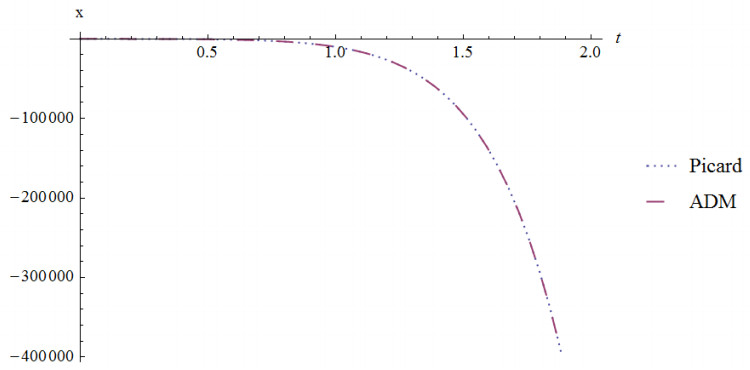
The demand for healthy food items with a high nutrient value of bioavailability and bioaccessibility has created a need for continuous development of technology and food ingredients like bioactive peptides. This study aimed to investigate seven proteolytic lactic acid bacteria (PLABs) isolated from the plaa-som (fermented fish) sample originated from silver BARB species for production of proteolytic enzymes. Proteolytic enzymes produced by (PLABs) were used further to create potent bioactive peptides by hydrolyzing proteins throughout PLAB-probiotics enhancer. Protein derived-bioactive peptides was tested the proteolytic activity on different protein sources and examined bioactivities including antioxidative and antimicrobial effect for further use in functional foods. Results of screened-PLAB strains showed high proteolytic activity namely Streptococcus thermophilus strains (KKUPA22 and KKUPK13). These strains have proteolytic system consisting of extracellular and cell-bound enzymes that used for degrading protein in fish flesh protein (FFP) and skim milk (SKM) broth media. Proteolytic activity of tested bacterial enzymes was estimated after incubation at 45, 37, and 50 °C. Furthermore, FFP hydrolysates were formed with various peptides and has small molecular weights (checked by SDS-PAGE) in the range of10.5 to 22 kDa), exhibiting strong activity. Data revealed that S. thermophilus strains (KKUPA22 and KKUPK13) had high antioxidant activity in term of 2,2-diphenyl-1-picrylhydrazyl (DPPH), 2,2-azinobis-(3-ethylbenzothiazoline-6-sulfonate) (ABTS) radical-scavenging inhibition, and ferric reducing antioxidant power (FRAP) reducing power capacity. Both strains (KKUPA22 and KKUPK13) of S. thermophilus have higher antimicrobial activity against Gram-negative bacteria than against Gram-positive bacteria. We have confirmed presence of proteolytic (prt) gene regions in S. thermophilus strains using specific primers via PCR amplification. Results showed highest homology (100%) with the prtS gene of S. thermophillus located on the cell envelope proteolytic enzymes (CEPEs) such as serine proteinase. Therefore, it concluded that the proteolytic system of tested PLAB strains able to generate bioactive peptides-derived proteins having active biological property, good mechanism of degradability, and bioaccessibility for further use in catalyzing protein of functional foods.
Citation: Srisan Phupaboon, Farah J. Hashim, Parichat Phumkhachorn, Pongsak Rattanachaikunsopon. Molecular and biotechnological characteristics of proteolytic activity from Streptococcus thermophilus as a proteolytic lactic acid bacteria to enhance protein-derived bioactive peptides[J]. AIMS Microbiology, 2023, 9(4): 591-611. doi: 10.3934/microbiol.2023031
[1] | Debao Yan . Existence results of fractional differential equations with nonlocal double-integral boundary conditions. Mathematical Biosciences and Engineering, 2023, 20(3): 4437-4454. doi: 10.3934/mbe.2023206 |
[2] | Abdon Atangana, Jyoti Mishra . Analysis of nonlinear ordinary differential equations with the generalized Mittag-Leffler kernel. Mathematical Biosciences and Engineering, 2023, 20(11): 19763-19780. doi: 10.3934/mbe.2023875 |
[3] | Allaberen Ashyralyev, Evren Hincal, Bilgen Kaymakamzade . Crank-Nicholson difference scheme for the system of nonlinear parabolic equations observing epidemic models with general nonlinear incidence rate. Mathematical Biosciences and Engineering, 2021, 18(6): 8883-8904. doi: 10.3934/mbe.2021438 |
[4] | Sebastian Builes, Jhoana P. Romero-Leiton, Leon A. Valencia . Deterministic, stochastic and fractional mathematical approaches applied to AMR. Mathematical Biosciences and Engineering, 2025, 22(2): 389-414. doi: 10.3934/mbe.2025015 |
[5] | Hardik Joshi, Brajesh Kumar Jha, Mehmet Yavuz . Modelling and analysis of fractional-order vaccination model for control of COVID-19 outbreak using real data. Mathematical Biosciences and Engineering, 2023, 20(1): 213-240. doi: 10.3934/mbe.2023010 |
[6] | Barbara Łupińska, Ewa Schmeidel . Analysis of some Katugampola fractional differential equations with fractional boundary conditions. Mathematical Biosciences and Engineering, 2021, 18(6): 7269-7279. doi: 10.3934/mbe.2021359 |
[7] | Jian Huang, Zhongdi Cen, Aimin Xu . An efficient numerical method for a time-fractional telegraph equation. Mathematical Biosciences and Engineering, 2022, 19(5): 4672-4689. doi: 10.3934/mbe.2022217 |
[8] | Yingying Xu, Chunhe Song, Chu Wang . Few-shot bearing fault detection based on multi-dimensional convolution and attention mechanism. Mathematical Biosciences and Engineering, 2024, 21(4): 4886-4907. doi: 10.3934/mbe.2024216 |
[9] | H. M. Srivastava, Khaled M. Saad, J. F. Gómez-Aguilar, Abdulrhman A. Almadiy . Some new mathematical models of the fractional-order system of human immune against IAV infection. Mathematical Biosciences and Engineering, 2020, 17(5): 4942-4969. doi: 10.3934/mbe.2020268 |
[10] | Guodong Li, Ying Zhang, Yajuan Guan, Wenjie Li . Stability analysis of multi-point boundary conditions for fractional differential equation with non-instantaneous integral impulse. Mathematical Biosciences and Engineering, 2023, 20(4): 7020-7041. doi: 10.3934/mbe.2023303 |
The demand for healthy food items with a high nutrient value of bioavailability and bioaccessibility has created a need for continuous development of technology and food ingredients like bioactive peptides. This study aimed to investigate seven proteolytic lactic acid bacteria (PLABs) isolated from the plaa-som (fermented fish) sample originated from silver BARB species for production of proteolytic enzymes. Proteolytic enzymes produced by (PLABs) were used further to create potent bioactive peptides by hydrolyzing proteins throughout PLAB-probiotics enhancer. Protein derived-bioactive peptides was tested the proteolytic activity on different protein sources and examined bioactivities including antioxidative and antimicrobial effect for further use in functional foods. Results of screened-PLAB strains showed high proteolytic activity namely Streptococcus thermophilus strains (KKUPA22 and KKUPK13). These strains have proteolytic system consisting of extracellular and cell-bound enzymes that used for degrading protein in fish flesh protein (FFP) and skim milk (SKM) broth media. Proteolytic activity of tested bacterial enzymes was estimated after incubation at 45, 37, and 50 °C. Furthermore, FFP hydrolysates were formed with various peptides and has small molecular weights (checked by SDS-PAGE) in the range of10.5 to 22 kDa), exhibiting strong activity. Data revealed that S. thermophilus strains (KKUPA22 and KKUPK13) had high antioxidant activity in term of 2,2-diphenyl-1-picrylhydrazyl (DPPH), 2,2-azinobis-(3-ethylbenzothiazoline-6-sulfonate) (ABTS) radical-scavenging inhibition, and ferric reducing antioxidant power (FRAP) reducing power capacity. Both strains (KKUPA22 and KKUPK13) of S. thermophilus have higher antimicrobial activity against Gram-negative bacteria than against Gram-positive bacteria. We have confirmed presence of proteolytic (prt) gene regions in S. thermophilus strains using specific primers via PCR amplification. Results showed highest homology (100%) with the prtS gene of S. thermophillus located on the cell envelope proteolytic enzymes (CEPEs) such as serine proteinase. Therefore, it concluded that the proteolytic system of tested PLAB strains able to generate bioactive peptides-derived proteins having active biological property, good mechanism of degradability, and bioaccessibility for further use in catalyzing protein of functional foods.
Fractional calculus is a main branch of mathematics that can be considered as the generalisation of integration and differentiation to arbitrary orders. This hypothesis begins with the assumptions of L. Euler (1730) and G. W. Leibniz (1695). Fractional differential equations (FDEs) have lately gained attention and publicity due to their realistic and accurate computations [1,2,3,4,5,6,7]. There are various types of fractional derivatives, including Riemann–Liouville, Caputo, Grü nwald–Letnikov, Weyl, Marchaud, and Atangana. This topic's history can be found in [8,9,10,11]. Undoubtedly, fractional calculus applies to mathematical models of different phenomena, sometimes more effectively than ordinary calculus [12,13]. As a result, it can illustrate a wide range of dynamical and engineering models with greater precision. Applications have been developed and investigated in a variety of scientific and engineering fields over the last few decades, including bioengineering [14], mechanics [15], optics [16], physics [17], mathematical biology, electrical power systems [18,19,20] and signal processing [21,22,23].
One of the definitions of fractional derivatives is Caputo-Fabrizo, which adds a new dimension in the study of FDEs. The new derivative's feature is that it has a nonsingular kernel, which is made from a combination of an ordinary derivative with an exponential function, but it has the same supplementary motivating properties with various scales as in the Riemann-Liouville fractional derivatives and Caputo. The Caputo-Fabrizio fractional derivative has been used to solve real-world problems in numerous areas of mathematical modelling for example, numerical solutions for groundwater pollution, the movement of waves on the surface of shallow water modelling [24], RLC circuit modelling [25], and heat transfer modelling [26,27] were discussed.
Rach (1987), Bellomo and Sarafyan (1987) first compared the Adomian Decomposition method (ADM) [28,29,30,31,32] to the Picard method on a variety of examples. These methods have many benefits: they effectively work with various types of linear and nonlinear equations and also provide an analytic solution for all of these equations with no linearization or discretization. These methods are more realistic compared with other numerical methods as each technique is used to solve a specific type of equations, on the other hand ADM and Picard are useful for many types of equations. In the numerical examples provided, we compare ADM and Picard solutions of multidimentional fractional order equations with Caputo-Fabrizio.
The fractional derivative of Caputo-Fabrizio for the function x(t) is defined as [33]
CFDα0x(t)=B(α)1−α∫t0dds(x(s)) e−α1−α(t−s)ds, | (1.1) |
and its corresponding fractional integral is
CFIαx(t)=1−αB(α)x(t)+αB(α)∫t0x (s)ds, 0<α<1, | (1.2) |
where x(t) be continuous and differentiable on [0, T]. Also, in the above definition, the function B(α)>0 is a normalized function which satisfy the condition B(0)=B(1)=0. The relation between the Caputo–Fabrizio fractional derivate and its corresponding integral is given by
(CFIα0)(CFDα0f(t))=f(t)−f(a). | (1.3) |
In this section, we will introduce a multidimentional FDE subject to the initial condition. Let α∈(0,1], 0<α1<α2<...,αm<1, and m is integer real number,
CFDx=f(t,x,CFDα1x,CFDα2x,...,CFDαmx,) ,x(0)=c0, | (2.1) |
where x=x(t),t∈J=[0,T],T∈R+,x∈C(J).
To facilitate the equation and make it easy for the calculation, we let x(t)=c0+X(t) so Eq (2.1) can be witten as
CFDαX=f(t,c0+X,CFDα1X,CFDα2X,...,CFDαmX), X(0)=0. | (2.2) |
the algorithm depends on converting the initial condition from a constant c0 to 0.
Let CFDαX=y(t) then X=CFIαy, so we have
CFDαiX= CFIα−αi CFDαX= CFIα−αiy, i=1,2,...,m. | (2.3) |
Substituting in Eq (2.2) we obtain
y=f(t,c0+ CFIαy, CFIα−α1y,..., CFIα−αmy). | (2.4) |
Assume f satisfies Lipschtiz condition with Lipschtiz constant L given by,
|f(t,y0,y1,...,ym)|−|f(t,z0,z1,...,zm)|≤Lm∑i=0|yi−zi|, | (2.5) |
which implies
|f(t,c0+CFIαy,CFIα−α1y,..,CFIα−αmy)−f(t,c0+CFIαz,CFIα−α1z,..,CFIα−αmz)|≤Lm∑i=0| CFIα−αiy− CFIα−αiz|. | (2.6) |
The solution algorithm of Eq (2.4) using ADM is,
y0(t)=a(t)yn+1(t)=An(t), j⩾0. | (2.7) |
where a(t) pocesses all free terms in Eq (2.4) and An are the Adomian polynomials of the nonlinear term which takes the form [34]
An=f(Sn)−n−1∑i=0Ai, | (2.8) |
where f(Sn)=∑ni=0Ai. Later, this accelerated formula of Adomian polynomial will be used in convergence analysis and error estimation. The solution of Eq (2.4) can be written in the form,
y(t)=∞∑i=0yi(t). | (2.9) |
lastly, the solution of the Eq (2.4) takes the form
x(t)=c0+X(t)=c0+ CFIαy(t). | (2.10) |
At which we convert the parameter to the initial form y to x in Eq (2.10), so we have the solution of the original Eq (2.1).
Define a mapping F:E→E where E=(C[J],‖⋅‖) is a Banach space of all continuous functions on J with the norm ‖x‖= maxtϵJx(t).
Theorem 3.1. Equation (2.4) has a unique solution whenever 0<ϕ<1 where ϕ=L(∑mi=0[(α−αi)(T−1)]+1B(α−αi)).
Proof. First, we define the mapping F:E→E as
Fy=f(t,c0+ CFIαy, CFIα−α1y,..., CFIα−αmy). |
Let y and z∈E are two different solutions of Eq (2.4). Then
Fy−Fz=f(t,c0+CFIαy,CFIα−α1y,..,CFIα−αmy)−f(t,c0+CFIαz,CFIα−α1z,...,CFIα−αmz) |
which implies that
|Fy−Fz|=|f(t,c0+ CFIαy, CFIα−α1y,..., CFIα−αmy)−f(t,c0+ CFIαz, CFIα−α1z,..., CFIα−αmz)|≤Lm∑i=0| CFIα−αiy− CFIα−αiz|≤Lm∑i=0|1−(α−αi)B(α−αi)(y−z)+α−αiB(α−αi)∫t0(y−z)ds|‖Fy−Fz‖≤Lm∑i=01−(α−αi)B(α−αi)maxtϵJ|y−z|+α−αiB(α−αi)maxtϵJ|y−z|∫t0ds≤Lm∑i=01−(α−αi)B(α−αi)‖y−z‖+α−αiB(α−αi)‖y−z‖T≤L‖y−z‖(m∑i=01−(α−αi)B(α−αi)+α−αiB(α−αi)T)≤L‖y−z‖(m∑i=0[(α−αi)(T−1)]+1B(α−αi))≤ϕ‖y−z‖. |
under the condition 0<ϕ<1, the mapping F is contraction and hence there exists a unique solution y∈C[J] for the problem Eq (2.4) and this completes the proof.
Theorem 3.2. The series solution of the problem Eq (2.4)converges if |y1(t)|<c and c isfinite.
Proof. Define a sequence {Sp} such that Sp=∑pi=0yi(t) is the sequence of partial sums from the series solution ∑∞i=0yi(t), we have
f(t,c0+ CFIαy, CFIα−α1y,..., CFIα−αmy)=∞∑i=0Ai, |
So
f(t,c0+ CFIαSp, CFIα−α1Sp,..., CFIα−αmSp)=p∑i=0Ai, |
From Eq (2.7) we have
∞∑i=0yi(t)=a(t)+∞∑i=0Ai−1 |
let Sp,Sq be two arbitrary sums with p⩾q. Now, we are going to prove that {Sp} is a Caushy sequence in this Banach space. We have
Sp=p∑i=0yi(t)=a(t)+p∑i=0Ai−1,Sq=q∑i=0yi(t)=a(t)+q∑i=0Ai−1. |
Sp−Sq=p∑i=0Ai−1−q∑i=0Ai−1=p∑i=q+1Ai−1=p−1∑i=qAi−1=f(t,c0+ CFIαSp−1, CFIα−α1Sp−1,..., CFIα−αmSp−1)−f(t,c0+ CFIαSq−1, CFIα−α1Sq−1,..., CFIα−αmSq−1) |
|Sp−Sq|=|f(t,c0+ CFIαSp−1, CFIα−α1Sp−1,..., CFIα−αmSp−1)−f(t,c0+ CFIαSq−1, CFIα−α1Sq−1,..., CFIα−αmSq−1)|≤Lm∑i=0| CFIα−αiSp−1− CFIα−αiSq−1|≤Lm∑i=0|1−(α−αi)B(α−αi)(Sp−1−Sq−1)+α−αiB(α−αi)∫t0(Sp−1−Sq−1)ds|≤Lm∑i=01−(α−αi)B(α−αi)|Sp−1−Sq−1|+α−αiB(α−αi)∫t0|Sp−1−Sq−1|ds |
‖Sp−Sq‖≤Lm∑i=01−(α−αi)B(α−αi)maxtϵJ|Sp−1−Sq−1|+α−αiB(α−αi)maxtϵJ|Sp−1−Sq−1|∫t0ds≤L‖Sp−Sq‖m∑i=0(1−(α−αi)B(α−αi)+α−αiB(α−αi)T)≤L‖Sp−Sq‖(m∑i=0[(α−αi)(T−1)]+1B(α−αi))≤ϕ‖Sp−Sq‖ |
let p=q+1 then,
‖Sq+1−Sq‖≤ϕ‖Sq−Sq−1‖≤ϕ2‖Sq−1−Sq−2‖≤...≤ϕq‖S1−S0‖ |
From the triangle inequality we have
‖Sp−Sq‖≤‖Sq+1−Sq‖+‖Sq+2−Sq+1‖+...‖Sp−Sp−1‖≤[ϕq+ϕq+1+...+ϕp−1]‖S1−S0‖≤ϕq[1+ϕ+...+ϕp−q+1]‖S1−S0‖≤ϕq[1−ϕp−q1−ϕ]‖y1(t)‖ |
Since 0<ϕ<1,p⩾q then (1−ϕp−q)≤1. Consequently
‖Sp−Sq‖≤ϕq1−ϕ‖y1(t)‖≤ϕq1−ϕmax∀tϵJ|y1(t)| | (3.1) |
but |y1(t)|<∞ and as q→∞ then, ‖Sp−Sq‖→0 and hence, {Sp} is a Caushy sequence in this Banach space then the proof is complete.
Theorem 3.3. The maximum absolute truncated error Eq (2.4)is estimated to be maxtϵJ|y(t)−∑qi=0yi(t)|≤ϕq1−ϕmaxtϵJ|y1(t)|
Proof. From the convergence theorm inequality (Eq 3.1) we have
‖Sp−Sq‖≤ϕq1−ϕmaxtϵJ|y1(t)| |
but, Sp=∑pi=0yi(t) as p→∞ then, Sp→y(t) so,
‖y(t)−Sq‖≤ϕq1−ϕmaxtϵJ|y1(t)| |
so, the maximum absolute truncated error in the interval J is,
maxtϵJ|y(t)−q∑i=0yi(t)|≤ϕq1−ϕmaxtϵJ|y1(t)| | (3.2) |
and this completes the proof.
In this part, we introduce several numerical examples with unkown exact solution and we will use inequality (Eq 3.2) to estimate the maximum absolute truncated error.
Example 4.1. Application of linear FDE
CFDx(t)+2aCFD1/2x(t)+bx(t)=0, x(0)=1. | (4.1) |
A Basset problem in fluid dynamics is a classical problem which is used to study the unsteady movement of an accelerating particle in a viscous fluid under the action of the gravity [36]
Set
X(t)=x(t)−1 |
Equation (4.1) will be
CFDX(t)+2aCFD1/2X(t)+bX(t)=0, X(0)=0. | (4.2) |
Appling Eq (2.3) to Eq (4.2), and using initial condition, also we take a = 1, b = 1/2,
y=−12−2I1/2y−12I y | (4.3) |
Appling ADM to Eq (4.3), we find the solution algorithm become
y0(t)=−12,yi(t)=−2 CFI1/2yi−1−12 CFI yi−1, i≥1. | (4.4) |
Appling Picard solution to Eq (4.2), we find the solution algorithm become
y0(t)=−12,yi(t)=−12−2 CFI1/2yi−1−12 CFI yi−1, i≥1. | (4.5) |
From Eq (4.4), the solution using ADM is given by y(t)=Limq→∞qi=0yi(t) while from Eq (4.5), the solution using Picard technique is given by y(t)=Limi→∞yi(t). Lately, the solution of the original problem Eq (4.2), is
x(t)=1+ CFI y(t). |
One the same processor (q = 20), the time consumed using ADM is 0.037 seconds, while the time consumed using Picard is 7.955 seconds.
Figure 1 gives a comparison between ADM and Picard solution of Ex. 4.1.
Example 4.2. Consider the following nonlinear FDE [35]
CFD1/2x=8t3/23√π−t7/44Γ(114)−t44+18 CFD1/4x+14x2, x(0)=0. | (4.6) |
Appling Eq (2.3) to Eq (4.6), and using initial condition,
y=8t3/23√π−t7/44Γ(114)−t44+18 CFI1/4y+14(CFI1/2y)2. | (4.7) |
Appling ADM to Eq (4.7), we find the solution algorithm will be become
y0(t)=8t3/23√π−t7/44Γ(114)−t44,yi(t)=18 CFI1/4yi−1+14(Ai−1), i≥1. | (4.8) |
at which Ai are Adomian polynomial of the nonliner term (CFI1/2y)2.
Appling Picard solution to Eq (4.7), we find the the solution algorithm become
y0(t)=8t3/23√π−t7/44Γ(114)−t44,yi(t)=y0(t)+18 CFI1/4yi−1+14(CFI1/2yi−1)2, i≥1. | (4.9) |
From Eq (4.8), the solution using ADM is given by y(t)=Limq→∞qi=0yi(t) while from Eq (4.9), the solution using Picard technique is given by y(t)=Limi→∞yi(t). Finally, the solution of the original problem Eq (4.7), is.
x(t)= CFI1/2y. |
One the same processor (q = 2), the time consumed using ADM is 65.13 seconds, while the time consumed using Picard is 544.787 seconds.
Table 1 showed the maximum absolute truncated error of of ADM solution (using Theorem 3.3) at different values of m (when t = 0:5; N = 2):
q | max. absolute error |
2 | 0.114548 |
5 | 0.099186 |
10 | 0.004363 |
Figure 2 gives a comparison between ADM and Picard solution of Ex. 4.2.
Example 4.3. Consider the following nonlinear FDE [35]
CFDαx=3t2−128125πt5+110(CFD1/2x)2,x(0)=0. | (4.10) |
Appling Eq (2.3) to Eq (4.10), and using initial condition,
y=3t2−128125πt5+110(CFI1/2y)2 | (4.11) |
Appling ADM to Eq (4.11), we find the solution algorithm become
y0(t)=3t2−128125πt5,yi(t)=110(Ai−1), i≥1 | (4.12) |
at which Ai are Adomian polynomial of the nonliner term (CFI1/2y)2.
Then appling Picard solution to Eq (4.11), we find the solution algorithm become
y0(t)=3t2−128125πt5,yi(t)=y0(t)+110(CFI1/2yi−1)2, i≥1. | (4.13) |
From Eq (4.12), the solution using ADM is given by y(t)=Limq→∞qi=0yi(t) while from Eq (4.13), the solution is y(t)=Limi→∞yi(t). Finally, the solution of the original problem Eq (4.11), is
x(t)=CFIy(t). |
One the same processor (q = 4), the time consumed using ADM is 2.09 seconds, while the time consumed using Picard is 44.725 seconds.
Table 2 showed the maximum absolute truncated error of of ADM solution (using Theorem 3.3) at different values of m (when t = 0:5; N = 4):
q | max. absolute error |
2 | 0.00222433 |
5 | 0.0000326908 |
10 | 2.88273*10−8 |
Figure 3 gives a comparison between ADM and Picard solution of Ex. 4.3 with α=1.
Example 4.4. Consider the following nonlinear FDE [35]
CFDαx=t2+12 CFDα1x+14 CFDα2x+16 CFDα3x+18x4,x(0)=0. | (4.14) |
Appling Eq (2.3) to Eq (4.10), and using initial condition,
y=t2+12(CFIα−α1y)+14(CFIα−α2y)+16(CFIα−α3y)+18(CFIαy)4, | (4.15) |
Appling ADM to Eq (4.15), we find the solution algorithm become
y0(t)=t2,yi(t)=12(CFIα−α1y)+14(CFIα−α2y)+16(CFIα−α3y)+18Ai−1, i≥1 | (4.16) |
where Ai are Adomian polynomial of the nonliner term (CFIαy)4.
Then appling Picard solution to Eq (4.15), we find the solution algorithm become
y0(t)=t2,yi(t)=t2+12(CFIα−α1yi−1)+14(CFIα−α2yi−1)+16(CFIα−α3yi−1)+18(CFIαyi−1)4 i≥1. | (4.17) |
From Eq (4.16), the solution using ADM is given by y(t)=Limq→∞qi=0yi(t) while from Eq (4.17), the solution using Picard technique is y(t)=Limi→∞yi(t). Finally, the solution of the original problem Eq (4.14), is
x(t)=CFIαy(t). |
One the same processor (q = 3), the time consumed using ADM is 0.437 seconds, while the time consumed using Picard is (16.816) seconds. Figure 4 shows a comparison between ADM and Picard solution of Ex. 4.4 atα=0.7,α1=0.1,α2=0.3,α3=0.5.
The Caputo-Fabrizo fractional deivative has a nonsingular kernel, and consequently, this definition is appropriate in solving nonlinear multidimensional FDE [37,38]. Since the selected numerical problems have an unkown exact solution, the formula (3.2) can be used to estimate the maximum absolute truncated error. By comparing the time taken on the same processor (i7-2670QM), it was found that the time consumed by ADM is much smaller compared with the Picard technique. Furthermore Picard gives a more accurate solution than ADM at the same interval with the same number of terms.
The authors declare there is no conflict of interest.
[1] |
Kieliszek M, Pobiega K, Piwowarek K, et al. (2021) Molecules characteristics of the proteolytic enzymes produced by lactic acid bacteria. Molecules 26: 1858. https://doi.org/10.3390/molecules26071858 ![]() |
[2] |
Muhsin MBY, Majeed HZ, Mohammed BB, et al. (2018) Lactic acid bacteria biosurfactant role that isolated from human breast milk in inhibit eyes pathogenic bacteria. Ibn Al-Haitham J Pure Appl Sci 31: 31-40. https://doi.org/10.30526/31.2.1959 ![]() |
[3] |
Phumkhachorn P, Rattanachaikunsopon P (2023) Probiotics: Sources, selection and health benefits. Res J Biotechnol 18: 102-113. https://doi.org/10.25303/1805rjbt1020113 ![]() |
[4] |
Punyauppa-path S, Kiatprasert P, Sawangkaew J, et al. (2022) Diversity of fermentative yeasts with probiotic potential isolated from Thai fermented food products. AIMS Microbiology 8: 575-594. https://doi.10.3934/microbiol.2022037 ![]() |
[5] |
Chang OK, Roux É, Awussi AA, et al. (2014) Use of a free form of the Streptococcus thermophilus cell envelope protease PrtS as a tool to produce bioactive peptides. Int Dairy J 38: 104-115. https://doi:10.1016/J.IDAIRYJ.2014.01.008 ![]() |
[6] |
Jemil I, Abdelhedi O, Nasri R, et al. (2017) Novel bioactive peptides from enzymatic hydrolysate of Sardinella, Sardinella aurita muscle proteins hydrolysed by Bacillus subtilis A26 proteases. Food Res Int 100: 121-133. https://doi:10.1016/j.foodres.2017.06.018 ![]() |
[7] |
Miclo L, Roux É, Genay M, et al. (2012) Variability of hydrolysis of β-, α S1-, and α S2-caseins by 10 strains of Streptococcus thermophilus and resulting bioactive peptides. J Agric Food Chem 60: 554-565. https://doi:10.1021/jf202176d ![]() |
[8] |
Raveschot C, Cudennec B, Coutte F, et al. (2018) Production of bioactive peptides by Lactobacillus species: From gene to application. Front Microbiol 9: 2354. https://doi.org/10.3389/fmicb.2018.02354 ![]() |
[9] |
Gué Don E, Renault P, Dusko Ehrlich S, et al. (2001) Transcriptional pattern of genes coding for the proteolytic system of Lactococcus lactis and evidence for coordinated regulation of key enzymes by peptide supply. J Bacteriol 183: 3614-3622. https://doi:10.1128/JB.183.12.3614-3622.2001 ![]() |
[10] |
Christensen JE, Dudley EG, Pederson JA, et al. (1990) Peptidases and amino acid catabolism in lactic acid bacteria. Antonie Van Leeuwenhoek 76: 217-246. https://doi:10.1007/978-94-017-2027-4_11 ![]() |
[11] |
Chang OK, Perrin C, Galia W, et al. (2012) Release of the cell-envelope protease PrtS in the growth medium of Streptococcus thermophilus 4F44. Int Dairy J 23: 91-98. https://doi:10.1016/j.idairyj.2011.10.014 ![]() |
[12] |
Lozo J, Strahinic I, Dalgalarrondo M, et al. (2011) Comparative analysis of β-casein proteolysis by PrtP proteinase from Lactobacillus paracasei subsp. paracasei BGHN14, PrtR proteinase from Lactobacillus rhamnosus BGT10 and PrtH Proteinase from Lactobacillus helveticus BGRA43. Int Dairy J 21: 863-868. https://doi:10.1016/J.IDAIRYJ.2011.05.002 ![]() |
[13] |
Vukotić G, Strahinić I, Begović J, et al. (2016) Survey on proteolytic activity and diversity of proteinase genes in mesophilic lactobacilli. Microbiology 85: 33-41. https://doi:10.1134/S002626171601015X ![]() |
[14] |
Genay M, Sadat L, Gagnaire V, et al. (2009) PrtH2, not PrtH, is the ubiquitous cell wall proteinase gene in Lactobacillus helveticus. Appl Environ Microbiol 75: 3238-3249. https://doi:10.1128/AEM.02395-08 ![]() |
[15] | Phupaboon S, Ratha J, Piyatheerawong W, et al. (2022) Application of a modified membrane-trapping technique to determine fish microflora species from Thai silver BARB (Barbonymus gonionotus). Asia-Pac J Sci Technol 27: 1-7. https://doi.org/10.14456/apst.2022.58 |
[16] |
Phupaboon S, Punyauppa-Path S, Kontongdee P, et al. (2022) Development and enhancement of antioxidant peptides from spontaneous plaa-som fermentation co-stimulated with Chiangrai-Phulae pineapple enzymatic reaction. Int Food Res J 29: 406-415. https://doi.org/10.47836/ifrj.29.2.18 ![]() |
[17] |
Phupaboon S, Kontongdee P, Hashim FJ, et al. (2022) Bioaccessibility and microencapsulation of Lactobacillus sp. to enhance nham protein hydrolysates in Thai fermented sausage. Foods 11: 3846. https://doi.org/10.3390/foods11233846 ![]() |
[18] | Phupaboon S, Piyatheerawong W, Yunchalard S (2020) Correlation of antioxidant activity and protein alteration of silver BARB protein hydrolysates. Asia-Pac J Sci Technol 25: 1-9. https://doi:10.14456/APST.2020.35 |
[19] |
Cao CC, Feng MQ, Sun J, et al. (2019) Screening of lactic acid bacteria with high protease activity from fermented sausages and antioxidant activity assessment of its fermented sausages. CYTA-J Food 17: 347-354. https://doi:10.1080/19476337.2019.1583687 ![]() |
[20] |
Keay L, Wildi BS (1970) Proteases of the genus Bacillus neutral proteases. Biotechnol Bioeng 12: 179-212. https://doi:10.1002/bit.260120205 ![]() |
[21] |
Cheon MJ, Lim SM, Lee NK, et al. (2020) Probiotic properties and neuroprotective effects of Lactobacillus buchneri KU200793 isolated from Korean fermented foods. Int J Mol Sci 21: 1227. https://doi:10.3390/ijms21041227 ![]() |
[22] |
Laemmli UK (1970) Cleavage of structural proteins during the assembly of the head of bacteriophage T4. Nature 227: 680-685. https://doi:10.1038/227680a0 ![]() |
[23] |
Arnao MB, Cano A, Acosta M (2001) The hydrophilic and lipophilic contribution to total antioxidant activity. Food Chem 73: 239-244. https://doi:10.1016/S0308-8146(00)00324-1 ![]() |
[24] |
Binsan W, Benjakul S, Visessanguan W, et al. (2008) Antioxidative activity of mungoong, an extract paste, from the cephalothorax of white shrimp (Litopenaeus vannamei). Food Chem 106: 185-193. https://doi:10.1016/J.FOODCHEM.2007.05.065 ![]() |
[25] |
Hashim FJ, Vichitphan S, Boonsiri P, et al. (2021) Neuroprotective assessment of Moringa oleifera leaves extract against oxidativ-stress-induced cytotoxicity in SHSY5Y neuroblastoma cells. Plants 10: 889. https://doi.org/10.3390/plants10050889 ![]() |
[26] |
Benzie IFF, Strain JJ (1996) The ferric reducing ability of plasma (FRAP) as a measure of “antioxidant power”: The FRAP assay. Anal Biochem 239: 70-76. https://doi:10.1006/ABIO.1996.0292 ![]() |
[27] |
Alsaraf KM, Abd ST, Husain NS (2016) An antimicrobial activity of Moringa oleifera extract in comparison to chlorhexidene gluconate (in vitro study). J Bagh Coll Dent 28: 183-187. https://doi.org/10.12816/0024732 ![]() |
[28] |
Drosinos EH, Paramithiotis S, Kolovos G, et al. (2007) Phenotypic and technological diversity of lactic acid bacteria and Staphylococci isolated from traditionally fermented sausages in southern greece. Food Microbiol 24: 260-270. https://doi:10.1016/J.FM.2006.05.001 ![]() |
[29] |
Pailin T, Kang DH, Schmidt K, et al. (2001) Detection of extracellular bound proteinase in EPS-producing lactic acid bacteria cultures on skim milk agar. Lett Appl Microbiol 33: 45-49. https://doi:10.1046/j.1472-765X.2001.00954.x ![]() |
[30] |
Kitaevskaya SV, Ponomarev VY, Yunusov ES (2022) Research of fermentation processes of protein substrates by consortiums of lactic acid bacteria. IOP Conf Ser: Earth Environ Sci 978: 012052. https://doi:10.1088/1755-1315/978/1/012052 ![]() |
[31] |
Wu Y, Min Cao S (2018) Study on endogenous protease and protein degradation of dry-salted. Decapterus maruadsi. CyTA-J Food 16: 350-356. https://doi:10.1080/19476337.2017.1406006 ![]() |
[32] |
Corsetti A, Gobbetti M, Marco BD, et al. (2000) Combined effect of sourdough lactic acid bacteria and additives on bread firmness and staling. J Agri Food Chem 48: 3044-3051. https://doi:10.1021/JF990853E ![]() |
[33] |
Dakwa S, Sakyi-Dawson E, Diako C, et al. (2005) Effect of boiling and roasting on the fermentation of soybeans into Dawadawa (soy-Dawadawa). Int J Food Microbiol 104: 69-82. https://doi:10.1016/J.IJFOODMICRO.2005.02.006 ![]() |
[34] |
Di Cagno R, De Angelis M, Lavermicocca P, et al. (2002) Proteolysis by sourdough lactic acid bacteria: Effects on wheat flour protein fractions and gliadin peptides involved in human cereal intolerance. Appl Environ Microbiol 68: 623-633. https://doi:10.1128/aem.68.2.623-633.2002 ![]() |
[35] |
Kazanas N (1968) Proteolytic activity of microorganisms isolated from freshwater fish. Appl Microbiol 16: 128-132. https://doi.org/10.1128/am.16.1.128-132.1968 ![]() |
[36] | Argyle PJ, Mathison GE, Chandan RC (1976) Production of cell-bound proteinase by Lactobacillus bulgaricus and its location in the bacterial cell. J Appl Microbiol 41: 175-184. https://doi:10.1111/j.1365-2672.1976.tb00616.x |
[37] |
Joo HS, Kumar CG, Park GC, et al. (2002) Optimization of the production of an extracellular alkaline protease from Bacillus horikoshii. Process Biochem 38: 155-159. https://doi:10.1016/S0032-9592(02)00061-4 ![]() |
[38] |
Patel R, Dodia M, Singh SP (2005) Extracellular alkaline protease from a newly isolated haloalkaliphilic Bacillus sp.: Production and optimization. Process Biochem 40: 3569-3575. https://doi:10.1016/J.PROCBIO.2005.03.049 ![]() |
[39] |
Chabasri T, Klanrit P, Yunchalard S (2010) Starters cultures development process by using their enzymatic activities on the carbohydrates constituents in plaa-som fermentation process as the primary selected criterion. J Biotechnol 150: 312-313. https://doi:10.1016/j.jbiotec.2010.09.291 ![]() |
[40] |
Zhang J, Liu M, Xu J, et al. (2020) First insight into the probiotic properties of ten Streptococcus thermophilus strains based on in vitro conditions. Curr Microbiol 77: 343-352. https://doi:10.1007/s00284-019-01840-3 ![]() |
[41] |
Paz D, Aleman RS, Cedillos R, et al. (2022) Probiotic characteristics of Streptococcus thermophilus and Lactobacillus delbrueckii ssp. bulgaricus as influenced by Carao (Cassia Grandis). Fermentation 8: 499. https://doi:10.3390/fermentation8100499 ![]() |
[42] |
Escamilla Rosales MF, Olvera Rosales L, Jara Gutiérrez CE, et al. (2023) Proteins of milk, egg and fish as a source of antioxidant peptides: production, mechanism of action and health benefits. Food Rev Int 39: 1-21. https://doi.org/10.1080/87559129.2023.2227974 ![]() |
[43] |
Kasankala LM, Xiong YL, Chen J (2012) Enzymatic activity and flavor compound production in fermented silver carp fish paste inoculated with Douchi starter culture. J Agric Food Chem 60: 226-233. https://doi:10.1021/jf203887x ![]() |
[44] |
Chamignon C, Guéneau V, Medina S, et al. (2020) Evaluation of the probiotic properties and the capacity to form biofilms of various Lactobacillus strains. Microorganisms 8: 1-15. https://doi:10.3390/microorganisms8071053 ![]() |
[45] |
Kim SK, Wijesekara I (2010) Development and biological activities of marine-derived bioactive peptides: A review. J Funct Foods 2: 1-9. https://doi:10.1016/j.jff.2010.01.003 ![]() |
[46] |
Najafian L, Babji AS (2012) A review of fish-derived antioxidant and antimicrobial peptides: Their Production, assessment, and applications. Peptides 33: 178-185. https://doi.org/10.1016/j.peptides.2011.11.013 ![]() |
[47] |
Geeta, Yadav AS (2017) Antioxidant and antimicrobial profile of chicken sausages prepared after fermentation of minced chicken meat with Lactobacillus plantarum and with additional dextrose and starch. LWT 77: 249-258. https://doi:10.1016/J.LWT.2016.11.050 ![]() |
[48] |
Ozyurt CE, Boga EK, Ozkutuk AS, et al. (2020) Bioconversion of discard fish (Equulites klunzingeri and Carassius gibelio) fermented with natural lactic acid bacteria; the chemical and microbiological quality of ensilage. Waste Biomass Valorization 11: 1435-1442. https://doi:10.1007/s12649-018-0493-5 ![]() |
[49] |
Zhang Q, Song C, Zhao J, et al. (2018) Molecules separation and characterization of antioxidative and angiotensin converting enzyme inhibitory peptide from jellyfish gonad hydrolysate. Molecules 23: 94. https://doi.org/10.3390/molecules23010094 ![]() |
[50] |
Dharmisthaben P, Basaiawmoit B, Sakure A, et al. (2021) Exploring potentials of antioxidative, anti-inflammatory activities and production of bioactive peptides in lactic fermented camel milk. Food Biosci 44: 101404. https://doi:10.1016/J.FBIO.2021.101404 ![]() |
[51] |
Homayouni-Tabrizi M, Shabestarin H, Asoodeh A, et al. (2016) Identification of two novel antioxidant peptides from camel milk using digestive proteases: Impact on expression gene of superoxide dismutase (SOD) in hepatocellular carcinoma cell line. Int J Pept Res Ther 22: 187-195. https://doi:10.1007/s10989-015-9497-1 ![]() |
[52] |
Wang Q, Liu FJ, Wang XM, et al. (2022) Preparation and hepatoprotective activities of peptides derived from mussels (Mytilus edulis) and clams (Ruditapes philippinarum). Mar Drugs 20: 719. https://doi:10.3390/md20110719 ![]() |
[53] |
Zhu Y, Lao F, Pan X, et al. (2022) Food protein-derived antioxidant peptides: Molecular mechanism, stability and bioavailability. Biomolecules 12: 1622. https://doi:10.3390/biom12111622 ![]() |
[54] |
Bulet P, Stö R, Menin L, et al. (2004) Anti-microbial peptides: From invertebrates to vertebrates. Immunol Rev 198: 169-184. https://doi:10.1111/j.0105-2896.2004.0124.x ![]() |
[55] |
Reddy KVR, Yedery RD, Aranha C (2004) Antimicrobial peptides: Premises and promises. Int J Antimicrob Agents 24: 536-547. https://doi:10.1016/J.IJANTIMICAG.2004.09.005 ![]() |
[56] |
Rajanbabu V, Chen JY (2011) Applications of antimicrobial peptides from fish and perspectives for the future. Peptides 32: 415-420. https://doi:10.1016/J.PEPTIDES.2010.11.005 ![]() |
[57] |
Chang WT, Pan CY, Rajanbabu V, et al. (2011) Tilapia (Oreochromis mossambicus) antimicrobial peptide, Hepcidin 1–5, shows antitumor activity in cancer cells. Peptides 32: 342-352. https://doi:10.1016/J.PEPTIDES.2010.11.003 ![]() |
[58] |
Aissaoui N, Chobert JM, Haertlé T, et al. (2017) Purification and biochemical characterization of a neutral serine protease from Trichoderma harzianum use in antibacterial peptide production from a fish by-product hydrolysate. Appl Biochem Biotechnol 182: 831-845. https://doi:10.1007/s12010-016-2365-4 ![]() |
[59] |
Lai C, Chen J, Liu J, et al. (2022) New polyketides from a hydrothermal vent sediment fungus Trichoderma sp. JWM29-10-1 and their antimicrobial effects. Mar Drugs 20: 720. https://doi:10.3390/md20110720 ![]() |
[60] |
Hou JC, Liu F, Ren DX, et al. (2015) Effect of culturing conditions on the expression of key enzymes in the proteolytic system of Lactobacillus Bulgaricus. J Zhejiang Univ-Sci B 16: 317-326. https://doi:10.1631/jzus.B1400230 ![]() |
[61] |
Alcántara C, Bäuerl C, Revilla-Guarinos A, et al. (2016) Peptide and amino acid metabolism is controlled by an OmpR-family response regulator in Lactobacillus casei. Mol Microbiol 100: 25-41. https://doi:10.1111/MMI.13299 ![]() |
[62] |
Griffiths MW, Tellez AM (2013) Lactobacillus Helveticus: The proteolytic system. Front Microbiol 4: 30. https://doi.org/10.3389/fmicb.2013.00030 ![]() |
[63] |
Courtin P, Monnet V, Rul F (2002) Cell-wall proteinases PrtS and PrtB have a different role in Streptococcus thermophilus/Lactobacillus bulgaricus mixed cultures in milk. Microbiology 148: 3413-3421. https://doi.org/10.1099/00221287-148-11-3413 ![]() |
[64] |
Kliche T, Li B, Bockelmann W, et al. (2017) Screening for proteolytically active lactic acid bacteria and bioactivity of peptide hydrolysates obtained with selected strains. Appl Microbiol Biotechnol 101: 7621-7633. https://doi: 10.1007/s00253-017-8369-3 ![]() |
[65] |
Sadat-Mekmene L, Genay M, Atlan D, et al. (2011) Original features of cell-envelope proteinases of Lactobacillus helveticus: A review. Int J Food Microbiol 146: 1-13. https://doi:10.1016/J.IJFOODMICRO.2011.01.039 ![]() |
[66] |
Najim NH (2010) Influence of mild pulsed electric field conditions on the growth and protease activity of Streptococcus thermophilus. Iraqi J Vet Med 34: 20-29. https://doi.org/10.30539/ijvm.v34i2.1396 ![]() |
1. | Eman A. A. Ziada, Salwa El-Morsy, Osama Moaaz, Sameh S. Askar, Ahmad M. Alshamrani, Monica Botros, Solution of the SIR epidemic model of arbitrary orders containing Caputo-Fabrizio, Atangana-Baleanu and Caputo derivatives, 2024, 9, 2473-6988, 18324, 10.3934/math.2024894 | |
2. | H. Salah, M. Anis, C. Cesarano, S. S. Askar, A. M. Alshamrani, E. M. Elabbasy, Fourth-order differential equations with neutral delay: Investigation of monotonic and oscillatory features, 2024, 9, 2473-6988, 34224, 10.3934/math.20241630 | |
3. | Said R. Grace, Gokula N. Chhatria, S. Kaleeswari, Yousef Alnafisah, Osama Moaaz, Forced-Perturbed Fractional Differential Equations of Higher Order: Asymptotic Properties of Non-Oscillatory Solutions, 2024, 9, 2504-3110, 6, 10.3390/fractalfract9010006 | |
4. | A.E. Matouk, Monica Botros, Hidden chaotic attractors and self-excited chaotic attractors in a novel circuit system via Grünwald–Letnikov, Caputo-Fabrizio and Atangana-Baleanu fractional operators, 2025, 116, 11100168, 525, 10.1016/j.aej.2024.12.064 | |
5. | Zahra Barati, Maryam Keshavarzi, Samaneh Mosaferi, Anatomical and micromorphological study of Phalaris (Poaceae) species in Iran, 2025, 68, 1588-4082, 9, 10.14232/abs.2024.1.9-15 |
q | max. absolute error |
2 | 0.114548 |
5 | 0.099186 |
10 | 0.004363 |
q | max. absolute error |
2 | 0.00222433 |
5 | 0.0000326908 |
10 | 2.88273*10−8 |