in all the ST121 seafood isolates. Isolates (42%) harbored transposon Tn1688_qac (ermC), conferring tolerance to quaternary ammonium compounds. Five plasmids were identified in 13 isolates from seafood and the FPE. This is the first One Health study reporting on L. monocytogenes genetic diversity, virulence and resistance profiles from various types of seafood and its FPE in South Africa.
As a minimally invasive interventional surgery, percutaneous puncture has the advantages of minimal trauma, fast recovery and strong targeting [1], and it is widely used in human tissue biopsies and drug-directed treatment. Among the various procedures, lumbar puncture is often used for the diagnosis of various central nervous system inflammatory diseases, vascular diseases, spinal cord diseases, intracranial-space-occupying diseases, unknown nervous system diseases and spinal angiography. In addition, lumbar puncture is also a common clinical diagnosis and treatment method for drug injection treatment and decompression due to the high cerebrospinal fluid pressure caused by central nervous system diseases [2,3].
In traditional lumbar puncture surgery, doctors use medical imaging equipment and their clinical experience to determine the location of the needle puncture point on the skin. Medical imaging mainly includes computed tomography, magnetic resonance imaging and ultrasound imaging [4]. Compared with computed tomography and magnetic resonance imaging, ultrasound imaging has the advantages of being low cost, no radiation and real-time image generation [5]. Therefore, it has become the best choice for doctors in lumbar puncture surgery. However, the time and results required to complete a scan are dependent exclusively on the skills and experience of the doctor. In addition, ultrasound scanning requires a complex posture of the hands of doctors to maintain a certain contact force; hence, long-term work may cause complications such as arthritis [6,7]. The use of electric power to operate a mechanical arm can reduce the burden on doctors.
During an operation, the robotic arm puncture system has the advantages of high precision, good stability and simple and efficient operation [8]. Aiming at the clinical needs of lumbar puncture, the present study involved the design of a robotic-assisted ultrasound scanning system for lumbar puncture. The computer-controlled robotic arm clamps the ultrasound probe and automatically scans the patient's lumbar spine.
The proposed system is shown in Figure 1, and it mainly included DP-50 portable ultrasound diagnostic equipment (Shenzhen Mindray Biomedical Electronics Company) for acquiring the two-dimensional ultrasound images, a six-degree-of-freedom robotic arm (UR3, Universal Robots A/S, Odense, Denmark) for controlling the movement of the ultrasound probe, a main control computer with an Intel Core i7-7700 processor to control the robot arm and process the image, a video capture card (Epiphan DVI2USB3.0) for acquiring two-dimensional ultrasound images and transmitting them to a computer and a GRIPKIT-E gripper (Weiss Robotic Company) for holding the ultrasonic probes.
The system was divided into three main parts: control, acquisition and visual processing. Among them, the control part mainly involved the main control computer (including software), the robot arm control box and the structure of the robot arm; the acquisition part mainly involved the portable ultrasonic scanning system and the video capture card; the visualization processing was mainly realized through the software on the main control computer in which the code was embedded. In the operation of the system, the operator controlled the control program interface through the peripheral equipment of the main control computer and monitored the running state of the system in real time through the display, and the robot arm and the control box communicated with the computer through the Transmission Control Protocol/Internet Protocol. When the operator determined the starting point and end point of the scan, the control program automatically controlled the robot arm holding the ultrasound probe to scan the lumbar vertebrae and collect two-dimensional ultrasound images. The collected ultrasound images were transmitted to the main control through the data acquisition card. The computer then processed and saved the images. After the scan was completed, the visualization toolkit (VTK) 3D image reconstruction program was employed to perform the 3D reconstruction of the scanned images. The reconstruction result can provide a reference and guidance, thereby enabling the doctor to determine the puncture point.
The control software was developed by using the Microsoft C# programming language, which mainly included five modules. The user interface module (LumbarPunctureSystem) was the main module of the system; it had the main following functions: parameter setting, operation control, process control, image display and system status display. The surgery module (SurgeryModule) was used to create new cases, hardware (robot arm, ultrasound) start and control and system settings. The robot control module (RobotModule) realized the motion control of the robot arm so that the robot arm could independently move along the axes of the base coordinate system and the tool coordinate system. The ultrasound imaging module (ImagingModule) was used to collect the ultrasound images and record information, such as the position of the robot arm and the size of the torque during the acquisition process, and to perform 3D reconstruction after the acquisition. The button control module (EnhancedGlassButton) was used to create button controls for the interface.
Initially, the control software was launched; the program automatically connected the robot arm and the acquisition card. After the connection was successfully established, the new case button in the operation control area was activated. The operator created a case and filled in relevant information by clicking the new case button. Subsequently, after the successful creation, the tool button was activated.
After the operator clicked the tool button, the program controlled the robot arm to grab the ultrasonic probe placed at a fixed position, activated the button to set the scanning start point and prompted the robot arm motion control panel to appear on screen. The operator adjusted the robot arm through the control panel by moving it to the starting point of the model scan and pressed the start button to activate the scanning end button. Similarly, the probe could be moved to the end point, after which the end point button could be pressed. Subsequently, the program launched the scan control interface, which displayed the corresponding information and supported the modification of some settings. Finally, when the operator pressed the scan button, the program automatically controlled the robot arm to move along a specific path from the scan start point to the scan end point and performed real-time image storage. During the scanning process, every time the robot arm moved 0.5 mm, the program acquired an ultrasound image, as shown in Figure 2. During this process, the robotic arm was set to run in force mode to ensure that the probe was in effective contact with the measured object. After artificial planning, the robotic arm moved in the lumbar spine direction and it automatically adjusted the position in the vertical direction, following force-limited parameters in grip, thereby acquiring a good ultrasound image. It is worth noting that, before the scan started, information such as the pressure of the robot arm and the interval of image acquisition was set on the scan control interface. In addition, once the new case was created, the program automatically activated the stop button in the operation control area. At any time during the scan, the program could be terminated by pressing this button to prevent accidents and ensure the safety of the scan.
The lumbar spine model used in this study was the Enovo Medical Model (Shanghai, China) shown in Figure 3. Ultrasound has a good propagation speed in hydrogel material [9], and it produces a good coupling between the model and the probe. As a result, the experimental model for scanning was made in an acrylic material container (made in our laboratory), the spine model was put in it and the mixture of hydrogel and pure water was poured for lumbar region modeling. The liquid surface was 1–2 cm above the model. The ultrasonic probe model included a 75L38EA linear array, and the ultrasonic frequency was 8.5 MHz, the scan width was 3.8 cm and the depth was 4.6 cm. According to clinical experience, lumbar puncture is usually performed at the 3rd to 4th lumbar vertebral space, and in children under 4 years old, it needs to be punctured at the 4th to 5th spinous process space of the lumbar vertebrae. Therefore, the scanning range of this experiment was L3–L5. In the experiment, the main control program controlled the robot arm to clamp onto the ultrasonic probe to determine the scanning start and end points. The acquisition interval (0.5 mm) and force mode value (2N) were set to perform the two-dimensional ultrasound image acquisition. Then, the obtained image was processed for the 3D reconstruction and visualization.
After successfully acquiring the two-dimensional ultrasound tomographic image, the 3D reconstruction button in the main control program was activated. The operator pressed this button to perform the VTK-based 3D ultrasound image reconstruction and display, which can provide a reference and serve as guidance for determining the location of the puncture point and surgical path planning.
The VTK is an open-source visualization development tool developed by the Kitware Company, and it is widely used in the fields of visualization and 3D graphics, as well as other fields. The 3D visualization process generally involves medical image data reading, data preprocessing, visual mapping, reconstruction and interactive operation. Image data acquisition is a two-dimensional tomographic medical image sequence obtained by scanning real objects by using an imaging device. The format of the read image included the DICOM format and BMP format. Data preprocessing was carried out because the quality of the image may be reduced to a certain extent during the image output, transmission and conversion, and due to the introduction of noise. Hence, the image needed to be preprocessed by filtering. Visual mapping converted the processed raw data into geometric pixels and attributes for drawing, including the display shape, color and other attribute settings. Common methods for reconstruction include surface and volume rendering. Surface rendering describes the 3D structure of organs or tissues by fitting the surface information of the structure by data splicing, and the key technology sets a threshold to extract the isosurface according to the needs [10]. Volume rendering directly synthesizes the 3D images by resampling the volume data based on the principle of vision. This method treats each voxel as a particle that can receive or emit light, and it configures the light intensity and opacity, integrates along the line of sight and finally forms a projected image. Because surface rendering may lead to the reduction of detailed information, resulting in poor authenticity, this study adopted the use of the volume rendering method for 3D reconstruction. The interactive operation was performed on the basis of the appearance of the 3D reconstruction results. By clicking the mouse, the reconstruction body could be rotated to any angle.
The main methods of volume rendering are ray casting, maximum intensity projection and texture mapping [11]. Since the image quality obtained by the ray-casting algorithm is high, it is most widely used. The flowchart of the ray-casting algorithm is shown in Figure 4. The specific steps of the ray-casting method in the VTK were as follows. First, the bitmap (BMP) picture was read through the vtkBMPReader class. Subsequently, the vtkPiecewiseFunction class was used to determine the opacity of each voxel through the addition of numerical points, and the vtkColorTransferFunction class was employed to implement the color transfer function to add color values or a gray degree value. Then, by selecting different vtkVolumeMapper classes in the drawing pipeline, different volume rendering algorithms were implemented, among which the ray-casting algorithm used the vtkVolumeRayCastMapper class. Finally, the vtkVolumeActor class was used to specify the scene lighting, perspective, focus and other information, and the vtkRender class was used to render the entities in the drawn scene. The VTK also provides the widget base class. In this experiment, the vtkImagePlaneWidget class was used to create cutting plane objects, and the cross section, coronal plane and sagittal plane were successfully displayed.
In this study, the two-dimensional ultrasound images collected by the control program were saved as an 8-bit BMP grayscale image. Subsequently, the reconstruction program was employed to visualize the 3D reconstruction, and the images were processed by using the median filtering method. According to the two-dimensional images collected every 0.5 mm, the ultrasound acquisition depth and probe width were set corresponding to the pixel points of the BMP image in order to calculate the difference between the data points. This was done to ensure that the image scale and size were consistent with the real object. Interactive operation was also set in the program. When the reconstruction result was obtained, the operator could place the mouse cursor in this area and hold down the left mouse button to move it arbitrarily. The operator could then rotate the 3D reconstruction body to any angle for full observation. The zoom function was also realized by rolling the mouse wheel.
A total of 136 two-dimensional ultrasound images were collected in the lumbar spine model experiment, and the dimensions (in pixels) were 393 × 473. After preprocessing by enhancing the contrast of and denoising the original ultrasound images, the ray-casting algorithm was used to perform the 3D reconstruction of the lumbar spine model. The reconstruction results are shown in Figure 5. Figure 5B is the 3D reconstruction result of the volume rendering ray-casting method, and Figure 5A, C, D are the cross section, sagittal plane and coronal plane after reconstruction, respectively.
Repeated experiments with different entry points were performed and recorded, following the parameters above; the results are shown in Figure 6. The 3D images and corresponding sagittal plane slice images were labeled to show the reproducibility for this model.
Due to the propagation characteristics of the ultrasound along a straight line, the information of the spinous processes perpendicular to the ultrasound linear array was lost. Thus, the reconstructed structure was defective; however, the location of the spinous process gap could be determined by using the sagittal and coronal images, which can be used as a follow-up reference basis for the puncture surgery. The repeated experiment data with different entry points (Figure 6) shows the anatomical structural changes in grey value and sharpness of boundary, which might have been caused by entry point changes, along with shape changing on the back during the scanning of the model.
In recent years, with the gradual maturity of the robotic technology, puncture surgery systems assisted by a robotic arm have developed rapidly. Nelson et al. [12] developed a set of ultrasound image-guided breast biopsy systems for the detection of early breast cancer; it was shown to have good repeatability and less than 0.5% variation. Podder et al. [13] designed a multichannel robot system based on the ultrasound guidance, and it comprised multiple particle implantation needles, especially for the implantation of radioactive particles in the prostate [14]. An experiment revealed a conveyor accuracy within 0.2 mm. Kojcev et al. [15] used both arms to design a set of ultrasound-guided auxiliary puncture systems; it implemented the closed-loop control of the planning-imaging-needle insertion to adjust and plan the puncture path in real time. Xu et al. [16] developed a minimally invasive robotic system for the ultrasound-guided microwave ablation of the liver. This system could perform 3D reconstruction of the liver tumor before surgery and plan the puncture path; it assisted the doctors in sending the puncture needle to the target point during an operation. The above-mentioned previous studies mainly involved ultrasound guidance and included location planning of the body or probe through the use of computed tomography, optical assisted technology and other imaging methods, instead of the robotic arm automatic guidance with force feedback. Our study provides an easier way to employ ultrasound scanning, find the precise location and simplify the complicated operation. In this study, the scanned ultrasound images were processed and reconstructed in all three dimensions. After experimental verification, the system was shown to be able to collect two-dimensional ultrasound images and reconstruct 3D lumbar spine images, thereby providing the doctors with stereoscopic visualization guidance, laying the foundation for subsequent robot-assisted puncture surgeries.
Regarding the usage of this methodology in actual clinical practice, more studies need to be done in the future, such as the use of an automatic drawing point and trajectory as a reference for the doctor, the addition of the modules for different types of conditions, like leukemia cerebrospinal fluid examination, scoliosis and larger body habitus and the redesign of different algorithms for robotic arm motion and puncture planning.
The present study was purposed to design a 3D visualization-enabled robotic-assisted ultrasound automatic scanning system for the lumbar spine. The control program for the robotic arm was developed using the C# language, and a 3D reconstruction program for the ultrasound images was developed based on the VTK. The system controlled the robotic arm to automatically scan and collect the ultrasound images of the lumbar spine model through the program; it subsequently performed the 3D reconstruction. The system could operate normally and achieved the target function. The experimental results for the lumbar spine model showed that the scanning system could effectively collect the images of the model, visualize the 3D reconstruction, realize a full-dimensional stereoscopic display and provide a reference basis for lumbar puncture surgery.
This study was supported by grants from the CAMS Innovation Fund for Medical Science (No. 2021-I2M-1-042, 2021-I2M-1-015, No. 2017-I2M-1-016).
The authors declare that there is no conflict of interest.
[1] |
Jami M, Ghanbari M, Zunabovic M, et al. (2014) Listeria monocytogenes in aquatic food products-A review. Compr Rev Food Sci Food Saf 13: 798-813. https://doi.org/10.1111/1541-4337.12092 ![]() |
[2] | Batt CA (2014) Listeria: Introduction. Encycl Food Microbiol Second Ed 2: 466-469. |
[3] |
Leong D, Alvarez-Ordóñez A, Jordan K (2014) Monitoring occurrence and persistence of Listeria monocytogenes in foods and food processing environments in the Republic of Ireland. Front Microbiol 5: 436. https://doi.org/10.3389/fmicb.2014.00436 ![]() |
[4] |
Chen BY, Wang CY, Wang CL, et al. (2016) Prevalence and persistence of Listeria monocytogenes in ready-to-eat tilapia sashimi processing plants. J Food Prot 79: 1898-1903. https://doi.org/10.4315/0362-028X.JFP-16-149 ![]() |
[5] |
Wagner E, Fagerlund A, Thalguter S, et al. (2022) Deciphering the virulence potential of Listeria monocytogenes in the Norwegian meat and salmon processing industry by combining whole genome sequencing and in vitro data. Int J Food Microbiol 383: 109962. https://doi.org/10.1016/j.ijfoodmicro.2022.109962 ![]() |
[6] |
Batt CA (2014) Listeria: Listeria monocytogenes. Encyclopedia of Food Microbiology.Academic Press 490-493. https://doi.org/10.1016/B978-0-12-384730-0.00191-9 ![]() |
[7] |
Abdollahzadeh E, Ojagh SM, Hosseini H, et al. (2016) Prevalence and molecular characterization of Listeria spp. and Listeria monocytogenes isolated from fish, shrimp, and cooked ready-to-eat (RTE) aquatic products in Iran. LWT 73: 205-211. https://doi.org/10.1016/j.lwt.2016.06.020 ![]() |
[8] |
Kathariou S (2002) Listeria monocytogenes virulence and pathogenicity, a food safety perspective. J Food Prot 65: 1811-1829. https://doi.org/10.4315/0362-028x-65.11.1811 ![]() |
[9] |
Matle I, Mbatha KR, Madoroba E (2020) A review of Listeria monocytogenes from meat and meat products: Epidemiology, virulence factors, antimicrobial resistance and diagnosis. Onderstepoort J Vet Res 87: 1-20. https://doi.org/10.4102/ojvr.v87i1.1869 ![]() |
[10] |
Orsi RH, den Bakker HC, Wiedmann M (2011) Listeria monocytogenes lineages: Genomics, evolution, ecology, and phenotypic characteristics. Int J Med Microbiol 301: 79-96. https://doi.org/10.1016/j.ijmm.2010.05.002 ![]() |
[11] |
Rip D, Gouws PA (2020) PCR–restriction fragment length polymorphism and pulsed-field gel electrophoresis characterization of Listeria monocytogenes isolates from ready-to-eat foods, the food processing environment, and clinical samples in South Africa. J Food Prot 83: 518-533. https://doi.org/10.4315/0362-028X.JFP-19-301 ![]() |
[12] |
Kaszoni-Rückerl I, Mustedanagic A, Muri-Klinger S, et al. (2020) Predominance of distinct Listeria innocua and Listeria monocytogenes in recurrent contamination events at dairy processing facilities. Microorganisms 8: 234. https://doi.org/10.3390/microorganisms8020234 ![]() |
[13] |
Lotfollahi L, Chaharbalesh A, Ahangarzadeh Rezaee M, et al. (2017) Prevalence, antimicrobial susceptibility and multiplex PCR-serotyping of Listeria monocytogenes isolated from humans, foods and livestock in Iran. Microb Pathog 107: 425-429. https://doi.org/10.1016/j.micpath.2017.04.029 ![]() |
[14] |
Alvarez-Molina A, Cobo-Díaz JF, López M, et al. (2021) Unraveling the emergence and population diversity of Listeria monocytogenes in a newly built meat facility through whole genome sequencing. Int J Food Microbiol 340: 109043. https://doi.org/10.1016/j.ijfoodmicro.2021.109043 ![]() |
[15] |
Camejo A, Carvalho F, Reis O, et al. (2011) The arsenal of virulence factors deployed by Listeria monocytogenes to promote its cell infection cycle. Virulence 2: 379-394. https://doi.org/10.4161/viru.2.5.17703 ![]() |
[16] |
Hernandez-Milian A, Payeras-Cifre A (2014) What is new in listeriosis?. Biomed Res Int 2014: 1-7. https://doi.org/10.1155/2014/358051 ![]() |
[17] | EFSA.The European Union One Health 2020 Zoonoses Report. EFSA J (2021) 19:. https://doi.org/10.2903/j.efsa.2021.6971 |
[18] |
Keet R, Rip D (2021) Listeria monocytogenes isolates from Western Cape, South Africa exhibit resistance to multiple antibiotics and contradicts certain global resistance patterns. AIMS Microbiol 7: 40-58. https://doi.org/10.3934/microbiol.2021004 ![]() |
[19] |
Basha KA, Kumar NR, Das V, et al. (2019) Prevalence, molecular characterization, genetic heterogeneity and antimicrobial resistance of Listeria monocytogenes associated with fish and fishery environment in Kerala, India. Lett Appl Microbiol 69: 286-293. https://doi.org/10.1111/lam.13205 ![]() |
[20] |
Jamali H, Paydar M, Ismail S, et al. (2015) Prevalence, antimicrobial susceptibility and virulotyping of Listeria species and Listeria monocytogenes isolated from open-air fish markets. BMC Microbiol 15: 144. https://doi.org/10.1186/s12866-015-0476-7 ![]() |
[21] |
Abdollahzadeh E, Ojagh SM, Hosseini H, et al. (2016) Antimicrobial resistance of Listeria monocytogenes isolated from seafood and humans in Iran. Microb Pathog 100: 70-74. https://doi.org/10.1016/j.micpath.2016.09.012 ![]() |
[22] |
Matle I, Pierneef R, Mbatha KR, et al. (2019) Genomic diversity of common sequence types of listeria monocytogenes isolated from ready-to-eat products of animal origin in South Africa. Genes (Basel) 10: 1007. https://doi.org/10.3390/genes10121007 ![]() |
[23] |
Wieczorek K, Osek J (2017) Prevalence, genetic diversity and antimicrobial resistance of Listeria monocytogenes isolated from fresh and smoked fish in Poland. Food Microbiol 64: 164-171. https://doi.org/10.1016/j.fm.2016.12.022 ![]() |
[24] |
Palaiodimou L, Fanning S, Fox EM (2021) Genomic insights into persistence of Listeria species in the food processing environment. J Appl Microbiol 131: 2082-2094. https://doi.org/10.1111/jam.15089 ![]() |
[25] |
Mullapudi S, Siletzky RM, Kathariou S (2008) Heavy-metal and benzalkonium chloride resistance of Listeria monocytogenes isolates from the environment of turkey-processing plants. Appl Environ Microbiol 74: 1464-1468. https://doi.org/10.1128/AEM.02426-07 ![]() |
[26] |
Kropac AC, Eshwar AK, Stephan R, et al. (2019) New Insights on the role of the plmst6 plasmid in Listeria monocytogenes biocide tolerance and virulence. Front Microbiol 10: 1-16. https://doi.org/10.3389/fmicb.2019.01538 ![]() |
[27] |
Elhanafi D, Dutta V, Kathariou S (2010) Genetic characterization of plasmid-associated benzalkonium chloride resistance determinants in a Listeria monocytogenes strain from the 1998-1999 Outbreak. Appl Environ Microbiol 76: 8231-8238. https://doi.org/10.1128/AEM.02056-10 ![]() |
[28] |
Tezel U, Pavlostathis SG (2015) Quaternary ammonium disinfectants: microbial adaptation, degradation and ecology. Curr Opin Biotechnol 33: 296-304. https://doi.org/10.1016/j.copbio.2015.03.018 ![]() |
[29] |
Møretrø T, Schirmer BCT, Heir E, et al. (2017) Tolerance to quaternary ammonium compound disinfectants may enhance growth of Listeria monocytogenes in the food industry. Int J Food Microbiol 241: 215-224. https://doi.org/10.1016/j.ijfoodmicro.2016.10.025 ![]() |
[30] |
Kremer PHC, Lees JA, Koopmans MM, et al. (2017) Benzalkonium tolerance genes and outcome in Listeria monocytogenes meningitis. Clin Microbiol Infect 23: 265.e1-265.e7. https://doi.org/10.1016/j.cmi.2016.12.008 ![]() |
[31] |
Daeschel D, Pettengill JB, Wang Y, et al. (2022) Genomic analysis of Listeria monocytogenes from US food processing environments reveals a high prevalence of QAC efflux genes but limited evidence of their contribution to environmental persistence. BMC Genomics 23: 488. https://doi.org/10.1186/s12864-022-08695-2 ![]() |
[32] |
Harrand AS, Jagadeesan B, Baert L, et al. (2020) Evolution of Listeria monocytogenes in a food processing plant involves limited single-nucleotide substitutions but considerable diversification by gain and loss of prophages. Appl Environ Microbiol 86: e02493-19. https://doi.org/10.1128/AEM.02493-19 ![]() |
[33] |
Hilliard A, Leong D, O'Callaghan A, et al. (2018) Genomic characterization of Listeria monocytogenes isolates associated with clinical listeriosis and the food production environment in Ireland. Genes (Basel) 9: 171. https://doi.org/10.3390/genes9030171 ![]() |
[34] |
Vilchis-Rangel RE, Espinoza-Mellado M del R, Salinas-Jaramillo IJ, et al. (2019) Association of Listeria monocytogenes LIPI-1 and LIPI-3 marker llsX with invasiveness. Curr Microbiol 76: 637-643. https://doi.org/10.1007/s00284-019-01671-2 ![]() |
[35] |
Lachtara B, Osek J, Wieczorek K (2021) Molecular typing of Listeria monocytogenes ivb serogroup isolated from food and food production environments in poland. Pathogens 10: 482. https://doi.org/10.3390/pathogens10040482 ![]() |
[36] |
Guidi F, Chiaverini A, Repetto A, et al. (2021) Hyper-virulent Listeria monocytogenes strains associated with respiratory infections in central Italy. Front Cell Infect Microbiol 11: 1-8. https://doi.org/10.3389/fcimb.2021.765540 ![]() |
[37] |
Maury MM, Tsai Y, Charlier C, et al. (2016) Uncovering Listeria monocytogenes hypervirulence by harnessing its biodiversity. Nat Genet 48: 308-313. https://doi.org/10.1038/ng.3501 ![]() |
[38] |
Wieczorek K, Bomba A, Osek J (2020) Whole-genome sequencing-based characterization of Listeria monocytogenes from fish and fish production environments in Poland. Int J Mol Sci 21: 9419. https://doi.org/10.3390/ijms21249419 ![]() |
[39] |
Wagner E, Zaiser A, Leitner R, et al. (2020) Virulence characterization and comparative genomics of Listeria monocytogenes sequence type 155 strains. BMC Genomics 21: 1-18. https://doi.org/10.1186/s12864-020-07263-w ![]() |
[40] |
Harter E, Wagner EM, Zaiser A, et al. (2017) Stress survival islet 2, predominantly present in Listeria monocytogenes strains of sequence type 121, is involved in the alkaline and oxidative stress responses. Appl Environ Microbiol 83. https://doi.org/10.1128/AEM.00827-17 ![]() |
[41] |
Ryan S, Begley M, Hill C, et al. (2010) A five-gene stress survival islet (SSI-1) that contributes to the growth of Listeria monocytogenes in suboptimal conditions. J Appl Microbiol 109: 984-995. https://doi.org/10.1111/j.1365-2672.2010.04726.x ![]() |
[42] |
Lakicevic BZ, Den Besten HMW, De Biase D (2022) Landscape of stress response and virulence genes among Listeria monocytogenes strains. Front Microbiol 12: 1-16. https://doi.org/10.3389/fmicb.2021.738470 ![]() |
[43] |
Toledo V, den Bakker H, Hormazábal J, et al. (2018) Genomic diversity of Listeria monocytogenes isolated from clinical and non-clinical samples in Chile. Genes (Basel) 9: 396. https://doi.org/10.3390/genes9080396 ![]() |
[44] |
Allerberger F (2003) Listeria: Growth, phenotypic differentiation and molecular microbiology. FEMS Immunol Med Microbiol 35: 183-189. https://doi.org/10.1016/S0928-8244(02)00447-9 ![]() |
[45] |
Hyden P, Pietzka A, Lennkh A, et al. (2016) Whole genome sequence-based serogrouping of Listeria monocytogenes isolates. J Biotechnol 235: 181-186. https://doi.org/10.1016/j.jbiotec.2016.06.005 ![]() |
[46] |
Bouymajane A, Rhazi Filali F, Oulghazi S, et al. (2021) Occurrence, antimicrobial resistance, serotyping and virulence genes of Listeria monocytogenes isolated from foods. Heliyon 7: e06169. https://doi.org/10.1016/j.heliyon.2021.e06169 ![]() |
[47] |
Seidl K, Leimer N, Palheiros Marques M, et al. (2015) Clonality and antimicrobial susceptibility of methicillin-resistant Staphylococcus aureus at the University Hospital Zurich, Switzerland between 2012 and 2014. Ann Clin Microbiol Antimicrob 14: 14. https://doi.org/10.1186/s12941-015-0075-3 ![]() |
[48] |
Enright MC, Day NPJ, Davies CE, et al. (2000) Multilocus sequence typing for characterization of methicillin-resistant and methicillin-susceptible clones of Staphylococcus aureus. J Clin Microbiol 38: 1008-1015. https://doi.org/10.1128/JCM.38.3.1008-1015.2000 ![]() |
[49] |
Enright MC, Robinson DA, Randle G, et al. (2002) The evolutionary history of methicillin-resistant Staphylococcus aureus (MRSA). Proc Natl Acad Sci 99: 7687-7692. https://doi.org/10.1073/pnas.122108599 ![]() |
[50] |
Blais BW, Phillippe LM (1993) A simple RNA probe system for analysis of Listeria monocytogenes polymerase chain reaction products. Appl Environ Microbiol 59: 2795-2800. https://doi.org/10.1128/aem.59.9.2795-2800.1993 ![]() |
[51] | EUCASTAntimicrobial susceptibility testing EUCAST disk diffusion method (2023). Available from: https://www.eucast.org/fileadmin/src/media/PDFs/EUCAST_files/Disk_test_documents/2023_manuals/Manual_v_11.0_EUCAST_Disk_Test_2023.pdf |
[52] |
Varsaki A, Ortiz S, Santorum P, et al. (2022) Prevalence and population diversity of Listeria monocytogenes isolated from dairy cattle farms in the cantabria region of Spain. Animals (Basel) 12: 2477. ![]() |
[53] | EUCASTEuropean Committee on Antimicrobial Susceptibility Testing Breakpoint tables for interpretation of MICs and zone diameters European Committee on Antimicrobial Susceptibility Testing Breakpoint tables for interpretation of MICs and zone diameters (2022). Version 12.0. Available from: http://www.eucast.org |
[54] |
Chen BY, Pyla R, Kim TJ, et al. (2010) Antibiotic resistance in Listeria species isolated from catfish fillets and processing environment. Lett Appl Microbiol 50: 626-632. https://doi.org/10.1111/j.1472-765X.2010.02843.x ![]() |
[55] |
Noll M, Kleta S, Al Dahouk S (2018) Antibiotic susceptibility of 259 Listeria monocytogenes strains isolated from food, food-processing plants and human samples in Germany. J Infect Public Health 11: 572-577. https://doi.org/10.1016/j.jiph.2017.12.007 ![]() |
[56] |
Maćkiw E, Modzelewska M, Mąka Ł, et al. (2016) Antimicrobial resistance profiles of Listeria monocytogenes isolated from ready-to-eat products in Poland in 2007–2011. Food Control 59: 7-11. https://doi.org/10.1016/j.foodcont.2015.05.011 ![]() |
[57] |
Wiggins GL, Albritton WL, Feeley JC (1978) Antibiotic susceptibility of clinical isolates of Listeria monocytogenes. Antimicrob Agents Chemother 13: 854-860. https://doi.org/10.1128/AAC.13.5.854 ![]() |
[58] |
Larsen M V, Cosentino S, Rasmussen S, et al. (2012) Multilocus sequence typing of total-genome-sequenced bacteria. J Clin Microbiol 50: 1355-1361. https://doi.org/10.1128/JCM.06094-11 ![]() |
[59] |
Bartual SG, Seifert H, Hippler C, et al. (2005) Development of a multilocus sequence typing scheme for characterization of clinical isolates of Acinetobacter baumannii. J Clin Microbiol 43: 4382-4390. https://doi.org/10.1128/JCM.43.9.4382-4390.2005 ![]() |
[60] |
Griffiths D, Fawley W, Kachrimanidou M, et al. (2010) Multilocus sequence typing of Clostridium difficile. J Clin Microbiol 48: 770-778. https://doi.org/10.1128/JCM.01796-09 ![]() |
[61] |
Lemee L, Dhalluin A, Pestel-Caron M, et al. (2004) Multilocus sequence typing analysis of human and animal Clostridium difficile isolates of various toxigenic types. J Clin Microbiol 42: 2609-2617. https://doi.org/10.1128/JCM.42.6.2609-2617.2004 ![]() |
[62] |
Wirth T, Falush D, Lan R, et al. (2006) Sex and virulence in Escherichia coli: an evolutionary perspective. Mol Microbiol 60: 1136-1151. https://doi.org/10.1111/j.1365-2958.2006.05172.x ![]() |
[63] |
Jaureguy F, Landraud L, Passet V, et al. (2008) Phylogenetic and genomic diversity of human bacteremic Escherichia coli strains. BMC Genomics 9: 560. https://doi.org/10.1186/1471-2164-9-560 ![]() |
[64] |
Camacho C, Coulouris G, Avagyan V, et al. (2009) BLAST+: architecture and applications. BMC Bioinformatics 10: 421. https://doi.org/10.1186/1471-2105-10-421 ![]() |
[65] |
Moura A, Criscuolo A, Pouseele H, et al. (2016) Whole genome-based population biology and epidemiological surveillance of Listeria monocytogenes. Nat Microbiol 2: 16185. https://doi.org/10.1038/nmicrobiol.2016.185 ![]() |
[66] |
Joensen KG, Scheutz F, Lund O, et al. (2014) Real-time whole-genome sequencing for routine typing, surveillance, and outbreak detection of verotoxigenic Escherichia coli. J Clin Microbiol 52: 1501-1510. https://doi.org/10.1128/JCM.03617-13 ![]() |
[67] |
Malberg Tetzschner AM, Johnson JR, Johnston BD, et al. (2020) In silico genotyping of Escherichia coli isolates for extraintestinal virulence genes by use of whole-genome sequencing data. J Clin Microbiol 58: 1-13. https://doi.org/10.1128/jcm.01269-20 ![]() |
[68] |
Carattoli A, Zankari E, García-Fernández A, et al. (2014) In silico detection and typing of plasmids using plasmidfinder and plasmid multilocus sequence typing. Antimicrob Agents Chemother 58: 3895-3903. https://doi.org/10.1128/AAC.02412-14 ![]() |
[69] |
Bortolaia V, Kaas RS, Ruppe E, et al. (2020) ResFinder 4.0 for predictions of phenotypes from genotypes. J Antimicrob Chemother 75: 3491-3500. https://doi.org/10.1093/jac/dkaa345 ![]() |
[70] |
Zankari E, Allesøe R, Joensen KG, et al. (2017) PointFinder: a novel web tool for WGS-based detection of antimicrobial resistance associated with chromosomal point mutations in bacterial pathogens. J Antimicrob Chemother 72: 2764-2768. https://doi.org/10.1093/jac/dkx217 ![]() |
[71] |
Cossart P, Vicente MF, Mengaud J, et al. (1989) Listeriolysin O is essential for virulence of Listeria monocytogenes: direct evidence obtained by gene complementation. Infect Immun 57: 3629-3636. https://doi.org/10.1128/iai.57.11.3629-3636.1989 ![]() |
[72] | Forsythe SJ (2010) The microbiology of safe food. Chichester, UK: Wiley-Blackwell Pub. |
[73] |
Maury MM, Bracq-Dieye H, Huang L, et al. (2019) Hypervirulent Listeria monocytogenes clones' adaption to mammalian gut accounts for their association with dairy products. Nat Commun 10: 2488. https://doi.org/10.1038/s41467-019-10380-0 ![]() |
[74] |
Fagerlund A, Wagner E, Møretrø T, et al. (2022) Pervasive Listeria monocytogenes is common in the norwegian food system and is associated with increased prevalence of stress survival and resistance determinants. Appl Environ Microbiol 88: e0086122. https://doi.org/10.1128/aem.00861-22 ![]() |
[75] |
Lee DY, Ha JH, Lee MK, et al. (2017) Antimicrobial susceptibility and serotyping of Listeria monocytogenes isolated from ready-to-eat seafood and food processing environments in Korea. Food Sci Biotechnol 26: 287-291. https://doi.org/10.1007/s10068-017-0038-x ![]() |
[76] |
Miettinen H, Wirtanen G (2005) Prevalence and location of Listeria monocytogenes in farmed rainbow trout. Int J Food Microbiol 104: 135-143. https://doi.org/10.1016/j.ijfoodmicro.2005.01.013 ![]() |
[77] |
Painset A, Björkman JT, Kiil K, et al. (2019) LiSEQ–whole-genome sequencing of a cross-sectional survey of Listeria monocytogenes in ready-to-eat foods and human clinical cases in Europe. Microb Genomics 5: e000257. https://doi.org/10.1099/mgen.0.000257 ![]() |
[78] |
Pontello M, Guaita A, Sala G, et al. (2012) Listeria monocytogenes serotypes in human infections (Italy, 2000–2010). Ann Ist Super Sanita 48: 146-150. https://doi.org/10.4415/ANN_12_02_07 ![]() |
[79] |
Fagerlund A, Idland L, Heir E, et al. (2022) Whole-genome sequencing analysis of Listeria monocytogenes from Rural, Urban, and farm environments in Norway: genetic diversity, persistence, and relation to clinical and food isolates. Appl Environ Microbiol 88: e0213621. https://doi.org/10.1128/aem.02136-21 ![]() |
[80] |
Fonnesbech Vogel B, Huss HH, Ojeniyi B, et al. (2001) Elucidation of Listeria monocytogenes Contamination Routes in Cold-Smoked Salmon Processing Plants Detected by DNA-Based Typing Methods. Appl Environ Microbiol 67: 2586-2595. https://doi.org/10.1128/AEM.67.6.2586-2595.2001 ![]() |
[81] |
Chen BY, Pyla R, Kim TJ, et al. (2010) Prevalence and contamination patterns of Listeria monocytogenes in catfish processing environment and fresh fillets. Food Microbiol 27: 645-652. https://doi.org/10.1016/j.fm.2010.02.007 ![]() |
[82] |
Rørvik LM, Aase B, Alvestad T, et al. (2000) Molecular epidemiological survey of Listeria monocytogenes in Seafood and Seafood-Processing Plants. Appl Environ Microbiol 66: 4779-4784. https://doi.org/10.1128/aem.66.11.4779-4784.2000 ![]() |
[83] |
Miettinen H, Wirtanen G (2006) Ecology of Listeria spp. in a fish farm and molecular typing of Listeria monocytogenes from fish farming and processing companies. Int J Food Microbiol 112: 138-146. https://doi.org/10.1016/j.ijfoodmicro.2006.06.016 ![]() |
[84] |
Pagadala S, Parveen S, Rippen T, et al. (2012) Prevalence, characterization and sources of Listeria monocytogenes in blue crab (Callinectus sapidus) meat and blue crab processing plants. Food Microbiol 31: 263-270. https://doi.org/10.1016/j.fm.2012.03.015 ![]() |
[85] |
Johansson T, Rantala L, Palmu L, et al. (1999) Occurrence and typing of Listeria monocytogenes strains in retail vacuum-packed fish products and in a production plant. Int J Food Microbiol 47: 111-119. https://doi.org/10.1016/s0168-1605(99)00019-7 ![]() |
[86] |
Palma F, Brauge T, Radomski N, et al. (2020) Dynamics of mobile genetic elements of Listeria monocytogenes persisting in ready-to-eat seafood processing plants in France. BMC Genomics 21: 1-21. https://doi.org/10.1186/s12864-020-6544-x ![]() |
[87] |
Leong D, Alvarez-Ordonez A, Zaouali S, et al. (2015) Examination of Listeria monocytogenes in seafood processing facilities and smoked salmon in the Republic of Ireland. J Food Prot 78: 2184-2190. https://doi.org/10.4315/0362-028X.JFP-15-233 ![]() |
[88] | Mahgoub SA, Elbahnasawy AAF, Abdelfattah HI, et al. (2022) Identification of non-Listeria and presence of Listeria in processing line production of cold-smoked salmon. Egypt J Chem 65: 241-250. https://doi.org/10.21608/ejchem.2021.102791.4762 |
[89] |
Osman KM, Kappell AD, Fox EM, et al. (2020) Prevalence, pathogenicity, virulence, antibiotic resistance, and phylogenetic analysis of biofilmproducing Listeria monocytogenes isolated from different ecological niches in Egypt: Food, humans, animals, and environment. Pathogens 9: 5. https://doi.org/10.3390/pathogens9010005 ![]() |
[90] |
Müller A, Rychli K, Zaiser A, et al. (2014) The Listeria monocytogenes transposon Tn6188 provides increased tolerance to various quaternary ammonium compounds and ethidium bromide. FEMS Microbiol Lett 361: 166-173. https://doi.org/10.1111/1574-6968.12626 ![]() |
[91] |
Cooper AL, Carrillo CD, DeschêNes M, et al. (2021) Genomic markers for quaternary ammonium compound resistance as a persistence indicator for Listeria monocytogenes contamination in food manufacturing environments. J Food Prot 84: 389-398. https://doi.org/10.4315/JFP-20-328 ![]() |
[92] |
Hurley D, Luque-Sastre L, Parker CT, et al. (2019) Whole-genome sequencing-based characterization of 100 Listeria monocytogenes isolates collected from food processing environments over a four-year period. mSphere 4: 1-14. https://doi.org/10.1128/msphere.00252-19 ![]() |
[93] |
Chmielowska C, Korsak D, Szuplewska M, et al. (2021) Benzalkonium chloride and heavy metal resistance profiles of Listeria monocytogenes strains isolated from fish, fish products and food-producing factories in Poland. Food Microbiol 98: 103756. https://doi.org/10.1016/j.fm.2021.103756 ![]() |
[94] |
Müller A, Rychli K, Muhterem-Uyar M, et al. (2013) Tn6188-a novel transposon in Listeria monocytogenes responsible for tolerance to benzalkonium chloride. PLoS One 8: e76835. https://doi.org/10.1371/journal.pone.0076835 ![]() |
[95] | Zhang X, Liu Y, Zhang P, et al. (2021) Genomic characterization of clinical Listeria monocytogenes isolates in Beijing, China. Front Microbiol 12: 1-12. https://doi.org/10.3389/fmicb.2021.751003 |
[96] |
Datta AR, Burall LS (2018) Serotype to genotype: The changing landscape of listeriosis outbreak investigations. Food Microbiol 75: 18-27. https://doi.org/10.1016/j.fm.2017.06.013 ![]() |
[97] |
Holch A, Webb K, Lukjancenko O, et al. (2013) Genome sequencing identifies two nearly unchanged strains of persistent Listeria monocytogenes isolated at two different fish processing plants sampled 6 years apart. Appl Environ Microbiol 79: 2944-2951. https://doi.org/10.1128/AEM.03715-12 ![]() |
[98] |
Maury MM, Tsai YH, Charlier C, et al. (2016) Uncovering Listeria monocytogenes hypervirulence by harnessing its biodiversity. Nat Genet 48: 308-313. https://doi.org/10.1038/ng.3501 ![]() |
[99] |
Myintzaw P, Pennone V, McAuliffe O, et al. (2023) Association of virulence, biofilm, and antimicrobial resistance genes with specific clonal complex types of Listeria monocytogenes. Microorganisms 11: 1603. https://doi.org/10.3390/microorganisms11061603 ![]() |
[100] | Fox EM, Allnutt T, Bradbury MI, et al. (2016) Comparative genomics of the Listeria monocytogenes ST204 subgroup. Front Microbiol 7: 1-12. https://doi.org/10.3389/fmicb.2016.02057 |
[101] |
Pasquali F, Palma F, Guillier L, et al. (2018) Listeria monocytogenes sequence types 121 and 14 repeatedly isolated within one year of sampling in a rabbit meat processing plant: Persistence and ecophysiology. Front Microbiol 9: 1-12. https://doi.org/10.3389/fmicb.2018.00596 ![]() |
[102] | Cheng Y, Dong Q, Liu Y, et al. (2022) Systematic review of Listeria monocytogenes from food and clinical samples in Chinese mainland from 2010 to 2019. Food Qual Saf 6: 1-10. https://doi.org/10.1093/fqsafe/fyac021 |
[103] |
Wang H, Luo L, Zhang Z, et al. (2018) Prevalence and molecular characteristics of Listeria monocytogenes in cooked products and its comparison with isolates from listeriosis cases. Front Med 12: 104-112. https://doi.org/10.1007/s11684-017-0593-9 ![]() |
[104] |
Ariza-Miguel J, Fernández-Natal MI, Soriano F, et al. (2015) Molecular epidemiology of invasive listeriosis due to Listeria monocytogenes in a Spanish Hospital over a nine-year study period, 2006–2014. Biomed Res Int 2015: 1-10. https://doi.org/10.1155/2015/191409 ![]() |
[105] |
Chenal-Francisque V, Lopez J, Cantinelli T, et al. (2011) Worldwide distribution of major clones of Listeria monocytogenes. Emerg Infect Dis 17: 1110-1112. https://doi.org/10.3201/eid1706.101778 ![]() |
[106] |
Bespalova TY, Mikhaleva T V, Meshcheryakova NY, et al. (2021) Novel sequence types of Listeria monocytogenes of different origin obtained in the Republic of Serbia. Microorganisms 9: 1289. https://doi.org/10.3390/microorganisms9061289 ![]() |
[107] |
Parisi A, Latorre L, Normanno G, et al. (2010) Amplified Fragment Length Polymorphism and Multi-Locus Sequence Typing for high-resolution genotyping of Listeria monocytogenes from foods and the environment. Food Microbiol 27: 101-108. https://doi.org/10.1016/j.fm.2009.09.001 ![]() |
[108] |
Sosnowski M, Lachtara B, Wieczorek K, et al. (2019) Antimicrobial resistance and genotypic characteristics of Listeria monocytogenes isolated from food in Poland. Int J Food Microbiol 289: 1-6. https://doi.org/10.1016/j.ijfoodmicro.2018.08.029 ![]() |
[109] |
Wagner M, Slaghuis J, Göbel W, et al. (2021) Virulence pattern analysis of three Listeria monocytogenes lineage i epidemic strains with distinct outbreak histories. Microorganisms 9: 1745. https://doi.org/10.3390/microorganisms9081745 ![]() |
[110] |
Dalton CB, Austin CC, Sobel J, et al. (1997) An outbreak of gastroenteritis and fever due to Listeria monocytogenes in milk. N Engl J Med 336: 100-106. https://doi.org/10.1056/NEJM199701093360204 ![]() |
[111] | Xu J, Wu S, Liu M, et al. (2023) Prevalence and contamination patterns of Listeria monocytogenes in Pleurotus eryngii (king oyster mushroom) production plants. Front Microbiol 14: 2944-2951. https://doi.org/10.3389/fmicb.2023.1064575 |
[112] |
Zhang X, Niu Y, Liu Y, et al. (2019) Isolation and characterization of clinical Listeria monocytogenes in Beijing, China, 2014–2016. Front Microbiol 10: 1-11. https://doi.org/10.3389/fmicb.2019.00981 ![]() |
[113] |
Lomonaco S, Verghese B, Gerner-Smidt P, et al. (2013) Novel epidemic clones of Listeria monocytogenes, United States, 2011. Emerg Infect Dis 19: 147-150. https://doi.org/10.3201/eid1901.121167 ![]() |
[114] |
Bergholz TM, Shah MK, Burall LS, et al. (2018) Genomic and phenotypic diversity of Listeria monocytogenes clonal complexes associated with human listeriosis. Appl Microbiol Biotechnol 102: 3475-3485. https://doi.org/10.1007/s00253-018-8852-5 ![]() |
[115] |
Smith AM, Tau NP, Smouse SL, et al. (2019) Outbreak of Listeria monocytogenes in South Africa, 2017-2018: Laboratory activities and experiences associated with whole-genome sequencing analysis of isolates. Foodborne Pathog Dis 16: 524-530. https://doi.org/10.1089/fpd.2018.2586 ![]() |
[116] |
Halbedel S, Prager R, Fuchs S, et al. (2018) Whole-genome sequencing of recent Listeria monocytogenes isolates from Germany reveals population structure and disease clusters. J Clin Microbiol 56: e00119-18. https://doi.org/10.1128/JCM.00119-18 ![]() |
[117] |
Papić B, Golob M, Kušar D, et al. (2019) Source tracking on a dairy farm reveals a high occurrence of subclinical mastitis due to hypervirulent Listeria monocytogenes clonal complexes. J Appl Microbiol 127: 1349-1361. https://doi.org/10.1111/jam.14418 ![]() |
[118] |
Matle I, Mafuna T, Madoroba E, et al. (2020) Population structure of Non-ST6 Listeria monocytogenes isolated in the red meat and poultry value chain in South Africa. Microorganisms 8: 1152. https://doi.org/10.3390/microorganisms8081152 ![]() |
[119] |
Mafuna T, Matle I, Magwedere K, et al. (2021) Whole genome-based characterization of Listeria monocytogenes isolates recovered from the food chain in South Africa. Front Microbiol 12: 1-12. https://doi.org/10.3389/fmicb.2021.669287 ![]() |
[120] |
Zhang H, Chen W, Wang J, et al. (2020) 10-year molecular surveillance of Listeria monocytogenes using whole-genome sequencing in Shanghai, China, 2009–2019. Front Microbiol 11: 551020. https://doi.org/10.3389/fmicb.2020.551020 ![]() |
[121] |
Yan S, Li M, Luque-Sastre L, et al. (2019) Susceptibility (re)-testing of a large collection of Listeria monocytogenes from foods in China from 2012 to 2015 and WGS characterization of resistant isolates. J Antimicrob Chemother 74: 1786-1794. https://doi.org/10.1093/jac/dkz126 ![]() |
[122] |
Chen M, Cheng J, Zhang J, et al. (2019) Isolation, potential virulence, and population diversity of Listeria monocytogenes from meat and meat products in China. Front Microbiol 10: 1-10. https://doi.org/10.3389/fmicb.2019.00946 ![]() |
[123] |
Schiavano GF, Ateba CN, Petruzzelli A, et al. (2021) Whole-genome sequencing characterization of virulence profiles of Listeria monocytogenes food and human isolates and in vitro adhesion/invasion assessment. Microorganisms 10: 62. https://doi.org/10.3390/microorganisms10010062 ![]() |
[124] |
Li W, Bai L, Fu P, et al. (2018) The epidemiology of Listeria monocytogenes in China. Foodborne Pathog Dis 15: 459-466. https://doi.org/10.1089/fpd.2017.2409 ![]() |
[125] |
Parra-Flores J, Holý O, Bustamante F, et al. (2022) Virulence and antibiotic resistance genes in Listeria monocytogenes strains isolated from ready-to-eat foods in Chile. Front Microbiol 12: 1-14. https://doi.org/10.3389/fmicb.2021.796040 ![]() |
[126] |
Schmitz-Esser S, Anast JM, Cortes BW (2021) A large-scale sequencing-based survey of plasmids in Listeria monocytogenes reveals global dissemination of plasmids. Front Microbiol 12: 1-17. https://doi.org/10.3389/fmicb.2021.653155 ![]() |
[127] |
García-Vela S, Ben Said L, Soltani S, et al. (2023) Targeting enterococci with antimicrobial activity against Clostridium perfringens from Poultry. Antibiotics 12: 231. https://doi.org/10.3390/antibiotics12020231 ![]() |
[128] |
Hashimoto Y, Suzuki M, Kobayashi S, et al. (2023) Enterococcal linear plasmids adapt to Enterococcus faecium and spread within multidrug-resistant clades. Antimicrob Agents Chemother 67: e0161922. https://doi.org/10.1128/aac.01619-22 ![]() |
[129] |
Centorotola G, Ziba MW, Cornacchia A, et al. (2023) Listeria monocytogenes in ready to eat meat products from Zambia: phenotypical and genomic characterization of isolates. Front Microbiol 14: 1228726. https://doi.org/10.3389/fmicb.2023.1228726 ![]() |
[130] |
Kuenne C, Voget S, Pischimarov J, et al. (2010) Comparative analysis of plasmids in the genus Listeria. PLoS One 5: e12511. https://doi.org/10.1371/journal.pone.0012511 ![]() |
[131] |
Hingston P, Brenner T, Truelstrup Hansen L, et al. (2019) Comparative analysis of Listeria monocytogenes plasmids and expression levels of plasmid-encoded genes during growth under salt and acid stress conditions. Toxins (Basel) 11: 426. https://doi.org/10.3390/toxins11070426 ![]() |
[132] |
Gilmour MW, Graham M, Van Domselaar G, et al. (2010) High-throughput genome sequencing of two Listeria monocytogenes clinical isolates during a large foodborne outbreak. BMC Genomics 11: 120. https://doi.org/10.1186/1471-2164-11-120 ![]() |
[133] |
Zhou M, Li Q, Yu S, et al. (2023) Co-proliferation of antimicrobial resistance genes in tilapia farming ponds associated with use of antimicrobials. Sci Total Environ 887: 164046. https://doi.org/10.1016/j.scitotenv.2023.164046 ![]() |
[134] |
Schwan CL, Lomonaco S, Bastos LM, et al. (2021) Genotypic and phenotypic characterization of antimicrobial resistance profiles in non-typhoidal Salmonella enterica strains isolated from cambodian informal markets. Front Microbiol 12: 711472. https://doi.org/10.3389/fmicb.2021.711472 ![]() |
[135] |
Lou Y, Liu H, Zhang Z, et al. (2016) Mismatch between antimicrobial resistance phenotype and genotype of pathogenic Vibrio parahaemolyticus isolated from seafood. Food Control 59: 207-211. https://doi.org/10.1016/j.foodcont.2015.04.039 ![]() |
[136] |
Neuert S, Nair S, Day MR, et al. (2018) Prediction of phenotypic antimicrobial resistance profiles from whole genome sequences of non-typhoidal Salmonella enterica. Front Microbiol 9: 1-11. https://doi.org/10.3389/fmicb.2018.00592 ![]() |
[137] |
Deekshit VK, Kumar BK, Rai P, et al. (2012) Detection of class 1 integrons in Salmonella Weltevreden and silent antibiotic resistance genes in some seafood-associated nontyphoidal isolates of Salmonella in south-west coast of India. J Appl Microbiol 112: 1113-1122. https://doi.org/10.1111/j.1365-2672.2012.05290.x ![]() |
[138] |
Liu X, Chen W, Fang Z, et al. (2022) Persistence of Listeria monocytogenes ST5 in Ready-to-Eat Food Processing Environment. Foods 11: 2561. https://doi.org/10.3390/foods11172561 ![]() |
[139] |
Pöntinen A, Aalto-Araneda M, Lindström M, et al. (2017) Heat resistance mediated by pLM58 plasmid-borne ClpL in Listeria monocytogenes. mSphere 2: 1-13. https://doi.org/10.1128/mSphere.00364-17 ![]() |
[140] |
Lungu B, O'Bryan CA, Muthaiyan A, et al. (2011) Listeria monocytogenes: antibiotic resistance in food production. Foodborne Pathog Dis 8: 569-578. https://doi.org/10.1089/fpd.2010.071 ![]() |
[141] |
Kapoor G, Saigal S, Elongavan A (2017) Action and resistance mechanisms of antibiotics: A guide for clinicians. J Anaesthesiol Clin Pharmacol 33: 300. https://doi.org/10.4103/joacp.JOACP_349_15 ![]() |
[142] |
Munoz-Lopez M, Garcia-Perez J (2010) DNA Transposons: Nature and applications in genomics. Curr Genomics 11: 115-128. https://doi.org/10.2174/138920210790886871 ![]() |
[143] |
Sévellec Y, Torresi M, Félix B, et al. (2020) First report on the finding of Listeria monocytogenes ST121 strain in a dolphin brain. Pathogens 9: 1-13. https://doi.org/10.3390/pathogens9100802 ![]() |
[144] |
Zuber I, Lakicevic B, Pietzka A, et al. (2019) Molecular characterization of Listeria monocytogenes isolates from a small-scale meat processor in Montenegro, 2011–2014. Food Microbiol 79: 116-122. https://doi.org/10.1016/j.fm.2018.12.005 ![]() |
[145] |
Manso B, Melero B, Stessl B, et al. (2019) Characterization of virulence and persistence abilities of Listeria monocytogenes strains isolated from food processing premises. J Food Prot 82: 1922-1930. https://doi.org/10.4315/0362-028X.JFP-19-109 ![]() |
[146] |
Ebner R, Stephan R, Althaus D, et al. (2015) Phenotypic and genotypic characteristics of Listeria monocytogenes strains isolated during 2011–2014 from different food matrices in Switzerland. Food Control 57: 321-326. https://doi.org/10.1016/j.foodcont.2015.04.030 ![]() |
[147] |
Kurpas M, Osek J, Moura A, et al. (2020) Genomic characterization of Listeria monocytogenes isolated from ready-to-eat meat and meat processing environments in Poland. Front Microbiol 11: 1-10. https://doi.org/10.3389/fmicb.2020.01412 ![]() |
[148] |
Koopmans MM, Bijlsma MW, Brouwer MC, et al. (2017) Listeria monocytogenes meningitis in the Netherlands, 1985–2014: A nationwide surveillance study. J Infect 75: 12-19. https://doi.org/10.1016/j.jinf.2017.04.004 ![]() |
[149] |
Piet J, Kieran J, Dara L, et al. (2016) Listeria monocytogenes in food: Control by monitoring the food processing environment. African J Microbiol Res 10: 1-14. https://doi.org/10.5897/AJMR2015.7832 ![]() |
![]() |
![]() |
1. | Xingmao Shao, Lun Xie, Chiqin Li, Zhiliang Wang, A Study on Networked Industrial Robots in Smart Manufacturing: Vulnerabilities, Data Integrity Attacks and Countermeasures, 2023, 109, 0921-0296, 10.1007/s10846-023-01984-2 | |
2. | Xiaoyu Wang, Tianbo Liu, Songping Mai, Respiratory motion tracking of the thoracoabdominal surface based on defect-aware point cloud registration, 2024, 14, 2093-9868, 1057, 10.1007/s13534-024-00390-3 | |
3. | Shuai Hu, Rongjian Lu, Yinlong Zhu, Wenhan Zhu, Hongzhe Jiang, Suzhao Bi, Application of Medical Image Navigation Technology in Minimally Invasive Puncture Robot, 2023, 23, 1424-8220, 7196, 10.3390/s23167196 | |
4. | Silong Zhang, Jicheng Chen, Hengkai Sun, Zhi Qi, Hui Zhang, A scientometric review of medical flexible needle systems in surgery: signal processing, navigation and control, 2024, 18, 1863-1703, 627, 10.1007/s11760-024-03179-0 |