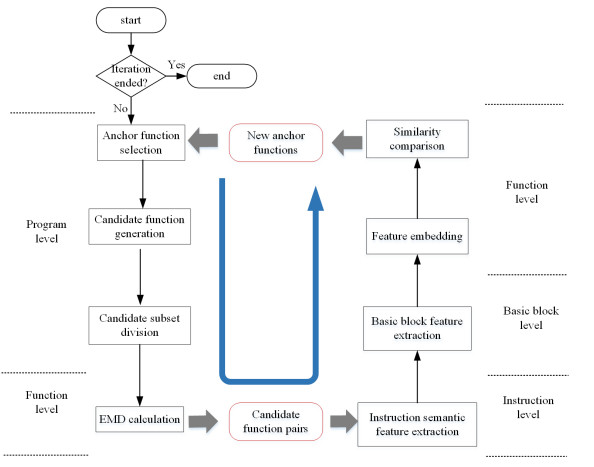
Citation: Marco Pegoraro, Elisabetta Benevento, Davide Aloini, Wil M.P. van der Aalst. Advances in computational methods for process and data mining in healthcare[J]. Mathematical Biosciences and Engineering, 2024, 21(7): 6603-6607. doi: 10.3934/mbe.2024288
[1] | Naila Naz, Muazzam A Khan, Suliman A. Alsuhibany, Muhammad Diyan, Zhiyuan Tan, Muhammad Almas Khan, Jawad Ahmad . Ensemble learning-based IDS for sensors telemetry data in IoT networks. Mathematical Biosciences and Engineering, 2022, 19(10): 10550-10580. doi: 10.3934/mbe.2022493 |
[2] | Hongan Li, Qiaoxue Zheng, Wenjing Yan, Ruolin Tao, Xin Qi, Zheng Wen . Image super-resolution reconstruction for secure data transmission in Internet of Things environment. Mathematical Biosciences and Engineering, 2021, 18(5): 6652-6671. doi: 10.3934/mbe.2021330 |
[3] | Jiushuang Wang, Ying Liu, Huifen Feng . IFACNN: efficient DDoS attack detection based on improved firefly algorithm to optimize convolutional neural networks. Mathematical Biosciences and Engineering, 2022, 19(2): 1280-1303. doi: 10.3934/mbe.2022059 |
[4] | Gang Cao, Antao Zhou, Xianglin Huang, Gege Song, Lifang Yang, Yonggui Zhu . Resampling detection of recompressed images via dual-stream convolutional neural network. Mathematical Biosciences and Engineering, 2019, 16(5): 5022-5040. doi: 10.3934/mbe.2019253 |
[5] | Xiaodong Zhu, Liehui Jiang, Zeng Chen . Cross-platform binary code similarity detection based on NMT and graph embedding. Mathematical Biosciences and Engineering, 2021, 18(4): 4528-4551. doi: 10.3934/mbe.2021230 |
[6] | Yong Tian, Tian Zhang, Qingchao Zhang, Yong Li, Zhaodong Wang . Feature fusion–based preprocessing for steel plate surface defect recognition. Mathematical Biosciences and Engineering, 2020, 17(5): 5672-5685. doi: 10.3934/mbe.2020305 |
[7] | Xiaoqing Lu, Jijun Tong, Shudong Xia . Entity relationship extraction from Chinese electronic medical records based on feature augmentation and cascade binary tagging framework. Mathematical Biosciences and Engineering, 2024, 21(1): 1342-1355. doi: 10.3934/mbe.2024058 |
[8] | Hongqiang Zhu . A graph neural network-enhanced knowledge graph framework for intelligent analysis of policing cases. Mathematical Biosciences and Engineering, 2023, 20(7): 11585-11604. doi: 10.3934/mbe.2023514 |
[9] | Noor Wali Khan, Mohammed S. Alshehri, Muazzam A Khan, Sultan Almakdi, Naghmeh Moradpoor, Abdulwahab Alazeb, Safi Ullah, Naila Naz, Jawad Ahmad . A hybrid deep learning-based intrusion detection system for IoT networks. Mathematical Biosciences and Engineering, 2023, 20(8): 13491-13520. doi: 10.3934/mbe.2023602 |
[10] | Xi Lu, Zejun You, Miaomiao Sun, Jing Wu, Zhihong Zhang . Breast cancer mitotic cell detection using cascade convolutional neural network with U-Net. Mathematical Biosciences and Engineering, 2021, 18(1): 673-695. doi: 10.3934/mbe.2021036 |
Nowadays, code reuse and sharing are becoming more and more popular, bringing convenience to developers and security problems at the same time. One problem is that the code vulnerabilities can spread with the code reuse. In the Synopsys 2020 open source security and risk analysis report [1], it is found that 99% of the codebases audited in 2019 use third-party components, and 75% of the audited codebases contain at least one public vulnerability. Recently, Apache Log4j, a widely used component, has exposed a high-risk vulnerability that has been exploited by the wild. Almost all industries are affected by the vulnerability, including many well-known technology companies and e-commerce website around the world. More and more software, especially commercial software, is released without source code, so the security analysis of binary files is very necessary. But at the same time, due to redundancy elimination, instruction reordering and conversion during compiling, it is more difficult to analyze binary files. In addition, vendors of IoT (Internet of things) devices rely heavily on the third-party library, making vulnerabilities in third-party libraries spread to files compiled in different architectures. U. S. Department of Homeland Security has warned that third-party code embedded in approximately 50 million networked devices worldwide was vulnerable to infiltration by malicious hackers [2]. Vulnerabilities in IoT devices are usually critical ones, opening IoT devices to attacks such as distributed denial of service (DDoS) [3]. Binary diffing, also known as binary similarity detection, aims to discover and measure similarities between two files whose codes are not open-sourced. The binary diffing technology across versions, optimization levels and architectures, can help detect vulnerabilities in IoT devices.
At present, there are many mature tools and research papers for binary diffing. Representative tools include Diaphora [4], BinDiff [5] and TurboDiff [6]. Diaphora compares binary files based on the primary keys (including functions address, function hash, etc.). TurboDiff uses basic block checksum and the number of instructions to compare two binary files. BinDiff takes the small-primes-products property of basic blocks to solve the slightly code changes that influenced the comparison result, such as the instruction reordering and branch inversion. The above three tools use relatively strict comparsion rules that are not robust to changes by different optimization levels or architectures. With the rise of machine learning, especially deep learning, researchers begin to apply machine learning methods to binary diffing. Genius [7] and Gemini [8] are outstanding methods of applying machine learning to vectorize features of code for similarity comparison. Genius [7] embeds the ACFG (attribute control flow graph) into feature vector to represent the functions in binary file and measure the similarity by bipartite graph matching algorithm. The generation of codebook during feature embedding is expensive and runtime overhead increases with the size of the codebook. Gemini [8] applies deep neural network to embed features of binary code into matrix. Both Gemini and Genius extract statistical features which are scalable, but they do not consider semantic features. Later works extract semantic features in basic block granularity to represent the code [9,10,11,12]. However, they all make function-wide comparison, ignoring the inter-function features which are also important representing the code. Reference [13] proposes a program-wide comparison method DEEPBINDIFF in basic block granularity, but the method of generating multiple paths based on random walk of each basic block has scalability problem. DEEPBINDIFF can not handle the cross-architecture binary diffing task. In addition, the more semantic features are extracted by neural network, the more they can reflect the behavior of code. However, the neural network based comparison method brings more time overhead than the traditional one based on the rigorous features. Acceptable time overhead is another challenge of neural network-based comparison method.
In this paper, we propose a program-wide binary diffing method to make similarity comparison across versions, optimization levels and architectures. The diffing analysis is conducted in four granularities: program granularity, function granularity, basic block granularity and instruction granularity. We take the analysis from program granularity to function granularity as a top down process. While the bottom up process is implemented from instruction granularity to function granularity. We make diffing through both top down and bottom up process. The top down comparison is to make preliminary relationship selection according to the inter-function features within the program scope. We apply the modified earth mover distance (EMD) to obtain the candidate function pair for further comparison. In the bottom up process, the features of binary code is represented by neural network to obtain a more accurate comparison. Semantic features in instruction granularity are extracted based on the skip-thoughts model in natural language processing (NLP). The structural features of basic blocks in a single function are represented by graph autoencoder (GAE) model. We can embed the semantic features of functions obtained from top down process and calculate the similarity score to obtain a more accurate comparison result. The neural network model based feature representation method can generate feature vectors that are robust to code variation across versions, optimization levels and architectures. The top down process is actually a function selection process which reduces the scope of comparison and brings acceptable time overhead, while the bottom up process uses neural network to represent code feature and reaches a relatively accurate comparison result.
We have implemented a prototype PBDiff (program-wide binary diffing) and evaluated it using the widely-used standard third-party library and firmware dataset used by Genius [7]. Experimental result shows that our tool outperforms state-of-the-art BinDiff [5], Asm2vec [14] and TurboDiff [6] on the diffing tasks across versions, optimization levels and architectures. When making diffing between binary files of Busybox 1.27.2 compiled in different architectures, the average recall and precision of PBDiff is 0.842 and 0.889, which is 69.8% and 60.5% higher than that of BinDiff. The performance of PBDiff is also verified by a case study that conducts diffing between the OpenSSL and Busybox standard third-party libraries and real-world firmware files containing the libraries. In addition, PBDiff is capable to detect CVE vulnerabilities in real-world firmware files.
Contributions. The contributions of this paper are as follows:
● We propose a program-wide binary diffing method across versions, optimization levels and architectures. The diffing is divided into four granularities, and we conduct the comparison in top down process and bottom up process according to the granularity.
● In the top down process, we make comparison mainly using the inter-function features. The modified EMD is applied to obtain the candidate function pair containing functions that are likely to be similar.
● Neural network model is applied in the bottom up process to extract and embed features of functions in candidate function pair. Based on the generated feature matrix, the similarity score is calculated to obtain the corresponding relationship of functions in the two comparison files.
● We have implemented a prototype PBDiff and proved it outperformed state-of-the-art tools BinDiff, Asm2vec and TurboDiff when making diffing across versions, optimization levels and architectures. The effectiveness of PBDiff is also proved by making diffing and detecting CVE vulnerabilities in real-world firmware files.
The program-wide binary diffing is to find the corresponding similar functions in the two binary files to be compared.We divide the binary diffing into two processes: top down preliminary relationship selection and bottom up similarity comparison. The two processes are executed alternately to complete the task of diffing.
The system workflow is shown in Figure 1. The left part of Figure 1 illustrates the top down preliminary relationship selection. The preliminary relationship selection proceeds from the program granularity to function granularity. We define the similar functions confirmed in the two comparison files as anchor functions. The initial anchor function selection is based on unique characteristics. Then functions that have calling relationship with the same anchor function are grouped together to form the candidate function subset. For candidate function subsets related to anchor functions in two files, we apply the EMD to find the relaxed relationship in functions of the subset. This relaxed relationship is represented by candidate function pair whose functions are from the two comparison files. For example, functions g1 and t1 are anchor functions in two comparison files f1 and f2. The candidate function subset of g1 and t1 are presented as G={g′1,g′2,...,g′n} and T={t′1,t′2,...,t′m}. The EMD is calculated to find the candidate function pairs (g′i,t′j) from G and T. Functions g′i and t′j in the two files are more likely to be similar. The generated candidate function pairs are further compared during the bottom up process.
The right part of Figure 1 is a bottom up similarity comparison process. It takes candidate function pairs as input, calculates the similarity between functions in the function pair to find the corresponding functions in two comparison files. The comparison process conduct analysis from instruction granularity to function granularity. Features of instruction and basic block granularity are extracted and embedded to represent candidate functions. Similarity calculation is implemented based on the embedding matrices. If the functions in the candidate function pair have a relatively high similarity score, they become new anchor functions for later comparison. We can start a new iteration by conducting a new top down process based on the new anchor functions.
We mark the corresponding functions in the two comparison files as anchor functions. The top down preliminary relationship selection aims to expand the comparison scope according to anchor functions and the calling relationship between functions. The comparison scope expansion takes the anchor functions as input and selects functions that have calling relationship with anchor functions. We select candidate function pairs from these functions by EMD.
Figure 2 shows an example of the comparison expansion based on anchor function. For partial function nodes in files F and G in Figure 2, we have obtained functions a and c have corresponding relationship and marked them as anchor functions. In file F, nodes b, d and e have calling relationships with a, while nodes g, h and f in file G have calling relationships with c. During the top down relationship selection, we compare the function nodes b,d,e with f,g,h using EMD and generate candidate function pair (d, h). Function nodes d and h in the candidate function pair might have corresponding relationship.
Anchor functions are the basis of comparison scope expansion. In the top down preliminary relationship selection process, the initial anchor function selection is different from subsequent anchor function selection. The initial anchor functions are selected using the unique features of functions, while subsequent anchor function selection is based on the comparison result in the bottom up similarity comparison process.
During the initial anchor function selection, we select the unique features of functions that are not affected by different optimization levels and architectures. For functions compiled by the same source code in different architectures, their statistical features (such as instruction number and basic block number) vary greatly and can not be used as the unique features. However, the string constants referenced in functions are usually unique, and the number of functions with string constants usually accounts for more than 15% of the total function number. On the other hand, integer constant can also be used for initial anchor selection [15]. However, there are a number of integer constants in a single function. We choose the largest frequency of integer constant as another unique feature. For example, the function ssl_parse_serverhello_renegotiate_ext has the unique string constant t1_reneg.c!expected_len||s−>s3−>previous_client_finished_len, which makes this function an initial anchor function. Functions asn1_enc_save, asn1_do_lock and asn1_do_adb all have the string constant "tasn_tuil.c". However, the largest frequency of integer constants in the three functions are different. The largest constant frequency is 2 for function asn1_enc_save. While the largest constant frequency of asn1_do_lock and asn1_do_adb is 4 and 3, so we can distinguish asn1_do_lock and asn1_do_adb by combining the features of string constant and largest integer constant frequency. In addition, we add the number of function parameters as the auxiliary comparison basis. Therefore, we choose three kinds of features including string constant, largest frequency of integer constant and the number of parameters as unique features when selecting the initial anchor functions.
The generation of candidate function pair is an important step in the comparison scope expansion. The functions that have calling relationship with the same anchor functions are grouped into the same candidate function subset. Candidate function pairs are chosen from these function subsets in two comparison files.
The selection of candidate function pair is based on the modified EMD. EMD was proposed by Rubner et al. [16] to measure the distance between two probability distributions. When applying EMD to select the candidate function pair, we replace the ground distance used by EMD with cosine distance. The structural features of functions including degree and betweenness are normalized to generate the feature matrix which is used as the input of EMD calculation. The modified EMD calculation outputs a flow matrix that represents the relaxed one-to-one mapping relationship of functions in the two comparison files. Candidate function pairs are obtained according to the relaxed one-to-one mapping relationship.
In addition, the function call graph [17,18] is a directed graph representing call relationships between functions. The node in the function call graph represents the function, with edge representing the call relationship between two nodes. We take advantage of the scale-free property of the function call graph [19], which means that the functions in the call graph are not even. Most function nodes in the call graph have fewer edges with others, while a few function nodes are connected with many other ones (with larger degree). The uneven property of nodes makes the call-relationship-based comparison scope expansion cover most of functions in limited iterations.
The bottom up similarity comparison uses the neural network model to extract and compare the finer function semantic features in the candidate function pair. Feature extraction is implemented from bottom to top in three different granularities: instruction granularity, basic block granularity and function granularity. The feature extraction and similarity comparison are shown in Figure 3. In instruction granularity, the semantic features are extracted by the skip-thoughts model. In basic block granularity, we obtain the structural features based on the control flow graph, and integrate the features of each function by graph neural network. The feature matrices of different granularities are concatenated to represent the function. Similarity score is calculated by the function matrix to verify whether the two functions in the candidate function pair have a corresponding relationship.
In this paper, we aim to extract semantic feature of instructions while most researches usually take the basic block as the minimum unit of feature extraction. We propose a semantic representation method to vectorize instructions in each basic block inspired by the skip-thoughts model in NLP. The structure of paragraph in natural languages process is somewhat like the structure of binary code. Instructions in binary files can be regarded as the words in document, basic blocks as the sentences and functions as paragraph.
In order to solve the same problem as out-of-vocabulary problem in NLP, we first normalize the disassembly instruction sequence when adopting the skip-thoughts model in binary instruction embedding. The immediate value is replaced with IMM and the base address of memory is replaced with MEM. We apply the encoder component of skip-thoughts model to embed the instructions into vector. When being applied to represent the features of binary code, skip-thoughts model takes each instruction in instruction sequence as input, generating a semantic vector that can represent not only the latent semantic features among instructions but also the contexual semantic features of basic blocks. The encoder component of skip-thoughts model is composed of gated recurrent units, which takes the instructions in the basic block as minimum input unit. Just like reference [20], we encode the instruction sequence with the following formula:
zt=σ(Wz⋅[ht−1,st]) | (2.1) |
rt=σ(Wr⋅[ht−1,st]) | (2.2) |
~ht=tanh(W⋅[rt∗ht−1,st]) | (2.3) |
ht=(1−zt)∗ht−1+zt∗~ht | (2.4) |
where zt is the update gate, rt is the reset gate. t represents the tth instructions in current basic block, corresponding to the tth time of GRU. ~ht is the hidden state of the tth instruction that is passed to the next state. ht is the hidden state that depends on ht−1 and ~ht. If there are N insructions in current basic block, hN is the vector represented current basic block. In this way, the generated vector represents the semantic features of instructions of single basic block.
Unlike previous works [8,9], we only consider the lightweight structural features for efficiency when extracting basic block features. To obtain the betweenness and offspring of each basic block, we construct the CFG (control flow graph) of each function. The vertices in CFG represent the basic blocks in the function with edges reflecting the control dependency between basic blocks. The betweenness of a node is the proportion of the number of shortest paths passing through the node to the total number of shortest paths, reflecting the impact of the node on the whole graph. The betweenness of basic block nodes can reflect their importance in the whole CFG. In addition, the offspring values of basic blocks are actually the number of other nodes connected to them, which can also reflect the structural features of graphs.
The features of basic blocks reflect partial structural features in functions. We obtain the global structural features of functions using a graph neural network based representation method. Traditional deep learning methods successfully extract the features of Euclidean spatial data, assuming the data samples are independent. However, the relationship between the vertex and its neighborhood in CFG is not independent. For CFG of a single function, the relationship between vertices can be represented by Graph Neural Network (GNN). Therefore, the structural features of functions are embedded based on GAE (graph autoencoder) [21]. GAE model consists of encoder and decoder part. The encoder part contains a two-layer graph convolutional network which can embed the structural feature into matrix. The embedded matrix Z is calculated by Eqs (2.5) and (2.6):
Z=GCN(X,A) | (2.5) |
GCN(X,A)=A′Relu(A′XW0)W1 | (2.6) |
where A′=D−1/2AD−1/2 is a symmetric normalized adjacency matrix of the adjacent matrix A. The vertex feature matrix X with dimension n×d records the features of n nodes in the graph.
To calculate the similarity score of two functions, we apply the widely-used siamese architecture. Siamese network has two input fields to compare two patterns and one output whose state value corresponds to the similarity between the two patterns [22]. When the siamese network is applied to the similarity calculation of functions, the input is the two functions in the candidate function pair and output is the similarity score of the two functions. The score is used to judge whether two functions have a corresponding relationship.
To conduct similarity score calculation, the siamese network should be trained first to generate the network model that can represent the code and make similarity comparison. The siamese architecture consists of two branches that share the same hyperparameters. During training process, each branch takes the feature matrix of different granularities as input, and generate the matrix that represents the function code.
We use IM and FM to record the instruction feature matrix and structural feature matrix of functions. The dimension of IM is d1×N where N is the number of basic blocks and d1 is the instruction sequence vector dimension. FM is a d2×N dimension matrix recording the features of basic block granularity and function granularity. B and OF represent the matrix of betweenness and offspring. The combination of matrices with different granularities is shown on the right part of Figure 3. The generated matrix M of each function is calculated by Eq (2.7):
M=tanh(W1×FM+W2×tanh(Con(IM,B,OF))) | (2.7) |
where W1 and W2 are hyperparameters, W1 is a p×d2 dimensional weight matrix, W2 is a p×(d1+2) dimension weight matrix.
After obtaining the output matrix Mi and Mj of function i and j, the cosine value of Mi and Mj, denoted as ˆy, is calculated to represent the similarity of two functions. For each function pair, the actual distance y is valued with 1 or -1, representing whether two functions are similar or not. The predicted distance ˆy calculated by siamese network is between -1 and 1. The training process is to optimize the hyperparameters W1 and W2 to minimize the gap between predicted value and actual value by Eq (2.8) until the network performance is good, where yi represents the ith function in the function list of comparsion file.
minW1,W2n∑i=1(yi−^yi)2 | (2.8) |
After the training process, the network model takes feature matrices of two functions as input and calculates a similarity score. If the two functions in the candidate function subset have high similarity score, they are regarded as new anchor functions which will be used as the basis for later comparison scope expansion during the top down process.
We implemented a proof-of-concept PBDiff (program-wide binary diffing). To evaluate the performance of PBDiff, we would like to answer the following four research questions:
RQ1. Performance of diffing across versions and optimization levels. Can PBDiff perform better than state-of-the-art tools when making diffing across versions and optimization levels?
RQ2. Performance of diffing across architectures. To detect vulnerabilities in real-world firmware, we need to make diffing across architectures because the files in firmware are complied in different architectures such as MIPS. Is PBDiff effective in making diffing across architectures and detecting vulnerabilities in real-world firmware files?
RQ3. Effectiveness of top down candidate selection process. The top down process is to select functions that have calling relationship with anchor functions and divide the comparison set into much smaller subsets. How does the top down process affect the performance of PBDiff?
RQ4. Time overhead. The candidate function selection is based on EMD and finer candidate function comparison is based on neural network. Is the time overhead related to EMD calculation, training time and runtime of neural network acceptable considering efficiency?
In the process of similarity comparison based on neural network, we need the GPU-support environment and the dataset for training. Our experiment was conducted on a server equipped with a GeForce 2080 GPU card which is used to accelerate the learning process.
Dataset. We generated the dataset utilizing OpenSSL, Busybox, coreutils, diffutils and findutils like [7,8,9] to train the neural model used in the bottom up process. The functions compiled in different versions, optimization levels and architectures are stored in the dataset and selected in pairs for training. In addition, real-world firmware files [7] are leveraged to verify the capability of PBDiff in detecting the real-world vulnerabilities.
Baseline techniques. We compared PBDiff with state-of-the-art baseline techniques BinDiff [5], TurboDiff [6] and Asm2vec [14]. Considering that Asm2vec is designed for single architecture, we compared PBDiff with Asm2vec only across versions and optimization levels. When verifying the performance of PBDiff across architectures, we compared PBDiff with BinDiff and TurboDiff. In order to evaluate the effectiveness of features extracted by neural network, we implemented PBDiff-S, which conducts diffing only using the statistical features instead of the semantic ones used by neural network model.
We evaluated the effectiveness of PBDiff by diffing binary files across versions, optimization levels and architectures. PBDiff was compared with BinDiff [5], TurboDiff [6] and Asm2vec [14]. We also use case study to make diffing between real-world firmware files and standard third-party library.
Precision and recall. In the classification problem, precision is defined as the ratio between all the instances that were correctly classified in the positive class against the total number of instances classified in the positive class. Recall is defined as the ratio between all the instances that were correctly classified in the positive class against the total number of actual members of the positive class. The precision and recall metrics are used to measure the performance of different tools across versions, optimization levels and architectures.
To compare the performance of BinDiff, Asm2vec, PBDiff and PBDiff-S, we took different versions of binaries including diffutils, findutils, OpenSSL and Busybox as analysis target. From the recall and precision result shown in Table 1, PBDiff outperforms BinDiff, Asm2vec and PBDiff-S. When diffing the binaries of different versions, the performance of PBDiff is relatively stable, while BinDiff and Asm2vec perform much differently. For example, BinDiff has a recall of 0.474 in diffing diffutils v2.8 and v3.6, with recall of 0.892 in diffing v3.4 and v3.6. The corresponding recall of PBDiff is 0.91 and 0.956, improving the recall by 91.2% and 7.2%. BinDiff makes diffing mainly based on the statistical features, including the basic block number, jumping instruction (such as jmp) number and total instruction number, failing to extract the semantic features. The performance of BinDiff between v3.4 and v3.6 is better than that between v2.8 and v3.6 because there are fewer code changes. Asm2vec and PBDiff extract semantic features based on neural network models, which make the two tools perform better than BinDiff. However, Asm2vec extracts the vector of each operand and averages them to generate the vector presenting the instruction sequence in functions. PBDiff, on the other hand, generates the vector representing not only the relationship between instructions in basic block but also the contextual relationship between basic blocks. Feature vector generated by PBDiff can represent more semantic information than Asm2vec, showing that our method has better performance in cross-version diffing. PBDiff-S performs not as good as PBDiff. However, there is less gap between the performance of PBDiff-S and PBDiff. In the diffing between findutils v4.4.1 and findutils v4.6.0, the recall of PBDiff-S and PBDiff is 0.849 and 0.852. There are not so many changes in codes of different versions, which mainly update certain functional modules. As a result, in the diffing across versions, the statistical features can represent codes, making PBDiff-S perform relatively good.
Recall | Precision | ||||||||
BinDiff | Asm2vec | PBDiff-S | PBDiff | BinDiff | Asm2vec | PBDiff-S | PBDiff | ||
diffutils | v2.8-v3.6 | 0.474 | 0.683 | 0.691 | 0.725 | 0.896 | 0.892 | 0.899 | 0.91 |
v3.1-v3.6 | 0.789 | 0.792 | 0.804 | 0.823 | 0.847 | 0.88 | 0.903 | 0.926 | |
v3.4-v3.6 | 0.892 | 0.897 | 0.917 | 0.945 | 0.936 | 0.941 | 0.949 | 0.956 | |
average | 0.718 | 0.791 | 0.804 | 0.831 | 0.893 | 0.904 | 0.917 | 0.931 | |
findutils | v4.2.33-v4.6.0 | 0.756 | 0.760 | 0.763 | 0.787 | 0.864 | 0.875 | 0.887 | 0.898 |
v4.4.1-v4.6.0 | 0.827 | 0.834 | 0.849 | 0.852 | 0.899 | 0.898 | 0.916 | 0.933 | |
average | 0.67 | 0.738 | 0.753 | 0.822 | 0.804 | 0.827 | 0.902 | 0.916 | |
Busybox | 1.20.0-1.30.0 | 0.625 | 0.671 | 0.665 | 0.761 | 0.766 | 0.799 | 0.827 | 0.864 |
1.20.0-1.27.2 | 0.714 | 0.804 | 0.840 | 0.883 | 0.842 | 0.856 | 0.855 | 0.878 | |
average | 0.67 | 0.738 | 0.753 | 0.822 | 0.804 | 0.827 | 0.841 | 0.871 | |
OpenSSL | 0.9.8a-1.0.2 | 0.315 | 0.492 | 0.585 | 0.604 | 0.524 | 0.623 | 0.675 | 0.682 |
1.0.1f-1.0.2 | 0.526 | 0.623 | 0.704 | 0.727 | 0.753 | 0.806 | 0.818 | 0.853 | |
average | 0.421 | 0.558 | 0.645 | 0.666 | 0.639 | 0.715 | 0.747 | 0.768 |
To measure the effectiveness of diffing across optimization levels, we use the same comparison tools and analysis files when making diffing across versions. Files are compiled in optimization levels O0, O1, O2 and O3. Table 2 shows the precision and recall of the diffing across optimization levels. For the same source files compiled in different optimization levels, there are not as many differences as the ones compiled in different versions. Consequently, there is less gap among the performance of BinDiff, Asm2vec and PBDiff. Still, PBDiff is better than the other two tools. However, the diffing result between files compiled in O2 and O3 is better than the diffing between O1 and O3. The recall of findutils v4.41 between O1 and O3 is 0.732 by BinDiff, while the recall between O2 and O3 is 0.819. For Asm2vec, its recall of findutils v4.41 between O1 and O3 is 0.834, and the corresponding recall between O2 and O3 is 0.879. This is mainly due to the optimization mechanism. Optimization level O1 is mainly related to the optimization of code branches, constants and expressions. Level O2 optimizes register and instruction levels and performs almost all optimizations without time and space trade-offs, such as rearrangement of basic blocks and instructions, which brings more changes to the code. This explains why the difference between O1 and O2 is greater than that between O2 and O3.
Recall | Precision | ||||||||
BinDiff | Asm2vec | PBDiff-S | PBDiff | BinDiff | Asm2vec | PBDiff-S | PBDiff | ||
diffutils | v2.8 O0 - O3 | 0.725 | 0.835 | 0.851 | 0.898 | 0.801 | 0.825 | 0.845 | 0.89 |
v2.8 O1 - O3 | 0.792 | 0.859 | 0.868 | 0.914 | 0.825 | 0.898 | 0.862 | 0.919 | |
v2.8 O2 - O3 | 0.833 | 0.88 | 0.902 | 0.935 | 0.848 | 0.912 | 0.892 | 0.926 | |
v3.1 O0 - O3 | 0.755 | 0.817 | 0.863 | 0.914 | 0.814 | 0.867 | 0.855 | 0.917 | |
v3.1 O1 - O3 | 0.782 | 0.829 | 0.875 | 0.922 | 0.833 | 0.892 | 0.872 | 0.936 | |
v3.1 O2 - O3 | 0.818 | 0.876 | 0.923 | 0.945 | 0.856 | 0.924 | 0.903 | 0.948 | |
v3.4 O0 - O3 | 0.744 | 0.832 | 0.899 | 0.923 | 0.82 | 0.872 | 0.891 | 0.905 | |
v3.4 O1 - O3 | 0.783 | 0.847 | 0.925 | 0.947 | 0.845 | 0.906 | 0.926 | 0.938 | |
v3.4 O2 - O3 | 0.805 | 0.902 | 0.938 | 0.969 | 0.868 | 0.933 | 0.947 | 0.967 | |
v3.6 O0 - O3 | 0.733 | 0.816 | 0.884 | 0.914 | 0.847 | 0.89 | 0.882 | 0.922 | |
v3.6 O1 - O3 | 0.768 | 0.825 | 0.899 | 0.925 | 0.875 | 0.925 | 0.942 | 0.947 | |
v3.6 O2 - O3 | 0.825 | 0.857 | 0.923 | 0.947 | 0.902 | 0.94 | 0.966 | 0.982 | |
Average | 0.78 | 0.848 | 0.896 | 0.929 | 0.845 | 0.899 | 0.899 | 0.933 | |
findutils | v4.233 O0 - O3 | 0.755 | 0.811 | 0.845 | 0.901 | 0.806 | 0.831 | 0.902 | 0.925 |
v4.233 O1 - O3 | 0.771 | 0.826 | 0.877 | 0.926 | 0.822 | 0.886 | 0.933 | 0.939 | |
v4.233 O2 - O3 | 0.812 | 0.834 | 0.892 | 0.938 | 0.837 | 0.893 | 0.947 | 0.954 | |
v4.41 O0 - O3 | 0.707 | 0.822 | 0.832 | 0.852 | 0.815 | 0.856 | 0.893 | 0.933 | |
v4.41 O1 - O3 | 0.732 | 0.834 | 0.849 | 0.893 | 0.835 | 0.874 | 0.925 | 0.94 | |
v4.41 O2 - O3 | 0.819 | 0.879 | 0.892 | 0.925 | 0.824 | 0.88 | 0.937 | 0.956 | |
v4.6 O0 - O3 | 0.714 | 0.81 | 0.844 | 0.901 | 0.829 | 0.845 | 0.872 | 0.882 | |
v4.6 O1 - O3 | 0.725 | 0.822 | 0.87 | 0.923 | 0.875 | 0.898 | 0.896 | 0.915 | |
v4.6 O2 - O3 | 0.799 | 0.859 | 0.885 | 0.937 | 0.916 | 0.922 | 0.903 | 0.933 | |
Average | 0.759 | 0.833 | 0.865 | 0.911 | 0.84 | 0.876 | 0.912 | 0.931 | |
Busybox | 1.20.0 O0-O3 | 0.823 | 0.838 | 0.902 | 0.933 | 0.878 | 0.864 | 0.89 | 0.915 |
1.20.0 O1-O3 | 0.856 | 0.856 | 0.915 | 0.946 | 0.899 | 0.9 | 0.926 | 0.937 | |
1.20.0 O2-O3 | 0.887 | 0.892 | 0.923 | 0.959 | 0.913 | 0.94 | 0.946 | 0.969 | |
1.30.0 O0-O3 | 0.801 | 0.823 | 0.834 | 0.856 | 0.822 | 0.835 | 0.903 | 0.915 | |
1.30.0 O1-O3 | 0.827 | 0.845 | 0.846 | 0.868 | 0.833 | 0.856 | 0.915 | 0.932 | |
1.30.0 O2-O3 | 0.845 | 0.872 | 0.872 | 0.893 | 0.849 | 0.866 | 0.922 | 0.946 | |
average | 0.840 | 0.854 | 0.882 | 0.909 | 0.866 | 0.877 | 0.917 | 0.936 | |
Openssl | 1.0.1f O0-O3 | 0.724 | 0.815 | 0.823 | 0.89 | 0.816 | 0.832 | 0.876 | 0.902 |
1.0.1f O1-O3 | 0.776 | 0.833 | 0.847 | 0.922 | 0.832 | 0.854 | 0.892 | 0.929 | |
1.0.1f O2-O3 | 0.789 | 0.852 | 0.88 | 0.946 | 0.849 | 0.866 | 0.923 | 0.954 | |
average | 0.763 | 0.833 | 0.85 | 0.919 | 0.832 | 0.851 | 0.897 | 0.928 |
On the whole, PBDiff performs better than PBDiff-S, and their performance gap is bigger than that in the comparison across versions. For example, the precision of PBDiff and PBDiff-S during the comparison between diffutils v2.8 in O1 level and O3 level is 0.862 and 0.919, while the precision of comparison between diffutils v2.8 and v3.6 is 0.899 and 0.91. For PBDiff and PBDiff-S, their performance is better in the comparison across versions than that across optimization levels. When making modifications in newer version, code changes focuse in limited number of functions. However, code changes occur in more functions when using different optimization levels, which affects the performance of diffing across optimization levels.
In cross-architecture diffing, since Asm2vec is only applicable in single architecture, we compare PBDiff with BinDiff and TurboDiff [6] instead of Asm2vec. TurboDiff is an IDA plugin used to discover and analyze differences between the functions of two binaries. BinDiff is also applicable for the cross-architecture diffing. We compared the performance of BinDiff, TurboDiff, PBDiff-S and PBDiff by recall and precision, using binary files of Busybox 1.27.2 and OpenSSL 1.0.1f compiled in MIPS, ARM, X86 and X64 architecture, and the comparison result is shown in Table 3. For TurboDiff, the recall and precision is relatively low. For example, the recall is 0.104 for comparison between Busybox binaries compiled in MIPS and ARM architectures, and 0.125 between MIPS and X86 architecture. TurboDiff uses the basic block checksum and instruction number in basic block as the comparison basis. However, this comparison basis is relatively strict and may be affected by different architectures. BinDiff performs better than TurboDiff because it uses the statistical features such as number of instructions as the comparison basis which is not as strict as that of TurboDiff. PBDiff-S and PBDiff have a stable performance when making diffing across architectures. For the comparison among binaries compiled by Busybox 1.27.2 in different architectures, the average recall and precision of PBDiff is 0.842 and 0.889, which is 69.8% and 60.5% higher than that of BinDiff, and PBDiff has the recall and precision 4.0% and 6.7% higher than PBDiff-S. For comparison tools BinDiff and TurboDiff, the comparison basis such as basic block checksum or instruction number is not robust to the changes made by different architectures, making their performance not as good as comparison between binaries across versions or optimization levels. PBDiff and PBDiff-S, on the other side, extract semantic features instead of statistical features which can present the code more than BinDiff and TurboDiff across architectures, making PBDiff and PBDiff-S obtain a more precise comparison result.
Recall | Precision | ||||||||
BinDiff | TurboDiff | PBDiff-S | PBDiff | BinDiff | TurboDiff | PBDiff-S | PBDiff | ||
Busybox 1.27.2 | MIPS-ARM | 0.572 | 0.104 | 0.761 | 0.794 | 0.623 | 0.125 | 0.722 | 0.824 |
MIPS-x86 | 0.472 | 0.125 | 0.703 | 0.745 | 0.526 | 0.205 | 0.769 | 0.869 | |
MIPS-x64 | 0.566 | – | 0.825 | 0.862 | 0.614 | – | 0.846 | 0.896 | |
ARM-x86 | 0.416 | 0.204 | 0.795 | 0.83 | 0.554 | 0.311 | 0.825 | 0.877 | |
ARM-x64 | 0.325 | – | 0.886 | 0.909 | 0.474 | – | 0.915 | 0.927 | |
X86-x64 | 0.622 | – | 0.892 | 0.915 | 0.533 | – | 0.923 | 0.942 | |
Average | 0.496 | 0.144 | 0.810 | 0.842 | 0.554 | 0.214 | 0.833 | 0.889 | |
OpenSSL 1.0.1f | MIPS-ARM | 0.474 | 0.205 | 0.788 | 0.825 | 0.358 | 0.304 | 0.826 | 0.921 |
MIPS-x86 | 0.429 | 0.196 | 0.725 | 0.885 | 0.469 | 0.101 | 0.816 | 0.916 | |
MIPS-x64 | 0.566 | – | 0.749 | 0.904 | 0.624 | – | 0.838 | 0.925 | |
ARM-x86 | 0.462 | 0.254 | 0.828 | 0.915 | 0.392 | 0.158 | 0.845 | 0.937 | |
ARM-x64 | 0.38 | – | 0.816 | 0.923 | 0.454 | – | 0.825 | 0.906 | |
X86-x64 | 0.625 | – | 0.912 | 0.927 | 0.633 | – | 0.931 | 0.944 | |
Average | 0.489 | 0.218 | 0.803 | 0.90 | 0.488 | 0.188 | 0.847 | 0.925 |
In the previous subsection, we made diffing between binary files compiled by standard library code. To apply our binary diffing technology to the vulnerability detection across architectures, especially taking the IoT (internet of things) devices as detection target, we verified the performance of PBDiff when diffing between the standard third-party library and the real-world firmware files. Third-party libraries, such as OpenSSL and Busybox, are widely used in the firmware files. However, firmware vendors usually delete the unnecessary third-party code due to the limitation of memory, making the diffing harder than the cross-architecture diffing discussed above.
We took the standard third-party library OpenSSL and Busybox and real-world firmware files used by Genius [7] as comparison target. Third-party library OpenSSL and Busybox are widely used in firmware files such as router and camera, and the firmware list of Genius contains the OpenSSL or Busybox library.
The detection rate is used as the metric to verify the effectiveness of diffing. For the two comparison files with m and n functions, the detection rate is defined as the ratio of the detected similar function number to the smaller value in m and n. To make diffing between two files, the top down process and the bottom up process are executed alternately, and we took the one execution of the top down and bottom up process as an execution round. We selected the firmware DIR−655_REVC_FIRMWARE_3.00B10 and R4500_V1.0.0.4_1.0.3 containing OpenSSL library to compare them with OpenSSL 1.0.1f library and firmware AirCam.gen1.v3.0.9.25 and DIR−808L_FIRMWARE_1.03B05 containing Busybox library to compare with Busybox 1.27.2 standard library. We conducted five comparison rounds with the initial anchor selection as the first round. However, anchor function based method uses calling relationship to expand the comparison scope. This makes functions that have no calling relationship with others excluded from the scope expansion. We call such kind of functions orphan functions and group them to one subset, using neural network model to compare the orphan function set in two files. We added the number of matched orphan nodes after the five rounds, naming the process as neural comparison in Figure 4.
Figure 4 records the detection rate in each round of the four firmware files DIR655_REVC_FIRMWARE_3.00B10, R4500_V1.0.0.4_1.0.3, AirCam.gen1.v3.0.9.25 and DIR−808L_FIRMWARE_1.03B05 when comparing with standard libraries compiled in MIPS, ARM, X86 and X64 architectures. It can be observed that Figure 4 has four characteristics. Firstly, the detection rate of the first round is the same, this is because the initial anchor selection strategy is not influenced by architectures.
Secondly, in the first five rounds, the increment of detection rate gradually decreases. In the first round, the anchor function is chosen and added to the empty anchor function set and there are no duplicates. However, during the second to the fifth round, the number of new anchor functions increased more and more slowly considering the duplicate functions. The other reason of the decrease in the number of new anchor functions is that although our method can detect more function relationship, there will still be wrongly judged anchor functions. The results of these errors will affect the corresponding detection results in the subsequent round. The third characteristic is that the neural comparison of orphan function nodes can find more similar functions. The orphan functions account for more than 10% of the total functions. For example, the percentage of orphan nodes in R4500_V1.0.0.4_1.0.3 is 17.2%. The last characteristic is that among the detection rate curves representing different architectures, one is better than the others, while the other three curves are close to each other. It was found that the curve with the best performance has the same architecture as that of the target firmware file. For example, in Figure 4 (a), the detection rate curve of function diffing for DIR655_REVC_FIRMWARE_3.00B10 and OpenSSL in MIPS architecture is the best. DIR655_REVC_FIRMWARE_3.00B10 file is also compiled in MIPS architecture.
Function name recovery. In addition to the overall performance of the program-wide diffing, we also explored the corresponding relationship of functions, which is also very practical in binary analysis. Information of functions in real-world firmware files, such as the function name, are usually stripped due to the compilation optimization. For example, the format of partial function names in AirCam.gen1.v3.0.9.25 firmware file is sub_XXX. We matched the functions in real-world firmware with the ones in standard third-party library file to get corresponding relationship and recovered the name of functions in firmware files. In the first round of diffing between Busybox in AirCam.gen1.v3.0.9.25 and the standard Busybox library, the function sub_14840 can be mapped to function telnet_main by signature and used as anchor function. Then after the bottom up similarity comparison, function sub_14650 and con_escape are marked as new anchor functions, and we can recover the name of function sub_14650 with con_escape. In the next iteration, these two functions are added to the anchor list, and we obtained another function pair sub_145CC and setConMode which have calling relationship with function con_escape.
Vulnerability detection in real-word firmware. Furthermore, we collected the CVE vulnerable functions and detected whether these vulnerabilities could affect real-world firmware. Among the four CVE vulnerabilities of Busybox (CVE-2015-9261, CVE-2017-15873, CVE-2017-16544 and CVE-2018-20679), there is a vulnerability CVE-2018-20679 in the AirCam firmware file. The related function in AirCam is sub_1FEFC, corresponding to the vulnerability udhcp_get_option. We also analyzed the OpenSSL related vulnerabilities CVE-2015-1791 and CVE-2015-0204 in 93 real-world firmware files and found that 22 files were affected by CVE-2015-1791 and 71 files were affected by CVE-2015-0204, indicating that our method can also help to find the vulnerability function. The affected firmware list is recorded in the appendix section.
To discuss the effectiveness of the top down process, we mark PBDiff without top down process as PBDiff-TD. PBDiff-TD is actually a pair-wise comparison method. For two comparison files f1 and f2, PBDiff-TD takes two functions from f1 and f2 as input, calculating the similarity score of the two functions to judge if they have corresponding relationship. In detail, one function in file f1 is compared with all the functions in the other file f2 to verify whether the corresponding function in file f2 has the highest similarity score. If the corresponding function in file f2 has the highest similarity score, the function pair is considered to be correctly matched. However, the corresponding function does not always have the highest score. We made experiments on PBDiff-TD across architectures, choosing commonly used cjpeg v9a, wget v1.19, curl v7.53.1 and OpenSSL v1.1.0 binaries compiled in X86/MIPS/ARM/X64 architectures. Then, we took certain function in binary of X86 architecture and compared it with all the functions in binaries compiled in MIPS/ARM/X64 architecture using the trained neural network model to verify whether the corresponding functions in different architectures have the highest similarity score. For example, we took function resp_new in binary of wget v1.19 compiled in X86 and compared it with all the functions in binaries of wget v1.19 compiled in MIPS/ARM/X64 architecture. The same function resp_new in MIPS, ARM and X64 architecture has 1st, 15th and 7th ranking score. The similarity score ranking of other functions in cjpeg, wget, curl and OpenSSL binaries is shown in Table 4.
Comparison function | ranking (MIPS) | ranking (ARM) | ranking (X64) | |
cjpeg9a | alloc_sarray | 3 | 1 | 16 |
cjpeg9a | get_text_gray_row | 2 | 2 | 49 |
wget1.19 | resp_new | 1 | 15 | 7 |
wget1.19 | url_parse | 2 | 16 | 4 |
curl-7.53.1 | ourWriteOut | 1 | 1 | 1 |
OpenSSL1.1.0 | ssl3_read_bytes | 1 | 1 | 1 |
Besides, we made experiment by comparing single function in standard third-party library with functions in real-world firmware files. We compared function ssl3_read_bytes in standard third-party library OpenSSL with all the functions in file DAP−2330_REVA_FIRMWARE_1.01RC014, and obtained the similarity score between function ssl3_read_bytes and all the functions in DAP−2330_REVA_FIRMWARE_1.01RC014. However, the corresponding ssl3_read_bytes function in firmware file does not have the highest similarity score, instead, it ranks 2nd. When making comparison between the function ssl3_read_bytes in standard library and firmware tomato−Cisco_M10v2−NVRAM32K−1.28.RT−N5x−MIPSR2−109−Mini, the corresponding ssl3_read_bytes function in tomato−Cisco_M10v2−NVRAM32K−1.28.RT−N5x−MIPSR2−109−Mini ranks 37th.
From Table 4 and the comparison result of the single function detection in real-world firmware, it can be concluded that using PBDiff-TD, the corresponding functions do not always rank first, which will affect the final recall and precision value. The ranking is not always the first mainly because there are a large number of functions in the entire comparison file and the limitation of only applying static-feature-based method, which makes multiple functions close in the vector mapping space. We use a simple example of close distance of several functions in vector mapping space in Figure 5 to illustrate the necessity and effectiveness of top down process in dividing the entire comparison set into small subsets. For two files f1 and f2 to be compared, the black nodes in vector space of Figure 5 represent functions that belong to file f1 while the red nodes represent functions belong to file f2. In Figure 5, function a and function b belong to the same file, while function a and function c belong to two comparison files and have corresponding relationships. Although a and c are close to each other, there is another function b that has a shorter distance with c than that between a and c. If the comparison is made in the entire comparison set, function b and c may be misjudged as similar functions. However, if we divide function a and c into a much smaller subset A and B, function a and c will be regarded as similar.
If we use the pair-wise method only, the recall and precision of the entire function set comparison could not be satisfying. But from the score ranking result in Table 4, the corresponding function usually have a high top-N ranking even if it cannot rank 1st, indicating that the pair-wise comparison based on neural network can still select some candidate functions that may be similar to certain function. Besides, we chose function ssl3_read_bytes in standard third-party library and compared it with all functions in real-world firmware files. Of the total 90 firmware files, there are 75 files having the corresponding function ssl3_read_bytes rank in top-10. The divided subsets usually have a much smaller number of functions, because each subset only contains functions that have calling relationship with the same anchor function. Therefore, if the pair-wise method is applied to a subset of functions, the possibility of grouping functions with close distance into the same subset is much lower, resulting in a more accurate comparison result in the experiments of previous sections.
We discussed the time overhead of both top down preliminary relation selection and bottom up similarity comparison to verify the availability of our method. The time cost of top down process is mainly related to the feature extraction and EMD calculation. However, the time cost of feature extraction is negligible compared with that of EMD calculation, because the features extracted in the top down process are simple, so only the time overhead of EMD calculation is discussed. In the bottom up similarity comparison process, the neural network is firstly trained and then used to make comparison and find new anchor functions. In the bottom up process, we focused on training time and comparison runtime.
The EMD time overhead is related to the size of candidate function subset to be compared in two files. To illustrate the relationship between time cost and the number of functions in the candidate function subset, we took the comparison between the OpenSSL binary files compiled in ARM and MIPS architectures as an example. The relationship between the time cost and the size of the two candidate function subset generated by anchor functions is shown in Figure 6 (a). It can be seen that for the function subset with functions less than 20, the time cost is less than 1 second. When comparing a candidate function subset with 20 to 40 functions, the time cost is less than 10 seconds. However, the time increases with the size of the candidate set, and the time cost exceeds 100 seconds for the candidate set with more than 60 functions. Actually, the size of candidate subset is usually small. To verify this, we studied the distribution of function number in actual candidate function subset. The candidate function subset contains the functions called by anchor function, so the size of candidate function subset is actually equal to the outdegree value of the anchor function. We compiled OpenSSL in ARM architecture and analyzed the outdegree distribution of its functions. It can be seen from Figure 6 (b) that although there are functions with outdegree more than 60, the number of such functions is small. Out of the 4,919 functions, 7 functions have outdegree values greater than 60. The outdegree values of 4,581 functions are less than 10, accounting for 93.13% of the total number. Candidate function subset is much smaller than the entire set of functions. Therefore, the larger candidate function subset has little impact on the total overhead of EMD calculation.
The training time based on siamese network during bottom up process is discussed. We set the training iteration to 500 and recorded the time cost of each iteration. Figure 7 shows the time cost of each iteration in 500 iterations. The average training cost is less than 2 seconds in each iteration. However, the training process only needs to be executed once. Even if the neural network is trained for 5000 iterations, the time cost is no more than 3 hours, which ensures the efficiency of the training process.
After the training of neural network, the two functions in candidate function pair are used as inputs to calculate the similarity score. We recorded the comparison time cost of each function pair in Figure 8. The x-axis represents the number of functions in the candidate function subset that related to comparison of candidate subset, and the y-axis records the time cost for each comparison. The average time cost of each comparison in Figure 8 is about 0.05 seconds. The runtime overhead is not greatly influenced by the function size, for the size of the function matrix that used as input of neural network model is fixed. There are also periodic peaks in Figure 8. This is mainly because the comparison is divided into several rounds and some preprocessing must be made in each round, resulting in a relatively large time overhead.
To make diffing between two files, earlier researches implement the semantic equivalence of code by graph comparison [23], symbolic execution and theorem proving [24,25]. However, graph isomorphism is a NP-complete problem, leading to a low efficiency, and the method based on theorem proving is not scalable. Later work take the execution tracelets [26,27], code signature [28] to measure the similarity of functions in binary files, but they either have low coverage or are not robust to the changes brought by compiler optimization.
Other machine learning methods are applied to binary diffing across optimization levels and architectures. Graph embedding [29,30,31] and graph neural network [32,33,34] models can be applied to the binary function diffing. Vulseeker [9] generates labeled semantic flow graph (LSFG) to represent code feature. Redmond et al. [10] convert the binary code to intermediate language and record the input/output as signature for comparison across architectures. Zhang et al. [35] and Wang et al. [36] focus on the change between patched and unpatched code and make similarity comparison in code snippet level. Duan et al. [13] combine the NLP and TADW (Text-associated DeepWalk algorithm) [37] to obtain the semantic cross-function dependency feature. However, the random walk in CFG within the scope of the program is time-consuming. Bai et al. [12] propose a graph similarity comparison method directly based on node embedding instead of the widely used graph-level embedding. Ding et al. [14] propose a vector representation method by learning the latent semantic information without preliminary knowledge of assembly code in X86 architecture. Some researchers believe that the execution path contains semantic information and apply it to the binary diffing [38,39,40,41]. However, the execution path based methods face the code coverage problem. Yu et al. [11] adopt the convolutional neural network to extract the order information as well as semantic information. They also make source-code to binary diffing combining Deep Pyramid Convolutional Neural Network (DPCNN) with GNN [15]. There are also local-preferenced methods to make binary function diffing. Kam1n0 [42] combines the subgraph matching and adaptive LSH to detect the code clone. Li et al. [43] propose a topology-aware hashing method to make CFG similarity analysis by extracting graph signature as the comparison basis.
This paper presents a program-wide binary diffing method which can conduct comparison between binary files compiled in different versions, optimization levels and architectures. In order to improve efficiency, we do not directly use neural network to embed all functions, but only the functions of candidate function pairs in the top down preliminary relationship selection process. In the bottom up analysis procedure, features of function in candidate function pairs are extracted from instruction granularity, basic block granularity and function granularity. We apply the skip-thoughts model and GAE model to extract the semantic features of instruction and structural features of functions, and use siamese network to calculate the similarity between functions to find more corresponding functions. This method can take advantage of neural network in representing codes without reducing the efficiency too much by the top down preliminary relationship selection. Experiments on efficiency and effectiveness show that the combination of top down and bottom up analysis methods can achieve better performance than the state-of-the-art tools under different versions, optimization levels and architectures. In particular, a case study is discussed by making diffing between real-world firmware files and standard third-party library. In addition, PBDiff can help find the vulnerabilities in real-world firmware files.
We thank the anonymous reviewers for the helpful comments. This work is supported by the National Key Research and Development Program of China (No. 2017YFB0802900).
The authors declare no conflict of interest.
tomato-E1500USB-NVRAM64K-1.28.RT-N5x-MIPSR2-093-BT-VPN
DIR-640L_FIRMWARE_1.02B02
DCS-1130_REVA_FIRMWARE_1.08.8707
tomato-E1500-NVRAM64K-1.28.RT-N5x-MIPSR2-105-PL-Max
AirCam.v1.2.17961.130609.0103
R7000_V1.0.1.22-1.0.15
DIR-885L-R_REVA_FIRMWARE_1.00.B20
tomato-E900USB-NVRAM64K-1.28.RT-N5x-MIPSR2-093-BT-VPN
R6250-V1.0.0.62_1.0.62
DGN1000v3-V1.0.0.4_0.0.4
DIR-505_FIRMWARE_1.01
tomato-Cisco-M10v2-NVRAM32K-1.28.RT-N5x-MIPSR2-110-PL-Mini
tomato-W1800R_RT-AC6x–120-AIO-64K
tomato-Cisco-M10v2-NVRAM32K-1.28.RT-N5x-MIPSR2-109-Mini
DIR-880L_REVA_FIRMWARE_1.05.B02_WW
tomato-E2000-NVRAM60K-1.28.RT-MIPSR2-107-Max
AC1450-V1.0.0.8_1.0.4
tomato-E1200v1-NVRAM64K-1.28.RT-N5x-MIPSR2-109-Mini
NWA1100-N_1.00UJG.0
tomato-E900-NVRAM64K-1.28.RT-N5x-MIPSR2-110-Max
DIR-506L_FIRMWARE_2.13
DCS-1100_FIRMWARE_1.06_US_8330
tomato-E1500USB-NVRAM64K-1.28.RT-N5x-MIPSR2-093-BT-VPN
DGN3500-V1.1.00.36_1.00.36NA
DIR-640L_FIRMWARE_1.02B02
dd-wrt.v24-21676_NEWD-2_K3.x_mega-R6300
DWL-3150_FIRMWARE_1.00
DIR-825_REVB_FIRMWARE_2.02
DCS-1130_REVA_FIRMWARE_1.08.8707
DIR-817LW_REVA_FIRMWARE_1.00B05
DAP-2660_REVA_FIRMWARE_1.00
DIR-826L_FIRMWARE_1.00
tomato-E1500-NVRAM64K-1.28.RT-N5x-MIPSR2-105-PL-Max
DAP-1650_REVA_FIRMWARE_1.02B02
DIR-636L_FIRMWARE_1.00
DIR-120_REVA_FIRMWARE_1.05.B01_RU
DAP-2690_FIRMWARE_1.02
AirCam.v1.2.17961.130609.0103
DAP-3525_REVA_FIRMWARE_1.01
DIR-835_FIRMWARE_1.03
DIR-657_BETA_FIRMWARE_1.02B07
DIR-665_FIRMWARE_1.00
DIR-855L_FIRMWARE_1.01
DAP-2360_REVB_FIRMWARE_2.00
R7000_V1.0.1.22-1.0.15
DIR-885L-R_REVA_FIRMWARE_1.00.B20
tomato-E900USB-NVRAM64K-1.28.RT-N5x-MIPSR2-093-BT-VPN
DIR-655_REVC_FIRMWARE_3.00B10
DAP-1533_FIRMWARE_1.01B
DCH-M225_REVA_FIRMWARE_1.00B27
dgnd3700v2-v1.1.00.12_1.00.12NA
V2.7.25_V1.7orV1.8
R6250-V1.0.0.62_1.0.62
DGN1000v3-V1.0.0.4_0.0.4
DIR-836L_FIRMWARE_1.04
DIR-505_FIRMWARE_1.01
tomato-Cisco-M10v2-NVRAM32K-1.28.RT-N5x-MIPSR2-110-PL-Mini
DIR-850L_FIRMWARE_1.03
tomato-W1800R_RT-AC6x–120-AIO-64K
DAP-2310_FIRMWARE_1.10
DHP-1565_FIRMWARE_1.01
tomato-Cisco-M10v2-NVRAM32K-1.28.RT-N5x-MIPSR2-109-Mini
Hub_swi_nhd1w_1.0.95.0
DAP-2553_FIRMWARE_1.01
DIR-880L_REVA_FIRMWARE_1.05.B02_WW
DAP-2590_FIRMWARE_1.10
DAP-3690_FIRMWARE_1.00
tomato-E2000-NVRAM60K-1.28.RT-MIPSR2-107-Max
AC1450-V1.0.0.8_1.0.4
tomato-E1200v1-NVRAM64K-1.28.RT-N5x-MIPSR2-109-Mini
DIR-626L_FIRMWARE_1.02
DAP-1350_FIRMWARE_1.11
DIR-865L_FIRMWARE_1.02
NWA1100-N_1.00UJG.0
DIR-810L_REVA_FIRMWARE_1.01B04
tomato-E900-NVRAM64K-1.28.RT-N5x-MIPSR2-110-Max
DAP-2555_FIRMWARE_1.00
DIR-808L_FIRMWARE_1.03B01
DCS-6111_FIRMWARE_1.01
DAP-3520_FIRMWARE_1.00
DIR-820LW_REVB_FIRMWARE_PATCH_2.03.B01_TC
DIR-506L_FIRMWARE_2.13
DCS-1100_FIRMWARE_1.06_US_8330
DIR-860L_REVA_FIRMWARE_1.08B02
DWL-8500AP_FIRMWARE_2.20.4
DAP-2565_FIRMWARE_1.00
IPC_V1.4_V1.7.25
DIR-818L_REVA_FIRMWARE_PATCH_1.05.B01_EN
EX6100_V1.0.0.22_1.0.51
DAP-2330_REVA_FIRMWARE_1.01RC014
DIR-816L_REVB_FIRMWARE_2.01B03_WW
DAP-2695_FIRMWARE_1.00RC015
DAP-1522_FIRMWARE_1.01
[1] | W.M.P. van der Aalst, Process mining: Data science in action, Second Edition, Springer International Publishing, (2016). https://doi.org/10.1007/978-3-662-49851-4 |
[2] |
E. Rojas, J. Munoz-Gama, M. Sepúlveda, D. Capurro, Process mining in healthcare: A literature review, J. Biomed. Inform., 61 (2016), 224–236. https://doi.org/10.1016/j.jbi.2016.04.007 doi: 10.1016/j.jbi.2016.04.007
![]() |
[3] |
M. Ghasemi, D. Amyot, Process mining in healthcare: A systematised literature review, Int. J. Electron. Healthcare, 9 (2016), 60–88. https://doi.org/10.1504/IJEH.2016.078745 doi: 10.1504/IJEH.2016.078745
![]() |
[4] |
T.G. Erdogan, A.K. Tarhan, Systematic mapping of process mining studies in healthcare, IEEE Access, 6 (2018), 24543–24567. https://doi.org/10.1109/ACCESS.2018.2831244 doi: 10.1109/ACCESS.2018.2831244
![]() |
[5] | C. Fernandez-Llatas, Interactive process mining in healthcare, Springer International Publishing, (2020). https://doi.org/10.1007/978-3-030-53993-1 |
[6] |
A. Guzzo, A. Rullo, E. Vocaturo, Process mining applications in the healthcare domain: A comprehensive review, WIREs Data Mining Knowledge Discovery, 12 (2022), e1442. https://doi.org/10.1002/widm.1442 doi: 10.1002/widm.1442
![]() |
[7] |
E. De Roock, N. Martin, Process mining in healthcare - An updated perspective on the state of the art, J. Biomed. Inform., 127 (2022), 103995. https://doi.org/10.1016/j.jbi.2022.103995 doi: 10.1016/j.jbi.2022.103995
![]() |
[8] | W. M. P. van der Aalst, A. Adriansyah, A. K. A. De Medeiros, F. Arcieri, T. Baier, T. Blickle, et al., Process mining manifesto, in Business Process Management Workshops: BPM 2011 International Workshops, Clermont-Ferrand, France, August 29, 2011, Revised Selected Papers, Part I (eds. F. Daniel, K. Barkaoui, S. Dustdar), 9 (2012), 169–194. https://doi.org/10.1007/978-3-642-28108-2_19 |
[9] | R. S. Mans, W. M. P. van der Aalst, R. J. B. Vanwersch, Process mining in healthcare: Evaluating and exploiting operational healthcare processes, Springer International Publishing, (2015). https://doi.org/10.1007/978-3-319-16071-9 |
[10] |
F. Daniel, Q. Z. Sheng, H. Motahari, Business process management workshops - BPM 2018 international workshops, Sydney, NSW, Australia, September 9-14, 2018, revised papers, Lecture Notes in Business Information Processing, 342 (2019). https://doi.org/10.1007/978-3-030-11641-5 doi: 10.1007/978-3-030-11641-5
![]() |
[11] |
J. Munoz-Gama, N. Martin, C. Fernandez-Llatas, O. Johnson, M. Sepúlveda, Innovative informatics methods for process mining in health care, J. Biomed. Inform., 134 (2022), 104203. https://doi.org/10.1016/j.jbi.2022.104203 doi: 10.1016/j.jbi.2022.104203
![]() |
[12] |
J. Munoz-Gama, N. Martin, C. Fernandez-Llatas, O. A. Johnson, M. Sepúlveda, E. Helm, et al., Process mining for healthcare: Characteristics and challenges, J. Biomed. Inform., 127 (2022), 103994. https://doi.org/10.1016/j.jbi.2022.103994 doi: 10.1016/j.jbi.2022.103994
![]() |
[13] | N. Martin, N. Wittig, J. Munoz-Gama, Using Process Mining in Healthcare, in Process Mining Handbook (eds. W. M. P. van der Aalst, J. Carmona), Lecture Notes in Business Information Processing, 448 (2022), 416–444, Springer. https://doi.org/10.1007/978-3-031-08848-3_14 |
[14] |
M. Jiang, B. Zhou, L. Chen, Identification of drug side effects with a path-based method, Math. Biosci. Eng., 19 (2022), 5754–5771. https://doi.org/10.3934/mbe.2022269 doi: 10.3934/mbe.2022269
![]() |
[15] |
M. N. Tiftik, T. G. Erdogan, A. K. Tarhan, A framework for multi-perspective process mining into a BPMN process model, Math. Biosci. Eng., 19 (2022), 11800–11820. https://doi.org/10.3934/mbe.2022550 doi: 10.3934/mbe.2022550
![]() |
[16] |
J. Grüger, M. Kuhn, R. Bergmann, Reconstructing invisible deviating events: A conformance checking approach for recurring events, Math. Biosci. Eng., 19 (2022), 11782–11799. https://doi.org/10.3934/mbe.2022549 doi: 10.3934/mbe.2022549
![]() |
[17] |
Y. Shi, X. Huang, Z. Du, J. Tan, Analysis of single-cell RNA-sequencing data identifies a hypoxic tumor subpopulation associated with poor prognosis in triple-negative breast cancer, Math. Biosci. Eng., 19 (2022), 5793–5812. https://doi.org/10.3934/mbe.2022271 doi: 10.3934/mbe.2022271
![]() |
1. | Jiyuan Xing, Senlin Luo, Limin Pan, Jingwei Hao, Yingdan Guan, Zhouting Wu, HGE-BVHD: Heterogeneous graph embedding scheme of complex structure functions for binary vulnerability homology discrimination, 2024, 238, 09574174, 121835, 10.1016/j.eswa.2023.121835 |
Recall | Precision | ||||||||
BinDiff | Asm2vec | PBDiff-S | PBDiff | BinDiff | Asm2vec | PBDiff-S | PBDiff | ||
diffutils | v2.8-v3.6 | 0.474 | 0.683 | 0.691 | 0.725 | 0.896 | 0.892 | 0.899 | 0.91 |
v3.1-v3.6 | 0.789 | 0.792 | 0.804 | 0.823 | 0.847 | 0.88 | 0.903 | 0.926 | |
v3.4-v3.6 | 0.892 | 0.897 | 0.917 | 0.945 | 0.936 | 0.941 | 0.949 | 0.956 | |
average | 0.718 | 0.791 | 0.804 | 0.831 | 0.893 | 0.904 | 0.917 | 0.931 | |
findutils | v4.2.33-v4.6.0 | 0.756 | 0.760 | 0.763 | 0.787 | 0.864 | 0.875 | 0.887 | 0.898 |
v4.4.1-v4.6.0 | 0.827 | 0.834 | 0.849 | 0.852 | 0.899 | 0.898 | 0.916 | 0.933 | |
average | 0.67 | 0.738 | 0.753 | 0.822 | 0.804 | 0.827 | 0.902 | 0.916 | |
Busybox | 1.20.0-1.30.0 | 0.625 | 0.671 | 0.665 | 0.761 | 0.766 | 0.799 | 0.827 | 0.864 |
1.20.0-1.27.2 | 0.714 | 0.804 | 0.840 | 0.883 | 0.842 | 0.856 | 0.855 | 0.878 | |
average | 0.67 | 0.738 | 0.753 | 0.822 | 0.804 | 0.827 | 0.841 | 0.871 | |
OpenSSL | 0.9.8a-1.0.2 | 0.315 | 0.492 | 0.585 | 0.604 | 0.524 | 0.623 | 0.675 | 0.682 |
1.0.1f-1.0.2 | 0.526 | 0.623 | 0.704 | 0.727 | 0.753 | 0.806 | 0.818 | 0.853 | |
average | 0.421 | 0.558 | 0.645 | 0.666 | 0.639 | 0.715 | 0.747 | 0.768 |
Recall | Precision | ||||||||
BinDiff | Asm2vec | PBDiff-S | PBDiff | BinDiff | Asm2vec | PBDiff-S | PBDiff | ||
diffutils | v2.8 O0 - O3 | 0.725 | 0.835 | 0.851 | 0.898 | 0.801 | 0.825 | 0.845 | 0.89 |
v2.8 O1 - O3 | 0.792 | 0.859 | 0.868 | 0.914 | 0.825 | 0.898 | 0.862 | 0.919 | |
v2.8 O2 - O3 | 0.833 | 0.88 | 0.902 | 0.935 | 0.848 | 0.912 | 0.892 | 0.926 | |
v3.1 O0 - O3 | 0.755 | 0.817 | 0.863 | 0.914 | 0.814 | 0.867 | 0.855 | 0.917 | |
v3.1 O1 - O3 | 0.782 | 0.829 | 0.875 | 0.922 | 0.833 | 0.892 | 0.872 | 0.936 | |
v3.1 O2 - O3 | 0.818 | 0.876 | 0.923 | 0.945 | 0.856 | 0.924 | 0.903 | 0.948 | |
v3.4 O0 - O3 | 0.744 | 0.832 | 0.899 | 0.923 | 0.82 | 0.872 | 0.891 | 0.905 | |
v3.4 O1 - O3 | 0.783 | 0.847 | 0.925 | 0.947 | 0.845 | 0.906 | 0.926 | 0.938 | |
v3.4 O2 - O3 | 0.805 | 0.902 | 0.938 | 0.969 | 0.868 | 0.933 | 0.947 | 0.967 | |
v3.6 O0 - O3 | 0.733 | 0.816 | 0.884 | 0.914 | 0.847 | 0.89 | 0.882 | 0.922 | |
v3.6 O1 - O3 | 0.768 | 0.825 | 0.899 | 0.925 | 0.875 | 0.925 | 0.942 | 0.947 | |
v3.6 O2 - O3 | 0.825 | 0.857 | 0.923 | 0.947 | 0.902 | 0.94 | 0.966 | 0.982 | |
Average | 0.78 | 0.848 | 0.896 | 0.929 | 0.845 | 0.899 | 0.899 | 0.933 | |
findutils | v4.233 O0 - O3 | 0.755 | 0.811 | 0.845 | 0.901 | 0.806 | 0.831 | 0.902 | 0.925 |
v4.233 O1 - O3 | 0.771 | 0.826 | 0.877 | 0.926 | 0.822 | 0.886 | 0.933 | 0.939 | |
v4.233 O2 - O3 | 0.812 | 0.834 | 0.892 | 0.938 | 0.837 | 0.893 | 0.947 | 0.954 | |
v4.41 O0 - O3 | 0.707 | 0.822 | 0.832 | 0.852 | 0.815 | 0.856 | 0.893 | 0.933 | |
v4.41 O1 - O3 | 0.732 | 0.834 | 0.849 | 0.893 | 0.835 | 0.874 | 0.925 | 0.94 | |
v4.41 O2 - O3 | 0.819 | 0.879 | 0.892 | 0.925 | 0.824 | 0.88 | 0.937 | 0.956 | |
v4.6 O0 - O3 | 0.714 | 0.81 | 0.844 | 0.901 | 0.829 | 0.845 | 0.872 | 0.882 | |
v4.6 O1 - O3 | 0.725 | 0.822 | 0.87 | 0.923 | 0.875 | 0.898 | 0.896 | 0.915 | |
v4.6 O2 - O3 | 0.799 | 0.859 | 0.885 | 0.937 | 0.916 | 0.922 | 0.903 | 0.933 | |
Average | 0.759 | 0.833 | 0.865 | 0.911 | 0.84 | 0.876 | 0.912 | 0.931 | |
Busybox | 1.20.0 O0-O3 | 0.823 | 0.838 | 0.902 | 0.933 | 0.878 | 0.864 | 0.89 | 0.915 |
1.20.0 O1-O3 | 0.856 | 0.856 | 0.915 | 0.946 | 0.899 | 0.9 | 0.926 | 0.937 | |
1.20.0 O2-O3 | 0.887 | 0.892 | 0.923 | 0.959 | 0.913 | 0.94 | 0.946 | 0.969 | |
1.30.0 O0-O3 | 0.801 | 0.823 | 0.834 | 0.856 | 0.822 | 0.835 | 0.903 | 0.915 | |
1.30.0 O1-O3 | 0.827 | 0.845 | 0.846 | 0.868 | 0.833 | 0.856 | 0.915 | 0.932 | |
1.30.0 O2-O3 | 0.845 | 0.872 | 0.872 | 0.893 | 0.849 | 0.866 | 0.922 | 0.946 | |
average | 0.840 | 0.854 | 0.882 | 0.909 | 0.866 | 0.877 | 0.917 | 0.936 | |
Openssl | 1.0.1f O0-O3 | 0.724 | 0.815 | 0.823 | 0.89 | 0.816 | 0.832 | 0.876 | 0.902 |
1.0.1f O1-O3 | 0.776 | 0.833 | 0.847 | 0.922 | 0.832 | 0.854 | 0.892 | 0.929 | |
1.0.1f O2-O3 | 0.789 | 0.852 | 0.88 | 0.946 | 0.849 | 0.866 | 0.923 | 0.954 | |
average | 0.763 | 0.833 | 0.85 | 0.919 | 0.832 | 0.851 | 0.897 | 0.928 |
Recall | Precision | ||||||||
BinDiff | TurboDiff | PBDiff-S | PBDiff | BinDiff | TurboDiff | PBDiff-S | PBDiff | ||
Busybox 1.27.2 | MIPS-ARM | 0.572 | 0.104 | 0.761 | 0.794 | 0.623 | 0.125 | 0.722 | 0.824 |
MIPS-x86 | 0.472 | 0.125 | 0.703 | 0.745 | 0.526 | 0.205 | 0.769 | 0.869 | |
MIPS-x64 | 0.566 | – | 0.825 | 0.862 | 0.614 | – | 0.846 | 0.896 | |
ARM-x86 | 0.416 | 0.204 | 0.795 | 0.83 | 0.554 | 0.311 | 0.825 | 0.877 | |
ARM-x64 | 0.325 | – | 0.886 | 0.909 | 0.474 | – | 0.915 | 0.927 | |
X86-x64 | 0.622 | – | 0.892 | 0.915 | 0.533 | – | 0.923 | 0.942 | |
Average | 0.496 | 0.144 | 0.810 | 0.842 | 0.554 | 0.214 | 0.833 | 0.889 | |
OpenSSL 1.0.1f | MIPS-ARM | 0.474 | 0.205 | 0.788 | 0.825 | 0.358 | 0.304 | 0.826 | 0.921 |
MIPS-x86 | 0.429 | 0.196 | 0.725 | 0.885 | 0.469 | 0.101 | 0.816 | 0.916 | |
MIPS-x64 | 0.566 | – | 0.749 | 0.904 | 0.624 | – | 0.838 | 0.925 | |
ARM-x86 | 0.462 | 0.254 | 0.828 | 0.915 | 0.392 | 0.158 | 0.845 | 0.937 | |
ARM-x64 | 0.38 | – | 0.816 | 0.923 | 0.454 | – | 0.825 | 0.906 | |
X86-x64 | 0.625 | – | 0.912 | 0.927 | 0.633 | – | 0.931 | 0.944 | |
Average | 0.489 | 0.218 | 0.803 | 0.90 | 0.488 | 0.188 | 0.847 | 0.925 |
Comparison function | ranking (MIPS) | ranking (ARM) | ranking (X64) | |
cjpeg9a | alloc_sarray | 3 | 1 | 16 |
cjpeg9a | get_text_gray_row | 2 | 2 | 49 |
wget1.19 | resp_new | 1 | 15 | 7 |
wget1.19 | url_parse | 2 | 16 | 4 |
curl-7.53.1 | ourWriteOut | 1 | 1 | 1 |
OpenSSL1.1.0 | ssl3_read_bytes | 1 | 1 | 1 |
Recall | Precision | ||||||||
BinDiff | Asm2vec | PBDiff-S | PBDiff | BinDiff | Asm2vec | PBDiff-S | PBDiff | ||
diffutils | v2.8-v3.6 | 0.474 | 0.683 | 0.691 | 0.725 | 0.896 | 0.892 | 0.899 | 0.91 |
v3.1-v3.6 | 0.789 | 0.792 | 0.804 | 0.823 | 0.847 | 0.88 | 0.903 | 0.926 | |
v3.4-v3.6 | 0.892 | 0.897 | 0.917 | 0.945 | 0.936 | 0.941 | 0.949 | 0.956 | |
average | 0.718 | 0.791 | 0.804 | 0.831 | 0.893 | 0.904 | 0.917 | 0.931 | |
findutils | v4.2.33-v4.6.0 | 0.756 | 0.760 | 0.763 | 0.787 | 0.864 | 0.875 | 0.887 | 0.898 |
v4.4.1-v4.6.0 | 0.827 | 0.834 | 0.849 | 0.852 | 0.899 | 0.898 | 0.916 | 0.933 | |
average | 0.67 | 0.738 | 0.753 | 0.822 | 0.804 | 0.827 | 0.902 | 0.916 | |
Busybox | 1.20.0-1.30.0 | 0.625 | 0.671 | 0.665 | 0.761 | 0.766 | 0.799 | 0.827 | 0.864 |
1.20.0-1.27.2 | 0.714 | 0.804 | 0.840 | 0.883 | 0.842 | 0.856 | 0.855 | 0.878 | |
average | 0.67 | 0.738 | 0.753 | 0.822 | 0.804 | 0.827 | 0.841 | 0.871 | |
OpenSSL | 0.9.8a-1.0.2 | 0.315 | 0.492 | 0.585 | 0.604 | 0.524 | 0.623 | 0.675 | 0.682 |
1.0.1f-1.0.2 | 0.526 | 0.623 | 0.704 | 0.727 | 0.753 | 0.806 | 0.818 | 0.853 | |
average | 0.421 | 0.558 | 0.645 | 0.666 | 0.639 | 0.715 | 0.747 | 0.768 |
Recall | Precision | ||||||||
BinDiff | Asm2vec | PBDiff-S | PBDiff | BinDiff | Asm2vec | PBDiff-S | PBDiff | ||
diffutils | v2.8 O0 - O3 | 0.725 | 0.835 | 0.851 | 0.898 | 0.801 | 0.825 | 0.845 | 0.89 |
v2.8 O1 - O3 | 0.792 | 0.859 | 0.868 | 0.914 | 0.825 | 0.898 | 0.862 | 0.919 | |
v2.8 O2 - O3 | 0.833 | 0.88 | 0.902 | 0.935 | 0.848 | 0.912 | 0.892 | 0.926 | |
v3.1 O0 - O3 | 0.755 | 0.817 | 0.863 | 0.914 | 0.814 | 0.867 | 0.855 | 0.917 | |
v3.1 O1 - O3 | 0.782 | 0.829 | 0.875 | 0.922 | 0.833 | 0.892 | 0.872 | 0.936 | |
v3.1 O2 - O3 | 0.818 | 0.876 | 0.923 | 0.945 | 0.856 | 0.924 | 0.903 | 0.948 | |
v3.4 O0 - O3 | 0.744 | 0.832 | 0.899 | 0.923 | 0.82 | 0.872 | 0.891 | 0.905 | |
v3.4 O1 - O3 | 0.783 | 0.847 | 0.925 | 0.947 | 0.845 | 0.906 | 0.926 | 0.938 | |
v3.4 O2 - O3 | 0.805 | 0.902 | 0.938 | 0.969 | 0.868 | 0.933 | 0.947 | 0.967 | |
v3.6 O0 - O3 | 0.733 | 0.816 | 0.884 | 0.914 | 0.847 | 0.89 | 0.882 | 0.922 | |
v3.6 O1 - O3 | 0.768 | 0.825 | 0.899 | 0.925 | 0.875 | 0.925 | 0.942 | 0.947 | |
v3.6 O2 - O3 | 0.825 | 0.857 | 0.923 | 0.947 | 0.902 | 0.94 | 0.966 | 0.982 | |
Average | 0.78 | 0.848 | 0.896 | 0.929 | 0.845 | 0.899 | 0.899 | 0.933 | |
findutils | v4.233 O0 - O3 | 0.755 | 0.811 | 0.845 | 0.901 | 0.806 | 0.831 | 0.902 | 0.925 |
v4.233 O1 - O3 | 0.771 | 0.826 | 0.877 | 0.926 | 0.822 | 0.886 | 0.933 | 0.939 | |
v4.233 O2 - O3 | 0.812 | 0.834 | 0.892 | 0.938 | 0.837 | 0.893 | 0.947 | 0.954 | |
v4.41 O0 - O3 | 0.707 | 0.822 | 0.832 | 0.852 | 0.815 | 0.856 | 0.893 | 0.933 | |
v4.41 O1 - O3 | 0.732 | 0.834 | 0.849 | 0.893 | 0.835 | 0.874 | 0.925 | 0.94 | |
v4.41 O2 - O3 | 0.819 | 0.879 | 0.892 | 0.925 | 0.824 | 0.88 | 0.937 | 0.956 | |
v4.6 O0 - O3 | 0.714 | 0.81 | 0.844 | 0.901 | 0.829 | 0.845 | 0.872 | 0.882 | |
v4.6 O1 - O3 | 0.725 | 0.822 | 0.87 | 0.923 | 0.875 | 0.898 | 0.896 | 0.915 | |
v4.6 O2 - O3 | 0.799 | 0.859 | 0.885 | 0.937 | 0.916 | 0.922 | 0.903 | 0.933 | |
Average | 0.759 | 0.833 | 0.865 | 0.911 | 0.84 | 0.876 | 0.912 | 0.931 | |
Busybox | 1.20.0 O0-O3 | 0.823 | 0.838 | 0.902 | 0.933 | 0.878 | 0.864 | 0.89 | 0.915 |
1.20.0 O1-O3 | 0.856 | 0.856 | 0.915 | 0.946 | 0.899 | 0.9 | 0.926 | 0.937 | |
1.20.0 O2-O3 | 0.887 | 0.892 | 0.923 | 0.959 | 0.913 | 0.94 | 0.946 | 0.969 | |
1.30.0 O0-O3 | 0.801 | 0.823 | 0.834 | 0.856 | 0.822 | 0.835 | 0.903 | 0.915 | |
1.30.0 O1-O3 | 0.827 | 0.845 | 0.846 | 0.868 | 0.833 | 0.856 | 0.915 | 0.932 | |
1.30.0 O2-O3 | 0.845 | 0.872 | 0.872 | 0.893 | 0.849 | 0.866 | 0.922 | 0.946 | |
average | 0.840 | 0.854 | 0.882 | 0.909 | 0.866 | 0.877 | 0.917 | 0.936 | |
Openssl | 1.0.1f O0-O3 | 0.724 | 0.815 | 0.823 | 0.89 | 0.816 | 0.832 | 0.876 | 0.902 |
1.0.1f O1-O3 | 0.776 | 0.833 | 0.847 | 0.922 | 0.832 | 0.854 | 0.892 | 0.929 | |
1.0.1f O2-O3 | 0.789 | 0.852 | 0.88 | 0.946 | 0.849 | 0.866 | 0.923 | 0.954 | |
average | 0.763 | 0.833 | 0.85 | 0.919 | 0.832 | 0.851 | 0.897 | 0.928 |
Recall | Precision | ||||||||
BinDiff | TurboDiff | PBDiff-S | PBDiff | BinDiff | TurboDiff | PBDiff-S | PBDiff | ||
Busybox 1.27.2 | MIPS-ARM | 0.572 | 0.104 | 0.761 | 0.794 | 0.623 | 0.125 | 0.722 | 0.824 |
MIPS-x86 | 0.472 | 0.125 | 0.703 | 0.745 | 0.526 | 0.205 | 0.769 | 0.869 | |
MIPS-x64 | 0.566 | – | 0.825 | 0.862 | 0.614 | – | 0.846 | 0.896 | |
ARM-x86 | 0.416 | 0.204 | 0.795 | 0.83 | 0.554 | 0.311 | 0.825 | 0.877 | |
ARM-x64 | 0.325 | – | 0.886 | 0.909 | 0.474 | – | 0.915 | 0.927 | |
X86-x64 | 0.622 | – | 0.892 | 0.915 | 0.533 | – | 0.923 | 0.942 | |
Average | 0.496 | 0.144 | 0.810 | 0.842 | 0.554 | 0.214 | 0.833 | 0.889 | |
OpenSSL 1.0.1f | MIPS-ARM | 0.474 | 0.205 | 0.788 | 0.825 | 0.358 | 0.304 | 0.826 | 0.921 |
MIPS-x86 | 0.429 | 0.196 | 0.725 | 0.885 | 0.469 | 0.101 | 0.816 | 0.916 | |
MIPS-x64 | 0.566 | – | 0.749 | 0.904 | 0.624 | – | 0.838 | 0.925 | |
ARM-x86 | 0.462 | 0.254 | 0.828 | 0.915 | 0.392 | 0.158 | 0.845 | 0.937 | |
ARM-x64 | 0.38 | – | 0.816 | 0.923 | 0.454 | – | 0.825 | 0.906 | |
X86-x64 | 0.625 | – | 0.912 | 0.927 | 0.633 | – | 0.931 | 0.944 | |
Average | 0.489 | 0.218 | 0.803 | 0.90 | 0.488 | 0.188 | 0.847 | 0.925 |
Comparison function | ranking (MIPS) | ranking (ARM) | ranking (X64) | |
cjpeg9a | alloc_sarray | 3 | 1 | 16 |
cjpeg9a | get_text_gray_row | 2 | 2 | 49 |
wget1.19 | resp_new | 1 | 15 | 7 |
wget1.19 | url_parse | 2 | 16 | 4 |
curl-7.53.1 | ourWriteOut | 1 | 1 | 1 |
OpenSSL1.1.0 | ssl3_read_bytes | 1 | 1 | 1 |