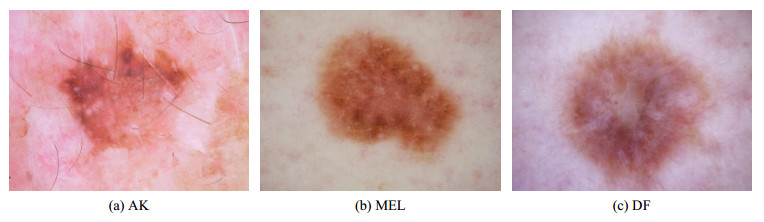
Methods based on deep learning have shown good advantages in skin lesion recognition. However, the diversity of lesion shapes and the influence of noise disturbances such as hair, bubbles, and markers leads to large intra-class differences and small inter-class similarities, which existing methods have not yet effectively resolved. In addition, most existing methods enhance the performance of skin lesion recognition by improving deep learning models without considering the guidance of medical knowledge of skin lesions. In this paper, we innovatively construct feature associations between different lesions using medical knowledge, and design a medical domain knowledge loss function (MDKLoss) based on these associations. By expanding the gap between samples of various lesion categories, MDKLoss enhances the capacity of deep learning models to differentiate between different lesions and consequently boosts classification performance. Extensive experiments on ISIC2018 and ISIC2019 datasets show that the proposed method achieves a maximum of 91.6% and 87.6% accuracy. Furthermore, compared with existing state-of-the-art loss functions, the proposed method demonstrates its effectiveness, universality, and superiority.
Citation: Li Zhang, Xiangling Xiao, Ju Wen, Huihui Li. MDKLoss: Medicine domain knowledge loss for skin lesion recognition[J]. Mathematical Biosciences and Engineering, 2024, 21(2): 2671-2690. doi: 10.3934/mbe.2024118
[1] | Xiao Ma, Xuemei Luo . Finger vein recognition method based on ant colony optimization and improved EfficientNetV2. Mathematical Biosciences and Engineering, 2023, 20(6): 11081-11100. doi: 10.3934/mbe.2023490 |
[2] | Hangle Hu, Chunlei Cheng, Qing Ye, Lin Peng, Youzhi Shen . Enhancing traditional Chinese medicine diagnostics: Integrating ontological knowledge for multi-label symptom entity classification. Mathematical Biosciences and Engineering, 2024, 21(1): 369-391. doi: 10.3934/mbe.2024017 |
[3] | Yu Li, Meilong Zhu, Guangmin Sun, Jiayang Chen, Xiaorong Zhu, Jinkui Yang . Weakly supervised training for eye fundus lesion segmentation in patients with diabetic retinopathy. Mathematical Biosciences and Engineering, 2022, 19(5): 5293-5311. doi: 10.3934/mbe.2022248 |
[4] | Ting Yao, Farong Gao, Qizhong Zhang, Yuliang Ma . Multi-feature gait recognition with DNN based on sEMG signals. Mathematical Biosciences and Engineering, 2021, 18(4): 3521-3542. doi: 10.3934/mbe.2021177 |
[5] | Xiaoliang Jiang, Junjian Xiao, Qile Zhang, Lihui Wang, Jinyun Jiang, Kun Lan . Improved U-Net based on cross-layer connection for pituitary adenoma MRI image segmentation. Mathematical Biosciences and Engineering, 2023, 20(1): 34-51. doi: 10.3934/mbe.2023003 |
[6] | Li Hou, Meng Wu, Hongyu Kang, Si Zheng, Liu Shen, Qing Qian, Jiao Li . PMO: A knowledge representation model towards precision medicine. Mathematical Biosciences and Engineering, 2020, 17(4): 4098-4114. doi: 10.3934/mbe.2020227 |
[7] | Cong Lin, Yiquan Huang, Wenling Wang, Siling Feng, Mengxing Huang . Lesion detection of chest X-Ray based on scalable attention residual CNN. Mathematical Biosciences and Engineering, 2023, 20(2): 1730-1749. doi: 10.3934/mbe.2023079 |
[8] | Guoli Wang, Pingping Wang, Jinyu Cong, Benzheng Wei . MRChexNet: Multi-modal bridge and relational learning for thoracic disease recognition in chest X-rays. Mathematical Biosciences and Engineering, 2023, 20(12): 21292-21314. doi: 10.3934/mbe.2023942 |
[9] | Huiying Zhang, Jiayan Lin, Lan Zhou, Jiahui Shen, Wenshun Sheng . Facial age recognition based on deep manifold learning. Mathematical Biosciences and Engineering, 2024, 21(3): 4485-4500. doi: 10.3934/mbe.2024198 |
[10] | Shuai Cao, Biao Song . Visual attentional-driven deep learning method for flower recognition. Mathematical Biosciences and Engineering, 2021, 18(3): 1981-1991. doi: 10.3934/mbe.2021103 |
Methods based on deep learning have shown good advantages in skin lesion recognition. However, the diversity of lesion shapes and the influence of noise disturbances such as hair, bubbles, and markers leads to large intra-class differences and small inter-class similarities, which existing methods have not yet effectively resolved. In addition, most existing methods enhance the performance of skin lesion recognition by improving deep learning models without considering the guidance of medical knowledge of skin lesions. In this paper, we innovatively construct feature associations between different lesions using medical knowledge, and design a medical domain knowledge loss function (MDKLoss) based on these associations. By expanding the gap between samples of various lesion categories, MDKLoss enhances the capacity of deep learning models to differentiate between different lesions and consequently boosts classification performance. Extensive experiments on ISIC2018 and ISIC2019 datasets show that the proposed method achieves a maximum of 91.6% and 87.6% accuracy. Furthermore, compared with existing state-of-the-art loss functions, the proposed method demonstrates its effectiveness, universality, and superiority.
Dermatology diagnosis is a crucial aspect of the medical field and remains an active area of research. As computer technology advances, artificial intelligence has increasingly infiltrated the medical sector, with one of its most promising applications being computer-assisted diagnosis [1]. Traditional computer-assisted methods mainly depended on hand-crafted features such as color, texture, and classifiers to achieve better results [2,3]. In this process, selecting the most suitable type of features and descriptors is crucial, which usually requires the expertise of practitioners. However, due to the constraints of manually designed features, it can be challenging to fully characterize skin lesions with intricate structures. The outcomes of traditional skin lesion recognition methods are often unsatisfactory. In recent years, deep learning (DL) has been successfully applied in various fields [4,5,6,7,8], becoming the core of mainstream tasks such as image classification [9,10,11], object detection [12,13], and image segmentation [14,15,16]. Compared with hand-crafted feature extraction, DL can achieve end-to-end feature extraction, effectively reducing the heavy workload caused by the manual selection of features. Therefore, researchers have proposed many methods for computer-assisted diagnosis based on DL [17].
Although these deep learning methods have effectively improved the recognition performance of skin lesions, there is still ample room for improvement. This is attributed to inter-class similarity and intra-class differences, the influence of markers, bubbles, and other factors, as well as the intricate characteristics of lesion areas. For example, as shown in Figure 1, there are three dermoscopic images selected from the ISIC 2019 dataset [18]. As we can see, Actinic keratosis (AK) (Figure 1(a)) shares great similarity with malignant melanoma (MEL) (Figure 1(b)) in color and morphology. The periphery of the Dermatofibroma (DF) (Figure 1(c)) shows a light brown color, as is the marginal color of the MEL, which might affect classification performance. Moreover, as we can see from the pictures in Figure 1, there will be hair, bubbles, marks, and other interference in the lesion area. This also increases the difficulty of accurately identifying skin lesions.
In light of the aforementioned problems, researchers have put forward some pertinent solutions. For instance, Tang et al. [19] proposed a GP-CNN model, which improved the classification performance of skin lesions by combining global and local feature information. He et al. [20] proposed a deep metric attention learning CNN (DeMAL-CNN) to address issues in skin lesion recognition. Golnoori et al. [21] proposed a metaheuristic algorithm-based hyper-parameters optimization to increase skin lesion recognition performance. Selen [22] proposed a swin transformer model based on end-to-end mapping without prior knowledge to ensure efficient learning in the classification of skin lesions. Wei et al. [23] proposed a skin lesion recognition network based on dual attention-assisted learning, which is able to focus on lesion area features with reduced irrelevant artifacts and is able to further refine the meaningful local pattern features contained in the lesion area. Wang et al. [24] proposed a novel loss function to enhance inter-class discriminative features and designed an independent module to focus on minimizing the intra-class distance. Moreover, to solve an image classification problem of engineering interest, Versaci et al. [25] proposed a classification procedure based on fuzzy similarity computations in order to create classes of images that are very close to each other.
These works have solved some problems relatively well in the recognition of skin lesions. However, these methods extracted features for classification from the input images without considering the application of medical knowledge of the skin disease itself. Therefore, in order to improve the performance of the feature extraction while further improving the accuracy of the classification network, this paper uses medical domain knowledge to guide DL models. The main contributions of this paper are as follows:
● We have innovatively proposed to integrate medical knowledge of skin lesions into deep learning (DL) models. By incorporating medical domain knowledge, deep learning models can learn more distinguishing features, which is conducive to more accurate recognition of skin lesions.
● Using the different color and shape characteristics of various skin lesions, we proposed a medical domain knowledge loss function (MDKLoss). By utilizing MDKLoss, we can further magnify the differences among various lesions, enhancing their distinguishability and aiding DL models in accurately identifying skin lesions.
● The proposed method is independent and can combine different DL models to improve the performance of skin lesion recognition. Experimental validation on two standard datasets has demonstrated the effectiveness of using different backbones, and a comparative analysis with other related methods shows that our method is superior.
The rest of the article is organized as below. The Section 2 reviews the related work. The proposed method is introduced in Section 3. Section 4 provides extensive experimental results and analyses, and the conclusion is given in Section 5.
The successful application of DL in the medical field brings more accurate and valuable reference information for doctors in clinical diagnoses. Intelligent and rapid screening greatly improves the diagnosis efficiency of doctors, which helps patients to receive the best time for diagnosis and treatment. Therefore, many DL methods for skin lesion recognition have been proposed. Zhuang et al. [26] proposed a cost-sensitive multi-classifier active fusion framework (CS-AF) to reduce the cost (e.g., money, time, or even life) of misclassifying more severe skin lesions as benign or less severe. Considering the relevant and complementary information of the two modalities, Wang et al. [27] proposed the anti-modal fusion attention mechanism (AMFAM), which can reduce intra-reader and inter-reader variability and improve diagnostic accuracy. Deng et al. [28] proposed a hierarchical representation learning method which is based on efficient structural pseudoinverse learning. Zhou et al. [29] proposed a semi-supervised framework which integrates four loss functions to reduce the large human effort involved in labeling data and then improve the performance of classification. Wan et al. [30] integrated semantic information at different levels and scales and designed an MSLANet which consisted of three long attention networks. At the same time, a deep data augmentation strategy was used to improve the generalization ability of the model. Kingsley et al. [31] designed a skin lesion diagnosis model named the OSSAE-BPNN model for automated skin lesion detection and classification. To overcome the data imbalance and insufficient data of deep learning networks problems on the training sets, Tsai et al. [32] proposed a method that utilizes multi-model ensemble with generated levels-of-detail images.
During the training of deep learning models, the loss function serves as a vital guide in directing the learning process. In order to achieve better results, researchers design a series of loss functions according to different learning tasks, including cross-entropy loss [33], commonly used in classification tasks, hinge loss [34], and focal loss [35], commonly used in target detection tasks. However, according to the uniqueness of different datasets, many researchers have designed different loss functions to better fit the DL models to achieve more accurate results. Yue et al. [36] introduced a multi-center skin lesion classification scheme by designing an adaptive weighted balance loss function. In order to achieve better segmentation effect and automatically pay more attention to difficult to segment boundaries (e.g., blurred boundary) to obtain more refined object boundaries, Du et al. [37] proposed a boundary sensitive loss function with the position constraint. Chaitanya et al. [38] proposed a local contrast loss to obtain semantic label information to learn good pixel-level features useful for segmentation. Although these methods can provide better results for their own work and have minimal impact on computational complexity, there is still plenty of room for improvement. Most loss functions not only fail to effectively address the issue of inter-class similarity and intra-class difference, but also exhibit certain limitations in specific domains.
Therefore, in this work, using the characteristic relationship in colors and patterns among skin lesions, we designed a loss to complete skin lesion recognition according to medical domain knowledge. This information mainly focus on the high similarity of color, texture, and morphology of different lesions and deal with the problem of intra-class difference and inter-class similarity through the strategy of optimizing the DL model.
In this section, we detail the skin lesion recognition method which integrates medical domain knowledge. The goal of our method is to train DL networks under the guidance of knowledge in the medical field that can effectively improve performance for skin lesion recognition. Moreover, we apply the proposed method to various models which have good performance on skin lesion recognition to prove the effectiveness and universality of our method. The following paragraphs will provide an overview of the framework and optimization strategy that will be discussed in detail.
In our study, we developed a strategy that leverages established deep learning architectures and integrates medical knowledge to construct a classifier for skin lesions. We first constructed a deep neural network model and used three established architectures, ResNet-18 [39], EfficientNetV2-S [40], and ConvNeXt-T [41], which are usually used for image classification tasks. The basic architecture and details of the solution we built are shown in Figure 2. First, input a mini-batch of skin lesion images. Second, a deep neural network model (such as ResNet-18, EfficientNetV2-S, ConvNeXt-T) is selected as the feature extractor for feature extraction. Then, output from a fully connected (FC) layer and conduct the parameter optimization which used the Cross-Entropy (CE) loss and the MDKLoss between samples. Finally, output the results of classification. The proposed MDKLoss enables the training of DL models in an end-to-end manner, without a significant increase in computational effort. Therefore, this method is worth popularizing and applying to computer-assisted diagnosis systems for skin lesions.
To optimize DL models, the proposed MDKLoss is used in the skin lesion recognition network. Inspired by the study of Wu et al. [42], we believe that domain knowledge provided by experts can be used as reliable prior knowledge to assist feature extraction directly. Therefore, it is reasonable to use and integrate medical knowledge into the DL model, which will be helpful for improving the recognition performance of skin lesions.
According to the similarity of different categories of skin lesions in color, texture, morphology, and other representations, we constructed the relationship information between different categories of samples, and designed the MDKLoss. To our knowledge, this is the first time medical knowledge of the relationships between skin lesions in a skin lesion recognition study has been applied. As the Reference [43], which describes the characteristics of different lesions under dermatoscopy, allows us to summarize the typical description of lesions, as shown in Table 1.
Type of lesion | Description | Examples |
Melanoma (MEL) | multiple colors (black, red, blue, gray, dark brown, light brown, and white); Irregular Streaks (Radial Streaming and Pseudopods) | ![]() |
Basal cell carcinoma (BCC) | blue-gray (sometimes brown); leaf-like structures (sometimes mimic streaks seen in MEL); pseudopods or brown aggregated globules | ![]() |
Melanocytic nevus (NV) | dark brown, light brown, or black; visible network pattern | ![]() |
Actinic keratosis (AK) | white-to-yellow surface scale; fine, wavy vessels (straight or coiled) surrounding the hair follicles; a background erythema sparing the hair follicles and resembling a pink-to-red pseudonetwork | ![]() |
Benign keratosis (BKL) | hairpin blood vessels with a white halo; milia-like cysts; network-like structures | ![]() |
Dermatofibroma (DF) | manifest different structures and patterns (some of which can simulate MEL) | ![]() |
Vascular lesion (VASC) | red/maroon lacunae and black lacunae; often exhibits a blue veil, erythema and/or ulceration | ![]() |
Squamous cell carcinoma (SCC) | typically reveals a central mass of keratin and ulceration surrounded by hairpin vessels, linear-irregular vessels and targetoid hair follicles | ![]() |
Following the semantic representation of skin lesions and doctors' analysis method, we can summarize the characteristics of the lesions in two aspects: the description of color and the description of pattern, as shown in Table 2. It is not uncommon to observe that various skin lesions may exhibit similar colors or patterns. This partly affects the correct discrimination to their categories. On the other hand, it also illustrates the same characteristic relationship between the different categories. For example, MEL has multiple colors such as black, red, brown, and so on, which looks like AK, DF, and SCC. In terms of pattern, MEL exhibits different structures and patterns, some of which can mimic DF. When there are similar colors or patterns, it can be easy to misclassify them. Based on this intuition, it can be concluded from Table 2 that MEL, AK, and DF are easily confused due to their similar feature representations. Consequently, we designed a novel medical domain knowledge loss strategy. By learning the information between samples in each mini-batch, the DL model recognizes the relationship between different categories and the same category. Under the guidance of the MDKLoss, the DL model can expand the distance among the samples of different lesion categories and make the samples of the same lesion category more clustered. The MDKLoss is conceptually similar to the triplet loss function [44], but there are differences in their meaning. The triplet loss function is centered around the anchor sample, positive sample, and negative sample, which are all generated by the same model. Through the model, the anchor sample and positive sample can cluster and move away from the negative sample. Therefore, our study achieves the MDKLoss by improving the triplet loss.
Type of lesion | Colors | Patterns | |||||||||||
black | dark brown | light brown | gray | blue | yellow | white | red | dots | clods | circles | radial | other | |
MEL | ✓ | ✓ | ✓ | ✓ | ✓ | ✓ | ✓ | ✓ | ✓ | ✓ | |||
BCC | ✓ | ✓ | ✓ | ✓ | ✓ | ||||||||
NV | ✓ | ✓ | ✓ | ✓ | ✓ | ||||||||
AK | ✓ | ✓ | ✓ | ✓ | ✓ | ✓ | ✓ | ||||||
BKL | ✓ | ✓ | ✓ | ||||||||||
DF | ✓ | ✓ | ✓ | ✓ | ✓ | ✓ | |||||||
VASC | ✓ | ✓ | |||||||||||
SCC | ✓ | ✓ | ✓ | ✓ | ✓ | ✓ | ✓ | ✓ |
In the DL model, the input skin lesion image x is mapped end-to-end to a multi-dimensional Euclidean space f(x), with the primary objective of optimizing the final feature representation. For each sample xi, three different features will be obtained through the DL model by the MDKLoss, which is recorded as f(xi), f(xsi), and f(xdi), respectively. f(xsi) represents the central characteristics between same categories, while f(xdi) represents the central characteristics of the different category. In the process of guiding the DL model, the purpose is to make the feature f(xi) closer to f(xsi), and as far away as possible from the f(xdi). The specific equation is as follows:
‖(f(xi)−f(xsi))‖22+α<‖(f(xi)−f(xdi))‖22 | (3.1) |
f(xsi)=1n(s)∑xi∈B,yi==sf(xi) | (3.2) |
f(xdi)=1n(d)∑xi∈B,yi==df(xi) | (3.3) |
n(y)=|{xi|xi∈B,yi==y}| | (3.4) |
The MDKLoss is defined as
LMDK=∑Ni[‖(f(xi)−f(xsi))‖22−∑yi≠d‖(f(xi)−f(xdi))‖22+α]+ | (3.5) |
where ‖.‖ represents the Euclidean distance, B={xi} stands for a mini-batch of training samples, yi corresponds to the category of skin lesions, and []+ is used to represent the loss value. If the value within the middle parentheses is greater than 0, then the loss value will be represented by this value. However, if the value within the brackets is less than 0, then the loss value will be 0. α is used as a margin to increase the distances between anchor picture pair and negative picture pair. And it represents the minimum interval of feature distances between xi and xdi, as well as the feature distances between xi and xsi.
To more clearly show the optimized effect of the MDKloss which was proposed according to the semantic representation of skin lesions, we present an example, as shown in Figure 3. The ds symbolizes the distance between samples of the same category, and du represents the distance between samples of different categories. By utilizing the MDKLoss to further magnify the differences among various lesions, DL models can enhance their distinguishability. Without the guidance of the MDKLoss, the information obtained by the DL model cannot accurately make a correct judgment for skin lesions. It is difficult for professional doctors to discriminate, because they visually showed a high similarity of intra-class and low differences of inter-class. After further guiding by the MDKloss, the DL model can bring the lesion samples of the same category as close as possible and the samples of different categories as far as possible according to the optimization goal. Therefore, under the guidance of the MDKLoss, the model can more accurately identify and classify the skin lesions.
Finally, given that skin lesion recognition is a multi-classification problem involving images, the cross-entropy loss function (LCE) [33] is typically incorporated into the classification model.
LCE=−1N∑it∑c=1yiclog(pic) | (3.6) |
pic=p(yi|xi) | (3.7) |
where t is the number of categories, and pic stands for the probability which the label of xi is yi. The loss function of the entire DL model is a combination of the cross-entropy loss and the MDKLoss, which can be expressed as
L=LCE+LMDK | (3.8) |
Furthermore, in addition to the cross-entropy loss, there are numerous other loss functions that can be combined with the MDKLoss using various combination rules.
In this study, we utilize two publicly available skin lesion recognition datasets, namely ISIC 2018 and ISIC 2019, to thoroughly compare the efficacy of our method with that of other existing methods.
● ISIC2018 is comprised of 10,208 labeled dermoscopic images, originating from the HAM10000 dataset [45]. Each image in the dataset has a resolution of 600 × 450 pixels and encompasses seven different types of skin lesions. These images were collected using various dermatoscopes from multiple institutions.
● ISIC2019 is available for training across eight different lesion types, which has 25,331 dermoscopic images. The images in the dataset were sourced from both the HAM10000 [45] and BCN20000 [46] datasets.
To evaluate and compare performance among state-of-the-art methods, we opted for widely recognized assessment metrics, such as accuracy, precision, recall, and F1-Score. In these metrics, true positives (TP) refer to instances where the model correctly predicted a positive outcome, while true negatives (TN) signify cases where the model accurately identified a negative outcome. False positive (FP) is a negative case predicted by the model as a positive class. False negative (FN) is a positive case predicted by the model as a negative class. Therefore, there are a total of four evaluation metrics in this study. For the final evaluation, we employed five-fold cross-validation and computed the mean value of each evaluation metric across the five trials as the results for all experiments. Generally speaking, the higher the value of the above indicators in a superior classification method, the better the classification effect is, and the maximum value is 1.
● Accuracy is the most commonly used indicator in the evaluation of image classification methods. In our work, we applied the accuracy to provide feedback on the classification results of each skin lesion. Formally, accuracy is defined as
Accuracy=TP+TNTP+FP+FN+TN | (4.1) |
● Precision, which describes the closeness of the measurements to each other, is a measure of statistical variability. Formally, precision is defined as
Precision=TPTP+FP | (4.2) |
● Recall represents the proportion of actual positives that are correctly detected as such. Formally, recall is defined as
Recall=TPTP+FN | (4.3) |
● F1-score is a harmonic mean considering both the precision and the recall. Formally, the F1-score is defined as
F1=2×Precision×RecallPrecision+Recall | (4.4) |
The method in this paper is implemented in the backend of PyTorch. All experimental results are obtained on a workstation equipped with a Nvidia GeForce RTX 3090 GPU card. We select three classical DL models as the feature extractor, including ResNet-18, EfficientNetV2-S, and ConvNeXt-T. These chosen deep learning models are initialized with weights that have been pre-trained on the ImageNet dataset [47]. All images are resized to 224×224. The SGD (stochastic gradient descent) optimizer is utilized with a learning rate of 0.001, and the momentum is 0.9. Additionally, the weight-decay parameter is set to 0.0001 in the optimizer. All DL models are trained for a total of 60 epochs and the mini-batch size is 64.
In this section, we compare the MDKLoss with CE loss, focal loss, and CBCE loss [48] for skin lesion recognition of ISIC2018 and ISIC2019 datasets. The CE loss is the classical loss function without parameter. Focal loss is a popular technique in the image domain that addresses model performance issues stemming from data imbalance. CBCE loss which introduced a weight factor, is a popular strategy for addressing the class-imbalance. Tables 3 and 4 present the experimental results of our method on two skin lesion image datasets, ISIC2018 and ISIC2019, respectively. The highest value is highlighted in bold of each evaluation metric for convenience.
Models | Loss Function | Accuracy | Precision | Recall | F1-score |
ResNet-18 | CE Loss | 0.883 | 0.853 | 0.738 | 0.785 |
ResNet-18 | Focal Loss | 0.887 | 0.831 | 0.748 | 0.784 |
ResNet-18 | CBCE Loss | 0.887 | 0.831 | 0.735 | 0.780 |
ResNet-18 | CE+MDKLoss (Ours) | 0.895 | 0.869 | 0.758 | 0.793 |
EfficientNetV2-S | CE Loss | 0.899 | 0.812 | 0.797 | 0.804 |
EfficientNetV2-S | Focal Loss | 0.903 | 0.825 | 0.812 | 0.815 |
EfficientNetV2-S | CBCE Loss | 0.896 | 0.802 | 0.685 | 0.729 |
EfficientNetV2-S | CE+MDKLoss (Ours) | 0.907 | 0.835 | 0.809 | 0.821 |
ConvNeXt-T | CE Loss | 0.901 | 0.812 | 0.797 | 0.804 |
ConvNeXt-T | Focal Loss | 0.909 | 0.866 | 0.836 | 0.845 |
ConvNeXt-T | CBCE Loss | 0.910 | 0.867 | 0.806 | 0.839 |
ConvNeXt-T | CE+MDKLoss (Ours) | 0.916 | 0.875 | 0.841 | 0.856 |
Models | Loss Function | Accuracy | Precision | Recall | F1-score |
ResNet-18 | CE Loss | 0.836 | 0.756 | 0.720 | 0.739 |
ResNet-18 | Focal Loss | 0.827 | 0.756 | 0.710 | 0.731 |
ResNet-18 | CBCE Loss | 0.841 | 0.755 | 0.721 | 0.738 |
ResNet-18 | CE+MDKLoss (Ours) | 0.842 | 0.770 | 0.722 | 0.744 |
EfficientNetV2-S | CE Loss | 0.859 | 0.762 | 0.714 | 0.735 |
EfficientNetV2-S | Focal Loss | 0.856 | 0.780 | 0.711 | 0.750 |
EfficientNetV2-S | CBCE Loss | 0.861 | 0.780 | 0.709 | 0.757 |
EfficientNetV2-S | CE+MDKLoss (Ours) | 0.873 | 0.791 | 0.724 | 0.757 |
ConvNeXt-T | CE Loss | 0.861 | 0.791 | 0.758 | 0.772 |
ConvNeXt-T | Focal Loss | 0.858 | 0.815 | 0.774 | 0.792 |
ConvNeXt-T | CBCE Loss | 0.856 | 0.788 | 0.757 | 0.771 |
ConvNeXt-T | CE+MDKLoss (Ours) | 0.876 | 0.821 | 0.785 | 0.799 |
As we can see from Table 3, the MDKLoss (ours) method is superior to other loss functions that were compared. For example, the proposed method achieved 91.6% accuracy with ConvNeXt-T on the ISIC-2018 dataset, while the existing techniques which used ResNet-18, EfficientNetV2-S, and ConvNeXt-T achieved nearly 90% accuracy. Moreover, in the precision, recall, and F1-Score evaluation metrics, the MDKLoss achieved 87.5%, 84.1%, and 85.6% with ConvNeXt-T, respectively, which achieved better performance than others. The results also show that our proposed loss strategy is more suitable and universal for skin lesion recognition with different DL models. The proposed method achieves better performance because the MDKLoss is able to integrate medical domain knowledge to increase the distance between different lesions.
To facilitate a more intuitive analysis, we present a graphical representation of various models' performance on the ISIC-2018 dataset using four evaluation metrics in Figure 4. Clearly, the MDKLoss has more obvious advantages, especially in the accuracy of the classification. This demonstrates that our method can more effectively guide and optimize the performance of DL models for identifying skin lesions.
To further verify the generalization ability of the MDKLoss (ours) method, we performed experiments with the same setup on the ISIC2019 dataset, and the results are tabulated in Table 4 and Figure 5. The results of the experiments clearly indicate that our approach is highly effective. The MDKLoss can improve the performance of any DL model, resulting in a maximum accuracy of 87.6%. As shown in Figure 5, we have separately compared the impact of the MDKLoss on different DL models. Our observations further confirm the effectiveness of this method.
The receiver operating characteristic (ROC) curve is a commonly used tool in medical statistics for comparing the effectiveness of different diagnostic methods for diseases. Therefore, we also present the ROC curve for analysis in Figure 6. Our method achieved an excellent ROC of 0.95 on the ISIC2018 dataset, and a superior ROC of 0.91 on the ISIC2019 dataset. However, due to the low proportion of DF categories in both datasets, the DF categories have a poor performance in the ROC results. Despite this, the overall average ROC results can still be above 0.9, which further validates the effectiveness of the MDKLoss (ours) method.
In addition, we also used the confusion matrix of the DL model (take ConvNeXt-T for example) on the ISIC2018 and 2019 datasets for analysis, as shown in Figure 7. It can be observed from Figure 7(c) that an accuracy of above 70% can be achieved for each category. This is because the MDKLoss (ours) utilizes the medicine knowledge of skin lesions to enlarge the distance of different skin lesions as much as possible, while reducing the distance of the same lesion category. Therefore, the DL model can classify these skin lesions more accurately and achieve better results. For instance, in Figure 7(a), the DL model can only identify AK which achieved 73.68% accuracy and we can observe that the probability of AK being mis-classified as MEL is as high as 5.26%. When we added the MDKLoss, the accuracy rate of AK recognition was increased to 75.76%, and the probability of mis-classifying MEL was reduced to 3.03%. Similarly, we also found that the error rate between DF and AK was reduced, which also verified that our method could be able to alleviate the problems raised in Figure 1. This indicates that using MDKLoss to assist the classification of skin lesions has practical significance and value, and thus it is a worthy reference for doctors.
To gain a deeper understanding of the impact of the MDKLoss, we selected some skin lesion images for visualization to prove the effectiveness of our method. As depicted in Figure 8, the first row displays the true labels, while the second row showcases the predicted labels provided by the baseline model. The third row represents the predictions made by the model incorporating the MDKLoss. From the results, we can see that the misclassification of these three categories appears without the MDKLoss. The inclusion of this guidance function enables the correction of misclassified categories, demonstrating that the MDKLoss possesses the capability to rectify mixed categories. In the visualization results, when the MDKLoss is not added, the model does identify AK as DF or DF as MEL. When the MDKLoss was added for training, the model was able to correctly recognize the skin lesions. This illustrates the effectiveness of MDKLoss.
Meanwhile, we visualize the attention maps of the ConvNeXt-T model with MDKLoss in Figure 9. These attention maps are used to locate meaningful sub-regions in the input image. The observation reveals that the MDKLoss-based model is highly resilient to hair or air bubble interference, allowing it to concentrate on the lesion site for accurate classification. Notably, this model exhibits enhanced learning and training capabilities when guided by the MDKLoss, which aligns with the description of skin lesions. Take Figure 9(b) for example. The attention maps of the ConvNeXt-T model focus entirely on the entire region of the DF lesion. The "Circles" attributes of the DF can be clearly resolved which was summarized in Table 2. Therefore, the visualization results further illustrate the significant role of MDKLoss and are able to make the model more interpretable.
The manual inspection of skin lesions has been a difficult task for doctors and healthcare professionals. Nevertheless, the emergence of computer-aided medical diagnosis has greatly facilitated the progress of intelligent medicine. In this study, we utilized a deep learning model to classify various types of skin lesions on the ISIC2018 and ISIC2019 datasets. By developing a novel training model loss strategy based on medical domain knowledge, we were able to distinguish between different classes of skin lesions with similar characteristics more effectively, which contributed to the recognition of skin lesions. Furthermore, our research can be applied to other skin lesion datasets, and we believe it will achieve better results. Additionally, reliable and effective deep learning methods that incorporate medical domain knowledge are deserving of promotion and application. However, it is undeniable that the extreme imbalance of datasets also has a significant impact on the performance of deep learning models. Therefore, in future work, we will continue to explore more advanced deep learning methods to improve the performance of the models and provide more efficient and cost-effective diagnostic algorithms for areas with limited medical resources to reduce the burden on healthcare systems.
The authors declare they have not used Artificial Intelligence (AI) tools in the creation of this article.
This research was funded by National Natural Science Foundation of China (No. 62006049), Basic and Applied Basic Research Foundation of Guangdong Province (No. 2023A1515010939), Project of Education Department of Guangdong Province (Nos. 2022KTSCX068 and 2021ZDZX1079).
The authors declare there is no conflict of interest.
[1] |
K. Doi, Computer-aided diagnosis in medical imaging: historical review, current status and future potential, Comput. Med. Imaging Graphics, 31 (2007), 198–211. https://doi.org/10.1016/j.compmedimag.2007.02.002 doi: 10.1016/j.compmedimag.2007.02.002
![]() |
[2] |
M. Ahammed, M. A. Mamun, M. S. Uddin, A machine learning approach for skin disease detection and classification using image segmentation, Healthcare Anal., 2 (2022), 100122. https://doi.org/10.1016/j.health.2022.100122 doi: 10.1016/j.health.2022.100122
![]() |
[3] |
J. Zhang, Y. Xia, Y. Xie, M. Fulham, D. D. Feng, Classification of medical images in the biomedical literature by jointly using deep and handcrafted visual features, IEEE J. Biomed. Health. Inf., 22 (2017), 1521–1530. https://doi.org/10.1109/JBHI.2017.2775662 doi: 10.1109/JBHI.2017.2775662
![]() |
[4] |
P. I. R. Jenifer, S. Kannan, Deep learning with optimal hierarchical spiking neural network for medical image classification, Comput. Syst. Sci. Eng., 44 (2023), 1081–1097. https://doi.org/10.32604/csse.2023.026128 doi: 10.32604/csse.2023.026128
![]() |
[5] |
J. Dominic, N. Bhaskhar, A. D. Desai, A. Schmidt, E. Rubin, B. Gunel, et al., Improving data-efficiency and robustness of medical imaging segmentation using inpainting-based self-supervised learning, Bioengineering, 10 (2023), 207. https://doi.org/10.3390/bioengineering10020207 doi: 10.3390/bioengineering10020207
![]() |
[6] |
L. Tan, H. Wu, J. Xia, Y. Liang, J. Zhu, Skin lesion recognition via global-local attention and dual-branch input network, Eng. Appl. Artif. Intell., 127 (2023), 107385. https://doi.org/10.1016/j.engappai.2023.107385 doi: 10.1016/j.engappai.2023.107385
![]() |
[7] |
F. Bozkurt, Skin lesion classification on dermatoscopic images using effective data augmentation and pre-trained deep learning approach, Multimedia Tools Appl., 82 (2023), 18985–19003. https://doi.org/10.1007/s11042-022-14095-1 doi: 10.1007/s11042-022-14095-1
![]() |
[8] |
X. Feng, T. Wang, X. Yang, M. Zhang, W. Guo, W. Wang, Convwin-unet: unet-like hierarchical vision transformer combined with convolution for medical image segmentation, Math. Biosci. Eng., 20 (2023), 128–144. https://doi.org/10.3934/mbe.2023007 doi: 10.3934/mbe.2023007
![]() |
[9] |
M. Abdar, M. A. Fahami, L. Rundo, P. Radeva, A. F. Frangi, U. R. Acharya, et al., Hercules: deep hierarchical attentive multilevel fusion model with uncertainty quantification for medical image classification, IEEE Trans. Ind. Inf., 19 (2023), 274–285. https://doi.org/10.1109/TII.2022.3168887 doi: 10.1109/TII.2022.3168887
![]() |
[10] |
Z. Yang, Y. Bao, Y. Liu, Q. Zhao, H. Zheng, Research on deep learning garbage classification system based on fusion of image classification and object detection classification, Math. Biosci. Eng., 20 (2022), 4741–4759. https://doi.org/10.3934/mbe.2023219 doi: 10.3934/mbe.2023219
![]() |
[11] |
H. Rastegar, D. Giveki, Designing a new deep convolutional neural network for skin lesion recognition, Multimedia Tools Appl., 82 (2023), 18907–18923. https://doi.org/10.1007/s11042-022-14181-4 doi: 10.1007/s11042-022-14181-4
![]() |
[12] |
Z. Wu, C. Liu, J. Wen, Y. Xu, J. Yang, X. Li, Selecting high-quality proposals for weakly supervised object detection with bottom-up aggregated attention and phase-aware loss, IEEE Trans. Image Process., 32 (2023), 682–693. https://doi.org/10.1109/TIP.2022.3231744 doi: 10.1109/TIP.2022.3231744
![]() |
[13] |
T. Diwan, G. Anirudh, J. V. Tembhurne, Object detection using yolo: challenges, architectural successors, datasets and applications, Multimedia Tools Appl., 82 (2023), 9243–9275. https://doi.org/10.1007/s11042-022-13644-y doi: 10.1007/s11042-022-13644-y
![]() |
[14] |
T. Shen, F. Huang, X. Zhang, CT medical image segmentation algorithm based on deep learning technology, Math. Biosci. Eng., 20 (2023), 10954–10976. https://doi.org/10.3934/mbe.2023485 doi: 10.3934/mbe.2023485
![]() |
[15] |
Y. Zhong, Z. Tang, H. Zhang, Y. Xie, X. Gao, A froth image segmentation method via generative adversarial networks with multi-scale self-attention mechanism, Multimedia Tools Appl., 2023. https://doi.org/10.1007/s11042-023-16397-4 doi: 10.1007/s11042-023-16397-4
![]() |
[16] |
S. Xue, H. Wang, X. Guo, M. Sun, K. Song, Y. Shao, et al., Cts-net: A segmentation network for glaucoma optical coherence tomography retinal layer images, Bioengineering, 10 (2023), 230. https://doi.org/10.3390/bioengineering10020230 doi: 10.3390/bioengineering10020230
![]() |
[17] |
H. Li, X. Tao, T. Liang, J. Jiang, J. Zhu, S. Wu, et al., Comprehensive ai-assisted tool for ankylosing spondylitis based on multicenter research outperforms human experts, Front. Public Health, 11 (2023), 1063633. https://doi.org/10.3389/fpubh.2023.1063633 doi: 10.3389/fpubh.2023.1063633
![]() |
[18] |
B. Cassidy, C. Kendrick, A. Brodzicki, J. Jaworek-Korjakowska, M. H. Yap, Analysis of the isic image datasets: usage, benchmarks and recommendations, Med. Image Anal., 75 (2022), 102305. https://doi.org/10.1016/j.media.2021.102305 doi: 10.1016/j.media.2021.102305
![]() |
[19] |
P. Tang, Q. Liang, X. Yan, S. Xiang, D. Zhang, Gp-cnn-dtel: Global-part cnn model with data-transformed ensemble learning for skin lesion classification, IEEE J. Biomed. Health. Inf., 24 (2020), 2870–2882. https://doi.org/10.1109/JBHI.2020.2977013 doi: 10.1109/JBHI.2020.2977013
![]() |
[20] |
X. He, Y. Wang, S. Zhao, C. Yao, Deep metric attention learning for skin lesion classification in dermoscopy images, Complex Intell. Syst., 8 (2022), 1487–1504. https://doi.org/10.1007/s40747-021-00587-4 doi: 10.1007/s40747-021-00587-4
![]() |
[21] |
F. Golnoori, F. Z. Boroujeni, A. Monadjemi, Metaheuristic algorithm based hyper-parameters optimization for skin lesion classification, Multimedia Tools Appl., 82 (2023), 25677–25709. https://doi.org/10.1007/s11042-023-14429-7 doi: 10.1007/s11042-023-14429-7
![]() |
[22] |
S. Ayas, Multiclass skin lesion classification in dermoscopic images using swin transformer model, Neural Comput. Appl., 35 (2023), 6713–6722. https://doi.org/10.1007/s00521-022-08053-z doi: 10.1007/s00521-022-08053-z
![]() |
[23] |
Z. Wei, Q. Li, H. Song, Dual attention based network for skin lesion classification with auxiliary learning, Biomed. Signal Process. Control, 74 (2022), 103549. https://doi.org/10.1016/j.bspc.2022.103549 doi: 10.1016/j.bspc.2022.103549
![]() |
[24] |
L. Wang, L. Zhang, X. Shu, Z. Yi, Intra-class consistency and inter-class discrimination feature learning for automatic skin lesion classification, Med. Image Anal., 85 (2023), 102746. https://doi.org/10.1016/j.media.2023.102746 doi: 10.1016/j.media.2023.102746
![]() |
[25] | M. Versaci, G. Angiulli, F. La Foresta, P. Crucitti, F. Lagana, D. Pellicano, et al., Innovative soft computing techniques for the evaluation of the mechanical stress state of steel plates, in Applied Intelligence and Informatics, (2022), 14–28. https://doi.org/10.1007/978-3-031-24801-6_2 |
[26] |
D. Zhuang, K. Chen, J. M. Chang, Cs-af: A cost-sensitive multi-classifier active fusion framework for skin lesion classification, Neurocomputing, 491 (2022), 206–216. https://doi.org/10.1016/j.neucom.2022.03.042 doi: 10.1016/j.neucom.2022.03.042
![]() |
[27] |
Y. Wang, Y. Feng, L. Zhang, J. T. Zhou, Y. Liu, R. S. M. Goh, et al., Adversarial multimodal fusion with attention mechanism for skin lesion classification using clinical and dermoscopic images, Med. Image Anal., 81 (2022), 102535. https://doi.org/10.1016/j.media.2022.102535 doi: 10.1016/j.media.2022.102535
![]() |
[28] |
X. Deng, Q. Yin, P. Guo, Efficient structural pseudoinverse learning-based hierarchical representation learning for skin lesion classification, Complex Intell. Syst., 8 (2022), 1445–1457. https://doi.org/10.1007/s40747-021-00588-3 doi: 10.1007/s40747-021-00588-3
![]() |
[29] |
S. Zhou, S. Tian, L. Yu, W. Wu, D. Zhang, Z. Peng, et al., Fixmatch-ls: Semi-supervised skin lesion classification with label smoothing, Biomed. Signal Process. Control, 84 (2023), 104709. https://doi.org/10.1016/j.bspc.2023.104709 doi: 10.1016/j.bspc.2023.104709
![]() |
[30] |
Y. Wan, Y. Cheng, M. Shao, Mslanet: Multi-scale long attention network for skin lesion classification, Appl. Intell., 53 (2023), 12580–12598. https://doi.org/10.1007/s10489-022-03320-x doi: 10.1007/s10489-022-03320-x
![]() |
[31] |
K. A. Ogudo, R. Surendran, O. I. Khalaf, Optimal artificial intelligence based automated skin lesion detection and classification model, Comput. Syst. Sci. Eng., 44 (2023), 693–707. https://doi.org/10.32604/csse.2023.024154 doi: 10.32604/csse.2023.024154
![]() |
[32] |
W. X. Tsai, Y. C. Li, C. H. Lin, Skin lesion classification based on multi-model ensemble with generated levels-of-detail images, Biomed. Signal Process. Control, 85 (2023), 105068. https://doi.org/10.1016/j.bspc.2023.105068 doi: 10.1016/j.bspc.2023.105068
![]() |
[33] |
F. Miao, L. Yao, X. Zhao, Adaptive margin aware complement-cross entropy loss for improving class imbalance in multi-view sleep staging based on eeg signals, IEEE Trans. Neural Syst. Rehabil. Eng., 30 (2022), 2927–2938. https://doi.org/10.1109/TNSRE.2022.3213848 doi: 10.1109/TNSRE.2022.3213848
![]() |
[34] |
D. Zabihzadeh, A. Tuama, A. Karami-Mollaee, S. J. Mousavirad, Low-rank robust online distance/similarity learning based on the rescaled hinge loss, Appl. Intell., 53 (2023), 634–657. https://doi.org/10.1007/s10489-022-03419-1 doi: 10.1007/s10489-022-03419-1
![]() |
[35] | T. Y. Lin, P. Goyal, R. Girshick, K. He, P. Dollár, Focal loss for dense object detection, in Proceedings of the IEEE International Conference on Computer Vision (ICCV), (2017), 2980–2988. |
[36] |
G. Yue, P. Wei, T. Zhou, Q. Jiang, W. Yan, T. Wang, Toward multicenter skin lesion classification using deep neural network with adaptively weighted balance loss, IEEE Trans. Med. Imaging, 42 (2022), 119–131. https://doi.org/10.1109/TMI.2022.3204646 doi: 10.1109/TMI.2022.3204646
![]() |
[37] |
J. Du, K. Guan, P. Liu, Y. Li, T. Wang, Boundary-sensitive loss function with location constraint for hard region segmentation, IEEE J. Biomed. Health. Inf., 27 (2022), 992–1003. https://doi.org/10.1109/JBHI.2022.3222390 doi: 10.1109/JBHI.2022.3222390
![]() |
[38] |
K. Chaitanya, E. Erdil, N. Karani, E. Konukoglu, Local contrastive loss with pseudo-label based self-training for semi-supervised medical image segmentation, Med. Image Anal., 87 (2023), 102792. https://doi.org/10.1016/j.media.2023.102792 doi: 10.1016/j.media.2023.102792
![]() |
[39] | K. He, X. Zhang, S. Ren, J. Sun, Deep residual learning for image recognition, in Proceedings of the IEEE Conference on Computer Vision and Pattern Recognition (CVPR), (2016), 770–778. |
[40] | M. Tan, Q. Le, Efficientnetv2: Smaller models and faster training, in Proceedings of the 38th International Conference on Machine Learning, 139 (2021), 10096–10106. |
[41] | Z. Liu, H. Mao, C. Y. Wu, C. Feichtenhofer, T. Darrell, S. Xie, A convnet for the 2020s, in Proceedings of the IEEE/CVF Conference on Computer Vision and Pattern Recognition (CVPR), (2022), 11976–11986. |
[42] |
X. Wu, Z. Tao, B. Jiang, T. Wu, X. Wang, H. Chen, Domain knowledge-enhanced variable selection for biomedical data analysis, Inf. Sci., 606 (2022), 469–488. https://doi.org/10.1016/j.ins.2022.05.076 doi: 10.1016/j.ins.2022.05.076
![]() |
[43] | A. Marghoob, R. Braun, An Atlas of Dermoscopy, CRC Press, 2012. https://doi.org/10.3109/9781841847627 |
[44] | F. Schroff, D. Kalenichenko, J. Philbin, Facenet: A unified embedding for face recognition and clustering, in 2015 IEEE Conference on Computer Vision and Pattern Recognition (CVPR), (2015), 815–823. https://doi.org/10.1109/CVPR.2015.7298682 |
[45] |
P. Tschandl, C. Rosendahl, H. Kittler, The ham10000 dataset, a large collection of multi-source dermatoscopic images of common pigmented skin lesions, Sci. Data, 5 (2018), 180161. https://doi.org/10.1038/sdata.2018.161 doi: 10.1038/sdata.2018.161
![]() |
[46] | M. Combalia, N. C. F. Codella, V. Rotemberg, B. Helba, V. Vilaplana, O. Reiter, et al., Bcn20000: Dermoscopic lesions in the wild, preprint, arXiv: 1908.02288. |
[47] | J. Deng, W. Dong, R. Socher, L. J. Li, K. Li, F. F. Li, Imagenet: A large-scale hierarchical image database, in 2009 IEEE Conference on Computer Vision and Pattern Recognition, (2009), 248–255. https://doi.org/10.1109/CVPR.2009.5206848 |
[48] | Y. Cui, M. Jia, T. Y. Lin, Y. Song, S. Belongie, Class-balanced loss based on effective number of samples, in Proceedings of the IEEE/CVF Conference on Computer Vision and Pattern Recognition (CVPR), (2019), 9268–9277. |
Type of lesion | Description | Examples |
Melanoma (MEL) | multiple colors (black, red, blue, gray, dark brown, light brown, and white); Irregular Streaks (Radial Streaming and Pseudopods) | ![]() |
Basal cell carcinoma (BCC) | blue-gray (sometimes brown); leaf-like structures (sometimes mimic streaks seen in MEL); pseudopods or brown aggregated globules | ![]() |
Melanocytic nevus (NV) | dark brown, light brown, or black; visible network pattern | ![]() |
Actinic keratosis (AK) | white-to-yellow surface scale; fine, wavy vessels (straight or coiled) surrounding the hair follicles; a background erythema sparing the hair follicles and resembling a pink-to-red pseudonetwork | ![]() |
Benign keratosis (BKL) | hairpin blood vessels with a white halo; milia-like cysts; network-like structures | ![]() |
Dermatofibroma (DF) | manifest different structures and patterns (some of which can simulate MEL) | ![]() |
Vascular lesion (VASC) | red/maroon lacunae and black lacunae; often exhibits a blue veil, erythema and/or ulceration | ![]() |
Squamous cell carcinoma (SCC) | typically reveals a central mass of keratin and ulceration surrounded by hairpin vessels, linear-irregular vessels and targetoid hair follicles | ![]() |
Type of lesion | Colors | Patterns | |||||||||||
black | dark brown | light brown | gray | blue | yellow | white | red | dots | clods | circles | radial | other | |
MEL | ✓ | ✓ | ✓ | ✓ | ✓ | ✓ | ✓ | ✓ | ✓ | ✓ | |||
BCC | ✓ | ✓ | ✓ | ✓ | ✓ | ||||||||
NV | ✓ | ✓ | ✓ | ✓ | ✓ | ||||||||
AK | ✓ | ✓ | ✓ | ✓ | ✓ | ✓ | ✓ | ||||||
BKL | ✓ | ✓ | ✓ | ||||||||||
DF | ✓ | ✓ | ✓ | ✓ | ✓ | ✓ | |||||||
VASC | ✓ | ✓ | |||||||||||
SCC | ✓ | ✓ | ✓ | ✓ | ✓ | ✓ | ✓ | ✓ |
Models | Loss Function | Accuracy | Precision | Recall | F1-score |
ResNet-18 | CE Loss | 0.883 | 0.853 | 0.738 | 0.785 |
ResNet-18 | Focal Loss | 0.887 | 0.831 | 0.748 | 0.784 |
ResNet-18 | CBCE Loss | 0.887 | 0.831 | 0.735 | 0.780 |
ResNet-18 | CE+MDKLoss (Ours) | 0.895 | 0.869 | 0.758 | 0.793 |
EfficientNetV2-S | CE Loss | 0.899 | 0.812 | 0.797 | 0.804 |
EfficientNetV2-S | Focal Loss | 0.903 | 0.825 | 0.812 | 0.815 |
EfficientNetV2-S | CBCE Loss | 0.896 | 0.802 | 0.685 | 0.729 |
EfficientNetV2-S | CE+MDKLoss (Ours) | 0.907 | 0.835 | 0.809 | 0.821 |
ConvNeXt-T | CE Loss | 0.901 | 0.812 | 0.797 | 0.804 |
ConvNeXt-T | Focal Loss | 0.909 | 0.866 | 0.836 | 0.845 |
ConvNeXt-T | CBCE Loss | 0.910 | 0.867 | 0.806 | 0.839 |
ConvNeXt-T | CE+MDKLoss (Ours) | 0.916 | 0.875 | 0.841 | 0.856 |
Models | Loss Function | Accuracy | Precision | Recall | F1-score |
ResNet-18 | CE Loss | 0.836 | 0.756 | 0.720 | 0.739 |
ResNet-18 | Focal Loss | 0.827 | 0.756 | 0.710 | 0.731 |
ResNet-18 | CBCE Loss | 0.841 | 0.755 | 0.721 | 0.738 |
ResNet-18 | CE+MDKLoss (Ours) | 0.842 | 0.770 | 0.722 | 0.744 |
EfficientNetV2-S | CE Loss | 0.859 | 0.762 | 0.714 | 0.735 |
EfficientNetV2-S | Focal Loss | 0.856 | 0.780 | 0.711 | 0.750 |
EfficientNetV2-S | CBCE Loss | 0.861 | 0.780 | 0.709 | 0.757 |
EfficientNetV2-S | CE+MDKLoss (Ours) | 0.873 | 0.791 | 0.724 | 0.757 |
ConvNeXt-T | CE Loss | 0.861 | 0.791 | 0.758 | 0.772 |
ConvNeXt-T | Focal Loss | 0.858 | 0.815 | 0.774 | 0.792 |
ConvNeXt-T | CBCE Loss | 0.856 | 0.788 | 0.757 | 0.771 |
ConvNeXt-T | CE+MDKLoss (Ours) | 0.876 | 0.821 | 0.785 | 0.799 |
Type of lesion | Description | Examples |
Melanoma (MEL) | multiple colors (black, red, blue, gray, dark brown, light brown, and white); Irregular Streaks (Radial Streaming and Pseudopods) | ![]() |
Basal cell carcinoma (BCC) | blue-gray (sometimes brown); leaf-like structures (sometimes mimic streaks seen in MEL); pseudopods or brown aggregated globules | ![]() |
Melanocytic nevus (NV) | dark brown, light brown, or black; visible network pattern | ![]() |
Actinic keratosis (AK) | white-to-yellow surface scale; fine, wavy vessels (straight or coiled) surrounding the hair follicles; a background erythema sparing the hair follicles and resembling a pink-to-red pseudonetwork | ![]() |
Benign keratosis (BKL) | hairpin blood vessels with a white halo; milia-like cysts; network-like structures | ![]() |
Dermatofibroma (DF) | manifest different structures and patterns (some of which can simulate MEL) | ![]() |
Vascular lesion (VASC) | red/maroon lacunae and black lacunae; often exhibits a blue veil, erythema and/or ulceration | ![]() |
Squamous cell carcinoma (SCC) | typically reveals a central mass of keratin and ulceration surrounded by hairpin vessels, linear-irregular vessels and targetoid hair follicles | ![]() |
Type of lesion | Colors | Patterns | |||||||||||
black | dark brown | light brown | gray | blue | yellow | white | red | dots | clods | circles | radial | other | |
MEL | ✓ | ✓ | ✓ | ✓ | ✓ | ✓ | ✓ | ✓ | ✓ | ✓ | |||
BCC | ✓ | ✓ | ✓ | ✓ | ✓ | ||||||||
NV | ✓ | ✓ | ✓ | ✓ | ✓ | ||||||||
AK | ✓ | ✓ | ✓ | ✓ | ✓ | ✓ | ✓ | ||||||
BKL | ✓ | ✓ | ✓ | ||||||||||
DF | ✓ | ✓ | ✓ | ✓ | ✓ | ✓ | |||||||
VASC | ✓ | ✓ | |||||||||||
SCC | ✓ | ✓ | ✓ | ✓ | ✓ | ✓ | ✓ | ✓ |
Models | Loss Function | Accuracy | Precision | Recall | F1-score |
ResNet-18 | CE Loss | 0.883 | 0.853 | 0.738 | 0.785 |
ResNet-18 | Focal Loss | 0.887 | 0.831 | 0.748 | 0.784 |
ResNet-18 | CBCE Loss | 0.887 | 0.831 | 0.735 | 0.780 |
ResNet-18 | CE+MDKLoss (Ours) | 0.895 | 0.869 | 0.758 | 0.793 |
EfficientNetV2-S | CE Loss | 0.899 | 0.812 | 0.797 | 0.804 |
EfficientNetV2-S | Focal Loss | 0.903 | 0.825 | 0.812 | 0.815 |
EfficientNetV2-S | CBCE Loss | 0.896 | 0.802 | 0.685 | 0.729 |
EfficientNetV2-S | CE+MDKLoss (Ours) | 0.907 | 0.835 | 0.809 | 0.821 |
ConvNeXt-T | CE Loss | 0.901 | 0.812 | 0.797 | 0.804 |
ConvNeXt-T | Focal Loss | 0.909 | 0.866 | 0.836 | 0.845 |
ConvNeXt-T | CBCE Loss | 0.910 | 0.867 | 0.806 | 0.839 |
ConvNeXt-T | CE+MDKLoss (Ours) | 0.916 | 0.875 | 0.841 | 0.856 |
Models | Loss Function | Accuracy | Precision | Recall | F1-score |
ResNet-18 | CE Loss | 0.836 | 0.756 | 0.720 | 0.739 |
ResNet-18 | Focal Loss | 0.827 | 0.756 | 0.710 | 0.731 |
ResNet-18 | CBCE Loss | 0.841 | 0.755 | 0.721 | 0.738 |
ResNet-18 | CE+MDKLoss (Ours) | 0.842 | 0.770 | 0.722 | 0.744 |
EfficientNetV2-S | CE Loss | 0.859 | 0.762 | 0.714 | 0.735 |
EfficientNetV2-S | Focal Loss | 0.856 | 0.780 | 0.711 | 0.750 |
EfficientNetV2-S | CBCE Loss | 0.861 | 0.780 | 0.709 | 0.757 |
EfficientNetV2-S | CE+MDKLoss (Ours) | 0.873 | 0.791 | 0.724 | 0.757 |
ConvNeXt-T | CE Loss | 0.861 | 0.791 | 0.758 | 0.772 |
ConvNeXt-T | Focal Loss | 0.858 | 0.815 | 0.774 | 0.792 |
ConvNeXt-T | CBCE Loss | 0.856 | 0.788 | 0.757 | 0.771 |
ConvNeXt-T | CE+MDKLoss (Ours) | 0.876 | 0.821 | 0.785 | 0.799 |