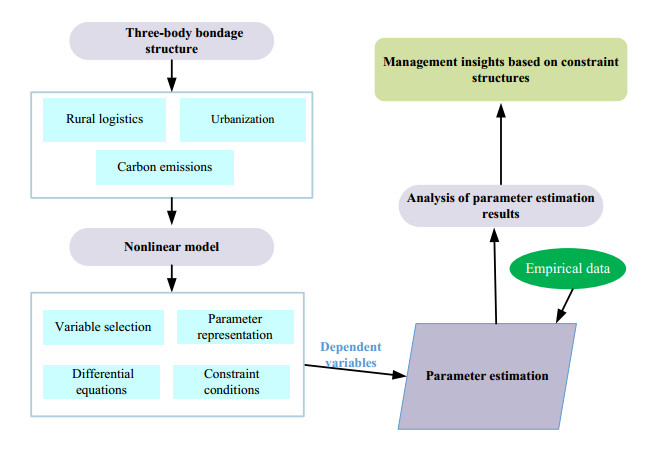
In an era where global focus intensifies on sustainable development, in this study, I investigate the interplay between rapid urbanization, rural logistics evolution, and carbon dynamics in China. We aim to bridge the gap in existing literature by examining the tripartite relationship between these areas and their collective impact on sustainable development. I explore the dynamic interaction mechanisms between urban construction, rural logistics development, and carbon emissions, assessing their joint influence on sustainable development. A detailed analysis of demand dynamics and market mechanisms supporting urbanization, rural logistics development, and carbon emissions has been initiated, leading to the establishment of a theoretical framework. This framework adeptly captures the interdependencies and constraints among these variables, offering a mathematical and bioscientific perspective to understand their complex interactions. Furthermore, a sophisticated nonlinear model based on key quantitative indicators like urbanization level, rural logistics development, and carbon emissions has been incorporated. Considering the multivariate nature, uncertainty, and dynamism presented by the nonlinear model, genetic algorithms have been employed for the estimation of model parameters. Through rigorous empirical testing using data from China spanning the years 1991–2021, I not only validate the effectiveness of the model but also accurately the interactions between urbanization processes, rural logistics progression, and carbon emissions. The findings demonstrate that urban construction significantly drives rural logistics development and uncover a pronounced nonlinear relationship among urbanization, rural logistics development (with a significant pull effect of 4.2), and carbon emissions growth. This research highlights the subtle balance between rural-urban development and environmental management, providing theoretical backing for the creation of sustainable policy frameworks in rural contexts and setting a foundation for future research in this domain.
Citation: Dongmei Zhang. Unveiling dynamics of urbanization, rural logistics, and carbon emissions: A study based on China's empirical data[J]. Mathematical Biosciences and Engineering, 2024, 21(2): 2731-2752. doi: 10.3934/mbe.2024121
[1] | Huanyu Chen, Jizheng Yi, Aibin Chen, Guoxiong Zhou . Application of PVAR model in the study of influencing factors of carbon emissions. Mathematical Biosciences and Engineering, 2022, 19(12): 13227-13251. doi: 10.3934/mbe.2022619 |
[2] | Xiangyang Ren, Xinxin Jiang, Liyuan Ren, Lu Meng . A multi-center joint distribution optimization model considering carbon emissions and customer satisfaction. Mathematical Biosciences and Engineering, 2023, 20(1): 683-706. doi: 10.3934/mbe.2023031 |
[3] | Hexiao Hu, Yalian Zhang, Chen Yao, Xin Guo, Zhijing Yang . Research on cost accounting of enterprise carbon emission (in China). Mathematical Biosciences and Engineering, 2022, 19(11): 11675-11692. doi: 10.3934/mbe.2022543 |
[4] | Zheng Liu, Hangxin Guo, Yuanjun Zhao, Bin Hu, Lihua Shi, Lingling Lang, Bangtong Huang . Research on the optimized route of cold chain logistics transportation of fresh products in context of energy-saving and emission reduction. Mathematical Biosciences and Engineering, 2021, 18(2): 1926-1940. doi: 10.3934/mbe.2021100 |
[5] | Wanli Zhou, Yingwen Gu, Xiulin Wang . Access to sanitary toilets and health outcomes: A panel data analysis using two-way fixed effects model. Mathematical Biosciences and Engineering, 2021, 18(6): 8815-8830. doi: 10.3934/mbe.2021435 |
[6] | Baotong Wu, Qi Tang . A sustainable scheduling system for medical equipment: Towards net zero goals for green healthcare. Mathematical Biosciences and Engineering, 2023, 20(10): 18960-18986. doi: 10.3934/mbe.2023839 |
[7] | Mitali Sarkar, Yong Won Seo . Biodegradable waste to renewable energy conversion under a sustainable energy supply chain management. Mathematical Biosciences and Engineering, 2023, 20(4): 6993-7019. doi: 10.3934/mbe.2023302 |
[8] | Shujuan Liu, Hui Jin, Yanbiao Di . A strategy for predicting waste production and planning recycling paths in e-logistics based on improved EMD-LSTM. Mathematical Biosciences and Engineering, 2023, 20(9): 17569-17588. doi: 10.3934/mbe.2023780 |
[9] | Yue Liu, Chunying Ma, Zhehao Huang . Can the digital economy improve green total factor productivity? An empirical study based on Chinese urban data. Mathematical Biosciences and Engineering, 2023, 20(4): 6866-6893. doi: 10.3934/mbe.2023296 |
[10] | Qinghua Liu, Siyu Yuan, Xinsheng Wang . A SEIARQ model combine with Logistic to predict COVID-19 within small-world networks. Mathematical Biosciences and Engineering, 2023, 20(2): 4006-4017. doi: 10.3934/mbe.2023187 |
In an era where global focus intensifies on sustainable development, in this study, I investigate the interplay between rapid urbanization, rural logistics evolution, and carbon dynamics in China. We aim to bridge the gap in existing literature by examining the tripartite relationship between these areas and their collective impact on sustainable development. I explore the dynamic interaction mechanisms between urban construction, rural logistics development, and carbon emissions, assessing their joint influence on sustainable development. A detailed analysis of demand dynamics and market mechanisms supporting urbanization, rural logistics development, and carbon emissions has been initiated, leading to the establishment of a theoretical framework. This framework adeptly captures the interdependencies and constraints among these variables, offering a mathematical and bioscientific perspective to understand their complex interactions. Furthermore, a sophisticated nonlinear model based on key quantitative indicators like urbanization level, rural logistics development, and carbon emissions has been incorporated. Considering the multivariate nature, uncertainty, and dynamism presented by the nonlinear model, genetic algorithms have been employed for the estimation of model parameters. Through rigorous empirical testing using data from China spanning the years 1991–2021, I not only validate the effectiveness of the model but also accurately the interactions between urbanization processes, rural logistics progression, and carbon emissions. The findings demonstrate that urban construction significantly drives rural logistics development and uncover a pronounced nonlinear relationship among urbanization, rural logistics development (with a significant pull effect of 4.2), and carbon emissions growth. This research highlights the subtle balance between rural-urban development and environmental management, providing theoretical backing for the creation of sustainable policy frameworks in rural contexts and setting a foundation for future research in this domain.
In the current global scenario, characterized by an intricate interplay between environmental and economic challenges [1,2], the phenomenon of climate change stands at the forefront, marked by a discernible increase in the frequency of natural disasters and extreme meteorological events [3,4]. These phenomena, while exerting significant impacts on both ecological systems and human societies, also pose a palpable threat to the stability of the global economic infrastructure [5]. Concurrently, the fabric of international relations is increasingly complicated by the variable nature of local conflicts and geopolitical tensions [6,7]. This complexity is further compounded by the widespread propagation of economic instability, which serves to amplify the uncertainties inherent in the global economic system [8].
Responding to this complex scenario, the Chinese government has proactively engaged in strategic planning to address these dual challenges. Central to this approach is the implementation of the Dual Circulation development strategy, complemented by the Dual Carbon objectives, forming the cornerstone of China's pursuit of sustainable development [9,10]. The Dual Circulation strategy endeavors to establish a harmonious balance between domestic and international economic flows [11], while concurrently addressing pressing environmental concerns. The Dual Carbon goals, on the other hand, are aimed at achieving carbon neutrality, a critical objective in mitigating the adverse impacts of climate change [12]. These strategies collectively embody a holistic approach, seeking not only to address environmental issues but also to stimulate economic growth, thereby representing a comprehensive response to the multifaceted challenges posed in the modern global context.
In the context of the strategic framework delineated for addressing contemporary global challenges, urbanization emerges as a pivotal element [13,14,15]. This process plays a dual role: First, by refining consumption patterns and advancing the consumption structure, urbanization effectively translates the demographic dividend into an expansive domestic market [16]. This transformation is instrumental in bolstering the domestic circulation economy. Second, urbanization enhances the connectivity between urban centers and international markets [17]. This improvement fosters national trade and investment. It also augments the global competitiveness of urban areas [18]. Consequently, urbanization acts not merely as a catalyst for domestic economic circulation but also synergizes with international economic flows, thus contributing significantly to the integration and interconnectedness of the global economy. However, this trajectory of urbanization, particularly in the context of China, is accompanied by a spectrum of environmental challenges, with carbon emissions being a focal concern. As urbanization intensifies, there is a concomitant increase in both energy consumption and carbon emissions [19]. This trend presents a formidable challenge in the pursuit of the Dual Carbon objectives, which aim for carbon peak and carbon neutrality. The dynamic interplay between urbanization and its environmental impact necessitates a comprehensive understanding and innovative approaches to ensure sustainable urban development while aligning with the broader goals of environmental conservation and carbon neutrality.
In the intricate urban-rural economic cycle, rural logistics serve as an indispensable conduit, playing a pivotal role in bolstering rural development and bridging the gap between rural economies and urban marketplaces [20]. The advancement of rural logistics is key to enhancing the distribution of agricultural produce, which in turn augments the income of farmers and catalyzes economic growth within rural domains [21]. This process, integral to the overall socioeconomic framework, contributes to the stabilization and prosperity of rural areas. However, the expansion of rural logistics systems presents a multifaceted challenge, primarily due to its association with environmental concerns, notably carbon emissions. As rural logistics networks grow, there is an observable increase in carbon emissions, a phenomenon that directly contradicts the objectives of China's Dual Carbon goals, which encompass both carbon peaking and carbon neutrality [22,23]. This presents a significant challenge in balancing the growth and efficiency of rural logistics with the imperative of environmental sustainability.
Therefore, it becomes imperative to dissect and understand the complex and dynamic interplay between urbanization, the evolution of rural logistics, and their consequent impact on carbon emissions. This understanding is crucial for devising strategies that can harmonize the objectives of economic development and environmental sustainability, particularly in the context of achieving the Dual Carbon targets. Such an analysis requires an interdisciplinary approach, combining insights from mathematical modeling, economic theory, and environmental science to create comprehensive solutions that align with the overarching goal of sustainable development.
In the realm of sustainable development, the interplay between urbanization, the evolution of rural logistics, and carbon emissions presents a multidimensional and intricate challenge. Historically, research in this domain has predominantly concentrated on the binary interactions between these elements. Nonetheless, a holistic understanding of their interdependencies and wider implications necessitates a thorough examination of several critical aspects:
1) How does urbanization impact carbon emissions? As the process of urbanization advances, do energy consumption and carbon emissions show an increasing trend, thereby posing challenges to the achievement of the Dual Carbon goals?
2) What is the correlation between the development of rural logistics and carbon emissions? Does the expansion of rural logistics lead to an increase in carbon emissions, and how can carbon neutrality be achieved while developing rural logistics?
The bondage structure and interactions between urbanization, rural logistics, and carbon emissions require more refined analysis in this study to deepen the understanding of these issues, as shown in Figure 1. We first construct a binding model based on the developmental demands and market laws of urbanization, rural logistics, and carbon emissions; second, we use a nonlinear model to capture the dynamic interactions among them. Next, based on empirical data from China from 1991 to 2021, we estimate parameters using advanced mathematical tools like genetic algorithms, ensuring the methodological rigor of the research. Finally, the interaction mechanisms between urbanization, rural logistics, and carbon emissions are revealed based on the parameter estimation results. The following subsections provide more detailed descriptions of the process. This research endeavors to bridge existing knowledge gaps and shed new light on the dynamic interplay between urbanization, rural logistics, and carbon emissions. We anticipate that our findings will elucidate the underlying mechanisms driving these interactions, thereby providing valuable insights for policymakers.
The paper is structured as follows: Section 3 introduces the binding structure between rural logistics development, urbanization, and carbon emissions, and establishes a nonlinear mathematical model. Section 4 utilizes empirical data from China to estimate the parameters of the nonlinear mathematical model according to the genetic algorithm. Section 5 uses the model parameters to validate the theoretical reasoning of the proposed model and presents management implications based on the binding structure. Finally, the concluding remarks are presented in Section 6.
The introduction of the concepts of dual carbon goals and the dual economic cycle has elevated the level of attention on urban construction, rural logistics, and carbon emissions to a new height. To systematically review the existing theoretical research in these three areas, based on the differences in the dimensions of the research elements, the content can be categorized into the following two types.
The foundational work of Behrends [24], Liu and Su [25], and Pradhan et al. [26] posited logistics development as a catalyst for urbanization, a premise further reinforced by Chanieabate et al. [27] through their identification of a positive correlation between logistics infrastructure and urbanization. Tan et al. [28], however, introduced the concept of a nonlinear relationship, where logistics' impact on urbanization varies in accordance with local demographic factors. Liu et al. [29] leveraged panel threshold models to uncover substantial interval effects, underscoring the complexity of interactions over mere linear correlations, thus opening avenues for intricate mathematical modeling and analysis in this field.
Pioneering research by Liu et al. [30], Lin et al. [31], and Tan et al. [32] positioned urbanization as a pivotal driver of carbon emissions, closely linked with environmental degradation [33,34]. Wang and Chen [35] illuminated a significant positive correlation between urbanization and carbon emissions, particularly in ASEAN countries, with land development playing a more crucial role than population growth [36]. The introduction of a U-shaped relationship between urbanization and carbon emissions by Shahbaz et al. [37], Wang et al. [38], and Peng et al. [39] suggests diverse patterns at different urbanization levels [40], indicating a complex system of variables that can be explored through advanced mathematical modeling in biosciences and engineering.
In exploring rural logistics, Lu and Liu [41] discerned a positive correlation with carbon emissions, marked by regional disparities. Zheng et al. [42] attributed high carbon emissions in Jiangsu to a larger scale of logistics output in employment, while Zhu et al. [43] emphasized the significant role of logistics' energy intensity in regional carbon emissions. The positive correlation between logistics performance and carbon emissions highlighted by Karaduman et al. [44], and the substantial contribution of road and air transport noted by Zhang et al. [45]. Zhang et al. [46] identified the expansion of logistics scale as a primary driver of emission growth, and Zhang and Li [47] revealed negative decoupling between logistics development and carbon emissions, all contribute to a nuanced understanding that can benefit from mathematical biosciences and engineering methodologies.
This comprehensive synthesis of existing binary relationships among urbanization, rural logistics, and carbon emissions delineates a complex and multifaceted interplay between these elements. Current research, however, indicates a notable gap in the theoretical exploration of their interconnectedness. Liang and Fang [48] scrutinized logistics development and urbanization as variables impacting logistics carbon emissions. Liu et al. [49] conducted an analysis from both national and regional perspectives, identifying logistics development as a benchmark for increased emissions on a national level, and land urbanization as a key regional factor. These studies, predominantly focusing on individual impacts, overlook the deeper interactive mechanisms between all three components. The mechanism of interplay between rural logistics, urbanization, and carbon emissions forms a complex and crucial area in environmental and economic sustainable development research. In the context of Dual-Carbon and Dual-Circulation strategies, understanding these interactions becomes increasingly crucial, presenting novel research perspectives and challenges for sustainable development. This scenario calls for a sophisticated approach in mathematical biosciences and engineering to model these complex relationships and contribute significantly to the field of sustainable development.
The interaction between rural logistics, urbanization, and carbon emissions forms a complex socio-economic environmental system. At a micro level of analysis, urbanization construction, accompanied by the aggregation of population and industries, optimizes spatial structure, enhances regional production capacity, and also stimulates a rise in the demand for rural logistics services. This growth in demand promotes the expansion of the rural logistics network, but it may also lead to reduced transportation efficiency and increased transportation costs. Additionally, urbanization might cause seasonal or cyclical fluctuations in the demand for rural logistics, adding complexity to logistics management. Due to the highly dispersed nature of rural logistics demand, it plays a key role in connecting rural areas with cities during the urbanization process.
As rural logistics develop, they address the issue of uneven infrastructure, enhance the efficiency of resource distribution between urban and rural areas, reduce logistics costs, and accelerate the penetration speed of agricultural products into cities and industrial goods into rural areas. This promotes the economic integration of urban and rural areas, advances the market integration of rural and urban areas, strengthens the market involvement of rural products, fosters the market expansion of products and services in urbanized areas, and narrows the social development gap between urban and rural areas, thus driving rapid urbanization. However, if rural logistics are not effective, it will inevitably hinder the economic development and market expansion of urbanized areas, affect the adjustment of rural industrial structure, fail to achieve effective aggregation of resources, manpower, and financial power, and delay the process of urbanization. Additionally, insufficient effectiveness of rural logistics will also inevitably lead to a large amount of unnecessary carbon emissions, increasing environmental constraints, and thereby restricting the development of rural logistics and urbanization.
At the macro level, urbanization construction triggers the aggregation of industries and resources, increasing societal consumption demand. This increase in demand directly impacts the development of rural logistics. Rural logistics provide logistic services for the needs of rural areas, and carbon emissions are a negative externality resulting from urbanization construction and the development of rural logistics. Due to insufficient development of rural logistics, the regional adaptability of rural logistics is reduced, limiting infrastructure construction, delaying the application and innovation of new logistics technologies, and weakening the spillover effects on economic, social, and spatial aspects of urbanization construction.
Moreover, weakened levels of urbanization construction fail to provide sufficient spillover effects for rural logistics, indirectly slowing down the development process of rural logistics, affecting the perfection of the rural logistics market mechanism, and failing to realize the spillover effects of rural logistics on urbanization construction. Both urbanization construction and the development of rural logistics rely on energy, leading to a large amount of carbon emissions. Excessive carbon emissions inevitably trigger environmental constraints, directly affecting resource allocation and environmental constraints. The aforementioned analysis reveals the cyclical feedback among rural logistics, urbanization, and carbon emissions. Urbanization drives the development of rural logistics, while effective rural logistics can support a more efficient urbanization process. Furthermore, increased carbon emissions may lead to changes in environmental policies, which in turn can affect the development modes of urbanization and rural logistics. Therefore, utilizing pattern recognition modeling methods to fundamentally grasp the structure between these three aspects, focusing on spillover effects, logistics effectiveness, and environmental constraints is crucial.
Despite the intricate and nonlinear interdependence between urbanization construction, rural logistics development, and carbon emissions, an analysis can be conducted from a goal-oriented perspective by considering their respective overarching objectives: the sustainable development of rural logistics, the improvement of urbanization quality, and carbon emission reduction. Taking into account the characteristics exhibited by each entity, such as the spillover effects of urbanization construction, the logistics efficiency of rural logistics, and the environmental constraints posed by carbon emissions, a framework depicting their interconnections can be constructed through the application of pattern recognition modeling methods. The specific configuration of this interrelated framework is illustrated in Figure 2.
The entities involved in urbanization construction, rural logistics development, and carbon emissions are driven by their individual objectives, necessitating them to make decisions that are relatively independent but contextually dependent. However, as integral components of the rural system, these entities are subject to inevitable influences from other entities. This bottom-up influence engenders a dynamic interplay among urbanization construction, rural logistics development, and carbon emissions, leading to their mutual permeation, interdependence, and reciprocal constraints. In order to further explore the intricate relationship among urbanization construction, rural logistics development, and carbon emissions, this study draws upon the theoretical framework of the Ternary Paradox. It distills the three nonlinear relationships of urbanization construction, rural logistics development, and carbon emissions into a coordination relationship between overall objectives and entity characteristics. As depicted in Figure 2, there exists a bondage relationship among urbanization construction, rural logistics development, and carbon emissions. The realization of the overall objectives of any two factors necessitates the coordination of the entity characteristics of the remaining factor. Furthermore, the interaction between any two factors exerts an impact on the third factor.
In a more nuanced perspective, urbanization construction exerts its influence on rural logistics development through the mechanism of spillover effects. However, it is important to note that the interplay between urbanization construction and rural logistics is not unilateral, as environmental constraints also exert their impact on both processes. Similarly, the development of rural logistics is contingent upon its ability to synchronize with urbanization construction by enhancing logistics efficiency. Nevertheless, this synergy is subject to the constraints imposed by the environment. Furthermore, the growth of carbon emissions plays a pivotal role in shaping the trajectory of urbanization construction and the sustainable development of rural logistics by means of environmental constraints. It is through the harmonization of logistics efficiency and environmental constraints that the level of urbanization construction can be elevated. The bondage relationship is manifested in the mutual constraints that exist among urbanization construction, rural logistics development, and carbon emissions, which impede the simultaneous attainment of their respective optimal objectives. It requires a process of negotiation and compromise among these three entities to find a balance and reconcile their divergent goals. Both urbanization construction and rural logistics development are constrained by environmental factors. The synergy between rural logistics development and carbon emissions growth is facilitated through spillover effects, while the mitigation of the impact of urbanization construction on carbon emissions growth necessitates the adjustment of logistics effectiveness. The relationship between urbanization construction, rural logistics development, and carbon emissions constitutes a complex network of constraints. This interplay can be comprehended by considering the spatial and temporal dimensions. From a spatial perspective, the effects between urbanization construction and rural logistics development can be examined within different spatial contexts but at the same point in time, encompassing various aspects such as positive or negative effects and causal relationships. Moreover, in the same spatial context but at different points in time, the non-linear and bifurcating relationship between the level of urbanization construction and carbon emissions can be explored. This nuanced understanding facilitates a deeper analysis of the intricate dynamics among these three components in the context of their spatial and temporal dimensions.
Urbanization construction, rural logistics development, and carbon emissions serve as central components in the study and implementation of China's carbon peak. These factors are pivotal for analyzing the dynamics of rural low-carbon development. In light of the proposed structural framework, it is imperative to construct a clear nonlinear model to comprehensively investigate the intricate interdependencies among these variables. Genetic algorithms are employed to identify the binding parameters of the nonlinear model, aiming to depict the strength of the constraints between urbanization construction, rural logistics development, and carbon emissions.
To quantitatively depict the bondage structure among urbanization construction, rural logistics development, and carbon emissions, a unified mathematical formulation is employed to represent their interdependencies.
y=f(x1,x2,x3,t) | (1) |
In this context, x1 denotes the magnitude of rural logistics development, x2 represents the level of urbanization construction, x3 signifies the quantity of carbon emissions, t denotes time, and f symbolizes the structural function that characterizes the interrelationships among these three variables.
From the analysis of the above structural constraints, it can be seen that the relationships between rural logistics, urbanization, and carbon emissions exhibit characteristics of cyclical feedback, dynamic changes, and complexity. Linear models are no longer sufficient to fully reveal the complex mechanisms of action. Nonlinear models, on the other hand, are a type of mathematical model that can more realistically reflect the complex relationships in the real world. This is particularly true in the analysis of the relationships among rural logistics, urbanization, and carbon emissions, where nonlinear models can reveal the complex and dynamic interactions among these factors. Therefore, this study utilizes the time-varying characteristics of differential equations to construct a data-driven nonlinear model based on differential formulas. As shown in Eq (2).
{dx1dt=∑3i=1a1,ixi+∑3i=1∑3j=1b1,i,jxixj+c1dx2dt=∑3i=1a2,ixi+∑3i=1∑3j=1b2,i,jxixj+c2dx3dt=∑3i=1a3,ixi+∑3i=1∑3j=1b3,i,jxixj+c3 | (2) |
In the given context, the coefficients a1,i, a2,i, a3,i, b1,i,j, b2,i,j, b3,i,j, and ci represent the influences and interactions between these variables. Specifically, coefficient a1,i quantifies the effects of rural logistics scale, urbanization construction level, and carbon emissions on the development of rural logistics. Coefficient a2,i characterizes the impacts of rural logistics development scale, urbanization construction level, and carbon emissions on urbanization construction. Coefficient a3,i captures the influence of rural logistics development scale, urbanization construction level, and carbon emissions growth on changes in carbon emissions. Coefficient b1,i,j describes the interaction among these variables and its impact on the scale of rural logistics development. Coefficient b2,i,j represents the interaction among these variables and its effect on the level of urbanization construction. Coefficient b3,i,j quantifies the interaction among these variables and its influence on carbon emissions. Last, coefficient ci represents the constant term.
The representation of the nonlinear model, as outlined in your description and depicted in the image, integrates both linear and nonlinear relationships between urbanization, rural logistics, and carbon emissions. The model takes into account the direct linear relations of each variable as well as the nonlinear interactions and cumulative effects, which are critical for a comprehensive understanding of these complex dynamics.
Urbanization construction, rural logistics development, and carbon emissions serve as central components in the study and implementation of China's carbon peak. These factors are pivotal for analyzing the dynamics of rural low-carbon development. In light of the proposed structural framework, it is imperative to construct a clear nonlinear model to comprehensively investigate the intricate interdependencies among these variables. Genetic algorithms are employed to identify the binding parameters of the nonlinear model, aiming to depict the strength of the constraints between urbanization construction, rural logistics development, and carbon emissions.
All the procedures for the GA have been implemented following [50,51]. The genetic algorithm is embedded within the optimization session for nonlinear model parameter estimation introduced in Section 3.2.1. This session is fed by supported by empirical data on urbanization, rural logistics, and carbon emissions in China, covering the period from 1991 to 2021. The learning session finds the best fitting values for the coefficients of the nonlinear model by minimizing the error between the model's estimated outputs for urbanization, rural logistics, and carbon emissions and the actual data. Using these automated procedures for tuning the coefficients of the nonlinear model, shown in Figure 3, a significant improvement in the accuracy of the parameters a, estimated by the nonlinear model, is expected.
In the proposed genetic algorithm, a sample (or individual) xi,j of the population is a vector whose elements (or genes) are the unknown coefficients to be inserted into the coefficients of the nonlinear model. Where the index i represents the sample of the population (individual) in each j iteration (or generation). The proposed genetic algorithm selects the individual xi,j with the best combination of genes through the procedure that is described step by step below.
1) Initialization. In the initial function, the number n of individuals of the population to observe is defined. At the initial step, the set of genes for the m individuals xi, within very wide predefined domains.
[x1,1⋮xn,1]=[a11,1,a21,1,a31,1,a111,1,a121,1,a131,1,a221,1,a231,1,a331,1,a1⋮a1n,1,a2n,1,a3n,1,a11n,1,a12n,1,a13n,1,a22n,1,a23n,1,a33n,1,an] | (3) |
2) Fitness. For each individual i:1→n, the corresponding set of genes, indicated in the rows of Eq (3), are used as coefficients of the nonlinear model presented in Section 3.2.1. For each set of genes, during the evolution of the corresponding nonlinear model parameter estimation algorithm, Eq (4) for i=1 is continuously updated. The Eq (4) represents the error between the actual and estimated output values of rural logistics, urbanization, and carbon emissions from the nonlinear model at each computational step k.
fitness(θki)=12√1n∑(xactual−xestimated)2 | (4) |
This choice of Eq (4) is crucial for obtaining the correct results of the optimization process. After applying the nonlinear model to the available measured data, by using the n sets of genes as coefficients of the nonlinear model, an initial vector containing the results of the fitness function is obtained.
3) Selection. With the selection function, the process enters in an iterative cycle, where each j cycle represents a new generation of individuals. In this function, the two individuals having the lowest fitness value are selected from the current population xj, i and defined as original individuals. To prevent premature convergence, the identifier is set to i≠j.
4) Crossover & Mutation. Cross the selected individuals with a probability of 0.8 to generate sub-individuals. Mutation operations are performed with a probability of 0.005 in the newly generated sub-individuals to introduce new genetic variations. Once the new vector of sub-individuals is obtained, a sequence of the nonlinear model elaborations is performed. Each nonlinear model elaboration uses the new coefficients given by the sub-genes and by using the same reference measured cell quantities. During the evolution of each nonlinear model, the new fitness values for the children are calculated using the same fitness function introduced in Eq (4).
5) Population Update. The next generation of the population k+1 consists of a combination of original individuals and sub-individuals, where worse original individuals are replaced by sub-individuals. In this way, the maximum amount is always n. The resulting fitness functions of the new population are simply obtained by merging the corresponding fitness functions of selected original individuals and sub-individuals. The fitness vector is used at the next iteration k+1 for the selection step, which selects the two parents of the new generation.
The genetic algorithm iterates generation after generation, continuously evolving until it reaches the predefined maximum number of iterations (k≤1000) or the fitness level meets a set threshold (ϵ<10−3). The iteration process stops upon satisfying one of these termination criteria, and specific results are then outputted. The algorithm selects the best-performing individual from the final generation as the optimal solution.
In this section, the time series data from 1991 to 2021 is utilized as the sample data to estimate the bondage parameters between urbanization, rural logistics development, and carbon emissions. The genetic algorithm is employed for this purpose in order to verify the rationality of the binding framework and the correctness of the nonlinear model.
The quantification of qualitative indicators in a scientific and rational manner is crucial for the validation of the structure and models of rural logistics development, urbanization, and carbon emissions. The principles of completeness and high frequency of usage were followed in the selection of indicators to maximize the reflection of the integrity and accuracy of the chosen quantitative indicators, as well as the representativeness and availability of the sample data [52,53]. x1 adopts the quantified indicator of rural logistics scale value, which reflects the overall development scale of rural logistics, as well as the ability to reduce logistics costs and promote the sustainable development of logistics [54]. x2 uses the quantified indicators of urbanization level, which are important measures for assessing China's economic development and industrialization [55]. It is a comprehensive reflection of regional social productivity development, technological progress, and industrial structure adjustment. It reflects the scale and achievements of urban construction over a certain period. This indicator is a composite index that can be measured by both urbanization rate and per capita gross domestic product. x3 adopts the quantified indicator of carbon emissions from rural logistics as a measure [56]. This indicator represents the absolute amount of carbon emissions generated by rural logistics over a certain period, providing an overall reflection of carbon reduction levels in rural logistics.
The data for rural logistics scale output, urbanization level, and rural logistics carbon emissions are sourced from the website of the National Bureau of Statistics of China, the China Urbanization Rate Survey Report, the China Energy Statistical Yearbook, and the China Rural Yearbook. The sample period is set from 1991 to 2021 in ordered to ensure data completeness.
By employing a randomness test method, 80% of the sample data was randomly selected for estimating the model parameters, while the remaining 20% of the sample data was used for validating the estimated parameter values. This approach ensures the robustness and reliability of the parameter estimation process using a representative portion of the data for estimation and an independent portion for testing the estimated values. Based on the genetic algorithm flowchart in Figure 3, the algorithm code was implemented using Matlab R2017b.
The genetic algorithm in this study was configured with a population size of 200 and a total of 1500 iterations. To enhance the clarity of the model structure and concentrate the parameters, a reduction was made in the number of components from 13 to 10 in the nonlinear model. This reduction aimed at eliminating redundant pairwise interactions that could potentially introduce duplicated influences. The estimated values of the nonlinear model parameters for the three groups were obtained through calculations, and the specific values are shown in Table 1.
Param | a11 | a12 | a13 | b111 | b112 | b113 | b122 | b123 | b133 | c1 | |
x1 | Set1 | –0.2629 | 0.854 | –0.259 | –0.912 | 5.052 | –2.631 | –3.06 | 0.63 | 0 | –0.008 |
Set2 | 3.517 | 1.078 | –4.36 | –7.406 | –4.220 | 4.433 | 0.785 | 2.938 | –2.609 | –0.034 | |
Set3 | 0.070 | 0.236 | –0.179 | –2.583 | 1.681 | 1.257 | –0.540 | 1.224 | –0.810 | 0.054 | |
Param | a21 | a22 | a23 | b211 | b212 | b213 | b222 | b223 | b233 | c2 | |
x2 | Set1 | 0.1564 | 0.344 | –0.340 | –0.562 | 4.317 | –3.613 | –2.511 | 1.124 | 0 | 0.001 |
Set2 | –0.062 | –1.284 | 1.327 | 0.075 | 0.000 | 0.000 | 9.077 | –16.495 | 7.353 | 0.042 | |
Set3 | –0.185 | –1.020 | 1.355 | 0.676 | 0.000 | 0.000 | 6.050 | –11.615 | 4.757 | 0.031 | |
Param | a31 | a32 | a33 | b311 | b312 | b313 | b322 | b323 | b333 | c3 | |
x3 | Set1 | –0.107 | 0.276 | 0.145 | 0.603 | 0.743 | –1.206 | –1.241 | 0.421 | 0 | –0.006 |
Set2 | 0.136 | –0.640 | 0.617 | –0.124 | 0.000 | 3.175 | 4.739 | –7.895 | 0.000 | 0.020 | |
Set3 | 0.145 | –0.634 | 0.606 | –0.134 | 0.000 | 0.000 | 4.653 | –7.624 | 2.995 | 0.019 |
To validate the effectiveness of the model parameter values, a sample analysis of the mathematical model is performed. The three sets of parameters are individually applied to the model to obtain calculated values, which are then compared with the actual values of the sample data.
Figure 4 represents a comparison between the sample values and calculated values of model parameter x1. In the Figure 4, curve 1 represents the sample curve of parameter x1, curve 2 represents the calculated curve of parameter x1 for the first set, curve 3 represents the calculated curve of parameter x1 for the second set, and curve 4 represents the calculated curve of parameter x1 for the third set. Based on the analysis of Figure 4, it can be observed that curve 2 and curve 4 have a similar magnitude of fluctuations, and both curves show a consistent overall trend without major variations. Curve 2 and curve 4 deviate significantly from the sample curve, and they do not align well with the observed trend of rural logistics development. This indicates that the first and third sets do not meet the requirements of parameter validity testing. Curve 3 and the sample curve exhibit a similar development trend, both experiencing significant fluctuations in the later stages of the sample period. This aligns well with the actual trend of rural logistics development, indicating that curve 3 satisfies the requirement of parameter validity testing. Therefore, the data from the second set can be considered as an alternative option for the model parameter x1.
Figure 5 is a comparison analysis between the sample values and calculated values of the model parameter x2. In the Figure 5, curve 1 represents the sample curve of parameter x2, curve 2 represents the calculated curve of parameter x2 for the first set, curve 3 represents the calculated curve of parameter x2 for the second set, and curve 4 represents the actual curve of parameter x2 for the third set. From Figure 5, it can be observed that curve 1 exhibits a similar development trend to curve 3 and curve 4. Throughout the entire sample period from 1991 to 2022, there are two peaks in urbanization development, indicating a significant non-linearity. This pattern aligns well with the trend of urbanization in China. Curve 2 exhibits relatively stable fluctuations and shows significant differences compared to the sample curve. This indicates that the first set of data does not meet the requirement of parameter validity testing. Therefore, the second and the third sets of data can be considered as alternative options for the model parameter x2.
Figure 6 is a comparison analysis between the sample values and calculated values of the model parameter x3. In the Figure 6, curve 1 represents the sample curve of parameter x3, curve 2 represents the calculated curve of parameter x3 for the first set, curve 3 represents the calculated curve of parameter x3 for the second set, and curve 4 represents the actual curve of parameter x3 for the third set. From Figure 6, it can be observed that all four curves exhibit significant non-linearity in their fluctuations. However, curve 2 and curve 4 share a similar fluctuation pattern with minor variations. Interestingly, they display opposite trends compared to the sample curve. This deviation from the sample curve indicates that curve 2 does not meet the requirement of parameter validity testing for the model parameter x3. Curve 1 and curve 3 exhibit a similar development trend, indicating that the second set of the calculated values can be considered as an alternative option for the model parameter x3.
To enhance the goodness of fit in parameter estimation, an analysis of the errors between the calculated values and the sample values is conducted for further optimization. Initially, the mean square errors (MSE) of the calculated values are compared with the MSE of the sample values for each of the three groups. The group with a higher MSE for the sample values compared to the calculated values is excluded. Next, the remaining two groups of calculated values are compared, and the group with the lower MSE is chosen as the optimal solution for parameter estimation.
Table 2 presents the three sets of error results for the parameters of the nonlinear model. From Table 2, it is observed that the mean square errors (MSE) of the first set of calculated values in the nonlinear model are greater than the MSE of the sample values. The MSE of the second set of calculated values in the nonlinear model is smaller than the MSE of the sample values. The MSE of the third set of calculated values in the nonlinear model shows both positive and negative deviations from the MSE of the sample values.
Parameters | First set (MSE) | Second set(MSE) | Third set(MSE) | |||
Calculated values | Sample values | Calculated values | Sample values | Calculated values | Sample values | |
x1 | 0.244 | 0.235 | 0.189 | 0.209 | 0.080 | 0.070 |
x2 | 0.251 | 0.102 | 0.214 | 0.245 | 0.036 | –0.06 |
x3 | 0.178 | 0.103 | 0.289 | 0.378 | 0.154 | 0.102 |
Therefore, the second set of calculated values in the nonlinear model is selected as the optimal solution for parameter estimation. The results of the parameter estimation for the nonlinear model are presented in Table 3.
a11 | a12 | a13 | b111 | b112 | b113 | b122 | b123 | b133 | c1 |
3.517 | 1.078 | –4.36 | –0.741 | 4.220 | –4.433 | 0.785 | 2.938 | –2.609 | –0.034 |
a21 | a22 | a23 | b211 | b212 | b213 | b222 | b223 | b233 | c2 |
0.063 | –1.284 | –1.327 | 0.075 | 0.001 | –0.001 | 9.077 | –1.645 | 7.353 | 0.042 |
a31 | a32 | a33 | b311 | b312 | b313 | b322 | b323 | b333 | c3 |
0.136 | 0.640 | –0.617 | 0.124 | 1.25 | 3.175 | 4.739 | –7.895 | 0.618 | 0.20 |
From Table 3, it can be observed that the numerical values of parameters a12 is 1.078 and the numerical values of parameters a21 is 0.063. The values indicate that rural logistics development and urbanization are interdependent, exhibiting a significant positive correlation. Moreover, the value of parameter of a12 further directly indicates that urbanization has a greater driving effect on rural logistics development. The numerical values of parameters a13 is –4.36, and the numerical values of parameters a23 is –1.327. These numerical values indicate that carbon emissions have constraint effects on rural logistics development and urbanization, but there are differences in the constraint effects between the two. It can be inferred that during the process of evolutionary development, rural logistics development, urbanization, and carbon emissions all exhibit accumulated cyclic effects from Table 3.
Coefficient b111 indicates the presence of an accumulated cyclic negative effect on rural logistics development, with its effect being –0.741. Therefore, rural logistics development should be adapted to local conditions. On the other hand, coefficients b222 and b333 suggest that both urbanization and carbon emissions show accumulated cyclic positive effects, with effects of 9.077 and 0.618, respectively. Thus, under the context of a dual carbon strategy, it is crucial to properly coordinate urbanization and carbon emissions.
Based on parameters derived from the nonlinear model, the evolutionary process of rural logistics development is analyzed. The numerical value of parameter b112 is 4.22, and the coefficient indicates that the interaction between rural logistics development and urbanization effectively promotes rural logistics development. The numerical value of parameter b113 is –4.433, and it demonstrates that the interaction between rural logistics development and carbon emissions constrains rural logistics development. The numerical value of parameter b123 is 2.938, indicating that the current interaction between urbanization and carbon emissions effectively drives rural logistics development, consistent with the rapid development of rural logistics in reality. Based on parameters derived from the nonlinear model, the evolutionary process of urbanization construction is analyzed. The numerical value of parameter b213 is −0.001, it demonstrates that the interaction between rural logistics and carbon emissions has a negative effect on urbanization. The numerical value of parameter b212 is 0.001, and the coefficient suggests that the interaction between urbanization and rural logistics development has a positive effect on urbanization. The numerical value of parameter b223 is 1.645, and the parameter illustrates that the interaction between urbanization and carbon emissions hinders the progress of urbanization, with a bondage effect of 1.645.
Based on parameters derived from the nonlinear model, the evolutionary process of carbon emissions is analyzed. The numerical value of parameter b312 is 1.25, the coefficient indicates that the interaction between urbanization and rural logistics promotes an increase in carbon emissions. The numerical value of parameter b313 is 3.175, and the coefficient indicates that the interaction between rural logistics development and carbon emissions promotes carbon emission growth. Parameter b323 shows that the interaction between urbanization and carbon emissions has a negative effect on carbon emissions, with an effect of –7.895. From the perspective of pairwise interactions, urbanization and carbon emissions have a more significant driving effect compared to rural logistics development, which is generally consistent with the actual situation. The nonlinear effects of the interaction between urbanization and rural logistics development have a more pronounced impact on promoting rural logistics development. The nonlinear effects of the interaction between urbanization and carbon emissions exert a significant inhibitory effect on carbon emission growth.
In conclusion, rural logistics development, urbanization, and carbon emission growth are all subject to both linear and nonlinear influences, validating the deductive logic within the bondage structure among the three factors as outlined in Section 3.1. The constructed nonlinear model has been used to verify the rationality of the bondage structure between rural logistics development, urbanization, and carbon emissions.
Based on the above research findings, we offer the following management policy recommendations for the implementation of the two major strategies and sustainable rural development.
Promoting the Green Logistics System in Rural Areas: Due to the constraining effect of carbon emissions on rural logistics and urbanization, it's crucial to advance sustainable development in rural logistics comprehensively. This involves re-planning and optimizing the rural logistics network to reduce transportation distances and times, thereby lowering energy use and carbon emissions. Introducing smart logistics systems can enhance efficiency and minimize unnecessary transport. Utilizing efficient logistics management software to optimize cargo loading and delivery routes is also key. Additionally, formulating policies that encourage the development of green logistics is essential, as it guides and promotes the widespread adoption of green logistics practices.
Implement Sustainable Urbanization Strategies: The dynamic nature of the relationship between rural logistics, urbanization, and carbon emissions necessitates continuous monitoring. Developing a circular economy model, promoting green building standards, and the use of energy-efficient building materials, as well as vigorously advancing the digital transformation of urbanization management, are key steps. Governments and organizations should adopt adaptive management strategies that integrate new data and insights in real time, allowing for the ongoing optimization of policies and practices.
Foster International Cooperation and Technological Exchange: Promote transnational carbon trading and the development of carbon markets, encourage international collaboration in low-carbon technology research and development, and share resources and outcomes. Strengthen the coordination and cooperation of international environmental protection policies, provide technical support and financial aid, and assist underdeveloped regions in building low-carbon infrastructure.
In this article, I delve into the intricate dynamics among urban development, advancement of rural logistics, and carbon emissions, forming an integrated structural framework to encapsulate these elements and employing a nonlinear model for analysis. This framework is substantiated with empirical data spanning from 1991 to 2019 in China. Key observations reveal a mutual dependency between rural logistics and urban development, both exhibiting a direct and positive correlation with the escalation of carbon emissions. Notably, urban development exerts a more pronounced impetus on rural logistics, whereas carbon emissions impose a regulatory effect on both domains. Furthermore, the interplay between urban development and carbon emissions significantly mitigates the increase of carbon emissions, while the interaction between the progression of rural logistics and carbon emissions curtails the expansion of rural logistics.
Therefore, two main research contributions are provided by this study. First, a bondage structure framework of urbanization, rural logistics and carbon emissions is established, which provides an analysis framework for the internal correlation analysis of urbanization, rural logistics and carbon emissions. Second, a new bondage model of low-carbon rural logistics, rural economy and carbon emissions is proposed, which effectively corrects the one-sided cognition of the "economy-social-ecological" synergy of the existing models, breaks the black box of the interaction between rural logistics, urbanization and carbon emissions, and provides theoretical support for the "dilemma" paradox of decoupling rural economic development and environmental protection.
Although the investigation yields valuable insights into the nexus of rural economics and ecological sustainability, it is not without its limitations. A primary constraint is the exclusive reliance on Chinese data from 1991 to 2019. Future studies should aim to incorporate a more expansive and diverse collection of international data, thereby broadening the research's scope and relevance. Additionally, forthcoming in-depth analyses should integrate a broader spectrum of potential determinants, including policy frameworks and technological advancements. Such an approach would pave the way for a more holistic examination, significantly elevating both the scientific rigor and practical applicability of the findings.
The author declares that they have not used Artificial Intelligence (AI) tools in the creation of this article.
This work is supported the China Grand Canal Research Institute of Yangzhou University (Grant No. DYH202205), and Jiangsu Provincial Federation of Philosophy and Social Sciences (Grant No. 23SSL027).
The author declares that there are no conflicts of interest.
[1] |
M. S. Anjum, S. M. Ali, M. Imad-Ud-Din, M. A. Subhani, M. N. Anwar, A.S. Nizami, et al., An emerged challenge of air pollution and ever-increasing particulate matter in Pakistan; a critical review, J. Hazard. Mater., 402 (2021), 123943. https://doi.org/10.1016/j.jhazmat.2020.123943 doi: 10.1016/j.jhazmat.2020.123943
![]() |
[2] |
X. Wu, Long La Niña events could rise in frequency as the planet warms, Nature, 619 (2023), 702–703. http://doi.org/10.1038/d41586-023-02331-z doi: 10.1038/d41586-023-02331-z
![]() |
[3] |
E. M. Fischer, U. Beyerle, L. Bloin-Wibe, C. Gessner, V. Humphrey, F. Lehner, et al., Storylines for unprecedented heatwaves based on ensemble boosting, Nat. Commun., 14 (2023), 4643. https://doi.org/10.1038/s41467-023-40112-4 doi: 10.1038/s41467-023-40112-4
![]() |
[4] |
C. Cao, X. Guan, W. Sun, S. Guo, B. Chen, Changes of extreme high temperature by global warming in the Northern Hemisphere, J. Appl. Meteorol. Climatol., 62 (2023), 3–11. https://doi.org/10.1175/JAMC-D-22-0043.1 doi: 10.1175/JAMC-D-22-0043.1
![]() |
[5] |
M. L. Tseng, T. D. Bui, M. K. Lim, M. Fujii, U. Mishra, Assessing data-driven sustainable supply chain management indicators for the textile industry under industrial disruption and ambidexterity, Int. J. Product. Econom., 245 (2022), 108401. https://doi.org/10.1016/j.ijpe.2021.108401 doi: 10.1016/j.ijpe.2021.108401
![]() |
[6] |
J. Pospisil, Dissolving conflict. Local peace agreements and armed conflict transitions, Peacebuilding, 10 (2022), 122–137. https://doi.org/10.1080/21647259.2022.2032945 doi: 10.1080/21647259.2022.2032945
![]() |
[7] |
U. Shahzad, K. S. Mohammed, S. Tiwari, J. Nakonieczny, R. Nesterowicz, Connectedness between geopolitical risk, financial instability indices and precious metals markets: novel findings from Russia Ukraine conflict perspective, Resour. Policy, 80 (2023), 103190. https://doi.org/10.1016/j.resourpol.2022.103190 doi: 10.1016/j.resourpol.2022.103190
![]() |
[8] |
I. Ali, A. Arslan, S. Tarba, T. Mainela, Supply chain resilience to climate change inflicted extreme events in agri-food industry: the role of social capital and network complexity, Int. J. Product. Econom., 264 (2023), 108968. https://doi.org/10.1016/j.ijpe.2023.108968 doi: 10.1016/j.ijpe.2023.108968
![]() |
[9] |
J. Fang, A. Collins, S. Yao, On the global COVID-19 pandemic and China's FDI, J. Asian Econom., 74 (2021), 101300. https://doi.org/10.1016/j.asieco.2021.101300 doi: 10.1016/j.asieco.2021.101300
![]() |
[10] |
M. Cai, J. Luo, Influence of COVID-19 on manufacturing industry and corresponding countermeasures from supply chain perspective, J. Shanghai Jiaotong Univ., 25 (2020), 409–416. https://doi.org/10.1007/s12204-020-2206-z doi: 10.1007/s12204-020-2206-z
![]() |
[11] |
J. J. Ou, L. Y. He, Opportunity or challenge? Carbon emissions reduction under new development pattern of dual circulation, Sustainability, 15 (2023), 1757. https://doi.org/10.3390/su15031757 doi: 10.3390/su15031757
![]() |
[12] |
L. Liu, X. Wang, Z. Wang, Recent progress and emerging strategies for carbon peak and carbon neutrality in China, Greenhouse Gases Sci. Technol., 13 (2023), 732–759. https://doi.org/10.1002/ghg.2235 doi: 10.1002/ghg.2235
![]() |
[13] |
B. Yang, A study of regional economic disparities and coordinated development based on improving grey model combinations, Scient. Programm. 2022 (2022), 3695361. https://doi.org/10.1155/2022/3695361 doi: 10.1155/2022/3695361
![]() |
[14] |
Q. Zhu, C. Xie, J. B. Liu, The impact of population agglomeration on ecological resilience: evidence from China, Math. Biosci. Eng., 20 (2023), 15898–15917. https://doi.org/10.3934/mbe.2023708 doi: 10.3934/mbe.2023708
![]() |
[15] |
L. Xu, D. Wang, J. Du, The heterogeneous influence of infrastructure construction on China's urban green and smart development—The threshold effect of urban scale, Land, 10 (2021), 1015. https://doi.org/10.3390/land10101015 doi: 10.3390/land10101015
![]() |
[16] |
M. Ahmad, G. Jabeen, Dynamic causality among urban agglomeration, electricity consumption, construction industry, and economic performance: generalized method of moments approach, Environ. Sci. Pollut. Res., 27 (2020), 2374–2385. https://doi.org/10.1007/s11356-019-06905-1 doi: 10.1007/s11356-019-06905-1
![]() |
[17] |
A. Yusuf, Dynamic effects of energy consumption, economic growth, international trade and urbanization on environmental degradation in Nigeria, Energy Strategy Rev., 50 (2023), 101228. https://doi.org/10.1016/j.esr.2023.101228 doi: 10.1016/j.esr.2023.101228
![]() |
[18] |
J. Wang, J. Chen, R. Li, Outward foreign direct investment and urban green productivity: promote or inhibit? International Rev. Econom. Finance, 88 (2023), 516–530. https://doi.org/10.1016/j.iref.2023.06.040 doi: 10.1016/j.iref.2023.06.040
![]() |
[19] |
Y. Long, S. Ayyoob, L. Huang, J. Chen, Urban carbon accounting: an overview, Urban Clim., 44 (2022), 101195. https://doi.org/10.1016/j.uclim.2022.101195 doi: 10.1016/j.uclim.2022.101195
![]() |
[20] |
H. Lu, J. Bao, Spatial differentiation effect of rural logistics in urban agglomerations in China based on the fuzzy neural network, Sustainability, 14 (2022), 9268. https://doi.org/10.3390/su14159268 doi: 10.3390/su14159268
![]() |
[21] |
D. Zhang, L. Wang, J. Xu, K. Chen, Simulation analysis of the evolutionary mechanism of low-carbon rural logistics, DYNA-Ingeniería e Industria, 96 (2021), 484–491. https://doi.org/10.6036/10236 doi: 10.6036/10236
![]() |
[22] |
W. Yao, W. Zhang, W. Li, Promoting the development of marine low carbon through the digital economy, J. Innov. Knowl., 8 (2023), 100285. https://doi.org/10.1016/j.jik.2022.100285 doi: 10.1016/j.jik.2022.100285
![]() |
[23] |
Z. Zhang, G. Hu, X. Mu, L. Kong, From low carbon to carbon neutrality: a bibliometric analysis of the status, evolution and development trend, J. Environ. Manage., 322 (2022), 116087. https://doi.org/10.1016/j.jenvman.2022.116087 doi: 10.1016/j.jenvman.2022.116087
![]() |
[24] |
S. Behrends, Recent developments in urban logistics research – A review of the proceedings of the international conference on city logistics 2009-2013, Transport. Res. Procedia, 12 (2016), 278–287. https://doi.org/10.1016/j.trpro.2016.02.065 doi: 10.1016/j.trpro.2016.02.065
![]() |
[25] |
T. Y. Liu, C. W. Su, Is transportation improving urbanization in China? Socio-Econom. Planning Sci., 77 (2021), 101034. https://doi.org/10.1016/j.seps.2021.101034 doi: 10.1016/j.seps.2021.101034
![]() |
[26] |
R. P. Pradhan, M. B. Arvin, M. Nair, Urbanization, transportation infrastructure, ICT, and economic growth: a temporal causal analysis, Cities, 115 (2021), 103213. https://doi.org/10.1016/j.cities.2021.103213 doi: 10.1016/j.cities.2021.103213
![]() |
[27] |
M. Chanieabate, H. He, C. Guo, B. Abrahamgeremew, Y. Huang, Examining the relationship between transportation infrastructure, urbanization level and rural-urban income gap in China, Sustainability, 15 (2023), 8410. https://doi.org/10.3390/su15108410 doi: 10.3390/su15108410
![]() |
[28] |
C. T. Tan, W. He, Y. R. Liu, Interregional transaction costs, industrial productivity and spatially-differentiated urbanization, Nankai Econom. Studies, 2021 (2021), 162–178+252. http://doi.org/10.14116/j.nkes.2021.06.010 doi: 10.14116/j.nkes.2021.06.010
![]() |
[29] |
H. C. Liu, X. L. Chen, X. F. Wang, Research on the threshold effect of urbanization and logistics total factor productivity, Rev. Econ. Manage., 36 (2020), 123–132. http://doi.org/10.13962/j.cnki.37-1486/f.2020.02.012 doi: 10.13962/j.cnki.37-1486/f.2020.02.012
![]() |
[30] | B. Liu, K. Cheng, Z. Ma, Research on the impacts of urbanization on logistics carbon emission changes, China Popul. Resour. Environ., 26 (2016), 54–60. |
[31] |
J. Lin, S. Lu, X. He, F. Wang, Analyzing the impact of three-dimensional building structure on CO2 emissions based on random forest regression, Energy, 236 (2021), 121502. https://doi.org/10.1016/j.energy.2021.121502 doi: 10.1016/j.energy.2021.121502
![]() |
[32] |
Y. Tan, Y. Liu, Y. Chen, Z. Zhang, D. Wu, H. Chen, et al., The impact of urban construction land change on carbon emissions—A case study of Wuhan city, Int. J. Environ. Res. Public Health, 20 (2023), 922. https://doi.org/10.3390/ijerph20020922 doi: 10.3390/ijerph20020922
![]() |
[33] |
S. Z. Huang, M. Sadiq, F. Chien, The impact of natural resource rent, financial development, and urbanization on carbon emission, Environ. Sci. Pollut. Res., 30 (2023), 42753–42765. https://doi.org/10.1007/s11356-021-16818-7 doi: 10.1007/s11356-021-16818-7
![]() |
[34] |
S. Zeng, A. Tanveer, X. Fu, Y. Gu, M. Irfan, Modeling the influence of critical factors on the adoption of green energy technologies, Renew. Sustain. Energy Rev., 168 (2022), 112817. https://doi.org/10.1016/j.rser.2022.112817 doi: 10.1016/j.rser.2022.112817
![]() |
[35] | X. Wang, Y. Cheng, Research on the influencing mechanism of urbanization on carbon emission efficiency—Based on an empirical study of 118 countries, World Regional Studies, 29 (2020), 503–511. |
[36] |
D. Zhang, Z. Wang, S. Li, H. Zhang, Impact of land urbanization on carbon emissions in urban agglomerations of the middle reaches of the Yangtze River, Int. J. Environ. Res. Public Health, 18 (2021), 1403. https://doi.org/10.3390/ijerph18041403 doi: 10.3390/ijerph18041403
![]() |
[37] |
M. Shahbaz, N. Loganathan, A. T. Muzaffar, K. Ahmed, M. A. Jabran, How urbanization affects CO2 emissions in Malaysia? The application of STIRPAT model, Renew. Sustain. Energy Rev., 57 (2016), 83–93. https://doi.org/10.1016/j.rser.2015.12.096 doi: 10.1016/j.rser.2015.12.096
![]() |
[38] |
Y. Wang, W. Chen, M. Zhao, B. Wang, Analysis of the influencing factors on CO2 emissions at different urbanization levels: regional difference in China based on panel estimation, Nat. Hazards, 96 (2019), 627–645. https://doi.org/10.1007/s11069-018-3561-z doi: 10.1007/s11069-018-3561-z
![]() |
[39] |
J. Peng, Y. Zheng, C. Liu, The impact of urban construction land use change on carbon emissions: evidence from the China land market in 2000-2019, Land, 11 (2022), 1440. https://doi.org/10.3390/land11091440 doi: 10.3390/land11091440
![]() |
[40] |
Z. Cheng, X. Hu, The effects of urbanization and urban sprawl on CO2 emissions in China, Environ. Dev. Sustain., 25 (2023), 1792–1808. https://doi.org/10.1007/s10668-022-02123-x doi: 10.1007/s10668-022-02123-x
![]() |
[41] |
S. Lu, Y. Liu, Evaluation system for the sustainable development of urban transportation and ecological environment based on SVM, J. Intell. Fuzzy Syst., 34 (2018), 831–838. https://doi.org/10.3233/JIFS-169376 doi: 10.3233/JIFS-169376
![]() |
[42] |
W. L. Zheng, J. W. Wang, X. H. Mao, J. F. Li, Ecological efficiency evaluation and spatiotemporal characteristics analysis of the linkage development of the logistics industry and manufacturing industry, Front. Energy Res., 9 (2022), 709582. https://doi.org/10.3389/fenrg.2021.709582 doi: 10.3389/fenrg.2021.709582
![]() |
[43] |
C. Zhu, M. Wang, Y. Yang, Analysis of the influencing factors of regional carbon emissions in the Chinese transportation industry, Energies, 13 (2020), 1100. https://doi.org/10.3390/en13051100 doi: 10.3390/en13051100
![]() |
[44] |
H. A. Karaduman, A. Karaman-Akgül, M. Çağlar, H. E. Akbaş, The relationship between logistics performance and carbon emissions: an empirical investigation on Balkan countries, Int. J. Clim. Change Strat. Manage., 12 (2020), 449–461. https://doi.org/10.1108/IJCCSM-05-2020-0041 doi: 10.1108/IJCCSM-05-2020-0041
![]() |
[45] |
S. Zhang, J. Wang, W. Zheng, Decomposition analysis of energy-related CO2 emissions and decoupling status in China's logistics industry, Sustainability, 10 (2018), 1340. https://doi.org/10.3390/su10051340 doi: 10.3390/su10051340
![]() |
[46] |
C. Zhang, W. Zhang, W. Luo, X. Gao, B. Zhang, Analysis of influencing factors of carbon emissions in China's logistics industry: a GDIM-based indicator decomposition, Energies, 14 (2021), 5742. https://doi.org/10.3390/en14185742 doi: 10.3390/en14185742
![]() |
[47] | G. L. Zhang, D. Li, An analysis of relationship among energy consumption, carbon emissions, and economic growth on China's logistics, Sci. Technol. Manage. Res., 35 (2015), 238–243. |
[48] |
W. Liang, Z.H. Fang, Study on the dynamic relationship between logistics industry growth, urbanization, and carbon emissions, Jianghan Academ., 38 (2019), 73–81. http://doi.org/10.16388/j.cnki.cn42-1843/c.2019.04.008 doi: 10.16388/j.cnki.cn42-1843/c.2019.04.008
![]() |
[49] |
B. Liu, Y. Wang, X. Chang, B. Nie, L. Meng, Y. Li, Does land urbanization affect the catch-up effect of carbon emissions reduction in China's logistics? Land, 11 (2022), 1503. https://doi.org/10.3390/land11091503 doi: 10.3390/land11091503
![]() |
[50] |
C. Rossi, C. Falcomer, L. Biondani, D. Pontara, Genetically optimized extended Kalman filter for state of health estimation based on Li-Ion batteries parameters, Energies, 15 (2022), 3404. https://doi.org/10.3390/en15093404 doi: 10.3390/en15093404
![]() |
[51] |
J. Lin, X. Li, Y. Wen, P. He, Modeling urban land-use changes using a landscape-driven patch-based cellular automaton (LP-CA), Cities, 132 (2023), 103906. https://doi.org/10.1016/j.cities.2022.103906 doi: 10.1016/j.cities.2022.103906
![]() |
[52] |
W. Liu, S. Wang, D. Dong, J. Wang, Evaluation of the intelligent logistics eco-index: evidence from China, J. Cleaner Prod., 274 (2020), 123127. https://doi.org/10.1016/j.jclepro.2020.123127 doi: 10.1016/j.jclepro.2020.123127
![]() |
[53] |
Y. C. Lin, C. L. Chen, C. F. Chao, W. H. Chen, H. Pandia, The study of evaluation index of growth evaluation of science and technological innovation micro-enterprises, Sustainability, 12 (2020), 6233. https://doi.org/10.3390/su12156233 doi: 10.3390/su12156233
![]() |
[54] |
G. X. Yao, X. Y. Bian, Y. He, Construction and simulation of rural logistics system dynamics model from the perspective of low-carbon economy, Soft Sci., 32 (2018), 60–66. http://doi.org/10.13956/j.ss.1001-8409.2018.02.13 doi: 10.13956/j.ss.1001-8409.2018.02.13
![]() |
[55] |
C. Gu, Urbanization: processes and driving forces, Sci. China Earth Sci., 62 (2019), 1351–1360. https://doi.org/10.1007/s11430-018-9359-y doi: 10.1007/s11430-018-9359-y
![]() |
[56] |
Y. Shen, J. Liu, W. Tian, Interaction between international trade and logistics carbon emissions, Energy Rep., 8 (2022), 10334–10345. https://doi.org/10.1016/j.egyr.2022.07.159 doi: 10.1016/j.egyr.2022.07.159
![]() |
Param | a11 | a12 | a13 | b111 | b112 | b113 | b122 | b123 | b133 | c1 | |
x1 | Set1 | –0.2629 | 0.854 | –0.259 | –0.912 | 5.052 | –2.631 | –3.06 | 0.63 | 0 | –0.008 |
Set2 | 3.517 | 1.078 | –4.36 | –7.406 | –4.220 | 4.433 | 0.785 | 2.938 | –2.609 | –0.034 | |
Set3 | 0.070 | 0.236 | –0.179 | –2.583 | 1.681 | 1.257 | –0.540 | 1.224 | –0.810 | 0.054 | |
Param | a21 | a22 | a23 | b211 | b212 | b213 | b222 | b223 | b233 | c2 | |
x2 | Set1 | 0.1564 | 0.344 | –0.340 | –0.562 | 4.317 | –3.613 | –2.511 | 1.124 | 0 | 0.001 |
Set2 | –0.062 | –1.284 | 1.327 | 0.075 | 0.000 | 0.000 | 9.077 | –16.495 | 7.353 | 0.042 | |
Set3 | –0.185 | –1.020 | 1.355 | 0.676 | 0.000 | 0.000 | 6.050 | –11.615 | 4.757 | 0.031 | |
Param | a31 | a32 | a33 | b311 | b312 | b313 | b322 | b323 | b333 | c3 | |
x3 | Set1 | –0.107 | 0.276 | 0.145 | 0.603 | 0.743 | –1.206 | –1.241 | 0.421 | 0 | –0.006 |
Set2 | 0.136 | –0.640 | 0.617 | –0.124 | 0.000 | 3.175 | 4.739 | –7.895 | 0.000 | 0.020 | |
Set3 | 0.145 | –0.634 | 0.606 | –0.134 | 0.000 | 0.000 | 4.653 | –7.624 | 2.995 | 0.019 |
Parameters | First set (MSE) | Second set(MSE) | Third set(MSE) | |||
Calculated values | Sample values | Calculated values | Sample values | Calculated values | Sample values | |
x1 | 0.244 | 0.235 | 0.189 | 0.209 | 0.080 | 0.070 |
x2 | 0.251 | 0.102 | 0.214 | 0.245 | 0.036 | –0.06 |
x3 | 0.178 | 0.103 | 0.289 | 0.378 | 0.154 | 0.102 |
a11 | a12 | a13 | b111 | b112 | b113 | b122 | b123 | b133 | c1 |
3.517 | 1.078 | –4.36 | –0.741 | 4.220 | –4.433 | 0.785 | 2.938 | –2.609 | –0.034 |
a21 | a22 | a23 | b211 | b212 | b213 | b222 | b223 | b233 | c2 |
0.063 | –1.284 | –1.327 | 0.075 | 0.001 | –0.001 | 9.077 | –1.645 | 7.353 | 0.042 |
a31 | a32 | a33 | b311 | b312 | b313 | b322 | b323 | b333 | c3 |
0.136 | 0.640 | –0.617 | 0.124 | 1.25 | 3.175 | 4.739 | –7.895 | 0.618 | 0.20 |
Param | a11 | a12 | a13 | b111 | b112 | b113 | b122 | b123 | b133 | c1 | |
x1 | Set1 | –0.2629 | 0.854 | –0.259 | –0.912 | 5.052 | –2.631 | –3.06 | 0.63 | 0 | –0.008 |
Set2 | 3.517 | 1.078 | –4.36 | –7.406 | –4.220 | 4.433 | 0.785 | 2.938 | –2.609 | –0.034 | |
Set3 | 0.070 | 0.236 | –0.179 | –2.583 | 1.681 | 1.257 | –0.540 | 1.224 | –0.810 | 0.054 | |
Param | a21 | a22 | a23 | b211 | b212 | b213 | b222 | b223 | b233 | c2 | |
x2 | Set1 | 0.1564 | 0.344 | –0.340 | –0.562 | 4.317 | –3.613 | –2.511 | 1.124 | 0 | 0.001 |
Set2 | –0.062 | –1.284 | 1.327 | 0.075 | 0.000 | 0.000 | 9.077 | –16.495 | 7.353 | 0.042 | |
Set3 | –0.185 | –1.020 | 1.355 | 0.676 | 0.000 | 0.000 | 6.050 | –11.615 | 4.757 | 0.031 | |
Param | a31 | a32 | a33 | b311 | b312 | b313 | b322 | b323 | b333 | c3 | |
x3 | Set1 | –0.107 | 0.276 | 0.145 | 0.603 | 0.743 | –1.206 | –1.241 | 0.421 | 0 | –0.006 |
Set2 | 0.136 | –0.640 | 0.617 | –0.124 | 0.000 | 3.175 | 4.739 | –7.895 | 0.000 | 0.020 | |
Set3 | 0.145 | –0.634 | 0.606 | –0.134 | 0.000 | 0.000 | 4.653 | –7.624 | 2.995 | 0.019 |
Parameters | First set (MSE) | Second set(MSE) | Third set(MSE) | |||
Calculated values | Sample values | Calculated values | Sample values | Calculated values | Sample values | |
x1 | 0.244 | 0.235 | 0.189 | 0.209 | 0.080 | 0.070 |
x2 | 0.251 | 0.102 | 0.214 | 0.245 | 0.036 | –0.06 |
x3 | 0.178 | 0.103 | 0.289 | 0.378 | 0.154 | 0.102 |
a11 | a12 | a13 | b111 | b112 | b113 | b122 | b123 | b133 | c1 |
3.517 | 1.078 | –4.36 | –0.741 | 4.220 | –4.433 | 0.785 | 2.938 | –2.609 | –0.034 |
a21 | a22 | a23 | b211 | b212 | b213 | b222 | b223 | b233 | c2 |
0.063 | –1.284 | –1.327 | 0.075 | 0.001 | –0.001 | 9.077 | –1.645 | 7.353 | 0.042 |
a31 | a32 | a33 | b311 | b312 | b313 | b322 | b323 | b333 | c3 |
0.136 | 0.640 | –0.617 | 0.124 | 1.25 | 3.175 | 4.739 | –7.895 | 0.618 | 0.20 |